- 1Department of Neurology, Affiliated Brain Hospital of Nanjing Medical University, Nanjing, China
- 2Department of Radiology, Affiliated Brain Hospital of Nanjing Medical University, Nanjing, China
- 3Department of Neurology, Taizhou People's Hospital, Taizhou, China
Cerebellar lesions can lead to a series of cognitive and emotional disorders by influencing cerebral activity via cerebro-cerebellar loops. To explore changes in cognitive function and structural brain networks in patients with posterior cerebellar infarction, we conducted the current study using diffusion-weighted MRI (32 cerebellar infarction patients, 29 controls). Moreover, a series of neuropsychological tests were used to assess the subject's cognitive performance. We found cognitive impairment following cerebellar infarction involving multiple cognitive domains, including memory, executive functions, visuospatial abilities, processing speed and language functions, and brain topological abnormalities, including changes in clustering coefficients, shortest path lengths, global efficiency, local efficiencies, betweenness centrality and nodal efficiencies. Our results indicated that measures of local efficiency, mainly in the precuneus, cingulate gyrus and frontal-temporal cortex, were significantly reduced with posterior cerebellar infarction. At the same time, The correlation analysis suggested thatthe abnormal alterations in the right PCG, bilateral DCG, right PCUN may play a core role in the cognitive impairment following cerebellar infarctions. The differences in topological features of the structural brain networks within the cerebro-cerebellar circuits may provide a new approach to explore the pathophysiological mechanisms of cognitive impairment following cerebellar infarction.
Introduction
The cerebellum has always been a mysterious structure in the history of neuroscience exploration, especially in the field of advanced cognition. Regarding dysfunction after cerebellar lesions, the main concern in the past has been with motor control and coordination, which mainly manifest as ataxia, balance disorder, vertigo nystagmus, and dysarthria (1). However, recent experimental and clinical evidence suggests that it may also play an important role in cognition and emotion (2–5). The cerebellum cognitive affective syndrome (CCAS), proposed by Schmahmann et al. was characterized by executive dysfunction, spatial cognitive impairment, language deficits, and personality changes (6).
Cerebellar infarction is common in cerebellar lesions, most of which are caused by blockage of the posterior inferior artery. The cerebellar infarction not only affects the patient's motor function and the functional independence but also impacts the patient's cognitive function (7). An increasing amount of evidence has demonstrated that the underlying mechanisms of cognitive impairment following cerebellar lesions might involve abnormal fiber connections between the cerebellum and the functional areas of the cerebrum, especially the frontal and temporal lobes, precuneus and cingulate gyrus (8–10), i.e., the “cerebro-cerebellar loops” (11–14). In particular, the posterior cerebellum is interrelated with the cognitive function areas of the cerebrum (15).
There are many neuroimaging methods for studying brain connections, of which diffusion imaging technology (DTI) is one of the non-invasive methods that can effectively observe and track white matter fiber bundles. It has been applied to the investigation of abnormal brain connections in various cognitive and neuropsychiatric disorders, such as Alzheimer's disease and schizophrenia (16–18). In a previous study, DTI-based fascicular imaging was performed in patients with cerebellar disease to study voxel differences in diffusion coefficient parameters, showing changes in white matter tracts of the cerebellum connecting the cerebellum to cognitive-related cortical areas (19). Furthermore, the relation between cerebellar white matter junction and cognitive impairment was also confirmed in patients with cerebellar neurodegenerative diseases (20). As far as we know, there is currently no DTI study on cognitive impairment after cerebellar infarction.
Whole-brain structural connectivity can be reconstructed with network methods of diffusion MR imaging tractography (21, 22). The present study is the first study of cognitive impairment following cerebellar infarction using the DTI method. The purpose of this study was to investigate topological differences of whole-brain structural connectivity in patients with acute cerebellar infarction and confirm that the cerebellum is involved in cognitive processes by regulating certain cognitive regions of the cerebrum.
Materials and Methods
Subjects
The current study recruited 61 subjects from the Affiliated Brain Hospital of Nanjing Medical University from July 2016 to March 2018. All subjects were Chinese and right-handed, including 32 subjects with cerebellar infarction (17 males and 15 females) and 29 age- and sex-matched healthy controls (17 males and 12 females). Eleven patients had right cerebellar infarction, fourteen patients had left cerebellar infarction, and the remaining patients had bilateral cerebellar infarction. The inclusion criteria for the patients with cerebellar infarction were as follows: (1) subjects were aged between 50 and 70 years old; (2) subjects provided informed consent and could cooperate and complete the MRI examination; (3) education period was >5 years, there was no mental retardation and subjects could cooperate and complete the neuropsychological assessment; (4) this was their first cerebellar infarction and the lesion was localized in the posterior cerebellum; and (5) the cerebellar infarction was in the acute phase (the course of disease had been <2 weeks). The exclusion criteria were as follows: (1) Stroke lesions also involve other brain areas besides the posterior cerebellar lobe; (2) could not complete the examination due to various symptoms; (3) previous history of stroke, leukoencephalopathy, brain tumor, brain trauma, encephalitis, metabolic disorders, and other dementia caused by degenerative diseases of the nervous system; (4) mental disorders and intellectual disabilities; and (5) a history of severe heart, brain, or kidney disease or alcohol and drug abuse. In addition, healthy subjects matched by age, sex, and education levels were recruited as healthy controls.
The current study was approved by the Ethics Committee of the Affiliated Brain Hospital of Nanjing Medical University. Each participant had Written informed consent.
Behavioral Assessment
All participants conducted a series of behavioral tests to assess cognitive performance, including episodic memory, working memory, processing speed, executive function, language, and balance function. General mental status was evaluated with the Mini-Mental Status Examination (MMSE) (23). Episodic memory was evaluated with the Rey auditory verbal learning test (RAVLT) (24). Verbal working memory was evaluated with digit span test (DST) (25). Executive function was evaluated with the Trail-Making Test (TMT) (26) and verbal fluency task (VFT) (27). Language function was evaluated using the Boston naming test (BNT) (28). Visuospatial abilities was evaluated with the clock drawing test (CDT) (29). To assess the emotional state of the patient, we used the Hamilton Depression Scale(HAMD) (30). The assessment of balance function used the Berg balance scale (BBS) (31) and International Cooperative Ataxia Rating Scale(ICARS) (32). Behavioral assessment were conducted by psychometricians and psychologists.
MRI Data Acquisition
The subjects underwent magnetic resonance scans using Siemens 3.0T scanner (Erlangen, Germany) with standard orthogonal head coils. For all subjects, Conventional T2-weighted MR images were obtained to rule out cortical atrophy and other brain abnormalities: repetition time (TR) = 3,500 ms; echo time (TE) = 103 ms; flip angle = 90°; thickness = 6.0 mm; gap = 0 mm; acquisition matrix = 320 × 192; field of view (FOV) = 240 × 240 mm2; number of excitations (NEX) = 2. the high resolution T1-weighted image were collected in a sagittal direction using a 3D-SPGR sequence. The T1 weighted image parameters were as follows: 176 sagittal slices; 1.0 mm slice thickness; TR = 1,900 ms; TE = 2.48 ms; flip angle = 9°; matrix = 512 × 512. The diffusion-weighted MR image were obtained using a spin echo planar imaging sequence, the parameters of which were as follows : TR = 6,600 ms; TE = 93 ms; 45 axial slices; slice thickness = 3.0 mm;gap = 0 mm; 30 gradient directions with a b-value = 1,000 sec/mm2; acquisition matrix = 128 × 128; FOV = 240 × 240 mm2. Imaging was performed by a physician with 16 years of experience in MR image acquisition.
Data Preprocessing
The data preprocessing steps were mainly as follows: Eddy current and head movement correction of the diffusion-weighted image data were conducted by applying affine correspondence of DTI to the image of b = 0. Excess scalp and brain tissue were removed, and then the three eigenvalues (λ1, λ2, λ3) and the eigenvectors were obtained by diagonalizing the tensor matrix. The fractional anisotropy (FA) were calculated. At the same time, the matrix, and inverse matrix from the individual space to the standard space were calculated. The AAL template was used to divide the cerebral cortex and the subcortical region into 90 brain regions, with each brain region as a network node (33). The AAL template by inverse matrix registration to the individual brain space completes the definition of individual brain network nodes. The AAL standard image under the individual spatial template was constructed with the FA image, and the fiber network was constructed to generate a 90 × 90 matrix of the average FA value of all the voxels along the fiber bundle. The above processing were completed by PANDA software (http://www.nitrc.org/projects/panda). The characteristic parameters of the brain network were constructed by Gretna software (http://www.nitrc.org/frs/download.php/5534/gretna.zip). Finally, BrainNet Viewer (http://www.nitrc.org/projects/bnv/) was used to render the multidimensional visualization of weighted networks. It is worth noting that in the construction of the brain network, we defined a threshold for the definition of the network edges, that is, if the number of streamlines between two nodes were greater than a certain threshold (T = 3), then there was an edge connection between the two regions. This threshold was used in previous network research because of its advantages that can reduce the false positive rate of network connection caused by noise or limitation in the study of deterministic tractography (34, 35).
Topological Characteristics
Graph theory is a graph composed of several nodes and edges, which is usually used to describe the connection between two nodes. In the brain networks, nodes and edges represent brain regions and connections between two regions, respectively. Graph theoretical analyses have revealed that brain networks have important topological parameters, the most striking of which was the small worldness (36). In the study of structural networks, several key parameters were used, including local efficiency (Eloc), global efficiency (Eglob), clustering coefficient (Cp), normalized clustering coefficient (γ), shortest path length(Lp), normalized characteristic path length (λ), small-worldness (σ), betweenness centrality, and regional efficiency.
The clustering coefficient C(i) of node i represents the ratio of the number of edges where the actual number of edges E(i) is completely connected to the subgraph Gi. Gi is the connection subgraph of all nodes connected to node i, and Ki is the degree of node i. The formula is as follows:
The clustering coefficient Cp of the entire network is defined as the average of the clustering coefficients of all nodes in the network and reflects the connection status of the entire network. The formula is as follows:
The shortest path length is indicated by L, and the shortest distance Lij from node i to node j refers to how many times the connection from node i can reach node j. The average of the distances of all nodes is the average shortest path length of the entire network. The shortest path length (Lp) measures the extent of network long-distance connections. The formula is as follows:
Global efficiency (Eglob) measures the global efficiency of the information transmit in the network. The global efficiency is defined as the average of the reciprocal of the shortest path of all nodes in the network. The formula is as follows:
The local efficiency(Eloc) is defined as the average of the inverse of the shortest path of all nodes in the subgraph Gi. The formula is as follows:
Regional efficiency, Enodal(i) is the nodal properties of the structural networks, which indicates the average shortest path length between node i and all other nodes in the structural network. The Enodal(i), is defined as follows:
Betweenness centrality of each node can be used to define the hub regions of the brain network (37). The centrality level of the node indicates the importance of node i in promoting connection between different regions of the brain network. The definition of the hub can be implemented in a variety of ways, such as node efficiency, betweenness centrality and nodal degree (37). The definition of the hub regions in the current study was based on betweenness centrality. If the centrality value of node i was >1.5 times the average centrality of the brain network, the node was identified as a hub region in the brain network.
Small-worldness network characteristics were proposed by Watts and Strogatz (38), including γ, λ, σ. The λ is defined by λ = / and the γ is defined by γ = /. Small-worldness, σ = γ/λ, is typically > 1 for small-world networks. Compared with random networks, the advantage of small-world networks is that it has higher interconnectivity and similar shortest path length.
Statistical Analysis
Using SPSS 20.0 statistical software, two-sample T-test was used to compare demographic and clinical data on the basis of the normal distribution test. The age, education level and neuropsychological test scores of the cerebellar infarction group and the control group were compared by independent two-sample t-tests. The sex distribution of the two groups were compared by chi-square test. Network parameters of the two groups were compared by an independent two-sample t-test. The false discovery rate correction was used to correct the statistical results of the network hubs and regional efficiency. Pearson correlation analyses were calculated between the differences in whole brain structural connectivity and neuropsychological test scores; among them, we only examined network characteristics with significant intergroup differences. The significance threshold in statistical analyses were P < 0.05.
Results
Demographics and Clinical Results
The demographic data and clinical characterizations for each group are shown in Table 1. There were no significant differences in age, sex, and educational level between the cerebellar infarction group and the control group. There were significant differences in MMSE scores (P < 0.001) between the two groups. For the domain of episodic memory, working memory, executive function, visuospatial abilities, language and balance function, the group effects were significant for AVLT (P < 0.01), DST (P < 0.05), TMT (TMT-A: P < 0.01), TMT-B (P < 0.001), VFT (P < 0.001), CDT(P < 0.05), BNT (P < 0.01), BBS (P < 0.001) and ICARS(P < 0.001). There was no significant difference in the HAMD scores between the two groups.
Structural Network Parameters
There were significant differences in the network parameters Eglob, Eloc, Cp, and Lp between the cerebellar infarction group and the control group. The cerebellar infarction group was found to have decreased parameters of global efficiency and local efficiency compared with the control group. In contrast, the clustering coefficient and shortest path length increased in patients with cerebellar infarction (Table 2, Figure 1). Other parameters, such as the normalized clustering coefficient, normalized characteristic path length and small-worldness, did not show significant differences between the groups.
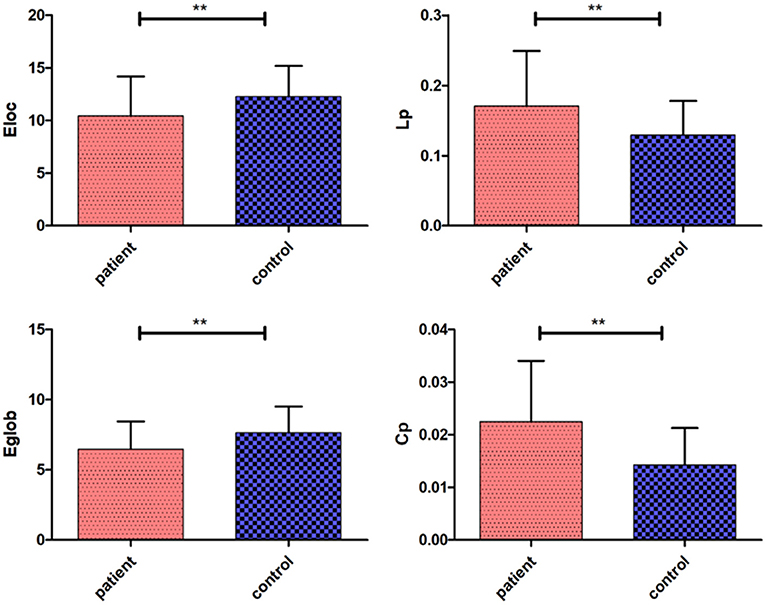
Figure 1. Significantly different network parameters at a threshold T = 3 between cerebellar infarction and healthy controls. Bars and error bars indicate the mean and standard deviation. The asterisk marks indicate a significant difference between the two groups (p < 0.05).
Network Hubs
Regarding brain networks, regions with high levels of centrality can be identified as network hubs. The definition of hub regions in the current study was based on betweenness centrality. If the betweenness centrality value of node was >1.5 times the average centrality of the network, the node was identified as a hub region in the brain network (37). The hub distributions of patients and controls are shown in Table 3 and Figure 2. There were 21 hub regions in the cerebellar infarction group and 22 in the control group. Among them, five hubs only existed in the patient group, including the right thalamus (THA.R), left middle temporal gyrus (MTG.L), left caudate nucleus (CAU.L), left middle frontal gyrus, orbital part (ORBmid.L), and right postcentral gyrus (PoCG.R). In contrast, the right inferior temporal gyrus (ITG.R), bilateral median cingulate and paracingulate gyri (DCG), right cuneus (CUN.R), right superior frontal gyrus, dorsolateral (SFGdor.R), and left calcarine fissure and surrounding cortex (CAL.L) were defined as hub regions only in the control group. Among all hub regions, the most prominent hub of the two groups was the right lenticular nucleus, putamen (PUT.R). Subsequently, the hubs in both groups were compared, and it was found that there was a significant difference only in the right precuneuses (PCUN.R) (P < 0.01; false discovery rate corrected). The details are shown in Figure 3.
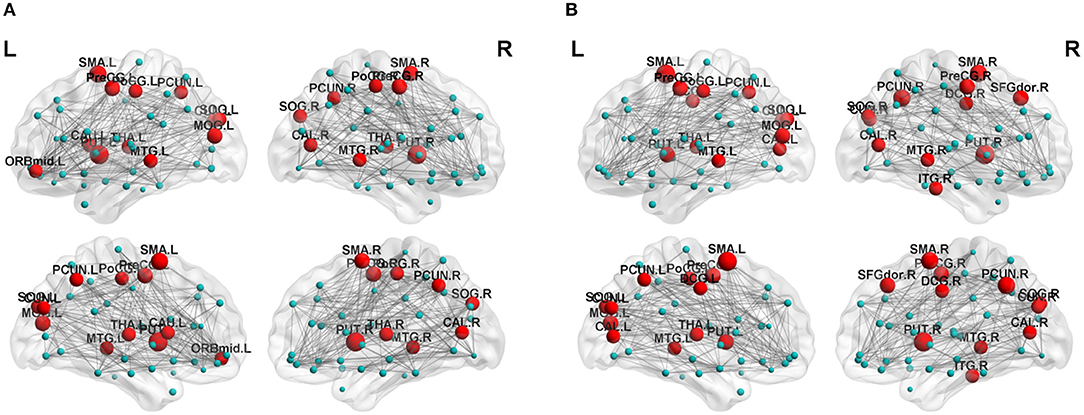
Figure 2. Distribution of hubs in the cerebellar infarction group (A) and control group (B). The hub nodes are shown in red, and node sizes represent their betweenness centrality values. For abbreviations of the hub regions, please see Table 2.
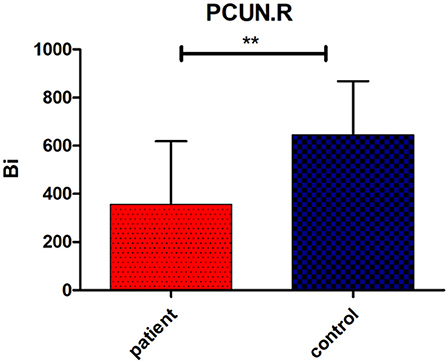
Figure 3. Significant group difference in betweenness centrality value (Bi) of right precuneus (PCUN.R). Bars and error bars indicate the mean and standard deviation. The asterisk marks indicates a significant difference between the two groups (p < 0.05; false discovery rate corrected).
Regional Efficiency
Regional efficiency in the cerebellar infarction group was lower than the control group (Table 4, Figure 4). Twelve brain regions with significant differences (P < 0.05; false discovery rate corrected) between the two groups were found, mainly distributed in the precuneus, cingulate gyrus and frontal-temporal cortex, including bilateral median cingulate and paracingulate gyri (DCG), right inferior frontal gyrus, opercular part (IFGoperc.R), left inferior frontal gyrus, orbital part (ORBinf.L), bilateral supplementary motor area (SMA), bilateral precuneus (PCUN), left temporal pole, superior temporal gyrus (TPOsup.L), left temporal pole, middle temporal gyrus (TPOmid.L), and bilateral posterior cingulate gyrus (PCG).
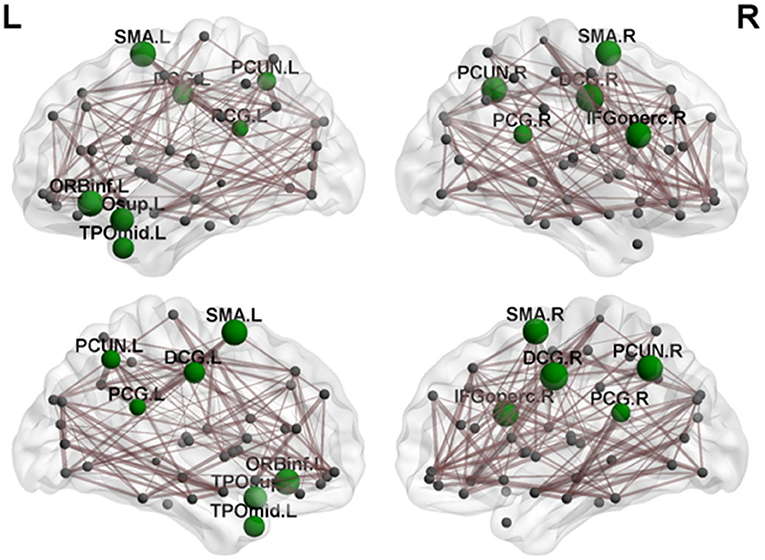
Figure 4. By comparing nodal efficiency between the cerebellar infarction and control group, 12 brain regions with significant differences (P < 0.05, false discovery rate corrected) between the two groups are shown in green. Node sizes represent the significance of between-group differences in regional nodal efficiency. For the abbreviations of the regions, please see Table 3.
Relationship Between Neuropsychological Scale Scores and Brain Network Characteristics
Pearson correlation analyses were calculated between the differences in whole brain structural connectivity and neuropsychological test scores. Among them, we only examined network characteristics with significant intergroup differences. MMSE scores were significantly negatively correlated with Lp (r = −0.370, P = 0.037) and significantly positively correlated with the nodal efficiency of DCG.R (r = 0.374, P = 0.035), PCG.R (r = 0.355, P = 0.046) and PCUN.R (r = 0.396, p = 0.025) (Figure 5A). RAVLT-B scores showed a correlation with Lp (r = −0.363, P = 0.041) (Figure 5B). DST scores were positively correlated with the nodal efficiency of DCG.L (r = 0.357, p = 0.045) (Figure 5C). CDT scores were positively correlated with the nodal efficiency of DCG.R (r = 0.424, p = 0.016) and PCG.R(r = 0.370, P = 0.037) (Figure 5D). TMT-A performance was negatively correlated with the nodal efficiency of PCG.R (r = −0.429, p = 0.014), DCG.R (r = −0.399, P = 0.024), and PCUN.R (r = −0.391, p = 0.027) (Figure 5E). VFT scores were significantly positively correlated with Eloc (r = 0.354, P = 0.047) and Eglob (r = 0.384, P = 0.03) and negatively correlated with Lp (r = −0.504, P = 0.003) (Figure 5F).
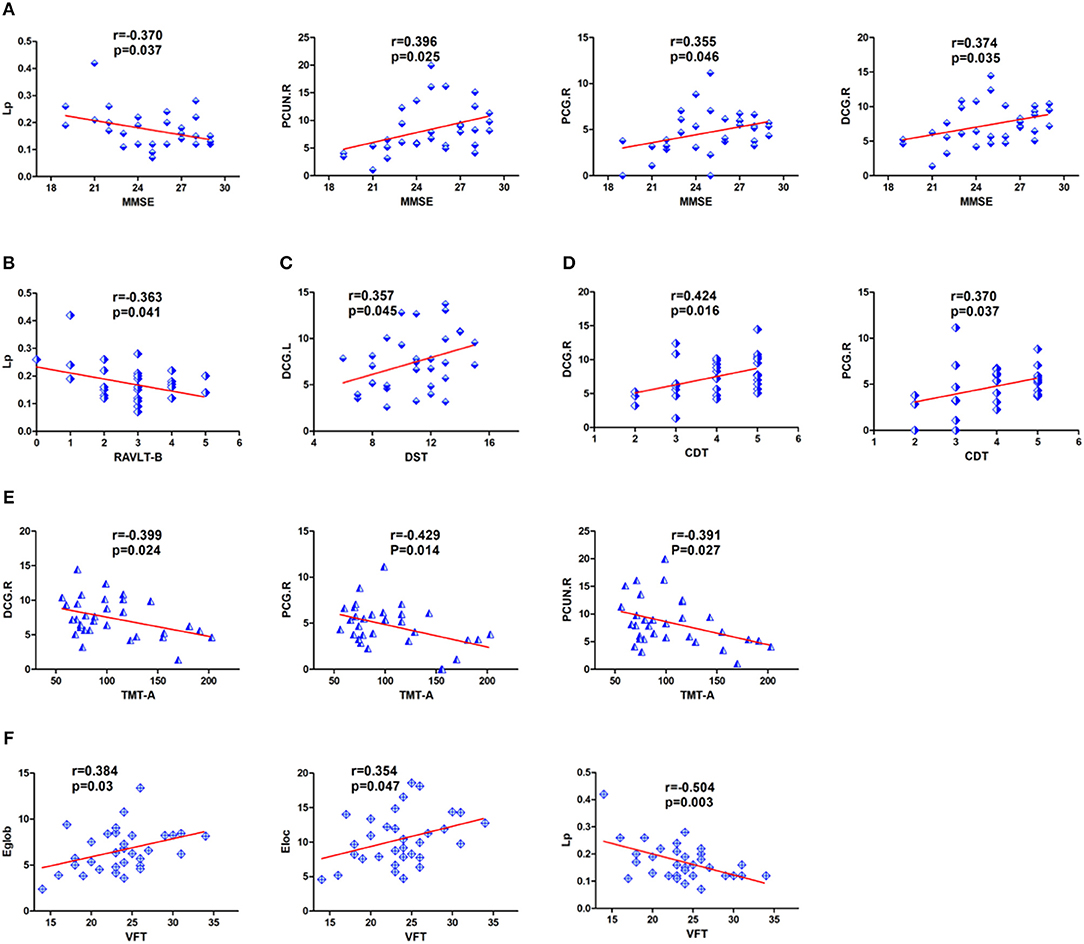
Figure 5. Detailed display of the correlations between neuropsychological scores and regional nodal efficiency and network parameters in patients with cerebral infarction. (A) MMSE was significantly correlated with nodal efficiency in DCG.R, PCUN.R, PCG.R and Lp. (B) RAVLT-B showed a significant negative correlation with Lp. (C) DST showed a significant positive correlation with nodal efficiency in DCG.L. (D) CDT scores were positively correlated with the nodal efficiency of DCG.R and PCG.R. (E) TMT-A showed a significant negative correlation with nodal efficiency in DCG.R, PCG.R, and PCUN.R. (F) VFT showed a significant correlation with Eloc, Lp and Eglob. For the abbreviations of nodes and neuropsychological scores, see Tables 1, 3.
Discussion
To the best of our knowledge, there have been no previous studies using DTI methods to investigate the correlations between cerebellar and cognitive functions in patients with cerebellar infarctions. In this study, we found that cognitive impairment after cerebellar infarction was involved in multiple cognitive domains and the topological alterations of brain networks, including Cp, Lp, Eglob, Eloc, betweenness centrality and nodal efficiency. Additionally, we studied the correlation between the differences in whole brain structural connectivity and neuropsychological scores, which suggested that brain topological abnormalities may have played an important role in the cognitive impairment following cerebellar infarctions.
In our study, Cp and Lp in the cerebellar infarction group were increased significantly compared to those measures in the control group, while Eglob and Eloc were decreased in the patient group compared to the control group. Decreased Eglob and Eloc indicated that brain network efficiency was diminished and integration of the brain networks was disrupted in patients with cerebellar infarction. Current research has also suggested that the cerebellum regulates the brain's higher-order cognitive processes by forming a wide range of neural circuits with specific brain regions (39). Meanwhile, many previous studies have shown that brain networks have small-world properties (40, 41). The small-world networks have quantified the balance between separation and integration of processing information and have enabled the brain to process information efficiently. Previous research on brain networks showed that small-world networks have both higher Cp and shorter Lp (38). Increased Lp and decreased Cp suggested a reduction in the global integration of the structural brain, i.e., destruction of small-world properties (42). Our study did not find abnormalities in the small-world properties, including γ, λ, σ. However, we found that Cp and Lp were significantly increased in the cerebellar infarction group. Increased Lp indicated that the integration of the brain networks was disrupted, which was consistent with previous research results. These measures may suggest a potential change in the small-world properties after cerebellar infarction. There is currently no reasonable explanation for the increased Cp. It may reflect the earliest adaptive mechanism in the brain network disruption, which requires further confirmation by future large-sample trials.
Previous research on brain networks has shown that hub regions were the core nodes in the structural network of the brain (35). The centrality plays an important role in global information transmission. The present study found that the distribution of hub regions in the cerebellar infarction group was similar with control group. Only a few central distributions were different between the two groups, which may indicate the changes of brain structure network after cerebellar infarction. Among all hub regions, the most prominent hub of the two groups was the right lenticular nucleus, putamen (PUT.R). The proposed brain region is an essential part of extrapyramidal system, which is consistent with the previous report that the important function of cerebellum is motor assistance and coordination. Among the hub regions in both groups, only the right precuneus showed a significant difference between the two groups. In a prior study, Dicks et al. used structural magnetic resonance imaging to study patients with mild cognitive impairment and found that the betweeness centrality value of the precuneus was significantly correlated with cognitive decline (43). In our exploration, the betweeness centrality value of precuneus in patients with cerebellar infarction was found to be remarkably lower than that in the control group, suggesting that the occurrence of cognitive impairment after cerebellar infarction may be intimately related to the change of structural connections in the precuneus.
In addition, there were significant differences in the nodal efficiency of some brain regions between the two groups, mainly in the precuneus, cingulate gyrus and frontal-temporal cortex. The reduced efficiency of nodes reflected disruption in the integration of structural connectivity and the dissemination of efficient information in these areas, which may be attributed to the disruption of brain network connection after cerebral infarction.
In the current study, local efficiency reductions in the bilateral PCUN, PCG and DCG were found. The PCUN and cingulate gyrus are the core components of the default mode network (DMN) (44). Previous studies on Alzheimer's disease have shown that the disintegration of the DMN was related to dysfunctions of cognition, such as memory and information processing (45, 46). The PCUN has been shown to be involved in many advanced functional activities in previous studies, such as episodic memory retrieval, visuospatial imagery, self-processing, consciousness (47–49). Cunningham et al used diffusion tensor imaging (DTI) and found that there was significant structural connectivity between the PCUN and the thalamus, hippocampus and middle prefrontal cortex (50). Another study also indicated that the cerebellum, especially Crus I and Crus II, had extensive neural connections with the PCUN (51). Halko et al indicated that intermittent theta-burst stimulation of the lateral cerebellum can increases functional connectivity of the default network (52). At present, multiple studies have shown that the cerebellum contributes significantly to the default network (53–55). Furthermore, the cingulate gyrus also plays an important role in the DMN. The cingulate gyrus, especially the PCG, had a rich connection with other brain regions, such as the thalamus and basal ganglia, which was also an important part of the Papez loop (mainly composed of the hippocampus, thalamus and cingulum) (56). Previous studies also showed that there were neural circuits between the cerebellum and specific regions of the cerebrum, such as the cingulate gyrus and frontal and temporal lobes (8). There was also a close relationship between the anterior, medial and posterior cingulate gyrus (57). Kobayashi found that the cingulate gyrus plays a key role in regulating spatial cognition, working memory processing and episodic memory formation (58). Regarding the study of the cingulate gyrus, most of the current studies have considered that the anterior cingulate gyrus was related to complex physical and visceral motor functions, cognitive function, emotional processing and pain responses, the PCG was related to cognitive function, mainly visual spatial ability and memory (59, 60), and the DCG was considered to have the function of regulating attention and executive functions (61).
There was also a reduction in local efficiency in some frontal and temporal cortices, including the inferior frontal gyrus, opercular part, inferior frontal gyrus, orbital part, temporal pole, superior temporal gyrus, and temporal pole, middle temporal gyrus. A meta-analysis showed that there were extensive neural connections between the cerebellum and the cerebrum, and one importantly connected mode was the connection between the posterior cerebellum and medial prefrontal cortex and temporoparietal junction (9). Franzmeier et al reported that the frontal lobe was specifically connected to the dorsal attention network and the DMN (two core cognitive networks) and plays various functions in learning, working memory, planning, selective attention, and cognitive flexibility (62). Findings in our study support that cognitive impairment may be mainly attributed to the decreased efficiency of local connections in the brain regions involved in default networks and frontotemporal lobe after cerebellar infarction. Furthermore, previous studies have documented that there are ipsilateral and contralateral fibrous connections between the cerebellum and the brain (9, 63). Current studies have also revealed that the changes of network connections in bilateral brain areas after cerebellar infarction may be associated with ipsilateral and contralateral regulation of the cerebellum, which requires to be further explored.
At the same time, correlation analysis was performed between the differences of whole brain structural connectivity and neuropsychological scores. In terms of general cognitive assessment, we found that the MMSE scores were negatively correlated with Lp and positively correlated with local efficiency of some brain regions, including the right DCG, right PCG and right PCUN. We also found a significant correlations between episodic memory, executive function, visuospatial abilities and processing speed with structural network abnormalities. In particular, increased Lp was manifested as a significant correlation with deficits of episodic memory and executive function; reduction in Eglob, Eloc and the efficiency of several brain regions (right DCG, right PCG, and right PCUN) showed significant correlations with impaired executive function; the local efficiency of right DCG and right PCG showed significant correlations with impaired visuospatial abilities; disrupted brain regions of the left DCG were significantly correlated with disrupted processing speed. The correlation analysis suggested that the abnormal alterations in the right PCG, bilateral DCG, right PCUN may play a core role in the cognitive impairment following cerebellar infarctions.
The cerebellum participated in the cognitive processing process, including memory, language functions, visuospatial abilities and processing speed. The cerebellum regulated the cognitive functional regions of the brain through a wide range of neural circuits formed between the cerebrum and cerebellum. The mechanisms of cognitive dysfunction after cerebellar lesions may be by interrupting the nodal efficiency of the structural network and disrupting integration of brain networks.
Limitations
Although we have achieved some considerable results, there are also some methodological problems in our research. First, owing to the small sample size, we have no subgroup of patients with cerebellar infarction based on the left and right location. Therefore, we will continue to recruit patients with cerebellar infarction and conduct in-depth explorations of the functional changes related to different aspects of the lesions. In addition, in our study, deterministic tractography was used to define the edge of the structural network. This analysis might have led to the loss of tracking existing fibers due to fiber crossing problems and limitations of adequately tracking long-distance fibers. An additional supplemental approach may be needed, such as probabilistic fiber tracking, to verify our results.
Conclusions
In general, we are the first to use diffusion-weighted MRI to study the brain structural network of patients with cognitive impairment following cerebellar infarction. Our findings suggested that cognitive impairment after cerebellar infarction was associated with decreased connectivity and information transmission in some brain regions, such as bilateral PCUN, PCG, and DCG, as well as frontotemporal lobes. Among them, abnormal changes of right PCG, bilateral DCG and right PCUN might play a central role in cognitive impairment. Our findings validate the concept of cerebro-cerebellar circuits and indicate that there will be disruptions in structural brain networks after cerebellar infarction. The mechanism of cognitive impairment after cerebellar injury may be the destruction in the connection efficiency and information integration ability of brain network, suggesting that cerebellum may only participate in the integration and regulation of brain network connection, so as to make it function more effectively. The disruptions in the topological features of the structural brain networks within the cerebro-cerebellar circuits may provide a new approach to explore the pathophysiological mechanisms of cognitive impairment following cerebellar infarction.
Data Availability
Datasets are available upon request to the authors.
Ethics Statement
This study was carried out in accordance with the recommendations of the Ethics Committee of the Affiliated Brain Hospital of Nanjing Medical University with written informed consent from all subjects. All subjects gave written informed consent in accordance with the Declaration of Helsinki. The protocol was approved by the Ethics Committee of the Affiliated Brain Hospital of Nanjing Medical University.
Author Contributions
JS designed the study and revised it critically for important intellectual content. DW performed the research and drafted the manuscript. QY, MY, and LF helped in data analyses. XL, CX, DZ, and MT help in clinical data collection, analyses, and made patients follow-ups.
Conflict of Interest Statement
The authors declare that the research was conducted in the absence of any commercial or financial relationships that could be construed as a potential conflict of interest.
Abbreviations
CCAS, Cerebellum cognitive affective syndrome; DTI, Diffusion imaging technology; MMSE, Mini-Mental Status Examination; RAVLT, Rey auditory verbal learning test; DST, Digit span test; TMT, Trail-Making Test; VFT, Verbal fluency task; BNT, Boston naming test; BBS, Berg balance scale; ICARS, International Cooperative Ataxia Rating Scale; CDT, Clock drawing test; HAMD, Hamilton Depression Scale; Eloc, Local efficiency; Eglob, Global efficiency; Cp, Clustering coefficient; γ, Normalized clustering coefficient; Lp, Shortest path length; λ, Normalized characteristic path length; σ, Small-worldness; THA, Thalamus; MTG, Middle temporal gyrus; CAU, Caudate nucleus; PoCG, Postcentral gyrus; ITG, Inferior temporal gyrus; DCG, Median cingulate and paracingulate gyri; CUN, Cuneus; SFGdor, Superior frontal gyrus, dorsolateral; CAL, Calcarine fissure and surrounding cortex; PUT, Lenticular nucleus, putamen; PCUN, Precuneuses; MOG, Middle occipital gyrus; ORBmid, Middle frontal gyrus, orbital part; PCG, Posterior cingulate gyrus; PreCG, Precentral gyrus; SOG, Superior occipital gyrus; IFGoperc, Inferior frontal gyrus, opercular part; ORBinf, Inferior frontal gyrus, orbital part; SMA, Supplementary motor area; TPOsup, Temporal pole, superior temporal gyrus; TPOmid, Temporal pole, middle temporal gyrus; DMN, Default mode network.
References
1. Datar S, Rabinstein AA. Cerebellar infarction. Neurol Clin. (2014) 32:979–91. doi: 10.1016/j.ncl.2014.07.007
2. Sui R, Zhang L. Cerebellar dysfunction may play an important role in vascular dementia. Med Hypotheses. (2012) 78:162–5. doi: 10.1016/j.mehy.2011.10.017
3. Schmahmann JD, Caplan D. Cognition, emotion and the cerebellum. Brain. (2006) 129(Pt 2):290–2. doi: 10.1093/brain/awh729
4. Schmahmann JD. Disorders of the cerebellum: ataxia, dysmetria of thought, and the cerebellar cognitive affective syndrome. J Neuropsyc Clin Neurosci. (2004) 16:367–78. doi: 10.1176/jnp.16.3.367
5. Garriga-Grimau L, Aznar Lain G, Nascimiento MT, Petrizan Aleman A. [Cerebellar cognitive affective syndrome]. Arch Argent Ped. (2015) 113:e268–70. doi: 10.5546/aap.2015.98
6. Schmahmann JD, Sherman JC. The cerebellar cognitive affective syndrome. Brain. (1998) 121(Pt 4):561–79. doi: 10.1093/brain/121.4.561
7. De Smet HJ, Paquier P, Verhoeven J, Marien P. The cerebellum: its role in language and related cognitive and affective functions. Brain Lang. (2013) 127:334–42. doi: 10.1016/j.bandl.2012.11.001
8. Rastogi A, Cash R, Dunlop K, Vesia M, Kucyi A, Ghahremani A, et al. Modulation of cognitive cerebello-cerebral functional connectivity by lateral cerebellar continuous theta burst stimulation. NeuroImage. (2017) 158:48–57. doi: 10.1016/j.neuroimage.2017.06.048
9. Van Overwalle F, Marien P. Functional connectivity between the cerebrum and cerebellum in social cognition: a multi-study analysis. NeuroImage. (2016) 124(Pt A):248–55. doi: 10.1016/j.neuroimage.2015.09.001
10. Clausi S, Olivito G, Lupo M, Siciliano L, Bozzali M, Leggio M. The cerebellar predictions for social interactions: theory of mind abilities in patients with degenerative cerebellar atrophy. Front Cell Neurosci. (2018) 12:510. doi: 10.3389/fncel.2018.00510
11. Strick PL, Dum RP, Fiez JA. Cerebellum and nonmotor function. Ann Rev Neurosci. (2009) 32:413–34. doi: 10.1146/annurev.neuro.31.060407.125606
12. Cantalupo C, Hopkins W. The cerebellum and its contribution to complex tasks in higher primates: a comparative perspective. Cortex. (2010) 46:821–30. doi: 10.1016/j.cortex.2009.10.004
13. Stoodley CJ, Schmahmann JD. Evidence for topographic organization in the cerebellum of motor control versus cognitive and affective processing. Cortex. (2010) 46:831–44. doi: 10.1016/j.cortex.2009.11.008
14. Salmi J, Pallesen KJ, Neuvonen T, Brattico E, Korvenoja A, Salonen O, et al. Cognitive and motor loops of the human cerebro-cerebellar system. J Cogn Neurosci. (2010) 22:2663–76. doi: 10.1162/jocn.2009.21382
15. Stoodley CJ, MacMore JP, Makris N, Sherman JC, Schmahmann JD. Location of lesion determines motor vs. cognitive consequences in patients with cerebellar stroke. NeuroImage Clin. (2016) 12:765–75. doi: 10.1016/j.nicl.2016.10.013
16. Kelly S, Jahanshad N, Zalesky A, Kochunov P, Agartz I, Alloza C, et al. Widespread white matter microstructural differences in schizophrenia across 4322 individuals: results from the ENIGMA Schizophrenia DTI Working Group. Mol Psychiat. (2018) 23:1261–9. doi: 10.1038/mp.2017.170
17. Kantarci K, Murray ME, Schwarz CG, Reid RI, Przybelski SA, Lesnick T, et al. White-matter integrity on DTI and the pathologic staging of Alzheimer's disease. Neurobiol Aging. (2017) 56:172–9. doi: 10.1016/j.neurobiolaging.2017.04.024
18. Nedelska Z, Schwarz CG, Boeve BF, Lowe VJ, Reid RI, Przybelski SA, et al. White matter integrity in dementia with Lewy bodies: a voxel-based analysis of diffusion tensor imaging. Neurobiol Aging. (2015) 36:2010–7. doi: 10.1016/j.neurobiolaging.2015.03.007
19. Olivito G, Lupo M, Iacobacci C, Clausi S, Romano S, Masciullo M, et al. Microstructural MRI basis of the cognitive functions in patients with spinocerebellar ataxia type 2. Neuroscience. (2017) 366:44–53. doi: 10.1016/j.neuroscience.2017.10.007
20. Olivito G, Brunamonti E, Clausi S, Pani P, Chiricozzi FR, Giamundo M, et al. Atrophic degeneration of cerebellum impairs both the reactive and the proactive control of movement in the stop signal paradigm. Exp Brain Res. (2017) 235:2971–81. doi: 10.1007/s00221-017-5027-z
21. Gong G, He Y, Concha L, Lebel C, Gross DW, Evans AC, et al. Mapping anatomical connectivity patterns of human cerebral cortex using in vivo diffusion tensor imaging tractography. Cereb Cort. (2009) 19:524–36. doi: 10.1093/cercor/bhn102
22. Hagmann P, Cammoun L, Gigandet X, Meuli R, Honey CJ, Wedeen VJ, et al. Mapping the structural core of human cerebral cortex. PLoS Biol. (2008) 6:e159. doi: 10.1371/journal.pbio.0060159
23. Tombaugh TN, McIntyre NJ. The mini-mental state examination: a comprehensive review. J Am Geriatr Soc. (1992) 40:922–35. doi: 10.1111/j.1532-5415.1992.tb01992.x
24. Vakil E, Blachstein H. Rey auditory-verbal learning test: structure analysis. J Clin Psychol. (1993) 49:883–90.
25. Bowden SC, Petrauskas VM, Bardenhagen FJ, Meade CE, Simpson LC. Exploring the dimensionality of digit span. Assessment. (2013) 20:188–98. doi: 10.1177/1073191112457016
26. Buck KK, Atkinson TM, Ryan JP. Evidence of practice effects in variants of the Trail Making Test during serial assessment. J Clin Exp Neuropsychol. (2008) 30:312–8. doi: 10.1080/13803390701390483
27. Piskunowicz M, Bielinski M, Zglinski A, Borkowska A. [Verbal fluency tests–application in neuropsychological assessment]. Psychiat Pol. (2013) 47:475–85.
28. Killgore WD, Adams RL. Prediction of boston naming test performance from vocabulary scores: preliminary guidelines for interpretation. Percept Mot Skills. (1999) 89:327–37. doi: 10.2466/pms.1999.89.1.327
29. Mainland BJ, Amodeo S, Shulman KI. Multiple clock drawing scoring systems: simpler is better. Int J Geriat Psychiat. (2014) 29:127–36. doi: 10.1002/gps.3992
30. Bagby RM, Ryder AG, Schuller DR, Marshall MB. The hamilton depression rating scale: has the gold standard become a lead weight? Am J Psychiat. (2004) 161:2163–77. doi: 10.1176/appi.ajp.161.12.2163
31. Smith PS, Hembree JA, Thompson ME. Berg balance scale and functional reach: determining the best clinical tool for individuals post acute stroke. Clin Rehabil. (2004) 18:811–8. doi: 10.1191/0269215504cr817oa
32. Trouillas P, Takayanagi T, Hallett M, Currier RD, Subramony SH, Wessel K, et al. International cooperative ataxia rating scale for pharmacological assessment of the cerebellar syndrome. The ataxia neuropharmacology committee of the world federation of neurology. J Neurol Sci. (1997) 145:205–11. doi: 10.1016/S0022-510X(96)00231-6
33. Tzourio-Mazoyer N, Landeau B, Papathanassiou D, Crivello F, Etard O, Delcroix N, et al. Automated anatomical labeling of activations in SPM using a macroscopic anatomical parcellation of the MNI MRI single-subject brain. NeuroImage. (2002) 15:273–89. doi: 10.1006/nimg.2001.0978
34. Wang Y, West JD, Flashman LA, Wishart HA, Santulli RB, Rabin LA, et al. Selective changes in white matter integrity in MCI and older adults with cognitive complaints. Biochim Biophys Acta. (2012) 1822:423–30. doi: 10.1016/j.bbadis.2011.08.002
35. Bai F, Shu N, Yuan Y, Shi Y, Yu H, Wu D, et al. Topologically convergent and divergent structural connectivity patterns between patients with remitted geriatric depression and amnestic mild cognitive impairment. J Neurosci. (2012) 32:4307–18. doi: 10.1523/JNEUROSCI.5061-11.2012
36. He Y, Evans A. Graph theoretical modeling of brain connectivity. Curr Opin Neurol. (2010) 23:341–50. doi: 10.1097/WCO.0b013e32833aa567
37. van den Heuvel MP, Sporns O. Network hubs in the human brain. Trends Cogn Sci. (2013) 17:683–96. doi: 10.1016/j.tics.2013.09.012
38. Watts DJ, Strogatz SH. Collective dynamics of ‘small-world’ networks. Nature. (1998) 393:440–2. doi: 10.1038/30918
39. Hoche F, Guell X, Vangel MG, Sherman JC, Schmahmann JD. The cerebellar cognitive affective/Schmahmann syndrome scale. Brain. (2018) 141:248–70. doi: 10.1093/brain/awx317
40. Humphries MD, Gurney K, Prescott TJ. The brainstem reticular formation is a small-world, not scale-free, network. Proc Biol Sci. (2006) 273:503–11. doi: 10.1098/rspb.2005.3354
41. Sporns O, Zwi JD. The small world of the cerebral cortex. Neuroinformatics. (2004) 2:145–62. doi: 10.1385/NI:2:2:145
42. Shu N, Liang Y, Li H, Zhang J, Li X, Wang L, et al. Disrupted topological organization in white matter structural networks in amnestic mild cognitive impairment: relationship to subtype. Radiology. (2012) 265:518–27. doi: 10.1148/radiol.12112361
43. Dicks E, Tijms BM, Ten Kate M, Gouw AA, Benedictus MR, Teunissen CE, et al. Gray matter network measures are associated with cognitive decline in mild cognitive impairment. Neurobiol Aging. (2018) 61:198–206. doi: 10.1016/j.neurobiolaging.2017.09.029
44. Vergara EF, Behrens MI. [Default mode network and Alzheimer's disease]. Rev Med Chile. (2013) 141:375–80. doi: 10.4067/S0034-98872013000300014
45. Dillen KNH, Jacobs HIL, Kukolja J, Richter N, von Reutern B, Onur OA, et al. Functional disintegration of the default mode network in prodromal Alzheimer's disease. J Alzheimer's Dis. (2017) 59:169–87. doi: 10.3233/JAD-161120
46. Toussaint PJ, Maiz S, Coynel D, Doyon J, Messe A, de Souza LC, et al. Characteristics of the default mode functional connectivity in normal ageing and Alzheimer's disease using resting state fMRI with a combined approach of entropy-based and graph theoretical measurements. NeuroImage. (2014) 101:778–86. doi: 10.1016/j.neuroimage.2014.08.003
47. Cavanna AE, Trimble MR. The precuneus: a review of its functional anatomy and behavioural correlates. Brain. (2006) 129(Pt 3):564–83. doi: 10.1093/brain/awl004
48. Krause BJ, Schmidt D, Mottaghy FM, Taylor J, Halsband U, Herzog H, et al. Episodic retrieval activates the precuneus irrespective of the imagery content of word pair associates. A PET study. Brain. (1999) 122(Pt 2):255–63. doi: 10.1093/brain/122.2.255
49. Kircher TT, Senior C, Phillips ML, Benson PJ, Bullmore ET, Brammer M, et al. Towards a functional neuroanatomy of self processing: effects of faces and words. Brain Res Cogn Brain Res. (2000) 10:133–44. doi: 10.1016/S0926-6410(00)00036-7
50. Cunningham SI, Tomasi D, Volkow ND. Structural and functional connectivity of the precuneus and thalamus to the default mode network. Hum Brain Mapp. (2017) 38:938–56. doi: 10.1002/hbm.23429
51. Chen G, Zhao L, Jia Y, Zhong S, Chen F, Luo X, et al. Abnormal cerebellum-DMN regions connectivity in unmedicated bipolar II disorder. J Affect Disord. (2019) 243:441–7. doi: 10.1016/j.jad.2018.09.076
52. Halko MA, Farzan F, Eldaief MC, Schmahmann JD, Pascual-Leone A. Intermittent theta-burst stimulation of the lateral cerebellum increases functional connectivity of the default network. J Neurosci. (2014) 34:12049–56. doi: 10.1523/JNEUROSCI.1776-14.2014
53. Guo W, Liu F, Liu J, Yu M, Zhang Z, Liu G, et al. Increased cerebellar-default-mode-network connectivity in drug-naive major depressive disorder at rest. Medicine. (2015) 94:e560. doi: 10.1097/MD.0000000000000560
54. Kucyi A, Hove MJ, Biederman J, Van Dijk KR, Valera EM. Disrupted functional connectivity of cerebellar default network areas in attention-deficit/hyperactivity disorder. Hum Brain Mapp. (2015) 36:3373–86. doi: 10.1002/hbm.22850
55. Wang L, Zou F, Shao Y, Ye E, Jin X, Tan S, et al. Disruptive changes of cerebellar functional connectivity with the default mode network in schizophrenia. Schizophr Res. (2014) 160:67–72. doi: 10.1016/j.schres.2014.09.034
56. Bhattacharyya KB. James wenceslaus papez, his circuit, and emotion. Ann Indian Acad Neurol. (2017) 20:207–10. doi: 10.4103/aian.AIAN_487_16
57. Tamburin S, Cacciatori C, Bonato C, Zanette G. Cingulate gyrus tumor presenting as panic attacks. Am J Psychiat. (2008) 165:651–2. doi: 10.1176/appi.ajp.2007.07061005
58. Kobayashi Y. [Spatial cognition and episodic memory formation in the limbic cortex]. Brain Nerve. (2017) 69:427–37. doi: 10.11477/mf.1416200760
59. Delano-Wood L, Stricker NH, Sorg SF, Nation DA, Jak AJ, Woods SP, et al. Posterior cingulum white matter disruption and its associations with verbal memory and stroke risk in mild cognitive impairment. J Alzheimer's Dis. (2012) 29:589–603. doi: 10.3233/JAD-2012-102103
60. Bubb EJ, Metzler-Baddeley C, Aggleton JP. The cingulum bundle: anatomy, function, and dysfunction. Neurosci Biobehav Rev. (2018) 92:104–27. doi: 10.1016/j.neubiorev.2018.05.008
61. Bush G, Luu P, Posner MI. Cognitive and emotional influences in anterior cingulate cortex. Trends Cogn Sci. (2000) 4:215–22. doi: 10.1016/S1364-6613(00)01483-2
62. Franzmeier N, Gottler J, Grimmer T, Drzezga A, Araque-Caballero MA, Simon-Vermot L, et al. Resting-state connectivity of the left frontal cortex to the default mode and dorsal attention network supports reserve in mild cognitive impairment. Front Aging Neurosci. (2017) 9:264. doi: 10.3389/fnagi.2017.00264
Keywords: cerebellar infarction, cognitive impairment, diffusion MRI, graph theory, brain network
Citation: Wang D, Yao Q, Yu M, Xiao C, Fan L, Lin X, Zhu D, Tian M and Shi J (2019) Topological Disruption of Structural Brain Networks in Patients With Cognitive Impairment Following Cerebellar Infarction. Front. Neurol. 10:759. doi: 10.3389/fneur.2019.00759
Received: 28 February 2019; Accepted: 01 July 2019;
Published: 19 July 2019.
Edited by:
Peter Sörös, University of Oldenburg, GermanyReviewed by:
Silvia Clausi, Fondazione Santa Lucia (IRCCS), ItalyGiusy Olivito, Fondazione Santa Lucia (IRCCS), Italy
Copyright © 2019 Wang, Yao, Yu, Xiao, Fan, Lin, Zhu, Tian and Shi. This is an open-access article distributed under the terms of the Creative Commons Attribution License (CC BY). The use, distribution or reproduction in other forums is permitted, provided the original author(s) and the copyright owner(s) are credited and that the original publication in this journal is cited, in accordance with accepted academic practice. No use, distribution or reproduction is permitted which does not comply with these terms.
*Correspondence: Jingping Shi, cHJvZnNoaWpwJiN4MDAwNDA7MTYzLmNvbQ==