- 1Department of Epidemiology, Erasmus University Medical Center, Rotterdam, Netherlands
- 2Department of Radiology and Nuclear Medicine, Erasmus University Medical Center, Rotterdam, Netherlands
- 3Department of Internal Medicine, Erasmus University Medical Center, Rotterdam, Netherlands
Background: It is known that obesity [measured with body mass index (BMI)] relates to brain structure and markers of cerebral small vessel disease (CSVD). However, BMI may not adequately represent body composition. Furthermore, whether those cross-sectional associations hold longitudinally remains uncertain.
Methods: Three thousand six hundred and fourty-eight participants underwent baseline (2006–2014) dual-energy X-ray absorptiometry (DXA)-scan to obtain detailed measures of body composition and a magnetic resonance imaging (MRI) scan to assess brain structure. One thousand eight hundred and fourty-four participants underwent a second MRI-scan at follow-up (2010–2017; median follow-up: 5.5 years). To assess cross-sectional and longitudinal associations (measures of change have been calculated) between body composition [BMI, fat mass index (FMI), fat-free mass index (FFMI)], and brain tissue volume (gray matter, white matter, hippocampus), white matter microstructure [fractional anisotropy (FA), mean diffusivity (MD)], and CSVD markers (white matter hyperintensity volume, lacunes, microbleeds) we used multivariable linear and logistic regression models.
Results: A higher BMI and FMI were cross-sectionally associated with smaller white matter volumes (difference in Z-score per SD higher BMI: −0.064 [95% CI: −0.094, −0.035]) and FMI: −0.067 [95% CI: −0.099, −0.034], higher FA and MD. A higher FFMI was associated larger gray matter volume (difference: 0.060 [95% CI: 0.018, 0.101]). There was no statistically significant or clinically relevant association between body composition and brain changes.
Conclusions: Body composition, distinguishing between fat mass and fat-free mass, does not directly influence changes in brain tissue volume, white matter integrity and markers of CSVD. Cross-sectional associations between body composition and brain tissue volume likely reflect cumulative risk or shared etiology.
Introduction
The increasing prevalence of obesity is accompanied by numerous adverse health effects, including cognitive decline and dementia (1, 2). Moreover, obesity has been linked with smaller brain tissue volumes, and decreased white matter integrity (2–5). On top of that, it has been found that obesity is associated with focal brain pathology in the form of cerebral small vessel disease (CSVD) (6).
An important limitation of previous studies is that obesity is generally assessed by body mass index (BMI) (2). However, aging is associated with a decrease in lean mass and an increase in fat mass, making BMI less suitable as an approximation of obesity in the elderly (2, 7). In a study looking at the association between body composition and metabolic syndrome the same “BMI problem” was encountered. Researchers found that fat mass index (FMI) was independently and positively associated with the presence of metabolic syndrome regardless of BMI and concluded that FMI is a better approximation of body composition (8). A second limitation is that previous studies mainly assessed cross-sectional relations, precluding inference on directionality.
We studied the association of body composition, divided into body mass, fat mass, and fat-free mass, with brain tissue volume, white matter integrity and markers of CSVD (white matter lesion hyperintensities (WMH), lacunes, and microbleeds) to evaluate whether fat mass and fat-free mass is a better approximation of body composition. Moreover, we aimed to assess the longitudinal association between body composition and brain health.
Materials and Methods
Setting
This study was embedded within the population-based Rotterdam Study. At study entry and subsequently every 3–4 years, all participants were invited to undergo extensive examinations. For the present study, 4,104 participants (2006–2014) underwent body composition assessment with a dual-energy X-ray absorptiometry (DXA) scan and a brain magnetic resonance imaging (MRI) scan (9). From this group we excluded participants with cortical infarcts on MRI (N = 164), cancer (N = 263), and dementia (N = 29), leaving 3,648 participants for the cross-sectional analyses. Between 2010 and 2017 in a subsequent examination-round, 1,844 participants had a follow-up MRI-scan available for the longitudinal analysis. Reasons of dropout at follow-up or unavailability of follow up MRI information have been included in Supplemental Figure 1.
The Rotterdam Study has received medical ethical approval according to the Population Screening Act: Rotterdam Study, executed by the Ministry of Health, Welfare, and Sports of the Netherlands. All participants provided written informed consent.
Body Composition
Body weight and length were measured and BMI was calculated (kg/m2) (9). A DXA—scan (iDXA, GE Lunar Healthcare, USA) was performed to determine fat-free mass and fat mass in kilograms (9). From this, we calculated fat mass index (FMI) (kg/m2), and fat-free mass index (FFMI) (kg/m2).
Brain Tissue Volume, White Matter Integrity, and Markers of CSVD
Brain-MRI was performed on a 1.5-tesla MRI scanner with a 8-channel head coil, using a standardized scan protocol which has been described in detail before (9, 10). To quantify brain tissue volume, gray matter volume, white matter volume, WMH volume, and intracranial volume, automated brain tissue classification was used. This quantification strategy was based on a k-nearest neighbor classifier algorithm, extended with an in-house-developed WMH segmentation (10). Total brain volume did not include the cerebellum (used MRI segmentation only segments supratentorial brain tissue and not all MRI scans incorporated the entire cerebellum in the field of view). T1-weighted MR images were processed using FreeSurfer (version 5.1) to obtain the hippocampus volume (9). White matter microstructure [fractional anisotropy (FA), mean diffusivity (MD)] was assessed with diffusion weighted imaging (9, 11). Visual ratings were performed for presence of lacunes or microbleeds (9).
Covariables
Information on energy intake, smoking, alcohol, education, and physical activity was obtained from questionnaires and interviews as described in detail in the supplement (9).
Statistics
We investigated associations of body composition with brain tissue volumes, WMH volume (log-transformed), and FA and MD as a ratio of intracranial volume (to adjust for absolute head size differences) using linear regression models. We calculated changes by subtracting baseline measurement from follow-up measurement, which were eventually standardized and used to assess the longitudinal association with linear regression models. The association of body composition with presence of lacunes and microbleeds at baseline and the progression of lacunes or microbleeds at follow-up for the longitudinal analysis was assessed with logistic regression models. Adjustments were made for age, age2, sex, and education (model 1). We additionally adjusted for energy intake, smoking, physical activity, and alcohol (model 2). Adding height, hypertension, hypercholesterolemia, and diabetes to the regression models did not change the effect estimates and were therefore left out of the final analysis. For the longitudinal analysis we additionally adjusted for time between MRI-scans. We ran the same analyses, with brain tissue volumes, WMH volume, and FA and MD unstandardized (while adjusting for intracranial volume in the regression model) to evaluate clinical relevance of the associations. We compared our results with previously reported brain tissue changes that ranged from 3.6 to 5.4 ml (these values represent estimated average changes over the head size distributions of the population studied) with 1 year of aging (12, 13).
We checked for interaction between body composition and sex and between body composition and age (<60 vs. ≥60 years) as effects of BMI might differ between mid- and late-life (7). We were powered (alpha level: 0.05; power level: 0.80) to detect minimal differences of 2.028 ml brain tissue volume. A p-value < 0.05 was considered statistically significant. IBM SPSS statistics version 24.0 (IBM Corp, Armonk, NY, USA) was used for analyses.
Results
Baseline characteristics are described in Table 1. Mean age was 65.9 years (standard deviation (SD): 9.8), 57.3% was female. Mean BMI was 27.2 kg/m2 (SD: 3.9). Participants with a follow-up MRI scan compared to those with only baseline MRI were younger, higher educated, and healthier (Supplemental Table 1).
We found that cross-sectionally a higher BMI and FMI were related with smaller white matter volumes (difference in Z-score per SD higher BMI: −0.064 [95% CI: −0.094, −0.035]; FMI: −0.067 [95% CI: −0.099, −0.034]), higher FA (BMI difference: 0.061 [95% CI: 0.031, 0.091]; FMI difference: 0.098 [95% CI: 0.065, 0.130]), and higher MD (BMI difference: 0.045 [95% CI: 0.018, 0.072]; FMI difference: 0.075 [95% CI: 0.045, 0.104]), and a higher FFMI was related with larger gray matter volumes (difference: 0.060 [95% CI: 0.018, 0.101]) (Table 2, model 1). Effect estimates did not change between the different models (Table 3, model 2), nor were there differences in effects of body composition indices between the various outcomes of interest. Though statistically significant, cross-sectional results might not be clinically relevant as effect estimates of change in brain tissue volumes, as well as their corresponding confidence intervals, reported in milliliter difference per one point increase in BMI, FMI, or FFMI are small and do not exceed clinically relevant amounts of tissue changes (Supplemental Tables 2, 3).
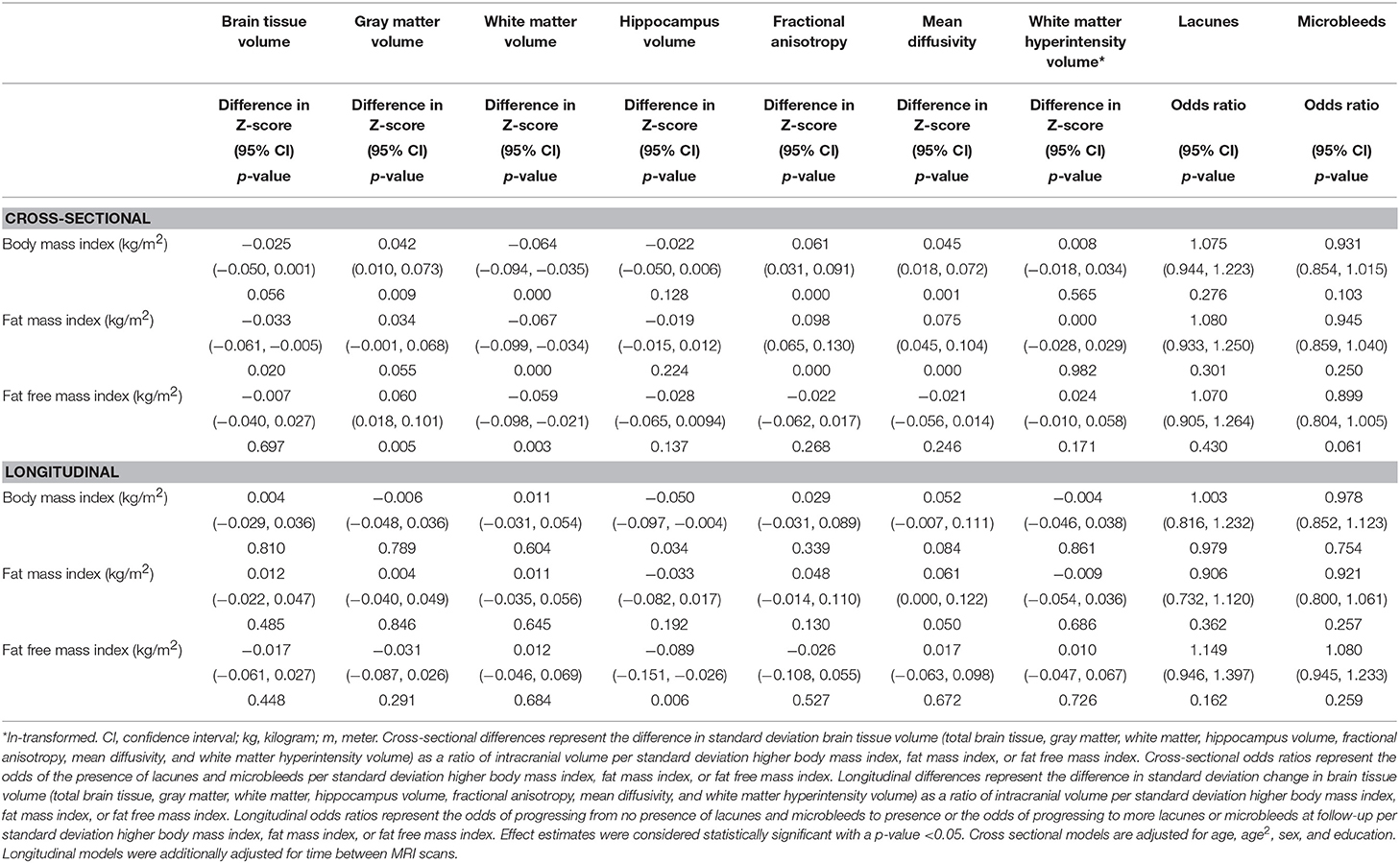
Table 2. The associations between body composition and brain tissue volume and CSVD markers—model 1.
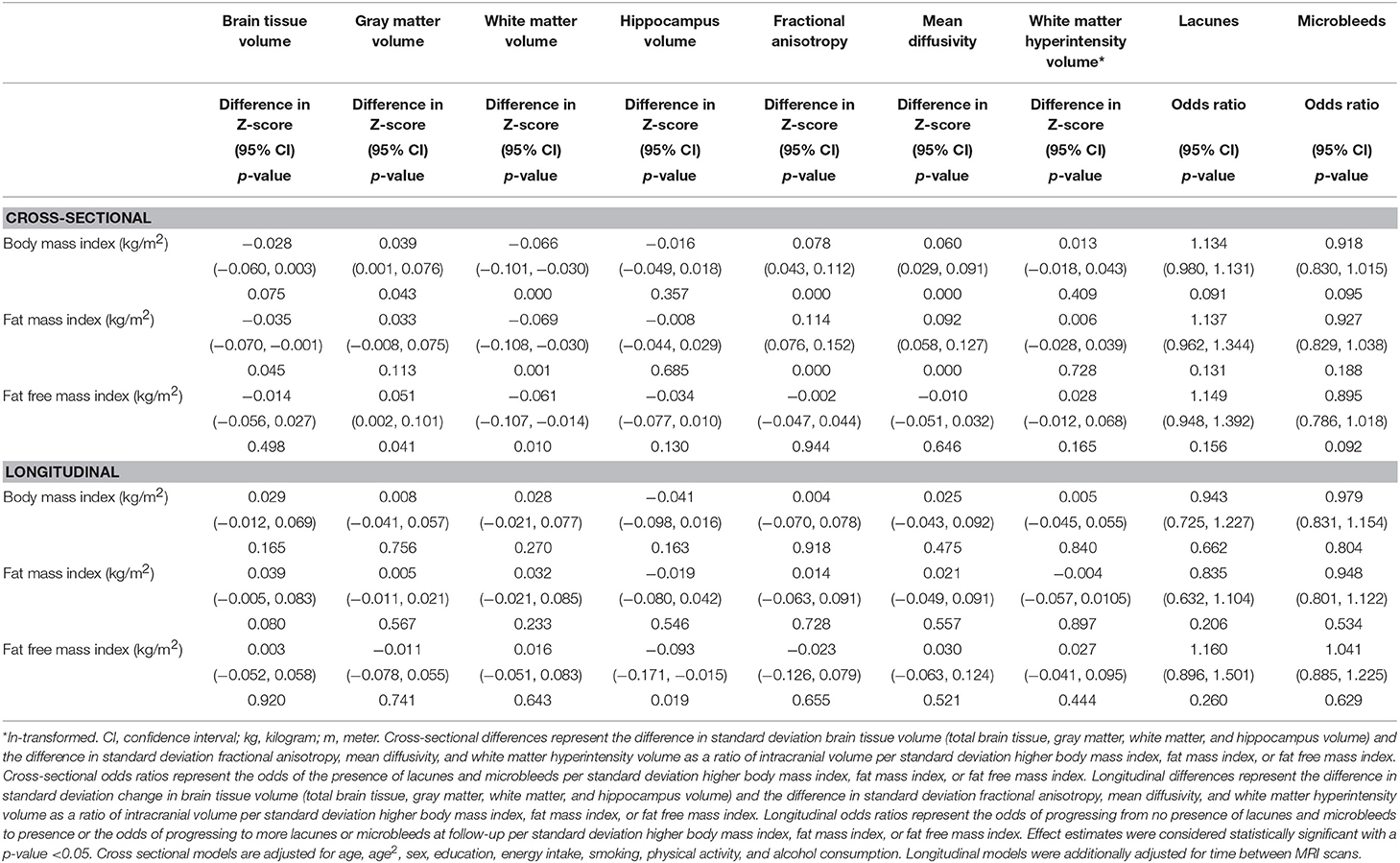
Table 3. The associations between body composition and brain tissue volume and CSVD markers—model 2.
Only the longitudinal association between FFMI and change in hippocampus volume was statistically significant (difference: −0.089 [95% CI: −0.151, −0.026]) (Table 2; model 1), and did not change across models. However, it is questionable whether the direction of this association is biological plausible, and when reporting this association in milliliter (Supplemental Table 3; model 2), it is borderline statistically significant (difference in milliliter per one point increase in FFMI: −0.027 [95% CI: −0.055, 0.000]). We did not find any statistically significant or clinically relevant longitudinal associations between body composition, brain tissue volumes, white matter integrity, or markers of CSVD (Tables 2, 3; Supplemental Tables 2, 3). Both cross-sectional and longitudinal results did not differ between midlife and late life (data not shown), or between males and females (Supplemental Table 4).
Discussion
We found that detailed measures of body composition (higher BMI and higher FMI) related to smaller white matter volume and decreased white matter microstructure and that higher FFMI related to higher gray matter volumes. However, expressing those cross-sectional associations in milliliter difference, raises the question whether those are also clinically relevant. No statistically significant or clinically relevant associations were found longitudinally (median follow-up: 5.5 years).
Strengths of this study are the large sample size, the distinction of body composition, the wide range of brain imaging markers, and the longitudinal design. However, some limitations should also be acknowledged. First, due to the observational design we cannot exclude the possibility of residual confounding. Second, we did not adjust for possible changes in body composition over time in the longitudinal analysis.
Previous studies showed that body composition relates to brain structure (14, 15), which is replicated in some of our cross-sectional results. Currently there are no established thresholds for rates of brain atrophy. However, in two other population-based studies, change in brain tissue volume with 1 year of aging were found to range from 3.6 to 5.4 ml (12, 13). Against this background, it is questionable whether a (cross-sectional) 0.496 ml smaller brain volume per one-point increase in BMI (independent of age) and other (longitudinal) effect estimates, are also clinically relevant. The absence of a longitudinal association did not result from insufficient power, as we were able to minimally detect a change of 2.028 ml brain tissue volume, which is smaller than a clinically relevant change of 5 ml brain volume (12). Though, the absence of a longitudinal association might result in part from selection bias. Indeed, it appeared that participants with MRI at follow-up compared to those without follow-up were younger, higher educated, and healthier. Another explanation might be that the effects of body composition in midlife and late life may be reversed (7, 16). But, we could not confirm effect modification by age in our study. Other longitudinal studies are inconclusive in their results, both reporting non-significant associations, and significant associations between body composition and various brain measurements (3, 5, 16–18), probably due to different methodologies. In a study investigating whether the effect of obesity on the brain increased with age they found no significant results and thus suggested that obesity may act as a modifier of brain atrophy rather than it being a direct cause (19). Cardiovascular risk factors (hypertension, glucose, cholesterol, plasma triglyceride) are known to co-occur frequently with obesity and have independent negative effects on brain structure (2, 20–22). Therefore, although we did not find significant associations between body composition and markers of CSVD, it might be that the effects of vascular risk factors may be less pronounced in their effects on brain structure. And it might also be that solely body composition does not influence brain health, but it is rather the interplay of cardiovascular risk factors altogether that may impact brain health negatively (23, 24).
Since BMI may not be a good approximation for body composition (25), we used FMI and FFMI. Surprisingly, both cross-sectionally and longitudinally, regression estimates between the different body composition measurement (BMI, FMI, and FFMI) and brain MRI data did not differ.
Based on our findings it seems that cross-sectional associations between body composition and brain health likely reflect cumulative risk or shared etiology. Moreover, it seems that body composition, decomposed into fat mass, and fat-free mass, does not influence brain changes or the presence of CSVD. Therefore, we cannot conclude that FMI and FFMI is a better approximation of adiposity than BMI to study brain health. Other longitudinal studies are needed to replicate our findings.
Ethics Statement
The Rotterdam Study has been approved by the medical ethics committee according to the Population Screening Act: Rotterdam Study, executed by the Ministry of Health, Welfare, and Sports of the Netherlands. All participants provided written informed consent.
Author Contributions
MI, FR, and MV: study design and funding. TV, MI, FR, DB, and MV: data collection. PC: data analysis. PC, TV, MI, DB, and MV: data interpretation. All authors: critical revision of the manuscript.
Funding
The Rotterdam Study is funded by Erasmus Medical Center and Erasmus University, Rotterdam, Netherlands Organization for the Health Research and Development (ZonMw), the Research Institute for Diseases in the Elderly (RIDE), the Ministry of Education, Culture and Science, the Ministry for Health, Welfare, and Sports, the European Commission (DG XII), and the Municipality of Rotterdam. FR is supported by the Netherlands Scientific Organization (NWO) and ZonMW Project number: NWO/ZONMW-VIDI-016-136-367.
Conflict of Interest Statement
The authors declare that the research was conducted in the absence of any commercial or financial relationships that could be construed as a potential conflict of interest.
Acknowledgments
We are grateful to all the participants of the Rotterdam Study, the staff from the Rotterdam Study, and the participating general practitioners and pharmacists.
Supplementary Material
The Supplementary Material for this article can be found online at: https://www.frontiersin.org/articles/10.3389/fneur.2019.00559/full#supplementary-material
References
1. Collaborators GBDO. Health effects of overweight and obesity in 195 countries over 25 years. N Engl J Med. (2017) 377:13–27. doi: 10.1056/NEJMoa1614362
2. Stillman CM, Weinstein AM, Marsland AL, Gianaros PJ, Erickson KI. Body-brain connections: the effects of obesity and behavioral interventions on neurocognitive aging. Front Aging Neurosci. (2017) 9:115. doi: 10.3389/fnagi.2017.00115
3. Cherbuin N, Sargent-Cox K, Fraser M, Sachdev P, Anstey KJ. Being overweight is associated with hippocampal atrophy: the PATH through life study. Int J Obes (Lond). (2015) 39:1509–14. doi: 10.1038/ijo.2015.106
4. Kullmann S, Schweizer F, Veit R, Fritsche A, Preissl H. Compromised white matter integrity in obesity. Obes Rev. (2015) 16:273–81. doi: 10.1111/obr.12248
5. Dekkers IA, Jansen PR, Lamb HJ. Obesity, brain volume, and white matter microstructure at MRI: a cross-sectional UK biobank study. Radiology. (2019). doi: 10.1148/radiol.2019181012. [Epub ahead of print].
6. Karcher HS, Holzwarth R, Mueller HP, Ludolph AC, Huber R, Kassubek J, et al. Body fat distribution as a risk factor for cerebrovascular disease: an MRI-based body fat quantification study. Cerebrovasc Dis. (2013) 35:341–8. doi: 10.1159/000348703
7. Spauwen PJ, Murphy RA, Jonsson PV, Sigurdsson S, Garcia ME, Eiriksdottir G, et al. Associations of fat and muscle tissue with cognitive status in older adults: the AGES-Reykjavik Study. Age Ageing. (2017) 46:250–7. doi: 10.1093/ageing/afw219
8. Liu P, Ma F, Lou H, Liu Y. The utility of fat mass index vs. body mass index and percentage of body fat in the screening of metabolic syndrome. BMC Public Health. (2013) 13:629. doi: 10.1186/1471-2458-13-629
9. Ikram MA, Brusselle GGO, Murad SD, van Duijn CM, Franco OH, Goedegebure A, et al. The Rotterdam study: 2018 update on objectives, design and main results. Eur J Epidemiol. (2017) 32:807–50. doi: 10.1007/s10654-017-0321-4
10. Ikram MA, van der Lugt A, Niessen WJ, Koudstaal PJ, Krestin GP, Hofman A, et al. The Rotterdam scan study: design update 2016 and main findings. Eur J Epidemiol. (2015) 30:1299–315. doi: 10.1007/s10654-015-0105-7
11. de Groot M, Ikram MA, Akoudad S, Krestin GP, Hofman A, van der Lugt A, et al. Tract-specific white matter degeneration in aging: the Rotterdam study. Alzheimers Dement. (2015) 11:321–30. doi: 10.1016/j.jalz.2014.06.011
12. Resnick SM, Pham DL, Kraut MA, Zonderman AB, Davatzikos C. Longitudinal magnetic resonance imaging studies of older adults: a shrinking brain. J Neurosci. (2003) 23:3295–301. doi: 10.1523/JNEUROSCI.23-08-03295.2003
13. Croll PH, Voortman T, Ikram MA, Franco OH, Schoufour JD, Bos D, et al. Better diet quality relates to larger brain tissue volumes: the Rotterdam study. Neurology. (2018) 90:e2166–73. doi: 10.1212/WNL.0000000000005691
14. Ronan L, Alexander-Bloch AF, Wagstyl K, Farooqi S, Brayne C, Tyler LK, et al. Obesity associated with increased brain age from midlife. Neurobiol Aging. (2016) 47:63–70. doi: 10.1016/j.neurobiolaging.2016.07.010
15. van Bloemendaal L, Ijzerman RG, Ten Kulve JS, Barkhof F, Diamant M, Veltman DJ, et al. Alterations in white matter volume and integrity in obesity and type 2 diabetes. Metab Brain Dis. (2016) 31:621–9. doi: 10.1007/s11011-016-9792-3
16. Shaw ME, Sachdev PS, Abhayaratna W, Anstey KJ, Cherbuin N. Body mass index is associated with cortical thinning with different patterns in mid- and late-life. Int J Obes (Lond). (2017) 42:455–61. doi: 10.1038/ijo.2017.254
17. Gustafson D, Lissner L, Bengtsson C, Bjorkelund C, Skoog I. A 24-year follow-up of body mass index and cerebral atrophy. Neurology. (2004) 63:1876–81. doi: 10.1212/01.WNL.0000141850.47773.5F
18. Brooks SJ, Benedict C, Burgos J, Kempton MJ, Kullberg J, Nordenskjold R, et al. Late-life obesity is associated with smaller global and regional gray matter volumes: a voxel-based morphometric study. Int J Obes (Lond). (2013) 37:230–6. doi: 10.1038/ijo.2012.13
19. Driscoll I, Beydoun MA, An Y, Davatzikos C, Ferrucci L, Zonderman AB, et al. Midlife obesity and trajectories of brain volume changes in older adults. Hum Brain Mapp. (2012) 33:2204–10. doi: 10.1002/hbm.21353
20. Ikram MA, Vrooman HA, Vernooij MW, van der Lijn F, Hofman A, van der Lugt A, et al. Brain tissue volumes in the general elderly population. The Rotterdam scan study. Neurobiol Aging. (2008) 29:882–90. doi: 10.1016/j.neurobiolaging.2006.12.012
21. Yates KF, Sweat V, Yau PL, Turchiano MM, Convit A. Impact of metabolic syndrome on cognition and brain: a selected review of the literature. Arterioscler Thromb Vasc Biol. (2012) 32:2060–7. doi: 10.1161/ATVBAHA.112.252759
22. Sala M, de Roos A, van den Berg A, Altmann-Schneider I, Slagboom PE, Westendorp RG, et al. Microstructural brain tissue damage in metabolic syndrome. Diabetes Care. (2014) 37:493–500. doi: 10.2337/dc13-1160
23. Debette S, Beiser A, Hoffmann U, DeCarli C, O'Donnell CJ, Massaro JM, et al. Visceral fat is associated with lower brain volume in healthy middle-aged adults. Ann Neurol. (2010) 68:136–44. doi: 10.1002/ana.22062
24. Pichler A, Khalil M, Langkammer C, Pinter D, Ropele S, Fuchs S, et al. The impact of vascular risk factors on brain volume and lesion load in patients with early multiple sclerosis. Mult Scler. (2017) 25:48–54. doi: 10.1177/1352458517736149
Keywords: body composition, brain volume, white matter integrity, cerebral small vessel disease (CSVD), fat mass index (FMI), fat-free mass index (FFMI)
Citation: Croll PH, Bos D, Ikram MA, Rivadeneira F, Voortman T and Vernooij MW (2019) Body Composition Is Not Related to Structural or Vascular Brain Changes. Front. Neurol. 10:559. doi: 10.3389/fneur.2019.00559
Received: 16 October 2018; Accepted: 09 May 2019;
Published: 28 May 2019.
Edited by:
Tracey Weiland, The University of Melbourne, AustraliaReviewed by:
Claudia Kimie Suemoto, University of São Paulo, BrazilDieter J. Meyerhoff, University of California, San Francisco, United States
Ernest Lo, Institut National de Santé Publique du Québec, Canada
Copyright © 2019 Croll, Bos, Ikram, Rivadeneira, Voortman and Vernooij. This is an open-access article distributed under the terms of the Creative Commons Attribution License (CC BY). The use, distribution or reproduction in other forums is permitted, provided the original author(s) and the copyright owner(s) are credited and that the original publication in this journal is cited, in accordance with accepted academic practice. No use, distribution or reproduction is permitted which does not comply with these terms.
*Correspondence: Meike W. Vernooij, m.vernooij@erasmusmc.nl