- 1Geriatrics, Neuroscience, Orthopaedics, Head and Neck Department, Fondazione Policlinico Universitario A. Gemelli IRCCS, Rome, Italy
- 2Department of Neuroscience, Mental Health and Sensory Organs, Faculty of Medicine and Psychology, Centre for Experimental Neurological Therapies, S. Andrea Hospital, Sapienza University, Rome, Italy
- 3Department of Human Science and Promotion of Quality of Life, San Raffaele Roma Open University, Rome, Italy
- 4Department of Informatics & Analytics, Dana-Farber Cancer Institute, Boston, MA, United States
- 5Massachusetts Institute of Technology, Cambridge, MA, United States
- 6IRCCS Istituto Neurologico Mediterraneo (INM) Neuromed, Pozzilli, Italy
The emerging concept of a crosstalk between hemostasis, inflammation, and immune system prompt recent works on coagulation cascade in multiple sclerosis (MS). Studies on MS pathology identified several coagulation factors since the beginning of the disease pathophysiology: fibrin deposition with breakdown of blood brain barrier, and coagulation factors within active plaques may exert pathogenic role, especially through the innate immune system. Studies on circulating coagulation factors showed complex imbalance involving several components of hemostasis cascade (thrombin, factor X, factor XII). To analyze the role of the coagulation process in connection with other pathogenic pathways, we implemented a systematic matching of genome-wide association studies (GWAS) data with an informative and unbiased network of coagulation pathways. Using MetaCore (version 6.35 build 69300, 2018) we analyzed the connectivity (i.e., direct and indirect interactions among two networks) between the network of the coagulation process and the network resulting from feeding into MetaCore the MS GWAS data. The two networks presented a remarkable over-connectivity: 958 connections vs. 561 expected by chance; z-score = 17.39; p-value < 0.00001. Moreover, genes coding for cluster of differentiation 40 (CD40) and plasminogen activator, urokinase (PLAU) shared both networks, pointed to an integral interplay between coagulation cascade and main pathogenic immune effectors. In fact, CD40 pathways is especially operative in B cells, that are currently a major therapeutic target in MS field. The potential interaction of PLAU with a signal of paramount importance for B cell pathogenicity, such as CD40, suggest new lines of research and pave the way to implement new therapeutic targets.
Introduction
Recent studies focused on the role of coagulation cascade in neuroinflammation and neurodegenerative disease, considering new suggestions on a crosstalk between hemostasis, inflammation and immune system (1). The majority of these studies regarded multiple sclerosis (MS), but others demonstrated a dysregulation of several proteins of the coagulation cascade in many other central nervous system (CNS) diseases: traumatic brain and spinal cord injury, Parkinson disease, amyotrophic lateral sclerosis, Huntington disease and Alzheimer dementia (2–6).
A recent review discussed the role of fibrinogen in some neurological diseases, with an emphasis on the cellular targets and the fibrinogen-induced signal transduction pathways in the CNS: fibrinogen has a pleiotropic role in the activation of inflammation and pathologies that share, as common change, the increased blood-brain barrier (BBB) permeability. This produces the extravasation of plasma proteins that are undetectable in a healthy CNS, but abundantly deposited in many neurological conditions, whereby they mediate both pathological inflammation and tissue repair (7).
In MS the BBB breakdown and activation of the innate immune system appears to be an early event in the diseases development, that may precede the clinical onset. Different studies showed that fibrin deposition is a leading feature of MS pathology and it is presents all over the disease course (7). Fibrinogen can directly activate microglia cells in vitro and increase their phagocytic ability by binding to the integrin receptor CD11b/CD18, which is specifically expressed in the CNS (8). Participation of the coagulation cascade to the neuropathology of MS was strongly suggested by a proteomic analysis on laser-micro dissected, post-mortem brain lesions. Comparative proteomic profiles identified tissue factor and protein C inhibitor within chronic active plaque samples. In vivo experiments with antagonists of the coagulation factors identified (hirudin or recombinant activated protein C) were capable of ameliorating animal models of MS and suppressing pathogenic immune effectors, confirming the impact of dysregulated coagulation factors on demyelinating processes and suggesting potential therapeutic targets (9).
Another approach focused on the study of circulating coagulation factors, as possible biomarkers and targets of treatment tactics in MS pathogenic process. Gobel et al. (10) studied different neurological diseases (all the forms of MS, neuro myelitis optica spectrum disorders, other inflammatory neurological diseases, and non-inflammatory neurological conditions) compared to healthy status. The plasma levels of different coagulation proteins measured and the results demonstrated significantly higher levels of prothrombin and factor X in MS patients, without significant changes in the other conditions. Thrombin produces different inflammatory responses, including platelet activation, vasodilatation, leukocyte attraction, production of cytokine, and chemokine (IL-1, IL-6, TNFα) (11). These effects in CNS are also dependent on thrombin concentration: at low-to-moderate concentrations, it protects hippocampal neurons and astrocytes from insults, while at higher concentrations thrombin induces cell death (12, 13). Another coagulation factor that proved to be somehow involved in MS pathogenic process was factor XII (FXII). Increased FXII levels and reduced function within the intrinsic coagulation pathway were evident in people with MS (14); Gobel et al. found high levels of FXII activity in the plasma of MS patients during relapse, and immune activating effects mediated by interactions between FXII and dendritic cells in a CD87-dependent manner (15).
The above studies [with the prominent exception of the proteomic analysis by Han et al. (9)] were planned with a hypothesis-driven approach focusing on single factors of coagulation cascade. The coming of genome-wide association studies (GWAS) data would allow unbiased approaches capable of disclosing a more extensive landscape of coagulation process involvement in MS pathogenesis. GWAS results are derived from population-based association studies, comparing disease cases and controls for common genetic variants, that have variable frequencies in the general population. Each common variants (signaled by a single nucleotide polymorphism) explain a small fraction of the risk/protection in a population. The overall MS genetic risk is multifaceted: many common variants of small effect spread throughout the genome, loci of stronger effects lying in the human leukocyte antigen (HLA) haplotype, that had been associated to disease risk since eighties, as well as recently described low-frequency and rare-coding variants all contribute to the complex genetic architecture of MS (16).
GWAS Studies And Coagulation
GWAS studies encompassing the last decade have identified more than 200 MS-associated loci across the human genome (17). Technological advances, adequate increase of sample size, and improved statistical approaches have all contributed to a substantial progress in the definition of the complex genetic architecture of MS. This prompted a significant extension of the view on MS genetics, that was essentially limited to the role of human histocompatibility haplo types until 15 years ago. At least two challenges remain: (i) the definition of a comprehensive etiological model, with the need of better understanding both the plausibly causal effects in altering disease risk for many of the susceptibility gene regions identified, and the impact of non-genetic factors, as demonstrated, among others, by twin studies (18, 19); (ii) the clinical translation of genomic data, that may exploit the relevance of pathogenic pathways, for which therapeutics is already available in clinical practice, or may drive the discovery of new druggable targets.
One potentially informative approach to deal with these issues includes bioinformatics attempts capable of extracting from GWAS data the biological consequences and the functional implications of individual disease-associated variants. Our group implemented analyses aimed at clarifying the interplay between diseases-associated genomic regions and presumed causal environmental factors (20–22). Another bioinformatics reworking allows to explore the reciprocal interactions of pathways resulting from GWAS data, to disclose unknown networks and to focus on previously under estimated pathways in MS etiology.
By applying the latter approach we used bioinformatics tools to analyze the role of the coagulation cascade in connection with other biological pathways contributing to the complex disease pathogenesis. Using MetaCore (version 6.35 build 69300, 2018) we analyzed the connectivity (i.e., direct and indirect interactions among two networks) between the network of the coagulation process (a standard map in MetaCore, presented in Figure 1, that includes 94 components) and the network resulting from feeding into MetaCore the MS GWAS data. In particular we considered genes that were reported in 19 MS GWAS studies (23–41) filed in the GWAS Catalog (https://www.ebi.ac.uk/gwas); such list (Supplementary Table 1) contains 398 genes, that were either reported as associated to MS in the aforementioned studies, or that were originally reported as hits on non-well specified regions, later mapped to better characterized regions and genes. The connectivity analysis in MetaCore takes place in two steps: first, the genes that are shared by the two networks (i.e., elements that appear in both the coagulation process network and the MS GWAS network) are identified; second, every element in each network is enriched with its interactors. A statistics is then computed counting how many interactions are observed among the two enriched networks, comparing this number to what would be expected by chance. MetaCore connectivity analysis showed the following results: the coagulation process network and the MS GWAS network presented a remarkable over-connectivity, showing 958 connections (561 were expected by chance) that lead to z-score of 17.39 and p-value < 0.00001; genes coding for cluster of differentiation 40 (CD40) and plasminogen activator, urokinase (PLAU) appeared both in the coagulation process network and the MS GWAS network (Figure 2).
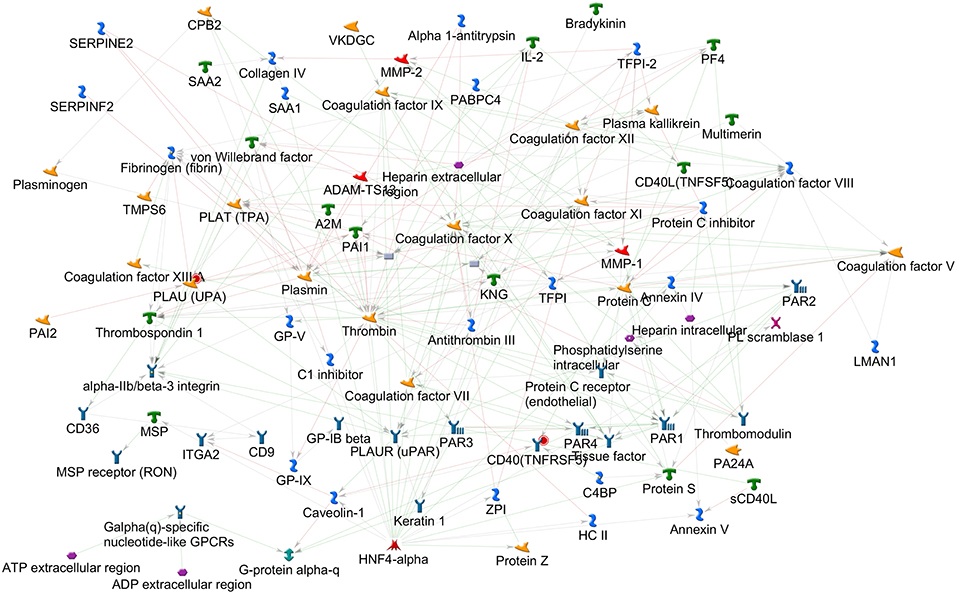
Figure 1. Coagulation process map, as available in MetaCore 2018. The elements on this map, together with their interactors, were used for the connectivity analysis.
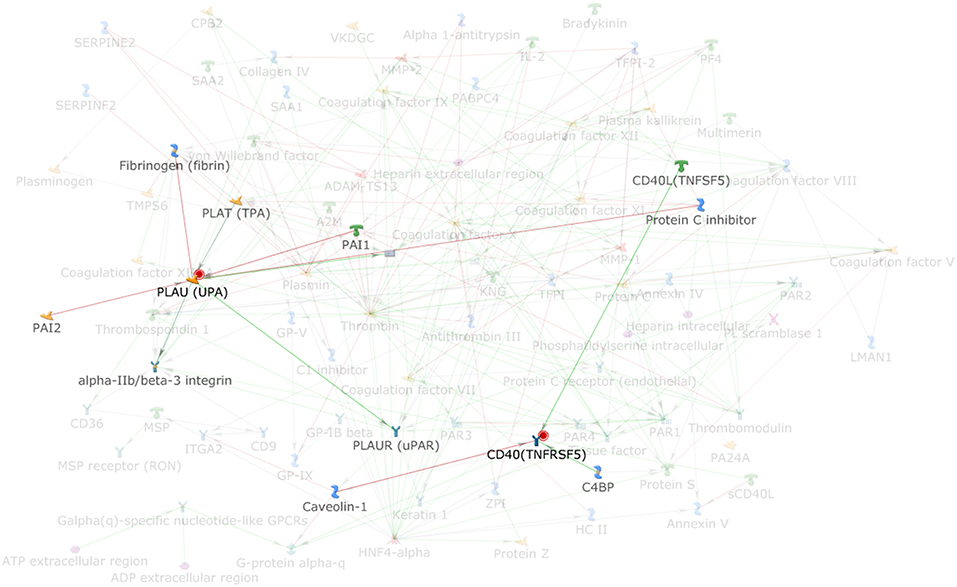
Figure 2. Highlight of the elements (PLAU and CD40) that are shared among the coagulation process map and the MS GWAS gene list; PLAU and CD40 interactors are also highlighted.
These analyses on one hand confirm that the coagulation cascade may have an impact on MS development, as already reported (see above), on the other hand fail to detect main coagulation components previously indicated by experimental studies. This limitation may pertain the analyses based on GWAS studies in general, which incorporate huge number of gene variants and several levels of possible functional complexities. Specifically, PLAU pathway has already been scrutinized for its role in the activation of matrix metallopeptidase9, that has in turn been associated with BBB breakdown, a crucial event in MS development (42). However, the network sharing by PLAU and CD40 pathways, resulting from our analysis, points to a more integral interplay between coagulation cascade and immune effectors, that are currently the main focus of research on MS etiopathogenesis and therapy. CD40 pathways is especially operative in B cells, being the typical signal mediating help by T cells (through CD40 ligand) on cognate B lymphocytes for antibody production and other important functions, such as antigen presenting cells and cells modulating the immune response. Recent studies show indeed that MS-associated genetic variants alter the expression of co-stimulatory molecule, including CD40 in B cells, as well as the level of steering cytokines such as interleukin-10, which is considered to have an immunoregulatory function downstream of CD40 (43). Moreover, the CD40-CD40 ligand dyadis intensively investigated for its essential role in the development of MS, with the aim of targeting it therapeutically and antagonize neuroinflammation (44).
The role of CD40 pathway in MS development refers to the more general topic of the role of B cells in neuroinflammation. Our recent works suggest that B lymphocytes, in an activated and pro-survival status, contribute to MS development with functions other than antibody-production (45). Indeed, B lymphocytes are professional antigen-presenting cells for autoreactive T cells (43, 46), as well as potent producers of steering cytokines and other immune effectors influencing both pathogenic (lymphotoxin, tumor necrosis factor, granulocyte macrophage-colony stimulating factor, and metallo-peptidases) and protective (interleukin 10) milieus in neuroinflammation (47–49). Accordingly, CD20-targeted monoclonal antibodies, that deplete B cells in their earlier stages of development, turned out to be highly and consistently effective in tackling the disease development (50, 51). Hence, the finding that PLAU pathway may potentially interact with a signal of paramount importance for B cell pathogenicity, such as CD40, may open new perspectives for translational research. Along this line, the protease activity of microglial cells activated by urokinase plasminogen activator coupled with its receptor seems very important for their pathogenic role in MS (52) and, notably, this pathogenic role is increasingly recognized also in a very recent GWAS study on MS.
Conclusion
The case of the relationship between coagulation pathway and MS molecular model may teach us how fruitful a bioinformatics reworking of GWAS data may be. In particular bioinformatics approaches that match GWAS data with other biological repositories of unbiased comprehensive records may shed light on the functional relevance of common diseases-associated single nucleotide polymorphism: each genetic variant is often located in regulatory genomic regions, and may be active in different ways in diverse tissues, making it very difficult to encompass a detailed understanding of the underpinning pathobiology.
Future works based on connectivity analyses may inform a number of questions that are still open in the context of MS heritability: the degree of epistasis and interaction with non-genetic causative factors; the existence of genetic interactors determining disease forms, clinical course, and response to diseases modifying therapies; the predictivity of endophenotypes, in particular the imaging data, that often segregate on a familiar basis. Moreover, the discovery of “clinically actionable genes” may represent a timely task in the current landscape of MS therapeutics.
Many new diseases modifying therapies, already available in clinical practice, show superior effectiveness compared to the treatments that were in place only a decade ago. The “cost” is the safety profile, being at least suboptimal. Approaches based on drugs targeting PLAU system, that have successfully been used to ameliorate CNS inflammation (53, 54), may be potential resources, with good therapeutic index and synergic action with currently available immune-modulators, potentially to be exploited in combination schemes.
Author Contributions
All authors listed have made a substantial, direct and intellectual contribution to the work, and approved it for publication.
Conflict of Interest Statement
The authors declare that the research was conducted in the absence of any commercial or financial relationships that could be construed as a potential conflict of interest.
Supplementary Material
The Supplementary Material for this article can be found online at: https://www.frontiersin.org/articles/10.3389/fneur.2019.00095/full#supplementary-material
Supplementary Table 1. List of genes that were gathered from the GWAS Catalog as associated with MS and that were used for this work.
Abbreviations
GWAS, genome-wide association studies; PLAU, plasminogen activator, urokinase.
References
1. Langer HF, Chavakis T. Platelets and neurovascular inflammation. Thromb Haemost. (2013) 110:888–93. doi: 10.1160/TH13-02-0096
2. Hay JR, Johnson VE, Young AM, Smith DH, Stewart W. Blood–brain barrier disruption is an early event that may persist for many years after traumatic brain injury in humans. J. NeuropatholExpNeurol. (2015) 74:1147–57. doi: 10.1097/NEN.0000000000000261
3. Gray MT, Woulfe JM. Striatal blood–brain barrier permeability in Parkinson's disease. J Cereb Blood Flow Metab. (2015) 35:747–50. doi: 10.1038/jcbfm.2015.32
4. Winkler EA, Sengillo JD, Sullivan JS, Henkel JS, Appel SH, Zlokovic BV. Blood-spinal cord barrier breakdown and pericyte reductions in amyotrophic lateral sclerosis. Acta Neuropathol. (2013) 125:111–20. doi: 10.1007/s00401-012-1039-8
5. Drouin-Ouellet J, Sawiak SJ, Cisbani G, Lagacé M, Kuan WL, Saint-Pierre M, et al. Cerebrovascular and blood-brain barrier impairments in Huntington's disease: potential implications for its pathophysiology. Ann Neurol. (2015) 2015:160–77. doi: 10.1002/ana.24406
6. Cortes-Canteli M, Mattei L, Richards AT, Norris EH, Strickland S. Fibrin deposited in the Alzheimer's disease brain promotes neuronal degeneration. Neurobiol Aging (2015) 36:608–17. doi: 10.1016/j.neurobiolaging.2014.10.030
7. Petersen MA, Kyu J, Akassoglou K. Fibrinogen in neurological disease: mechanisms, imaging and therapeutics. Nat Rev Neurosci. (2018) 19:283–301. doi: 10.1038/nrn.2018.13
8. Adams RA, Bauer J, Flick MJ, Sikorski SL, Nuriel T, Lassmann H, et al. The fibrin-derived gamma377–395 peptide inhibits microglia activation and suppresses relapsing paralysis in central nervous system autoimmune disease. J Exp Med. (2007) 204:571–82. doi: 10.1084/jem.20061931
9. Han MH, Hwang SI, Roy DB, Lundgren DH, Price JV, Ousman SS, et al. Proteomic analysis of active multiple sclerosis lesions reveals therapeutic targets. Nature (2008) 451:1076–81. doi: 10.1038/nature06559
10. Göbel K, Kraft P, Pankratz S, Gross CC, Korsukewitz C, Kwiecien R, et al. Prothrombin and factor X are elevated in multiple sclerosis patients. Ann Neurol. (2016) 80:946–51. doi: 10.1002/ana.24807
11. Schoenmakers SH, Reitsma PH, Spek CA. Blood coagulation factors as inflammatory mediators. Blood Cells Mol Dis. (2005) 34:30–7. doi: 10.1016/j.bcmd.2004.09.001
12. Donovan FM, Cunningham DD. Signaling pathways involved in thrombin-induced cell protection. J BiolChem. (1998) 273:12746–52. doi: 10.1074/jbc.273.21.12746
13. Pike CJ, Vaughan PJ, Cunningham DD, Cotman CW. Thrombin attenuates neuronal cell death and modulates astrocyte reactivity induced by beta-amyloid in vitro. J Neurochem. (1996) 66:1374–82. doi: 10.1046/j.1471-4159.1996.66041374.x
14. Ziliotto N, Baroni M, Straudi S, Manfredini F, Mari R, Menegatti E, et al. Coagulation factor XII levels and intrinsic thrombin generation in multiple sclerosis. Front Neurol. (2018) 9:245. doi: 10.3389/fneur.2018.00245
15. Göbel K, Pankratz S, Asaridou CM, Herrmann AM, Bittner S, Merker M, et al. Blood coagulation factor XII drives adaptive immunity during neuroinflammation via CD87-mediated modulation of dendritic cells. Nat Commun. (2016) 7:11626. doi: 10.1038/ncomms11626
16. International Multiple Sclerosis Genetics Consortium. Low-Frequency and Rare-Coding Variation Contributes to Multiple Sclerosis Risk. Cell (2018) 175:1679–87.e7. doi: 10.1016/j.cell.2018.09.049
17. Cotsapas C, Mitrovic M. Genome-wide association studies of multiple sclerosis. Clin Transl Immunol. (2018) 7:e1018. doi: 10.1002/cti2.1018
18. Fagnani C, Neale MC, Nisticò L, Stazi MA, Ricigliano VA, Buscarinu MC, et al. Twin studies in multiple sclerosis: a meta-estimation of heritability and environmentality. Mult Scler. (2015) 21:1404–13. doi: 10.1177/1352458514564492
19. Ristori G, Cannoni S, Stazi MA, Vanacore N, Cotichini R, Alfò M, et al. Multiple sclerosis in twins from continental Italy and Sardinia: a nationwide study. Ann Neurol. (2006) 59:27–34. doi: 10.1002/ana.20683
20. Mechelli R, Umeton R, Policano C, Annibali A International Multiple Sclerosis Genetics Consortium Wellcome Trust Case Control Consortium, et al. A “candidate-interactome” aggregate analysis of genome-wide association data in multiple sclerosis. PLoS ONE (2013) 8:e63300. doi: 10.1371/journal.pone.0063300
21. Ricigliano VA, Handel AE, Sandve GK, Annibali V, Ristori G, Mechelli R, et al. EBNA2 binds to genomic intervals associated with multiple sclerosis and overlaps with vitamin D receptor occupancy. PLoS ONE (2015) 10:e011960. doi: 10.1371/journal.pone.0119605
22. Mechelli R, Manzari C, Policano C, Annese A, Picardi E, Umeton R, et al. Epstein-Barr virus genetic variants are associated with multiple sclerosis. Neurology (2015) 84:1362–8. doi: 10.1212/WNL.0000000000001420
23. Briggs FB, Shao X, Goldstein BA, Oksenberg JR, Barcellos LF, de Jager PL. International Multiple Sclerosis Genetics Consortium. Genome-wide association study of severity in multiple sclerosis. Genes Immun. (2011) 12:615–25. doi: 10.1038/gene.2011.34
24. Wang JH, Pappas D, De Jager PL, Pelletier D, de Bakker PI, Kappos L, et al. Australian and New Zealand Multiple Sclerosis Genetics Consortium (ANZgene). Modeling the cumulative genetic risk for multiple sclerosis from genome-wide association data. Genome Med. (2011) 3:3. doi: 10.1186/gm217
25. Nischwitz S, Cepok S, Kroner A, Wolf C, Knop M, Müller-Sarnowski F, et al. Evidence for VAV2 and ZNF433 as susceptibility genes for multiple sclerosis. J Neuroimmunol. (2010) 227:162–6. doi: 10.1016/j.jneuroim.2010.06.00
26. Aulchenko YS, Hoppenbrouwers IA, Ramagopalan SV, Broer L, Jafari N, Hillert J, et al. Genetic variation in the KIF1B locus influences susceptibility to multiple sclerosis. Nat Genet. (2008) 40:1402–3. doi: 10.1038/ng.251
27. Comabella M, Craig DW, Camiña-Tato M, Morcillo C, Lopez C, Navarro A, et al. Identification of a novel risk locus for multiple sclerosis at 13q31.3 by a pooled genome-wide scan of 500,000 single nucleotide polymorphisms. PLoS ONE (2008) 3:e3490. doi: 10.1371/journal.pone.0003490
28. International Multiple Sclerosis Genetics Consortium Hafler DA, Compston A, Sawcer S, Lander ES, Daly MJ, et al. Risk alleles for multiple sclerosis identified by a genomewide study. N Engl J Med. (2007) 357:851–62. doi: 10.1056/NEJMoa073493
29. Gourraud PA, Sdika M, Khankhanian P, Henry RG, Beheshtian A, Matthews PM, et al. A genome-wide association study of brain lesion distribution in multiple sclerosis. Brain (2013) 136(Pt 4):1012–24. doi: 10.1093/brain/aws363
30. Matesanz F, González-Pérez A, Lucas M, Sanna S, Gayán J, Urcelay E, et al. Genome-wide association study of multiple sclerosis confirms a novel locus at 5p13.1. PLoS ONE (2012) 7:e36140. doi: 10.1371/journal.pone.0036140
31. Martinelli-Boneschi F, Esposito F, Brambilla P, Lindström E, Lavorgna G, Stankovich J, et al. A genome-wide association study in progressive multiple sclerosis. Mult Scler. (2012) 18:1384–94. doi: 10.1177/1352458512439118
32. Sanna S, Pitzalis M, Zoledziewska M, Zara I, Sidore C, Murru R, et al. Variants within the immunoregulatory CBLB gene are associated with multiple sclerosis. Nat Genet. (2010) 42:495–7. doi: 10.1038/ng.584
33. Jakkula E, Leppä V, Sulonen AM, Varilo T, Kallio S, Kemppinen A, et al. Genome-wide association study in a high-risk isolate for multiple sclerosis reveals associated variants in STAT3 gene. Am J Hum Genet. (2010) 86:285–91. doi: 10.1016/j.ajhg.2010.01.017
34. Baranzini SE, Wang J, Gibson RA, Galwey N, Naegelin Y, Barkhof F, et al. Genome-wide association analysis of susceptibility and clinical phenotype in multiple sclerosis. Hum Mol Genet. (2009) 18:767–78. doi: 10.1093/hmg/ddn388
35. Bahlo M, Booth DR, Broadley SA, Brown MA, Foote SJ, Griffiths LR, et al. Australia and New Zealand Multiple Sclerosis Genetics Consortium (ANZgene). Genome-wide association study identifies new multiple sclerosis susceptibility loci on chromosomes 12 and 20. Nat Genet. (2009) 41:824–8. doi: 10.1038/ng.396
36. De Jager PL, Jia X, Wang J, de Bakker PI, Ottoboni L, Aggarwal NT International MS Genetics Consortium, et al. Meta-analysis of genome scans and replication identify CD6, IRF8 and TNFRSF1A as new multiple sclerosis susceptibility loci. Nat Genet. (2009) 41:776–82. doi: 10.1038/ng.401
37. Steri M, Orrù V, Idda ML, Pitzalis M, Pala M, Zara I, et al. Overexpression of the Cytokine BAFF and Autoimmunity Risk. N Engl J Med. (2017) 376:1615–26. doi: 10.1056/NEJMoa1610528
38. Patsopoulos NA, Bayer Pharma MS Genetics Working Group Steering Committees of Studies Evaluating IFNβ-1b and a CCR1-Antagonist ANZgene Consortium GeneMSA International Multiple Sclerosis Genetics Consortium Esposito F, et al. Genome-wide meta-analysis identifies novel multiple sclerosis susceptibility loci. Ann Neurol. (2011) 70:897–912. doi: 10.1002/ana.22609
39. Andlauer TF, Buck D, Antony G, Bayas A, Bechmann L, Berthele A, et al. Novel multiple sclerosis susceptibility loci implicated in epigenetic regulation. Sci Adv. (2016) 2:e1501678. doi: 10.1126/sciadv.1501678
40. International Multiple Sclerosis Genetics Consortium (IMSGC) Trust Case Control Consortium 2 (WTCCC2); International IBD Genetics Consortium (IIBDGC) Beecham AH, et al. Analysis of immune-related loci identifies 48 new susceptibility variants for multiple sclerosis. Nat Genet. (2013) 45:1353–60. doi: 10.1038/ng.2770
41. International Multiple Sclerosis Genetics Consortium; Welcome Trust Case Control Consortium 2 Sawcer S, Hellenthal G, Pirinen M, Spencer CC. Genetic risk and a primary role for cell-mediated immune mechanisms in multiple sclerosis. Nature (2011) 476:214–9. doi: 10.1038/nature10251
42. Cox MB, Bowden NA, Scott RJ, Lechner-Scott. Altered expression of the plasminogen activation pathway in peripheral blood mononuclear cells in multiple sclerosis: possible pathomechanism of matrix metalloproteinase activation. J Mult Scler. (2013) 19:1268–74. doi: 10.1177/1352458513475493
43. Smets I, Fiddes B, Garcia-Perez JE, He D, Mallants K, Liao W, et al. Multiple sclerosis risk variants alter expression of co-stimulatory genes in B cells. Brain (2018) 141:786–96. doi: 10.1093/brain/awx372
44. Aarts SABM, Seijkens TTP, van Dorst KJF, Dijkstra CD, Kooij G, Lutgens E. The CD40-CD40L dyad in experimental autoimmune encephalomyelitis and multiple sclerosis. Front Immunol. (2017) 8:1791. doi: 10.3389/fimmu.2017.01791
45. Annibali V, Umeton R, Palermo A, Severa M, Etna MP, Giglio S, et al. Analysis of coding and non-coding transcriptome of peripheral B cells reveals an altered interferon response factor (IRF)-1 pathway in multiple sclerosis patients. J Neuroimmunol. (2018) 324:165–71. doi: 10.1016/j.jneuroim.2018.09.005
46. Mathias A, Perriard G, Canales M, Soneson C, Delorenzi M, Schluep M, et al. Increased ex vivo antigenpresentation profile of B cells in Multiple Sclerosis. Mult Scler. (2017) 23:802–9. doi: 10.1177/1352458516664210
47. Miyazaki Y, Li R, Rezk A, Misirliyan H, Moore C, Farooqi N, et al. A novel microRNA-132-sirtuin-1 axis underlies aberrant B-cell cytokine regulation in patients with relapsing-remitting multiple sclerosis. PLoS ONE (2014) 9:e10542. doi: 10.1371/journal.pone.0105421
48. Li R, Patterson KR, Bar-Or A. Reassessing B cell contributions in multiple sclerosis. Nat Immunol. (2018) 19:696–707. doi: 10.1038/s41590-018-0135-x
49. Aung LL, Mouradian MM, Dhib-Jalbut S, Balashov KE. MMP-9 expression is increased in B lymphocytes during multiple sclerosis exacerbation and is regulated by microRNA-320a. J Neuroimmunol. (2015) 278:185–9. doi: 10.1016/j.jneuroim.2014.11.004
50. Montalban X, Hauser SL, Kappos L, Arnold DL, Bar-Or A, Comi G, et al. ORATORIO Clinical Investigators. Ocrelizumab versus placebo in primary progressive multiple sclerosis. N Engl J Med. (2017) 376:209–20. doi: 10.1056/NEJMoa1606468
51. Hauser SL, Bar-Or A, Comi G, Giovannoni G, Hartung HP, Hemmer B, et al. OPERA I and OPERA II clinical investigators. Ocrelizumab versus interferon beta-1a in relapsing multiple sclerosis. N Engl J Med. (2017) 376:221–34. doi: 10.1056/NEJMoa1601277
52. Washington RA, Becher B, Balabanov R, Antel J, Dore-Duffy P. Expression of the activation marker urokinase plasminogen-activator receptor in cultured human central nervous system microglia.J Neurosci Res. (1996) 45:392–9. doi: 10.1002/(SICI)1097-4547(19960815)45:4/<392::AID-JNR8>3.0.CO;2-4
53. Gur-Wahnon D, Mizrachi T, Maaravi-Pinto FY, Lourbopoulos A, Grigoriadis N, Higazi AA, et al. The plasminogen activator system: involvement in central nervous system inflammation and a potential site for therapeutic intervention. J Neuroinflamm. (2013) 10:124. doi: 10.1186/1742-2094-10-124.
Keywords: multiple sclerosis, genome-wide association studies, cluster of differentiation 40, plasminogen activator, urokinase gene, connectivity analysis
Citation: La Starza S, Ferraldeschi M, Buscarinu MC, Romano S, Fornasiero A, Mechelli R, Umeton R, Ristori G and Salvetti M (2019) Genome-Wide Multiple Sclerosis Association Data and Coagulation. Front. Neurol. 10:95. doi: 10.3389/fneur.2019.00095
Received: 30 September 2018; Accepted: 24 January 2019;
Published: 14 February 2019.
Edited by:
Matilde Inglese, Icahn School of Medicine at Mount Sinai, United StatesReviewed by:
Kerstin Göbel, Universität Münster, GermanyFrancesco Bernardi, University of Ferrara, Italy
Copyright © 2019 La Starza, Ferraldeschi, Buscarinu, Romano, Fornasiero, Mechelli, Umeton, Ristori and Salvetti. This is an open-access article distributed under the terms of the Creative Commons Attribution License (CC BY). The use, distribution or reproduction in other forums is permitted, provided the original author(s) and the copyright owner(s) are credited and that the original publication in this journal is cited, in accordance with accepted academic practice. No use, distribution or reproduction is permitted which does not comply with these terms.
*Correspondence: Renato Umeton, Renato_Umeton@DFCI.Harvard.edu
Giovanni Ristori, giovanni.ristori@uniroma1.it