- 1Clinical Imaging Sciences Centre, Brighton and Sussex Medical School, Falmer, United Kingdom
- 2Hurstwood Park Neurological Centre, Haywards Heath, West Sussex, United Kingdom
- 3Department of Neuroscience, Trafford Centre for Biomedical Research, Brighton and Sussex Medical School, Falmer, United Kingdom
Objective markers of disease sensitive to the clinical activity, symptomatic progression, and underlying substrates of neurodegeneration are highly coveted in amyotrophic lateral sclerosis in order to more eloquently stratify the highly heterogeneous phenotype and facilitate the discovery of effective disease modifying treatments for patients. Magnetic resonance imaging (MRI) is a promising, non-invasive biomarker candidate whose acquisition techniques and analysis methods are undergoing constant evolution in the pursuit of parameters which more closely represent biologically-applicable tissue changes. Neurite Orientation Dispersion and Density Imaging (NODDI; a form of diffusion imaging), and quantitative Magnetization Transfer Imaging (qMTi) are two such emerging modalities which have each broadened the understanding of other neurological disorders and have the potential to provide new insights into structural alterations initiated by the disease process in ALS. Furthermore, novel neuroimaging data analysis approaches such as Event-Based Modeling (EBM) may be able to circumvent the requirement for longitudinal scanning as a means to comprehend the dynamic stages of neurodegeneration in vivo. Combining these and other innovative imaging protocols with more sophisticated techniques to analyse ever-increasing datasets holds the exciting prospect of transforming understanding of the biological processes and temporal evolution of the ALS syndrome, and can only benefit from multicentre collaboration across the entire ALS research community.
Neuroimaging modalities sensitive to the dynamics and patterns of tissue degeneration in amyotrophic lateral sclerosis (ALS) are required as objective biological markers of disease activity in vivo. Standard clinical assessment is usually adequate for diagnosis, however there is a pressing need for non-invasive neuroimaging biomarkers that may differentiate between the various phenotypes within the ALS syndrome, provide more accurate prognostic information, and monitor responses to therapeutic interventions. There is also a need for neuroimaging techniques which have the potential to interrogate the specific mechanisms of neurodegeneration, given that conventional MRI primarily aims to exclude alternative diagnoses (1). As such, it will be important to integrate new modalities of structural and functional imaging (including MRI and PET) with molecular biomarkers of neuronal damage, and indicators of neuroinflammation if the therapeutic impasse for more effective disease treatments is to be broken. Diffusion MRI, particularly diffusion tensor imaging (DTI), has been extensively researched in patients with ALS to infer structural alterations within the brain and spinal cord by virtue of the movement of water molecules induced by magnetic field gradients. Fractional anisotropy (FA) is consistently reduced, often alongside increased mean or radial diffusivity (MD or RD, respectively), within the corticospinal tracts (CSTs) (2–15) and body of the corpus callosum through which pass the fibers connecting hemispheric motor areas (3, 5–8, 10, 12, 16, 17). Indeed, DTI changes are perhaps most reliably encountered within the posterior limb of the internal capsule (18, 19) which forms a common conduit for several descending motor pathways including the CST, cortico-rubro-spinal, and cortico-reticulo-spinal connections (20). Additional areas within the frontal, temporal (11, 21, 22), and parietal areas (11, 23) have shown reduced FA, all of which is consistent with the multisystem motor and extra-motor regions involved clinically and neuropathologically (24–26). Nevertheless, establishing the precise substrate or substrates underlying these changes observed on MRI is not straightforward and may be complimented by novel magnetic resonance imaging techniques and emerging big data analysis methods.
Neurite Orientation Dispersion and Density Imaging (NODDI)
Diffusion MRI is sensitive to the motion of water molecules at microscopic level. Nevertheless the signal it measures is averaged across volumes of 1–2 mm3 (the so-called “voxel”). For this reason, any interpretation of the signal and its origin requires some degree of “modeling.” More than one model has been proposed and each typically incorporates slightly differing mathematical assumptions to interpret and model the signal, thus providing only indirect inferences on anatomical configurations. For instance, DTI assumes that water movement will obey Gaussian properties and is widely accepted to lose consistency when neuronal fibers bend or fan out within a voxel, or where otherwise aligned fiber tracts are crossing each other (5) which is common to areas such as the centrum semiovale and even regions of the foliated corpus callosum (27, 28). Moreover, a reduction in FA signifies changes in both neurite density and orientation dispersion without distinguishing their individual contributions (28, 29). Therefore, variations on the diffusion tensor model have been created in an attempt to address these limitations. One such model is neurite orientation dispersion and density imaging (NODDI).
NODDI requires acquisition over a longer time than DTI and compartmentalizes non-Gaussian water diffusion into three geometric spaces encompassing isotropic (or free), hindered anisotropic and restricted anisotropic components. These are known as VISO, VIC, and VEC and each broadly correspond to free water/CSF, intra-neurite water (of axons and dendrites), and extra-neurite water (but potentially including glial cells and neuronal somata), respectively (29–31). The NODDI parameters ISO, NDI (neurite density index), and ODI (orientation dispersion index; a marker of the geometric complexity of neurites) can then be derived, the latter two of which are considered to provide a more structurally useful breakdown of single FA values (29) (see Figure 1). NODDI is able to better delineate white from gray matter, in which normal white matter displays higher NDI and lower ODI with the reverse in gray matter (33), and differentiate between different gray matter structures although might be more susceptible to changes in field strength in these areas (31). Compared to DTI, NODDI indices, particularly ODI, have been shown to correlate with histological measures of orientation dispersion in the spinal cord and might also display more inter-subject variability with implications for the sample sizes required for group analyses (33, 34). However, this may not necessarily be an inaccuracy in modeling rather a more accurate depiction of tissue composition (31). In addition, regions which might be expected to demonstrate considerable axon density and higher NDI values might counterintuitively show higher ISO due to the larger diameter axons enabling more freedom of water movement (31, 34).
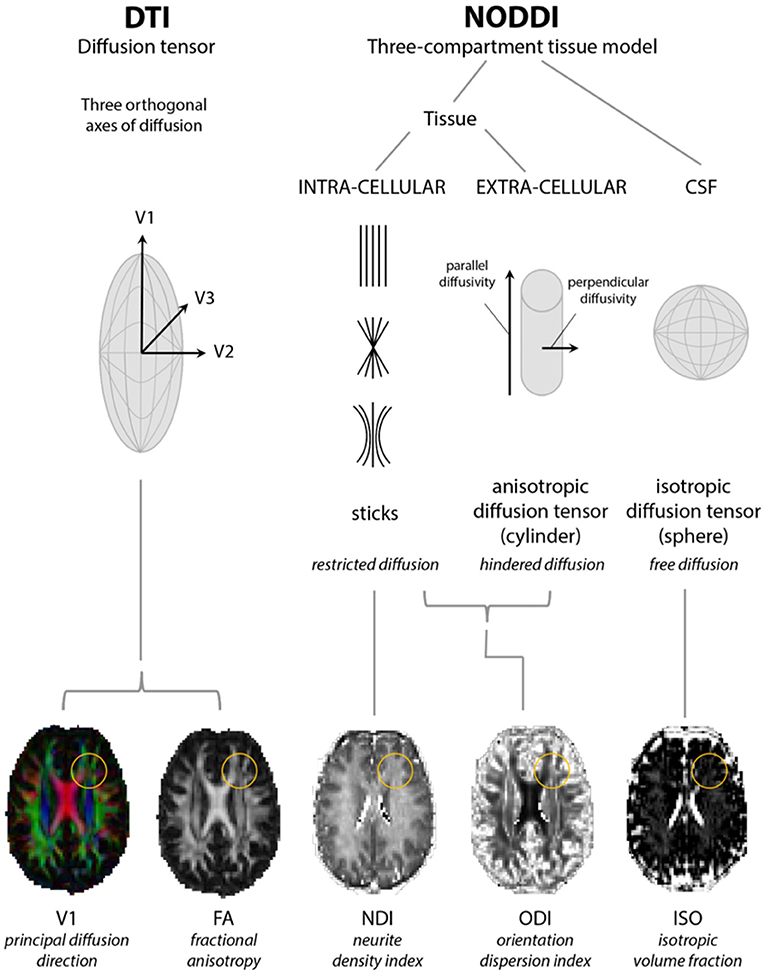
Figure 1. Models of diffusion for neurite orientation dispersion and density imaging (NODDI) and diffusion tensor imaging (DTI). The diffusion tensor model of DTI is based upon three orthogonal axes of diffusion (V1, V2, and V3) yielding radial, axial, and mean diffusivity from which fractional anisotropy (FA) can be estimated. NODDI considers diffusion within three compartments: restricted diffusion in the intracellular compartment, hindered diffusion in the extracellular compartment, and free diffusion in cerebrospinal fluid (CSF), from which parameter maps representing neurite density (NDI), orientation dispersion (ODI), and isotropic fraction (ISO) indices can be estimated. Yellow circles highlight a region where changes in FA can be accompanied by changes in both NDI and ODI. Adapted from Rae et al. (32).
NODDI has been used to demonstrate tissue alterations associated with normal aging (35–37) and in a range of conditions including focal cortical dysplasia (38), stroke (39), Wilson's disease (40), multiple sclerosis (33), neurofibromatosis type 1 (38, 41), and neurodegenerative diseases. Reduction in NDI and ODI of the contralateral substantia nigra pars compacta has been shown to correlate negatively with clinical severity of Parkinson's disease (42) whereas in pre-manifest Huntington's disease reductions in NDI and ODI are seen in a range of white matter tracts with reduced NDI in the corpus callosum correlating positively with markers of severity (43). In patients with young onset Alzheimer's disease reduction in NDI and ODI is seen corrected for reduced thickness within several relevant cortical areas, with lower NDI values in patients scoring less well on cognitive tests (44), while in a rodent model NODDI indices correlate more consistently than DTI parameters with the burden of tau pathology harbored by the cortex, corpus callosum, and hippocampus (45).
Use of NODDI imaging in ALS has only recently been undertaken. Whole brain analysis in patients with manifest disease has demonstrated a significant NDI reduction throughout the intracranial CSTs up to the subcortical matter of the precentral gyri and across the corpus callosum, with increased ODI in the anterior limb of right internal capsule and increased ISO adjacent to the right lateral ventricle relative to healthy controls (46). NDI within the right corona radiata and precentral subcortical white matter was decreased to a greater extent in those patients with both limb and bulbar involvement compared to limb alone, and longer disease durations correlated with reduced ODI in the precentral gyri, dorsolateral prefrontal cortices, and precuneus. Furthermore, at the statistical threshold used, FA was reduced as expected within the CSTs but less extensively than NDI, and changes were not observed within the corpus callosum, implying NODDI may be more sensitive than DTI. Indeed, combined NODDI and DTI has also been performed in pre-manifest C9orf72 mutation carriers alongside first degree relatives not possessing the pathological repeat expansion (47). The effect size relating to detectable reductions of NDI within 7 of 11 white matter tracts, including the CSTs, is greater than that for DTI metrics (in this case increased axial diffusivity, RD, and MD rather than decreased FA) albeit statistically significant in just two. Therefore, the results appear to corroborate the implication that lowered FA (or increased diffusivity) in the CSTs and corpus callosum results from the loss of axon fibers rather than increased complexity or dispersion within tracts. Longitudinal NODDI scans have not yet been investigated although results from an ancillary imaging study to the Modifying Immune Response and Outcomes in ALS (MIROCALS) trial of low dose Interleukin-2 treatment are awaited.
In any case, neuroimaging techniques are constantly evolving with a raft of acronyms and employing different protocols aiming to reflect the true histological framework of gray and white matter. Although NODDI is considered non-inferior to other MRI modalities of high-angular resolution in this regard (48), it may be that acquisition protocols or MRI data modeling methods undertaken in NODDI, such as spherical (rather than linear) tensor encoding (49) along with tract-based (50), gray matter based (37), and gray matter surface based (51) spatial statistics are further refined in due course to overcome its own recognized limitations.
Quantitative Magnetization Transfer Imaging (qMTi)
Magnetization transfer imaging, unlike the NODDI model of diffusion MRI, essentially utilizes a “two pool” model in which hydrogen protons are either free or bound to macromolecules (lipids and proteins) within the semisolid tissue. The latter protons do not directly contribute to the MRI signal and are “silent” in diffusion sequences (increased radial diffusivity with DTI is not specific for demyelination) (52), but can be indirectly probed thanks to their interaction with the free protons following off-frequency radiofrequency pulses. The exchange in magnetization between the two compartments allows the state of the semisolid pool (saturated) to affect that of the free protons, resulting in partial saturation and in a decrease of its overall magnetization (53). The magnetization transfer (MT) effect can thereby produce a qualitative magnetization transfer tissue contrast (MTC) image and is already clinically utilized as part of MR angiography and gadolinium-enhanced T1-weighted sequences, for instance. Indeed, MTC T1 images in patients with ALS have shown hyperintensity along the CST (54, 55) and CC (54) in a proportion of cases (and more conspicuously than FLAIR) (55) compared to control subjects which was significantly related to the degree of reduced FA in the same regions and presumed to reflect damage to the white matter tracts, although with no clear association with clinical rating scales or disease duration (54). Acquiring a proton-density image with and without a MT pulse renders it possible to semi-quantify the MT effect and produce a voxel-wise magnetization transfer ratio (MTR) to reflect changes in macromolecular integrity. Accordingly, reduced MTR within the brain has been reported within the CSTs (56), the precentral and other frontal and extramotor gyri (57, 58), in patients with ALS compared to healthy controls, and independently of gray matter atrophy as measured by voxel-base morphometry (57). Significantly reduced average MTR within the spinal cord has also been reported with respect to controls (59–61), accompanied by diminished cord cross-sectional area and average FA (60), and with a longitudinal decline between sequential scans (59). More recent segmentation of the cord into gray and white matter areas, and using a particular adjusted MT protocol called inhomogeneous MT, has demonstrated localized reductions in MTR to the CSTs and dorsal columns in addition to the anterior horns at several non-contiguous cervical levels (62). However, the MTC and MTR are dependent on a range of imaging variables and their biophysical basis is undefined (53).
The development of mathematical models able to describe the MT-weighted signal as a function of the saturating pulses has enabled more biologically applicable parameters to be derived from quantitative magnetization transfer imaging (qMTi), including the macromolecular pool fraction [f; modeled to essentially represent myelin content], forward exchange rate of magnetization transfer [kf], and transverse relaxation time of the free pool [T2F]. Although qMTi is yet to be explored in patients with ALS, studies in multiple sclerosis (MS) have demonstrated reductions in f and kf, and increased T2F in acute inflammatory lesions with a subsequent return to baseline over several months (63). Compared to healthy controls, normal appearing white matter (NAWM) has reduced f, kf, and MTR (64), and reduced MTR in chronic MS plaques and has been shown to correlate with greater disability (65). Incidentally, reduced MTR in the context of MS is generally considered to be a marker of demyelination, although a small study subdivided NAWM according to distance from a T2 hyper-intense plaque and degree of MTR reduction and found that, whereas at the edge of plaques reduced MTR correlates with reduced myelin content reduced MTR in NAWM may be result from to swollen microglia and, perhaps, axons (66), thus highlighting the uncertainty of its interpretation. MTR in normal appearing gray matter is also reduced in patients with relapsing-remitting MS (67–69) and may also correlate with disability, although variable results are reported (68). Acute increases in kf (but without change in f or T2f) on qMTi have also been induced within the insula in the context of a systemic inflammatory stimulus comprising intramuscular injection of typhoid vaccination and are associated with increased levels of reported fatigue, in addition to a co-localized increase in glucose metabolism measured by FGD-PET (70). Although the mechanisms underlying changes in magnetization transfer parameters are likely to be very different between diseases, it is plausible that qMTi would be sensitive to structural alterations in ALS given the likely role for the immune system in its pathogenesis (71, 72).
Multimodal MRI
Furthermore, it may be that performing simultaneous qMTi with several other MR neuroimaging sequences, such as diffusion and (resting state) functional MRI, will be most helpful in building a better understanding how both tissue structure and function are affected by the disease process and, ultimately, the difference between certain phenotypes to guide more personalized treatments. Indeed, this is exemplified by the estimations of the myelinated fiber “g-ratio,” the axon diameter divided by the diameter of its ensheathing myelin, which is estimated to ideally be around 0.7 in the central nervous system (73). As diffusion MRI is insensitive to myelin, the combination of intraneurite and isotropic fractions from NODDI and the f value from qMTi is required to calculate the g-ratio across the brain. Following adolescence, white matter g-ratio tends to steadily increase with age inferring myelin reduction and knock on effects with respect to the velocity of neuronal conduction (74) and premature increases in the g-ratio are accordingly seen within MS plaques (75, 76). Although ALS is not primarily a demyelinating disease, new insights into the secondary effects of the neurodegenerative process may be revealed with these techniques and correlate with clinical measures.
Event-Based Modeling
Aside from interpreting the deviations of imaging parameters in terms of current tissue configuration, collecting longitudinal data is, at least conceptually, the most straightforward approach to understanding the temporal evolution of neurodegenerative pathology. However, patient tolerability for repeated MRI acquisition remains challenging in ALS, particularly, due to the rapid accumulation of symptoms and perhaps accounts for the relatively few studies conducted to date (5). Furthermore, it can be argued that participants who are included would be those harboring more slowly-progressing disease, and therefore may not be representative of the majority of patients with ALS.
Given these limitations, alternative methods such as “big data” analysis techniques and new modeling approaches have the potential to greatly increase our understanding of the mechanisms of disease progression. One such approach is the Event-Based Model (EBM) (77–79), a generative probabilistic model originally developed for use in Alzheimer's disease (AD) for which it has been validated in addition to Huntington's disease (80) and recently in ALS using oculomotor data (81). The EBM is designed to extract temporal information from cross-sectional data sets and, unlike traditional models of disease progression, does not rely on a priori staging of patients but instead extracts the event ordering directly from the data, thereby minimizing subjective bias.
The EBM defines a disease as a series of “events,” where each event is the change of a biomarker reading from a “healthy” to a “diseased” state. Crucially, biomarker cut-off points are not determined beforehand, but are derived from the data during the modeling process. This not only reduces subjective bias, but also allows for much finer temporal characterization of disease progression than is possible under existing clinically-based staging systems. Healthy control data are used as a fixed reference, and each biomarker is modeled as a mixture of two Gaussian distributions (Figure 2). In order to perform temporal modeling, the EBM assumes that the disease progression is monotonic for individual biomarkers (i.e., the severity of disease burden can only increase). Thus, for biomarkers affected early on in the course of the disease, there will be larger differences between patient and control readings, while biomarkers that are affected late on will have smaller differences between patients and controls. Markov Chain Monte Carlo (MCMC) techniques can then be used to determine the most likely event order across the entire cohort (77).
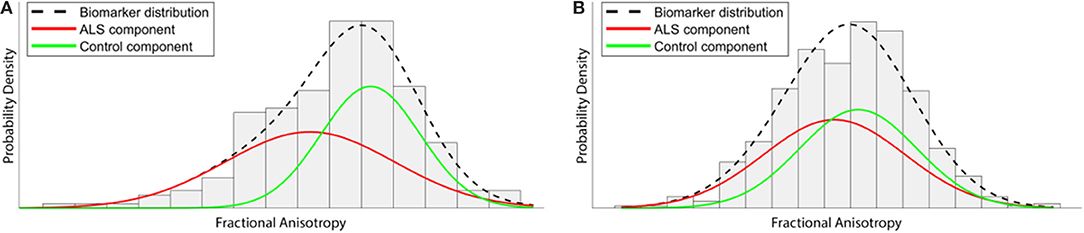
Figure 2. Illustration of how the event-based model (EBM) aims to extract temporal information from a cross-sectional data set. Gaussian distributions of fractional anisotropy (FA) biomarker readings within a tract affected early in the course of the disease, such as the corticospinal tract, would be expected to demonstrate substantial separation between ALS and Control imaging data (A). However, FA from another area affected at later stages demonstrates much less separation between distributions (B). By exploiting and characterizing these differences across all biomarkers, the EBM attempts to order the change from “normal” to “diseased” across the entire disease course.
As with any modeling approach, the EBM has strengths and weaknesses. The ability to extract fine-grained temporal information from cross-sectional data is exceptionally novel and valuable. Use of MCMC techniques also enables the model to quantify the positional variance of individual biomarkers across the cohort, thereby allowing a comparison of their relative importance and variability. In its current form, the EBM reveals aspects of disease progression that are common across the entire cohort (an “average” disease progression). The heterogeneity of ALS means that EBM analyses of stratified subgroups, based on genetic/prognostic factors, are an important future area for investigation.
The accuracy of the EBM output, as with any modeling process, will depend on the quality of the input biomarker data. As a consequence, ALS event-based modeling can require large quantities of data, particularly as individual mean cerebral CST FA values are known to have modest diagnostic power for ALS [found to have a pooled sensitivity and specificity of 0.68 and 0.73, respectively, in a meta-analysis (82)]. Current applications of the EBM to ALS data in progress include analysis of mean FA of white matter (WM) fiber bundles, modeling of patterns of cortical thinning, volumetric changes of brain structures, and oculomotor data. Future areas for development include the application of the EBM to multi-modal ALS biomarker data. Excitingly, the application of the EBM to higher order models of diffusion such as NODDI has the potential to give greater insight into ALS degeneration by simultaneously modeling the changes within ISO, NDI, and ODI parameters.
Conclusion
Ultimately, all modeling is an attempt to separate meaningful information from randomness. MRI techniques differentially model the signal to derive parameters that plausibly relate to tissue microstructure properties; these parameters can then be modeled further using the EBM to reveal patterns that exist within the data, but which still require human assessment and interpretation (as well as clinical and histological validation). Although the innovative imaging and data analysis techniques presented here constitute a selection of available methods or protocols, their use singly and in combination has the potential to transform our understanding of the biological processes and temporal evolution of ALS, which is likely to benefit further from multicenter collaboration across the entire ALS research community.
Author Contributions
AWB and MCG performed a review of the literature and drafted the paper. MC and PNL provided specialized expertise and critical appraisal of the article for submission.
Funding
MCG was funded by the MND Association (MNDA Gabel/Oct16/966-799).
Conflict of Interest Statement
The authors declare that the research was conducted in the absence of any commercial or financial relationships that could be construed as a potential conflict of interest.
References
1. Turner MR, Talbot K. Mimics and chameleons in motor neurone disease. Pract Neurol. (2013) 13:153–64. doi: 10.1136/practneurol-2013-000557
2. Blain CR, Williams VC, Johnston C, Stanton BR, Ganesalingam J, Jarosz JM, et al. A longitudinal study of diffusion tensor MRI in ALS. Amyotroph Lateral Scler. (2007) 8:348–55. doi: 10.1080/17482960701548139
3. Douaud G, Filippini N, Knight S, Talbot K, Turner MR. Integration of structural and functional magnetic resonance imaging in amyotrophic lateral sclerosis. Brain (2011) 134:3470–9. doi: 10.1093/brain/awr279
4. Huynh W, Simon NG, Grosskreutz J, Turner MR, Vucic S, Kiernan MC. Assessment of the upper motor neuron in amyotrophic lateral sclerosis. Clin Neurophysiol. (2016) 127:2643–60. doi: 10.1016/j.clinph.2016.04.025
5. Menke RA, Agosta F, Grosskreutz J, Filippi M, Turner MR. Neuroimaging endpoints in amyotrophic lateral sclerosis. Neurotherapeutics (2016) 14:11–23. doi: 10.1007/s13311-016-0484-9
6. Simon NG, Turner MR, Vucic S, Al-Chalabi A, Shefner J, Lomen-Hoerth C, et al. Quantifying disease progression in amyotrophic lateral sclerosis. Ann Neurol. (2014) 76:643–57. doi: 10.1002/ana.24273
7. Turner MR, Verstraete E. What does imaging reveal about the pathology of amyotrophic lateral sclerosis? Curr Neurol Neurosci Rep. (2015). 15:45. doi: 10.1007/s11910-015-0569-6
8. Verstraete E, Foerster BR. Neuroimaging as a new diagnostic modality in amyotrophic lateral sclerosis. Neurotherapeutics (2015) 12:403–16. doi: 10.1007/s13311-015-0347-9
9. Grolez G, Moreau C, Danel-Brunaud V, Delmaire C, Lopes R, Pradat PF, et al. The value of magnetic resonance imaging as a biomarker for amyotrophic lateral sclerosis: a systematic review. BMC Neurol. (2016) 16:155. doi: 10.1186/s12883-016-0672-6
10. Müller HP, Turner MR, Grosskreutz J, Abrahams S, Bede P, Govind V, et al. A large-scale multicentre cerebral diffusion tensor imaging study in amyotrophic lateral sclerosis. J Neurol Neurosurg Psychiatry (2016) 87:570–9. doi: 10.1136/jnnp-2015-311952
11. Sage CA, Peeters RR, Görner A, Robberecht W, Sunaert S. Quantitative diffusion tensor imaging in amyotrophic lateral sclerosis. Neuroimage (2007) 34:486–99. doi: 10.1016/j.neuroimage.2006.09.025
12. Iwata NK, Kwan JY, Danielian LE, Butman JA, Tovar-Moll F, Bayat E, et al. White matter alterations differ in primary lateral sclerosis and amyotrophic lateral sclerosis. Brain (2011) 134:2642–55. doi: 10.1093/brain/awr178
13. Iwata NK, Aoki S, Okabe S, Arai N, Terao Y, Kwak S, et al. Evaluation of corticospinal tracts in ALS with diffusion tensor MRI and brainstem stimulation. Neurology (2008) 70:528–32. doi: 10.1212/01.wnl.0000299186.72374.19
14. Stagg CJ, Knight S, Talbot K, Jenkinson M, Maudsley AA, Turner MR. Whole-brain magnetic resonance spectroscopic imaging measures are related to disability in ALS. Neurology (2013) 80:610–5. doi: 10.1212/WNL.0b013e318281ccec
15. Sarica A, Cerasa A, Valentino P, Yeatman J, Trotta M, Barone S, et al. The corticospinal tract profile in amyotrophic lateral sclerosis. Hum Brain Mapp. (2017) 38:727–39. doi: 10.1002/hbm.23412
16. Kollewe K, Körner S, Dengler R, Petri S, Mohammadi B. Magnetic resonance imaging in amyotrophic lateral sclerosis. Neurol Res Int. (2012) 2012:608501. doi: 10.1155/2012/608501
17. Agosta F, Pagani E, Rocca MA, Caputo D, Perini M, Salvi F, et al. Voxel-based morphometry study of brain volumetry and diffusivity in amyotrophic lateral sclerosis patients with mild disability. Hum Brain Mapp. (2007) 28:1430–8. doi: 10.1002/hbm.20364
18. Turner MR, Agosta F, Bede P, Govind V, Lulé D, Verstraete E. Neuroimaging in amyotrophic lateral sclerosis. Biomark Med. (2012) 6:319–37. doi: 10.2217/bmm.12.26
19. Li J, Pan P, Song W, Huang R, Chen K, Shang H. A meta-analysis of diffusion tensor imaging studies in amyotrophic lateral sclerosis. Neurobiol Aging (2012) 33:1833–8. doi: 10.1016/j.neurobiolaging.2011.04.007
20. Lindenberg R, Renga V, Zhu LL, Betzler F, Alsop D, Schlaug G. Structural integrity of corticospinal motor fibers predicts motor impairment in chronic stroke. Neurology (2010) 74:280–7. doi: 10.1212/WNL.0b013e3181ccc6d9
21. Sage CA, Van Hecke W, Peeters R, Sijbers J, Robberecht W, Parizel P, et al. Quantitative diffusion tensor imaging in amyotrophic lateral sclerosis: revisited. Hum Brain Mapp. (2009) 30:3657–75. doi: 10.1002/hbm.20794
22. Agosta F, Ferraro PM, Riva N, Spinelli EG, Chiò A, Canu E, et al. Structural brain correlates of cognitive and behavioral impairment in MND. Hum Brain Mapp. (2016) 37:1614–26. doi: 10.1002/hbm.23124
23. Senda J, Kato S, Kaga T, Ito M, Atsuta N, Nakamura T, et al. Progressive and widespread brain damage in ALS: MRI voxel-based morphometry and diffusion tensor imaging study. Amyotroph Lateral Scler. (2011) 12:59–69. doi: 10.3109/17482968.2010.517850
24. Brownell B, Oppenheimer DR, Hughes JT. The central nervous system in motor neurone disease. J Neurol Neurosurg Psychiatry (1970) 33:338–57.
25. Martin JE, Swash M. The pathology of motor neuron disease. In: Leigh PN, Swash M, editors. Motor Neuron Disease: Biology and Management. London: Springer-Verlag (1995). p. 93–118. doi: 10.1007/978-1-4471-1871-8_5
26. Smith M.C. Nerve fibre degeneration in the brain in amyotrophic lateral sclerosis. J Neurol Neurosurg Psychiatry (1960) 23:269–82. doi: 10.1136/jnnp.23.4.269
27. Budde MD, Annese J. Quantification of anisotropy and fiber orientation in human brain histological sections. Front Integr Neurosci. (2013) 7:3. doi: 10.3389/fnint.2013.00003
28. Jones DK, Knösche TR, Turner R. White matter integrity, fiber count, and other fallacies: the do's and don'ts of diffusion MRI. Neuroimage (2013) 73:239–54. doi: 10.1016/j.neuroimage.2012.06.081
29. Zhang H, Schneider T, Wheeler-Kingshott CA, Alexander DC. NODDI: practical in vivo neurite orientation dispersion and density imaging of the human brain. Neuroimage (2012) 61:1000–16. doi: 10.1016/j.neuroimage.2012.03.072
30. Caverzasi E, Papinutto N, Castellano A, Zhu AH, Scifo P, Riva M, et al. Neurite orientation dispersion and density imaging color maps to characterize brain diffusion in neurologic disorders. J Neuroimaging (2016) 26:494–8. doi: 10.1111/jon.12359
31. Chung AW, Seunarine KK, Clark CA. NODDI reproducibility and variability with magnetic field strength: a comparison between 1.5 T and 3 T. Hum Brain Mapp. (2016) 37:4550–65. doi: 10.1002/hbm.23328
32. Rae CL, Davies G, Garfinkel SN, Gabel MC, Dowell NG, Cercignani M, et al. Deficits in neurite density underlie white matter structure abnormalities in first-episode psychosis. Biol Psychiatry (2017) 82:716–25. doi: 10.1016/j.biopsych.2017.02.008
33. Grussu F, Schneider T, Tur C, Yates RL, Tachrount M, Ianuş A, et al. Neurite dispersion: a new marker of multiple sclerosis spinal cord pathology? Ann Clin Transl Neurol. (2017) 4:663–79. doi: 10.1002/acn3.445
34. Grussu F, Schneider T, Zhang H, Alexander DC, Wheeler-Kingshott CA. Neurite orientation dispersion and density imaging of the healthy cervical spinal cord in vivo. Neuroimage (2015) 111:590–601. doi: 10.1016/j.neuroimage.2015.01.045
35. Billiet T, Vandenbulcke M, Mädler B, Peeters R, Dhollander T, Zhang H, et al. Age-related microstructural differences quantified using myelin water imaging and advanced diffusion MRI. Neurobiol Aging (2015) 36:2107–21. doi: 10.1016/j.neurobiolaging.2015.02.029
36. Merluzzi AP, Dean DC, Adluru N, Suryawanshi GS, Okonkwo OC, Oh JM, et al. Age-dependent differences in brain tissue microstructure assessed with neurite orientation dispersion and density imaging. Neurobiol Aging (2016) 43:79–88. doi: 10.1016/j.neurobiolaging.2016.03.026
37. Nazeri A, Chakravarty MM, Rotenberg DJ, Rajji TK, Rathi Y, Michailovich OV, et al. Functional consequences of neurite orientation dispersion and density in humans across the adult lifespan. J Neurosci. (2015) 35:1753–62. doi: 10.1523/JNEUROSCI.3979-14.2015
38. Winston GP, Micallef C, Symms MR, Alexander DC, Duncan JS, Zhang H. Advanced diffusion imaging sequences could aid assessing patients with focal cortical dysplasia and epilepsy. Epilepsy Res. (2014) 108:336–9. doi: 10.1016/j.eplepsyres.2013.11.004
39. Adluru G, Gur Y, Anderson JS, Richards LG, Adluru N, DiBella EV. Assessment of white matter microstructure in stroke patients using NODDI. Conf Proc IEEE Eng Med Biol Soc. (2014) 2014:742–5. doi: 10.1109/EMBC.2014.6943697
40. Song YK, Li XB, Huang XL, Zhao J, Zhou XX, Wang YL, et al. A study of neurite orientation dispersion and density imaging in wilson's disease. J Magn Reson Imaging (2018) 48:423–30. doi: 10.1002/jmri.25930
41. Billiet T, Mädler B, D'Arco F, Peeters R, Deprez S, Plasschaert E, et al. Characterizing the microstructural basis of “unidentified bright objects” in neurofibromatosis type 1: A combined in vivo multicomponent T2 relaxation and multi-shell diffusion MRI analysis. Neuroimage Clin. (2014) 4:649–58. doi: 10.1016/j.nicl.2014.04.005
42. Kamagata K, Hatano T, Okuzumi A, Motoi Y, Abe O, Shimoji K, et al. Neurite orientation dispersion and density imaging in the substantia nigra in idiopathic Parkinson disease. Eur Radiol. (2016) 26:2567–77. doi: 10.1007/s00330-015-4066-8
43. Zhang J, Gregory S, Scahill RI, Durr A, Thomas DL, Lehericy S, et al. In vivo characterization of white matter pathology in pre-manifest Huntington's disease. Ann Neurol. (2018) 84:497–504. doi: 10.1002/ana.25309
44. Parker TD, Slattery CF, Zhang J, Nicholas JM, Paterson RW, Foulkes AJM, et al. Cortical microstructure in young onset Alzheimer's disease using neurite orientation dispersion and density imaging. Hum Brain Mapp. (2018) 39:3005–17. doi: 10.1002/hbm.24056
45. Colgan N, Siow B, O'Callaghan JM, Harrison IF, Wells JA, Holmes HE, et al. Application of neurite orientation dispersion and density imaging (NODDI) to a tau pathology model of Alzheimer's disease. Neuroimage (2016) 125:739–44. doi: 10.1016/j.neuroimage.2015.10.043
46. Broad RJ, Gabel MC, Dowell NG, Schwartzman DJ, Seth AK, Zhang H, et al. Neurite orientation and dispersion density imaging (NODDI) detects cortical and corticospinal tract degeneration in ALS. J Neurol Neurosurg Psychiatry (2018). doi: 10.1136/jnnp-2018-318830. [Epub ahead of print].
47. Wen J, Zhang H, Alexander DC, Durrleman S, Routier A, Rinaldi D, et al. Neurite density is reduced in the presymptomatic phase of C9orf72 disease. J Neurol Neurosurg Psychiatry (2018). doi: 10.1136/jnnp-2018-318994. [Epub ahead of print].
48. Schilling KG, Janve V, Gao Y, Stepniewska I, Landman BA, Anderson AW. Histological validation of diffusion MRI fiber orientation distributions and dispersion. Neuroimage (2018) 165:200–21. doi: 10.1016/j.neuroimage.2017.10.046
49. Lampinen B, Szczepankiewicz F, Mårtensson J, van Westen D, Sundgren PC, Nilsson M. Neurite density imaging versus imaging of microscopic anisotropy in diffusion MRI: a model comparison using spherical tensor encoding. Neuroimage (2017) 147:517–31. doi: 10.1016/j.neuroimage.2016.11.053
50. Smith SM, Jenkinson M, Johansen-Berg H, Rueckert D, Nichols TE, Mackay CE, et al. Tract-based spatial statistics: voxelwise analysis of multi-subject diffusion data. Neuroimage (2006) 31:1487–505. doi: 10.1016/j.neuroimage.2006.02.024
51. Parvathaneni P, Rogers BP, Huo Y, Schilling KG, Hainline AE, Anderson AW, et al. Gray matter surface based spatial statistics (GS-BSS) in diffusion microstructure. Med Image Comput Comput Assist Interv. (2017) 10433:638–46. doi: 10.1007/978-3-319-66182-7_73
52. Cohen-Adad J. Microstructural imaging in the spinal cord and validation strategies. Neuroimage (2018) 182:169–83. doi: 10.1016/j.neuroimage.2018.04.009
53. Cercignani M, Dowell NG, Tofts P. Quantitative MRI of the Brain : Principles of Physical Measurement. Series in Medical Physics and Biomedical Engineering. CRC Press; Taylor & Francs Group (2018).
54. Carrara G, Carapelli C, Venturi F, Ferraris MM, Lequio L, Chiò A, et al. A distinct MR imaging phenotype in amyotrophic lateral sclerosis: correlation between T1 magnetization transfer contrast hyperintensity along the corticospinal tract and diffusion tensor imaging analysis. AJNR Am J Neuroradiol. (2012) 33:733–9. doi: 10.3174/ajnr.A2855
55. da Rocha AJ, Oliveira AS, Fonseca RB, Maia AC, Buainain RP, Lederman HM. Detection of corticospinal tract compromise in amyotrophic lateral sclerosis with brain MR imaging: relevance of the T1-weighted spin-echo magnetization transfer contrast sequence. AJNR Am J Neuroradiol. (2004) 25:1509–15.
56. Tanabe JL, Vermathen M, Miller R, Gelinas D, Weiner MW, Rooney WD. Reduced MTR in the corticospinal tract and normal T2 in amyotrophic lateral sclerosis. Magn Reson Imaging (1998) 16:1163–9. doi: 10.1016/S0730-725X(98)00129-5
57. Cosottini M, Cecchi P, Piazza S, Pesaresi I, Fabbri S, Diciotti S, et al. Mapping cortical degeneration in ALS with magnetization transfer ratio and voxel-based morphometry. PLoS ONE (2013) 8:e68279. doi: 10.1371/journal.pone.0068279
58. Cosottini M, Pesaresi I, Piazza S, Diciotti S, Belmonte G, Battaglini M, et al. Magnetization transfer imaging demonstrates a distributed pattern of microstructural changes of the cerebral cortex in amyotrophic lateral sclerosis. AJNR Am J Neuroradiol. (2011) 32:704–8. doi: 10.3174/ajnr.A2356
59. El Mendili MM, Cohen-Adad J, Pelegrini-Issac M, Rossignol S, Morizot-Koutlidis R, Marchand-Pauvert V, et al. Multi-parametric spinal cord MRI as potential progression marker in amyotrophic lateral sclerosis. PLoS ONE (2014) 9:e95516. doi: 10.1371/journal.pone.0095516
60. Querin G, El Mendili MM, Bede P, Delphine S, Lenglet T, Marchand-Pauvert V, et al. Multimodal spinal cord MRI offers accurate diagnostic classification in ALS. J Neurol Neurosurg Psychiatry (2018) 89:1220–1. doi: 10.1136/jnnp-2017-317214
61. Querin G, El Mendili MM, Lenglet T, Delphine S, Marchand-Pauvert V, Benali H, et al. Spinal cord multi-parametric magnetic resonance imaging for survival prediction in amyotrophic lateral sclerosis. Eur J Neurol. (2017) 24:1040–6. doi: 10.1111/ene.13329
62. Rasoanandrianina H, Grapperon AM, Taso M, Girard OM, Duhamel G, Guye M, et al. Region-specific impairment of the cervical spinal cord (SC) in amyotrophic lateral sclerosis: a preliminary study using SC templates and quantitative MRI (diffusion tensor imaging/inhomogeneous magnetization transfer). NMR Biomed. (2017) 30:e3801. doi: 10.1002/nbm.3801
63. Levesque IR, Giacomini PS, Narayanan S, Ribeiro LT, Sled JG, Arnold DL, et al. Quantitative magnetization transfer and myelin water imaging of the evolution of acute multiple sclerosis lesions. Magn Reson Med. (2010) 63:633–40. doi: 10.1002/mrm.22244
64. Liu Z, Pardini M, Yaldizli Ö, Sethi V, Muhlert N, Wheeler-Kingshott CA, et al. Magnetization transfer ratio measures in normal-appearing white matter show periventricular gradient abnormalities in multiple sclerosis. Brain (2015) 138:1239–46. doi: 10.1093/brain/awv065
65. Amann M, Papadopoulou A, Andelova M, Magon S, Mueller-Lenke N, Naegelin Y, et al. Magnetization transfer ratio in lesions rather than normal-appearing brain relates to disability in patients with multiple sclerosis. J Neurol. (2015) 262:1909–17. doi: 10.1007/s00415-015-7793-5
66. Moll NM, Rietsch AM, Thomas S, Ransohoff AJ, Lee JC, Fox R, et al. Multiple sclerosis normal-appearing white matter: pathology-imaging correlations. Ann Neurol. (2011) 70:764–73. doi: 10.1002/ana.22521
67. Davies GR, Altmann DR, Hadjiprocopis A, Rashid W, Chard DT, Griffin CM, et al. Increasing normal-appearing grey and white matter magnetisation transfer ratio abnormality in early relapsing-remitting multiple sclerosis. J Neurol. (2005) 252:1037–44. doi: 10.1007/s00415-005-0808-x
68. Gracien RM, Jurcoane A, Wagner M, Reitz SC, Mayer C, Volz S, et al. Multimodal quantitative MRI assessment of cortical damage in relapsing-remitting multiple sclerosis. J Magn Reson Imaging (2016) 44:1600–7. doi: 10.1002/jmri.25297
69. Samson RS, Cardoso MJ, Muhlert N, Sethi V, Wheeler-Kingshott CA, Ron M, et al. Investigation of outer cortical magnetisation transfer ratio abnormalities in multiple sclerosis clinical subgroups. Mult Scler. (2014) 20:1322–30. doi: 10.1177/1352458514522537
70. Harrison NA, Cooper E, Dowell NG, Keramida G, Voon V, Critchley HD, et al. Quantitative magnetization transfer imaging as a biomarker for effects of systemic inflammation on the brain. Biol Psychiatry (2015) 78:49–57. doi: 10.1016/j.biopsych.2014.09.023
71. Schwartz M, Baruch K. Breaking peripheral immune tolerance to CNS antigens in neurodegenerative diseases: boosting autoimmunity to fight-off chronic neuroinflammation. J Autoimmun. (2014) 54:8–14. doi: 10.1016/j.jaut.2014.08.002
72. Zhao W, Beers DR, Appel SH. Immune-mediated mechanisms in the pathoprogression of amyotrophic lateral sclerosis. J Neuroimmune Pharmacol. (2013) 8:888–99. doi: 10.1007/s11481-013-9489-x
73. Chomiak T, Hu B. What is the optimal value of the g-ratio for myelinated fibers in the rat CNS? a theoretical approach. PLoS ONE (2009) 4:e7754. doi: 10.1371/journal.pone.0007754
74. Cercignani M, Giulietti G, Dowell NG, Gabel M, Broad R, Leigh PN, et al. Characterizing axonal myelination within the healthy population: a tract-by-tract mapping of effects of age and gender on the fiber g-ratio. Neurobiol Aging (2017) 49:109–18. doi: 10.1016/j.neurobiolaging.2016.09.016
75. Hagiwara A, Hori M, Yokoyama K, Nakazawa M, Ueda R, Horita M, et al. Analysis of white matter damage in patients with multiple sclerosis via a novel in vivo MR method for measuring myelin, axons, and G-Ratio. AJNR Am J Neuroradiol. (2017) 38:1934–40. doi: 10.3174/ajnr.A5312
76. Stikov N, Campbell JS, Stroh T, Lavelée M, Frey S, Novek J, et al. In vivo histology of the myelin g-ratio with magnetic resonance imaging. Neuroimage (2015) 118:397–405. doi: 10.1016/j.neuroimage.2015.05.023
77. Fonteijn HM, Clarkson MJ, Modat M, Barnes J, Lehmann M, Ourselin S, et al. An event-based disease progression model and its application to familial Alzheimer's disease. Inf Process Med Imaging (2011) 22:748–59. doi: 10.1007/978-3-642-22092-0_61
78. Oxtoby NP, Young AL, Cash DM, Benzinger TLS, Fagan AM, Morris JC, et al. Data-driven models of dominantly-inherited Alzheimer's disease progression. Brain (2018) 141:1529–44. doi: 10.1093/brain/awy050
79. Young AL, Oxtoby NP, Daga P, Cash DM, Fox NC, Ourselin S, et al. A data-driven model of biomarker changes in sporadic Alzheimer's disease. Brain (2014) 137:2564–77. doi: 10.1093/brain/awu176
80. Fonteijn HM, Modat M, Clarkson MJ, Barnes J, Lehmann M, Hobbs NZ, et al. An event-based model for disease progression and its application in familial Alzheimer's disease and Huntington's disease. Neuroimage (2012) 60:1880–9. doi: 10.1016/j.neuroimage.2012.01.062
81. Gabel MC, Schmidt, DG, Leigh, NP, Pinkhardt, EH, Ludolph, AC, Kassubek, J, et al. Event-Based Modelling Resembles the Sequential Development of Eye Movement Dysfunctions in Amyotrophic Lateral Sclerosis. Berlin: Neurowoche (2018). Available online at: https://www.dgnkongress.org/
Keywords: motor neuron disease, MRI—magnetic resonance imaging, event-based model, quantitative magnetization transfer imaging, neurite orientation dispersion and density imaging (NODDI)
Citation: Barritt AW, Gabel MC, Cercignani M and Leigh PN (2018) Emerging Magnetic Resonance Imaging Techniques and Analysis Methods in Amyotrophic Lateral Sclerosis. Front. Neurol. 9:1065. doi: 10.3389/fneur.2018.01065
Received: 29 October 2018; Accepted: 22 November 2018;
Published: 04 December 2018.
Edited by:
Peter Bede, Trinity College Dublin, IrelandReviewed by:
Efstratios Karavasilis, National and Kapodistrian University of Athens Medical School, GreeceCristina Moglia, Dipartimento di Neuroscienze Rita Levi Montalcini, Università degli Studi di Torino, Italy
Copyright © 2018 Barritt, Gabel, Cercignani and Leigh. This is an open-access article distributed under the terms of the Creative Commons Attribution License (CC BY). The use, distribution or reproduction in other forums is permitted, provided the original author(s) and the copyright owner(s) are credited and that the original publication in this journal is cited, in accordance with accepted academic practice. No use, distribution or reproduction is permitted which does not comply with these terms.
*Correspondence: Andrew W. Barritt, a.barritt@bsms.ac.uk