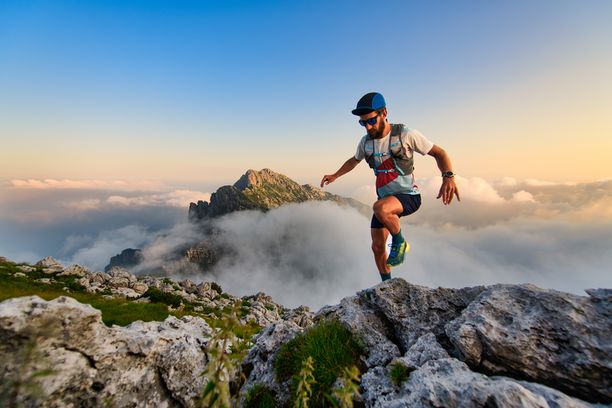
94% of researchers rate our articles as excellent or good
Learn more about the work of our research integrity team to safeguard the quality of each article we publish.
Find out more
REVIEW article
Front. Neurol., 26 November 2018
Sec. Movement Disorders
Volume 9 - 2018 | https://doi.org/10.3389/fneur.2018.00958
This article is part of the Research TopicHereditary Spastic Paraplegias: Current Knowledge and Future PerspectiveView all 6 articles
Hereditary Spastic Paraplegia is an extraordinarily heterogeneous disease caused by over 50 Mendelian genes. Recent applications of next-generation sequencing, large scale data analysis, and data sharing/matchmaking, have discovered a quickly expanding set of additional HSP genes. Since most recently discovered HSP genes are rare causes of the disease, there is a growing concern of a persisting diagnostic gap, estimated at 30–40%, and even higher for sporadic cases. This missing heritability may not be fully closed by classic Mendelian mutations in protein coding genes. Here we show strategies and published examples of broadening areas of attention for Mendelian and non-Mendelian causes of HSP. We suggest a more inclusive perspective on the potential final architecture of HSP genomics. Efforts to narrow the heritability gap will ultimately lead to more precise and comprehensive genetic diagnoses, which is the starting point for emerging, highly specific gene therapies.
Hereditary spastic paraplegias (HSPs) are a genetically heterogeneous group of neurodegenerative disorders with a prevalence of ~3-9/100,000 in most populations and a clinical hallmark of progressive lower limb weakness and spasticity (1, 2). HSPs result from genetic alterations resulting in dysfunction of the long axons in the corticospinal tract and posterior columns (3). Consistent with the cardinal clinical features of HSP, the primary pathological mechanism is distal axonal degeneration in a “dying-back” manner (4). HSPs are broadly categorized into pure and complicated forms based on the presence of additional clinical features such as ataxia, parkinsonism, peripheral neuropathy, cognitive dysfunction, cataracts, and icthyosis (3). HSPs segregate in several modes of inheritance, including autosomal dominant, autosomal recessive, X-linked, or mitochondrial (3). Although there is no evidence that HSP overall is more prevalent in one ethnic group over another, HSP does show ethnic differences in many of the mutated genes (Table 1).
Table 1. Critical review of the current SPG loci curated from the Online Mendelian Inheritance in Man (OMIM) database and a PubMed literature review of “hereditary spastic paraplegia” and each HSP gene and/or loci number.
As with many other Mendelian diseases, the introduction of next-generation sequencing (NGS) revolutionized the genetic diagnosis of HSPs with over 76 genomic loci and 58 corresponding genes (5). The autosomal dominant (AD) HSPs lead mostly to the pure form of disease and are linked to 19 spastic gait (SPG) genes. The most common AD genes are SPG4/SPAST, SPG3A/ATL1, SPG31/REEP1, and SPG10/KIF5A. Complicated HSPs more frequently occur in autosomal recessive (AR) families and are linked to 57 loci and 52 genes. The most common AR genes are SPG11/KIAA1840, SPG5A/CYP7B1, SPG7, and SPG15/ZFYVE26. Though rare, X-linked and mitochondrial inheritance are also observed. In the past 5 years alone >15 novel HSP and HSP-related genes have been reported. However, the number of families identified in the initial and follow-on papers is typically low; for example, the original REEP2 publication had 2 families with a follow-on of a single family (6, 7). Furthermore, a large number of recent SPG loci are supported by a single publication only (Table 1). This has led to the concern that we are in an asymptotic situation where, even with many new genes, the diagnostic yield may not get close to 100%. This potential gap in heritability is observed in other rare disorders as well and might be referred to as “dark matter” of clinical genomics. This review will focus on potential causes and efforts to overcome these challenges.
It is usually assumed that HSP is caused by Mendelian mechanisms and that eventually nearly all patients will receive a single-gene diagnosis. However, this is not necessarily true. Related disorders illustrate a diverse situation: inherited neuropathies (Charcot-Marie-Tooth disease, CMT) are also highly heterogeneous Mendelian disorders whereas amyotrophic lateral sclerosis (ALS) is largely not explained by Mendelian genes. The proportion of Mendelian genes is even lower in late onset neurodegenerative diseases, such as Parkinson and Alzheimer disease.
The reported diagnostic yield for exome sequencing in the general clinical setting ranges from 25 to 50% (8, 9). In hereditary spastic paraplegias, Schüle et al. identified a molecular diagnosis in 46% of families (240/519 families), despite extensive whole-exome sequencing efforts, with low success in simplex families (28%) (2). Similarly, a 20% diagnostic yield was reported in a cohort of 98 previously unsolved HSP families analyzed by a custom sequencing panel of 70 HSP and HSP-related genes (10).
While the genetics of certain neurodegenerative diseases are deemed “complex,” the field, thus far, has not applied similar models of inheritance to HSP. We believe that it is too early to tell whether non-Mendelian effects have a major contribution to HSP. There are a number of valid pro-Mendelian hypotheses as to how to close the diagnostic gap, and the coming years will allow us to test these ideas (Figure 1). These include non-coding regions of the genome, unorthodox types of mutations (such as repeat expansions) and digenic inheritance models. However, the search for risk genes and alleles will likely contribute to the understanding of HSP in multiple ways, from exploring oligogenic causation, gene/environment interactions, and phenotype modifying genes; thus, expanding the inheritance models in HSP.
Figure 1. The diagnostic heritability gap in HSP. (A) Despite unprecedented success in the identification of additional Mendelian genes, the diagnostic yield may not get close to 100% in HSP, but rather reach an asymptotic ceiling. (B) Areas that are potentially understudied in HSP thus far for cost and technical challenges. These include uncommon Mendelian causation, but also modifier and oligogenic risk alleles. The colored bars represent genes, the black lines connecting genes represent noncoding regions, and each “X” represents a mutation.
Systematic reanalysis of unresolved clinical exomes can reveal causative variants that were not prioritized in the initial analysis and increase the diagnostic yield; however, the search should ideally be expanded beyond mutations in the protein-coding regions (11). Full exploration of the non-coding space will require whole-genome sequencing; however, fortunately, the untranslated regions and regions adjacent to exons are typically covered by whole-exome sequencing. Though sparse annotation poses considerable challenges in interpreting these variants, Minnerop et al. found deep intronic mutations in POLR3A to be a frequent cause of HSP and cerebellar ataxia. After identifying intronic variants in a single recessive spastic ataxia family, the authors screened a cohort of 618 cases. They found that compound heterozygous POLR3A mutations accounted for ~3.1% of genetically unclassified autosomal recessive and sporadic index cases. Over 80% of these cases shared the same deep-intronic mutation which activates a cryptic splice site. This study nicely demonstrates the potential held within the non-coding genome.
As the limitation of non-coding coverage is overcome by whole-genome sequencing, the clinical and functional interpretation of variants will remain a challenge. Recently, the complementation of genetic sequencing with transcriptome sequencing (RNA-seq) has successfully improved the diagnostic yield in Mendelian disorders (12, 13). RNA-seq adds a functional layer to help prioritize genes and variants by providing information about aberrant splicing, abnormal gene expression levels, and extreme cases of mono-allelic expression (13). Since gene expression and mRNA isoforms vary widely across tissues, sequencing disease-relevant tissue is critical to the potential value with this approach (12). Due to the difficulties in obtaining the disease-relevant tissue, transcriptome sequencing may have limited potential in HSP. However, the recent mapping of the axonal transcriptome from induced pluripotent stem cell derived motor neurons may provide an avenue to overcoming this challenge (14).
Another contributor of genome variability that could help resolve the diagnostic gap is structural variation, including copy number variations, translocations, and inversions (15). Copy number variations (CNVs), in particular, are known to play an important role in HSPs, causing 8%-41% of SPG4 due to the Alu genomic architecture of SPAST (16–19). Screening of approximately 600 independent HSP cases over a 5-year period in ATL1, SPAST, NIPA1, SPG7, and REEP1 has detected numerous deletions (20). Recently, a complex homozygous 4-kb deletion/20-bp insertion that removes the last two exons and part of the 3′UTR was identified in DSTYK (21). These studies exemplify the relevance of structural variation in HSPs. However, major challenges still exist that hinder full exploration of these mutations. Since CNVs are also a large source of normal variation and their pathogenic potential can depend on genetic ancestry and environmental factors, determining whether a CNV is benign or pathogenic remains a considerable clinical challenge (15, 22). Additionally, CNV detection from whole-exome sequencing remains immature and unstable, as indicated by the low concordance between CNV variant callers (23). Another popular method for CNV detection is high-resolution microarray, which is commonly used in clinical cytogenetics where sequencing based analysis can overwhelm a typical laboratory's workflow (24). Though research laboratories largely rely on NGS, the additional high-resolution microarrays may lead to diagnoses in cases with suspected CNV. Improved sequencing technology and structural variation interpretation and detection will resolve more HSP families in the future.
Standard clinical genomic analysis focuses on typical modes of inheritance, such as autosomal dominant, autosomal recessive, and X-linked, while unusual inheritance modes are often ignored. Inclusion of these “genomic” mechanisms in analysis pipelines, for example genomic imprinting, repeat expansions, and uniparental disomy, can lead to successful identification of overlooked molecular diagnoses.
Uniparental isodisomy is particularly relevant to HSPs as this mechanism has been observed in two autosomal recessive spastic ataxia of Charlevoix-Saguenay (ARSACS) cases, one SPG18 case, and four SPG35 cases (25–27). Uniparental isodisomy is the inheritance of both chromosomes from the same parent, resulting from errors in meiosis and/or mitosis (28). Isodisomic events generate regions of homozygosity in the offspring, allowing for the inheritance of a homozygous variant from one heterozygous carrier-parent (29). During trios analysis, these homozygous variants are usually discarded as sequencing errors since only one parent is a carrier. The occurrence of uniparental isodisomy can be inferred from very long regions of homozygosity that are isolated to a single chromosome; therefore, uniparental isodisomy detection can be incorporated into a standard whole-exome pipeline by modifying existing methods for long regions of homozygosity (30, 31). The high number of SPG35 cases resulting from UPD suggests that this may be a frequent genetic mechanism for FA2H homozygous mutations in non-consanguineous families (27). Uniparental isodisomy is important to detect because of its impact on molecular diagnosis and recurrence risk in families.
The awareness of the genetic overlap between HSPs and spinocerebellar ataxias (SCAs) has increased as the number of loci causing both diseases expands; however, the overlap of HSP and SCA caused by triplet-repeat expansions is less emphasized (32). Bettencourt et al. reviewed the literature of triplet-repeat expansions mimicking spastic paraplegias: expansions have been observed at the ATXN1, ATXN2, ATXN3, ATXN7, ATN1, and FXN loci (32). The authors recommend incorporating triplet-repeat ataxia analysis into diagnostic algorithms, especially ATXN3 in autosomal dominant complicated HSP and FXN in autosomal recessive or sporadic spastic paraplegias (32). It is conceivable that additional, yet to be identified, coding and non-coding repeat expansion loci cause HSP.
Based on the assumption of fully penetrant alleles, traditional Mendelian disease analysis focuses on the rare DNA variation that segregates within a family. However, these locus-specific family studies treat Mendelian traits as distinct entities and disregard a more comprehensive genetic model for human disease in which variants of varying effect size as well as environmental influences contribute to disease (33). The challenge is the unexpected large amount of variation in the human genome on a population level, where >99% of all variants show a minor allele frequency of < 1% (34). Since many of these variants are without phenotypic consequence, some certainly are very harmful, and a considerable number must have effect sizes that are below the threshold of a Mendelian gene but contribute significantly to phenotypic expression. Identification of strong effect sizes in the background of mostly minor effects is the next big challenge in human genetics. Recent method developments in statistical genetics allow for unbiased genome-wide screens for non-Mendelian alleles, and surprisingly, are able to re-identify bona fide Mendelian genes as well (35). The application to HSP genomics will eventually generate a more complete genetic architecture of the disease.
Contrary to general expectations for HSP families, asymptomatic carriers are not infrequent, in which case, the genotype is said to be incompletely penetrant (36, 37). Reduced and age-dependent penetrance is a diagnostically challenging situation observed in autosomal dominant HSPs, especially SPG3A, which can lead to misinterpretation of inheritance patterns due to asymptomatic carriers and exclusion of the disease-causing allele (38–40). Additionally, sex-dependent penetrance is suspected in SPAST and ATL1 based on the excess of affected males (41). Incomplete penetrance can also manifest in autosomal recessive disorders when the primary mutation leads to varying phenotypic effects depending on the secondary mutation (36). For example, novel compound heterozygous mutations in SPG11 let to an atypical late onset and mild form of SPG11 (42).
To distinguish reduced-penetrance alleles, which have caused HSP in at least some individuals under a Mendelian trait, risk alleles have been defined as variants with smaller effect sizes that are part of a multifactorial model of disease causation (36). However, since the possibility of risk alleles is only recently recognized in rare Mendelian disease, the line between penetrance and risk is often blurred. In this context, risk alleles more broadly refer to rare variants that may lead to a less severe, later-onset form of disease or contribute to an individual's susceptibility to disease, likely through an oligogenic model. For example, heterozygous mutations in SPG7 were identified as a potential susceptibility factor for late-onset neurodegenerative disorder (43). Similarly, heterozygous mutations in MME were recently shown to predispose carriers to late-onset axonal neuropathy (44). In MME, the comparison of the “rare variant load” of missense and loss of function changes in late-onset CMT to the general population showed a significant enrichment of such variation (44).
Systematic identification of rare variant associations are usually limited by low statistical power unless sample sizes or variant effect sizes are very large (45). To illustrate, >60,000 cases (and an equal number of controls) would be necessary to detect a disease association for a rare variant (0.1% frequency) with an odds ratio of 2.0 for a disease with a 5% population prevalence (45). Fortunately, powerful study designs can alleviate the sample size requirement to more reasonable numbers (46). One approach that can be explored in HSPs is the gene-based variant burden test which collapses the number of minor alleles into one genetic score (gene), thus reducing multiple testing and increasing power (45, 47). One successful example of this approach was the identification of a new ALS gene, TBK1, in 2,869 sporadic ALS patients (35). Remarkably, other known ALS genes showed strong associations, indicating that additional variation in known familial ALS genes also contribute to sporadic ALS forms (35). The rare variant association studies are particularly useful for identifying risk genes and novel gene associations.
An increasing number of exceptions to the fundamental “one gene, one phenotype” paradigm are being published across Mendelian phenotypes (48). The oversimplified view that phenotypic expression, even for classically monogenic disorders, is driven exclusively by mutations at a single locus is being replaced by the concept of genetic modification (49). Though several types of genetic modification are possible, the simple definition is the effect of one allele on the phenotypic outcome of a second allele (49). If the primary allele is sufficient to cause disease, then the secondary allele is a “modifier” that modulates phenotypic expression, such as disease severity or progression.
Given the high clinical variability observed across HSP patients, genetic modification of the primary allele was anticipated. Over a decade ago, intragenic polymorphisms were suggested to modify the age at onset of SPAST mutations (50, 51). More recently, SPAST deletions spanning the adjacent DPY30 gene were shown to have significantly reduced age at onset (52). Furthermore, in a study of a large Cuban spinocerebellar ataxia type 2 (SCA2) cohort, 33% of the residual age at onset variance was attributed to genetic modifiers (53). Examples of genetic modifiers from related Mendelian disorders exist in the literature; for instance, a polymorphism in miR-149 was recently associated with onset age and severity in Charcot-Marie-Tooth disease type 1A (CMT1A) (54).
Another study design that increases the statistical power for association testing of rare variants is the extreme phenotype sampling (EPS) approach (46). Based on the assumption that rare causal variants are more likely found in the extremes of a quantitative trait such as age of onset or severity of a symptom, EPS can increase the power to detect rare variants over random sampling (46). For example, Emond et al. utilized an extreme phenotype sampling approach to identify an association between rare coding variants in DCTN4 and time to first Pseudomonas infection (measure of cystic fibrosis severity) (47, 55). Additionally, Tao et al. identified SIPA1L2 as a genetic modifier of muscle strength impairment in CMT1A. In vitro knock down of SIPA1L2 in Schwannoma cells lead to a significant reduction in PMP22 expression, offering a potential pathway for therapeutic strategies (56). Application of EPS to an HSP cohort may also reveal modifier alleles that contribute to disease.
Digenic or oligogenic inheritance refers to instances when one primary allele is insufficient to cause disease, instead requiring the combined consequence of multiple alleles (49). Evidence of oligogenic inheritance has emerged in other neurological disorders. In both sporadic and familial amyotrophic lateral sclerosis (ALS) cases, patients harboring two or more rare variants had lower survival or earlier age at onset, suggesting that the combined effect of rare variants affects ALS development and progression (57–59). Similarly, over 30% of Parkinson's disease (PD) patients carried additional rare variants in Mendelian PD genes and had younger ages at onset (60). An increased rare variant burden was also observed in two cohorts of inherited neuropathy cases, which was followed up in vivo zebrafish experiments (61). In zebrafish, more severe phenotypic outcomes were observed as a consequence of increased mutational burden in neuropathy genes, consistent with a positive genetic interaction mechanism of oligogenic inheritance (61).
Demonstrating oligogenic inheritance from family studies is challenging without experimental models. However, one trending approach to assessing oligogenic inheritance—which has been explored in Parkinson, ALS, Frontotemporal Dementia, Congenital Hypothyroidism, Inherited Neuropathy, and more—is to evaluate the mutational burden across known disease genes through Fisher's exact test or logistic regression (60–64). However, caution should be used with this approach as Koegh et al. warns that systematic bias can lead to the apparent enrichment of “oligogenic” variants in familial cases and controlling such bias is essential for investigating an oligogenic role in neurodegenerative diseases (65).
Though the high amount of locus heterogeneity present in HSPs complicates clinical diagnosis, it does provide an opportunity to study the overarching biological pathways through analysis of molecular networks. Genes and their products form complex networks within cells that are governed by specific laws and principles (66). These complex networks model the non-linear genotype-phenotype relationships observed in HSPs, such as incomplete penetrance and epistasis, that deviate from the “one gene, one phenotype” principle (66, 67). Functionally related proteins interact with each other to accomplish similar biological mechanisms, thus forming cellular pathways (66). Network medicine capitalizes on these interactions and hypothesizes that perturbation of a single gene product will propagate along the entire network (68). The interactions between a set of disease-causing genes can be summarized into a disease module for further study (69). A disease module can be analyzed for differences in complete loss of gene products vs. interaction-specific perturbations, global relationships to other human diseases, novel candidate disease genes, and emerging biological pathways (68, 70–73). Novarino et al. combined exome sequencing with network analysis to summarize a global view of HSP (74). A HSPome was created from previously published HSP seed genes and candidate genes from whole exome sequencing. From the HSPome, they extracted subnetworks of functionally related proteins that form pathological modules, including ER-associated degradation, endosomal and membrane-trafficking, and purine nucleotide metabolism. Three candidate genes arose from the HSPome that were found to be mutated in HSP patients. Lastly, the authors discovered the HSP seed genes were significantly overlapping with amyotrophic lateral sclerosis, Alzheimer's disease, and Parkinson's disease, while no overlap was observed between HSP and neurodevelopmental disorders nor non-neurological disorders (74). Recently, the relationship between the inherited axonopathies, HSP and Charcot-Marie-Tooth 2 (CMT2), was explored through a network analysis of protein-protein interactions. The HSP disease module was found to significantly overlap both the CMT2 and hereditary ataxia modules. Pathway analysis revealed ribosomal protein and viral infection response pathways (75). With the rapid pace of gene discovery in HSPs, network analysis will continue to be a powerful approach for deciphering the complex interactions underlying the phenotype.
Historically, many movement disorders, including HSPs, have been clinicogenetically classified based on the predominant phenotype of the first gene locus (76). These classification systems have similar shortcomings, including erroneously assigned loci, duplicated loci, missing loci, and unconfirmed loci (76, 77). Furthermore, these classification systems suggest that HSPs are a distinct and isolated disorder, when in fact HSPs exist on a spectrum between inherited ataxias and axonal Charcot-Marie-Tooth disease (CMT2) (76, 78). Not only do these disorders share clinical symptoms, such as prominent lower extremity spasticity, but they can also be caused by mutations within the same genes (76, 78, 79). Next-generation sequencing greatly facilitated the appreciation of these genetic overlaps by providing an unbiased approach that broke through the prior clinical and diagnostic preconceptions (76). Phenotypic expansions continue to blur the lines drawn in neurological disorders; for example, HSP was recently associated to PLA2G6, the causative gene underlying heterogeneous PLA2G6-associated neurodegeneration (PLAN) (80). Additionally, KIF5A, known to cause both HSP and CMT2, contains a C-terminal hotspot of mutations that can cause a classical amyotrophic lateral sclerosis phenotype (81). Furthermore, mutations in ATP13A2, originally linked to a rare form of juvenile-onset atypical Parkinson disease (Kufor-Rakeb syndrome), are now also associated with neurodegeneration with brain iron accumulation, neuronal ceroid lipofuscinosis, and most recently, complicated HSP (82–84). Awareness and consideration of phenotypic expansions will be essential for both individual genetic diagnoses as well as revealing common pathways underlying neurodegeneration (80). As the phenotypic spectrum broadens across the neurologic community, these historical classifications are being reconsidered (78). To address this issue, Synofzik and Schüle have proposed a mechanism based classification system for the ataxia-spasticity spectrum, based on unbiased modular phenotyping, that captures nuanced phenotypic expression, opens ataxia and spasticity to a multisystem neuronal dysfunction, and help to prioritize research on shared pathways (76).
The above-mentioned approaches increasingly require larger datasets which contradicts, of course, the low prevalence of rare disease. This requirement exceeds what single labs have traditionally been able to collect from local clinics. It will be insufficient to exchange candidate gene information or candidate alleles. To apply statistical approaches, one needs to gather hundred if not thousands of HSP samples and adequate controls. This led to the notion of raw genetic data aggregation as the next frontier for HSP gene discovery. The most prominent example of systematic data aggregate in HSP is the GENESIS Project database (tgp-foundation.org). Over 600 HSP, 500 ataxia, and 890 CMT exomes or genomes have been aggregated from several dozen laboratories in 22 different countries(85). We have begun to complement Mendelian gene discovery efforts with modifier gene studies and rare variant burden analyses. It is still early, but it appears that significant results can be achieved beginning with 200+ exomes and sufficient control samples. For example, an exome-wide association study with 202 cases and 6,905 controls successfully found a signal in GREB1L (joint p-value = 2.3 × 10−7) in renal agenesis and hypodysplasia (86). Another exome-wide association study in CMT, with 343 cases and 935 controls, identified a significant association in EXOC4 (p-value = 6.9 × 10−6, OR = 2.1) and nominal associations with other known CMT genes (87).
The current period is a remarkable time for HSP research. Studies from many countries are reporting a steady pace of novel Mendelian genes, complementing existing multigene clinical panels. The diagnostic yield has never been higher; albeit it is hindered by an increasing burden of Variants of Uncertain Significance. In addition, gene therapy approaches are maturing in related disorders, and it appears only a matter of time before they are applied to specific HSP genes. These novel therapeutic approaches include gene replacement, antisense oligonucleotides (ASO), and soon gene editing. Most of them require a specific genetic diagnosis. This emphasizes the need to fill the diagnostic gap currently estimated at 30-40%, but larger for sporadic HSP cases.
Contemplating the details of recently discovered HSP genes suggests that traditional Mendelian mutations may not be able to account for the majority of yet to be diagnosed patients. We have outlined the various possibilities of non-protein coding Mendelian variation but also an increasing interest in multigene causation or phenotypic modification. It appears to soon to tell how the genetic architecture of HSP will look like in 10 years' time. However, we are certain that non-Mendelian elements will play a role, if only as secondary protective/worsening factors. Still, cohort approaches now enabled through extensive collaborations and data aggregations will likely also hold surprises for non-traditional disease causation in HSP.
All authors listed have made a substantial, direct and intellectual contribution to the work, and approved it for publication.
The authors declare that the research was conducted in the absence of any commercial or financial relationships that could be construed as a potential conflict of interest.
This work was supported by grants from NIH (R01NS072248 to SZ and F31NS106859 to DB-B).
1. Blackstone, C Cellular pathways of hereditary spastic paraplegia. Annu Rev Neurosci. (2012) 35:25–47. doi: 10.1146/annurev-neuro-062111-150400
2. Schule, R, Wiethoff, S, Martus, P, Karle, KN, Otto, S, Klebe, S, et al. Hereditary spastic paraplegia: clinicogenetic lessons from 608 patients. Ann Neurol. (2016) 79:646–58. doi: 10.1002/ana.24611
3. Faber, I, Pereira, ER, Martinez, ARM, França, M Jr, and Teive, HAG Hereditary spastic paraplegia from 1880 to 2017: an historical review. Arquivos de Neuro-Psiquiatria (2017) 75:813–8. doi: 10.1590/0004-282x20170160
4. DeLuca, GC, Ebers, GC, and Esiri, MM The extent of axonal loss in the long tracts in hereditary spastic paraplegia. Neuropathol Appl Neurobiol. (2004) 30:576–84. doi: 10.1111/j.1365-2990.2004.00587.x
5. Parodi, L, Fenu, S, Stevanin, G, and Durr, A Hereditary spastic paraplegia: more than an upper motor neuron disease. Rev Neurol (Paris) (2017) 173:352–60. doi: 10.1016/j.neurol.2017.03.034
6. Esteves, T, Durr, A, Mundwiller, E, Loureiro, JL, Boutry, M, Gonzalez, MA, et al. Loss of association of REEP2 with membranes leads to hereditary spastic paraplegia. Am J Hum Genet. (2014) 94:268–77. doi: 10.1016/j.ajhg.2013.12.005
7. Roda, RH, Schindler, AB, and Blackstone, C De novo REEP2 missense mutation in pure hereditary spastic paraplegia. Ann Clin Transl Neurol. (2017) 4:347–50. doi: 10.1002/acn3.404
8. Eldomery, MK, Coban-Akdemir, Z, Harel, T, Rosenfeld, JA, Gambin, T, Stray-Pedersen, A, et al. Lessons learned from additional research analyses of unsolved clinical exome cases. Genome Med. (2017) 9:26. doi: 10.1186/s13073-017-0412-6
9. Chong, JX, Buckingham, KJ, Jhangiani, SN, Boehm, C, Sobreira, N, Smith, JD, et al. The Genetic basis of Mendelian phenotypes: discoveries, challenges, and opportunities. Am J Hum Genet. (2015) 97:199–215. doi: 10.1016/j.ajhg.2015.06.009
10. Morais, S, Raymond, L, Mairey, M, Coutinho, P, Brandão, E, Ribeiro, P, et al. Massive sequencing of 70 genes reveals a myriad of missing genes or mechanisms to be uncovered in hereditary spastic paraplegias. Eur J Hum Genet. (2017) 25:1217–28. doi: 10.1038/ejhg.2017.124
11. Wenger, AM, Guturu, H, Bernstein, JA, and Bejerano, G Systematic reanalysis of clinical exome data yields additional diagnoses: Implications for providers. Genet Med (2017) 19:209–14. doi: 10.1038/gim.2016.88
12. Cummings, BB, Marshall, JL, Tukiainen, T, Lek, M, Donkervoort, S, Foley, AR, et al. Improving genetic diagnosis in Mendelian disease with transcriptome sequencing. Sci Transl Med. (2017) 9:eaal5209. doi: 10.1126/scitranslmed.aal5209
13. Kremer, LS, Bader, DM, Mertes, C, Kopajtich, R, Pichler, G, Iuso, A, et al. Genetic diagnosis of Mendelian disorders via RNA sequencing. Nat Commun. (2017) 8:15824. doi: 10.1038/ncomms15824
14. Maciel, R, Bis, D, Rebelo, AP, Saghira, C, Zuchner, S, and Saporta, MA The human motor neuron axonal transcriptome is enriched for transcripts related to mitochondrial function and microtubule-based axonal transport. Exp Neurol. (2018) 307:155–63. doi: 10.1016/j.expneurol.2018.06.008
15. Zarrei, M, MacDonald, JR, Merico, D, and Scherer, SW A copy number variation map of the human genome. Nat Rev Genet. (2015) 16:172–183. doi: 10.1038/nrg3871
16. Depienne, C, Fedirko, E, Forlani, S, Cazeneuve, C, Ribaï, P, Feki, I, et al. Exon deletions of SPG4 are a frequent cause of hereditary spastic paraplegia. J Med Genet. (2007) 44:281–4. doi: 10.1136/jmg.2006.046425
17. Vandebona, H, Kerr, NP, Liang, C, and Sue, CM SPAST mutations in Australian patients with hereditary spastic paraplegia. Intern Med J. (2012) 42:1342–7. doi: 10.1111/j.1445-5994.2012.02941.x
18. Beetz, C, Nygren, AOH, Schickel, J, Auer-Grumbach, M, Bürk, K, Heide, G, et al. High frequency of partial SPAST deletions in autosomal dominant hereditary spastic paraplegia. Neurology (2006) 67:1926–30. doi: 10.1212/01.wnl.0000244413.49258.f5
19. Boone, PM, Yuan, B, Campbell, IM, Scull, JC, Withers, MA, Baggett, BC, et al. The Alu-rich genomic architecture of SPAST predisposes to diverse and functionally distinct disease-associated CNV alleles. Am J Hum Genet. (2014) 95:143–61. doi: 10.1016/j.ajhg.2014.06.014
20. Varga, R-E, Mumtaz, R, Jahic, A, Rudenskaya, GE, Sánchez-Ferrero, E, Auer-Grumbach, M, et al. MLPA-based evidence for sequence gain: pitfalls in confirmation and necessity for exclusion of false positives. Anal Biochem. (2012) 421:799–801. doi: 10.1016/j.ab.2011.12.002
21. Lee, JYW, Hsu, C-K, Michael, M, Nanda, A, Liu, L, McMillan, JR, et al. Large intragenic deletion in DSTYK underlies autosomal-recessive complicated spastic paraparesis, SPG23. Am J Hum Genet. (2017) 100:364–70. doi: 10.1016/j.ajhg.2017.01.014
22. Nowakowska, B Clinical interpretation of copy number variants in the human genome. J Appl Genet. (2017) 58:449–57. doi: 10.1007/s13353-017-0407-4
23. Yao, R, Zhang, C, Yu, T, Li, N, Hu, X, Wang, X, et al. Evaluation of three read-depth based CNV detection tools using whole-exome sequencing data. Mol Cytogenet. (2017) 10:30. doi: 10.1186/s13039-017-0333-5
24. Haraksingh, RR, Abyzov, A, and Urban, AE Comprehensive performance comparison of high-resolution array platforms for genome-wide Copy Number Variation (CNV) analysis in humans. BMC Genomics (2017) 18:321. doi: 10.1186/s12864-017-3658-x
25. Anesi, L, de, Gemmis P, Pandolfo, M, and Hladnik, U Two novel homozygous SACS mutations in unrelated patients including the first reported case of paternal UPD as an etiologic cause of ARSACS. J Mol Neurosci. (2011) 43:346–9. doi: 10.1007/s12031-010-9448-4
26. Srivastava, S, Cohen, JS, Vernon, H, Baranano, K, McClellan, R, Jamal, L, et al. Clinical whole exome sequencing in child neurology practice. Ann Neurol. (2014) 76:473–83. doi: 10.1002/ana.24251
27. Soehn, AS, Rattay, TW, Beck-Woedl, S, Schaeferhoff, K, Monk, D, Doebler-Neumann, M, et al. Uniparental disomy of chromosome 16 unmasks recessive mutations of FA2H/SPG35 in 4 families. Neurology (2016) 87:186–91. doi: 10.1212/WNL.0000000000002843
28. Eggermann, T, Soellner, L, Buiting, K, and Kotzot, D Mosaicism and uniparental disomy in prenatal diagnosis. Trends Mol Med. (2015) 21:77–87. doi: 10.1016/j.molmed.2014.11.010
29. Papenhausen, P, Schwartz, S, Risheg, H, Keitges, E, Gadi, I, Burnside, RD, et al. UPD detection using homozygosity profiling with a SNP genotyping microarray. Am J Med Genet A (2011) 155A:757–68. doi: 10.1002/ajmg.a.33939
30. Magi, A, Tattini, L, Palombo, F, Benelli, M, Gialluisi, A, Giusti, B, et al. H3M2: detection of runs of homozygosity from whole-exome sequencing data. Bioinformatics (2014) 30:2852–9. doi: 10.1093/bioinformatics/btu401
31. Bis, DM, Sch, le R, Reichbauer, J, Synofzik, M, Rattay, TW, Soehn, A, et al. Uniparental disomy determined by whole-exome sequencing in a spectrum of rare motoneuron diseases and ataxias. Mol Genet Genomic Med. (2017) 5:280–6. doi: 10.1002/mgg3.285
32. Bettencourt, C, Quintáns, B, Ros, R, Ampuero, I, Yáñez, Z, Pascual, SI, et al. Revisiting genotype-phenotype overlap in neurogenetics: triplet-repeat expansions mimicking spastic paraplegias. Hum Mutat. (2012) 33:1315–23. doi: 10.1002/humu.22148
33. Lupski, JR, Belmont, JW, Boerwinkle, E, and Gibbs, RA Clan genomics and the complex architecture of human disease. Cell (2011) 147:32–43. doi: 10.1016/j.cell.2011.09.008
34. Lek, M, Karczewski, KJ, Minikel, EV, Samocha, KE, Banks, E, Fennell, T, et al. Analysis of protein-coding genetic variation in 60,706 humans. Nature (2016) 536:285–91. doi: 10.1038/nature19057
35. Cirulli, ET, Lasseigne, BN, Petrovski, S, Sapp, PC, Dion, PA, Leblond, CS, et al. Exome sequencing in amyotrophic lateral sclerosis identifies risk genes and pathways. Science (2015) 347:1436–41. doi: 10.1126/science.aaa3650
36. Cooper, DN, Krawczak, M, Polychronakos, C, Tyler-Smith, C, and Kehrer-Sawatzki, H Where genotype is not predictive of phenotype: towards an understanding of the molecular basis of reduced penetrance in human inherited disease. Hum Genet. (2013) 132:1077–130. doi: 10.1007/s00439-013-1331-2
37. Zlotogora, J Penetrance and expressivity in the molecular age. Genet Med. (2003) 5:347–52. doi: 10.1097/01.GIM.0000086478.87623.69
38. Khan, TN, Klar, J, Tariq, M, Anjum, Baig S, Malik, NA, Yousaf, R, et al. Evidence for autosomal recessive inheritance in SPG3A caused by homozygosity for a novel ATL1 missense mutation. Eur J Hum Genet. (2014) 22:1180–4. doi: 10.1038/ejhg.2014.5
39. Klebe, S, Stevanin, G, and Depienne, C Clinical and genetic heterogeneity in hereditary spastic paraplegias: from SPG1 to SPG72 and still counting. Rev Neurol (Paris) (2015) 171:505–30. doi: 10.1016/j.neurol.2015.02.017
40. Varga, R-E, Schule, R, Fadel, H, Valenzuela, I, Speziani, F, Gonzalez, M, et al. Do not trust the pedigree: reduced and sex-dependent penetrance at a novel mutation hotspot in ATL1Blurs autosomal dominant inheritance of spastic paraplegia. Hum Mutat. (2013) 34:860–3. doi: 10.1002/humu.22309
41. Tesson, C, Koht, J, and Stevanin, G Delving into the complexity of hereditary spastic paraplegias: how unexpected phenotypes and inheritance modes are revolutionizing their nosology. Hum Genet. (2015) 134:511–38. doi: 10.1007/s00439-015-1536-7
42. Giannoccaro, MP, Liguori, R, Arnoldi, A, Donadio, V, Avoni, P, and Bassi, MT Atypical late-onset hereditary spastic paraplegia with thin corpus callosum due to novel compound heterozygous mutations in the SPG11 gene. J Neurol. (2014) 261:1825–7. doi: 10.1007/s00415-014-7443-3
43. Klebe, S, Depienne, C, Gerber, S, Challe, G, Anheim, M, Charles, P, et al. Spastic paraplegia gene 7 in patients with spasticity and/or optic neuropathy. Brain (2012) 135:2980–3. doi: 10.1093/brain/aws240
44. Auer-Grumbach, M, Toegel, S, Schabhüttl, M, Weinmann, D, Chiari, C, Bennett, DLH, et al. Rare variants in MME, encoding metalloprotease neprilysin, are linked to late-onset autosomal-dominant axonal polyneuropathies. Am J Hum Genet. (2016) 99:607–23. doi: 10.1016/j.ajhg.2016.07.008
45. Kiryluk, K challenges in rare variant association studies for complex kidney traits: CFHR5 and IgA nephropathy. J Am Soc Nephrol. (2016) 27:2547–51. doi: 10.1681/ASN.2016040383
46. Barnett, IJ, Lee, S, and Lin, X Detecting rare variant effects using extreme phenotype sampling in sequencing association studies. Genet Epidemiol. (2013) 37:142–51. doi: 10.1002/gepi.21699
47. Auer, PL Rare variant association studies: considerations, challenges and opportunities. (2015) 7:16. doi: 10.1186/s13073-015-0138-2
48. Katsanis, N The continuum of causality in human genetic disorders. Genome Biol. (2016) 17:233. doi: 10.1186/s13059-016-1107-9
49. Kousi, M, and Katsanis, N Genetic modifiers and oligogenic inheritance. Cold Spring Harbor Perspect Med. (2015) 5:a017145. doi: 10.1101/cshperspect.a017145
50. Svenson, IK, Kloos, MT, Gaskell, PC, Nance, MA, Garbern, JY, Hisanaga, S-I, et al. Intragenic modifiers of hereditary spastic paraplegia due to spastin gene mutations. Neurogenetics (2004) 5:157–64. doi: 10.1007/s10048-004-0186-z
51. Erichsen, AK, Inderhaug, E, Mattingsdal, M, Eiklid, K, and Tallaksen, CME Seven novel mutations and four exon deletions in a collection of Norwegian patients with SPG4 hereditary spastic paraplegia. Eur J Neurol. (2007) 14:809–14. doi: 10.1111/j.1468-1331.2007.01861.x
52. Newton, T, Allison, R, Edgar, JR, Lumb, JH, Rodger, CE, Manna, PT, and et, l Mechanistic basis of an epistatic interaction reducing age at onset in hereditary spastic paraplegia. Brain (2018) 141:1286–99. doi: 10.1093/brain/awy034
53. Figueroa, KP, Coon, H, Santos, N, Velazquez, L, Mederos, LA, and Pulst, SM Genetic analysis of age at onset variation in spinocerebellar ataxia type 2. (2017) 3:e155. doi: 10.1212/NXG.0000000000000155
54. Nam, SH, Kanwal, S, Nam, DE, Lee, MH, Kang, TH, Jung, S-C, et al. Association of miR-149 polymorphism with onset age and severity in Charcot-Marie-Tooth disease type 1A. Neuromuscul Disord (2018) 28:502–7. doi: 10.1016/j.nmd.2018.04.002
55. Emond, MJ, Louie, T, Emerson, J, Zhao, W, Mathias, RA, Knowles, MR, et al. Exome sequencing of extreme phenotypes identifies DCTN4 as a modifier of chronic Pseudomonas aeruginosa infection in cystic fibrosis. Nat Genet. (2012) 44:886–9. doi: 10.1038/ng.2344
56. Tao, F, Beecham, G, Rebelo, AP, Blanton, SH, Abreu, L, Baas, F, et al. Genome-Wide Association Study Identifies Potential Genetic Modifiers in Charcot-Marie-Tooth Disease Type 1A. Orlando, FL: Presented at the Annual Meeting of The American Society of Human Genetics. (2017).
57. Pang, SY-Y, Hsu, JS, Teo, K-C, Li, Y, Kung, MHW, Cheah, KSE, et al. Burden of rare variants in ALS genes influences survival in familial and sporadic ALS. Neurobiol Aging (2017) 58:238.e9–e15. doi: 10.1016/j.neurobiolaging.2017.06.007
58. Cady, J, Allred, P, Bali, T, Pestronk, A, Goate, A, Miller, TM, et al. Amyotrophic lateral sclerosis onset is influenced by the burden of rare variants in known amyotrophic lateral sclerosis genes. Ann Neurol. (2014) 77:100–13. doi: 10.1002/ana.24306
59. Pang, SY-Y, Teo, K-C, Hsu, JS, Chang, RS-K, Li, M, Sham, PC, et al. The role of gene variants in the pathogenesis of neurodegenerative disorders as revealed by next generation sequencing studies: a review. Transl Neurodegener. (2017) 6:27. doi: 10.1186/s40035-017-0098-0
60. Lubbe, SJ, Escott-Price, V, Gibbs, JR, Nalls, MA, Bras, J, Price, TR, et al. Additional rare variant analysis in Parkinson's disease cases with and without known pathogenic mutations: evidence for oligogenic inheritance. Hum Mol Genet. (2016) 25:5483–9. doi: 10.1093/hmg/ddw348
61. Gonzaga-Jauregui, C, Harel, T, Gambin, T, Kousi, M, Griffin, LB, Francescatto, L, et al. Exome sequence analysis suggests that genetic burden contributes to phenotypic variability and complex neuropathy. CellRep. (2015) 12:1169–83. doi: 10.1016/j.celrep.2015.07.023
62. Dols-Icardo, O, García-Redondo, A, Rojas-García, R, Borrego-Hernández, D, Illán-Gala, I, Muñoz-Blanco, JL, et al. Analysis of known amyotrophic lateral sclerosis and frontotemporal dementia genes reveals a substantial genetic burden in patients manifesting both diseases not carrying the C9orf72 expansion mutation. J Neurol Neurosurg Psychiatr. (2018) 89:162–8. doi: 10.1136/jnnp-2017-316820
63. Ferrari, R, Wang, Y, Vandrovcova, J, Guelfi, S, Witeolar, A, Karch, CM, et al. Genetic architecture of sporadic frontotemporal dementia and overlap with Alzheimer“s and Parkinson”s diseases. J Neurol Neurosurg Psychiatr. (2017) 88:152–64. doi: 10.1136/jnnp-2016-314411
64. de, Filippis T, Gelmini, G, Paraboschi, E, Vigone, MC, Di, Frenna M, Marelli, F, et al. A frequent oligogenic involvement in congenital hypothyroidism. Hum Mol Genet. (2017) 26:2507–14. doi: 10.1093/hmg/ddx145
65. Keogh, MJ, Wei, W, Aryaman, J, Wilson, I, Talbot, K, Turner, MR, et al. Oligogenic genetic variation of neurodegenerative disease genes in 980 postmortem human brains. J Neurol Neurosurg Psychiatr. (2018) 89:813–6. doi: 10.1136/jnnp-2017-317234
66. Vidal, M, Cusick, ME, and Barabási, A-L Interactome networks and human disease. Cell (2011) 144:986–98. doi: 10.1016/j.cell.2011.02.016
67. Beadle, GW, and Tatum, EL Genetic control of biochemical reactions in neurospora. Proc Natl Acad Sci U.S.A. (1941) 27:499–506.
68. Barabási, A-L, Gulbahce, N, and Loscalzo, J Network medicine: a network-based approach to human disease. Nat Rev Genet. (2011) 12:56–68. doi: 10.1038/nrg2918
69. Menche, J, Sharma, A, Kitsak, M, Ghiassian, SD, Vidal, M, Loscalzo, J, et al. Uncovering disease-disease relationships through the incomplete interactome. Science (2015) 347:1257601. doi: 10.1126/science.1257601
70. Limviphuvadh, V, Tanaka, S, Goto, S, Ueda, K, and Kanehisa, M The commonality of protein interaction networks determined in neurodegenerative disorders (NDDs). Bioinformatics (2007) 23:2129–38. doi: 10.1093/bioinformatics/btm307
71. Ghiassian, SD, Menche, J, and Barabási, A-L A DIseAse MOdule Detection (DIAMOnD) algorithm derived from a systematic analysis of connectivity patterns of disease proteins in the human interactome. PLoS Comput Biol. (2015) 11:e1004120. doi: 10.1371/journal.pcbi.1004120
72. Sharma, A, Menche, J, Huang, CC, Ort, T, Zhou, X, Kitsak, M, et al. A disease module in the interactome explains disease heterogeneity, drug response and captures novel pathways and genes in asthma. Hum Mol Genet. (2015) 24:3005–20. doi: 10.1093/hmg/ddv001
73. Zhong, Q, Simonis, N, Li, Q-R, Charloteaux, B, Heuze, F, Klitgord, N, et al. Edgetic perturbation models of human inherited disorders. Mol Syst Biol. (2009) 5:1–10. doi: 10.1038/msb.2009.80
74. Novarino, G, Fenstermaker, AG, Zaki, MS, Hofree, M, Silhavy, JL, Heiberg, AD, et al. Exome sequencing links corticospinal motor neuron disease to common neurodegenerative disorders. Science (2014) 343:506–11. doi: 10.1126/science.1247363
75. Bis, DM, Wuchty, S, Danzi, M, and Zuchner, S A Network Biology Approach to Unraveling Inherited Axonopathies. Scientific Reports. (in press).
76. Synofzik, M, and Schule, R Overcoming the divide between ataxias and spastic paraplegias: Shared phenotypes, genes, and pathways. Mov Disord. (2017) 32:332–45. doi: 10.1002/mds.26944
77. Marras, C, Lang, A, van, de Warrenburg BP, Sue, CM, Tabrizi, SJ, Bertram, L, et al. Nomenclature of genetic movement disorders: recommendations of the International Parkinson and Movement Disorder Society task force. Mov Disord. (2017) 32:724–25. doi: 10.1002/mds.27045
78. Fridman, V, and Murphy, SM The spectrum of axonopathies: from CMT2 to HSP. Neurology (2014) 83:580–1. doi: 10.1212/WNL.0000000000000700
79. Blackstone, C Converging cellular themes for the hereditary spastic paraplegias. Curr Opin Neurobiol. (2018) 51:139–46. doi: 10.1016/j.conb.2018.04.025
80. Ozes, B, Karagoz, N, Schüle, R, Rebelo, A, Sobrido, MJ, Harmuth, F, et al. PLA2G6 mutations associated with a continuous clinical spectrum from neuroaxonal dystrophy to hereditary spastic paraplegia. Clin Genet. (2017) 92:534–9. doi: 10.1111/cge.13008
81. Brenner, D, Yilmaz, R, Mueller, K, Grehl, T, Petri, S, Meyer, T, et al. Hot-spot KIF5A mutations cause familial ALS. Brain (2018) 141:688–97. doi: 10.1093/brain/awx370
82. Estrada-Cuzcano, A, Martin, S, Chamova, T, Synofzik, M, Timmann, D, Holemans, T, et al. Loss-of-function mutations in the ATP13A2/PARK9 gene cause complicated hereditary spastic paraplegia (SPG78). Brain (2017) 140:287–305. doi: 10.1093/brain/aww307
83. Kara, E, Tucci, A, Manzoni, C, Lynch, DS, Elpidorou, M, Bettencourt, C, et al. Genetic and phenotypic characterization of complex hereditary spastic paraplegia. Brain (2016) 139:1904–18. doi: 10.1093/brain/aww111
84. van, de Warrenburg BP, Schouten, MI, De, Bot ST, Vermeer, S, Meijer, R, Pennings, M, et al. Clinical exome sequencing for cerebellar ataxia and spastic paraplegia uncovers novel gene-disease associations and unanticipated rare disorders. Eur J Hum Genet. (2016) 24:1460–6. doi: 10.1038/ejhg.2016.42
85. Gonzalez, M, Falk, MJ, Gai, X, Postrel, R, Schuele, R, and Zuchner, S Innovative genomic collaboration using the GENESIS (GEM.app) platform. Hum Mutat. (2015) 36:950–6. doi: 10.1002/humu.22836
Keywords: heredit spastic paraplegia, genomics, risk allele, Mendelian, non-Mendelian inheritance
Citation: Bis-Brewer DM and Züchner S (2018) Perspectives on the Genomics of HSP Beyond Mendelian Inheritance. Front. Neurol. 9:958. doi: 10.3389/fneur.2018.00958
Received: 05 August 2018; Accepted: 25 October 2018;
Published: 26 November 2018.
Edited by:
Toshitaka Kawarai, Tokushima University, JapanCopyright © 2018 Bis-Brewer and Züchner. This is an open-access article distributed under the terms of the Creative Commons Attribution License (CC BY). The use, distribution or reproduction in other forums is permitted, provided the original author(s) and the copyright owner(s) are credited and that the original publication in this journal is cited, in accordance with accepted academic practice. No use, distribution or reproduction is permitted which does not comply with these terms.
*Correspondence: Stephan Züchner, c3p1Y2huZXJAbWVkLm1pYW1pLmVkdQ==
Disclaimer: All claims expressed in this article are solely those of the authors and do not necessarily represent those of their affiliated organizations, or those of the publisher, the editors and the reviewers. Any product that may be evaluated in this article or claim that may be made by its manufacturer is not guaranteed or endorsed by the publisher.
Research integrity at Frontiers
Learn more about the work of our research integrity team to safeguard the quality of each article we publish.