- 1Traumatic Brain Injury Research, Kessler Foundation, East Hanover, NJ, United States
- 2Department of Physical Medicine and Rehabilitation, Rutgers New Jersey Medical School, Newark, NJ, United States
- 3Neuropsychology and Neuroscience Research, Kessler Foundation, East Hanover, NJ, United States
- 4Department of Anatomy & Neurosciences, University of Amsterdam, Amsterdam, Netherlands
Outcome anticipation is not only a mental preparation for upcoming consequences, but also an essential component of learning and decision-making. Thus, anticipation of consequences is a key process in everyday functioning. The striatum and the ventromedial prefrontal cortex are among the key regions that have been shown to be involved in outcome anticipation. However, while structural abnormalities of these regions as well as altered decision-making have been noted in individuals with multiple sclerosis (MS), neural correlates of outcome anticipation have not been explored in this population. Thus, we examined the neural correlates of outcome anticipation in MS by analyzing brain activation in individuals with MS while they performed a modified version of a card-guessing task. Seventeen MS and 13 healthy controls performed the task while functional magnetic resonance imaging (fMRI) was obtained. To achieve maximal anticipatory response and prevent the possibility of differential performance on the task, participants were presented with monetary rewards only on 50% of the trials. While replicating previous evidence of structural abnormalities of the striatum in MS, our results further showed that individuals with MS exhibited greater activation in the putamen, right hippocampus, and posterior cingulate cortex during outcome anticipation compared to healthy controls. Furthermore, even though there was no strategy that participants could learn in order to predict outcomes, 76% of participants with MS indicated that they used strategies while performing the task. We thus propose that the increased neural activation observed in MS during outcome anticipation might be explained by a failure in recognizing the lack of regularity in the task structure that could result in using strategies to perform the task.
Introduction
Multiple sclerosis (MS) is a neuro-inflammatory disease characterized by the demyelination and neurodegeneration of the central nervous system (1, 2). Such neuronal damage can result in symptoms commonly seen in MS, such as difficulties in vision and ambulation, numbness, dizziness, and changes in affect and cognition. Indeed, a wide range of cognitive changes can occur following MS, including changes in attention, memory, and sensory information processing. Specifically, the process of learning, which gives the ability to acquire new information and modulate behavior accordingly, has been reported to be impaired in MS (3, 4).
Learning entails building action-outcome associations. The initial anticipation of what will happen in the future is an important component of action-outcome associations, since it indicates that an organism is aware of the link between an action and an outcome, and is prepared to process the outcome caused by the action. Thus, impairments in such learning can lead to altered outcome anticipation, which in turn can interfere with adaptive decision-making 5, 6. Altered decision making has been previously reported in individuals with MS (7–10). Specifically, several studies found that individuals with MS not only made poorer decisions while trying to maximize rewards, but were also less sensitive to the risks associated with their decisions. Such poor decision-making suggests the possibility that outcome anticipation in MS might be impaired. However, the process of outcome anticipation in MS has yet to be fully examined.
The striatum, the primary nucleus of the basal ganglia (BG) which can be subdivided into the caudate nucleus and putamen, has been linked to learning, decision-making, and outcome anticipation (11–13). The striatum is topographically connected with the prefrontal cortex (PFC), with specific regions of the PFC being engaged in outcome anticipation and decision-making, such as the ventromedial prefrontal cortex (vmPFC). While it has been shown that individuals with MS exhibit structural abnormalities in these regions (14, 15), the neural correlates of outcome anticipation in MS have not been examined.
Thus, the current study examined the neural correlates involved in outcome anticipation in individuals with MS. We expected that individuals with MS will show altered activation during outcome anticipation compared to healthy controls. We also assessed motivational tendencies in our participants because of the close relationship between outcome anticipation and motivation. In addition, we controlled for depressive symptomatology, as depression frequently occurs in MS (16), and has been shown to interfere with outcome anticipation processes (17, 18).
Methods
Participants
Magnetic resonance imaging (MRI) and functional MRI (fMRI) data of 17 individuals with MS and 13 healthy controls (HCs) from a previous study (19) were used for analysis (AgeMeanMS = 44.81 [SD = 8.82] AgeMeanHC = 37.85 [SD = 12.58]). MS participants had no other neurological or psychiatric disorders besides MS; HC participants had no neurological or psychiatric disorders. All participants were female in order to more accurately study the population most frequently affected with MS. Participants had no history of substance or drug abuse, no MRI contraindications, and were all right handed. To avoid the effect of aging, only participants under the age of 65 were recruited. In the MS group, 16 participants were diagnosed with relapsing-remitting, and one with secondary progressive MS. The mean disease duration for the MS group was 14.53 years (SD = 7.76). Participants with MS were at least 4 weeks post most recent exacerbation and use of steroids, benzodiazepines, or neuroleptics. Furthermore, due to the high prevalence of depression in MS populations, the MS and HC groups were screened for depressive symptomatology by the Chicago Multiscale Depression Inventory (CMDI). The two groups showed no differences in CMDI scores. Demographic information and CMDI scores are shown in Table 1.
The study was approved by the Institutional Review Board of Kessler Foundation and is in accordance with the ethical standards laid down in the 1964 Declaration of Helsinki and its later amendments. All participants signed an informed consent and received a compensation of $50 as well as a $5 bonus from the card-guessing task.
Behavioral Paradigm
We examined brain activity in individuals with MS while they performed a modified version of the card-guessing task (20) with two types of conditions: the reward and control (no reward) conditions. During the reward condition, participants were told that they can win a monetary bonus if they correctly guess a number on a card. Participants were presented with monetary rewards only on 50% of the trials. This was done in order to achieve maximal unpredictability of rewarding outcomes necessary to motivate gambling and elicit anticipatory response in the brain (21, 22) as well as to eliminate the confound of differential task performance on brain activity. Specifically, during the reward condition of the task, a card with a question mark was presented on the screen (Figure 1). Participants were told that the number on the card could range from one to nine (but would never be five), and to make a guess of whether the number on each card was higher or lower than five. Participants made their guess by pressing either the left or right button on a response box placed under their right hand within 3 s. After participants responded, a blank screen with a fixation point was presented for a randomly determined period of time between 1 and 5 s (i.e., anticipation phase). After the anticipation phase, participants were presented with a green checkmark for correct guesses and a red “X” for incorrect guesses, both accompanied with the presentation of the correct number. Participants earned $1.00 reward for each correct guess and lost $0.50 for each incorrect guess. Unknown to the participants, the outcome on each card was predetermined after they made the guess, so that participants' guesses were correct on half of the trials and incorrect on the other half of the trials. Therefore, participants could not learn to predict the value of the cards and would simply be waiting to find out about the result during the anticipation phase. The procedure of this task was the same in the control condition with one exception. Instead of guessing the values on the cards, participants were presented with the values of the cards. They then used the response box to indicate whether the value on the card was higher or lower than five, a condition in which the participant only confirmed the card's value. There was no monetary rewards or losses after each trial in the control condition. The order of the runs and trials within each run was randomized across participants using E-Prime software (23).
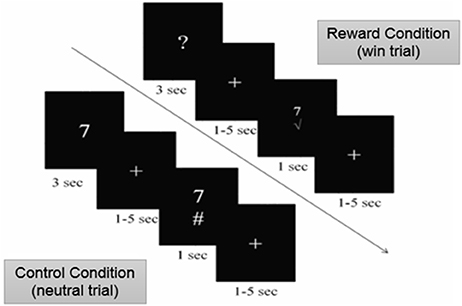
Figure 1. Card-Guessing Task paradigm shown to participants within MRI. During the reward condition, participants were asked to guess if a card's value was more or < 5 when prompted with a question mark. If guessed correctly, participants received a green check mark, indicating the win of $1.00 (shown above). If guessed incorrectly, participants received a red X mark, indicating a loss of $0.50. During the control condition, participants were shown numbers and indicated whether or not the number was higher or lower than five (shown above).
Every participant performed two runs of the reward condition and two runs of the control condition. Each run consisted of 60 trials. Every participant received a fixed bonus of $5 at the end of the study.
After the scan, participants filled out questionnaires to measure motivational tendencies and depression. First, we used the Behavioral Inhibition System and Behavioral Activation System scales (BIS/BAS) (24, 25), which consists of 24 statements, measuring both appetitive motivation (i.e. reward responsiveness, fun seeking and drive; BAS scale) and aversive motivation (BIS scale). The Chicago Multiscale Depression Inventory (26) was then administered to gain an overall score of depressive symptomatology in order to control for differences in depression between groups.
Data Acquisition
All MRI data was acquired on a Siemens Skyra 3.0T scanner equipped with a standard 20-channel radio-frequency head coil. Thirty-two slices were obtained in an oblique orientation of 30° to the anterior commissure-posterior commissure line to prevent signal drop-out from the prefrontal cortex (27).
High-resolution structural images were collected using a standard T1-weighted pulse sequence (TR = 2,100 ms, TE = 3.43 ms; 32 slices; 1 × 1 × 1 mm voxels). Using these images, functional activation could be localized. Functional data for four runs, consisting of 172 volumes each, was collected by using a standard T2*-weighted echo planar sequence (TR = 2,000 ms; TE = 30 ms; 3 × 3 × 3 mm voxels; flip angle = 90°; FoV = 256 mm; slice gap = 1 mm) sensitive to the blood oxygen level dependent (BOLD) contrast.
Data Analysis
fMRI Data
FMRIB Software Library (FSL; www.fmrib.ox.ac.uk/fsl) v5.0 was used to analyze data (28). The FMRI Expert Analysis Tool (FEAT) v6.00 was used for general linear model-based fMRI analysis. Data passed through a high pass filter of 90 s to keep out low frequency noise. The brain extraction tool was used to skull strip raw BOLD images (29). The effect of head motion was controlled by MCFLIRT by estimating mean displacement (30). Motion higher than 1.0 mm in rotation or translation was reason for exclusion. Images were spatially smoothed with a 6 mm full-width at half-maximum (FWHM) kernel to reduce noise without reducing valid activation. The boundary-based algorithm was used for registration (31). This requires both whole head and brain extracted input images. High resolution structural standard MNI space registration was performed using FLIRT (30, 32) and further refined with FNIRT nonlinear registration (12° of freedom and a 10 mm wrap resolution) (33, 34).
A first-level analysis was performed on each task run and included two regressors from the reward and control condition corresponding to the anticipation phase with the duration between 1 and 5 s. Time-series statistical analysis was carried out using FILM with local autocorrelation correction (35). Convolution was set to a double-gamma hemodynamic response function. Six motion parameters were included as regressors of no interest in addition to the derivatives of the original motion parameters and the squares of the derivatives, amounting to 18 regressors. Additional confound EVs were added to remove residual effects of motion that are still left in the data. For this we used the FSL tool called fsl_motion_outliers. This tool identifies outlier volumes or spikes in functional data and censors them based on certain criteria. For the current analysis, volumes were censored if framewise displacement was >0.9 (36). Runs were excluded from the analysis when more than 35 volumes were censored.
A second-level analysis was performed within subjects to combine the different runs using a fixed-effects model, by forcing the random effects variance to zero in FLAME (FMRIB's Local analysis of mixed effects) (37–39). These data were used for the group analysis to compare activity between MS and HC subjects ([MS anticipation – HC anticipation] and [MS control – HC control]). A whole-brain, voxel-wise independent samples t-test was performed using FLAME1 and 2 (38, 39). To correct for multiple comparisons, Z statistical images were thresholded using a cluster threshold of z > 2.3 and a corrected cluster significance threshold of p < 0.05 (40).
Structural MRI Data
All T1-weighted images used for structural analyses for the MS group were first lesion-filled using FSL's lesion_filling protocol (41).
Segmentation of the hippocampus, caudate, putamen and nucleus accumbens was accomplished using FIRST (FSL v3.2.0), which is based on a Bayesian framework that allows for the relationships between the subcortical structures' sizes and shapes to be investigated. Linear registration using FLIRT (30–32) on T1-weighted images was performed in a two stage manner in which the images were first aligned on the MNI152 template with a 1 mm resolution using 12° of freedom, and then a subcortical mask was created in which excluded any unwanted regions outside of the subcortical structures being segmented. Once the mask was complete, all segmentation outputs were quality checked. Volumetric analyses were then performed on each structure bilaterally by using fslstats with the appropriate structure intensity. Volume was gained in mm3. SIENAX (40, 42), which is part of FSL (28), was then used to obtain a volumetric scaling factor per participant in order to normalize all brain tissue volume for differences in head size. SIENAX also performed tissue type segmentation, including estimates of white matter. Independent samples t-tests were then conducted between the region volumes of both groups.
Grey Matter (GM) volume using T1-weighted images was analyzed using FSL-VBM (43), an optimized VBM protocol (44) carried out with FSL tools (28). Structural images were registered to the MNI 152 standard space using non-linear registration (34) after brain-extraction and GM segmentation was completed. These images were then used to create a study-specific GM template which all native GM images were non-linearly registered to. All images were modulated to correct for local expansion/contraction and then smoothed with an isotropic Gaussian kernel with a sigma of 3 mm. Lastly, voxelwise general linear modeling was applied using permutation-based non-parametric testing. All tests were corrected for multiple comparisons across space.
Questionnaire Data
Independent samples t-tests were performed on questionnaire data to see if there were differences between groups on the BIS/BAS scales. Pearson's correlations between questionnaire data and both subcortical volumes and averaged beta weights of the anticipation phase were then done to examine the relationships between motivational tendencies and both structure volume and activation between groups. For the MS group specifically, we controlled for disease duration by performing partial correlations.
Previous studies have shown that even with the use of tasks with non-contingent outcome presentation, individuals still often try to form strategies in order to feel more in control (45, 46). Thus, at the end of the experiment, we also asked participants about whether they used any strategies during task performance. Specifically, participants were asked the following question: “What strategies did you use while performing the task?” The questionnaire was coded as (1) if participants indicated using a strategy during the card task and [0] if participants indicated not using a strategy. The percentage of participants utilizing strategies was calculated for each group.
Results
Whole Brain Analysis
In order to examine the neural mechanisms associated with outcome anticipation in individuals with MS, we performed an independent samples t-test, comparing activation during the anticipation periods of the reward and control conditions. In the reward condition, activations in the vmPFC was not different between MS and HC participants. However, we observed greater activation in the MS group than in the HC group in the right hippocampus, putamen and posterior cingulate cortex (PCC; Figure 2), among other regions (Table 2A; all p-values were corrected for cluster significance at p < 0.05 threshold). This finding replicates previous studies of clinical populations that showed increased hippocampal activation during outcome anticipation (43, 47). In the control condition, only areas of the occipital lobe and the right postcentral gyrus showed increased activation during outcome anticipation (Table 2B).
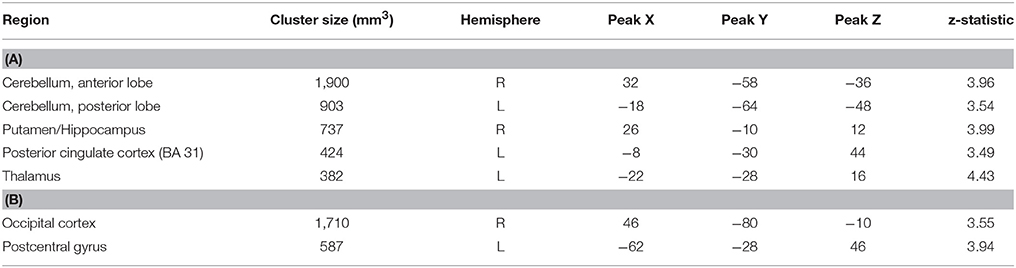
Table 2. (A) Brain regions showing increased activation in the MS group compared to the HC group during the anticipation period of the reward condition in MS vs. HC. (B) Brain regions showing increased activation in the MS group compared to the HC group during the anticipation period of the control condition.
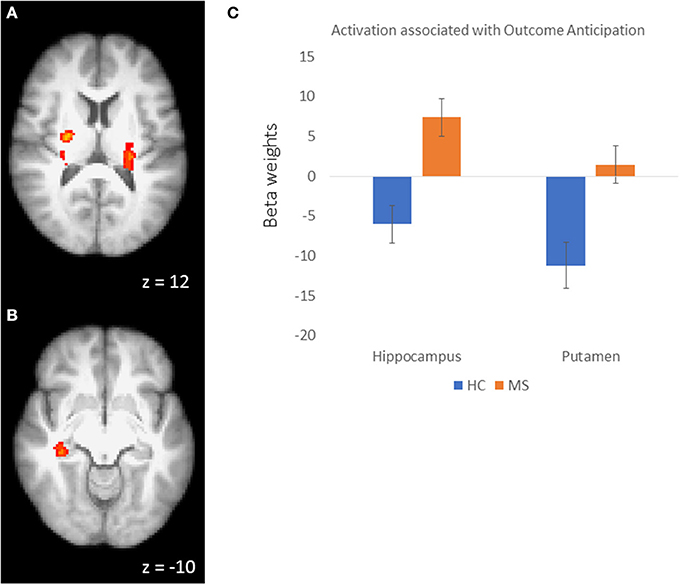
Figure 2. (A) Activation clusters in the putamen and thalamus during outcome anticipation in MS vs HC. (B) Activation cluster in the hippocampus during outcome anticipation in MS vs. HC. (C) For illustrative purposes, beta weights from the hippocampus and putamen clusters showing sensitivity during outcome anticipation in MS vs. HC.
Volumetric Analysis
A volumetric analysis of subcortical structures (deep GM) revealed significant bilateral differences of the hippocampus (right: p = 0.006; left: p = 0.017), caudate nucleus (right: p = 0.011; left: p = 0.004), and putamen (right: p = 0.017; left: p = 0.015) between groups, with the MS group having less volume than HCs on average. Additionally, the MS group showed significantly less volume in the left nucleus accumbens (p = 0.028) compared to HCs (see Table 3).
The VBM analysis showed no significant differences in cortical GM volume between groups. Significant white matter volume differences were observed (p = 0.006), with the MS group showing less white matter volume compared to HCs on average. All reported results were corrected for multiple comparisons.
Brain-Behavior Relationships
We examined the relationship between brain parameters and participants' motivation levels. Specifically, we focused on areas in which MS participants showed greater activity than HCs during outcome anticipation: the putamen, hippocampus, and PCC. To start, independent samples t-tests revealed that MS participants and HCs showed no differences on either BIS (p = 0.71) or BAS (p = 0.29) questionnaires, indicating no differences in motivation levels. However, while controlling for disease duration, partial correlations revealed negative associations between BIS scores and activation of the putamen (rpartial = −0.633, p = 0.011), hippocampus (rpartial = −0.639, p = 0.010) and PCC (rpartial = −0.600, p = 0.018) in the MS group, but not with HCs. These results suggest that less aversive motivation is associated with greater activity in the putamen, hippocampus, and PCC during outcome anticipation in MS.
For the HC group, Pearson's correlations revealed a positive association between caudate volume and BAS scores (r = 0.700, p = 0.008), indicating that those who had more volume in their caudate nuclei also showed higher appetitive motivation.
Strategy Use During Task Performance
At the end of the experiment, we asked participants to indicate whether they used any strategies to predict the number on the face of the card. While outcome presentation during the task was random, some participants reported the use of strategies. Responses included statements such as “I tried to come up with a pattern,” “I tried to think of a pattern of numbers,” or “I was thinking about the pattern the examples showed.” Based on participants' self-reports about the use of strategies, we calculated the percentage of participants reporting the use of strategies during task performance. The results showed that 76% of participants in the MS group indicated that they used strategies during the card-guessing game in order to try to predict the outcome. Only 46% of participants in the HC group indicated that they used strategies during the task.
Discussion
In the current study, we examined the neural mechanisms associated with outcome anticipation in individuals with MS. We observed that, compared to HCs, individuals with MS had reduced volumes of the striatal sub-regions and increased activation during outcome anticipation in the putamen. While we did not observe differences in the vmPFC activation, we observed greater activation in the right hippocampus and PCC during outcome anticipation in MS participants compared to their healthy counterparts. In addition, MS participants also exhibited reduced hippocampal volume compared to HCs.
Putamen
In the current study, the outcomes of participants' guesses in the card-guessing task were predetermined to be 50% gains and 50% losses. That is, there were no patterns that participants could have learned to predict outcomes. However, 76% of the MS participants reported the employment of strategies while guessing during the reward condition to increase their gains, while only 46% of the healthy participants reported doing so. These results might suggest that MS participants are less aware of the lack of regularity between their guesses and the outcomes compared to healthy participants. In the current study, we found increased activation in the putamen during outcome anticipation in individuals with MS compared to HCs. Previous research has demonstrated that the putamen is involved in learning and outcome anticipation (48, 49). Thus, one potential explanation for the observed increased activation of the putamen in the MS, compared to HC, group might be that participants with MS were trying to use strategies and identify patterns in task structure, while HC participants were able to recognize a lack of regularity in the task structure. Failure to recognize a lack of task regularity might have resulted in stronger outcome anticipation, and thus greater activation in the putamen, as we observed in MS participants. From this perspective, the increased activation in the putamen might indicate impairment in learning about the reward structure, which manifested as redundant effort during outcome anticipation.
Another potential explanation for increased putamen activation is a lack of inhibition or impulsivity in the MS group. Previous studies have shown that individuals with MS show neural compensation during response inhibition by exhibiting higher activation in some brain regions including the putamen (50). Further, as previously discussed, individuals with MS have poor decision making processes and show less sensitivity to risky decisions (7–10). This could be a sign of impulsive or habitual behavior in MS. That is, increased putamen activation could also be associated with impulsivity, which is the tendency to select choices without forethought (51–53), or dominant habitual response, which is the proclivity to choose actions with no regard for their consequences (54). Interestingly, response inhibition, impulsive behavior and habitual responding share overlapping neural circuits that might be difficult to disentangle (55). Therefore, putamen activation in our MS sample could be a manifestation of these behaviors or due to patients' preparation for response (by using strategies to perform the cognitive task). More research is needed in order to tease apart these potential explanations of putamen involvement during decision-making and outcome anticipation in MS.
Hippocampus and PCC
Structural and functional alterations of the hippocampus are well-documented in MS (56–58). Related to these previous findings, we observed differences in hippocampal activation during outcome anticipation between MS and healthy individuals. We discuss two possible explanations that may underlie the increased hippocampal activity in our MS group. First, the hippocampus may have been more active during outcome anticipation in order to compensate for the impaired activity in the striatum seen in MS. The hippocampus is a plausible candidate to cover for the striatum, as suggested by animal studies showing that the hippocampus and the striatum receive the same input information from dopaminergic midbrain areas (59). At the same time, it has been suggested that the hippocampus is involved in feedback learning and works in parallel with the frontal and striatal regions (60). Recent neuroimaging studies showed that hippocampal activation during outcome anticipation facilitates hippocampal coupling with the vmPFC (61). Furthermore, one study demonstrated that enhanced hippocampal activity compensates for the dysfunction of the caudate nucleus in patients with Huntington's disease (62).
Further, the hippocampus has been shown to play an important role in learning of regularities (63). As in the case of the putamen discussed above, the increased hippocampal activation might suggest that MS participants were actively engaging in determining regularities in task structure. As previously mentioned, participants with MS indicated trying to figure out a pattern to predict outcomes and maximize gains while performing the reward condition. Failing to learn the lack of regularity in the reward structure of the current task, MS participants might have tried harder to determine whether there were regularities, as individuals often do during gambling [e.g., gambler's fallacy and illusion of control 46, 64).
Third, together with the hippocampus, we also observed greater activation of the PCC in our MS group. The hippocampus and the PCC are part of the default mode network (DMN). This network has been shown to be deactivated during task performance, while activated during self-referential processing (65, 66). Reward processing during the card task can fall under the umbrella of self-referential processing as subjects have a goal to win monetary rewards for themselves. Thus, the current results might suggest that individuals with MS do not deactivate the DMN regions as do HCs during task performance. Such interpretation will be consistent with previous investigations of DMN activation in MS (67).
Motivational Tendencies
With the caudate nucleus being involved in anticipation and outcome processing (68), it comes as no surprise that we found a positive association between caudate volume and appetitive motivation in HCs. As for the MS group, a negative relationship was found between aversive motivation and the activation of the putamen, hippocampus, and PCC. That is, the more avoidant tendencies an individual has, the lower the activation of brain regions involved in outcome processing and learning of regularities, which goes hand in hand with the MS group being more strategic than the HCs. Furthermore, these observed relationships can be further interpreted as being due to impulsivity. Specifically, for the MS group, higher activation in the putamen might indicate a higher reflexive response, which means participants with MS may have responded to cards impulsively without motivation for the outcome in mind. With the HC group, however, more considerate responses should be made with motivation included in the thought process before selecting an action. While we did not observe functional differences in the caudate between groups, volumetric differences support this potential interpretation between appetitive motivation and caudate volume in the HC group. Future studies should utilize a paradigm which looks more closely at motivational aspects in order to more directly assess group differences in functional activation and individual differences in motivation.
Limitations and Future Directions
Because our study is one of the first reports of structural and functional substrates related to outcome anticipation in MS, future studies should attempt to replicate our findings. This is particularly important since limited sample size and a homogenous gender profile of the sample (females only) are obvious limitations of the current study. While MS is more common in females [20–30% males 69), future studies should attempt to recruit a more representative sample.
Further, data collection for the current study did not entail any neuropsychological evaluations or assessment of the disability status (e.g., EDSS scores). Thus, future investigations of processes related to outcome anticipation should include the examination of the cognitive and disability profiles of MS patients to better characterize the sample. In addition, while we did not observe significant differences between groups in age, the HCs were somewhat older. Thus, future studies should better match the groups on age. Despite these limitations, the current study paves the way for further investigation of motivational tendencies and outcome anticipation and processing in individuals with MS as well as functional and structural correlates of these processes.
Conclusion
The current study is the first to investigate functional architecture associated with outcome anticipation in individuals with MS. We observed (1) increased activation of several brain regions during outcome anticipation in MS compared to HCs, and (2) participants with MS not being able to identify the lack of regularity during the task and attempting to use strategies. While the present results require replication, they also add to the growing body of evidence suggesting structural and functional alterations of brain regions associated with anticipation and outcome processing in individuals with MS.
Author Contributions
ED conceived of the presented idea. AS, P-PL, and ED performed computations. AS, P-PL, JYN, EN, and ED contributed to final manuscript.
Conflict of Interest Statement
The authors declare that the research was conducted in the absence of any commercial or financial relationships that could be construed as a potential conflict of interest.
The reviewer PV and handling Editor declared their shared affiliation.
Funding
This work was partially supported by The National Multiple Sclerosis Society (Grant # RG-1501-02630), awarded to Ekaterina Dobryakova, Ph.D.
References
1. Tomassini V, Matthews PM, Thompson AJ, Fuglø D, Geurts JJ, Johansen-Berg H, et al. Neuroplasticity and functional recovery in multiple sclerosis. Nat Rev Neurol. (2012) 8:635–46. doi: 10.1038/nrneurol.2012.179
2. Haider L, Zrzavy T, Hametner S, Hö R, Bagnato F, Grabner GN, et al. The topograpy of demyelination and neurodegeneration in the multiple sclerosis brain. Brain (2016) 139:807–18. doi: 10.1093/brain/awv398
3. Chiaravalloti ND, Deluca J. Cognitive impairment in multiple sclerosis. Lancet Neurol. (2008) 7:1139–51. doi: 10.1016/S1474-4422(08)70259-X
4. DeLuca J, Gaudino EA, Diamond BJ, Christodoulou C, Engel RA. Acquisition and storage deficits in multiple sclerosis. J Clin Exp Neuropsychol. (1998) 20:376–90. doi: 10.1076/jcen.20.3.376.819
5. Balodis IM, Potenza MN. Anticipatory reward processing in addicted populations: a focus on the monetary incentive delay task. Biol Psychiatry. (2015) 77:434–44. doi: 10.1016/j.biopsych.2014.08.020
6. Rizvi SJ, Pizzagalli DA, Sproule BA, Kennedy SH. Assessing anhedonia in depression: potentials and pitfalls. Neurosci Biobehav Rev. (2016) 65:21–35. doi: 10.1016/j.neubiorev.2016.03.004
7. Nagy H, Bencsik K, Rajda C, Benedek K, Beniczky S, Kéri S, et al. The effects of reward and punishment contingencies on decision-making in multiple sclerosis. J Int Neuropsychol Soc. (2006) 12:559–65. doi: 10.1017/S1355617706060644
8. Farez MF, Crivelli L, Leiguarda R, Correale J. Decision-making impairment in patients with multiple sclerosis: a case-control study. BMJ Open. (2014) 4:e004918–e004918. doi: 10.1136/bmjopen-2014-004918
9. Simioni S, Ruffieux C, Kleeberg J, Bruggimann L, Pasquier RADU, Annoni J-M, et al. Progressive decline of decision-making performances during multiple sclerosis. J Int Neuropsychol Soc. (2009) 15:291–5. doi: 10.1017/S1355617709090262
10. Kleeberg J, Bruggimann L, Annoni J-M, van Melle G, Bogousslavsky J, Schluep M. Altered decision-making in multiple sclerosis: a sign of impaired emotional reactivity? Ann Neurol. (2004) 56:787–95. doi: 10.1002/ana.20277
11. Haber SN, Knutson B. The reward circuit: linking primate anatomy and human imaging. Neuropsychopharmacology (2010) 35:4–26. doi: 10.1038/npp.2009.129
12. Seger CA. How do the basal ganglia contribute to categorization? Their roles in generalization, response selection, and learning via feedback. Neurosci Biobehav Rev. (2008) 32:265–78. doi: 10.1016/j.neubiorev.2007.07.010
13. Graybiel AM. The basal ganglia: learning new tricks and loving it. Curr Opin Neurobiol. (2005) 15:638–44. doi: 10.1016/j.conb.2005.10.006
14. Cavallari M, Ceccarelli A, Wang G-Y, Moscufo N, Hannoun S, Matulis CR, et al. Microstructural changes in the striatum and their impact on motor and neuropsychological performance in patients with multiple sclerosis. PLoS ONE (2014) 9:e101199. doi: 10.1371/journal.pone.0101199
15. Muhlert N, Sethi V, Cipolotti L, Haroon H, Parker GJM, Yousry T, et al. The grey matter correlates of impaired decision-making in multiple sclerosis. J Neurol Neurosurg Psychiatry (2014) 86:530–6. doi: 10.1136/jnnp-2014-308169
16. Strober LB, Arnett PA. Depression in multiple sclerosis: the utility of common self-report instruments and development of a disease-specific measure. J Clin Exp Neuropsychol. (2015) 37:722–32. doi: 10.1080/13803395.2015.1063591
17. Olino TM, McMakin DL, Dahl RE, Ryan ND, Silk JS, Birmaher B, et al. “I won, but I'm not getting my hopes up”: depression moderates the relationship of outcomes and reward anticipation. Psychiatry Res. (2011) 194:393–5. doi: 10.1016/j.pscychresns.2011.04.009
18. Smoski MJ, Felder J, Bizzell J, Green SR, Ernst M, Lynch TR, et al. fMRI of alterations in reward selection, anticipation, and feedback in major depressive disorder. J Affect Disord. (2009) 118:69–78. doi: 10.1016/j.jad.2009.01.034
19. Dobryakova E, Hulst HE, Spirou A, Chiaravalloti N, Genova HM, Wylie GR, et al. Fronto-striatal network activation leads to less fatigue in multiple sclerosis. Mult Scler J. (2017). doi: 10.1177/1352458517717087. [Epub ahead of print].
20. Delgado MR, Nystrom LE, Fissell C, Noll DC, Fiez JA, Arbor A. Tracking the hemodynamic responses to reward and punishment in the striatum. J Neurophysiol. (2000) 84:3072–7. doi: 10.1152/jn.2000.84.6.3072
21. Anselme P, Robinson MJF. What motivates gambling behavior? Insight into dopamine's role. Front Behav Neurosci. (2013) 7:1–4. doi: 10.3389/fnbeh.2013.00182
22. Mirenowicz J, Schultz W. Importance of unpredictability for reward responses in primate dopamine neurons. J Neurophysiol. (1994) 72:1024–7. doi: 10.1152/jn.1994.72.2.1024
24. Carver CS, White TL. Behavioral inhibition, behavioral activation, and affective responses to impending reward and punishment: the BIS/BAS scales. J Pers Soc Psychol. (1994) 67:319–33. doi: 10.1037/0022-3514.67.2.319
25. Scheres A, Sanfey AG. Individual differences in decision making: drive and reward responsiveness affect strategic bargaining in economic games. Behav Brain Funct. (2006) 2:35. doi: 10.1186/1744-9081-2-35
26. Nyenhuis DL, Luchetta T, Yamamoto C, Terrien A, Bernardin L, Rao SM, et al. The development, standardization, and initial validation of the Chicago multiscale depression inventory. J Pers Assess. (1998) 70:386–401. doi: 10.1207/s15327752jpa7002_14
27. Deichmann R, Gottfried JA, Hutton C, Turner R. Optimized EPI for fMRI studies of the orbitofrontal cortex. Neuroimage (2003) 19:430–41. doi: 10.1016/S1053-8119(03)00073-9
28. Smith S, Jenkinson M, Woolrich M, Behrens T, Johansen-Berg H, Bannister P, et al. Advances in functional and structural MR image analysis and implementation as FSL. Neuroimage (2004) 23:S208–19. doi: 10.1016/j.neuroimage.2004.07.051
29. Jenkinson M, Pechaud M, Smith S. BET2-MR-Based Estimation of Brain, Skull and Scalp Surfaces. Oxford, UK (2005).
30. Jenkinson M, Bannister P, Brady M, Smith S. Improved optimization for the robust and accurate linear registration and motion correction of brain images. Neuroimage (2002) 17:825–41. doi: 10.1006/nimg.2002.1132
31. Greve DN, Fischl B. Accurate and robust brain image alignment using boundary-based registration. Neuroimage (2009) 48:63–72. doi: 10.1016/j.neuroimage.2009.06.060
32. Jenkinson M, Smith S. A global optimisation method for robust affine registration of brain images. Med Image Anal. (2001) 5:143–56. doi: 10.1016/S1361-8415(01)00036-6
33. Andersson JLR, Jenkinson M, Smith S, Andersson J. Non-linear Optimisation FMRIB Technial Report TR07JA1. Oxford, UK (2007).
34. Andersson JLR, Jenkinson M, Smith S, Andersson J. Non-linear Registration aka Spatial Normalisation FMRIB Technial Report TR07JA2. Oxford, UK (2007).
35. Woolrich MW, Ripley BD, Brady M, Smith SM. Temporal autocorrelation in univariate linear modeling of FMRI data. Neuroimage (2001) 14:1370–86. doi: 10.1006/nimg.2001.0931
36. Siegel JS, Power JD, Dubis JW, Vogel AC, Church JA, Schlaggar BL, et al. Statistical improvements in functional magnetic resonance imaging analyses produced by censoring high-motion data points. Hum Brain Mapp. (2014) 35:1981–96. doi: 10.1002/hbm.22307
37. Beckmann CF, Jenkinson M, Smith SM. General multilevel linear modeling for group analysis in FMRI. Neuroimage (2003) 20:1052–63. doi: 10.1016/S1053-8119(03)00435-X
38. Woolrich M. Robust group analysis using outlier inference. Neuroimage (2008) 41:286–301. doi: 10.1016/j.neuroimage.2008.02.042
39. Woolrich MW, Behrens TEJ, Beckmann CF, Jenkinson M, Smith SM. Multilevel linear modelling for FMRI group analysis using Bayesian inference. Neuroimage (2004) 21:1732–47. doi: 10.1016/j.neuroimage.2003.12.023
40. Forman SD, Cohen JD, Fitzgerald M, Eddy WF, Mintun MA, Noll DC. Improved assessment of significant activation in functional magnetic resonance imaging (fMRI): use of a cluster-size threshold. Magn Reson Med. (1995) 33:636–47.
41. Battaglini M, Jenkinson M, De Stefano N. Evaluating and reducing the impact of white matter lesions on brain volume measurements. Hum Brain Mapp. (2012) 33:2062–71. doi: 10.1002/hbm.21344
42. Smith SM, De Stefano N, Jenkinson, M, Matthews PM. Normalized accurate measurement of longitudinal brain change. J Comput Assist Tomogr. (2001) 25:466–75. doi: 10.1016/S1053-8119(00)91589-1
43. Douaud G, Smith S, Jenkinson M, Behrens T, Johansen-Berg H, Vickers J, et al. Anatomically related grey and white matter abnormalities in adolescent-onset schizophrenia. Brain (2007) 130:2375–86. doi: 10.1093/brain/awm184
44. Good CD, Johnsrude IS, Ashburner J, Henson RNA, Friston KJ, Frackowiak RSJ. A voxel-based morphometric study of ageing in 465 normal adult human brains. Neuroimage (2001) 14:21–36. doi: 10.1006/nimg.2001.0786
45. Leotti LA, Iyengar SS, Ochsner KN. Born to choose: the origins and value of the need for control. Trends Cogn Sci. (2010) 14:457–63. doi: 10.1016/j.tics.2010.08.001
46. Clark L. Disordered gambling: the evolving concept of behavioral addiction. Ann N Y Acad Sci. (2014) 1327:46–61. doi: 10.1111/nyas.12558
47. Smith SM, Zhang Y, Jenkinson M, Chen J, Matthews PM, Federico A, et al. Accurate, robust, and automated longitudinal and cross-sectional brain change analysis. Neuroimage (2002) 17:479–89. doi: 10.1006/nimg.2002.1040
48. Balleine BW, Delgado MR, Hikosaka O. The role of the dorsal striatum in reward and decision-making. J Neurosci. (2007) 27:8161–5. doi: 10.1523/JNEUROSCI.1554-07.2007
49. Haruno M, Kawato M. Different neural correlates of reward expectation and reward expectation error in the putamen and caudate nucleus during stimulus-action-reward association learning. J Neurophysiol. (2006) 95:948–59. doi: 10.1152/jn.00382.2005
50. Smith AM, Walker LAS, Freedman MS, DeMeulemeester C, Hogan MJ, Cameron I. fMRI investigation of disinhibition in cognitively impaired patients with multiple sclerosis. J Neurol Sci. (2009) 281:58–63. doi: 10.1016/j.jns.2009.02.366
51. Jentsch JD, Taylor JR. Impulsivity resulting from frontostriatal dysfunction in drug abuse: implications for the control of behavior by reward-related stimuli. Psychopharmacology (Berl). (1999) 146:373–90. doi: 10.1007/PL00005483
52. Dalley JW, Mar AC, Economidou D, Robbins TW. Neurobehavioral mechanisms of impulsivity: fronto-striatal systems and functional neurochemistry. Pharmacol Biochem Behav. (2008) 90:250–60. doi: 10.1016/j.pbb.2007.12.021
53. Davis FC, Knodt AR, Sporns O, Lahey BB, Zald DH, Brigidi BD, et al. Impulsivity and the modular organization of resting-state neural networks. Cereb Cortex (2013) 23:1444–52. doi: 10.1093/cercor/bhs126
54. Tricomi E, Balleine BW, O'Doherty JP. A specific role for posterior dorsolateral striatum in human habit learning. Eur J Neurosci. (2009) 29:2225–32. doi: 10.1111/j.1460-9568.2009.06796.x
55. Torregrossa MM, Quinn JJ, Taylor JR. Impulsivity, Compulsivity, and Habit: The Role of Orbitofrontal Cortex Revisited. Biol Psychiatry (2008) 63:253–5. doi: 10.1016/j.biopsych.2007.11.014
56. Koenig KA, Sakaie KE, Lowe MJ, Lin J, Stone L, Bermel RA, et al. Hippocampal volume is related to cognitive decline and fornicial diffusion measures in multiple sclerosis. Magn Reson Imaging. (2014) 32:354–8. doi: 10.1016/j.mri.2013.12.012
57. Roosendaal SD, Hulst HE, Vrenken H, Feenstra HEM, Castelijns JA, Pouwels PJW, et al. Structural and functional hippocampal changes in multiple sclerosis patients with intact memory function. Radiology (2010) 255:595–604. doi: 10.1148/radiol.10091433
58. Geurts JJG, Bö L, Roosendaal SD, Hazes T, Daniëls R, Barkhof F, et al. Extensive hippocampal demyelination in multiple sclerosis. J Neuropathol Exp Neurol. (2007) 66:819–27. doi: 10.1097/nen.0b013e3181461f54
59. White NM, McDonald RJ. Multiple parallel memory systems in the brain of the rat. Neurobiol Learn Mem. (2002) 77:125–84. doi: 10.1006/nlme.2001.4008
60. Dickerson KC, Delgado MR. Contributions of the hippocampus to feedback learning. Cogn Affect Behav Neurosci. (2015) 15:861–77. doi: 10.3758/s13415-015-0364-5
61. Murty VP, LaBar KS, Adcock RA. Distinct medial temporal networks encode surprise during motivation by reward versus punishment. Neurobiol Learn Mem. (2016) 134:55–64. doi: 10.1016/j.nlm.2016.01.018
62. Voermans NC, Petersson KM, Daudey L, Weber B, van Spaendonck KP, Kremer HPH, et al. Interaction between the human hippocampus and the caudate nucleus during route recognition. Neuron (2004) 43:427–35. doi: 10.1016/j.neuron.2004.07.009
63. Doeller CF, Opitz B, Krick CM, Mecklinger A, Reith W. Prefrontal-hippocampal dynamics involved in learning regularities across episodes. Cereb Cortex. (2005) 15:1123–33. doi: 10.1093/cercor/bhh211
64. Oskarsson AT, Van Boven L, McClelland, GH, Hastie R. What's next? Judging sequences of binary events. Psychol Bull. (2009) 135:262–85. doi: 10.1037/a0014821
65. Kim H. Dissociating the roles of the default-mode, dorsal, and ventral networks in episodic memory retrieval. Neuroimage (2010) 50:1648–57. doi: 10.1016/j.neuroimage.2010.01.051
66. Acikalin MY, Gorgolewski KJ, Poldrack RA. A coordinate-based meta-analysis of overlaps in regional specialization and functional connectivity across subjective value and default mode networks. Front Neurosci. (2017) 11:1. doi: 10.3389/fnins.2017.00001
67. Rocca MA, Valsasina P, Hulst HE, Abdel-Aziz K, Enzinger C, Gallo A, et al. Functional correlates of cognitive dysfunction in multiple sclerosis: a multicenter fMRI Study. Hum Brain Mapp. (2014) 35:5799–814. doi: 10.1002/hbm.22586
68. Delgado MR, Stenger VA, Fiez JA. Motivation-dependent responses in the human caudate nucleus. Cereb Cortex. (2004) 14:1022–30. doi: 10.1093/cercor/bhh062
Keywords: fMRI, reward, anticipation, multiple sclerosis, striatum, hippocampus, motivation
Citation: Spirou A, Liu P-P, Natsheh JY, Neuteboom E and Dobryakova E (2018) Neural Correlates of Outcome Anticipation in Multiple Sclerosis. Front. Neurol. 9:572. doi: 10.3389/fneur.2018.00572
Received: 14 March 2018; Accepted: 25 June 2018;
Published: 25 July 2018.
Edited by:
Federica Agosta, Università Vita-Salute San Raffaele, ItalyReviewed by:
Menno Michiel Schoonheim, VU University Medical Center, NetherlandsPaola Valsasina, San Raffaele Scientific Institute (IRCCS), Italy
Copyright © 2018 Spirou, Liu, Natsheh, Neuteboom and Dobryakova. This is an open-access article distributed under the terms of the Creative Commons Attribution License (CC BY). The use, distribution or reproduction in other forums is permitted, provided the original author(s) and the copyright owner(s) are credited and that the original publication in this journal is cited, in accordance with accepted academic practice. No use, distribution or reproduction is permitted which does not comply with these terms.
*Correspondence: Ekaterina Dobryakova, edobryakova@kesslerfoundation.org
† These authors have contributed equally to this work.