- 1Neuroradiology Unit, Fondazione IRCCS Istituto Neurologico “Carlo Besta,”, Milan, Italy
- 2Center for Mind/Brain Sciences (CIMeC), University of Trento, Trento, Italy
Preoperative mapping of brain functions is the most common clinical application of functional MRI (fMRI) (1). The pre-surgical localization of eloquent areas has a positive impact for maximizing the extent of resection while reducing intra-operative mapping time (2) and improving patient outcome (3). In the pre-surgical setting, the typical fMRI approach employs conventional tasks which require patients to execute simple tasks in the scanner (4–8). This task-based fMRI (tb-fMRI) approach is well established and widely used in clinical routine, but has limitations: patients must be able to perform the tasks appropriately, implementation has a cost, and trained personnel is needed to select the proper task and instruct the patient.
One straightforward alternative to task-based fMRI is resting-state fMRI (rs-fMRI). This technique allows the study of spontaneous, low-frequency fluctuations that occur throughout the brain and has been widely used to characterize the healthy brain and neurological diseases. With rs-fMRI it is possible to identify a number of networks, or components, which are strongly functionally connected at rest and highly reproducible across subjects and sessions (9–11). Some of them show similar topographies to tb-fMRI networks, that is regions activated during cognitive functions, visual or sensory-motor tasks (12, 13). Measures of functional connectivity allow the study of brain functional reorganization and neuronal changes associated with brain disease (14). Alterations of rs-fMRI networks have been identified in many neurological and psychiatric disorders, even in absence of structural modifications, and in some studies have been shown to correlate with disease progression and severity (15).
Rs-fMRI has been applied also in the preoperative setting to overcome the limitations of task-based fMRI, including the need of active patient participation (16). rs-fMRI has the additional advantage that acquisition is brief (~6–10 min) and can be easily managed by MRI technicians. Initial studies to validate the technique show good concordance with the gold standard intraoperative electro-cortical stimulation (ECS) (17–20). The most commonly identified networks for pre-surgical planning are the sensori-motor component, encompassing pre- and post-central cortex with the supplementary motor area, and the language network including Broca and Wernicke's areas, often corresponding to the fronto-parietal network (21–23).
Open Issues for Presurgical rs-fMRI
Despite promising results, there remain open issues for the use of rs-fMRI in the preoperative setting. The methodology is the most critical issue: with rs-fMRI the acquisition is easy, but analyses are complex and have not been standardized yet. Multiple analysis techniques are available and, according to the method, different results can be obtained from the same dataset (11, 24, 25). Identification of the network is a critical step and can occur: (i) automatically or semi-automatically with Independent Component Analysis (ICA), a data-driven method commonly used: a network among a set of components is selected either visually (26) or through a spatial matching with respect to network templates (17, 27, 28, 29); (ii) manually, with a seed-based approach, where pre-defined region-of-interest (ROI)s or seeds are selected based on a-priori hypothesis; (iii) with alternative methods, such as machine learning approaches (18, 30, 31), cortical parcellating approach (19) or graph analyses (15).
Networks are not all as easily identifiable. The sensori-motor component is one of the lower-level networks with a known functional correlate (i.e., it corresponds to the sensori-motor system) which is robust and easy to identify at individual level (32). On the other hand, the identification of the language component is more challenging. Language is a “higher-order” function with substantial variability across subjects and several cognitive functions such as comprehension, production and perception (if a word is presented visually) are involved in language. For this reason, it remains to be determined whether a single language network can be observed. In addition, when language is mapped with ICA, the network showing the highest spatial overlap with the task-based map or known language areas is used (26, 28, 33, 34), while when it is mapped with the seed-based analysis, language ROIs of the left or right hemisphere or electrically stimulated points as seeds are used (17, 35, 36).
Language lateralization is also important in preoperative mapping, as surgical interventions in the dominant hemisphere are at higher risk of post-operative language deficits than interventions in the non-dominant hemisphere (37). rs-fMRI has been shown to provide results that are generally concordant with the Wada test for language dominance, similarly to tb-fMRI (33, 38). However, the degree of language lateralization measured with rs-fMRI varies considerably, depending on regions and methods used (35, 36, 39, 40). More studies assessing rs-fMRI lateralization with clinical, neuropsychological and post-surgical outcome evaluations are needed in order to determine which method (ICA or seed) provides the most reliable results (34, 41). At present, it is still unclear which method of analysis produces the most reliable results for mapping eloquent areas.
The second issue concerns the differences between rs-fMRI and tb-fMRI. The rs-fMRI map is different from the tb-fMRI one, as the two techniques are intrinsically different. As recently reported by Derks et al. (42), the two imaging methods measure different aspects of brain function. Tb-fMRI is related to the performance of a task or administration of a stimulus, and the resulting maps represent the brain areas involved. By contrast, rs-fMRI refers to brain's intrinsic activity, the degree of communication between areas and the resulting maps represent networks of synchronous BOLD activity (43). This may explain why the degree of overlap between the two techniques is not complete (30, 44, 45). In particular, the motor network observed at rest often covers a larger portion of the motor cortex compared to the more focal region identified with tb-fMRI (30, 44). Understanding whether a region is necessary for a function might be more difficult with rs-fMRI; in practice, future studies comparing rs-fMRI with ECS data will evaluate the accuracy of rs-fMRI results, using sensitivity and specificity measures (17–20). In other cases, the rs-fMRI motor network covers a smaller portion of the motor cortex when, for instance, a high number of components is selected with ICA, and the foot or mouth motor areas can be difficult to identify with ICA (44, 46).
An example of motor and language mapping performed with rs-fMRI and ECS is illustrated in Figure 1. Motor mapping performed with rs-fMRI through a motor seed (placed in the healthy Rolandic cortex), provided a larger portion of sensori-motor areas compared to the finger tapping map (in green). In the same patient, ICA showed a different localization of the hand motor area compared to tb-fMRI and seed-based analysis. Nevertheless, the stimulation site which elicited motor responses was included in both rs-fMRI and tb-fMRI activations (patient 12 in Rosazza et al. (44)). In another patient, language mapping performed with rs-fMRI data through a language seed (placed in the left inferior frontal gyrus) and ICA, localized, to some extent, different language areas, even if the stimulation site was included in the activation pattern (patient 6 in Zacà et al. 47).
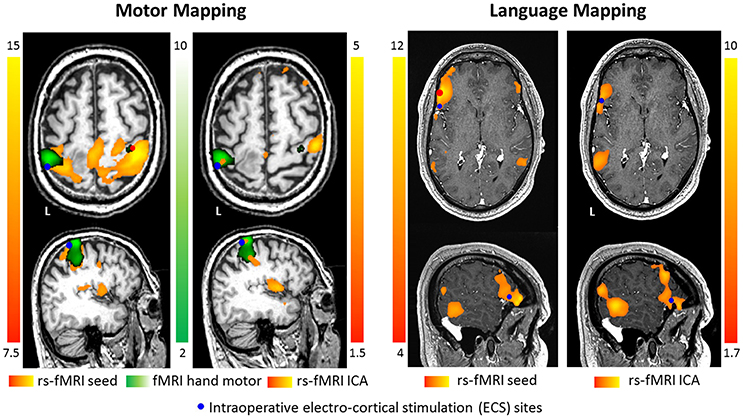
Figure 1. Motor mapping was performed in a surgical patient using rs-fMRI with motor seed (in red, placed in the healthy Rolandic cortex), rs-fMRI ICA, a finger tapping motor task and ECS data (patient 12 in Rosazza et al. (44)). Results showed that seed-based analysis, ICA and tb-fMRI, provide comparable but not equivalent results; nevertheless, the stimulation site (in blue) was included in each activation pattern. In another surgical patient language mapping was performed using rs-fMRI with language seed (in red, placed in the left inferior frontal gyrus), rs-fMRI ICA and ECS data (patient 6 in Zacà et al. (47)). Results showed that seed-based analysis and ICA provided similar but not equivalent results; however, stimulation site (in blue) was included in the pattern of correlated activity.
Among the factors that contribute to different rs-fMRI patterns, there is the placement of seeds when using the seed-based analysis, as seed size and location can bias the network, especially in lesioned brains. By contrast, when using ICA, networks can be combined or split, according to the small or big number of components used. In general, although ICA has ambiguity in choosing the number of components and identifying the components of interest, it is more explorative and less subjective than seed-based approach. Therefore, it may work better in patient populations, especially when large lesions may prevent the identification of reliable seeds in the eloquent cortex. In comparison with rs-fMRI, also tb-fMRI has some drawbacks, including: the choice of tasks used in particular for language mapping, the use of active vs. control conditions where it can be difficult to match the cognitive demands of the conditions and finally the use of two tasks that can be more time consuming than a single rs-fMRI sequence.
The third issue concerns the interpretation of rs-fMRI results. The pattern of correlated activity observed with rs-fMRI is not easy to interpret. With tb-fMRI, a map is associated to a behavior or cognitive function and this makes the results quite clear: in language mapping, for example, anterior regions are expected to be activated for production and so are posterior regions for comprehension; in addition, a differential involvement of anterior vs. posterior temporal regions is typically observed depending on the task (48, 49).
With rs-fMRI it is more difficult to understand the functional specificity of the map and interpret the results. The interpretation is even more difficult when rs-fMRI maps do not include expected areas, such as the paracentral lobule of the sensori-motor network. Most importantly, correlations of rs-fMRI pattern with cognitive or behavioral data have been established only occasionally. For instance, the rs-fMRI study by Otten et al. (50) has shown that in patients with brain tumors, motor weakness was associated with reduced connectivity of the sensori-motor network between inter-hemispheric motor areas. Further studies correlating measures of functional connectivity with clinical and cognitive data are necessary to understand the value of rs-fMRI in the clinical setting.
Future Development of rs-fMRI
Rs-fMRI has begun to make clinically meaningful contribution to the localization of eloquent areas. To make rs-fMRI standard of care for pre-surgical mapping, further developments in the methodological and theoretical setting are needed. From a methodological point of view, analyses must be reliable, quick and easily applicable in the preoperative routine for single patients. Initial steps have been made in this direction (51–53); however the procedure of analysis must be standardized, validated with ECS data and replicated in large population studies.
We can hypothesize that for motor mapping, the manual approach with the seed-based analysis will be better suited to localize the area of interest (foot, hand or mouth) with respect to the lesion. In fact, the seed-based analysis offers the flexibility necessary to explore the functional connectivity from different ROIs, even if it is more sensitive to the type of preprocessing performed. However, when the identification of motor seeds is confounded by pathology, ICA could provide a valid alternative approach (30, 53). In contrast, for language mapping, a semi-automatic approach with ICA could be better suited to assess lateralization and also localize language. ICA could be repeated setting a different number of components (e.g., 10, 20, 30.) and selecting the network that best matches a pre-defined template. This approach has been shown to provide reliable results because it takes into account both the variability of optimal number of components and localization of language areas across patients (52). When possible, the two methods of analysis should be applied jointly to obtain an independent confirmation of findings, similarly to the tb-fMRI procedure where two or more tasks are typically administered to map a function.
Considering the current limitations, we believe that, at present, tb-fMRI represents the first paradigm to choose for preoperative mapping of brain functions. Tb-fMRI is more robust with respect to noise, different tasks can be employed to map specific areas, analyses are quick, widely applied in the clinical routine, and maps are easy to interpret. When tb-fMRI cannot be used for clinical or logistic reasons, for instance because patients cannot perform the task or the stimulus delivery device is not available, then rs-fMRI will be used for the same purpose.
However, looking forward, we believe that, in the future, conceptual and methodological advances in neuroimaging techniques will allow a broader application of rs-fMRI functional connectivity in different neurological disease, including surgical practice. We are in fact shifting from a localizationist vision to a network-centric perspective, according to which the brain is organized into hierarchical, integrated, and interconnected large-scale networks, and neurological diseases are described as neuronal circuits dysfunctions (54–56). Network modeling of neuro-pathological conditions will be widely performed through connectivity analyses within and between networks, and results will be easily visualized to make rapid clinical decisions (15, 57). This is going to be accompanied by important theoretical advancements in clinical neuroscience: the functional relevance of rs-fMRI measures and their clinical correlates will be elucidated, together with a clearer definition of the areas of networks indicating eloquent cortex. rs-fMRI is going to be used also to localize glioma-related alterations and delineate the degree of tumor infiltration (14). From longitudinal studies it is going to be possible to understand network changes occurring throughout surgery and this will allow the development of personalized treatments (58). In this context, we can imagine that rs-fMRI will be used in the preoperative setting not only to map eloquent areas but also to get information about changes on neuronal circuits caused by lesions, and eventually it will provide the basis for a multi-network assessment for diagnosis, prognosis and treatment of single patients.
In conclusion, methodological and theoretical limitations currently prevent a routine use of rs-fMRI in the pre-surgical setting. However, there is a wider potential for this technique that it is likely to be realized in the future also for preoperative mapping.
Author Contributions
CR and MB suggested the idea of the work. CR and DZ drafted the manuscript. CR, DZ, and MB revised the manuscript. All authors have read and approved the manuscript in its final form.
Conflict of Interest Statement
The authors declare that the research was conducted in the absence of any commercial or financial relationships that could be construed as a potential conflict of interest.
References
1. Matthews PM, Honey GD, Bullmore ET. Applications of fMRI in translational medicine and clinical practice. Nat Rev Neurosci. (2006) 7:732–44. doi: 10.1038/nrn1929
2. Petrella JR, Shah LM, Harris KM, Friedman AH, George TM, Sampson JH, et al. Preoperative functional MR imaging localization of language and motor areas: effect on therapeutic decision making in patients with potentially resectable brain tumors. Radiology (2006) 240:793–802. doi: 10.1148/radiol.2403051153
3. Vysotski S, Madura C, Swan B, Holdsworth R, Lin Y, Munoz Del Rio A, et al. Preoperative FMRI associated with decreased mortality and morbidity in brain tumor patients. Interdiscip Neurosurg. (2018) 13:40–5. doi: 10.1016/j.inat.2018.02.001
4. Sunaert S. Presurgical planning for tumor resectioning. J Magn Reson Imaging (2006) 23:887–905. doi: 10.1002/jmri.20582
5. Pillai JJ. The evolution of clinical functional imaging during the past 2 decades and its current impact on neurosurgical planning. Am J Neuroradiol. (2010) 31:219–25. doi: 10.3174/ajnr.A1845
6. Silva MA, See AP, Essayed WI, Golby AJ, Tie Y. Challenges and techniques for presurgical brain mapping with functional MRI. NeuroImage Clin. (2018) 17:794–803. doi: 10.1016/j.nicl.2017.12.008
7. Gabriel M, Brennan NP, Peck KK, Holodny AI. Blood oxygen level dependent functional magnetic resonance imaging for presurgical planning. Neuroimaging Clin N Am. (2014) 24:557–71. doi: 10.1016/j.nic.2014.07.003
8. Ulmer JL, Klein AP, Mark LP, Tuna I, Agarwal M, DeYoe E. Functional and dysfunctional sensorimotor anatomy and imaging. Semin Ultrasound CT MRI (2015) 36:220–33. doi: 10.1053/j.sult.2015.05.014
9. Beckmann CF, DeLuca M, Devlin JT, Smith SM. Investigations into resting-state connectivity using independent component analysis. Philos Trans R Soc B Biol Sci. (2005) 360:1001–13. doi: 10.1098/rstb.2005.1634
10. Biswal BB, Mennes M, Zuo X-N, Gohel S, Kelly C, Smith SM, et al. Toward discovery science of human brain function. Proc Natl Acad Sci USA. (2010) 107:4734–4739. doi: 10.1073/pnas.0911855107
11. Jovicich J, Minati L, Marizzoni M, Marchitelli R, Sala-Llonch R, Bartrés-Faz D, et al. Longitudinal reproducibility of default-mode network connectivity in healthy elderly participants: a multicentric resting-state fMRI study. Neuroimage (2016) 124:442–54. doi: 10.1016/j.neuroimage.2015.07.010
12. Calhoun VD, Kiehl KA, Pearlson GD. Modulation of temporally coherent brain networks estimated using ICA at rest and during cognitive tasks. Hum Brain Mapp. (2008) 29:828–38. doi: 10.1002/hbm.20581
13. Smith SM, Fox PT, Miller KL, Glahn DC, Fox PM, Mackay CE, et al. Correspondence of the brain's functional architecture during activation and rest. Proc Natl Acad Sci USA. (2009) 106:13040–5. doi: 10.1073/pnas.0905267106
14. Ghinda DC, Wu JS, Duncan NW, Northoff G. How much is enough—can resting state fMRI provide a demarcation for neurosurgical resection in glioma? Neurosci Biobehav Rev. (2018) 84:245–61. doi: 10.1016/j.neubiorev.2017.11.019
15. Barkhof F, Haller S, Rombouts SARB. Resting-State Functional MR Imaging: a new window to the brain. Radiology (2014) 272:29–49. doi: 10.1148/radiol.14132388
16. Leuthardt EC, Allen M, Kamran M, Hawasli AH, Snyder AZ, Hacker CD, et al. Resting-state blood oxygen level-dependent functional MRI: a paradigm shift in preoperative brain mapping. Stereotact Funct Neurosurg. (2015) 93:427–39. doi: 10.1159/000442424
17. Cochereau J, Deverdun J, Herbet G, Charroud C, Boyer A, Moritz-Gasser S, et al. Comparison between resting state fMRI networks and responsive cortical stimulations in glioma patients. Hum Brain Mapp. (2016) 37:3721–32. doi: 10.1002/hbm.23270
18. Mitchell TJ, Hacker CD, Breshears JD, Szrama NP, Sharma M, Bundy DT, et al. A novel data-driven approach to preoperative mapping of functional cortex using resting-state functional magnetic resonance imaging. Neurosurgery (2013) 73:969–83. doi: 10.1227/NEU.0000000000000141
19. Wang D, Buckner RL, Fox MD, Holt DJ, Holmes AJ, Stoecklein S, et al. Parcellating cortical functional networks in individuals. Nat Neurosci. (2015) 18:1853–60. doi: 10.1038/nn.4164
20. Qiu TM, Yan CG, Tang WJ, Wu JS, Zhuang DX, Yao CJ, et al. Localizing hand motor area using resting-state fMRI: validated with direct cortical stimulation. Acta Neurochir (Wien). (2014) 156:2295–302. doi: 10.1007/s00701-014-2236-0
21. Liu H, Stufflebeam SM, Sepulcre J, Hedden T, Buckner RL. Evidence from intrinsic activity that asymmetry of the human brain is controlled by multiple factors. Proc Natl Acad Sci USA. (2009) 106:20499–503. doi: 10.1073/pnas.0908073106
22. Zhang D, Raichle ME. Disease and the brain's dark energy. Nat Rev Neurol. (2010) 6:15–28. doi: 10.1038/nrneurol.2009.198
23. Rosazza C, Minati L. Resting-state brain networks: literature review and clinical applications. Neurol Sci. (2011) 32:773–85. doi: 10.1007/s10072-011-0636-y
24. Hale JR, Mayhew SD, Mullinger KJ, Wilson RS, Arvanitis TN, Francis ST, et al. Comparison of functional thalamic segmentation from seed-based analysis and ICA. Neuroimage (2015) 114:448–65. doi: 10.1016/j.neuroimage.2015.04.027
25. Rosazza C, Minati M, Ghielmetti F, Mandelli ML, Bruzzone MG. Functional connectivity during resting-state functional MR imaging: study of the correspondence between independent component analysis and region-of-interest - Based methods. Am J Neuroradiol. (2012) 33:180–7. doi: 10.3174/ajnr.A2733
26. Sair HI, Yahyavi-Firouz-Abadi N, Calhoun VD, Airan RD, Agarwal S, Intrapiromkul J, et al. Presurgical brain mapping of the language network in patients with brain tumors using resting-state fMRI: comparison with task fMRI. Hum Brain Mapp. (2016) 37:913–923. doi: 10.1002/hbm.23075
27. Tie Y, Rigolo L, Norton IH, Huang RY, Wu W, Orringer D, et al. Defining language networks from resting-state fMRI for surgical planning- a feasibility study. Hum Brain Mapp. (2014) 35:1018–1030. doi: 10.1002/hbm.22231
28. Branco P, Seixas D, Castro SL. Temporal reliability of ultra-high field resting-state MRI for single-subject sensorimotor and language mapping. Neuroimage (2016) 168:499–508. doi: 10.1016/j.neuroimage.2016.11.029
29. Lu J, Zhang H, Hameed NUF, Zhang J, Yuan S, Qiu T, et al. An automated method for identifying an independent component analysis-based language-related resting-state network in brain tumor subjects for surgical planning. Sci Rep. (2017) 7:1–16. doi: 10.1038/s41598-017-14248-5
30. Dierker D, Roland JL, Kamran M, Rutlin J, Hacker CD, Marcus DS, et al. Resting-state functional magnetic resonance imaging in presurgical functional mapping: sensorimotor localization. Neuroimaging Clin N Am. (2017) 27:621–33. doi: 10.1016/j.nic.2017.06.011
31. Vergun S, Gaggl W, Nair VA, Suhonen JI, Birn RM, Ahmed AS, et al. Classification and extraction of resting state networks using healthy and epilepsy fMRI data. Front Neurosci. (2016) 10:440. doi: 10.3389/fnins.2016.00440
32. Kollndorfer K, Fischmeister FPS, Kasprian G, Prayer D, Schöpf V. A systematic investigation of the invariance of resting-state network patterns: is resting-state fMRI ready for pre-surgical planning? Front Hum Neurosci. (2013) 7:95. doi: 10.3389/fnhum.2013.00095
33. DeSalvo MN, Tanaka N, Douw L, Leveroni CL, Buchbinder BR, Greve DN, et al. Resting-state functional MR imaging for determining language laterality in intractable epilepsy. Radiology (2016) 281:264–9. doi: 10.1148/radiol.2016141010
34. Piervincenzi C, Petrilli A, Marini A, Caulo M, Committeri G, Sestieri C. Multimodal assessment of hemispheric lateralization for language and its relevance for behavior. Neuroimage (2016) 142:351–70. doi: 10.1016/j.neuroimage.2016.08.018
35. Doucet GE, Pustina D, Skidmore C, Sharan A, Sperling MR, Tracy JI. Resting-state functional connectivity predicts the strength of hemispheric lateralization for language processing in temporal lobe epilepsy and normals. Hum Brain Mapp. (2015) 36:288–303. doi: 10.1002/hbm.22628
36. Teghipco A, Hussain A, Tivarus ME. Disrupted functional connectivity affects resting state based language lateralization. Neuroimage Clin. (2016) 12:910–27. doi: 10.1016/j.nicl.2016.10.015
37. Zacà D, Jarso S, Pillai JJ. Role of semantic paradigms for optimization of language mapping in clinical fMRI studies. Am J Neuroradiol. (2013) 34:1966–71. doi: 10.3174/ajnr.A3628
38. Janecek JK, Swanson SJ, Sabsevitz DS, Hammeke TA, Raghavan ME, Rozman M, et al. Language lateralization by fMRI and Wada testing in 229 patients with epilepsy: rates and predictors of discordance. Epilepsia (2013) 54:314–22. doi: 10.1111/epi.12068
39. Takaya S, Kuperberg GR, Liu H, Greve DN, Makris N, Stufflebeam SM. Asymmetric projections of the arcuate fasciculus to the temporal cortex underlie lateralized language function in the human brain. Front Neuroanat. (2015) 9:119. doi: 10.3389/fnana.2015.00119
40. Smitha K, Akhil Raja K, Arun K, Rajesh P, Thomas B, Kapilamoorthy T, et al. Resting state fMRI: A review on methods in resting state connectivity analysis and resting state networks. Neuroradiol J. (2017) 30:305–17. doi: 10.1177/1971400917697342
41. Bradshaw AR, Thompson PA, Wilson AC, Bishop DVM, Woodhead ZVJ. Measuring language lateralisation with different language tasks: a systematic review. PeerJ. (2017) 5:e3929. doi: 10.7717/peerj.3929
42. Derks J, Dirkson AR, de Witt Hamer PC, van Geest Q, Hulst HE, Barkhof F, et al. Connectomic profile and clinical phenotype in newly diagnosed glioma patients. Neuroimage Clin. (2017) 14:87–96. doi: 10.1016/j.nicl.2017.01.007
43. Deco G, Corbetta M. The dynamical balance of the brain at rest. Neuroscientist (2011) 17:107–23. doi: 10.1177/1073858409354384
44. Rosazza C, Aquino D, D'Incerti L, Cordella R, Andronache A, Zacà D, et al. Preoperative mapping of the sensorimotor cortex: comparative assessment of task-based and resting-state fMRI. PLoS ONE (2014) 9:e98860. doi: 10.1371/journal.pone.0098860
45. Hou BL, Bhatia S, Carpenter JS. Quantitative comparisons on hand motor functional areas determined by resting state and task BOLD fMRI and anatomical MRI for pre-surgical planning of patients with brain tumors. Neuroimage Clin. (2016) 11:378–387. doi: 10.1016/j.nicl.2016.03.003
46. Kristo G, Rutten GJ, Raemaekers M, de Gelder B, Rombouts SARB, Ramsey NF. Task and task-free FMRI reproducibility comparison for motor network identification. Hum Brain Mapp. (2014) 35:340–352. doi: 10.1002/hbm.22180
47. Zacà D, Jovicich J, Corsini F, Rozzanigo U, Chioffi F, Sarubbo S. ReStNeuMap: a tool for automatic extraction of resting state fMRI networks in neurosurgical practice. J Neurosurg (in press).
48. Rosazza C, Ghielmetti F, Minati L, Vitali P, Giovagnoli AR, Deleo F, et al. Preoperative language lateralization in temporal lobe epilepsy (TLE) predicts peri-ictal, pre- and post-operative language performance: an fMRI study. NeuroImage Clin. (2013) 3:73–83 doi: 10.1016/j.nicl.2013.07
49. Bizzi A. Presurgical mapping of verbal language in brain tumors with functional MR imaging and MR tractography. Neuroimaging Clin N Am. (2009) 19:573–96. doi: 10.1016/j.nic.2009.08.010
50. Otten ML, Mikell CB, Youngerman BE, Liston C, Sisti MB, Bruce JN, et al. Motor deficits correlate with resting state motor network connectivity in patients with brain tumours. Brain (2012) 135:1017–26. doi: 10.1093/brain/aws041
51. Böttger J, Margulies DS, Horn P, Thomale UW, Podlipsky I, Shapira-Lichter I, et al. A software tool for interactive exploration of intrinsic functional connectivity opens new perspectives for brain surgery. Acta Neurochir (Wien) (2011) 153:1561–72. doi: 10.1007/s00701-011-0985-6
52. Huang H, Ding Z, Mao D, Yuan J, Zhu F, Chen S, et al. PreSurgMapp: a MATLAB toolbox for presurgical mapping of eloquent functional areas based on task-related and resting-state functional MRI. Neuroinformatics (2016) 14:421–38. doi: 10.1007/s12021-016-9304-y
53. Lang S, Duncan N, Northoff G. Resting-state functional magnetic resonance imaging: review of neurosurgical applications. Neurosurgery (2014) 74:453–64. doi: 10.1227/NEU.0000000000000307
54. Stam CJ. Modern network science of neurological disorders. Nat Rev Neurosci. (2014) 15:683–95. doi: 10.1038/nrn3801
55. Park HJ, Friston K. Structural and functional brain networks: from connections to cognition. Science (80-) (2013) 342:1238411. doi: 10.1126/science.1238411
56. Petersen SE, Sporns O. Brain networks and cognitive architectures. Neuron (2015) 88:207–219. doi: 10.1016/j.neuron.2015.09.027
57. Bullmore E. The future of functional MRI in clinical medicine. Neuroimage (2012) 62:1267–1. doi: 10.1016/j.neuroimage.2012.01.026
Keywords: resting-state fMRI, task-based fMRI, preoperative setting, motor mapping, language mapping, functional connectivity
Citation: Rosazza C, Zacà D and Bruzzone MG (2018) Pre-surgical Brain Mapping: To Rest or Not to Rest? Front. Neurol. 9:520. doi: 10.3389/fneur.2018.00520
Received: 28 February 2018; Accepted: 12 June 2018;
Published: 03 July 2018.
Edited by:
Giorgio Sandrini, University of Pavia, ItalyReviewed by:
Yanmei Tie, Harvard Medical School, United StatesCopyright © 2018 Rosazza, Zacà and Bruzzone. This is an open-access article distributed under the terms of the Creative Commons Attribution License (CC BY). The use, distribution or reproduction in other forums is permitted, provided the original author(s) and the copyright owner(s) are credited and that the original publication in this journal is cited, in accordance with accepted academic practice. No use, distribution or reproduction is permitted which does not comply with these terms.
*Correspondence: Cristina Rosazza, cristina.rosazza@istituto-besta.it