- Social, Cognitive, and Affective Neuroscience Laboratory, Department of Psychiatry, College of Medicine, University of Arizona, Tucson, AZ, United States
Background: Mild traumatic brain injuries (mTBIs) are a significant social, sport, and military health issue. In spite of advances in the clinical management of these injuries, the underlying pathophysiology is not well-understood. There is a critical need to advance objective biomarkers, allowing the identification and tracking of the long-term evolution of changes resulting from mTBI. Diffusion-weighted imaging (DWI) allows for the assessment of white-matter properties in the brain and shows promise as a suitable biomarker of mTBI pathophysiology.
Methods: 34 individuals within a year of an mTBI (age: 24.4 ± 7.4) and 18 individuals with no history of mTBI (age: 23.2 ± 3.4) participated in this study. Participants completed self-report measures related to functional outcomes, psychological health, post-injury symptoms, and sleep, and underwent a neuroimaging session that included DWI. Whole-brain white matter was skeletonized using tract-based spatial statistics (TBSS) and compared between groups as well as correlated within-group with the self-report measures.
Results: There were no statistically significant anatomical differences between the two groups. After controlling for time since injury, fractional anisotropy (FA) demonstrated a negative correlation with sleep quality scores (higher FA was associated with better sleep quality) and increasing depressive symptoms in the mTBI participants. Conversely, mean (MD) and radial diffusivity (RD) demonstrated positive correlations with sleep quality scores (higher RD was associated with worse sleep quality) and increasing depressive symptoms. These correlations were observed bilaterally in the internal capsule (anterior and posterior limbs), corona radiata (anterior and superior), fornix, and superior fronto-occipital fasciculi.
Conclusion: The results of this study indicate that the clinical presentation of mTBI, particularly with respect to depression and sleep, is associated with reduced white-matter integrity in multiple areas of the brain, even after controlling for time since injury. These areas are generally associated not only with sleep and emotion regulation but also cognition. Consequently, the onset of depression and sleep dysfunction as well as cognitive impairments following mTBI may be closely related to each other and to white-matter integrity throughout the brain.
Introduction
Concussions and mild traumatic brain injuries (mTBI) represent a significant public and military health crisis. 1.6 to 3.8 million sport-related concussions (SRCs) are reported annually (1, 2), and more than 300,000 mTBIs (hereafter referring to both mTBIs and SRCs) have been documented in military personnel since the year 2000 (3). The actual incidence of these injuries is likely much higher, as estimates reflect only those for which treatment is sought (4). Treatment costs for mTBIs top $22 billion annually in the United States (5). Individuals sustaining an mTBI may exhibit any number of clinical features, including changes in cognitive and motor function as well as post-injury depression, somatic symptoms, and sleep-wake cycle disruption (6). However, these clinical signs and symptoms are not generally associated with visible structural abnormalities when using traditional diagnostic/clinical neuroimaging techniques (e.g., structural magnetic resonance imaging (MRI) or computed tomography (CT) in the emergency department). Furthermore, although many of the clinical signs and symptoms resolve within the first month post-injury (6), many individuals continue to experience symptoms well beyond this clinical timeframe.
Among those persistent symptoms, sleep disruption and depression are among the most common. Estimates of the prevalence of sleep disruption following mTBI ranges from 30 to 80% (7–9), with complaints of insomnia, hypersomnia, and pleiosomnia all reported (8, 10–12). Individuals with prior mTBI also often report and exhibit depressive symptoms, with an estimated 6% per year being clinically diagnosed with depression (13) and many more exhibiting depressive symptoms (14). Notably, depression may additionally cause altered sleep patterns (15). Collectively, both sleep disruption and depression can impair cognitive and physical function (16–20) and may therefore exacerbate the symptoms of and delay the recovery from an mTBI. However, to date, there are have a limited number of studies that have identified the neural correlates of both sleep disruption (21) or depression (22, 23) following mTBI. Consequently, it is needful to identify objective biomarkers of both the pathophysiology and post-injury recovery that underpin the evolution of post-mTBI sleep disruption and depression.
One imaging methodology that is particularly sensitive to altered brain structure is diffusion tensor imaging (DTI). In DTI, water molecule diffusion properties in the brain are quantified, principally by fractional anisotropy (FA) and mean diffusivity (MD). FA quantifies molecular diffusion along three dimensions (FA = 0: diffusion is equally likely in any direction; FA = 1: diffusion occurs along one direction), while MD quantifies the average three-dimensional diffusion rate. Additionally, radial diffusivity (RD) and axial diffusivity (AD) reflect the rates of diffusion perpendicular and parallel to the underlying tissue, respectively. These diffusion metrics, and most prevalently FA, are thought to provide an index of white matter integrity. Mouse models of neural trauma have demonstrated decreased AD concomitant with axonal damage (24, 25) and negative correlations between RD and myelination [e.g., higher RD is associated with reduced myelination; (25, 26)]. MD, the average of AD and RD, is non-specific with respect to the direction of diffusion. Increases in MD and coincident decreases in FA are often associated with neural trauma and neurodegeneration (27, 28), including mTBI (29–31).
Mild traumatic brain injuries may reflect a model of diffuse axonal injury (DAI), characterized by damage to, and subsequently the loss of, axons, myelin, or both (32–34). Demyelination has been observed in animal models of mTBI (35) and may be secondary to axonal loss or loss of oligodendrocytes supplying undamaged axons (36–39). Regardless of mechanism, white matter integrity may be compromised following mTBI. Therefore, DTI metrics may provide a suitable biomarker of both microstructural changes following mTBI as well as clinical presentation.
With respect to mTBI, numerous publications have featured DTI-related findings in civilian, military, and sport populations, spanning timeframes from acute to remote (years) post-injury (40–42). Despite the density of publications, there is little consistency in the findings with respect to directional changes in DTI metrics. While some studies, for instance, report lower FA following mTBI (43–47), this is not always the case (48–51). Such inconsistent patterns are also present with respect to MD, AD, and RD (43, 46, 48, 52). Given the heterogeneous nature of mechanistic/neural changes in mTBI and generally small study sample sizes, such inconsistency is not unexpected and necessitates additional exploration.
Despite the inconsistency in directional findings for DTI diffusion metrics following mTBI, several affected white matter pathways do exhibit some consistency. Changes or differences in FA and MD in the corpus callosum, anterior and posterior corona radiata, anterior and posterior thalamic radiations, superior and inferior longitudinal fasciculi, corticospinal tracts, and internal capsule, are commonly reported (40, 41, 53). Such consistency of reporting suggests that these white matter tracts may be particularly susceptible to the multiple mechanisms (focal injuries, shearing) that may result in an mTBI (40, 41, 53). Importantly, prior work related to major depressive disorder (54) and insomnia (55, 56), as well as sleep quality (57) and variability (58) in generally healthy populations, has consistently demonstrated correlations with FA, such that more negative outcomes (e.g., greater depressive symptoms, lower sleep quality, and increased variability) are associated with lower FA in these same tracts. Given the overlapping tracts identified on DTI following mTBI and those related to sleep quality and depression from other populations, it is likely that these tracts play an important role in post-mTBI symptom presentation. However, to date, there are no DTI-related findings specific to mTBI and sleep quality or depression.
The purpose of this study was to use DTI to correlate diffusion metrics with self-report indices of sleep quality and depression in individuals with a recent mTBI. This study is part of a larger, on-going project aimed at identifying structural and neural correlates of self-report, neurocognitive, and behavioral outcomes following mTBI. Here, we compared DTI metrics between individuals within a year of an mTBI and individuals with no self-reported history of mTBI. Additionally, we computed within-group correlations between diffusion metrics and self-report outcomes in the National Institute of Neurological Disorders and Stroke (NINDS) Common Data Elements (CDEs; http://www.commondataelements.ninds.nih.gov/) psychiatric and psychological status [e.g., depression; (59)] domain and self-reported sleep quality (60). We hypothesized, consistent with the view that mTBI reflects aspects of DAI (32–34), that FA would be lower, and MD, AD, and RD higher in the mTBI group than in the healthy controls. Further we hypothesized that this pattern would extend to symptom presentation, with greater post-mTBI depressive symptoms, and lower sleep quality associated with lower FA and greater MD, AD, and RD.
Materials and Methods
Participants
A total of 52 individuals (n = 34 with a history of a recent (within 12 months) mTBI; n = 18 healthy control individuals with no documented history of head trauma) participated in the present study. Participants were recruited from the Tucson metropolitan area via multiple methods including Internet advertisements, posted flyers, and referral through local emergency departments. The presence of mTBI was defined in agreement with the American Congress of Rehabilitation Medicine guidelines including (1) alteration of mental status related to specific head trauma lasting up to 24 h; (2) loss of consciousness <30 min; (3) post-traumatic amnesia lasting <1 day; and (4) initial Glasgow Coma Scale between 13 and 15 (61). To be eligible, participants were required to provide written documentation from a medical provider or other professional who either witnessed the injury or provided immediate treatment or care as a result of the injury.
All participants were right-handed and reported English as their first language. Individuals were not eligible to participate in this study in the presence of (1) any contraindications for MRI, (2) education <9th grade, (3) history of alcoholism or substance abuse, (4) colorblindness, or (5) lifetime history of DSM-IV Axis I disorder. Healthy control participants were additionally ineligible with any lifetime history of TBI or sport participation in concussion high-risk sports (e.g., football, rugby, boxing, ice hockey, wrestling, soccer, or martial arts) for longer than 1 month. All participants were compensated for their time. All study procedures were approved by The University of Arizona Institutional Review Board and the US Army Human Research Protections Office. All participants provided written informed consent prior to participation. Participant demographics are further summarized in Table 1.
Materials and Procedure
As part of a larger, on-going study, individuals in the current sample were evaluated at one of six pre-specified time points relative to their injury date: 2-weeks, 4-weeks, 3-months, 6-months, or 12-months post-injury (see Table 1). The purpose of the larger study is to examine structural and neural correlates of neuropsychological, behavioral, and self-reported outcomes over the first year following an mTBI. Individuals are included at only one of six time points and treated as exemplars of recovery at that time. We report here on a subset of the outcomes.
All participants underwent a comprehensive neuropsychological evaluation, including several self-report measures (described below), followed by a neuroimaging session that included diffusion-weighted imaging (DWI). Only a subset of the outcome measures are presented here. In addition to indices of depressive symptoms and sleep quality, we included NINDS CDEs related to global outcomes, post-mTBI symptom presentation, and perceived health-related quality of life, all of which may be impacted by depression and/or lower sleep quality.
Self-Report Outcomes
Glasgow Outcome Scale - Extended (GOS-E)
The GOS-E is a structured interview commonly used to assess overall disability and recovery following TBI (62). It is a core element of the NINDS CDEs for all levels of severity of TBI, including sport-related concussion. Scores on subscales for the GOS-E quantify disability in cognition, independence, employability, and social or community participation. These subscales are cumulatively reported as a single overall outcome, ranging from 1 (death) to 8 (upper good recovery). Reliability for the GOS-E is high [κ = 0.85; (62)].
Beck Depression Inventory-II (BDI-II)
The BDI-II is a self-administered survey requiring self-appraisal of mood over the preceding 2 weeks (63, 64). Increasing scores on the BDI-II are associated with increasing levels of depression symptoms. The BDI-II has high test-retest reliability (r > 0.9), as well as construct (vs. the original BDI) and moderate concurrent (vs. the State-Trait Anxiety Inventory Anxiety and Depression factors) validity (r > 0.68; (65, 66)). Previous work has demonstrated that individuals with both recent mTBIs and a history of mTBI report higher levels of depression and increased likelihood for depression by comparison to individuals without mTBI (22, 67–71).
Pittsburgh Sleep Quality Index (PSQI)
The PSQI is an 18-item self-report questionnaire yielding information about overall sleep quality, latency, duration, efficiency, disturbances, medication use, and the effects on daytime function (60). Better sleep quality is associated with lower scores, with scores of >5 indicating poor sleep and >8 indicating insomnia. Prior work has indicated good test-retest reliability (r > 0.80; (60)) and sensitivity to sleep disruption following mTBI (72, 73).
Satisfaction With Life Survey (SWLS)
The SWLS is a self-administered survey in which individuals assess current life satisfaction based on five questions. Questions are scored “Strongly Disagree” (1) to “Strongly Agree” (7) and summed with a maximum value of 35 (74). Higher scores indicate greater life satisfaction. The SWLS has demonstrated test-retest reliability [r > 0.80; (75)]. It is a basic element of the NINDS CDEs for concussion and mTBI (59). Previous research has indicated that individuals with prior mTBIs report greater life dissatisfaction (76, 77).
Rivermead Post-Concussion Symptom Questionnaire (RPCSQ)
The RPCSQ is a common post-mTBI assessment of symptom presentation and is a basic element of the NINDS CDEs for concussion and mTBI (59). Participants self-report the extent to which 16 symptoms currently affect them compared to preinjury-levels. Ratings range from “Not experienced at all” (0) and “No more of a problem” (1) to “A severe problem (4) (78). Previous analyses of the RPCSQ have identified a two-factor structure, such that the first three questions (RPQ3) are sensitive to acute injury symptoms while the final 13 (RPQ13) are sensitive to chronic symptoms. These two scales have good test-retest reliability (r > 0.70) and external validity [ρ > 0.60; (79)], and the RPQ is a significant predictor of 3-month outcomes (80).
Diffusion-Weighted Imaging
We acquired DWI data using single-shot echo planar imaging (EPI) (TE = 88 ms; TR = 9600 ms; acquisition matrix = 128 × 128; FOV: 256 × 256; slice thickness = 2 mm with no gap) on a Siemens Skyra 3.0 Tesla MRI machine (32-channel head coil; MAGNETO Skyra Siemens Healthcare). Images were acquired following a within-lab standardized process, including cross checks on image acquisition parameters at the time of scanning, with a single MRI technician overseeing all scanning procedures. Diffusion gradients were applied along 72 directions, with b = 1000 s/mm2 and six non-diffusion weighted images (b0). Preprocessing followed the standard pipeline available through FMRIB Software Library's [FSL; (81)], including EPI distortion correction using TOPUP (82), motion and eddy current distortion correction using eddy (83), skull-stripping with the brain extraction tool [BET; (84)], and diffusion tensor model fitting using DTIFIT (85). Output from DTIFIT includes separate images for FA, MD and three eigenvalues (λ1, λ2, λ3). λ1 is the axial diffusivity and radial diffusivity is the average of λ2 and λ3.
The four DTI-metric images (FA, MD, AD, and RD) were then nonlinearly registered to a standard template (FMRIB-58) followed by affine-alignment to standard space (MNI, 1 × 1 × 1 mm) using Tract-Based Spatial Statistics [TBSS; (86)]. A study-specific, averaged whole-brain skeletonized FA mask was created through TBSS (threshold = 0.2). Skeletonizing in this way reduces the number of voxels considered in statistical modeling by only including voxels near the center of white matter tracts.
Whole-brain voxel-wise statistical analysis was conducted via FSL's randomise using threshold-free cluster enhancement (87) with 5000 permutations. Significant voxels were identified as those with p < 0.05 after family-wise error rate (FWER) adjustment for multiple comparison. We tested between-group differences using a two-sample T-test for each of the DTI metrics (FA, MD, AD, RD), controlling for age, sex, and days post-injury. For the self-report measures, we fit within-group GLMs for each combination of measure and metric, controlling for age, sex, and days since injury (mTBI only). Within-group correlations for the relationships between DTI metrics and self-report outcome measures were examined.
A mask of all significant voxels was created for each of the DTI metrics. These masks were then used to compute the mean DTI-metric value for each participant, which was then extracted for post-hoc analyses. Mean DTI-metric values and self-report measures were scatter-plotted and the partial correlation coefficient, after controlling for age, sex, and days post-injury, was computed. Significant white matter voxels were anatomically identified using the Johns Hopkins University (JHU) ICBM-DTI-81 White-Matter Labels atlas (88).
Statistical Analyses
Between-group comparisons for continuous demographic characteristics and self-report measures were analyzed using a two-tailed T-test in R [v. 3.4.2; (89)]. The between-group gender and ethnicity comparisons were computed using a Chi-square test. Average values over all significant voxels were plotted in R as scatterplots with the relevant self-report outcomes using ggplot2 (90). For the healthy control participants, taking an inverse transformation of the BDI scores [)] and a square transformation (y = x2) of the SWLS scores was necessary to reduce skewness. No GOS-E data were collected for the healthy control participants, as this measure is specific to brain injury.
Results
Demographic and Self-Report Measures
Demographic characteristics and self-report outcomes are summarized in Table 1. No participants were active duty military; however, we did not query for Veteran status. The groups did not differ in age, height, weight, or gender. Brain injured participants reported a median number of mTBIs, including the one used for referral, of 2 (range: 1–4). Most individuals (n = 13) reported a sports-related mechanism of injury for the referring mTBI. The mTBI participants reported significantly more post-concussive symptoms, poorer sleep quality, and greater depressive symptoms than the healthy control participants. The majority of the mTBI participants (n = 23, 68%) reported a good recovery as assessed via the GOS-E.
Given the dynamic nature of post-mTBI recovery and the role that time since injury may play, we additionally report the means and standard deviations for the self-reported outcomes for each of the six distinct time points in Table 2. Given the small sample sizes across all of the post-mTBI groups, we do not report any between-group statistical comparisons at this time.
Diffusion Metrics
Whole-Brain Group Differences
After correcting for multiple comparisons, there were no statistically significant differences observed between the healthy control participants and those with a history of mTBI for any of the diffusion metrics at the whole brain level (a priori α = 0.05). However, there were voxels with a trend toward greater radial diffusivity in the mTBI participants than the healthy control participants (FWER 0.064 ≤ p ≤ 0.094; Supplementary Figures 1, 5, top row).
Correlation Between Diffusion Metrics and Self-Report Measures
There were no significant correlations within the healthy control group between any of the DTI metrics and any of the self-report outcomes. However, a positive trend association between AD and SWLS scores was observed (0.068 ≤ corrected p ≤ 0.099, Supplementary Figures 2, 5, second row). We found voxels with significant correlations in the mTBI participant group for the BDI-FA (corrected p < 0.05, Figures 1, 6A), BDI-MD (corrected p < 0.05, Figures 2, 6B), BDI-RD (corrected p < 0.05, Figures 3, 6C), PSQI-FA (corrected p < 0.05, Figures 4, 6D) and PSQI-RD (corrected p < 0.05, Figures 5, 6E) relationships. Finally, trend associations were observed for RPQ3-AD (0.093 ≤ corrected p ≤ 0.1, Supplementary Figures 3, 5, third row) and PSQI-MD (0.079 ≤ corrected p ≤ 0.1, Supplementary Figures 4, 5, bottom row). No statistically significant correlations were present in the mTBI group between any diffusion metric and the GOS-E, RPQ13, or SWLS scores.
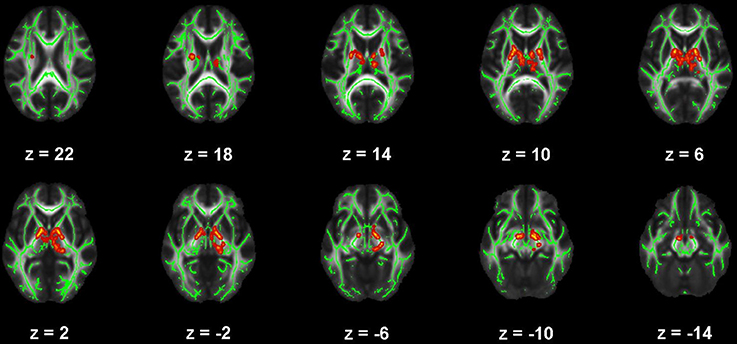
Figure 1. Map of voxels with significant correlations between fractional anisotropy (FA) and Beck Depression Inventory – II (BDI) total scores in the mild traumatic brain injury (mTBI) participants. The average white-matter skeleton is presented in green. Yellow voxels indicate significant, negative correlations between FA and BDI total score (family-wise error rate corrected p < 0.05). Surrounding voxels are filled in red for visual purposes only. Images are in neurological orientation and Z-coordinates are presented in MNI standard space.
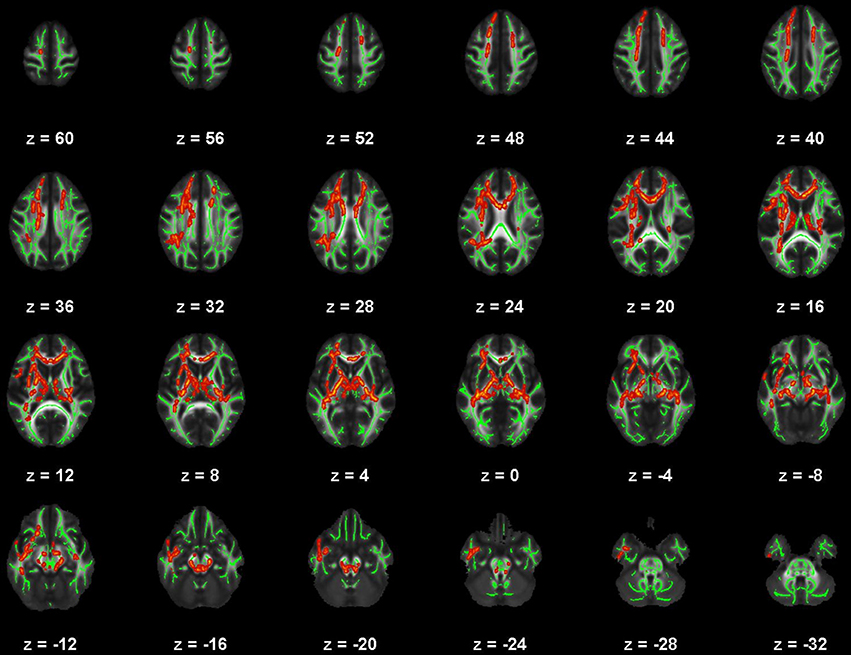
Figure 2. Map of voxels with significant correlations between radial diffusivity (RD) and Beck Depression Inventory – II (BDI) total scores in the mild traumatic brain injury (mTBI) participants. The average white-matter skeleton is presented in green. Yellow voxels indicate significant, positive correlations between RD and BDI total score (family-wise error rate corrected p < 0.05). Surrounding voxels are filled in red for visual purposes only. Images are in neurological orientation and Z-coordinates are presented in MNI standard space.
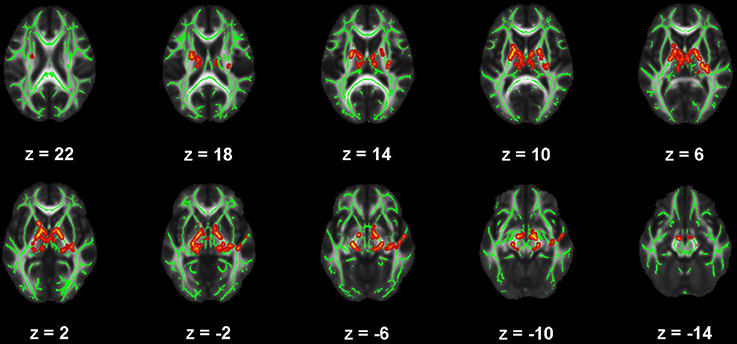
Figure 3. Map of voxels with significant correlations between mean diffusivity (MD) and Beck Depression Inventory – II (BDI) total scores in the mild traumatic brain injury (mTBI) participants. The average white-matter skeleton is presented in green. Yellow voxels indicate significant, positive correlations between MD and BDI total score (family-wise error rate corrected p < 0.05). Surrounding voxels are filled in red for visual purposes only. Images are in neurological orientation and Z-coordinates are presented in MNI standard space.
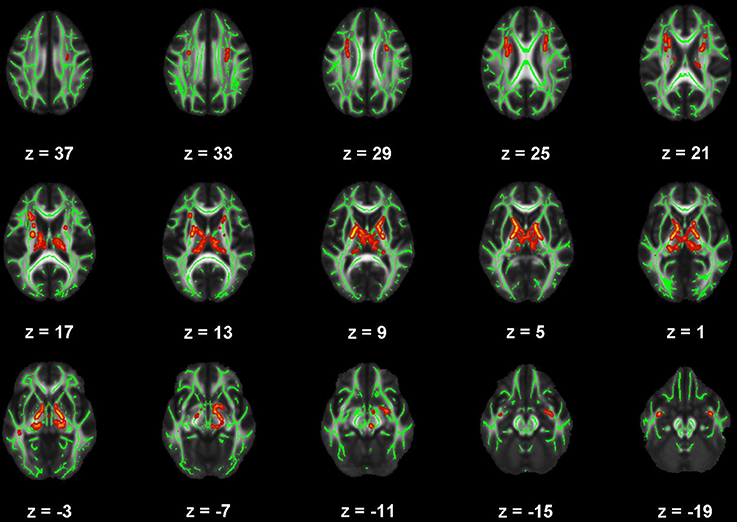
Figure 4. Map of voxels with significant correlations between fractional anisotropy (FA) and Pittsburgh Sleep Quality Index (PSQI) total scores in the mild traumatic brain injury (mTBI) participants. The average white-matter skeleton is presented in green. Yellow voxels indicate significant, negative correlations between FA and PSQI total score (family-wise error rate corrected p < 0.05). Surrounding voxels are filled in red voxels for visual purposes only. Images are in neurological orientation and Z-coordinates are presented in MNI standard space.
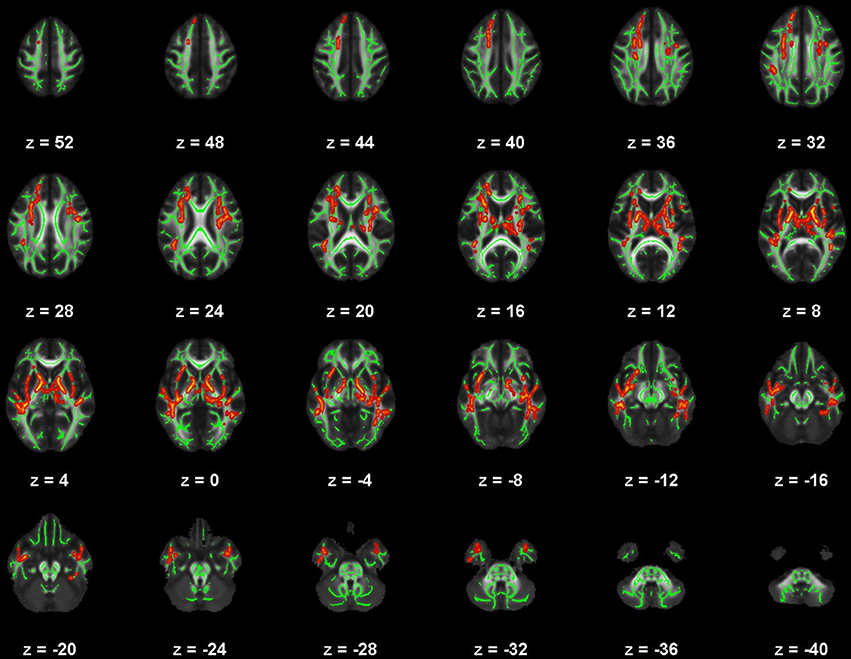
Figure 5. Map of voxels with significant correlations between radial diffusivity (RD) and Pittsburgh Sleep Quality Index (PSQI) total scores in the mild traumatic brain injury (mTBI) participants. The average white-matter skeleton is presented in green. Yellow voxels indicate significant, positive correlations between RD and PSQI total score (family-wise error rate corrected p < 0.05). Surrounding voxels are filled in red for visual purposes only. Images are in neurological orientation and Z-coordinates are presented in MNI standard space.
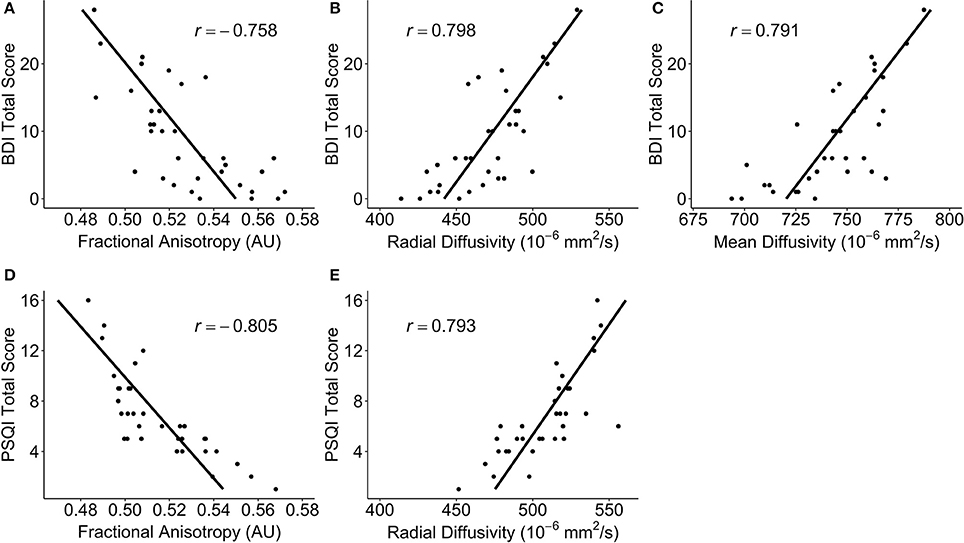
Figure 6. Scatterplots of post-hoc partial correlations between diffusivity measures and self-reported outcomes for participants with a mild traumatic brain injury. The points in each plot are the average DTI measure over all of the significant voxels. The black line is a regression line predicting the self-report outcomes from the diffusivity measures, controlling for age, sex, and days post-injury. Correlation coefficients are noted as r. (A) The Beck Depression Inventory – II (BDI) total score vs. fractional anisotropy (FA); (B) BDI total score vs. radial diffusivity (RD); (C) BDI total score vs. mean diffusivity (MD); (D) Pittsburgh Sleep Quality Index (PSQI) total score vs. FA; (E) PSQI total score vs. RD.
Anatomical locations of significant correlations were automatically determined using FSL's atlasquery function and the JHU ICBM-DTI-81 White-Matter Labels atlas (88). These locations are summarized in Figure 7 and the abbreviations detailed in Supplementary Table 1. Atlasquery returns the probability (and, in the case of the JHU ICBM-DTI-81 atlas, the proportion) of voxels in a mask belonging to a region identified in a given atlas. The JHU ICBM-DTI-81 atlas does not encompass all white matter, and consequently some voxels remain unclassified. Figure 7 has been rescaled to reflect only the classified voxels (e.g., 25% = 25% of the classified voxels). Across all five of the significant correlation pairs (BDI-FA, BDI-MD, BDI-RD, PSQI-FA, and PSQI-RD), correlations were consistently observed bilaterally within the internal capsules (anterior and posterior limbs), corona radiata (anterior and superior), fornix, and superior fronto-occipital fasciculi.
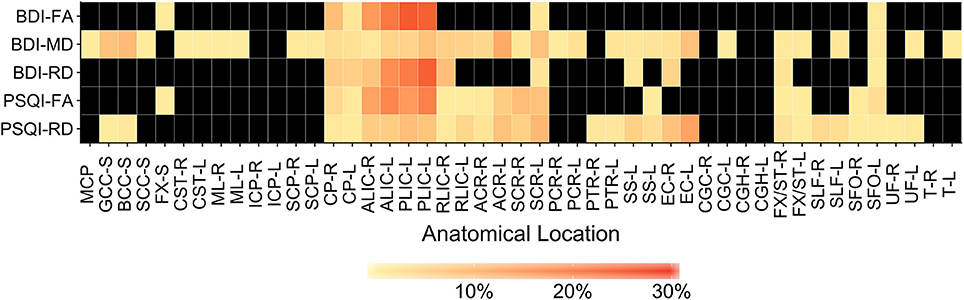
Figure 7. Heatmap showing distribution of labeled, significantly correlated voxels (family-wise error rate corrected p < 0.05) for each correlation pair. Anatomical labels are drawn from the JHU ICBM-DTI-81 White-Matter Labels atlas and retrieved using the FMRIB Software Library (FSL) atlasquery function. Atlasquery returns the probability (and, in the case of the JHU ICBM-DTI-81 atlas, the proportion) of voxels in a mask belonging to a region identified in a given atlas. The JHU ICBM-DTI-81 atlas does not encompass all white matter, and consequently some voxels remain unclassified. Colors reflect the percentage of labeled voxels identified within each anatomical location (. Black boxes indicate no voxels with a significant correlation were present in that anatomical location. BDI, Beck Depression Inventory; PSQI, Pittsburgh Sleep Quality Index; FA, Fractional Anisotropy; MD, Mean Diffusivity; RD, Radial Diffusivity. Anatomical location abbreviations are summarized in Supplementary Table 1.
Confirmatory Post-Hoc Analyses
We performed two additional post-hoc analyses to further strengthen these findings. To confirm the lack of PSQI-DTI and BDI-DTI correlation in the healthy controls, we conducted an ROI analysis. Using the significant voxel masks created for the mTBI participants, the healthy control participants' mean DTI-metric values were extracted in the same manner as for the mTBI participants. Post-hoc partial correlations for the healthy controls were computed as described above, controlling for age and sex. None of these post-hoc correlations were statistically significant, as anticipated based on the results from randomise. Additionally, we compared the partial correlation coefficients between the healthy control and mTBI participants (91). These analyses confirmed that the observed correlations in the mTBI participants were significantly different from those in the healthy controls (see Supplementary Table 2).
Additionally, the BDI-II includes two questions that specifically address sleep. We observed significant correlations between sleep quality (PSQI) and DTI metrics, as well as depressive symptoms (BDI) and the same DTI metrics (FA and RD). Additionally, there was overlap in the structural areas exhibiting significance. Consequently, it was important to examine whether the BDI-DTI correlations for the mTBI participants depended upon perceived sleep quality. We computed a modified BDI total score that ignored items 16 (changes in sleeping pattern) and 20 (tiredness or fatigue) and re-calculated the partial correlations between BDI and the mean FA, MD, and RD over the previously identified significant voxels, while controlling for age, sex and time since injury. We then compared the two sets of correlation coefficients (91). There were no significant differences between the original and adjusted BDI correlations (see Supplementary Table 2), suggesting that the BDI-FA, BDI-MD, and BDI-RD correlations were not necessarily dependent on self-reported sleep characteristics.
Discussion
The focus of this study was to identify neural correlates of clinically-relevant self-report outcomes related to global outcomes, psychiatric and psychological status, perceived health-related quality of life, and post-concussive related symptoms (59) as well as self-reported sleep quality (60). Specifically, the emphasis here was on metrics related to white-matter integrity, including FA as well as MD, AD, and RD. We hypothesized that individuals with a recent mTBI would exhibit lower FA and greater MD and RD than individuals with no prior history of mTBI. Additionally, we hypothesized that within-group correlations would be present such that poorer outcomes (e.g., poorer sleep quality, more depression symptoms) would be associated with lower FA and greater MD and RD. These hypotheses were partially confirmed.
DTI Sensitivity to mTBI
Contrary to our hypotheses, we did not observe any statistically significant differences between the healthy control and mTBI participants for any of the four diffusion metrics. This lack of difference occurred despite participants with mTBI reporting statistically greater sleep disturbances, depression symptoms, and post-concussive symptoms than the healthy controls. Recent systematic reviews and meta-analyses have generally highlighted the sensitivity of FA and MD to mTBI (40, 41, 53), however, the directional difference relative to non-mTBI participants is unclear, with lower, higher, and no differences reported (31, 43, 46, 48, 50–52). Our findings here are consistent with those of “no differences” at a whole brain corrected level, however it is important to note that generalization across DTI studies in mTBI is limited by cross-study heterogeneity in sample sizes, participant ages and populations, imaging protocols, and processing methods (40, 41). Please see Exploratory trends for a further discussion of group differences.
Correlations With Self-Report Outcomes
Consistent with our hypotheses, both sleep quality and depressive symptoms in the participants with mTBIs in the present study were correlated with DTI metrics. The relationships with depressive symptoms remained significant after removing sleep-related items from the BDI total score, suggesting that these were not driven by sleep issues per se. These measures exhibited negative correlations with FA and positive correlations with RD in projection and association tracts, including the internal capsule (IC), superior and anterior corona radiata (SCR, ACR), anterior and posterior thalamic radiations (ATR, PTR), and superior fronto-occipital fasciculus (SFO). Collectively, these white-matter tracts are integral aspects of neural circuits connecting deep brain structures, specifically the thalamus, parietal, and occipital cortical regions with frontal and prefrontal cortex areas. These connections not only play a critical role in sleep-wake regulation (thalamo-cortical circuits; (92–94), but also facilitate information processing, cognitive control, attention, executive function, and emotion regulation (95–97), all of which may be impaired following mTBI. Recent models of the neural basis of depression have further illustrated how alterations in the information processing supported by these prefrontal-posterior cortical/subcortical pathways (e.g., schema-guided attention, interpretation, and cognitive control processes) may bi-directionally interact with sleep quality to produce/maintain depressive symptoms (98). Consequently, damage in these pathways may precipitate the clinical presentation of mTBI, especially with respect to the correlated sleep and depression-related symptoms we observed in our sample.
There is substantial evidence that prior mTBI is associated with poor sleep quality, both self-reported (8, 12, 99, 100) as well as when measured via actigraphy (11, 101) and/or polysomnography (102). Poor sleep quality may manifest in numerous ways, including insomnia (8, 12), hypersomnia (10), pleiosomnia (11), and increased night-to-night sleep variability (101). Proposed mechanisms for sleep-wake disturbances following mTBI include reduced sleep-wake regulation neurotransmitter availability, specifically low hypocretin/orexin, and lower counts of wake-promoting neurons in the hypothalamus (103–105). To our knowledge, the findings here are the first to link sleep quality with white-matter integrity following mTBI, and suggest that there are likely overlapping relationships between these mechanisms.
The results reported here are consistent with findings from both depression- and sleep-related studies apart from mTBI [i.e., where sleep disturbance is thought to both promote, and be promoted by, underlying neural processing abnormalities in depression; (98)]. Lower FA in the SLF, IC, and corpus callosum are frequently observed in major depressive disorder (54). Additionally, individuals with poor sleep quality (57), increased sleep variability (58), and insomnia (55, 56) all exhibit lower FA, particularly in the IC, SLF, and thalamic radiations. Importantly, previous work has indicated that poor sleep quality is associated with lower FA and increased RD even in healthy individuals and can cause reduced myelination and limit oligodendrocyte precursor proliferation (57, 58). In light of mouse models indicating that mTBI can directly result in loss of myelination (35–38), it is unclear to what extent post-mTBI sleep quality leads to white-matter damage vs. trauma-induced white-matter damage leading to poor sleep. Regardless, white-matter damage in these pathways may explain overlapping presentations of poor sleep quality, psychological distress, and cognitive impairment typically associated with mTBI. Identifying the independent contributions of traumatic insult vs. sleep loss-induced alterations in white-matter remains an open area of investigation in this population.
Exploratory Trends
Surprisingly, and generally contrary to the bulk of the literature on mTBI and DTI, there were no statistically significant differences (at a whole brain FWER corrected p < 0.05 level) between the mTBI participants and healthy controls. We did, however, observe a trend toward greater RD in the mTBI participants (at a FWER corrected p < 0.1), primarily in the right hemisphere. While areas did not overlap exactly with the significant voxels from the mTBI group correlations, they do exist within the same pathways, particularly the corona radiata, longitudinal fasciculi, and the corpus callosum. While these differences do not meet the conventional level of significance, they do point to the possibility of myelin-related damage following mTBI.
Similarly, despite the observed relationships between FA/RD and both sleep quality and depressive symptoms, there were no statistically significant correlations (at a FWER corrected p < 0.05 level) between diffusion measures and post-concussive symptom presentation on the Rivermead Post-concussion Symptom Questionnaire. However, a negative trend did exist between AD and post-concussive symptoms on the RPQ3 (which identifies somatic symptoms; i.e., headaches, dizziness, nausea), such that lower AD was associated with greater symptom presentation. This result is in line with other recent findings (106), suggesting that somatic symptom presentation may be related to axonal damage.
Limitations
The present study indicates an association between diffusion metrics and self-report outcomes subsequent to mTBI, particularly with regard to sleep and depressive symptoms. However, a number of challenges remain. First, our overall sample was relatively small, which may contribute to the lack of statistically significant differences observed between healthy control participants and mTBI participants. Secondly, despite significant correlations between diffusion metrics and both sleep quality and depressive symptoms, the findings here present only a cross-sectional view of post-mTBI outcomes. Consequently, we cannot make any assertions about causation with respect to either the white matter integrity or self-report outcomes.
Third, our group had considerable heterogeneity of time since injury, ranging from 2-weeks to 12-months post injury. There is reasonable evidence that diffusion-related metrics may change over the weeks to months following an mTBI (40). Given the exclusively cross-sectional nature of our data, we addressed this potential limitation in the following ways. First, both our between-group and within-group models controlled for days since injury. Second, a post-hoc mTBI participant within-group correlation between the mean significant voxel values for PSQI and BDI-II scores reported earlier and time since injury revealed a non-significant correlation (r = 0.026, p = 0.146). Therefore, while intra-individual DTI-metrics may typically change over the course of mTBI recovery (40), the relationships between DTI measures, sleep quality, and depression we observed in the present sample appear independent of time since injury.
Finally, the white matter skeleton created during TBSS is based upon FA local maxima, generally near the midline of the white-matter tract (107). Thus, group differences between controls and mTBI participants may be present in non-maxima areas of the tracts, but these potential differences would not be detectable using the methods employed here. Finally, there are no established cutoffs, or reliable change indices, for DTI metrics after mTBI to identify whether the observed relationships reflect clinically meaningful changes in diffusion. Future work should address longitudinal outcomes, ideally with pre-injury DTI (though we recognize the inherent challenge in that), as well as machine-learning-based modeling methods (e.g., cross-validated logistic regression, classification trees) to identify discriminative post-mTBI changes in DTI metrics.
Conclusion
The results of this study contribute to a growing body of literature indicating that there are correlations between white-matter structure and clinical measures related to sleep quality and depression following mTBI. We have identified that the self-reported presentation of poor sleep quality and depressive symptoms following mTBI correlates with lower white-matter integrity in multiple areas of the brain involved in sleep-wake cycle and emotion regulation, in addition to information processing, cognitive control, attention, and executive function. Finally, trends in our data suggest that there may be alterations in white-matter structure that distinguish individuals with a history of mTBI from those without. Future work should emphasize identifying cutoff values in DTI metrics that provide clinically meaningful distinctions between individuals. Such findings will help not only to continue to increase what is known about mTBI pathophysiology and recovery, but will also help to guide best practices for the diagnosis and treatment of mTBI.
Author Contributions
AR conducted the MRI data processing, statistical analyses, and drafted the initial manuscript. SB, ND, RS, and AA assisted with data interpretation and manuscript revisions. BS assisted with manuscript revisions. WK designed the study, assisted with data interpretation and critique, as well as manuscript review and revisions.
Funding
This work was supported by a grant to WK from the Office of the Assistant Secretary of Defense for Health Affairs and the Defense Health Agency J9, Research and Development Directorate, through the US Army Medical Research and Materiel Command (USAMRMC, Award #W81XWH-12-0386). The opinions, interpretations, conclusions and recommendations in this paper are solely those of the authors and are not necessarily endorsed by the Department of Defense or the U.S. Army Medical Research and Materiel Command.
Conflict of Interest Statement
The authors declare that the research was conducted in the absence of any commercial or financial relationships that could be construed as a potential conflict of interest.
Supplementary Material
The Supplementary Material for this article can be found online at: https://www.frontiersin.org/articles/10.3389/fneur.2018.00468/full#supplementary-material
Supplementary Figure 1. Map of voxels with greater radial diffusivity (RD; family-wise error rate corrected 0.064 ≤ p ≤ 0.094) in mild traumatic brain injury (mTBI) participants compared to healthy control participants. The average white-matter skeleton is presented in green. Yellow voxels indicate voxels with a trend toward statistical significance. Surrounding voxels are filled with red for visual purposes only. Images are in neurological orientation and Z-coordinates are presented in MNI standard space.
Supplementary Figure 2. Map of voxels indicating a positive trend between axial diffusivity (AD) and Satisfaction with Life Scale (SWLS) total scores in the healthy control participants. The average white-matter skeleton is presented in green. Yellow voxels indicate positive correlations between AD and SWLS total score (family-wise error rate corrected 0.068 ≤ p ≤ 0.099). Surrounding voxels are filled in red for visual purposes only. Images are in neurological orientation and Z-coordinates are presented in MNI standard space.
Supplementary Figure 3. Map of voxels indicating a positive trend between mean diffusivity (MD) and Pittsburgh Sleep Quality Index (PSQI) total scores in the mild traumatic brain injury (mTBI) participants. The average white-matter skeleton is presented in green. Yellow voxels indicate positive correlations between MD and PSQI total score (family-wise error rate corrected 0.079 ≤ p ≤ 0.1). Surrounding voxels are filled in red for visual purposes only. Images are in neurological orientation and Z-coordinates are presented in MNI standard space.
Supplementary Figure 4. Map of voxels indicating a positive trend between axial diffusivity (AD) and Rivermead Post-concussion Symptom Questionnaire − 3 (RPQ3) scores in the mild traumatic brain injury (mTBI) participants. The average white-matter skeleton is presented in green. Yellow voxels indicate negative correlations between AD and RPQ3 total score (family-wise error rate corrected 0.093 ≤ p ≤ 0.1). Surrounding voxels are filled in red for visual purposes only. Images are in neurological orientation and Z-coordinates are presented in MNI standard space.
Supplementary Figure 5. Heatmap showing the distribution of labeled voxels (family-wise error rate corrected 0.05 < p ≤ 0.1). Anatomical labels are drawn from the JHU ICBM-DTI-81 White-Matter Labels atlas and retrieved using the FMRIB Software Library (FSL) atlasquery function. Atlasquery returns the probability (and, in the case of the JHU ICBM-DTI-81 atlas, the proportion) of voxels in a mask belonging to a region identified in a given atlas. The JHU ICBM-DTI-81 atlas does not encompass all white matter, and consequently some voxels remain unclassified. Colors reflect the percentage of labeled voxels identified within each anatomical location (. Black boxes indicate no voxels with a trend (family-wise error rate corrected 0.05 < p ≤ 0.1) were present in that anatomical location. BDI, Beck Depression Inventory; PSQI, Pittsburgh Sleep Quality Index; FA, Fractional Anisotropy; MD, Mean Diffusivity; RD, Radial Diffusivity. Anatomical location abbreviations are summarized in Supplementary Table 1.
Supplementary Table 1. JHU ICBM-DTI-81 White-Matter Labels atlas abbreviations.
Supplementary Table 2. Confirmatory post-hoc correlations.
References
1. Daneshvar DH, Nowinski CJ, McKee AC, Cantu RC. The epidemiology of sport-related concussion. Clin Sports Med. (2011) 30:1–17. doi: 10.1016/j.csm.2010.08.006
2. Langlois JA, Rutland-Brown W, Wald MM. The epidemiology and impact of traumatic brain injury: a brief overview. J Head Trauma Rehabil. (2006) 21:375–8. doi: 10.1097/00001199-200609000-00001
3. Defense Veterans Brain Injury Center DoD Worldwide Numbers for TBI. Available online at: http://dvbic.dcoe.mil/files/tbi-numbers/worldwide-totals-2000-2017_feb-14-2018_v1.0_2018-03-08.pdf (Accessed October 6, 2017).
4. Kerr ZY, Register-Mihalik JK, Kroshus E, Baugh CM, Marshall SW. Motivations associated with nondisclosure of self-reported concussions in former collegiate athletes. Am J Sports Med. (2016) 44:220–5. doi: 10.1177/0363546515612082
5. Finkelstein EA, Corso PS, Miller TR. Incidence and Economic Burden of Injuries in the United States. New York, NY: Oxford University Press (2006).
6. McCrory P, Meeuwisse W, Dvorak J, Aubry M, Bailes J, Broglio S, et al. Consensus statement on concussion in sport - The 5th international conference on concussion in sport held in Berlin, October 2016. Br J Sports Med. (2017) 51:838–47. doi: 10.1136/bjsports-2017-097699
7. Beetar JT, Guilmette TJ, Sparadeo FR. Sleep and pain complaints in symptomatic traumatic brain injury and neurologic populations. Arch Phys Med Rehabil. (1996) 77:1298–302. doi: 10.1016/S0003-9993(96)90196-3
8. Fichtenberg NL, Zafonte RD, Putnam S, Mann NR, Millard AE. Insomnia in a post-acute brain injury sample. Brain Inj. (2002) 16:197–206. doi: 10.1080/02699050110103940
9. Ouellet MC, Beaulieu-Bonneau S, Morin CM. Insomnia in patients with traumatic brain injury: frequency, characteristics, and risk factors. J Head Trauma Rehabil. (2006) 21:199–212. doi: 10.1097/00001199-200605000-00001
10. Billiard M, Podesta C. Recurrent hypersomnia following traumatic brain injury. Sleep Med. (2013) 14:462–5. doi: 10.1016/j.sleep.2013.01.009
11. Imbach LL, Valko PO, Li T, Maric A, Symeonidou ER, Stover JF, et al. Increased sleep need and daytime sleepiness 6 months after traumatic brain injury: a prospective controlled clinical trial. Brain (2015) 138:726–35. doi: 10.1093/brain/awu391
12. Ouellet MC, Morin CM. Subjective and objective measures of insomnia in the context of traumatic brain injury: a preliminary study. Sleep Med. (2006) 7:486–97. doi: 10.1016/j.sleep.2006.03.017
13. Jorge R, Robinson RG. Mood disorders following traumatic brain injury. NeuroRehabilitation (2002) 17:311–24. doi: 10.1080/09540260310001606700
14. Kreutzer JS, Seel RT, Gourley E. The prevalence and symptom rates of depression after traumatic brain injury: A comprehensive examination. Brain Inj. (2001) 15:563–76. doi: 10.1080/02699050010009108
15. Riemann D, Berger M, Voderholzer U. Sleep and depression — results from psychobiological studies: an overview. Biol Psychol. (2001) 57:67–103. doi: 10.1016/S0301-0511(01)00090-4
16. Kontos AP, Covassin T, Elbin RJ, Parker T. Depression and neurocognitive performance after concussion among male and female high school and collegiate athletes. Arch Phys Med Rehabil. (2012) 93:1751–6. doi: 10.1016/j.apmr.2012.03.032
17. Rapoport MJ, McCullagh S, Shammi P, Feinstein A. Cognitive impairment associated with major depression following mild and moderate traumatic brain injury. J Neuropsychiatry Clin Neurosci. (2005) 17:61–5. doi: 10.1176/jnp.17.1.61
18. Rock PL, Roiser JP, Riedel WJ, Blackwell AD. Cognitive impairment in depression: a systematic review and meta-analysis. Psychol Med. (2014) 44:2029–40. doi: 10.1017/S0033291713002535
19. Sufrinko A, Johnson EW, Henry LC. The influence of sleep duration and sleep-related symptoms on baseline neurocognitive performance among male and female high school athletes. Neuropsychology (2015) 30:484–91. doi: 10.1037/neu0000250
20. Thomas M, Sing H, Belenky G, Holcomb H, Mayberg H, Dannals R, et al. Neural basis of alertness and cognitive performance impairments during sleepiness. I. Effects of 24 h of sleep deprivation on waking human regional brain activity. J Sleep Res. (2000) 9:335–52. doi: 10.1046/j.1365-2869.2000.00225.x
21. Datta SGS, Pillai SV, Rao SL, Kovoor JME, Chandramouli BA. Post-concussion syndrome: Correlation of neuropsychological deficits, structural lesions on magnetic resonance imaging and symptoms. Neurol India (2009) 57:594–8. doi: 10.4103/0028-3886.57810
22. Chen JK, Johnston KM, Petrides M, Ptito A. Neural substrates of symptoms of depression following concussion in male athletes with persisting postconcussion symptoms. Arch Gen Psychiatry (2008) 65:81–9. doi: 10.1001/archgenpsychiatry.2007.8
23. Matthews SC, Strigo IA, Simmons AN, O'Connell RM, Reinhardt LE, Moseley SA. A multimodal imaging study in U.S. veterans of Operations Iraqi and Enduring Freedom with and without major depression after blast-related concussion. NeuroImage (2011) 54:S69–75. doi: 10.1016/j.neuroimage.2010.04.269
24. Budde MD, Xie M, Cross AH, Song SK. Axial diffusivity is the primary correlate of axonal injury in the experimental autoimmune encephalomyelitis spinal cord: A quantitative pixelwise analysis. J Neurosci. (2009) 29:2805–13. doi: 10.1523/JNEUROSCI.4605-08.2009
25. Song SK, Sun SW, Ju WK, Lin SJ, Cross AH, Neufeld AH. Diffusion tensor imaging detects and differentiates axon and myelin degeneration in mouse optic nerve after retinal ischemia. NeuroImage (2003) 20:1714–22. doi: 10.1016/j.neuroimage.2003.07.005
26. Song SK, Yoshino J, Le TQ, Lin SJ, Sun SW, Cross AH, et al. Demyelination increases radial diffusivity in corpus callosum of mouse brain. NeuroImage (2005) 26:132–40. doi: 10.1016/j.neuroimage.2005.01.028
27. Kasper E, Schuster C, Machts J, Kaufmann J, Bittner D, Vielhaber S, et al. Microstructural white matter changes underlying cognitive and behavioural impairment in ALS - An in vivo study using DTI. PLoS ONE (2014) 9:e114543. doi: 10.1371/journal.pone.0114543
28. Longoni G, Brown RA, MomayyezSiahkal P, Elliott C, Narayanan S, Bar-Or A, et al. White matter changes in paediatric multiple sclerosis and monophasic demyelinating disorders. Brain (2017) 140:1300–15. doi: 10.1093/brain/awx041
29. Ryan NP, Genc S, Beauchamp MH, Yeates KO, Hearps S, Catroppa C, et al. White matter microstructure predicts longitudinal social cognitive outcomes after paediatric traumatic brain injury: A diffusion tensor imaging study. Psychol Med. (2017) 48:679–91. doi: 10.1017/S0033291717002057
30. Ting WKC, Schweizer TA, Topolovec-Vranic J, Cusimano MD. Antisaccadic eye movements are correlated with corpus callosum white matter mean diffusivity, Stroop performance, and symptom burden in mild traumatic brain injury and concussion. Front Neurol. (2016) 6:271. doi: 10.3389/fneur.2015.00271
31. Wilde EA, Li X, Hunter JV, Narayana PA, Hasan K, Biekman B, et al. Loss of consciousness is related to white matter injury in mild traumatic brain injury. J Neurotrauma (2016) 33:2000–10. doi: 10.1089/neu.2015.4212
32. Browne KD, Chen XH, Meaney DF, Smith DH. Mild traumatic brain injury and diffuse axonal injury in swine. J Neurotrauma (2011) 28:1747–55. doi: 10.1089/neu.2011.1913
33. Kirov I, Tal A, Babb J, Lui Y, Grossman R, Gonen O. Diffuse axonal injury in mild traumatic brain injury: a 3D multivoxel proton MR spectroscopy study. J Neurol. (2013) 260:242–52. doi: 10.1007/s00415-012-6626-z
34. Lipton ML, Kim N, Park YK, Hulkower MB, Gardin TM, Shifteh K, et al. Robust detection of traumatic axonal injury in individual mild traumatic brain injury patients: Intersubject variation, change over time and bidirectional changes in anisotropy. Brain Imaging Behav. (2012) 6:329–42. doi: 10.1007/s11682-012-9175-2
35. Taib T, Leconte C, Steenwinckel JV, Cho AH, Palmier B, Torsello E, et al. Neuroinflammation, myelin and behavior: temporal patterns following mild traumatic brain injury in mice. PLoS ONE (2017) 12:e0184811. doi: 10.1371/journal.pone.0184811
36. Hall ED, Sullivan PG, Gibson TR, Pavel KM, Thompson BM, Scheff SW. Spatial and temporal characteristics of neurodegeneration after controlled cortical impact in mice: More than a focal brain injury. J Neurotrauma (2005) 22:252–65. doi: 10.1089/neu.2005.22.252
37. Hall ED, Bryant YD, Cho W, Sullivan PG. Evolution of post-traumatic neurodegeneration after controlled cortical impact traumatic brain injury in mice and rats as assessed by the de Olmos silver and fluorojade staining methods. J Neurotrauma (2008) 25:235–47. doi: 10.1089/neu.2007.0383
38. Mouzon B, Chaytow H, Crynen G, Bachmeier C, Stewart J, Mullan M, et al. Repetitive mild traumatic brain injury in a mouse model produces learning and memory deficits accompanied by histological changes. J Neurotrauma (2012) 29:2761–73. doi: 10.1089/neu.2012.2498
39. Mouzon BC, Bachmeier C, Ferro A, Ojo JO, Crynen G, Acker CM, et al. Chronic neuropathological and neurobehavioral changes in a repetitive mild traumatic brain injury model. Ann Neurol. (2014) 75:241–54. doi: 10.1002/ana.24064
40. Asken BM, DeKosky ST, Clugston JR, Jaffee MS, Bauer RM. Diffusion tensor imaging (DTI) findings in adult civilian, military, and sport-related mild traumatic brain injury (mTBI): A systematic critical review. Brain Imaging Behav. (2017) 12:585–612. doi: 10.1007/s11682-017-9708-9
41. Eierud C, Craddock RC, Fletcher S, Aulakh M, King-Casas B, Kuehl D, et al. Neuroimaging after mild traumatic brain injury: review and meta-analysis. NeuroImage Clin. (2014) 4:283–94. doi: 10.1016/j.nicl.2013.12.009
42. Hulkower MB, Poliak DB, Rosenbaum SB, Zimmerman ME, Lipton ML. A decade of DTI in traumatic brain injury: 10 years and 100 articles later. Am J Neuroradiol. (2013) 34:2064–74. doi: 10.3174/ajnr.A3395
43. Bazarian JJ, Zhu T, Blyth B, Borrino A, Zhong J. Subject-specific changes in brain white matter on diffusion tensor imaging after sports-related concussion. Magn Reson Imaging (2012) 30:171–80. doi: 10.1016/j.mri.2011.10.001
44. Inglese M, Makani S, Johnson G, Cohen BA, Silver JA, Gonen O, et al. Diffuse axonal injury in mild traumatic brain injury: a diffusion tensor imaging study. J Neurosurg. (2005) 103:298–303. doi: 10.3171/jns.2005.103.2.0298
45. Lipton ML, Gulko E, Zimmerman ME, Friedman BW, Kim M, Gellella E, et al. Diffusion-tensor imaging implicates prefrontal axonal injury in executive function impairment following very mild traumatic brain injury. Radiology (2009) 252:816–24. doi: 10.1148/radiol.2523081584
46. Meier TB, Bergamino M, Bellgowan PSF, Teague TK, Ling JM, Jeromin A, et al. Longitudinal assessment of white matter abnormalities following sports-related concussion. Hum Brain Mapp. (2016) 37:833–45. doi: 10.1002/hbm.23072
47. Messé A, Caplain S, Pélégrini-Issac M, Blancho S, Montreuil M, Lévy R, et al. Structural integrity and postconcussion syndrome in mild traumatic brain injury patients. Brain Imaging Behav. (2012) 6:283–92. doi: 10.1007/s11682-012-9159-2
48. Bazarian JJ, Zhong J, Blyth B, Zhu T, Kavcic V, Peterson D. Diffusion tensor imaging detects clinically important axonal damage after mild traumatic brain injury: a pilot study. J. Neurotrauma (2007) 24:1447–59. doi: 10.1089/neu.2007.0241
49. Dretsch MN, Lange RT, Katz JS, Goodman A, Daniel TA, Deshpande G, et al. Examining microstructural white matter in active duty soldiers with a history of mild traumatic brain injury and traumatic stress. Open Neuroimaging J. (2017) 11:46–57. doi: 10.2174/1874440001711010046
50. Veeramuthu V, Narayanan V, Kuo TL, Delano-Wood L, Chinna K, Bondi MW, et al. Diffusion tensor imaging parameters in mild traumatic brain injury and its correlation with early neuropsychological impairment: a longitudinal study. J Neurotrauma (2015) 32:1497–509. doi: 10.1089/neu.2014.3750
51. Wilde EA, McCauley SR, Hunter JV, Bigler ED, Chu Z, Wang ZJ, et al. Diffusion tensor imaging of acute mild traumatic brain injury in adolescents. Neurology (2008) 70:948–55. doi: 10.1212/01.wnl.0000305961.68029.54
52. Lancaster MA, Olson DV, McCrea MA, Nelson LD, LaRoche AA, Muftuler LT. Acute white matter changes following sport-related concussion: A serial diffusion tensor and diffusion kurtosis tensor imaging study. Hum Brain Mapp. (2016) 37:3821–34. doi: 10.1002/hbm.23278
53. Shenton ME, Hamoda HM, Schneiderman JS, Bouix S, Pasternak O, Rathi Y, et al. A review of magnetic resonance imaging and diffusion tensor imaging findings in mild traumatic brain injury. Brain Imaging Behav. (2012) 6:137–92. doi: 10.1007/s11682-012-9156-5
54. Murphy ML, Frodl T. Meta-analysis of diffusion tensor imaging studies shows altered fractional anisotropy occurring in distinct brain areas in association with depression. Biol Mood Anxiety Disord. (2011) 1:3. doi: 10.1186/2045-5380-1-3
55. Li S, Tian J, Bauer A, Huang R, Wen H, Li M, et al. Reduced integrity of right lateralized white matter in patients with primary insomnia: A diffusion-tensor imaging study. Radiology (2016) 280:520–8. doi: 10.1148/radiol.2016152038
56. Spiegelhalder K, Regen W, Prem M, Baglioni C, Nissen C, Feige B, et al. Reduced anterior internal capsule white matter integrity in primary insomnia. Hum Brain Mapp. (2014) 35:3431–8. doi: 10.1002/hbm.22412
57. Sexton CE, Zsoldos E, Filippini N, Griffanti L, Winkler A, Mahmood A, et al. Associations between self-reported sleep quality and white matter in community-dwelling older adults: a prospective cohort study. Hum Brain Mapp. (2017) 38:5465–73. doi: 10.1002/hbm.23739
58. Telzer EH, Goldenberg D, Fuligni AJ, Lieberman MD, Gálvan A. Sleep variability in adolescence is associated with altered brain development. Dev Cogn Neurosci. (2015) 14:16–22. doi: 10.1016/j.dcn.2015.05.007
59. Grinnon ST, Miller K, Marler JR, Lu Y, Stout A, Odenkirchen J, et al. National institute of neurological disorders and stroke common data element project – approach and methods. Clin Trials (2012) 9:322–9. doi: 10.1177/1740774512438980
60. Buysse DJ, Reynolds CF, Monk TH, Berman SR, Kupfer DJ. The pittsburgh sleep quality index: a new instrument for psychiatric practice and research. Psychiatry Res. (1989) 28:193–213. doi: 10.1016/0165-1781(89)90047-4
61. American Congress of Rehabilitation Medicine. Definition of mild traumatic brain injury. J. Head Trauma Rehabil. (1993) 8:86–87. doi: 10.1097/00001199-199309000-00010
62. Wilson JTL, Pettigrew LEK, Teasdale GM. Structured interviews for the glasgow outcome scale and the extended glasgow outcome scale: guidelines for their use. J Neurotrauma (1998) 15:573–85. doi: 10.1089/neu.1998.15.573
63. Beck AT, Epstein N, Brown G, Steer RA. An inventory for measuring clinical anxiety: Psychometric properties. J Consult Clin Psychol. (1988) 56:893. doi: 10.1037/0022-006X.56.6.893
64. Beck AT, Steer R, Brown G. Manual for The Beck Depression Inventory Second Edition (BDI-II). San Antonio, TX: Psycholoigcal Corporation (1996).
65. Beck AT, Steer RA, Ball R, Ranieri WF. Comparison of beck depression inventories-ia and-ii in psychiatric outpatients. J Pers Assess. (1996) 67:588–97. doi: 10.1207/s15327752jpa670313
66. Storch EA, Roberti JW, Roth DA. Factor structure, concurrent validity, and internal consistency of the beck depression inventory—second edition in a sample of college students. Depress Anxiety (2004) 19:187–9. doi: 10.1002/da.20002
67. Chrisman SPD, Richardson LP. Prevalence of diagnosed depression in adolescents with history of concussion. J Adolesc Health (2014) 54:582–6. doi: 10.1016/j.jadohealth.2013.10.006
68. Covassin T, Elbin RJ, Larson E, Kontos AP. Sex and age differences in depression and baseline sport-related concussion neurocognitive performance and symptoms. Clin J Sport Med. (2012) 22:98–104. doi: 10.1097/JSM.0b013e31823403d2
69. Strain J, Didehbani N, Cullum CM, Mansinghani S, Conover H, Kraut MA, et al. Depressive symptoms and white matter dysfunction in retired NFL players with concussion history. Neurology (2013) 81:25–32. doi: 10.1212/WNL.0b013e318299ccf8
70. Vanderploeg RD, Curtiss G, Luis CA, Salazar AM. Long-term morbidities following self-reported mild traumatic brain injury. J Clin Exp Neuropsychol. (2007) 29:585–98. doi: 10.1080/13803390600826587
71. Yang J, Peek-Asa C, Covassin T, Torner JC. Post-concussion symptoms of depression and anxiety in Division I collegiate athletes. Dev Neuropsychol. (2015) 40:18–23. doi: 10.1080/87565641.2014.973499
72. Mani A, Dastgheib SA, Chanor A, Khalili H, Ahmadzadeh L, Ahmadi J. Sleep quality among patients with mild traumatic brain injury: A cross-sectional study. Bull. Emerg. Trauma (2015) 3:93–6. doi: 10.1177/1545968315619697
73. Theadom A, Cropley M, Parmar P, Barker-Collo S, Starkey N, Jones K, et al. Sleep difficulties one year following mild traumatic brain injury in a population-based study. Sleep Med. (2015) 16:926–32. doi: 10.1016/j.sleep.2015.04.013
74. Diener E, Emmons RA, Larsen RJ, Griffin S. The satisfaction with life scale. J Pers Assess. (1985) 49:71–5. doi: 10.1207/s15327752jpa490113
75. Pavot W, Diener E. Review of the satisfaction with life scale. Psychol Assess. (1993) 5:164–72. doi: 10.1037/1040-3590.5.2.164
76. Dams-O'Connor K, Spielman L, Singh A, Gordon WA, Lingsma HF, Maas AIR, et al. The impact of previous traumatic brain injury on health and functioning: A TRACK-TBI study. J. Neurotrauma (2013) 30:2014–20. doi: 10.1089/neu.2013.3049
77. McMahon P, Hricik A, Yue JK, Puccio AM, Inoue T, Lingsma HF, et al. Symptomatology and functional outcome in mild traumatic brain injury: Results from the prospective TRACK-TBI study. J Neurotrauma (2014) 31:26–33. doi: 10.1089/neu.2013.2984
78. King NS, Crawford S, Wenden FJ, Moss NEG, Wade DT. The rivermead post concussion symptoms questionnaire: a measure of symptoms commonly experienced after head injury and its reliability. J Neurol. (1995) 242:587–92. doi: 10.1007/BF00868811
79. Eyres S, Carey A, Gilworth G, Neumann V, Tennant A. Construct validity and reliability of the rivermead post-concussion symptoms questionnaire. Clin Rehabil. (2005) 19:878–87. doi: 10.1191/0269215505cr905oa
80. Guise E, de Bélanger S, Tinawi S,erson K, LeBlanc J, Lamoureux J, et al. Usefulness of the rivermead postconcussion symptoms questionnaire and the trail-making test for outcome prediction in patients with mild traumatic brain injury. Appl Neuropsychol Adult (2016) 23:213–22. doi: 10.1080/23279095.2015.1038747
81. Smith SM, Jenkinson M, Woolrich MW, Beckmann CF, Behrens TEJ, Johansen-Berg H, et al. Advances in functional and structural MR image analysis and implementation as FSL. NeuroImage (2004) 23:S208–19. doi: 10.1016/j.neuroimage.2004.07.051
82. Andersson JLR, Skare S, Ashburner J. How to correct susceptibility distortions in spin-echo echo-planar images: application to diffusion tensor imaging. NeuroImage (2003) 20:870–88. doi: 10.1016/S1053-8119(03)00336-7
83. Andersson JLR, Sotiropoulos SN. An integrated approach to correction for off-resonance effects and subject movement in diffusion MR imaging. NeuroImage (2016) 125:1063–78. doi: 10.1016/j.neuroimage.2015.10.019
84. Smith SM. Fast robust automated brain extraction. Hum Brain Mapp. (2002) 17:143–55. doi: 10.1002/hbm.10062
85. Behrens TEJ, Woolrich MW, Jenkinson M, Johansen-Berg H, Nunes RG, Clare S, et al. Characterization and propagation of uncertainty in diffusion-weighted MR imaging. Magn Reson Med. (2003) 50:1077–88. doi: 10.1002/mrm.10609
86. Smith SM, Jenkinson M, Johansen-Berg H, Rueckert D, Nichols TE, Mackay CE, et al. Tract-based spatial statistics: voxelwise analysis of multi-subject diffusion data. NeuroImage (2006) 31:1487–05. doi: 10.1016/j.neuroimage.2006.02.024
87. Smith SM, Nichols TE. Threshold-free cluster enhancement: Addressing problems of smoothing, threshold dependence and localisation in cluster inference. NeuroImage (2009) 44:83–98. doi: 10.1016/j.neuroimage.2008.03.061
88. Oishi K, Zilles K, Amunts K, Faria A, Jiang H, Li X, et al. Human brain white matter atlas: Identification and assignment of common anatomical structures in superficial white matter. NeuroImage (2008) 43:447–57. doi: 10.1016/j.neuroimage.2008.07.009
89. R Core Team. R: A Language and Environment for Statistical Computing. Vienna: R Foundation for Statistical Computing (2016). Available online at: https://www.R-project.org/
91. Diedenhofen B, Musch J. cocor: A Comprehensive Solution for the Statistical Comparison of Correlations. PLoS ONE (2015) 10:e0121945. doi: 10.1371/journal.pone.0121945
92. Brown RE, Basheer R, McKenna JT, Strecker RE, McCarley RW. Control of sleep and wakefulness. Physiol Rev. (2012) 92:1087–187. doi: 10.1152/physrev.00032.2011
93. Larson-Prior LJ, Ju YE, Galvin JE. Cortical–subcortical interactions in hypersomnia disorders: mechanisms underlying cognitive and behavioral aspects of the sleep–wake cycle. Front Neurol. (2014) 5:165. doi: 10.3389/fneur.2014.00165
94. Steriade M. Corticothalamic resonance, states of vigilance and mentation. Neuroscience (2000) 101:243–76. doi: 10.1016/S0306-4522(00)00353-5
95. Asato MR, Terwilliger R, Woo J, Luna B. White matter development in adolescence: A DTI study. Cereb Cortex (2010) 20:2122–131. doi: 10.1093/cercor/bhp282
96. Schmahmann J, Pandya D. Fiber Pathways of the Brain. New York, NY: Oxford University Press (2009).
97. Sherman SM, Guillery RW. The role of the thalamus in the flow of information to the cortex. Philos Trans R Soc B Biol Sci. (2002) 357:1695–708. doi: 10.1098/rstb.2002.1161
98. Smith R, Alkozei A, Killgore WDS, Lane RD. Nested positive feedback loops in the maintenance of major depression: an integration and extension of previous models. Brain Behav Immun. (2018) 67:374–97. doi: 10.1016/j.bbi.2017.09.011
99. Gosselin N, Lassonde M, Petit D, Leclerc S, Mongrain V, Collie A, et al. Sleep following sport-related concussions. Sleep Med. (2009) 10:35–46. doi: 10.1016/j.sleep.2007.11.023
100. Kaufman Y, Tzischinsky O, Epstein R, Etzioni A, Lavie P, Pillar G. Long-term sleep disturbances in adolescents after minor head injury. Pediatr Neurol. (2001) 24:129–34. doi: 10.1016/S0887-8994(00)00254-X
101. Raikes AC, Schaefer SY. Sleep quantity and quality during acute concussion: A pilot study. SLEEP (2016) 39:2141–7. doi: 10.5665/sleep.6314
102. Imbach LL, Büchele F, Valko PO, Li T, Maric A, Stover JF, et al. Sleep–wake disorders persist 18 months after traumatic brain injury but remain underrecognized. Neurology (2016) 86:1945–9. doi: 10.1212/WNL.0000000000002697
103. Baumann CR, Stocker R, Imhof H, Trentz O, Hersberger M, Mignot E, et al. Hypocretin-1 (orexin A) deficiency in acute traumatic brain injury. Neurology (2005) 65:147–9. doi: 10.1212/01.wnl.0000167605.02541.f2
104. Baumann CR, Werth E, Stocker R, Ludwig S, Bassetti CL. Sleep-wake disturbances 6 months after traumatic brain injury: A prospective study. Brain (2007) 130:1873–83. doi: 10.1093/brain/awm109
105. Baumann CR, Bassetti CL, Valko PO, Haybaeck J, Keller M, Clark E, et al. Loss of hypocretin (orexin) neurons with traumatic brain injury. Ann Neurol. (2009) 66:555–9. doi: 10.1002/ana.21836
106. Mustafi SM, Harezlak J, Koch KM, Nencka AS, Meier T, West JD, et al. Acute white-matter abnormalities in sports-related concussion: a diffusion tensor imaging study from the NCAA-DoD CARE Consortium. J Neurotrauma. (2017). doi: 10.1089/neu.2017.5158. [Epub ahead of print].
Keywords: white matter integrity, Pittsburgh sleep quality index, beck depression inventory, fractional anisotropy, radial diffusivity, internal capsule, superior fronto-occipital fasciculus, corona radiata
Citation: Raikes AC, Bajaj S, Dailey NS, Smith RS, Alkozei A, Satterfield BC and Killgore WDS (2018) Diffusion Tensor Imaging (DTI) Correlates of Self-Reported Sleep Quality and Depression Following Mild Traumatic Brain Injury. Front. Neurol. 9:468. doi: 10.3389/fneur.2018.00468
Received: 20 December 2017; Accepted: 31 May 2018;
Published: 20 June 2018.
Edited by:
Denes V. Agoston, Karolinska Institutet (KI), SwedenReviewed by:
Ramon Diaz-Arrastia, University of Pennsylvania, United StatesRalph George Depalma, US Department of Veterans Affairs, United States
Copyright © 2018 Raikes, Bajaj, Dailey, Smith, Alkozei, Satterfield and Killgore. This is an open-access article distributed under the terms of the Creative Commons Attribution License (CC BY). The use, distribution or reproduction in other forums is permitted, provided the original author(s) and the copyright owner are credited and that the original publication in this journal is cited, in accordance with accepted academic practice. No use, distribution or reproduction is permitted which does not comply with these terms.
*Correspondence: Adam C. Raikes, cmFpa2VzLnJlc2VhcmNoQGdtYWlsLmNvbQ==