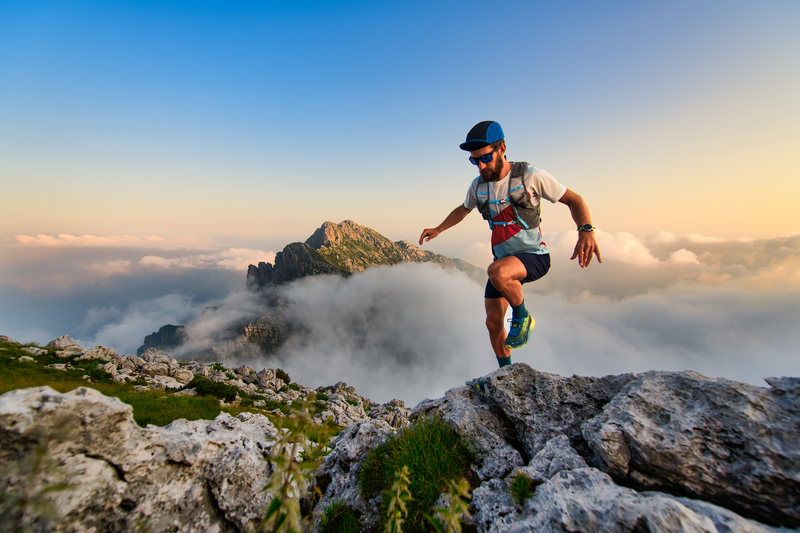
94% of researchers rate our articles as excellent or good
Learn more about the work of our research integrity team to safeguard the quality of each article we publish.
Find out more
CORRECTION article
Front. Comput. Neurosci. , 15 April 2020
Volume 14 - 2020 | https://doi.org/10.3389/fncom.2020.00028
This article is part of the Research Topic Understanding and Bridging the Gap between Neuromorphic Computing and Machine Learning View all 16 articles
This article is a correction to:
A Curiosity-Based Learning Method for Spiking Neural Networks
A Corrigendum on
A Curiosity-Based Learning Method for Spiking Neural Networks
by Shi, M., Zhang, T., and Zeng, Y. (2020). Front. Comput. Neurosci. 14:7. doi: 10.3389/fncom.2020.00007
In the original article, there was an error. In the original main text, there was an inaccurate statement sentence the result of NETalk in Table 3.
A correction has been made to Experiments, The validation of CBSNN on other datasets:
• NETtalk (Sejnowski and Rosenberg, 1987) is usually used for speech generation, consisting 5,033 training and 500 test. The input is a string of letters with fixed length of 7, which is encoded into 189 dimensions (each character has a 27 length one-hot vector). The output is 26 dimensions which represent 72 phonetic principles. For this mapping task with strong global regularities, VPSNN reaches 0.8680 accuracy. Although CBSNN is only slightly higher than VPSNN, it saves about half of the computation cost.
The authors apologize for this error and state that this does not change the scientific conclusions of the article in any way. The original article has been updated.
Keywords: curiosity, spiking neural network, novelty, STDP, voltage-driven plasticity-centric SNN
Citation: Shi M, Zhang T and Zeng Y (2020) Corrigendum: A Curiosity-Based Learning Method for Spiking Neural Networks. Front. Comput. Neurosci. 14:28. doi: 10.3389/fncom.2020.00028
Received: 21 February 2020; Accepted: 23 March 2020;
Published: 15 April 2020.
Edited and reviewed by: Huajin Tang, Zhejiang University, China
Copyright © 2020 Shi, Zhang and Zeng. This is an open-access article distributed under the terms of the Creative Commons Attribution License (CC BY). The use, distribution or reproduction in other forums is permitted, provided the original author(s) and the copyright owner(s) are credited and that the original publication in this journal is cited, in accordance with accepted academic practice. No use, distribution or reproduction is permitted which does not comply with these terms.
*Correspondence: Yi Zeng, eWkuemVuZ0BpYS5hYy5jbg==
†These authors have contributed equally to this work
Disclaimer: All claims expressed in this article are solely those of the authors and do not necessarily represent those of their affiliated organizations, or those of the publisher, the editors and the reviewers. Any product that may be evaluated in this article or claim that may be made by its manufacturer is not guaranteed or endorsed by the publisher.
Research integrity at Frontiers
Learn more about the work of our research integrity team to safeguard the quality of each article we publish.