- 1Center for Biomedical Image Computing and Analytics, Perelman School of Medicine, University of Pennsylvania, Philadelphia, PA, United States
- 2Department of Radiology, Perelman School of Medicine, University of Pennsylvania, Philadelphia, PA, United States
- 3Department of Pathology and Laboratory Medicine, Perelman School of Medicine, University of Pennsylvania, Philadelphia, PA, United States
- 4Division of Neurosurgery, Children Hospital of Philadelphia, Philadelphia, PA, United States
- 5Christiana Care Health System, Philadelphia, PA, United States
- 6Brigham and Women's Hospital, Boston, MA, United States
- 7Department of Biomedical Informatics, Northwestern University Feinberg School of Medicine, Chicago, IL, United States
- 8Department of Neurological Surgery, Weill Cornell Medicine, New York, NY, United States
- 9Department of Neurosurgery, Perelman School of Medicine, University of Pennsylvania, Philadelphia, PA, United States
Glioblastoma, the most frequent primary malignant brain neoplasm, is genetically diverse and classified into four transcriptomic subtypes, i. e., classical, mesenchymal, proneural, and neural. Currently, detection of transcriptomic subtype is based on ex vivo analysis of tissue that does not capture the spatial tumor heterogeneity. In view of accumulative evidence of in vivo imaging signatures summarizing molecular features of cancer, this study seeks robust non-invasive radiographic markers of transcriptomic classification of glioblastoma, based solely on routine clinically-acquired imaging sequences. A pre-operative retrospective cohort of 112 pathology-proven de novo glioblastoma patients, having multi-parametric MRI (T1, T1-Gd, T2, T2-FLAIR), collected from the Hospital of the University of Pennsylvania were included. Following tumor segmentation into distinct radiographic sub-regions, diverse imaging features were extracted and support vector machines were employed to multivariately integrate these features and derive an imaging signature of transcriptomic subtype. Extracted features included intensity distributions, volume, morphology, statistics, tumors' anatomical location, and texture descriptors for each tumor sub-region. The derived signature was evaluated against the transcriptomic subtype of surgically-resected tissue specimens, using a 5-fold cross-validation method and a receiver-operating-characteristics analysis. The proposed model was 71% accurate in distinguishing among the four transcriptomic subtypes. The accuracy (sensitivity/specificity) for distinguishing each subtype (classical, mesenchymal, proneural, neural) from the rest was equal to 88.4% (71.4/92.3), 75.9% (83.9/72.8), 82.1% (73.1/84.9), and 75.9% (79.4/74.4), respectively. The findings were also replicated in The Cancer Genomic Atlas glioblastoma dataset. The obtained imaging signature for the classical subtype was dominated by associations with features related to edge sharpness, whereas for the mesenchymal subtype had more pronounced presence of higher T2 and T2-FLAIR signal in edema, and higher volume of enhancing tumor and edema. The proneural and neural subtypes were characterized by the lower T1-Gd signal in enhancing tumor and higher T2-FLAIR signal in edema, respectively. Our results indicate that quantitative multivariate analysis of features extracted from clinically-acquired MRI may provide a radiographic biomarker of the transcriptomic profile of glioblastoma. Importantly our findings can be influential in surgical decision-making, treatment planning, and assessment of inoperable tumors.
Introduction
Glioblastoma is the most frequent primary malignant brain tumor with grim prognosis, despite aggressive combination of therapies (Stupp et al., 2017), and is characterized by inter- and intra-patient heterogeneity at radiographic, histologic, and molecular fronts, thereby providing opportunities for sub-classification, prognostication, and adoption of targeted therapeutic approaches (Aum et al., 2014; Lemée et al., 2015).
There is mounting evidence that different glioblastoma patients show different levels of sensitivity to therapeutic approaches depending on their distinct genetic characterization. It has been suggested earlier that glioblastoma should not be considered a single disease, but rather should be categorized into four transcriptomic subtypes, i.e., classical, mesenchymal, proneural, and neural (Verhaak et al., 2010). These subtypes present very distinct molecular biomarkers such as collective loss in chromosome 10 and amplification of chromosome 7 in classical subtype, largest occurrence of focal hemizygous deletions of a region at 17q11.2, encompassing NF1 gene, in mesenchymal subtype, aberrations in PDGFRA and mutations in IDH1 in proneural subtype, and presence of GABRA1, SYT1, NEFL, and SLC12A5 in neural subtype (Verhaak et al., 2010). In a recent study by Park et al. it has been shown that subtype-specific genetic aberrations have potential to serve as predictive markers and therapeutic targets (Park et al., 2019).
The determination of the molecular profile of the tumors leads to personalized diagnosis and treatment, as different treatment options may be considered depending on the characteristics of each subtype (Phillips et al., 2006; Verhaak et al., 2010; Bhat et al., 2011). Up until now, the assessment of transcriptomic subtypes was done via molecular profiling of surgical or biopsy tissue. However, such assessment has inherent limitations of: (i) tissue sampling error that sometimes leads to missing the tumor mutation, and (ii) inability to acquire multiple specimens over the course of the disease due to invasiveness of the tissue collection procedure, thereby leading to the failure in determining molecular subtype of the tumor over the course of the treatment.
Analysis of multi-parametric magnetic resonance imaging (mpMRI) data via advanced pattern analytics methods has been progressively shown to provide rich classifications of glioblastoma and its surrounding brain tissue, and has helped identifying relationships between MRI biomarkers and transcriptomic subtypes in gliomas (Gutman et al., 2013; Naeini et al., 2013; Gevaert et al., 2014; Pisapia et al., 2015; Macyszyn et al., 2016; Khened et al., 2019). For instance, the proneural subtype has shown lower levels of contrast enhancement; the mesenchymal subtype has presented lower levels of non-enhanced tumors and intensity in peritumoral edema region (Gutman et al., 2013); the classical subtype has associated necrosis and sharped edges of the edema region (Gevaert et al., 2014). A model to predict the mesenchymal subtype was also proposed (Naeini et al., 2013).
However, this classification scheme has been difficult to translate into clinical practice due to several complicating factors. First, existing literature has found associations between imaging features and individual subtypes (Naeini et al., 2013). Second, most studies to date have used basic imaging sequences only or have used very few hand-crafted imaging features, failing to leverage the power of computationally extracting and selecting imaging features (recently called radiomics), and analyzing them through advanced pattern analysis methods to build a more powerful predictive model (Gutman et al., 2013; Gevaert et al., 2014; Macyszyn et al., 2016). As literature increasingly acknowledges the tumor spatial and temporal heterogeneity, there is a parallel focus on extracting extensive features of the tumor and its surrounding peritumoral region toward providing a better characterization of patients. Furthermore, analysis of advanced mpMRI data can provide more details, which might not be available in conventional imaging.
This study aims to determine the transcriptomic subtypes of de novo glioblastoma patients by multivariately assessing imaging features from routine clinically-acquired scans, reflecting tumor biological properties such as angiogenesis, proliferation, cellularity, and peritumoral infiltration. Identifying these transcriptomic subtypes may allow enrollment of patients into targeted clinical trials, longitudinal profiling of the tumor, and assessment of treatment response.
Materials and Methods
Study Setting and Data Source
This study evaluates a group of 112 primary glioblastoma patients, diagnosed between 2006 and 2013 at the Hospital of the University of Pennsylvania (HUP), having pre-operative mpMRI (T1, T2, T1-Gd, T2-FLAIR). A subset of these patients (n = 89) had additional diffusion tensor imaging (DTI) and dynamic susceptibility contrast-enhanced (DSC) MRI imaging available. The proposed classification models were developed on n = 112 patients using conventional imaging only (T1, T2, T1-Gd, T2-FLAIR), whereas the subset of the patients (n = 89) was used to further analyze the imaging properties of different subtypes. The overall analysis was carried out on HUP dataset, and the findings were then replicated independently in The Cancer Genomic Atlas glioblastoma (TCGA-GBM) dataset (Clark et al., 2013; Scarpace et al., 2013) (n = 60), part of the International Brain Tumor Segmentation (BraTS) challenge dataset (Menze et al., 2015; Bakas et al., 2018), having the same set of pre-operative mpMRI. Expert manual segmentations for this dataset were downloaded from The Cancer Imaging Archive (TCIA) website (Bakas et al., 2017a,b). The study population was uniformly distributed and did not have any statistically significant difference based on clinical and demographical factors. All experiments were approved by the Institutional Review Board (IRB) of the University of Pennsylvania (approval no: 706564) and written informed consent was obtained from all patients. All experiments were carried out in accordance with the guidelines and regulations of the approved IRB.
Transcriptomic Subtyping
After pathologic confirmation of glioblastoma diagnosis, all tumors underwent subtyping into one of the four transcriptomic subtypes (classical = 21, mesenchymal = 31, proneural = 26, neural = 34). For this subtyping, we used an isoform-level assay classifier initially constructed using exon array data from glioblastoma samples in TCIA (Verhaak et al., 2010). It was then translated into a clinically applicable platform, where expression of desired transcripts was measured using reverse transcriptase–quantitative polymerase chain reaction (RT-qPCR) (Pal et al., 2014). RNA was isolated from the tissue samples using Tri Reagent (Sigma). A high-capacity complementary DNA reverse transcriptase kit (Applied Biosystems) was used to reverse-transcribe the RNA, and qPCR was then performed to designate the subtype. The assay was based on the expression of 121 transcripts with four housekeeping genes as controls.
Pre-processing Applied on the Dataset
All MRI of each patient were pre-processed using a series of image processing steps, including: (i) smoothing (i.e., reducing high frequency noise variations while preserving underlying anatomical structures) using Smallest Univalue Segment Assimilating Nucleus (SUSAN) denoising (Smith et al., 1997); (ii) correction for magnetic field inhomogeneity using N3 bias correction (Tustison et al., 2010); (iii) co-registration of all MRIs of each patient at 12-degrees of freedom for examining anatomically aligned signals at the voxel level using affine registration through the Linear Image Registration Tool (Jenkinson and Smith, 2001); (iv) skull stripping using the Brain Extraction Tool (Smith, 2002); and (v) matching of intensity profiles (histogram matching) of all MRIs of all patients to the corresponding MRIs of a reference patient.
Following the pre-processing, all tumors were segmented in distinct radiographic sub-regions of peritumoral edema region (ED), enhancing tumor (ET), and non-enhancing tumor (NET) (Figure 1) using a computational algorithm [namely GLISTRboost (Gooya et al., 2011; Bakas et al., 2016)]. The segmentations were assessed by two expert readers (H.A., G.S.) and revised before image analysis, when necessary. The segmentations were transformed into a standard atlas space to produce a standardized statistical distribution atlas for quantifying the tumor spatial location.
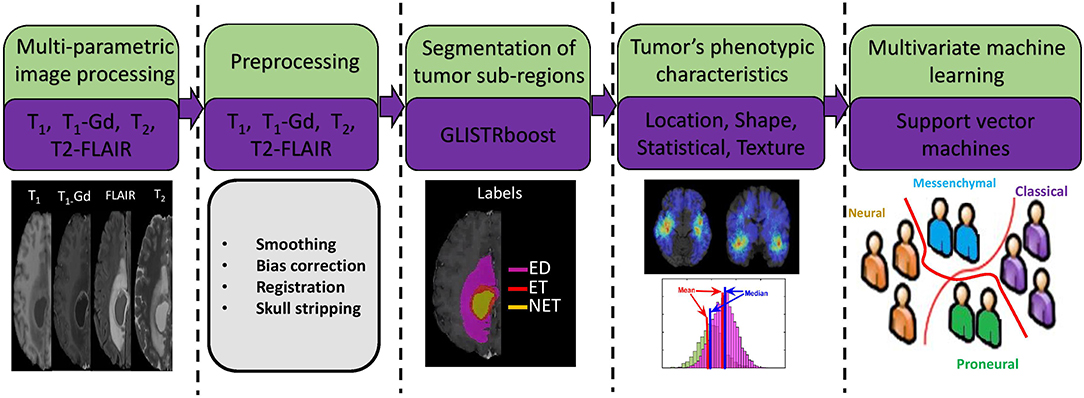
Figure 1. Image post-processing workflow. I. Preoperative imaging sequences (examples: T1-Gd, T2-FLAIR, T1, T2). II. Preprocessing. III. Segmentation of various subregions of tumor such as peritumoral edema region (ED), non-enhancing tumor core (NET), and enhancing tumor (ET). IV. Extraction of radiomic features in the segmented regions (ED, ET, NET) using all the imaging sequences. V. Multivariate machine learning model of support vector machines with 5-fold cross validation and sequential feature selection.
Radiophenotypic Tumor Characterization
The radiophenotypic characteristics of each tumor were quantified using a comprehensive and diverse set of imaging features, extracted from all tumor sub-regions (i.e., ED, ET, NET) and all MRI sequences using the Cancer Imaging Phenomics Toolkit (CaPTk) (Davatzikos et al., 2018). The feature set extracted to build the predictive model for this study comprised of (i) volumetric measurements, (ii) morphology parameters, (iii) location information, and (iv) statistical moments of the intensity distributions. The volumetric, location, and intensity statistics were calculated in 3D. The volumetric measurements include volume and surface area measurements of ED, NET, ET, tumor core (TC), which is the union of NET and ET, and whole tumor (WT), which is the combination of TC with ED. In addition, ratios of the volumes of the various tumor sub-regions and their union over the brain volume, were also calculated.
To capture the spatial distribution of each tumor, eight spatial distribution atlases were constructed as introduced in Akbari et al. (2018), two for each molecular subtype, i.e., P(+) and P(−) for proneural and non-proneural tumors, respectively. These distribution atlases were generated by superimposing the TC (ET+NET) segmentation labels of all patients according to their transcriptomic subtype status, i.e., superimposing the TC labels of proneural and non-proneural tumors. The similarity of the distribution pattern of an unseen tumor is then calculated by considering the intersection area between the tumor and the spatial map (Figure S1). Maximum and average frequency for each spatial distribution atlas in the intersected area are estimated, and four discrete relative values (L1, L2, L3, and L4) are used to evaluate any new unseen patient, for each subtype, thereby leading to a total of 16 location features.
Moreover, the distance of various tumor sub-regions, e.g., ED, TC, from the ventricles, and the proportions of TC in each lobe of the brain have also been utilized as additional location features. The proportion of TC in various brain regions, including temporal, frontal, parietal, occipital, basal ganglia, cc fornix, insula, cerebellum, and brain stem, was calculated by mapping each image to an atlas template via a deformable registration method (Gooya et al., 2011) that not only accounts for mass effect but also takes care of inter-individual anatomical variations (Kwon et al., 2014).
Furthermore, we used first-order statistical moments of intensity distributions to quantify the phenotypic characteristics of each tumor sub-region, along with second-order statistics that describe textural properties in tumor sub-regions. A gray-level co-occurrence matrix was calculated by considering the voxels within a radius of 1 and in the 13 main directions, and texture features of contrast, correlation, energy, and homogeneity were extracted. The intensity profiles of various sub-regions of tumor were also quantified using histograms. These histograms are reflective of the changes caused by the tumor both at functional and anatomical levels, which in turn change the corresponding imaging signals, and have shown strong association with various outcome of interest (Macyszyn et al., 2016; Rathore et al., 2018). Here, each intensity distribution is divided in to 5 bins and percentage of voxels in each bin are calculated.
Morphology parameters, comprising area, perimeter, extent, solidity, and length of major- and minor-axis, were extracted from one 2D slice per tumor. In order to pick the 2D slice for extraction of morphological features, we traversed the image in the axial direction and found the slice that had largest area of tumor core.
Feature Selection and Predictive Model Development
Support Vector Machines (SVM) (Chang and Lin, 2011), that has been extensively used in the past in medical image classification/segmentation (Lao et al., 2008; Haller et al., 2013), was used for predictive modeling in this study. We dealt the problem of classification as 4 one-vs. -rest classification problems. We trained a separate SVM to discriminate between one transriptomic subtype and the rest of the subtypes, such as classical vs. others, mesenchymal vs. others, neural vs. others, and proneural vs. others. To confirm the robustness of the method and to ensure that estimates of accuracy would be likely to generalize to new patients, we evaluated all classifiers through 5-fold cross-validation. In each iteration of the cross-validation, feature selection and classifier's parameters optimization was performed on the training folds and the resulting classification model, developed solely on the training folds, was applied on new/unseen test fold. Sequential forward feature selection was employed at each iteration until convergence, i.e., there was no improvement over a specific threshold. The final classification performance was obtained by combining the predictions of individual classifiers. For each classifier, the particular subtype was considered positive class and the rest of the subtypes were considered negative class. The distance of the sample from the hyperplane was noted for each classifier and highest distance was chosen as the final label of the sample. For example, if proneural, neural, mesenchymal, and classical have 0.45, 3.54, −2.43, and 5.32, then the classical label was assigned to the sample.
The classification performance of the proposed models was evaluated in terms of accuracy, balanced accuracy, sensitivity, and specificity. Sensitivity and specificity refer to the percentage of correctly classified samples of positive and negative classes, respectively. Balanced accuracy is the average of the proportion corrects of each class individually, whereas accuracy is the total proportion corrects of the population.
Statistical Analysis
The statistical analysis was performed with R (version 3.3.2, http://www.R-project.org), SPSS (version 25.0.0.0, IBM), and MatLab (version R2014b, Mathworks). For evaluation of statistically significant imaging features associated with each subtype, we used Kruskal-Wallis test (Chan et al., 1997).
Results
Performance of the Transcriptomic Subtype Prediction Model
The cross-validated accuracy and balanced accuracy [BA] of the obtained classifiers for classical, mesenchymal, proneural, and neural subtypes was 88.4% [BA: 81.9%], 75.9% [BA: 78.4%], 82.1% [BA: 78.9%], and 75.9% [BA: 76.9%], respectively. The overall 4-way classification among the four transcriptomic subtypes was 71% under 5-fold cross-validation experiment. Performance of the proposed prediction model is given in Table 1 where the first four columns show the result for binary classification, wherein each transcriptomic subtype is classified against the rest of the subtypes, and the last column shows the final 4-way classification accuracy obtained by combining the predictions of individual classifiers.
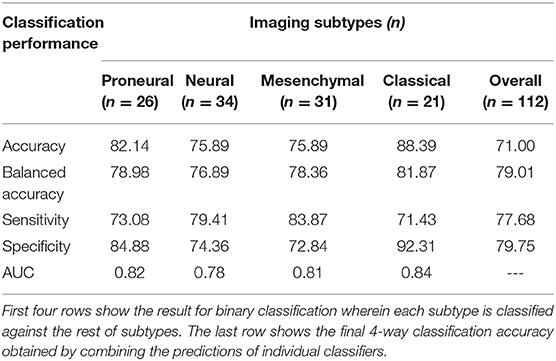
Table 1. Performance of the proposed transcriptomic subtype prediction model in terms of various performance measures.
Receiver-operating-characteristic (ROC) analysis on the given dataset yielded an area-under the-curve (AUC) of 0.82, 0.78, 0.81, and 0.84, for proneural, neural, mesenchymal, and classical subtypes, respectively (Figure 2).
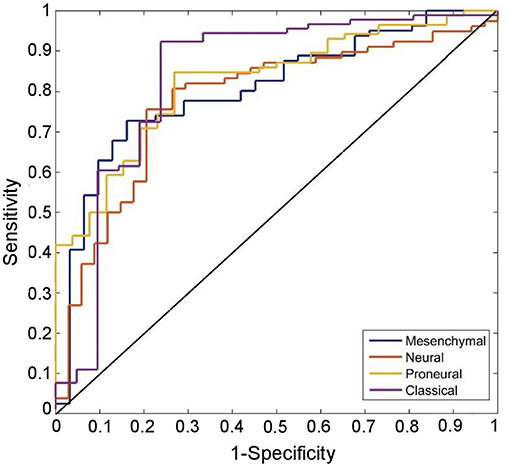
Figure 2. ROC curves of the predicted transriptomic subtypes are compared with chance (the diagonal line). ROC curves correctly classify proneural, neural, mesenchymal, and classical subtypes with 82.1% (sensitivity: 73.1, specificity = 84.9), 75.9% (sensitivity: 79.4, specificity = 74.4), 75.9% (sensitivity: 83.9, specificity = 72.8), and 88.4% (sensitivity: 71.4, specificity = 92.3) classification success rate, respectively.
Important Phenotypic Characteristics of Different Transcriptomic Subtypes
Along with evaluating the predictive performance of the model, we assessed individual features with the most predictive value. Our results have shown that specific subtypes have quite distinct quantitative imaging features, which can be utilized (Table 2, Figure 3). The main characteristics of the obtained imaging signature show that the mesenchymal subtype (in comparison with other subtypes) have lower T2 and T2-FLAIR signal in peritumoral edematous/invaded region, ET of lower eccentricity, NET of higher eccentricity, and higher volumes of ET, ED and WT. The proneural subtype, compared with the other subtypes, included signals of lower and uniform T1-Gd in ET. The neural subtype showed signals of higher T2-FLAIR in ED and lower eccentricity of NET, and the classical subtype showed smaller surface area of ED and WT.
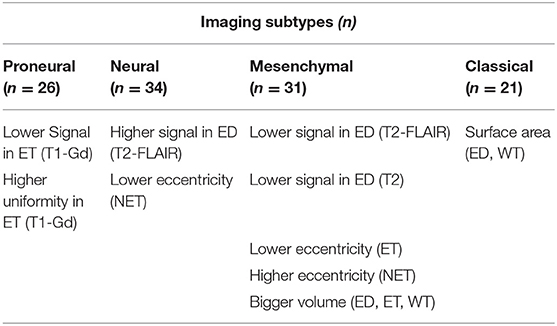
Table 2. Important imaging characteristics that distinguish each subtype from the rest of the subtypes.
Replication of the Proposed Model in TCIA Dataset
The predictive performance of the proposed model was also evaluated in an independent replication dataset of pre-operative glioblastoma patients, downloaded from TCIA (Bakas et al., 2017a), by applying the model trained on the discovery (i.e., HUP) dataset. The information about the molecular subtypes of TCIA patients was acquired from existing studies (Verhaak et al., 2010; Park et al., 2019). The four models, pertaining to four different molecular subtypes, trained on HUP dataset were applied to the patients in the replication (i.e., TCIA) cohort. The final molecular status of each patient in the replication dataset was obtained by combining the predictions of individual classifiers, as done in the discovery dataset, leading to 69% classification success rate compared to 25% chance in 4-way classification accuracy.
Discussion
We identified an in vivo radiographic signature of transcriptomic subtypes in glioblastoma by using quantitative multivariate analysis of mpMRI in a non-invasive manner, and further attempted to provide patho-physiological associations of the most distinctive imaging features. An important existing study has demonstrated the potential that deep learning techniques can be used for identifying associations between brain imaging phenotypes and genomic characteristics (Khened et al., 2019). The hereby proposed method is different from existing literature (Macyszyn et al., 2016; Khened et al., 2019) on the breadth of extracted mpMRI-based features, leading to an extensive radiographic signature. The proposed signature sheds light into the anatomical and pathological characteristics of the tumor, via macroscopic imaging features summarizing tumor characteristics related to water concentration, blood-brain barrier breakage, cell density, uniformity/heterogeneity, and geometric variations. We have achieved these findings utilizing routine mpMRI scans acquired under current clinical practice for glioblastoma, without the need to utilize any molecular imaging methods. We evaluated our model via a cross-validation mechanism in the HUP dataset, and also performed a multi-institutional validation to demonstrate generalizability. Potential applications of this signature include facilitating the assessment of transcriptomic status for patients with inadequate tissue. In a recent study by Park et al., it has been shown that subtype-specific genetic aberrations have potential to serve as predictive markers and therapeutic targets (Park et al., 2019). Therefore, in case of subtype-targeted clinical trials, it becomes very important to distinguish one particular subtype from the rest. The automatic distinction of these subtypes leads to personalized diagnosis and treatment, as different options may be considered depending on the histologic characteristics of different subtypes.
Biological Explanation of Quantitative Features of Different Subtypes
Toward gaining an understanding about the biological developments that induce different mutation status, we analyzed in isolation each individual feature that we used to develop our classification models. The analysis revealed that each subtype had an accompanying distinct and comprehensive set of radiographically relatable features (Table 2). The main findings from comparing the features of different transcriptomic subtypes, in ET, NET, and ED, are as follows:
1. Regions of lower and uniform T1-Gd signal in proneural subtype, suggestive of less blood–brain barrier compromise;
2. Areas of lower water content in mesenchymal subtype, reflected by T2-FLAIR and T2-weighted imaging, consistent with the characteristics of dense tissue;
3. Larger surface area of ED and WT in mesenchymal subtype, which points toward deep infiltration and migratory nature of the tumor;
4. Smaller surface area of ED and WT in classical subtype, supporting a radiographic phenotype of compact and less migratory nature of the tumor;
5. Major to minor axes ratios, associated with NET in neural subtype and ET/NET in mesenchymal subtype, were different from other subtypes (Table 2). The major axis was characterized by the longest possible 2D distance in a region; minor axis is vertical to the major axis. This eccentricity measure is suggestive of regular/spherical NET in neural subtype and irregular NET in mesenchymal subtype.
6. Regions of relatively lower contrast of T1 imaging sequence in ET in neural subtype, suggestive of more uniform T1 signal (Table 2).
It is important to note that despite several discriminative features, neither of these features is sufficient enough to predict transcriptomic subtype on each patient basis. However, synergistic integration of these features via appropriate machine learning yielded reasonable sensitivity and specificity in predicting subtype on an individual patient basis, thereby underscoring the potential of multivariate analysis methods.
Discriminative Power of Advanced MRI (DTI and DSC-MRI) Modalities
Advanced MRI sequences were evaluated to probe their discriminative power, compared to that of structural (conventional) imaging, i.e., T1, T2, T2-Flair, and T1-Gd. It is worth mentioning that these imaging sequences were not utilized to develop the classification models, rather only to analyze the diffusion and perfusion characteristics of a subset of these patients. These additional sequences comprised derivatives of DTI [i.e., fractional anisotropy (FA), apparent diffusion coefficient (ADC), radial diffusivity (RAD), axial diffusivity (AX)], as well as DSC-MRI derivatives, i.e., percentage signal recovery (PSR), peak height (PH), and relative cerebral blood volume (rCBV).
Imaging derivatives of DTI are reflective of the water diffusion process, which is partially affected by the architecture and density of tumor cells (Lu et al., 2003), in brain. The classical subtype has larger regions of lower ADC determined by the histograms (Figure 4) in NET (p = 2.27 × 10−08) and ET (p = 1.97 × 10−07) of the tumor, suggestive of less watery, and denser tumors. Imaging derivatives of DSC-MRI enumerate microvasculature and hemodynamics characteristics of the tumor (Wintermark et al., 2005; Tykocinski et al., 2012). When volume of brain tumors exceeds a certain critical limit, the consequential ischemia activates the discharge of angiogenic factors, which in turn endorses vascular proliferation and eventually leads to the formation of leaky and torturous tumor vessels (Lev and Hochberg, 1998; McDonald and Choyke, 2003; Bullitt et al., 2005; Hicklin et al., 2005; Essock-Burns et al., 2011; Thompson et al., 2011; Swami, 2013; Jensen et al., 2014). These imaging derivatives also steered toward some key findings. The classical subtype showed imaging features in agreement with highly vascular tumor, as shown by the PH in ET (p = 1.54 × 10−15) and NET (p = 4.00 × 10−06), revealing increased and compromised micro-vascularity compared to other subtypes. On the other hand, the proneural subtype had increased PSR in ET, indicative of lower micro-vascularity compared to other subtype.
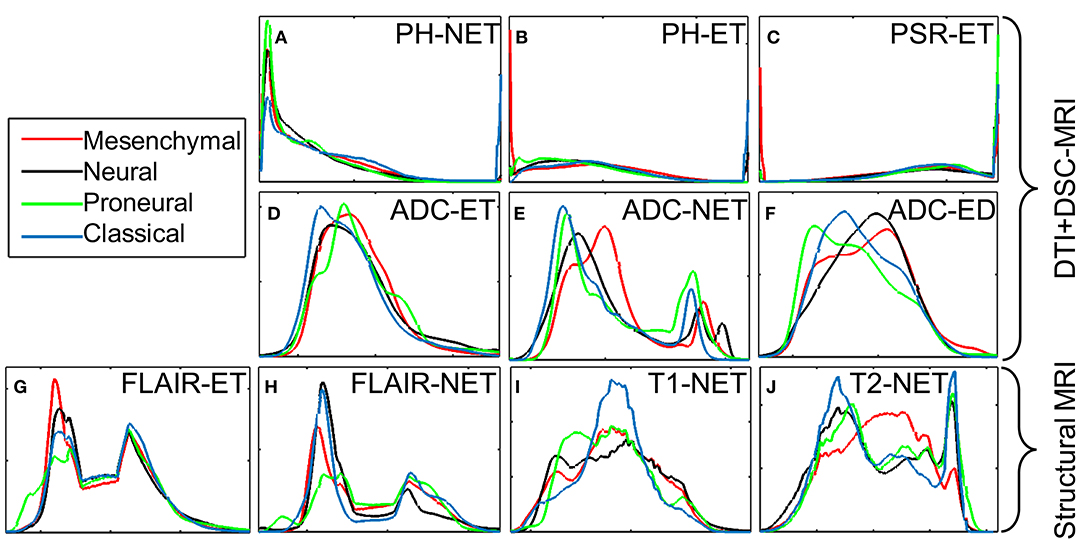
Figure 4. Intensity histograms display the diffusion and perfusion measures of different transcriptomic subtypes as measured by the DTI and DSC-MRI signals. (A) PH intensity in NET; (B) PH intensity in ET; (C) PSR intensity in ET; (D) ADC intensity in ET; (E) ADC intensity in NET; (F) ADC intensity in ED; (G) T2-Flair intensity in ET; (H) T2-Flair intensity in NET; (I) T1 intensity in NET; (J) T2 intensity in NET. The measures displayed in the first two rows are for analysis only; these measures have not been used for building the model.
Clinical Relevance and Impact
The assessment of transcriptomic subtype of glioblastoma via analysis of tissue specimen can be limited due to sampling error, and reluctance for longitudinal assessment of the status due to invasive nature of surgery. Our proposed imaging signature has potential to address both these limitations, since mpMRI facilitates assessment and monitoring of the tumor in its entirety in a repeatable manner. Further, the non-invasive imaging signature captures the heterogeneity of the whole tumor extent, instead of the analysis of one tissue specimen, therefore provides a global perspective of the transcriptomic status of a tumor. Our imaging signature is derivative of mpMRI that is routinely acquired for glioblastoma patients, therefore, is ready for immediate translation to the clinic. While the current method focuses on non-invasive assessment of transcriptomic subtype status, the same approach could also be used for molecular assessment in general. Further, the proposed non-invasive imaging signature can be applied to recurrent glioblastoma, with the goal of determining transcriptomic subtype status before, during, and after the treatment. This would help in non-invasive monitoring of dynamic changes in transcriptomic subtypes as response to targeted therapeutic approaches and consequently would in turn allow for tailoring the adopted therapies.
Conclusion
We can quantify important imaging characteristics within various sub-regions of the tumor and detect its transcriptomic subtype only by examining mpMRI data using advanced analytical methods and without the need of advanced genetic testing. The present study extracts an extensive set of quantitative imaging phenomic features from structural MRI sequences, and employs these variables via machine learning techniques to non-invasively distinguish transcriptomic glioblastoma subtypes. This molecular classification, due to its distinct phenotypic pattern derived from routine MRI, renders our imaging signature of increased likelihood for effective and immediate translation into clinical practice. The use of cross-validation within HUP dataset and the replication of our findings on TCIA dataset provide confidence in the generalizability of these subtypes and the proposed method on other datasets.
Data Availability Statement
Publicly available datasets were analyzed in this study. This data can be found here: TCGA.
Ethics Statement
The studies involving human participants were reviewed and approved by the Institutional Review Board (IRB) of University of Pennsylvania (approval no: 706564). The patients/participants provided their written informed consent to participate in this study.
Author Contributions
SR, HA, SB, and CD: conceptualization. SR: methodology, software, formal analysis, and writing—original draft. HA, SB, JP, GS, JR, XD, RD, ND, DO'R, and CD: validation. SR and CD: resources. SR, RD, and ND: data curation. SR, HA, SB, JP, GS, JR, XD, RD, ND, DO'R, and CD: writing—review and editing. CD: supervision and funding acquisition.
Funding
This research was funded by National Institutes of Health Grants (R01-NS042645, U24-CA189523) and Abramson Cancer Center, Hospital of the University of Pennsylvania Grant (ACC 040-0427-4-572593-xxxx-2433-8348).
Conflict of Interest
The authors declare that the research was conducted in the absence of any commercial or financial relationships that could be construed as a potential conflict of interest.
Supplementary Material
The Supplementary Material for this article can be found online at: https://www.frontiersin.org/articles/10.3389/fncom.2019.00081/full#supplementary-material
References
Akbari, H., Bakas, S., Pisapia, J. M., Nasrallah, M. P., Rozycki, M., Martinez-Lage, M., et al. (2018). In vivo evaluation of EGFRvIII mutation in primary glioblastoma patients via complex multiparametric MRI signature. Neuro-Oncology 20, 1068–1079. doi: 10.1093/neuonc/noy033
Aum, D. J., Kim, D. H., Beaumont, T. L., Leuthardt, E. C., Dunn, G. P., Kim, A., et al. (2014). Molecular and cellular heterogeneity: the hallmark of glioblastoma. Neurosurg. Focus 37:E11. doi: 10.3171/2014.9.FOCUS14521
Bakas, S., Akbari, H., Sotiras, A., Bilello, M., Rozycki, M., Kirby, J., et al. (2017b). Segmentation labels and radiomic features for the pre-operative scans of the TCGA-GBM collection. Cancer Imaging Arch. doi: 10.7937/K9/TCIA.2017.KLXWJJ1Q
Bakas, S., Akbari, H., Sotiras, A., Bilello, M., Rozycki, M., Kirby, J. S., et al. (2017a). Advancing the cancer genome atlas glioma MRI collections with expert segmentation labels and radiomic features. Scient. Data 4:170117. doi: 10.1038/sdata.2017.117
Bakas, S., Reyes, M., and Jakab, A. (2018). Identifying the best machine learning algorithms for brain tumor segmentation, progression assessment, and overall survival prediction in the BRATS challenge. arXiv:1811.02629. [Preprint].
Bakas, S., Zeng, K., Sotiras, A., Rathore, S., Akbari, H., Gaonkar, B., et al. (2016). GLISTRboost: combining multimodal MRI segmentation, registration, and biophysical tumor growth modeling with gradient boosting machines for glioma segmentation. Brainlesion 9556, 144–155. doi: 10.1007/978-3-319-30858-6_13
Bhat, K. P., Salazar, K. L., Balasubramaniyan, V., Wani, K., Heathcock, L., Hollingsworth, F., et al. (2011). The transcriptional coactivator TAZ regulates mesenchymal differentiation in malignant glioma. Genes Dev. 25, 2594–2609. doi: 10.1101/gad.176800.111
Bullitt, E., Zeng, D., Gerig, G., Aylward, S., Joshi, S., Smith, J. K., et al. (2005). Vessel tortuosity and brain tumor malignancy: a blinded study1. Acad. Radiol. 12, 1232–1240. doi: 10.1016/j.acra.2005.05.027
Chan, Y., and Walmsley, R. P. (1997). Learning and understanding the Kruskal-Wallis one-way analysis-of-variance-by-ranks test for differences among three or more independent groups. Phys. Ther. 77, 1755–1762. doi: 10.1093/ptj/77.12.1755
Chang, C.-C., and Lin, C.-J. (2011). LIBSVM: a library for support vector machines. ACM Trans. Intell. Syst. Technol. 2:27. doi: 10.1145/1961189.1961199
Clark, K., Vendt, B., Smith, K., Freymann, J., Kirby, J., Koppel, P., et al. (2013). The cancer imaging archive (TCIA): maintaining and operating a public information repository. J. Digit. Imaging 26, 1045–1057. doi: 10.1007/s10278-013-9622-7
Davatzikos, C., Rathore, S., Bakas, S., Pati, S., Bergman, M., Kalarot, R., et al. (2018). Cancer imaging phenomics toolkit: quantitative imaging analytics for precision diagnostics and predictive modeling of clinical outcome. J. Med. Imaging 5:011018. doi: 10.1117/1.JMI.5.1.011018
Essock-Burns, E., Lupo, J. M., Cha, S., Polley, M.-Y., Butowski, N. A., Chang, S. M., et al. (2011). Assessment of perfusion MRI-derived parameters in evaluating and predicting response to antiangiogenic therapy in patients with newly diagnosed glioblastoma. Neuro-oncology 13, 119–131. doi: 10.1093/neuonc/noq143
Gevaert, O., Mitchell, L. A., Achrol, A. S., Xu, J., Echegaray, S., Steinberg, G. K., et al. (2014). Glioblastoma multiforme: exploratory radiogenomic analysis by using quantitative image features. Radiology 273, 168–174. doi: 10.1148/radiol.14131731
Gooya, A., Biros, G., and Davatzikos, C. (2011). Deformable registration of glioma images using EM algorithm and diffusion reaction modeling. IEEE Trans. Med. Imaging 30, 375–390. doi: 10.1109/TMI.2010.2078833
Gutman, D. A., Cooper, L. A., Hwang, S. N., Holder, C. A., Gao, J., Aurora, T. D., et al. (2013). MR imaging predictors of molecular profile and survival: multi-institutional study of the TCGA glioblastoma data set. Radiology 267, 560–569. doi: 10.1148/radiol.13120118
Haller, S., Missonnier, P., Herrmann, F., Rodriguez, C., Deiber, M., Nguyen, D., et al. (2013). Individual classification of mild cognitive impairment subtypes by support vector machine analysis of white matter DTI. Am. J. Neuroradiol. 34, 283–291. doi: 10.3174/ajnr.A3223
Hicklin, D. J., and Ellis, L. M. (2005). Role of the vascular endothelial growth factor pathway in tumor growth and angiogenesis. J. Clin. Oncol. 23, 1011–1027. doi: 10.1200/JCO.2005.06.081
Jenkinson, M., and Smith, S. (2001). A global optimisation method for robust affine registration of brain images. Med. Image Anal. 5, 143–156. doi: 10.1016/S1361-8415(01)00036-6
Jensen, R. L., Mumert, M. L., Gillespie, D. L., Kinney, A. Y., Schabel, M. C., Salzman, K., et al. (2014). Preoperative dynamic contrast-enhanced MRI correlates with molecular markers of hypoxia and vascularity in specific areas of intratumoral microenvironment and is predictive of patient outcome. Neuro-oncology 16, 280–291. doi: 10.1093/neuonc/not148
Khened, M., Anand, V. K., Acharya, G., Shah, N., and Krishnamurthi, G. (2019). “3D convolution neural networks for molecular subtype prediction in glioblastoma multiforme,” in SPIE Medical Imaging (San Diego, CA), 10954.
Kwon, D., Shinohara, R. T., Akbari, H., and Davatzikos, C. (2014). “Combining generative models for multifocal glioma segmentation and registration,” in International Conference on Medical Image Computing and Computer-Assisted Intervention, Vol. 2014 (Boston, MA), 763–770. doi: 10.1007/978-3-319-10404-1_95
Lao, Z., Shen, D., Liu, D., Jawad, A. F., Melhem, E. R., Launer, L. J., et al. (2008). Computer-assisted segmentation of white matter lesions in 3D MR images, using support vector machine. Acad. Radiol. 15, 300–313. doi: 10.1016/j.acra.2007.10.012
Lemée, J.-M., Clavreul, A., and Menei, P. (2015). Intratumoral heterogeneity in glioblastoma: don't forget the peritumoral brain zone. Neuro Oncol. 17, 1322–1332. doi: 10.1093/neuonc/nov119
Lev, M. H., and Hochberg, F. (1998). Perfusion magnetic resonance imaging to assess brain tumor responses to new therapies. Cancer Control 5, 115–123. doi: 10.1177/107327489800500202
Lu, S., Ahn, D., Johnson, G., and Cha, S. (2003). Peritumoral diffusion tensor imaging of high-grade gliomas and metastatic brain tumors. Am. J. Neuroradiol. 24, 937–941.
Macyszyn, L., Akbari, H., Pisapia, J. M., Da, X., Attiah, M., Pigrish, V., et al. (2016). Imaging patterns predict patient survival and molecular subtype in glioblastoma via machine learning techniques. Neuro-oncology 18, 417–425. doi: 10.1093/neuonc/nov127
McDonald, D. M., and Choyke, P. L. (2003). Imaging of angiogenesis: from microscope to clinic. Nat. Med. 9, 713–725. doi: 10.1038/nm0603-713
Menze, B. H., Jakab, A., Bauer, S., Kalpathy-Cramer, J., Farahani, K., Kirby, J., et al. (2015). The multimodal brain tumor image segmentation benchmark (BRATS). IEEE Trans. Med. Imaging 34, 1993–2024. doi: 10.1109/TMI.2014.2377694
Naeini, K. M., Pope, W. B., Cloughesy, T. F., Harris, R. J., Lai, A., Eskin, A., et al. (2013). Identifying the mesenchymal molecular subtype of glioblastoma using quantitative volumetric analysis of anatomic magnetic resonance images. Neuro-oncology 15, 626–634. doi: 10.1093/neuonc/not008
Pal, S., Bi, Y., Macyszyn, L., Showe, L. C., O'Rourke, D. M., Davuluri, R., et al. (2014). Isoform-level gene signature improves prognostic stratification and accurately classifies glioblastoma subtypes. Nucleic Acids Res. 42:e64. doi: 10.1093/nar/gku121
Park, A. K., Kim, P., Ballester, L. Y., Esquenazi, Y., and Zhao, Z. (2019). Subtype-specific signaling pathways and genomic aberrations associated with prognosis of glioblastoma. Neuro-oncology 21, 59–70. doi: 10.1093/neuonc/noy120
Phillips, H. S., Kharbanda, S., Chen, R., Forrest, W. F., Soriano, R. H., Wu, T. D., et al. (2006). Molecular subclasses of high-grade glioma predict prognosis, delineate a pattern of disease progression, and resemble stages in neurogenesis. Cancer Cell 9, 157–173. doi: 10.1016/j.ccr.2006.02.019
Pisapia, J. M., Macyszyn, L., Akbari, H., Da, X., Pigrish, V., Attiah, M. A., et al. (2015). 135 imaging patterns predict patient survival and molecular subtype in glioblastoma using machine learning techniques. Neurosurgery 62(Suppl. 1):209. doi: 10.1227/01.neu.0000467097.06935.d9
Rathore, S., Akbari, H., Rozycki, M., Abdullah, K. G., Nasrallah, M. P., Binder, Z. A., et al. (2018). Radiomic MRI signature reveals three distinct subtypes of glioblastoma with different clinical and molecular characteristics, offering prognostic value beyond IDH1. Nat. Scient. Rep. 8:5087. doi: 10.1038/s41598-018-22739-2
Scarpace, L., Mikkelsen, T., Cha, S., Rao, S., Tekchandani, S., Gutman, D., et al. (2013). Radiology data from the cancer genome atlas glioblastoma multiforme [TCGA-GBM] collection. Cancer Imaging Arch. 2016. doi: 10.7937/K9/TCIA.2016.RNYFUYE9
Smith, S. M. (2002). Fast robust automated brain extraction. Hum. Brain Mapp. 17, 143–155. doi: 10.1002/hbm.10062
Smith, S. M., and Brady, J. M. (1997). SUSAN - a new approach to low level image processing. Int. J. Comput. Vis. 23, 45–78. doi: 10.1023/A:1007963824710
Stupp, R., Taillibert, S., Kanner, A., Read, W., Steinberg, D., Lhermitte, B., et al. (2017). Effect of tumor-treating fields plus maintenance temozolomide vs maintenance temozolomide alone on survival in patients with glioblastoma: a randomized clinical trial. JAMA 318, 2306–2316. doi: 10.1001/jama.2017.18718
Thompson, G., Mills, S., Coope, D., O'connor, J., and Jackson, A. (2011). Imaging biomarkers of angiogenesis and the microvascular environment in cerebral tumours. Br. J. Radiol. 84, S127–S144. doi: 10.1259/bjr/66316279
Tustison, N. J., Avants, B. B., Cook, P. A., Zheng, Y., Egan, A., Yushkevich, P. A., et al. (2010). N4ITK: improved N3 bias correction. IEEE Trans. Med. Imaging 29, 1310–1320. doi: 10.1109/TMI.2010.2046908
Tykocinski, E. S., Grant, R. A., Kapoor, G. S., Krejza, J., Bohman, L.-E., Gocke, T. A., et al. (2012). Use of magnetic perfusion-weighted imaging to determine epidermal growth factor receptor variant III expression in glioblastoma. Neuro-oncology 14, 613–623. doi: 10.1093/neuonc/nos073
Verhaak, R. G., Hoadley, K. A., Purdom, E., Wang, V., Qi, Y., Wilkerson, M. D., et al. (2010). Integrated genomic analysis identifies clinically relevant subtypes of glioblastoma characterized by abnormalities in PDGFRA, IDH1, EGFR, and NF1. Cancer Cell 17, 98–110. doi: 10.1016/j.ccr.2009.12.020
Keywords: transcriptomic classification, glioblastoma, multivariate analysis, brain tumors classification, biomarkers
Citation: Rathore S, Akbari H, Bakas S, Pisapia JM, Shukla G, Rudie JD, Da X, Davuluri RV, Dahmane N, O'Rourke DM and Davatzikos C (2019) Multivariate Analysis of Preoperative Magnetic Resonance Imaging Reveals Transcriptomic Classification of de novo Glioblastoma Patients. Front. Comput. Neurosci. 13:81. doi: 10.3389/fncom.2019.00081
Received: 06 July 2019; Accepted: 12 November 2019;
Published: 12 December 2019.
Edited by:
Abdelmalik Moujahid, University of the Basque Country, SpainReviewed by:
Muhammad Tahir, Saudi Electronic University, Saudi ArabiaMadhura Ingalhalikar, Symbiosis International University, India
Copyright © 2019 Rathore, Akbari, Bakas, Pisapia, Shukla, Rudie, Da, Davuluri, Dahmane, O'Rourke and Davatzikos. This is an open-access article distributed under the terms of the Creative Commons Attribution License (CC BY). The use, distribution or reproduction in other forums is permitted, provided the original author(s) and the copyright owner(s) are credited and that the original publication in this journal is cited, in accordance with accepted academic practice. No use, distribution or reproduction is permitted which does not comply with these terms.
*Correspondence: Christos Davatzikos, christos.davatzikos@pennmedicine.upenn.edu