- 1Department of Child and Adolescent Mental Health, University Hospital Erlangen, Friedrich-Alexander University Erlangen-Nürnberg, Erlangen, Germany
- 2Research Unit of Molecular Epidemiology, German Research Center for Environmental Health – Institute of Epidemiology II, Helmholtz Zentrum München, Munich, Germany
- 3Division of Human Genetics, Department of Paediatrics, Inselspital University of Bern, Bern, Switzerland
- 4Department of Obstetrics and Gynecology, University Hospital Erlangen, Friedrich-Alexander University Erlangen-Nürnberg, Erlangen, Germany
- 5Department of Obstetrics and Gynecology, RWTH Aachen University, Aachen, Germany
- 6kbo-Heckscher-Klinikum, Munich, Germany
- 7Department of Psychiatry and Psychotherapy, University Hospital Erlangen, Friedrich-Alexander University Erlangen-Nürnberg, Erlangen, Germany
- 8Department of Child and Adolescent Psychiatry, Faculty of Medicine of the TU Dresden, Dresden, Germany
Prenatal alcohol exposure (PAE) is known to elicit a broad range of systemic effects, including neurophysiological alterations that result in adverse behavioral and cognitive outcomes. However, molecular pathways underlying these long-term intrauterine effects remain to be investigated. Here, we tested a hypothesis that PAE may lead to epigenetic alterations to the DNA resulting in attentional and cognitive alterations of the children. We report the results of the study that included 156 primary school children of the Franconian Cognition and Emotion Studies (FRANCES) cohort which were tested for an objective marker of PAE, ethyl glucuronide (EtG) in meconium at birth. Thirty-two newborns were found to be exposed to alcohol with EtG values above 30 ng/g (EtG+). Previously we described PAE being associated with lower IQ and smaller amplitude of the event-related potential component P3 in go trials (Go-P3), which indicates a reduced capacity of attentional resources. Whole-genome methylation analysis of the buccal cell DNA revealed 193 differentially methylated genes in children with positive meconium EtG, that were clustered into groups involved in epigenetic modifications, neurodegeneration, neurodevelopment, axon guidance and neuronal excitability. Furthermore, we detected mediation effects of the methylation changes in DPP10 and SLC16A9 genes on the EtG related cognitive and attention-related deficits. Our results suggest that system-wide epigenetic changes are involved in long-term effects of PAE. In particular, we show an epigenetic mediation of PAE effects on cognition and attention-related processes.
Introduction
Adverse prenatal conditions have a potential to induce permanent changes in the developmental trajectories of the fetal brain. PAE is one of the most common in utero insults leading to a broad spectrum of structural, neurophysiological, cognitive and behavioral abnormalities (Hoyme et al., 2016). The degree to which alcohol affects development depends on a variety of factors including timing and the level of alcohol exposure (Pollard, 2007). At the most severe end of the spectrum is a FAS, which is described as a result of chronic exposure to high doses of alcohol (Jones and Smith, 1973; Del Campo and Jones, 2017). Whereas a clinical picture of FAS including pre- and postnatal growth retardation, facial dysmorphology, central nervous system alterations and intellectual impairment is well described, less is known about the impact of PAE that does not lead to the development of classic FAS. Newborns of mothers who consumed alcohol during pregnancy and seem to have no somatic abnormalities may reveal alterations in brain development (Flak et al., 2014). Cognitive and behavioral impairments and in particular attention deficits (Burden et al., 2005; Burger et al., 2011) in combination with hyperactivity, impulsivity (Mattson and Riley, 2000) and reduced inhibitory control have been described in children prenatally exposed to alcohol (Kingdon et al., 2016).
Prenatal adversity, such as substance exposure elicits its’ effects through long term alterations in the expression patterns of neurodevelopmental genes resulting in changed trajectories of both structural and functional brain development. A number of key studies have demonstrated that gestational alcohol exposure results in persistent genome-wide alterations to the transcriptome (Downing et al., 2012; Kleiber et al., 2012, 2013).
Increasing evidence suggests that epigenetic mechanisms are potential mediators linking environmental conditions; gene transcription and the phenotypic outcome (see for review, Murgatroyd and Spengler, 2012; Murgatroyd et al., 2015; Lussier et al., 2017). Indeed, numerous lines of evidence now point to epigenetic alterations in the etiology of FAS, including evidence from cell culture, various animal models, as well as a few clinical investigations on FAS (Rojas-Mayorquin et al., 2016; Lussier et al., 2017).
Three recent studies have characterized DNA methylation patterns of buccal epithelial cells of children with FAS (Bakdash et al., 2010; Laufer et al., 2015; Portales-Casamar et al., 2016). Laufer et al. (2015) identified a characteristic DNA methylation signature including novel genes and genes previously associated with alcohol exposure of the protocadherin cluster in children with FAS. Portales-Casamar et al. (2016) described 658 significantly differentially methylated sites between FAS cases and controls with enrichment for genes involved in neurodevelopmental processes and diseases, such as anxiety, epilepsy, and autism spectrum disorders.
Most studies on the effects of PAE investigated children with distinct FAS and/or based their work on a mother’s self-report regarding alcohol consumption during pregnancy (e.g., Furtado and Roriz, 2016), which is prone to systematic underreporting, due to retrospective recall bias and social stigmas (e.g., Eichler et al., 2016).
As a part of our FRANCES (Hein et al., 2014; Schneider et al., 2014; Eichler et al., 2016), we have studied attention and cognitive development of the 6- to 9-year-old children who were positive for EtG in meconium at birth, a child-related biomarker of intrauterine alcohol exposure (Eichler et al., 2018). Children with a positive meconium EtG, were found to have a 6-point lower IQ and a positive correlation between EtG value and ADHD symptoms (predominantly inattention) (Eichler et al., 2018). Considering ERPs in the EEG, which capture different aspects of attention and response control, EtG positive children (≥10 ng/g) were found to have a smaller P3 amplitude in go trials (Go-P3) indicating a reduced capacity of attentional resources.
In order to understand the mechanisms of these alcohol-mediated alterations, we compare the whole-genome DNA-methylation patterns in EtG positive children and children with no meconium EtG. Furthermore, we study how these epigenetic changes are related to the cognitive and attention-related deficits of the EtG positive children. Contrary to other studies all children included in the EtG-positive group did not fulfill the criteria of the FAS diagnose but were parentally exposed to measurable amounts of alcohol.
We hypothesized that (1) EtG values above the threshold are associated with differential genome-wide DNA methylation; (2) the effects of alcohol on IQ and the Go-P3 ERP component indexing attentional resources are mediated via alterations in gene methylation.
Indeed, we showed an association between meconium EtG with changes in the CpGs of 193 genes that were clustered into groups involved in epigenetic modifications, neurodegeneration, neurodevelopment and axon guidance, as well as neuronal excitability. In particular, methylation changes in the DPP10 gene were associated with the IQ score and the differential methylation of several CpGs in the SLC16A9 gene were linked to the Go-P3 ERP component that describes attention performance.
Materials and Methods
Study Design
Our data was obtained from a subset of the longitudinal Franconian Maternal Health Evaluation Studies (FRAMES, Erlangen, Germany; Reulbach et al., 2009) and the follow-up study FRANCES (Eichler et al., 2016). During the first assessment in the years 2005 till 2007, 1,100 pregnant women older than 18 years were recruited. Perinatal maternal health data was collected during the third trimester (Reulbach et al., 2009; Goecke et al., 2014) at the Department of Obstetrics and Gynecology, University Hospital Erlangen, Germany. Mothers received information material including the statement that alcohol-related metabolites (e.g., EtG) will be analyzed in meconium samples of their newborns (Figure 1).
From 2012 to 2015, 618 families were contacted again for a follow up study. 198 families agreed to re-participate (35%) in the FRANCES study. The second assessment took place at the Department of Child and Adolescent Mental Health in Erlangen, Germany, where each child was tested for IQ and ERPs recorded during a cued Go/No-go task. Moreover, the information on maternal psychopathology and the socioeconomic status of the family was obtained during an appointment with trained psychologists who were blinded to the respective EtG results (Figure 1). The study design is described in detail in Eichler et al. (2017). Methylation sets of all participants with valid EtG measurements were divided into EtG + (EtG ≥ 30 ng/g) and control groups (Table 1).
This study is consistent with the Declaration of Helsinki and was approved by the Local Ethics Committee of the University Hospital Erlangen (no. 4596). All parents gave written consent to this study and all children gave additionally their informed assent.
Sample Characteristics
Two hundred and forty-five mothers with their children re-participated in the FRANCES study. DNA samples were obtained from 215 of their children. After exclusion of twins (n = 3), missing data (n = 7) and DNA samples with low quality (n = 13), 192 participants remained for the methylation analysis.
The 192 participating mothers did not differ in their marital status [χ2(1) = 0.42, p = 0.519], educational level [χ2(1) = 0.04, p = 0.856] or family income [χ2(2) = 2.58, p = 0.276] from the women who rejected the participation, nor did the group of children with EtG measurements [maternal marital status, χ2(1) = 0.42, p = 0.519; educational level χ2(1) = 0.04, p = 0.856; family income χ2(2) = 2.58, p = 0.276] at the time of birth. There were no significant group differences regarding child age, sex or socioeconomic status (Table 1).
Furthermore 13 methylation sets were excluded during pre-processing of the methylation data (for detailed information, see pre-processing of the methylation data), reducing the sample size to n = 179. After exclusion of missing EtG data (n = 23) the final data set comprised whole-genome methylation data of 156 children, among whom n = 32 children had meconium EtG ≥ 30 ng/g and n = 124 EtG < 30 ng/g. IQ data was available for all 156 methylation datasets (Figure 1). However, calculations with Go-P3 measurements were performed with a sample size of n = 148 due to missing Go-P3 data (n = 31 children with EtG ≥ 30 ng/g and n = 117 controls). DNA sampling, IQ-testing and recording of ERPs took place on the same day. Based on the recruiting strategy, risk factors were overrepresented in this cohort. All children included in the final methylation analysis (n = 179) were from single pregnancies and had a Caucasian ethnicity.
EtG Measurement
Alcohol consumption during pregnancy was measured via the EtG- biomarker in 1 g meconium of the newborns. All samples were collected 2–24 h after birth. The subsequent EtG measurements were performed by Bakdash et al. (2010). EtG is a metabolic by-product of alcohol degradation commonly used to detect alcohol consumption (Palmer, 2009). About 75% of the meconium accumulates in the last 8 weeks of pregnancy in the fetal gut (Bakdash et al., 2010). Thus, positive EtG values represent an alcohol exposure during a time span of about 8 weeks (Bakdash et al., 2010).
The EtG value represents alcohol exposure but cannot be used for an estimation of the alcohol amount due to the individual metabolic rates. Furthermore, EtG measurements are sensitive to alcohol containing food and medication. To avoid false positives, we set the threshold for EtG+ group at 30 ng/g (Himes et al., 2015; Abernethy et al., 2017). Drinking self-report was not included as a variable in this analysis since earlier studies showed self-report results being inconsistent and less reliable than the EtG data (Lange et al., 2014; Eichler et al., 2016).
Recording and Analyzing ERP
The Go-P3 component was measured in a motivational Go/No-go task which comprised four blocks of 36 trials each. Using Presentation (Neurobehavioral Systems, United States), visual stimuli were presented on a monitor. In go trials, a danger traffic sign (cue stimulus, S1) was followed by a green figure of pedestrian traffic lights (go stimulus, S2); for details, see Supplementary Figure S1. In the second and third task blocks, a monetary reward of 10 ct was given for fast responses in Go-trials to increase motivation (Supplementary Figure S1).
During the task, EEG activity was recorded from 25 sites (10–20 system plus additional midline electrodes and mastoid electrodes; recording reference: Fcz, ground electrode: CP2). Standard electrode caps with sintered Ag/AgCl electrodes (Easycap, Herrsching, Germany) were used for EEG activity measurement.
Electrooculogram electrodes were placed below and above the right eye and at the outer canthi. Filter bandwidth was set to 0.016–120 Hz; the sampling frequency was 500 Hz. Impedances were kept under 20 kΩ.
The following preprocessing steps were applied: downsampling to 250 Hz, filtering (bandpass: 0.5–20 Hz; 24 dB/Oct Butterworth filters and 50 Hz notch filter) and an ocular correction procedure (Gratton et al., 1983). Trials with performance errors (responses faster than 200 ms or slower than 1,500 ms) and amplitudes outside a range of ±150 μV were excluded from further analysis. Go-segments lasted from -150 to 1,150 ms (related to S2). Signals were re-referenced to linked mastoids. After signal averaging, the maximum amplitude of the Go-P3 component was determined at electrode Pz (where the Go-P3 showed its maximum) in a time window from 300 to 700 ms.
As Go-P3 effects of EtG-positive vs. EtG-negative children did not depend on the reward condition as described in Eichler et al. (2018), the Go-P3 averaged over blocks with and without incentives was used for the analysis in the present manuscript.
Cognitive Development
Cognitive development was measured by standardized IDS (Grob et al., 2009). Total IQ score is based on the sum of seven subtest scores including visual perception, attention, phonological memory, spatio-visual memory, auditory memory, abstract reasoning and conceptual reasoning. The test is validated including gender- and age-specific norms, has an internal consistency of α = 0.92 and a retest-reliability (15 months) of rtt = 0.83 (Grob et al., 2009). Testing was performed by experienced psychologists.
Other Characteristics
Birth weight was recorded directly after delivery. Maternal smoking behavior was assessed by a self-report: here, ≥1 cigarette per day was set as a threshold for smoking.
Estimation of the socioeconomic status was based on maternal and paternal education level (4-level: <9, 9, 10, or 13 years) and net family income (6-level: <1,000 to >5,000) (sum-index, theoretical range: 3–14) as described in Geißler (1994).
Recording and analysis of the event related potentials as well as the methylation analysis procedure are explained in detail in the supplement.
DNA Extraction and Methylation Analysis
DNA for methylation analysis was extracted from buccal cells, which were collected with OmniSwabs (Whatman®, kat. no. 28421853) from the children’s inner cheek. Subsequently, DNA was isolated with the QIAamp DNA Mini Kit (Qiagen, kat. no. 51306) according to the manufacturer’s protocol and stored at +4°C. 500 ng gDNA per sample was send to the Helmholtz-Zentrum München for genome-wide DNA methylation analysis. DNA methylation was analyzed using the Infinium Human Methylation 450K BeadChip array (Illumina).
Pre-processing of Methylation Data
Pre-processing of methylation data were executed with R (version 3.2.2) using the packages Minfi (Aryee et al., 2014) and wateRmelon (Pidsley et al., 2013) according to Lehne et al. (2015) with minor modification. In particular, we analyzed all data sets for age and sex mismatches by shinyMethyl (Fortin et al., 2014) and DNAm age calculator (Horvath, 2013) prior to the data processing. Preprocessing was performed with the dataset of N = 179 samples. To account for technical variation in background fluorescence signals Illumina Background correction was applied to the raw intensity values. Subsequently, raw intensity values were normalized with quantile as implemented in Minfi and converted to beta values. These represent the proportion of DNA methylation at each single CpG. Probes were excluded if they fulfilled one of the following criteria: call rate < 97%, probes located on sex chromosomes or SNPs and a detection p-value > 0.001, which represents a low signal-detection rate from the background. Further exclusion criteria were mean beta <0.01 or >0.99 and a standard deviation less than 0.1 or above 4.0. To reduce technical bias we applied control probe adjustment to normalized beta values as recommended by Lehne et al. (2015). Control probe adjustment was done by a principal component analysis of the control probes with 22 factors explaining 95% of the variance (Supplementary Figure S3). Gender, age, maternal smoking behavior during pregnancy, and birth weight were added as cofactors to the regression model. The obtained residuals were used for a second PCA to explain further biological variance. The first two factors of the second PCA were added as predictors to a final regression model representing the values for further analysis. After exclusion of samples with missing EtG measurements (n = 23), 156 boys and girls were finally included in the analysis.
Statistics
Differentially methylated CpGs were identified in an epigenome-wide analysis by independent t-tests (EtG+ group vs. control group) assuming unequal variances with the software R (version 3.2.2). A threshold of p < 10-3 was applied. To test a functional relevance of differentially methylated CpGs regarding total IQ score, the score of the abstract reasoning subtest, and Go-P3 we conducted three independent mediation models have been calculated using the SPSS macro PROCESS (version 2.16; Hayes, 2013). Our hypothesis was that alcohol exposure causes methylation changes, which in turn affect IQ, abstract reasoning, or Go-P3, which were tested by mediation analysis. Here EtG+ vs. control groups served as predictors, differentially methylated CpGs between these groups were considered mediators, and total IQ, the subtest abstract reasoning, or Go-P3 were considered outcome variables. Indirect effects were considered statistically significant at p-value < 0.05 as calculated by implemented Sobel test and bootstrapped 95% confidence intervals (CI) based on 10,000 samples without 0. In calculations containing total IQ or subset abstract reasoning as outcome variable, socioeconomic status was added as covariate to control for maternal and parental IQ. CpGs were included in the mediation analysis if they were identified by whole-genome analysis with a p-value less than 10-3 or located in a gene affected by the aforementioned criterion. A mediation model is a multilevel regression model between an independent variable, a mediator variable, and a dependent variable. In contrast to a direct relationship between the dependent and the independent variable the mediation model analyses an influence of the independent variable on the mediator variable, which in turn influences the dependent variable. The term “mediation effect” describes the two-level effect between independent variable and mediator as well as mediator and dependent variable. The “mediation effect” is represented by correlations between PAE and the methylation status and between the changes in methylation and IQ or Go-P3.
The second rule was solely applied if matching CpGs’ methylation status showed significant differences in a t-test between EtG+ and the control group.
If indirect models by Sobel test revealed a significant p-value, all CpGs of the respective genes were checked for methylation differences by a t-test. (Supplementary Table S2). Here, p-values were adjusted by FDR procedure (Benjamini and Hochberg, 1995). Additionally, thresholds for Bonferroni-correction of CpGs per gene were calculated.
Functional gene clustering was performed with online tool provided by the Database for Annotation, Visualization and Integrated Discovery (DAVID, v6.7; Huang da et al., 2009a,b).
The classification stringency used for clustering was set to medium (as recommended) and contained the following parameters: similarity overlap = 3, similarity threshold = 0.5, initial group membership = 3, and multiple linkage threshold = 0.5. DAVID calculated 68 clusters ranging between an enrichment score of 0.08 and 1.89.
Results
EtG+ Children Reveal Lower IQ Test Results and Alterations in the Attention-Related ERP
Using the FRANCES cohort, Eichler et al. (2018) showed IQ differences in children with an EtG concentration in meconium above the 50th percentile. Here, we report this effect to be also persistent in the group of the EtG positive children with a threshold set at ≥30 ng/g.
Controlling for socioeconomic status EtG+ children (n = 32) had a lower total IQ than controls (n = 124; F = 6.04, p = 0.015, = 0.04).
Looking at the subtests of the IDS, a significant effect was obtained for abstract reasoning (t = 2.03, p = 0.049, d = 0.40), which reflects the aptitude or ability to reason logically in non-verbal tests.
Moreover, the observed differences in the Go-P3 (Eichler et al., 2018) as reported recently were also stable using the threshold of EtG ≥ 30 ng/g (Table 1). The differences between the EtG+ group (n = 31) and controls (n = 117) were analyzed by t-test (t = 2.43, p = 0.004, d = 0.49). Here, one child of the EtG+ and 7 of the control group were excluded due to the insufficient quality of the ERP data.
EtG+ Children Show Differential Genome-Wide DNA Methylation
After data processing, we obtained a list of CpGs and corresponding genes that showed significant differences in methylation between EtG+ and controls. The list was filtered down to stringent (p < 10-3, Supplementary Figures S4, S5), but not FDR corrected differentially methylated CpGs (193 genes in total, Supplementary Table S1). A p-value of 0.001 with the said sample size corresponds roughly to a medium effect size of 0.65 (Cohen’s d).
Gene Ontology (GO) Results
To get insights in the affected biological processes we clustered our results into groups based on the functional annotations provided by the DAVID (v6.7; Huang da et al., 2009a,b).
In summary, the affected genes belonged to several groups primarily involved in regulating epigenetic modifications (SET Domain), neurodegeneration and development including cell growth and axon guidance as well as neuronal excitability. Moreover several genes involved in nucleotide binding, cell adhesion, transmembrane transport and exopeptidase function were affected by methylation changes (Table 2). 19% of these identified genes function in neuronal processes (Supplementary Figure S2). According to the analysis, the SET Domain group was enriched the most followed by nucleotide binding, cell adhesion and neuron differentiation.
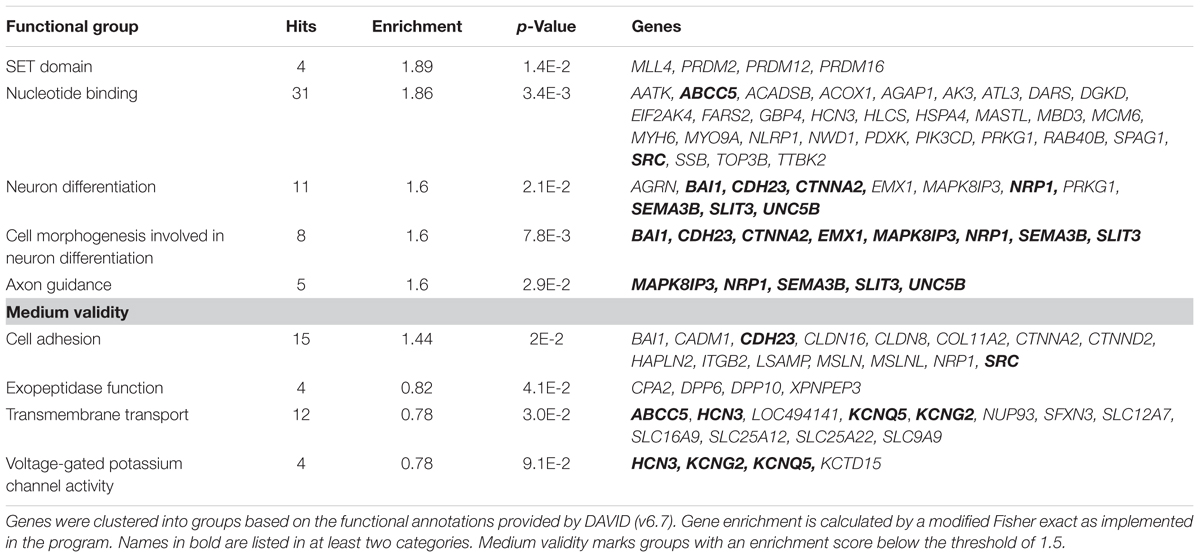
TABLE 2. Functional groups of genes that show alterations in methylated CpG sites (p < 10-3) between EtG+ (≥30 ng/g) and control children.
The Methylation Changes in the Genes DPP10 and SLC16A9 Mediate the Differences in IQ and Go-P3 in Gene-Specific Analyses
Our hypothesis was that the direct effect of alcohol exposure on IQ and Go-P3 can be (partially) explained by an indirect effect containing methylation changes.
We set EtG+ as predictor, the ethanol induced methylation changes of the 193 CpGs obtained by whole-genome analysis (Supplementary Table S1) as mediating variable, and IQ, its subtest abstract reasoning, or Go-P3 as outcome variable. The mediation analysis is a multilevel regression model revealing correlations between PAE, and the methylation status of the respective CpGs. Solely 2 of 193 CpGs were statistically significant in the indirect effect of the mediation analysis; CpG cg26842423 (DPP10) and CpG cg06578117 (SLC16A9). CpG cg26842423 (DPP10) could be identified as mediating variable for the association of EtG+, IQ (indirect effect: b = 1.67∗, 95% CI [0.69; 3.34], p = 0.026) and abstract reasoning (indirect effect: b = 0.46∗, 95% CI [0.17; 0.95], p = 0.030), respectively (Figure 2A). Another mediation analysis revealed the EtG-dependent (EtG ≥ 30 ng/g vs. controls) methylation of CpG cg06578117 (SLC16A9) as mediator for the effect of EtG on Go-P3 (indirect effect: b = 1.06∗, 95% CI [0.25; 2.28], p = 0.04; Figure 2B).
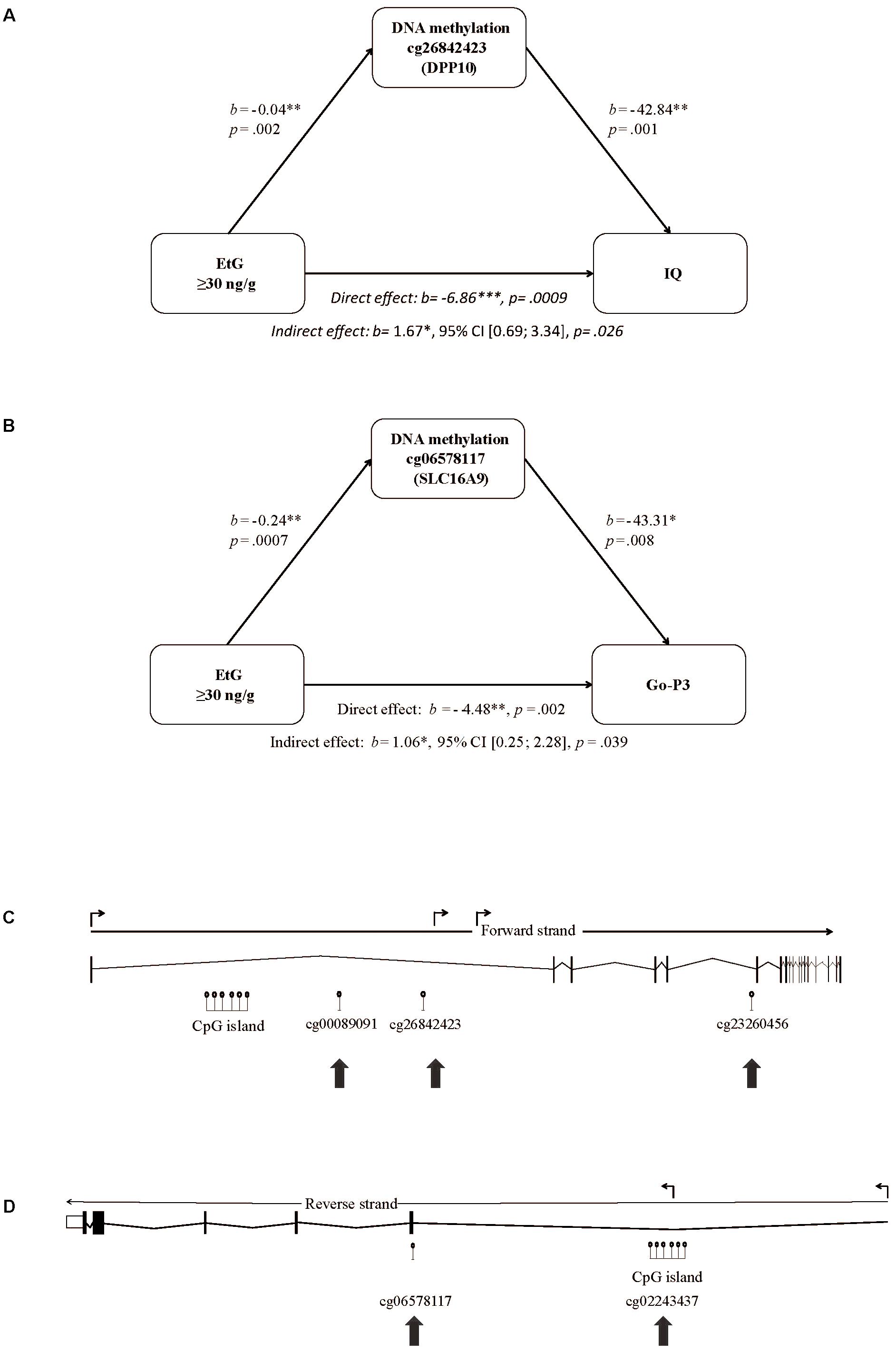
FIGURE 2. Mediation model of EtG as predictor for reduced (A) IQ, (B) Go-P3, and localization of the CpGs in the DPP10 gene and SLC16A9 affected by prenatal alcohol exposure. (C) The model consists of EtG as predictor, the methylation changes in cg26842423 (DPP10) between EtG+ (n = 32) and controls (n = 124) as mediator, and IQ as outcome. (B) The model consists of EtG+ as predictor, the methylation changes in cg06578117 (SLC16A9) between EtG+ (n = 31) and controls (n = 117) as mediator, and Go-P3 as outcome. The analysis was conducted with PROCESS (version 2.16; Hayes, 2013). Socioeconomic status was used as covariate. ∗p < 0.05, ∗∗p < 0.01, ∗∗∗p < 0.001. (C) Significant DNA methylation changes in the EtG+ group were observed in intron 1 and 5 of DPP10 and (D) in the 5′UTR and exon 1 of the SLC16A9 gene as indicated with arrows. Shown are the full-length genes with introns and exons according to UCSC (http://genome.ucsc.edu, hg38; Aken et al., 2016). The angled arrows above the transcription direction mark the positions for transcription variants.
CpGs are known to be more likely methylated/demethylated in line with other CpGs. Thus, we investigated if other CpGs located in DPP10 (n = 50) or SLC16A9 (n = 22) were differentially methylated depending on the EtG+ threshold (Supplementary Table S2). Two additional CpGs in DPP10 (4% of tested CpGs) and one more in SLC16A9 (5% of tested CpGs) showed methylation differences between EtG+ vs. control group. None of these showed positive results in the mediation analysis with IQ, abstract reasoning or Go-P3 as outcome variable (Supplementary Table S2).
Moreover, CpGs’ positions determine its influence on the gene regulation and function. The positions of the aforementioned three CpGs of DPP10 (cg26842423, cg00089091, and cg23260456) are shown in Figure 2C.
The second differentially methylated gene we report here is SLC16A9. CpG cg06578117 and cg02243437 are increased in methylation in the EtG+ group (Supplementary Table S2). The respective CpGs are both located in a region with enhancer function and thus may directly influence the expression of the SLC16A9 gene. The positions of the CpGs of SLC16A9 affected in the EtG+ group are shown in Figure 2D.
SLC16A9 and DPP10 Are Indirectly Linked by Co-expression and Genetic Interaction
Our study revealed that, alcohol exposure might influence children’s IQ and attentional capacity/Go-P3 by methylation changes in DPP10 and SLC16A9 genes. To identify possible links between these two genes, the geneMANIA network analysis was performed (Figure 3 and Supplementary Figure S6). This analysis revealed a connection by co-expression and genetic interaction of DPP10 and SLC16A9 via expression of LRRN1, CRISPLD1, and GIPC2 (Chen et al., 2002; Roth et al., 2006; Warde-Farley et al., 2010; Mallon et al., 2013).
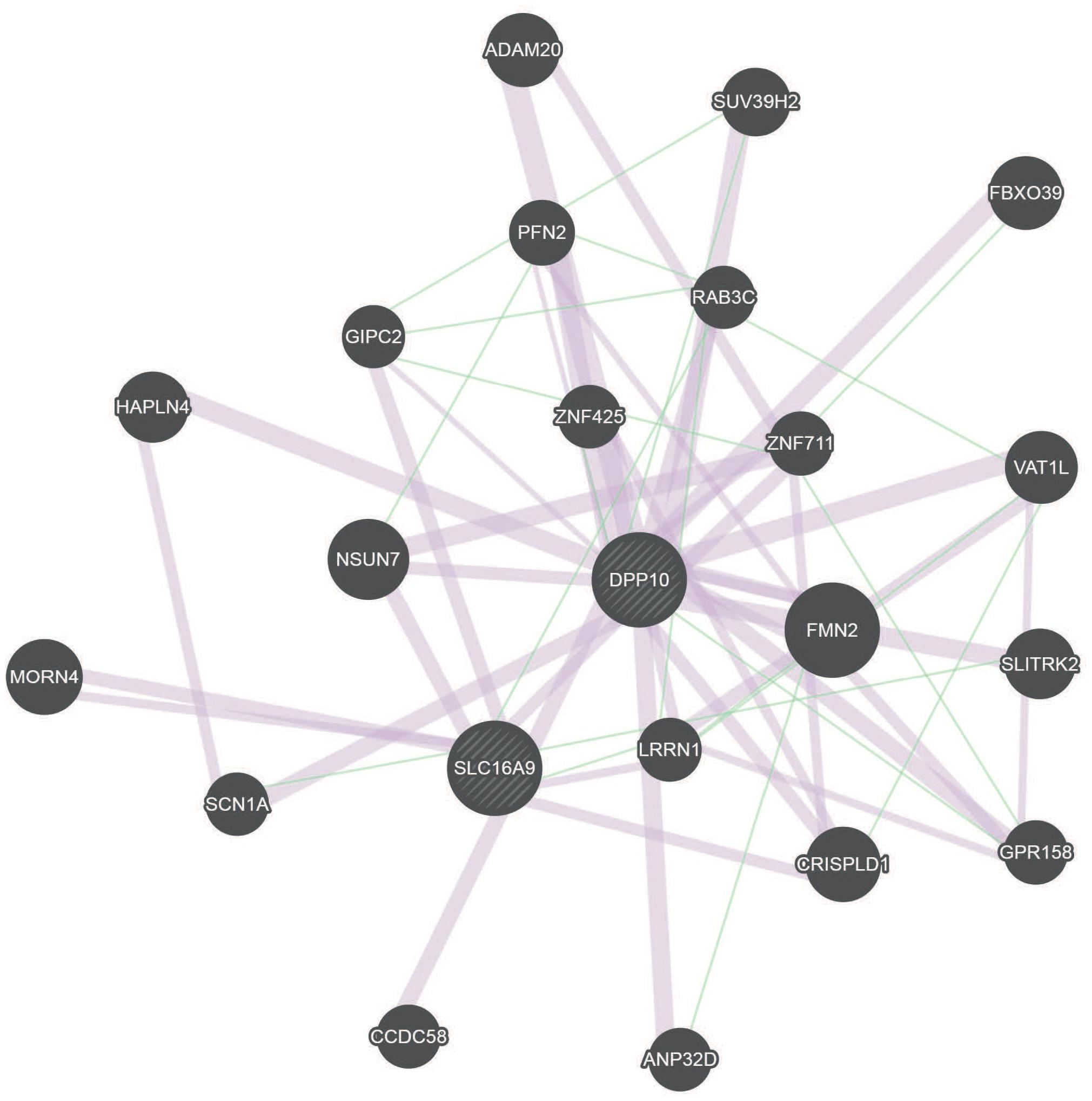
FIGURE 3. GeneMANIA network for DPP10 and SLC16A9 based on genetic interactions (green) and co-expression (red). DPP10 and SLC16A9 are indirectly connected over genetic interaction and co-expression.
Discussion
Prenatal alcohol exposure is a major risk factor for adverse cognitive and behavioral phenotypes. Increasing evidence suggests that epigenetic mechanisms potentially mediate the effects of this in utero adversity on the neurodevelopmental processes (Lussier et al., 2017).
In the FRANCES cohort, we could previously demonstrate the negative impact of PAE on child’s cognitive development and ADHD-related behavior on the basis of the ethanol metabolite EtG in newborn’s meconium (Eichler et al., 2018). In this study, we present a comprehensive genome-wide analysis of DNA methylation in buccal cells of children of the same FRANCES cohort both positive and negative for meconium EtG. Furthermore, we examine the hypothesis whether the EtG associated effects on the cognitive and attentional processes are mediated by alterations in DNA methylation. In contrast to other studies, we focused on methylation changes in children without diagnosed FAS but measurable PAE.
In children with PAE we report 193 genes with differentially methylated CpGs (p < 10-3), which could be clustered into nine biological groups playing a crucial role in the epigenetic processes, neuronal differentiation, and neuronal excitability.
Several genes belong to the SET – domain and to the “nucleotide binding” cluster, both containing components of the epigenetic regulatory machinery for neural growth and differentiation (Cui et al., 1998). In particular, the methyl-CpG-binding domain protein 3 (MBD3) seems to be relevant for maintenance and establishment of 5-hydroxymethylcytosin (5hmC) by interaction with Tet proteins (Yildirim et al., 2011), last being previously linked to FAS (Cheng et al., 2015). In line with the results of Laufer et al. (2015), we observe methylation changes in genes coding for cell adhesion and genes of the protocadherins family. Interestingly, mutations in the CDH23 gene of the cell adhesion domain result in deafness (Woo et al., 2014) and hearing loss is closely associated with FAS (Church, 1987).
Among 193 differentially methylated genes of the EtG positive group, 11 have an important function in neuronal differentiation and axon guidance. For instance, CTNNA2 and EMX1 function as transcription factors and are implicated in the cellular identity of cortical neurons. EMX1 deletion in mice results in a smaller hippocampus and a lack of the corpus callosum (Qiu et al., 1996). In addition, Lim et al. (2015) showed that EMX1 functions in “cingulate callosal axon guidance” by regulating the expression of NRP1, which in turn, was also identified as differentially methylated in the EtG+ group. Differentially methylated are also several genes coding for the proteins of the voltage-gated potassium channels. These are widely distributed in all brain areas and play an important role in neuronal excitability. Data was provided suggesting that potassium channel gene KCNQ1 may contribute to the shared risk for diminished processing speed and white mater integrity (Bruce et al., 2017).
Several hits detected in our study were previously associated with alcohol-related disorders. PRDM2 gene, for instance, involved in neuronal differentiation, has recently been associated with compulsive drinking and alcohol self-administration in rats (Barbier et al., 2016). NLRP1 gene was described to play a role in alcohol-induced inflammation in neuronal cells (Youm et al., 2013; de Rivero Vaccari et al., 2014). CTNNA2 has previously been identified as a risk gene for alcohol addiction (Song and Zhang, 2014). ITGB2 in rat was hypothesized to contribute to high alcohol drinking behavior (McBride et al., 2013). SRC signaling was linked to alcohol-induced neuroinflammation and neurodegeneration (Floreani et al., 2010).
Prenatal alcohol exposure has been shown to perturb the epigenetic machinery in several animal (Tulisiak et al., 2017) and human studies (Laufer et al., 2015; Portales-Casamar et al., 2016). Laufer et al. (2015) characterized DNA methylation in a discovery cohort consisted of six FAS patients and five matched controls and validated their results in a replication cohort of six different FAS patients and six controls. They identified 269 significant (p < 0.005) differentially methylated CpG sites, in genes related to protocadherins, glutamatergic synapses, and hippo signaling (Laufer et al., 2015). An involvement of such functional clusters as neurodevelopment and axonal guidance, as well as cell adhesion, was observed in our study and in the study of Laufer et al. (2015).
Another recent work of Portales-Casamar et al. (2016) described 658 significantly differentially methylated sites between FAS cases and controls with enrichment for genes involved in neurodevelopmental processes. A further study validated 161 of these as possible predictors for FAS (Lussier et al., 2018).
Within the 193 differentially methylated genes of the EtG positive group two genes are associated with the IQ and alterations in the attention-related processes. In particular, our mediation analysis showed that the methylation of the CpG cg26842423 in the DPP10 gene influences total IQ and abstract reasoning capacity. DPP10 is a single-pass type II membrane protein that binds specific voltage gated potassium channels. Thus, it plays an important role in a variety of cellular processes, such as neurotransmitter release, neuronal excitability and repolarization of action potentials. DPP10 has been linked to ADHD (Lasky-Su et al., 2008) and neurodegenerative processes in Parkinson’s and Alzheimer’s diseases (Chen et al., 2014). Several findings support DPP10 to be a target for multiple toxins, such as nicotine, alcohol, and phthalates (Blakey et al., 2009; Wu et al., 2010; Chhabra et al., 2014; Machtinger et al., 2018). More than 50 potential targets for DNA methylation have been identified within this gene so far. Among those Heinrich et al. (2017) reported an association between CpG cg19651219 (DPP10) with the Cue-P3, reflecting attentional orienting in ERPs, and ADHD behavior using the same cohort.
Consistently recurrent CNVs in the DPP10 are enriched in autism-spectrum-disorders (Marshall et al., 2008; Girirajan et al., 2013). Interestingly, as the size of deletions increases, non-verbal IQ significantly decreases, but there is no impact on autism severity; and as the size of duplications increases, autism severity significantly increases but non-verbal IQ is not affected (Girirajan et al., 2013).
Other authors reported associations between PAE and ADHD symptoms (Mick et al., 2002; Knopik et al., 2006, 2009). It is important to mention that Eichler et al. (2018) found evidence of ADHD symptoms derived from PAE to be related to different pathways than ‘classical’ ADHD. This might explain the difference in affected CpGs in DPP10 between ADHD and PAE. Interestingly, Knopik et al. (2016) also published an association between prenatal smoke exposure and ADHD symptoms. One explanation could be that DPP10 is a key target for multiple prenatal risks connected to ADHD. This seems quite realistic since DPP10 is a peptidase with a broad range of functions that modulate the electrophysiological properties of voltage-gated potassium channels via Kv4 subunit [primary K(+) channel pore-forming subunit], and plays a crucial role in the neuronal excitability. The Kv4 subunit contributes to the somatic and dendritic A-type currents regulating of neuronal excitability and dendritic processing of incoming synaptic information. It was repeatedly shown to be involved synaptic plasticity and learning and memory (Alexander et al., 2009). In particular, deletion of Kv4.2 gene eliminates dendritic A-type K+ current and enhances induction of long-term potentiation in hippocampal CA1 pyramidal neurons (Chen and Johnston, 2006; Chen et al., 2006). Lugo et al. (2012) show Kv4.2 knockout mice having hippocampal-dependent learning and memory deficits.
We propose the model that multiple toxins affect DPP10 which in turn has an effect on cognition and behavior.
There is no data on the functional changes on the expression regulation of the DPP10 gene through the affected CpG (cg00089091 and cg26842423). All affected CpGs are located within introns. Several authors suggested that DNA methylation in the gene body could increase gene expression by blocking the initiation of intragenic promotors (Maunakea et al., 2010). Moreover, gene body methylation is also hypothesized to influence splicing and thus, defining splice variants (Laurent et al., 2010). Targeted cell culture studies are necessary in order understand the functional significance of this methylation change.
Previously we described, that children with a meconium EtG above the detection limit show reduced Go-P3 amplitudes, an ERP component that is related to the allocation of attentional resources and executive response control (Eichler et al., 2018). These results indicate a potential risk for specific attentional impairments (Spronk et al., 2008), although no other ADHD related neural or neuropsychological function was negatively influenced in EtG+ group. Several other studies found an association between maternal alcohol use during pregnancy and offspring ADHD symptoms (Infante et al., 2015; Eilertsen et al., 2017). Even after controlling for the impact of the postnatal environment, elementary school children, who experienced PAE, are highly vulnerable to develop mood disorders, anxiety disorders, ADHD, oppositional defiant disorder or conduct disorder (Burger et al., 2011).
Here, we report positive meconium EtG as a predictor of Go-P3 by cg06578117 (SLC16A9) methylation. SLC16A9 is a monocarboxylate transporter and member of the soluble carrier family 16. This gene changes carnitine levels in blood by functioning as carnitine efflux transporter (Suhre et al., 2011). SLC16A9 was hypothesized to be a risk gene for neurodegeneration (Gonzalez-Aramburu et al., 2013). Sutin et al. (2014) showed a correlation between uric acid concentrations and impulsivity in humans and mice. Other studies found a positive correlation between uric acid concentrations and ADHD symptoms and thus uric acid may play a role in hyperactivity (Barrera et al., 1988; Johnson et al., 2011). Since SLC16A9 as carnitine efflux transporter directly influences the uric concentration, its activity could possibly influence ADHD symptoms.
The geneMANIA network analysis revealed an indirect connection between DPP10 and SLC16A9 by co-expression which is linked via LRRN1. LRRN1 has previously been identified as neurite growth regulator in early development (Buchser et al., 2010).
Interestingly, aside from co-expression, DPP10 and SLC16A9 also genetically interact (Lin et al., 2010) via the Solute Carrier Family 9 member SLC9A9, which has also been identified as differentially methylated in our EtG+ group (Supplementary Table S2). SLC9A9 functions an ion transport and is a risk gene for ADHD (de Silva et al., 2003). This is consistent with findings, that FAS is accompanied by an increased risk for ADHD (Burd et al., 2003; Rojas-Mayorquin et al., 2016). This connection may offer a model how PAE and finally FAS are linked to the increased risk of ADHD.
The main limitation of our study is a modest sample size for detecting small effects in a genome-wide analysis. Second limitation is a missing replication cohort though at least some of the results are in line with findings of recent epigenome-wide studies on FAS. Third, no reliable data on the correlations of the EtG levels and an amount of alcohol consumed by the mother exist so far; thus not allowing to predict alcohol induced adverse effects depending on the consume amount/type. Forth, EtG levels can depend on the metabolism of both fetus and the mother, another variable that might be associated with the methylation changes detected. Fifth, we made substantial efforts to eliminate Type I errors by setting the discovery threshold at p = 10-3. However, investigating over 450,000 sites in a sample of n = 156, these efforts might not be sufficient especially as this sample size did not allow to correct for FDR (Supplementary Figures S4, S5). A threshold of p = 10-3 corresponding to a medium effect size of 0.65 (Cohen’s d) was applied instead. Sixth, we examined methylation changes in buccal cells samples that might not or not exactly reflect changes in the brain tissue, we are concerned with. However, easily available samples are favorable when searching for potential biomarkers of prenatal adversity.
Conclusion
This study reports 193 differentially methylated genes in buccal cells of children with positive meconium EtG, an objective marker of maternal alcohol consume. Additionally we show mediation effects of the methylation changes in DPP10 and SLC16A9 genes on the EtG-related cognitive and attention-related alterations in IQ and ERP. Our data shows that maternal alcohol consumption has a long-term impact on epigenetic programming of key neurodevelopmental genes resulting in adverse cognitive and behavioral outcome. Further studies are needed to validate our findings and to identify associated pathways.
Availability of Data and Material
Methylation data have been deposited in the ArrayExpress database at EMBL-EBI1 under accession number E-MTAB-6730.
Author Contributions
SF, VS, YG, HH, and AE analyzed the data and/or interpreted the results. JKr and SW were involved in the 450k methylation analysis. TG, JKo, PF, and MB initiated and designed the FRAMES project. TG and PF supervised the clinical data acquisition in FRAMES. OK, GM, HH, and AE were responsible for the study design of FRANCES. SG contributed to the design of the epigenetic part of FRANCES. SF and YG wrote the manuscript. All authors reviewed the manuscript.
Funding
This work was initially supported by the ELAN Fonds of the University Hospital of Erlangen under grant 56750001/JP-12-25-29-01; and the Staedtler-Stiftung, Nürnberg under grant WW/eh 10/15.
Conflict of Interest Statement
The authors declare that the research was conducted in the absence of any commercial or financial relationships that could be construed as a potential conflict of interest.
Acknowledgments
The authors all families who participated in FRANCES as well as the colleagues and student assistants contributing to the project, particularly Martin Deinzer, Jörg Distler, and Ruth Steigleder. They acknowledge support by Deutsche Forschungsgemeinschaft and Friedrich-Alexander-Universität Erlangen-Nürnberg (FAU) within the funding program Open Access Publishing.
Supplementary Material
The Supplementary Material for this article can be found online at: https://www.frontiersin.org/articles/10.3389/fnbeh.2018.00125/full#supplementary-material
Abbreviations
ADHD, attention deficit hyperactivity disorder; CNVs, copy number variations; DPP10, dipeptidyl peptidase 10; ERPs, event-related potentials; EtG, ethyl glucuronide; FAS, fetal alcohol syndrome; FDR, false discovery rate; FRANCES, Franconian Cognition and Emotion Studies; Go-P3, event-related potential component P3 in go trials; IDS, Intelligence and Development Scales; IQ, intelligence quotient; PAE, prenatal alcohol exposure; SLC16A9, solute carrier family 16 member 9.
Footnotes
References
Abernethy, C., Mccall, K. E., Cooper, G., Favretto, D., Vaiano, F., Bertol, E., et al. (2017). Determining the pattern and prevalence of alcohol consumption in pregnancy by measuring biomarkers in meconium. Arch. Dis. Child. Fetal. Neonatal. Ed. 103, F216–F220. doi: 10.1136/archdischild-2016-311686
Aken, B. L., Ayling, S., Barrell, D., Clarke, L., Curwen, V., Fairley, S., et al. (2016). The Ensembl gene annotation system. Database 2016:baw093. doi: 10.1093/database/baw093
Alexander, J. C., Mcdermott, C. M., Tunur, T., Rands, V., Stelly, C., Karhson, D., et al. (2009). The role of calsenilin/DREAM/KChIP3 in contextual fear conditioning. Learn. Mem. 16, 167–177. doi: 10.1101/lm.1261709
Aryee, M. J., Jaffe, A. E., Corrada-Bravo, H., Ladd-Acosta, C., Feinberg, A. P., Hansen, K. D., et al. (2014). Minfi: a flexible and comprehensive Bioconductor package for the analysis of Infinium DNA methylation microarrays. Bioinformatics 30, 1363–1369. doi: 10.1093/bioinformatics/btu049
Bakdash, A., Burger, P., Goecke, T. W., Fasching, P. A., Reulbach, U., Bleich, S., et al. (2010). Quantification of fatty acid ethyl esters (FAEE) and ethyl glucuronide (EtG) in meconium from newborns for detection of alcohol abuse in a maternal health evaluation study. Anal. Bioanal. Chem. 396, 2469–2477. doi: 10.1007/s00216-010-3474-5
Barbier, E., Johnstone, A. L., Khomtchouk, B. B., Tapocik, J. D., Pitcairn, C., Rehman, F., et al. (2016). Dependence-induced increase of alcohol self-administration and compulsive drinking mediated by the histone methyltransferase PRDM2. Mol. Psychiatry 22, 1746–1758. doi: 10.1038/mp.2016.131
Barrera, C. M., Ruiz, Z. R., and Dunlap, W. P. (1988). Uric acid: a participating factor in the symptoms of hyperactivity. Biol. Psychiatry 24, 344–347. doi: 10.1016/0006-3223(88)90205-3
Benjamini, Y., and Hochberg, Y. (1995). Controlling the false discovery rate: a practical and powerful approach to multiple testing. J. R. Stat. Soc. B 57, 289–300.
Blakey, J. D., Sayers, I., Ring, S. M., Strachan, D. P., and Hall, I. P. (2009). Positionally cloned asthma susceptibility gene polymorphisms and disease risk in the British 1958 Birth Cohort. Thorax 64, 381–387. doi: 10.1136/thx.2008.102053
Bruce, H. A., Kochunov, P., Paciga, S. A., Hyde, C. L., Chen, X., Xie, Z., et al. (2017). Potassium channel gene associations with joint processing speed and white matter impairments in schizophrenia. Genes Brain Behav. 16, 515–521. doi: 10.1111/gbb.12372
Buchser, W. J., Slepak, T. I., Gutierrez-Arenas, O., Bixby, J. L., and Lemmon, V. P. (2010). Kinase/phosphatase overexpression reveals pathways regulating hippocampal neuron morphology. Mol. Syst. Biol. 6, 391. doi: 10.1038/msb.2010.52
Burd, L., Klug, M. G., Martsolf, J. T., and Kerbeshian, J. (2003). Fetal alcohol syndrome: neuropsychiatric phenomics. Neurotoxicol. Teratol. 25, 697–705. doi: 10.1016/j.ntt.2003.07.014
Burden, M. J., Jacobson, S. W., Sokol, R. J., and Jacobson, J. L. (2005). Effects of prenatal alcohol exposure on attention and working memory at 7.5 years of age. Alcohol. Clin. Exp. Res. 29, 443–452. doi: 10.1097/01.ALC.0000156125.50577.EC
Burger, P. H., Goecke, T. W., Fasching, P. A., Moll, G., Heinrich, H., Beckmann, M. W., et al. (2011). [How does maternal alcohol consumption during pregnancy affect the development of attention deficit/hyperactivity syndrome in the child]. Fortschr. Neurol. Psychiatr. 79, 500–506. doi: 10.1055/s-0031-1273360
Chen, T., Gai, W. P., and Abbott, C. A. (2014). Dipeptidyl peptidase 10 (DPP10(789)): a voltage gated potassium channel associated protein is abnormally expressed in Alzheimer’s and other neurodegenerative diseases. Biomed. Res. Int. 2014:209398. doi: 10.1155/2014/209398
Chen, X., Cheung, S. T., So, S., Fan, S. T., Barry, C., Higgins, J., et al. (2002). Gene expression patterns in human liver cancers. Mol. Biol. Cell 13, 1929–1939. doi: 10.1091/mbc.02-02-0023
Chen, X., and Johnston, D. (2006). Voltage-gated ion channels in dendrites of hippocampal pyramidal neurons. Pflugers Arch. 453, 397–401. doi: 10.1007/s00424-006-0097-y
Chen, X., Yuan, L. L., Zhao, C., Birnbaum, S. G., Frick, A., Jung, W. E., et al. (2006). Deletion of Kv4.2 gene eliminates dendritic A-type K+ current and enhances induction of long-term potentiation in hippocampal CA1 pyramidal neurons. J. Neurosci. 26, 12143–12151. doi: 10.1523/JNEUROSCI.2667-06.2006
Cheng, Y., Bernstein, A., Chen, D., and Jin, P. (2015). 5-Hydroxymethylcytosine: a new player in brain disorders? Exp Neurol. 268, 3–9. doi: 10.1016/j.expneurol.2014.05.008
Chhabra, D., Sharma, S., Kho, A. T., Gaedigk, R., Vyhlidal, C. A., Leeder, J. S., et al. (2014). Fetal lung and placental methylation is associated with in utero nicotine exposure. Epigenetics 9, 1473–1484. doi: 10.4161/15592294.2014.971593
Church, M. W. (1987). Chronic in utero alcohol exposure affects auditory function in rats and in humans. Alcohol 4, 231–239. doi: 10.1016/0741-8329(87)90017-6
Cui, X., De Vivo, I., Slany, R., Miyamoto, A., Firestein, R., and Cleary, M. L. (1998). Association of SET domain and myotubularin-related proteins modulates growth control. Nat. Genet. 18, 331–337. doi: 10.1038/ng0498-331
de Rivero Vaccari, J. P., Dietrich, W. D., and Keane, R. W. (2014). Activation and regulation of cellular inflammasomes: gaps in our knowledge for central nervous system injury. J. Cereb. Blood Flow Metab. 34, 369–375. doi: 10.1038/jcbfm.2013.227
de Silva, M. G., Elliott, K., Dahl, H. H., Fitzpatrick, E., Wilcox, S., Delatycki, M., et al. (2003). Disruption of a novel member of a sodium/hydrogen exchanger family and DOCK3 is associated with an attention deficit hyperactivity disorder-like phenotype. J. Med. Genet. 40, 733–740. doi: 10.1136/jmg.40.10.733
Del Campo, M., and Jones, K. L. (2017). A review of the physical features of the fetal alcohol spectrum disorders. Eur. J. Med. Genet. 60, 55–64. doi: 10.1016/j.ejmg.2016.10.004
Downing, C., Flink, S., Florez-Mcclure, M. L., Johnson, T. E., Tabakoff, B., and Kechris, K. J. (2012). Gene expression changes in C57BL/6J and DBA/2J mice following prenatal alcohol exposure. Alcohol. Clin. Exp. Res. 36, 1519–1529. doi: 10.1111/j.1530-0277.2012.01757.x
Eichler, A., Grunitz, J., Grimm, J., Walz, L., Raabe, E., Goecke, T. W., et al. (2016). Did you drink alcohol during pregnancy? Inaccuracy and discontinuity of women’s self-reports: On the way to establish meconium ethyl glucuronide (EtG) as a biomarker for alcohol consumption during pregnancy. Alcohol 54, 39–44. doi: 10.1016/j.alcohol.2016.07.002
Eichler, A., Hudler, L., Grunitz, J., Grimm, J., Raabe, E., Goecke, T. W., et al. (2018). Effects of prenatal alcohol consumption on cognitive development and ADHD-related behaviour in primary-school age: A multilevel study based on meconium ethyl glucuronide. J. Child Psychol. Psychiatry 59, 110–118. doi: 10.1111/jcpp.12794
Eichler, A., Walz, L., Grunitz, J., Grimm, J., Van Doren, J., Raabe, E., et al. (2017). Children of prenatally depressed mothers: externalizing and internalizing symptoms are accompanied by reductions in specific social-emotional competencies. J. Child Fam. Stud. 26, 3135–3144. doi: 10.1007/s10826-017-0819-0
Eilertsen, E. M., Gjerde, L. C., Reichborn-Kjennerud, T., Orstavik, R. E., Knudsen, G. P., Stoltenberg, C., et al. (2017). Maternal alcohol use during pregnancy and offspring attention-deficit hyperactivity disorder (ADHD): a prospective sibling control study. Int. J. Epidemiol. 46, 1633–1640. doi: 10.1093/ije/dyx067
Flak, A. L., Su, S., Bertrand, J., Denny, C. H., Kesmodel, U. S., and Cogswell, M. E. (2014). The association of mild, moderate, and binge prenatal alcohol exposure and child neuropsychological outcomes: a meta-analysis. Alcohol. Clin. Exp. Res. 38, 214–226. doi: 10.1111/acer.12214
Floreani, N. A., Rump, T. J., Abdul Muneer, P. M., Alikunju, S., Morsey, B. M., Brodie, M. R., et al. (2010). Alcohol-induced interactive phosphorylation of Src and toll-like receptor regulates the secretion of inflammatory mediators by human astrocytes. J. Neuroimmune Pharmacol. 5, 533–545. doi: 10.1007/s11481-010-9213-z
Fortin, J. P., Fertig, E., and Hansen, K. (2014). shinyMethyl: interactive quality control of Illumina 450k DNA methylation arrays in R. F1000Res. 3:175. doi: 10.12688/f1000research.4680.2
Furtado, E. F., and Roriz, S. T. (2016). Inattention and impulsivity associated with prenatal alcohol exposure in a prospective cohort study with 11-years-old Brazilian children. Eur. Child Adolesc. Psychiatry 25, 1327–1335. doi: 10.1007/s00787-016-0857-y
Geißler, R. (1994). Soziale Schichtung und Lebenschancen in Deutschland. Stuttgart: Ferdinand Enke Verlag. doi: 10.1515/9783110508925
Girirajan, S., Dennis, M. Y., Baker, C., Malig, M., Coe, B. P., Campbell, C. D., et al. (2013). Refinement and discovery of new hotspots of copy-number variation associated with autism spectrum disorder. Am. J. Hum. Genet. 92, 221–237. doi: 10.1016/j.ajhg.2012.12.016
Goecke, T. W., Burger, P., Fasching, P. A., Bakdash, A., Engel, A., Häberle, L., et al. (2014). Meconium indicators of maternal alcohol abuse during pregnancy and association with patient characteristics. Biomed. Res. Int. 2014:702848. doi: 10.1155/2014/702848
Gonzalez-Aramburu, I., Sanchez-Juan, P., Jesus, S., Gorostidi, A., Fernandez-Juan, E., Carrillo, F., et al. (2013). Genetic variability related to serum uric acid concentration and risk of Parkinson’s disease. Mov. Disord. 28, 1737–1740. doi: 10.1002/mds.25507
Gratton, G., Coles, M. G., and Donchin, E. (1983). A new method for off-line removal of ocular artifact. Electroencephalogr. Clin. Neurophysiol. 55, 468–484. doi: 10.1016/0013-4694(83)90135-9
Grob, A., Meyer, C. S., and Hagmann-Von Arx, P. (2009). Intelligence and Development Scales. Intelligenz- und Entwicklungsskalen für Kinder von 5-10 Jahren. Bern: Hogrefe.
Hayes, A. F. (2013). Introduction to Mediation, Moderation, and Conditional Process Analysis: A Regression-Based Approach. New York, NY: Guilford Press.
Hein, A., Rauh, C., Engel, A., Häberle, L., Dammer, U., Voigt, F., et al. (2014). Socioeconomic status and depression during and after pregnancy in the Franconian Maternal Health Evaluation Studies (FRAMES). Arch. Gynecol. Obstet. 289, 755–763. doi: 10.1007/s00404-013-3046-y
Heinrich, H., Grunitz, J., Stonawski, V., Frey, S., Wahl, S., Albrecht, B., et al. (2017). Attention, cognitive control and motivation in ADHD: Linking event-related brain potentials and DNA methylation patterns in boys at early school age. Sci. Rep. 7:3823. doi: 10.1038/s41598-017-03326-3
Himes, S. K., Dukes, K. A., Tripp, T., Petersen, J. M., Raffo, C., Burd, L., et al. (2015). Clinical sensitivity and specificity of meconium fatty acid ethyl ester, ethyl glucuronide, and ethyl sulfate for detecting maternal drinking during pregnancy. Clin. Chem. 61, 523–532. doi: 10.1373/clinchem.2014.233718
Horvath, S. (2013). DNA methylation age of human tissues and cell types. Genome Biol. 14:R115. doi: 10.1186/gb-2013-14-10-r115
Hoyme, H. E., Kalberg, W. O., Elliott, A. J., Blankenship, J., Buckley, D., Marais, A. S., et al. (2016). Updated clinical guidelines for diagnosing fetal alcohol spectrum disorders. Pediatrics 138:e20154256. doi: 10.1542/peds.2015-4256
Huang da, W., Sherman, B. T., and Lempicki, R. A. (2009a). Bioinformatics enrichment tools: paths toward the comprehensive functional analysis of large gene lists. Nucleic Acids Res. 37, 1–13. doi: 10.1093/nar/gkn923
Huang da, W., Sherman, B. T., and Lempicki, R. A. (2009b). Systematic and integrative analysis of large gene lists using DAVID bioinformatics resources. Nat. Protoc. 4, 44–57. doi: 10.1038/nprot.2008.211
Infante, M. A., Moore, E. M., Nguyen, T. T., Fourligas, N., Mattson, S. N., and Riley, E. P. (2015). Objective assessment of ADHD core symptoms in children with heavy prenatal alcohol exposure. Physiol. Behav. 148, 45–50. doi: 10.1016/j.physbeh.2014.10.014
Johnson, R. J., Gold, M. S., Johnson, D. R., Ishimoto, T., Lanaspa, M. A., Zahniser, N. R., et al. (2011). Attention-deficit/hyperactivity disorder: is it time to reappraise the role of sugar consumption? Postgrad. Med. 123, 39–49. doi: 10.3810/pgm.2011.09.2458
Jones, K. L., and Smith, D. W. (1973). Recognition of the fetal alcohol syndrome in early infancy. Lancet 302, 999–1001. doi: 10.1016/S0140-6736(73)91092-1
Kingdon, D., Cardoso, C., and Mcgrath, J. J. (2016). Research review: executive function deficits in fetal alcohol spectrum disorders and attention-deficit/hyperactivity disorder - a meta-analysis. J. Child Psychol. Psychiatry 57, 116–131. doi: 10.1111/jcpp.12451
Kleiber, M. L., Laufer, B. I., Wright, E., Diehl, E. J., and Singh, S. M. (2012). Long-term alterations to the brain transcriptome in a maternal voluntary consumption model of fetal alcohol spectrum disorders. Brain Res. 1458, 18–33. doi: 10.1016/j.brainres.2012.04.016
Kleiber, M. L., Mantha, K., Stringer, R. L., and Singh, S. M. (2013). Neurodevelopmental alcohol exposure elicits long-term changes to gene expression that alter distinct molecular pathways dependent on timing of exposure. J. Neurodev. Disord. 5:6. doi: 10.1186/1866-1955-5-6
Knopik, V. S., Heath, A. C., Jacob, T., Slutske, W. S., Bucholz, K. K., Madden, P. A., et al. (2006). Maternal alcohol use disorder and offspring ADHD: disentangling genetic and environmental effects using a children-of-twins design. Psychol. Med. 36, 1461–1471. doi: 10.1017/S0033291706007884
Knopik, V. S., Jacob, T., Haber, J. R., Swenson, L. P., and Howell, D. N. (2009). Paternal alcoholism and offspring ADHD problems: a children of twins design. Twin Res. Hum. Genet. 12, 53–62. doi: 10.1375/twin.12.1.53
Knopik, V. S., Marceau, K., Bidwell, L. C., Palmer, R. H., Smith, T. F., Todorov, A., et al. (2016). Smoking during pregnancy and ADHD risk: A genetically informed, multiple-rater approach. Am. J. Med. Genet. B Neuropsychiatr. Genet. 171, 971–981. doi: 10.1002/ajmg.b.32421
Lange, S., Shield, K., Koren, G., Rehm, J., and Popova, S. (2014). A comparison of the prevalence of prenatal alcohol exposure obtained via maternal self-reports versus meconium testing: a systematic literature review and meta-analysis. BMC Pregnancy Childbirth 14:127. doi: 10.1186/1471-2393-14-127
Lasky-Su, J., Neale, B. M., Franke, B., Anney, R. J., Zhou, K., Maller, J. B., et al. (2008). Genome-wide association scan of quantitative traits for attention deficit hyperactivity disorder identifies novel associations and confirms candidate gene associations. Am. J. Med. Genet. B Neuropsychiatr. Genet. 147B, 1345–1354. doi: 10.1002/ajmg.b.30867
Laufer, B. I., Kapalanga, J., Castellani, C. A., Diehl, E. J., Yan, L., and Singh, S. M. (2015). Associative DNA methylation changes in children with prenatal alcohol exposure. Epigenomics 7, 1259–1274. doi: 10.2217/epi.15.60
Laurent, L., Wong, E., Li, G., Huynh, T., Tsirigos, A., Ong, C. T., et al. (2010). Dynamic changes in the human methylome during differentiation. Genome Res. 20, 320–331. doi: 10.1101/gr.101907.109
Lehne, B., Drong, A. W., Loh, M., Zhang, W., Scott, W. R., Tan, S. T., et al. (2015). A coherent approach for analysis of the Illumina HumanMethylation450 BeadChip improves data quality and performance in epigenome-wide association studies. Genome Biol. 16:37. doi: 10.1186/s13059-015-0600-x
Lim, J. W., Donahoo, A. L., Bunt, J., Edwards, T. J., Fenlon, L. R., Liu, Y., et al. (2015). EMX1 regulates NRP1-mediated wiring of the mouse anterior cingulate cortex. Development 142, 3746–3757. doi: 10.1242/dev.119909
Lin, A., Wang, R. T., Ahn, S., Park, C. C., and Smith, D. J. (2010). A genome-wide map of human genetic interactions inferred from radiation hybrid genotypes. Genome Res. 20, 1122–1132. doi: 10.1101/gr.104216.109
Lugo, J. N., Brewster, A. L., Spencer, C. M., and Anderson, A. E. (2012). Kv4.2 knockout mice have hippocampal-dependent learning and memory deficits. Learn. Mem. 19, 182–189. doi: 10.1101/lm.023614.111
Lussier, A. A., Morin, A. M., Macisaac, J. L., Salmon, J., Weinberg, J., Reynolds, J. N., et al. (2018). DNA methylation as a predictor of fetal alcohol spectrum disorder. Clin Epigenet. 10:5. doi: 10.1186/s13148-018-0439-6
Lussier, A. A., Weinberg, J., and Kobor, M. S. (2017). Epigenetics studies of fetal alcohol spectrum disorder: where are we now? Epigenomics 9, 291–311. doi: 10.2217/epi-2016-0163
Machtinger, R., Zhong, J., Mansur, A., Adir, M., Racowsky, C., Hauser, R., et al. (2018). Placental lncRNA expression is associated with prenatal phthalate exposure. Toxicol. Sci. 163, 116–122. doi: 10.1093/toxsci/kfy013
Mallon, B. S., Chenoweth, J. G., Johnson, K. R., Hamilton, R. S., Tesar, P. J., Yavatkar, A. S., et al. (2013). StemCellDB: the human pluripotent stem cell database at the National Institutes of Health. Stem. Cell Res. 10, 57–66. doi: 10.1016/j.scr.2012.09.002
Marshall, C. R., Noor, A., Vincent, J. B., Lionel, A. C., Feuk, L., Skaug, J., et al. (2008). Structural variation of chromosomes in autism spectrum disorder. Am. J. Hum. Genet. 82, 477–488. doi: 10.1016/j.ajhg.2007.12.009
Mattson, S. N., and Riley, E. P. (2000). Parent ratings of behavior in children with heavy prenatal alcohol exposure and IQ-matched controls. Alcohol. Clin. Exp. Res. 24, 226–231. doi: 10.1111/j.1530-0277.2000.tb04595.x
Maunakea, A. K., Nagarajan, R. P., Bilenky, M., Ballinger, T. J., D’souza, C., Fouse, S. D., et al. (2010). Conserved role of intragenic DNA methylation in regulating alternative promoters. Nature 466, 253–257. doi: 10.1038/nature09165
McBride, W. J., Kimpel, M. W., Mcclintick, J. N., Ding, Z. M., Hauser, S. R., Edenberg, H. J., et al. (2013). Changes in gene expression within the ventral tegmental area following repeated excessive binge-like alcohol drinking by alcohol-preferring (P) rats. Alcohol 47, 367–380. doi: 10.1016/j.alcohol.2013.04.002
Mick, E., Biederman, J., Faraone, S. V., Sayer, J., and Kleinman, S. (2002). Case-control study of attention-deficit hyperactivity disorder and maternal smoking, alcohol use, and drug use during pregnancy. J. Am. Acad. Child Adolesc. Psychiatry 41, 378–385. doi: 10.1097/00004583-200204000-00009
Murgatroyd, C., and Spengler, D. (2012). Genetic variation in the epigenetic machinery and mental health. Curr. Psychiatry Rep. 14, 138–149. doi: 10.1007/s11920-012-0255-1
Murgatroyd, C. A., Taliefar, M., Bradburn, S., Carini, L. M., Babb, J. A., and Nephew, B. C. (2015). Social stress during lactation, depressed maternal care, and neuropeptidergic gene expression. Behav. Pharmacol. 26, 642–653. doi: 10.1097/FBP.0000000000000147
Palmer, R. B. (2009). A review of the use of ethyl glucuronide as a marker for ethanol consumption in forensic and clinical medicine. Semin. Diagn. Pathol. 26, 18–27. doi: 10.1053/j.semdp.2008.12.005
Pidsley, R., Wong, Y., Volta, M., Lunnon, K., Mill, J., and Schalkwyk, L. C. (2013). A data-driven approach to preprocessing Illumina 450K methylation array data. BMC Genomics 14:293. doi: 10.1186/1471-2164-14-293
Pollard, I. (2007). Neuropharmacology of drugs and alcohol in mother and fetus. Semin. Fetal Neonatal Med. 12, 106–113. doi: 10.1016/j.siny.2006.12.001
Portales-Casamar, E., Lussier, A. A., Jones, M. J., Macisaac, J. L., Edgar, R. D., Mah, S. M., et al. (2016). DNA methylation signature of human fetal alcohol spectrum disorder. Epigenet. Chromatin 9:25. doi: 10.1186/s13072-016-0074-4
Qiu, M., Anderson, S., Chen, S., Meneses, J. J., Hevner, R., Kuwana, E., et al. (1996). Mutation of the Emx-1 homeobox gene disrupts the corpus callosum. Dev. Biol. 178, 174–178. doi: 10.1006/dbio.1996.0207
Reulbach, U., Bleich, S., Knorr, J., Burger, P., Fasching, P. A., Kornhuber, J., et al. (2009). [Pre-, peri- and postpartal depression]. Fortsch. Neurol. Psychiat. 77, 708–713. doi: 10.1055/s-0028-1109822
Rojas-Mayorquin, A. E., Padilla-Velarde, E., and Ortuno-Sahagun, D. (2016). Prenatal alcohol exposure in rodents as a promising model for the study of ADHD molecular basis. Front. Neurosci. 10:565. doi: 10.3389/fnins.2016.00565
Roth, R. B., Hevezi, P., Lee, J., Willhite, D., Lechner, S. M., Foster, A. C., et al. (2006). Gene expression analyses reveal molecular relationships among 20 regions of the human CNS. Neurogenetics 7, 67–80. doi: 10.1007/s10048-006-0032-6
Schneider, M., Engel, A., Fasching, P. A., Häberle, L., Binder, E. B., Voigt, F., et al. (2014). Genetic variants in the genes of the stress hormone signalling pathway and depressive symptoms during and after pregnancy. Biomed. Res. Int. 2014:469278. doi: 10.1155/2014/469278
Song, C., and Zhang, H. (2014). TARV: tree-based analysis of rare variants identifying risk modifying variants in CTNNA2 and CNTNAP2 for alcohol addiction. Genet. Epidemiol. 38, 552–559. doi: 10.1002/gepi.21843
Spronk, M., Jonkman, L. M., and Kemner, C. (2008). Response inhibition and attention processing in 5- to 7-year-old children with and without symptoms of ADHD: an ERP study. Clin. Neurophysiol. 119, 2738–2752. doi: 10.1016/j.clinph.2008.09.010
Suhre, K., Shin, S. Y., Petersen, A. K., Mohney, R. P., Meredith, D., Wagele, B., et al. (2011). Human metabolic individuality in biomedical and pharmaceutical research. Nature 477, 54–60. doi: 10.1038/nature10354
Sutin, A. R., Cutler, R. G., Camandola, S., Uda, M., Feldman, N. H., Cucca, F., et al. (2014). Impulsivity is associated with uric acid: evidence from humans and mice. Biol. Psychiatry 75, 31–37. doi: 10.1016/j.biopsych.2013.02.024
Tulisiak, C. T., Harris, R. A., and Ponomarev, I. (2017). DNA modifications in models of alcohol use disorders. Alcohol 60, 19–30. doi: 10.1016/j.alcohol.2016.11.004
Warde-Farley, D., Donaldson, S. L., Comes, O., Zuberi, K., Badrawi, R., Chao, P., et al. (2010). The GeneMANIA prediction server: biological network integration for gene prioritization and predicting gene function. Nucleic Acids Res. 38, W214–W220. doi: 10.1093/nar/gkq537
Woo, H. M., Park, H. J., Park, M. H., Kim, B. Y., Shin, J. W., Yoo, W. G., et al. (2014). Identification of CDH23 mutations in Korean families with hearing loss by whole-exome sequencing. BMC Med. Genet. 15:46. doi: 10.1186/1471-2350-15-46
Wu, H., Romieu, I., Shi, M., Hancock, D. B., Li, H., Sienra-Monge, J. J., et al. (2010). Evaluation of candidate genes in a genome-wide association study of childhood asthma in Mexicans. J. Allergy Clin. Immunol. 125, 321–327.e13. doi: 10.1016/j.jaci.2009.09.007
Yildirim, O., Li, R., Hung, J. H., Chen, P. B., Dong, X., Ee, L. S., et al. (2011). Mbd3/NURD complex regulates expression of 5-hydroxymethylcytosine marked genes in embryonic stem cells. Cell 147, 1498–1510. doi: 10.1016/j.cell.2011.11.054
Keywords: prenatal alcohol exposure, whole-genome DNA methylation, dipeptidyl peptidase 10 (DPP10), SLC16A9, attention, ERP, IQ, FRANCES
Citation: Frey S, Eichler A, Stonawski V, Kriebel J, Wahl S, Gallati S, Goecke TW, Fasching PA, Beckmann MW, Kratz O, Moll GH, Heinrich H, Kornhuber J and Golub Y (2018) Prenatal Alcohol Exposure Is Associated With Adverse Cognitive Effects and Distinct Whole-Genome DNA Methylation Patterns in Primary School Children. Front. Behav. Neurosci. 12:125. doi: 10.3389/fnbeh.2018.00125
Received: 18 December 2017; Accepted: 06 June 2018;
Published: 26 June 2018.
Edited by:
Liana Fattore, Consiglio Nazionale delle Ricerche (CNR), ItalyReviewed by:
David Peter Gavin, Jesse Brown VA Medical Center, United StatesDaniel Ortuño-Sahagún, Universidad de Guadalajara, Mexico
Copyright © 2018 Frey, Eichler, Stonawski, Kriebel, Wahl, Gallati, Goecke, Fasching, Beckmann, Kratz, Moll, Heinrich, Kornhuber and Golub. This is an open-access article distributed under the terms of the Creative Commons Attribution License (CC BY). The use, distribution or reproduction in other forums is permitted, provided the original author(s) and the copyright owner are credited and that the original publication in this journal is cited, in accordance with accepted academic practice. No use, distribution or reproduction is permitted which does not comply with these terms.
*Correspondence: Stefan Frey, stefan.frey@uk-erlangen.de
†Co-senior-authorship