- 1School of Medical Sciences, Edith Cowan University, Joondalup, WA, Australia
- 2The McCusker Alzheimer's Research Foundation, Nedlands, WA, Australia
- 3School of Psychiatry and Clinical Neurosciences, University of Western Australia, Crawley, Australia
- 4The School of Animal Biology, University of Western Australia, Crawley, WA, Australia
- 5School of Psychology, University of Western Australia, Crawley, WA, Australia
- 6School of Pathology and Laboratory Medicine, University of Western Australia, Nedlands, WA, Australia
- 7PathWest Laboratory Medicine of WA, Nedlands, WA, Australia
- 8Western Medicine, Hollywood Specialist Centre, Nedlands, WA, Australia
- 9School of Public Health, Curtin University of Technology, Perth, WA, Australia
- 10Division of Immunology, Department of Developmental Neurobiolog,Institute for Basic Research in Developmental Disabilities, Staten Island, NY, USA
- 11Neurosciences Unit, Health Department of WA, School of Psychology and Speech Pathology, Curtin University of Technology, Perth, WA, Australia
- 12Academic Unit for Psychiatry of Old Age, St. Vincent's Health, Department of Psychiatry, University of Melbourne, Parkville, VIC, Australia
- 13The WA Centre for Health and Ageing, University of Western Australia, Crawley, Australia
- 14Institute for Immunology and Infectious Diseases, Murdoch University, WA, Australia
Cognitive decline and dementia due to Alzheimer's disease (AD) have been associated with genetic, lifestyle, and environmental factors. A number of potentially modifiable risk factors should be taken into account when preventive or ameliorative interventions targeting dementia and its preclinical stages are investigated. Bone mineral density (BMD) and body composition are two such potentially modifiable risk factors, and their association with cognitive decline was investigated in this study. 164 participants, aged 34–87 years old (62.78 ± 9.27), were recruited for this longitudinal study and underwent cognitive and clinical examinations at baseline and after 3 years. Blood samples were collected for apolipoprotein E (APOE) genotyping and dual energy x-ray absorptiometry (DXA) was conducted at the same day as cognitive assessment. Using hierarchical regression analysis, we found that BMD and lean body mass, as measured using DXA were significant predictors of episodic memory. Age, gender, APOE status, and premorbid IQ were controlled for. Specifically, the List A learning from California Verbal Learning Test was significantly associated with BMD and lean mass both at baseline and at follow up assessment. Our findings indicate that there is a significant association between BMD and lean body mass and episodic verbal learning. While the involvement of modifiable lifestyle factors in human cognitive function has been examined in different studies, there is a need for further research to understand the potential underlying mechanisms.
Introduction
Dementia is a major debilitating disorder and a cause of significant concern for the currently aging population. In 2010, more than 35.6 million individuals were diagnosed with dementia worldwide (Prince et al., 2013). Prevalence projections indicate that dementia cases will dramatically increase worldwide by 2050 (Norton et al., 2014). In particular, a report from the Australian Institute of Health and Welfare estimates that the number of dementia patients in Australia will increase from 175,000 to 465,000 by the year 2031 (Australian Institute of Health and Welfare, 2007). Preventative research to reduce dementia-related burden is essential to tackle the financial as well as social consequences of this condition. Based on recent modeling reported by Alzheimer's Australia, if the onset of dementia was delayed by 2 years, a reduction of 13% or 398,000 cumulative new cases by 2050 would be achieved. Further, a delay of 5 years would reduce the number of cumulative new cases by 30%, or 935,000 individuals by 2050. Dementia-prevention programs would have a significant economic impact and improve the quality of life for affected individuals and their family (Vickland et al., 2012). Identification of potentially modifiable risk factors, including lifestyle factors, is a promising avenue for facilitating reductions in dementia incidence.
Dementia due to Alzheimer's disease (AD) is the most common form of dementia worldwide (Di Carlo et al., 2012). While the underlying causes of the late-onset form of the disease remain poorly understood, a complex mix of genetic, lifestyle, and hormonal factors is thought to contribute to the cerebral accumulation of a small peptide, beta amyloid (Aβ) (Butterfield et al., 2002; Isacson et al., 2002; Verdile et al., 2004; Wirths et al., 2004), resulting in the formation of extracellular amyloid deposits (Glenner and Wong, 1984; Masters et al., 1985). Research suggests that one third of AD cases are preventable (Norton et al., 2014). Because lifestyle and hormonal factors are potentially modifiable risk factors for AD, they remain a focus of intense research scrutiny. One such hormone-related risk-factor is osteoporosis, which is defined as bone mineral density (BMD) more than 2.5 standard deviations below the mean for healthy adults aged between 20 and 40 years (W.H.O, 1994). The prevalence of osteoporosis increases with age. According to Osteoporosis Australia, 1 in 2 women and 1 in 3 men over the age of 60 will experience an osteoporotic fracture. In addition to age, female sex and menopause-related changes, previous fragility, previous fragility fractures, family history of hip fracture, and the use of oral corticosteroids are also significant risk factors for low BMD (Kanis, 2002; Finkelstein et al., 2008).
Osteoporosis and low BMD (osteopenia) have been associated with cognitive impairment and dementia (Lui et al., 2003; Rothman et al., 2007). BMD is regulated through the brain (Haberland et al., 2001; Karsenty and Oury, 2010), and this may partially explain the underlying relationship between BMD, cognitive dysfunction, and dementia. The brain regions involved in adiposity, (specifically the hypothalamus), also regulate bone remodeling through complicated and slow processes involving hormones including leptin (Haberland et al., 2001; Crockett et al., 2011). Leptin is thought to mediate BMD via binding to relevant receptors in the ventromedial hypothalamus (Haberland et al., 2001; Yang and Barouch, 2007) suggesting that osteoporosis may represent a neuro-skeletal condition (Takeda, 2009). Of note, plasma leptin level has been negatively associated with dementia and AD risk (Lieb et al., 2009). Additionally, the relationship between lower BMD and dementia may be modulated through cumulative exposure to estrogen as it was found in the Framingham Study, that lower femoral neck BMD was associated with a two-fold increase in risk of AD in women, potentially due to estrogen exposure (Tan et al., 2005).
Adiposity or body fat is another potentially modifiable risk factor associated with cognitive decline and dementia; however, research has produced somewhat conflicting results in this area. While most studies have supported a significant association between adiposity and cognitive decline (Luchsinger et al., 2007; Kerwin et al., 2011) other studies have failed to identify a significant relationship between these two on some of the cognitive functions associated with AD, including verbal memory (Wolf et al., 2007). In some studies, adiposity has been associated with cognitive decline only in men (Kanaya et al., 2009), in individuals above 70 years old (Levine and Crimmins, 2012), or in participants below age 70 (Yoon et al., 2012). Adiposity is a risk factor for diabetes, hypertension, and cardiovascular changes; conditions which themselves contribute to significantly increased risk of AD (for a review see: Gustafson and Luchsinger, 2013) and cognitive decline due to vascular pathologies (Gustafson, 2012). For example, it has been suggested that adiposity, as a risk factor for insulin resistance and hyperinsulinemia may increase amyloid deposits in the brain resulting in AD (Luchsinger and Mayeux, 2007). In sum, the available evidence suggests that midlife central obesity plays a significant role in age-related cognitive decline and significantly increases the risk of dementia (Whitmer et al., 2008).
It is important to note that both increased adiposity and osteoporosis have been associated with cardiovascular disease (CVD) (Banks et al., 1994), which is associated with AD-plasma amyloid-β protein (Bates et al., 2009) and has been shown to increase the risk of cognitive decline and dementia (Qiu et al., 2010; Norton et al., 2014). Interestingly, subclinical CVD increases the risk of bone loss and fracture (den Uyl et al., 2011) and BMD has been inversely associated with CVD (Farhat et al., 2007). Further, cardiovascular problems are associated with osteoporosis; moreover, lipid-related problems may play a role in increasing osteoporosis risk (Brown and Sharpless, 2004). Animal models support the association between osteoporosis and atherosclerosis (Parhami et al., 2000; Price et al., 2001). Observational studies have shown that higher atherogenic lipid profile and lipoproteins are inversely associated with bone density (Dimic et al., 2012; Sarkis et al., 2012) but the exact mechanisms underlying this relationship are unclear (Farhat and Cauley, 2008).
Cholesterol metabolism has been linked to apolipoprotein E epsilon 4 allele (APOE ε 4), a major genetic risk factor for late-onset AD (Corder et al., 1993; Saunders et al., 1993; Roses, 1997). The ApoE protein is the major cholesterol transport protein in the brain, with allelic polymorphism in the APOE gene resulting in isoform-specific functional effects (e.g., higher risk of AD for ε 4 carriers and more resistance to AD in ε 2 carriers) (Weisgraber, 1994; Mahley et al., 1996). Some studies have indicated that, in addition to increased risk of AD, APOE can also be involved in osteoporosis through mediating vitamin K transportation and/or inhibition of osteoblast differentiation (Kohlmeier et al., 1996; Parhami et al., 1997). However, a more recent study did not support the involvement of APOE genotype in BMD, increased bone loss, or higher risk of osteoporotic fractures (Schoofs et al., 2004).
The current study evaluated the relationship between adiposity, BMD and subsequent cognitive decline with respect to both screening of functional capacity and, more specifically, verbal episodic memory. We assessed BMD, adiposity and cognition, controlling for the potential effects of age, gender, and possession of the APOE ε 4 allele. Specific hypotheses included: (i) higher BMD would be significantly associated with better current and future cognitive functioning, particularly verbal memory; (ii) higher adiposity and lower lean body mass would be related to current cognitive function and predict subsequent cognitive function.
Materials and Methods
Study Design and Cohort Selection
One hundred and sixty four participants aged 34–87 years old (62.78 ± 9.27) were recruited from a larger, longitudinal, community-based study (the Western Australia Memory Study), investigating molecular and neuropsychological predictors of cognitive decline within younger and older adults (Clarnette et al., 2001; Sohrabi et al., 2009). The results were analyzed at baseline and after a 3-year follow-up. Participants completed annual blood and cognitive testing using standardized, validated screening and diagnostic measures. Exclusion criteria at the recruitment included: Mini Mental State Examination (MMSE) score ≤ 24 (Folstein et al., 1975); clinically diagnosed dementia; untreated depression [Geriatric Depression Scale (GDS) score ≥ 11]; history of neurological or psychiatric disorders affecting cognitive functions (e.g., stroke, Parkinson's disease, epilepsy, schizophrenia) and difficulty understanding or speaking English.
All participants provided written, informed consent to the study procedures including a body composition/BMD scan, using dual energy x-ray absorptiometry (DXA). Cognitive and clinical assessments, along with venous blood sampling occurred on the same day as DXA was performed. The study was approved by the Human Ethics Committees of Edith Cowan University, University of Western Australia, and Hollywood Private Hospital, Western Australia.
DXA Analysis
The DXA technique quantifies bone mineral content by comparing the attenuation that occurs as a result of absorption of photons at two different energy levels, thereby creating a two dimensional BMD (aBMD) map (Van Loan and Mayclin, 1992). This analysis allows for the separation of body mass into bone, lean, and fat components.
In this study, DXA bone density and body composition scans were conducted on a central, whole body, Norland XR-46, pencil beam scanner using software version 4.1.1. The instrument was calibrated daily using a 77-step calibration standard QC phantom. The mean coefficient of variation (over 5 days) was 0.40 for BMD, 0.20 for lean mass and 0.14 for fat mass. Bone density measures were taken at the spine (L2-L4) and at the hip (femoral neck and total hip). The software generated: (i) t-scores, which compared each individual against a group, defined as possessing peak bone mass (i.e., normative data from healthy adults aged 20–40 years); and (ii) z-scores which compared an individual with data from their own age group.
Whole body scans were divided into regions of interest including head, chest, midriff, pelvis, and limbs. The measures taken included fat and lean mass (in kg), total body fat percentage, Siri formula, and Brozek formula, for underwater weight equivalents (UWE) (Guerra et al., 2010). To estimate body fat %, body mass density is calculated and converted to body fat %, using the Siri or Brozek equations. These are the most commonly equations available (Guerra et al., 2010).
Most of the scores produced by DXA are highly inter-correlated. In order to address potential multicollinearity, we created composite, sample-based z scores derived from the sum of all the z scores calculated for lean mass and BMD raw scores, divided by the number of scores. The following raw scores were converted to z scores and divided by six (number of scores) to compute the composite lean mass z score: Midriff lean mass + Pelvis lean mass + Left leg lean mass + Right leg lean mass + Left arm lean mass + Right arm lean mass. The bone density composite z score was calculated by summing the DXA Spine L2-L4, Femoral Neck, and trochanter computed z scores divided by three. We did not use a Fat mass composite z score, but instead used the Siri UWE as this score is strongly associated with fat % and other fat mass-related scores derived from DXA.
Clinical and Cognitive Measures
Participants completed a comprehensive set of clinical and neuropsychological assessments lasting between 1.5 and 2.5 h and were offered breaks as needed. Depression at baseline was measured using the GDS (Yesavage et al., 1982). Premorbid cognitive ability was assessed using the Cambridge Contextual Reading Test (CCRT) (Beardsall, 1998). General cognitive functioning was assessed using the CAMCOG-R (Roth et al., 1998). Verbal episodic memory was assessed using the California Verbal Learning Test (CVLT) (Delis et al., 1988). Baseline and 3-year follow-up scores for the CVLT were calculated as follows: List Learning (List A; trials 1–5 total score), short delay free recall (SDFR), short delay cued recall (SDCR), long delay free recall (LDFR), long delay cued recall (LDCR), and recognition discriminability (RecDisc).
Biochemical and Genetic Analysis
On the same day as the DXA scan and cognitive/clinical assessment, a fasted venous blood sample was collected into serum, EDTA (containing prostaglandin E to prevent platelet activation) and heparin blood collection tubes (Interpath Services, Australia). The whole blood was then separated into different components using standard centrifugation techniques. DNA was isolated from leukocytes, and APOE genotype was determined via polymerase chain reaction (PCR) amplification and restriction enzyme digestion using the method originally described by Hixson and Vernier (1990), and outlined in Laws et al. (2002).
Statistical Analysis
Data were entered into Microsoft Excel and statistical analyses conducted using IBM SPSS Version 19 (IBM SPSS Statistics, 2010 New York, IBM Corp). After testing for normality, descriptive sample characteristics were analyzed. Next, a series of two-step hierarchical linear regressions were conducted in order to explore the relationships between biological variables, general cognitive function (as assessed by the CAMCOG-R) and episodic verbal memory (as measured by the above-mentioned CVLT sub scores). In step one, potential covariates including age at scan time, gender, APOE ε 4 allele status, and premorbid IQ (CCRT) were entered into the analysis. In step 2, variables derived from DXA were entered into the model. These variables included (1) Siri UWE Fat percentage, (2) Lean mass composite score, and (3) BMD composite score.
Results
In the current study, participants included 69% women and 38% of participants were APOE ε 4 carriers. Descriptive data for males/females, and APOE ε 4 +/APOE ε 4 – groups are presented in Tables 1, 2. The percentage of participants who were APOE ε 4 + did not differ by gender, χ2(1; N = 162) = 0.010, p = 0.919. There were significant differences between men and women on most of the DXA measures. The differences are presented in Table 2 with respect to gender and APOE ε 4 status. APOE ε 4 status and GDS (depression level) were not significantly associated with any of the DXA or cognitive variables.
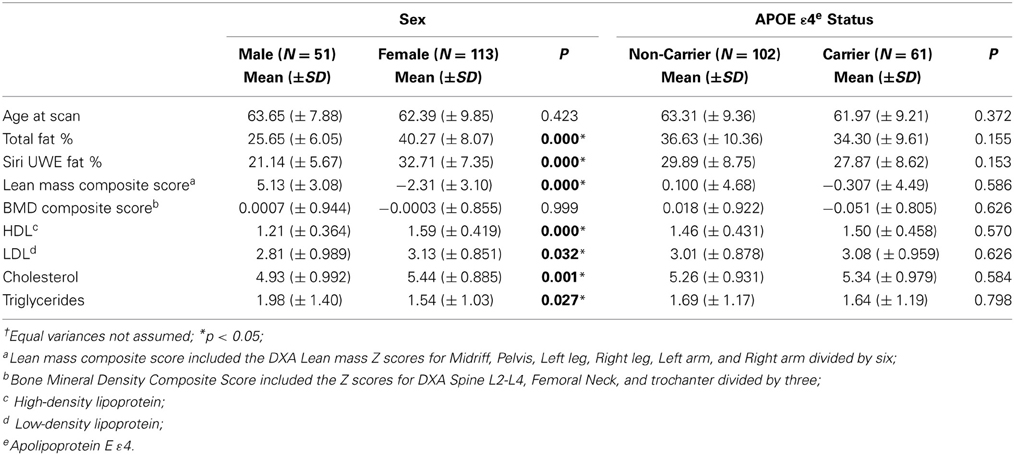
Table 1. Descriptive findings of dual energy x-ray absorptiometry (DXA) including the p-values corresponding to independent t†.
Correlations
Table 3 presents the correlations between DXA, adiposity, and cognitive measures at baseline and follow up. Premorbid IQ (as measured using the CCRT) was not associated with any of the DXA measures, but was a significant correlate of CAMCOG Baseline and FU scores, and CVLT SDCR and RecD follow up scores. GDS score was also not significantly associated with any of the DXA or other biomarkers and therefore was not included as a covariate in regression analyses. However, we found significant associations between overall cognitive status, as measured by CAMCOG-R, and various DXA measures. A significant, negative association was found between baseline CAMCOG-R and Siri UWE (r = −0.204, p < 0.01). Lean mass and BMD composite scores were positively associated with verbal learning (CVLT scores) and general cognitive functioning (CAMCOG-R) (Table 3) and therefore included in the subsequent regression analyses. HDL was significantly associated with baseline CVLT subscales including, SDFR (r = 0.195, p < 0.05), SDCR (r = 0.160, p < 0.05), and LDCR (r = 0.162, p < 0.05) but not with the CVLT follow up results except for SDCR (r = 0.184, p < 0.05). Interestingly, HDL was negatively associated with the total perseveration score for List A learning trials 1–5 (r = −0.160, p < 0.05), indicating that greater perseveration was associated with lower HDL.
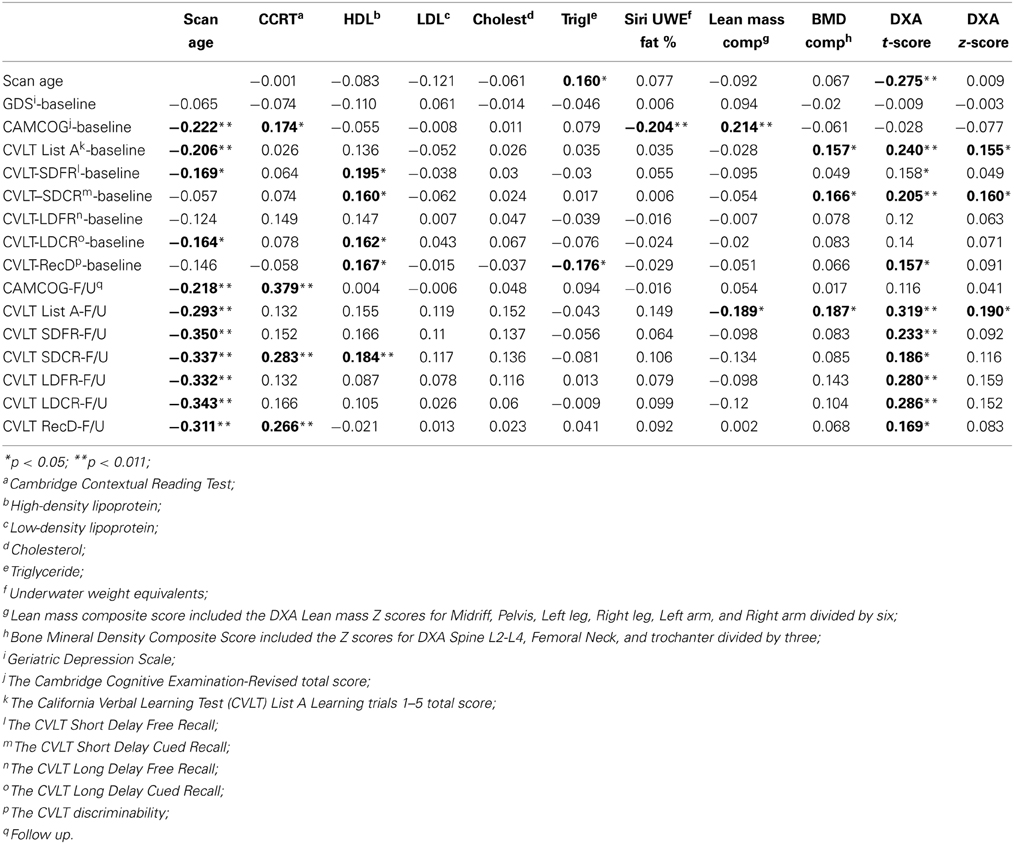
Table 3. Associations between Dual-energy X-ray absorptiometry (DXA), baseline, and follow up cognitive measures (2-tailed).
Clinical and Cognitive Data
Clinical and cognitive data were available for all participants. The mean clinical and cognitive scores for all participants are outlined in Tables 1, 2. The means for all neuropsychological measures were within expected age-related norms (Yesavage et al., 1982; Delis et al., 2000). We did not find any significant differences between APOE ε 4 carriers and non-carriers on any of the DXA or neuropsychological measures. Depression was not significantly associated with any of the DXA measures (Table 3) or with cognitive measures at baseline or follow up.
Regression Analysis
A series of hierarchical multiple regressions was conducted to examine the associations between DXA and adiposity measures and cognitive test results whilst controlling for the effects of relevant covariates at both baseline and follow up assessments. As discussed, demographic factors including age, gender, APOE ε 4 carriage, and premorbid IQ (as measured using the CCRT) were entered in Step 1. In Step 2, variables derived from DXA were entered into the model including the Siri UWE Fat percentage, Lean mass composite score, and the BMD composite score.
Cognitive Results
The DXA scores in our two-step hierarchical regression model were not significantly associated with general cognitive functioning at baseline (R2-change = 0.007; F change = 0.359, p = 0.782) or follow up (R2-change = 0.002; F change = 0.110, p = 0.954). In predicting baseline CVLT List A trials 1–5, the first model containing covariates only was not significant [F(4, 141) = 2.014, p = 0.096, R2 = 0.054]. However, the second model was significant [F(7, 138) = 2.359, p < 0.05, R2 = 0.107]. Specifically, as seen in Table 4, the addition of the DXA variables provided unique predictive variance (R2-change = 0.53). Higher BMD was significantly associated with higher learning scores, and there was a trend for lower Lean mass composite score (B = −0.644; t = −1.926; p = 0.056).
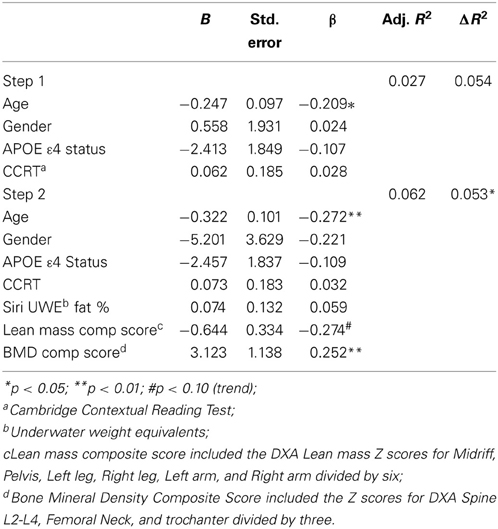
Table 4. Hierarchical linear regression predicting baseline CVLT List A trials 1–5 total score from lean mass and bone density composite scores.
Baseline CVLT discriminability (recognition) performance was not significantly predicted by the first model containing covariates only [F(4, 140) = 1.115, p = 0.352, R2 = 0.031, but the second model was significant, F(7, 137) = 2.422, p < 0.05, R2 = 0.110]. Both lean mass composite score and BMD were significant predictors of RecDisc in the final model, with higher BMD and lower lean mass being associated with better performance (Table 5). Adding DXA variables contributed an additional 8% of predictive variance.
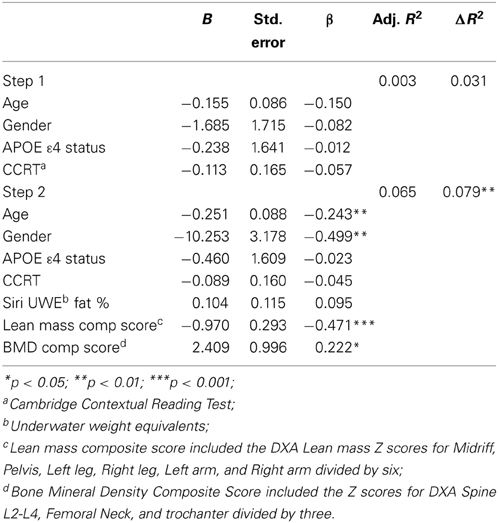
Table 5. Hierarchical linear regression predicting baseline CVLT discriminability score from lean mass and bone density composite scores.
CVLT List A learning at follow-up was also significantly predicted by DXA variables. The first model containing covariates only was significant [F(4, 124) = 6.005, p < 0.001]. The second model was also significant [F(7, 121) = 5.375, p < 0.001, R2 = 0.237]. Specifically, the Lean mass composite score (t = −2.297, p < 0.05), and BMD composite score (t = 3.351, p < 0.001) were significant predictors (Table 6), accounting for an additional 8% of variance.
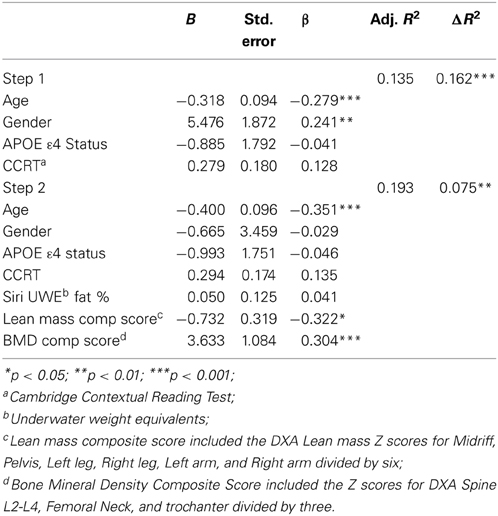
Table 6. Hierarchical linear regression predicting follow up CVLT List A trials 1–5 total score from lean mass and bone density composite scores.
Due to significant differences between men and women on DXA measures, we examined the interaction of gender and DXA measures (i.e., Siri UWE Fat %, lean mass composite score, and BMD composite score) in predicting cognitive functions. The cognitive functions examined here included baseline and follow up CAMCOG-R, List A learning trials 1–5, SDFR, SDCR, LDCR, LDFR, and Recognition Disc results. Interestingly, we did not find any significant results for these interactions except for Gender X BMD on the follow up LDFR [R2-change = 0.052; F change = 2.707, p = 0.048; F(10, 118) = 3.944, p < 0.001; B = −1.302, SE = 0.606, Beta = −0.698; t = −2.150, p < 0.034]. Further analysis indicated that higher BMD was significantly associated with better follow up LDFR in men, but not in women (r = 0.459, p < 0.002 and r = −0.039, p = 0.707, respectively).
Discussion
The results of this longitudinal study lend further support to the mounting body of evidence suggesting that lifestyle factors may be involved in cognitive functions and, in turn, may modulate the risk of future cognitive decline. We report a significant link between potentially modifiable indicators of systemic health, namely BMD and Lean body mass on cognitive performance in a group of community-dwelling, healthy adults. These findings have a number of potential implications for understanding the underlying, modifiable mechanisms involved in pathological cognitive decline and for developing preventive and potentially ameliorative clinical trials targeting AD, specifically with respect to the possible influence of metabolic, cardiovascular, and general health factors.
BMD was a predictor for cognitive performance with respect to CVLT measures (Tables 4–6) in a cross-sectional as well as longitudinal context. Interestingly, the findings from the present study indicate that the effect of APOE ε4 was limited, i.e., we did not observe any significant differences between the APOE ε4 carriers and non-carriers in the study on DXA or cognitive assessment results (Table 1).
In this study, we found significant differences between men and women on BMD and body composition (as expected from previous studies), but we did not find significant differences on cognitive measures at baseline. However, after 3 years of follow up, women outperformed men on most of the CVLT measures (Table 2) but their performance was similar on follow up global cognitive function. This may be consistent with findings implying differential cognitive decline rate in women vs. men (Maylor et al., 2007; Holland et al., 2013).
Low BMD and osteoporosis restrict morbidity amongst the elderly and have been associated to the risk of future cognitive decline (Yaffe et al., 1999; Lui et al., 2003) and dementia due to AD (Tan et al., 2005). Low BMD has been associated with lower cognitive function in cross-sectional study of post-menopausal women (Brownbill and Ilich, 2004) and with verbal memory performance in both men and women (Zhang et al., 2001). It has been suggested that BMD may be reflective of cumulative estrogen exposure, thus providing a molecular mechanism for the link between BMD and cognition as longer estrogen exposure has been associated with lower dementia risk (Fox et al., 2013). However, the evidence supporting a role for estrogen replacement in protecting against AD is currently uncertain due to a number of methodological factors and possible confounding variables including education, general health and physical activity (as reviewed in: Yaffe et al., 1998). As such, the debate surrounding the efficacy of estrogen replacement as a therapeutic target for AD continues.
We found that BMD and lean mass were significantly associated with memory abilities as measured using the CVLT (Tables 4–6). This is consistent with a previous report that BMD and verbal memory impairment were significantly associated (Zhang et al., 2001). Zhang et al. (2001) has postulated that this relationship may be potentially a function of cumulative exposure to estrogen. In fact, our findings, indirectly, support the notion of BMD as a marker of cumulative estrogen exposure, particularly because we observed a significant association between sex and CVLT Learning abilities at follow-up in the present study (Table 2). It should be noted that we did not measure estrogen exposure in this study. However, it has previously been shown that body fat serves as a source of endogenous estrogen in post-menopausal women (Bagger et al., 2004).
The relationship between memory, BMD, and body composition were more significantly pronounced on performance in the List A learning trials. In the current study, BMD and lean mass measures of DXA were significant predictors of CVLT scores representing learning capabilities. This finding is important, as encoding deficits in episodic verbal memory i.e., mental representation of new information–are the primary memory problems seen in preclinical dementia and AD (Golby et al., 2005; Twamley et al., 2006; Beck et al., 2012), and such encoding problems have been shown to correlate with cholinergic deficits that are commonly seen in AD (White and Ruske, 2002). Interestingly, the cholinergic system plays a pivotal role in BMD regulation, as has recently been reviewed (Eimar et al., 2013). These considerations are consistent with our findings discussed earlier concerning the association between learning ability and BMD.
It has been reported that executive functions are associated with episodic memory (Duff et al., 2005). More specifically, the attention component of executive function plays a significant role in verbal learning and memory (Brooks et al., 2006) and has been associated with difficulties in CVLT List A learning (Hill et al., 2012). Our findings show a significant association between List A learning and BMD (as discussed earlier). This may indirectly suggest an underlying mechanism involved in both BMD and executive functioning, although this has to be carefully examined in future studies.
While the current study furthers our understanding of the relationship between age-related memory capacities and BMD, the cohort size and the very wide age range represent the main limitations. In addition, it would be advantageous to have baseline and follow up DXA results, and general morbidity and physical activity to examine the potential differences with negative changes in the DXA results in terms of memory and other cognitive functions. Additionally, examining the sex hormones will add value to a longitudinal study investigating the relationship between DXA scores and cognitive functions.
Further research is warranted investigating the relationship between BMD and cognitive dysfunctions in various dementia patient groups and in preclinical stages, and the potential mechanisms underlying these relationships. Such research could significantly improve our knowledge of the association between bone mineral content and higher cortical capabilities, and the relevance of these factors in aging and dementia. Future research may investigate the relationship between BMD and cognitive decline in aging while controlling for the effects of general mobility and current/previous physical activity as contributing factors.
The non-significant relationship between APOE alleles carriage, BMD and cognition in this study may indicate differential contributing pathways for genetic vs. lifestyle factors. Of course, we requires further research in a larger cohort where various genes identified as contributing to dementia risk can be examined in relation to BMD and cognitive decline measures.
Author Contributions
Study design: HRS, KAB, JKF, SML, and RNM. Study conduct: HRS, KAB, MAR, MH, KT, GM, AP, JKF. Data collection: HRS, KAB, MAR, AP, GM. Data analysis: HRS, KAB, SSD, MW. Data interpretation: HRS, KAB, TS, RSB. Drafting manuscript: HRS, KAB. Revising manuscript content: MW, RSB, SRRS, JB, AC, MW, BMB, SMB, KT, TS, PDM, JKF, IJM, NLL, FM, SML. Approving final version of manuscript: HRS, KAB, MW, SSD, FM, NLL, and RNM. HRS, SSD, MW, and RNM take responsibility for the integrity of the data analysis.
Conflict of Interest Statement
RNM is the founder and owns stock in Alzhyme. HRS has received personal compensation for activities with Pfizer and Wyeth and currently with Takeda Pharmaceuticals. The authors declare that the research was conducted in the absence of any commercial or financial relationships that could be construed as a potential conflict of interest.
Acknowledgments
The WA Memory Study was supported by a grant from the National Health and Medical Research Council (NHMRC) of Australia (Grant Number: 324100) to RNM.
References
Australian Institute of Health and Welfare. (2007). Dementia in Australia: National Data Analysis and Development. Canberra, ACT: Australian Institute of Health and Welfare, 315.
Bagger, Y. Z., Tanko, L. B., Alexandersen, P., Qin, G., and Christiansen, C. (2004). The implications of body fat mass and fat distribution for cognitive function in elderly women. Obes. Res. 12, 1519–1526. doi: 10.1038/oby.2004.189
Pubmed Abstract | Pubmed Full Text | CrossRef Full Text | Google Scholar
Banks, L. M., Lees, B., MacSweeney, J. E., and Stevenson, J. C. (1994). Effect of degenerative spinal and aortic calcification on bone density measurements in post-menopausal women: links between osteoporosis and cardiovascular disease? Eur. J. Clin. Invest. 24, 813–817. doi: 10.1111/j.1365-2362.1994.tb02024.x
Bates, K. A., Sohrabi, H. R., Rodrigues, M., Beilby, J., Dhaliwal, S. S., Taddei, K., et al. (2009). Association of cardiovascular factors and Alzheimer's disease plasma amyloid-beta protein in subjective memory complainers. J. Alzheimers Dis. 17, 305–318. doi: 10.3233/JAD-2009-1050
Pubmed Abstract | Pubmed Full Text | CrossRef Full Text | Google Scholar
Beardsall, L. (1998). Development of the Cambridge Contextual Reading Test for improving the estimation of premorbid verbal intelligence in older persons with dementia. Br. J. Clin. Psychol. 37(Pt 2), 229–240. doi: 10.1111/j.2044-8260.1998.tb01297.x
Pubmed Abstract | Pubmed Full Text | CrossRef Full Text | Google Scholar
Beck, I. R., Gagneux-Zurbriggen, A., Berres, M., Taylor, K. I., and Monsch, A. U. (2012). Comparison of verbal episodic memory measures: consortium to establish a registry for Alzheimer's disease–Neuropsychological Assessment Battery (CERAD-NAB) versus California Verbal Learning Test (CVLT). Arch. Clin. Neuropsychol. 27, 510–519. doi: 10.1093/arclin/acs056
Pubmed Abstract | Pubmed Full Text | CrossRef Full Text | Google Scholar
Brooks, B. L., Weaver, L. E., and Scialfa, C. T. (2006). Does impaired executive functioning differentially impact verbal memory measures in older adults with suspected dementia? Clin. Neuropsychol. 20, 230–242. doi: 10.1080/13854040590947461
Pubmed Abstract | Pubmed Full Text | CrossRef Full Text | Google Scholar
Brownbill, R. A., and Ilich, J. Z. (2004). Cognitive function in relation with bone mass and nutrition: cross-sectional association in postmenopausal women. BMC Womens Health 4:2. doi: 10.1186/1472-6874-4-2
Pubmed Abstract | Pubmed Full Text | CrossRef Full Text | Google Scholar
Brown, S. A., and Sharpless, J. L. (2004). Osteoporosis: an under-appreciated complication of diabetes. Clin. Diabetes 22, 10–20. doi: 10.2337/diaclin.22.1.10
Butterfield, D. A., Griffin, S., Munch, G., and Pasinetti, G. M. (2002). Amyloid beta-peptide and amyloid pathology are central to the oxidative stress and inflammatory cascades under which Alzheimer's disease brain exists. J. Alzheimers Dis. 4, 193–201.
Clarnette, R. M., Almeida, O. P., Forstl, H., Paton, A., and Martins, R. N. (2001). Clinical characteristics of individuals with subjective memory loss in Western Australia: results from a cross-sectional survey. Int. J. Geriatr. Psychiatry 16, 168–174. doi: 10.1002/1099-1166(200102)16:2<168::AID-GPS291>3.0.CO;2-D
Pubmed Abstract | Pubmed Full Text | CrossRef Full Text | Google Scholar
Corder, E. H., Saunders, A. M., Strittmatter, W. J., Schmechel, D. E., Gaskell, P. C., Small, G. W., et al. (1993). Gene dose of apolipoprotein E type 4 allele and the risk of Alzheimer's disease in late onset families. Science 261, 921–923. doi: 10.1126/science.8346443
Pubmed Abstract | Pubmed Full Text | CrossRef Full Text | Google Scholar
Crockett, J. C., Rogers, M. J., Coxon, F. P., Hocking, L. J., and Helfrich, M. H. (2011). Bone remodelling at a glance. J. Cell Sci. 124, 991–998. doi: 10.1242/jcs.063032
Pubmed Abstract | Pubmed Full Text | CrossRef Full Text | Google Scholar
Delis, D. C., Freeland, J., Kramer, J. H., and Kaplan, E. (1988). Integrating clinical assessment with cognitive neuroscience: construct validation of the California Verbal Learning Test. J. Consult. Clin. Psychol. 56, 123–130. doi: 10.1037/0022-006X.56.1.123
Pubmed Abstract | Pubmed Full Text | CrossRef Full Text | Google Scholar
Delis, D. C., Kramer, J. H., Kaplan, A., and Ober, N. A. (2000). CVLT-II California Verbal Learning Test Manual Adult Version, 2nd Edn. Oxford: Harcourt Assessment Inc.
den Uyl, D., Nurmohamed, M. T., van Tuyl, L. H. D., Raterman, H. G., and Lems, W. F. (2011). (Sub) clinical cardiovascular disease is associated with increased bone loss and fracture risk; a systematic review of the association between cardiovascular disease and osteoporosis. Arthritis Res. Ther. 13:R5. doi: 10.1186/ar3224
Di Carlo, M., Giacomazza, D., and San Biagio, P. L. (2012). Alzheimer's disease: biological aspects, therapeutic perspectives and diagnostic tools. J. Phys. Condens. Matter 24:244102. doi: 10.1088/0953-8984/24/24/244102
Pubmed Abstract | Pubmed Full Text | CrossRef Full Text | Google Scholar
Dimic, A., Popovic, M. R., Tasic, I., Djordjevic, D., Stojanovic, S., Stamenkovic, B., et al. (2012). Relation between bone density and certain parameters of lipid status in postmenopausal women. Cent. Eur. J. Med. 7, 642–649. doi: 10.2478/s11536-012-0044-6
Duff, K., Schoenberg, M. R., Scott, J. G., and Adams, R. L. (2005). The relationship between executive functioning and verbal and visual learning and memory. Arch. Clin. Neuropsychol. 20, 111–122. doi: 10.1016/j.acn.2004.03.003
Pubmed Abstract | Pubmed Full Text | CrossRef Full Text | Google Scholar
Eimar, H., Tamimi, I., Murshed, M., and Tamimi, F. (2013). Cholinergic regulation of bone. J. Musculoskelet. Neuronal Interact. 13, 124–132.
Farhat, G. N., and Cauley, J. A. (2008). The link between osteoporosis and cardiovascular disease. Clin. Cases Miner. Bone Metab. 5, 19–34.
Farhat, G. N., Newman, A. B., Sutton-Tyrrell, K., Matthews, K. A., Boudreau, R., Schwartz, A. V., et al. (2007). The association of bone mineral density measures with incident cardiovascular disease in older adults. Osteoporos. Int. 18, 999–1008. doi: 10.1007/s00198-007-0338-8
Pubmed Abstract | Pubmed Full Text | CrossRef Full Text | Google Scholar
Finkelstein, J. S., Brockwell, S. E., Mehta, V., Greendale, G. A., Sowers, M. R., Ettinger, B., et al. (2008). Bone mineral density changes during the menopause transition in a multiethnic cohort of women. J. Clin. Endocrinol. Metab. 93, 861–868. doi: 10.1210/jc.2007-1876
Pubmed Abstract | Pubmed Full Text | CrossRef Full Text | Google Scholar
Folstein, M. F., Folstein, S. E., and McHugh, P. R. (1975). “Mini-mental state.” A practical method for grading the cognitive state of patients for the clinician. J. Psychiatr. Res. 12, 189–198. doi: 10.1016/0022-3956(75)90026-6
Pubmed Abstract | Pubmed Full Text | CrossRef Full Text | Google Scholar
Fox, M., Berzuini, C., and Knapp, L. A. (2013). Cumulative estrogen exposure, number of menstrual cycles, and Alzheimer's risk in a cohort of British women. Psychoneuroendocrinology 38, 2973–2982. doi: 10.1016/j.psyneuen.2013.08.005
Pubmed Abstract | Pubmed Full Text | CrossRef Full Text | Google Scholar
Glenner, G. G., and Wong, C. W. (1984). Alzheimer's disease: initial report of the purification and characterization of a novel cerebrovascular amyloid protein. Biochem. Biophys. Res. Commun. 120, 885–890. doi: 10.1016/S0006-291X(84)80190-4
Pubmed Abstract | Pubmed Full Text | CrossRef Full Text | Google Scholar
Golby, A., Silverberg, G., Race, E., Gabrieli, S., O'Shea, J., Knierim, K., et al. (2005). Memory encoding in Alzheimer's disease: an fMRI study of explicit and implicit memory. Brain 128(Pt 4), 773–787. doi: 10.1093/brain/awh400
Pubmed Abstract | Pubmed Full Text | CrossRef Full Text | Google Scholar
Guerra, R. S., Amaral, T. F., Marques, E., Mota, J., and Restivo, M. T. (2010). Accuracy of Siri and Brozek equations in the percent body fat estimation in older adults. J. Nutr. Health Aging 14, 744–748. doi: 10.1007/s12603-010-0112-z
Pubmed Abstract | Pubmed Full Text | CrossRef Full Text | Google Scholar
Gustafson, D. R. (2012). Adiposity and cognitive decline: underlying mechanisms. J. Alzheimers Dis. 30, S97–S112. doi: 10.3233/JAD-2012-120487
Pubmed Abstract | Pubmed Full Text | CrossRef Full Text | Google Scholar
Gustafson, D. R., and Luchsinger, J. A. (2013). High adiposity: risk factor for dementia and Alzheimer's disease? Alzheimers Res. Ther. 5:57. doi: 10.1186/alzrt221
Pubmed Abstract | Pubmed Full Text | CrossRef Full Text | Google Scholar
Haberland, M., Schilling, A. F., Rueger, J. M., and Amling, M. (2001). Brain and bone: central regulation of bone mass. A new paradigm in skeletal biology. J. Bone Joint Surg. Am. 83, 1871–1876.
Hill, B. D., Alosco, M., Bauer, L., and Tremont, G. (2012). The relation of executive functioning to CVLT-II learning, memory, and process indexes. Applied neuropsychology Adult. 19, 198–206. doi: 10.1080/09084282.2011.643960
Pubmed Abstract | Pubmed Full Text | CrossRef Full Text | Google Scholar
Hixson, J. E., and Vernier, D. T. (1990). Restriction isotyping of human apolipoprotein E by gene amplification and cleavage with HhaI. J. Lipid Res. 31, 545–548.
Holland, D., Desikan, R. S., Dale, A. M., and McEvoy, L. K. (2013). Higher rates of decline for women and apolipoprotein Eε 4 carriers. AJNR Am. J. Neuroradiol. 34, 2287–2293. doi: 10.3174/ajnr.A3601
Pubmed Abstract | Pubmed Full Text | CrossRef Full Text | Google Scholar
Isacson, O., Seo, H., Lin, L., Albeck, D., and Granholm, A. C. (2002). Alzheimer's disease and Down's syndrome: roles of APP, trophic factors and ACh. Trends Neurosci. 25, 79–84. doi: 10.1016/S0166-2236(02)02037-4
Pubmed Abstract | Pubmed Full Text | CrossRef Full Text | Google Scholar
Kanaya, A. M., Lindquist, K., Harris, T. B., Launer, L., Rosano, C., Satterfield, S., et al. (2009). Total and regional adiposity and cognitive change in older adults: the Health, Aging and Body Composition (ABC) study. Arch. Neurol. 66, 329–335. doi: 10.1001/archneurol.2008.570
Pubmed Abstract | Pubmed Full Text | CrossRef Full Text | Google Scholar
Kanis, J. A. (2002). Diagnosis of osteoporosis and assessment of fracture risk. Lancet 359, 1929–1936. doi: 10.1016/S0140-6736(02)08761-5
Pubmed Abstract | Pubmed Full Text | CrossRef Full Text | Google Scholar
Karsenty, G., and Oury, F. (2010). The central regulation of bone mass, the first link between bone remodeling and energy metabolism. J. Clin. Endocrincol. Metab. 95, 4795–4801. doi: 10.1210/jc.2010-1030
Pubmed Abstract | Pubmed Full Text | CrossRef Full Text | Google Scholar
Kerwin, D. R., Gaussoin, S. A., Chlebowski, R. T., Kuller, L. H., Vitolins, M., Coker, L. H., et al. (2011). Interaction between body mass index and central adiposity and risk of incident cognitive impairment and dementia: results from the Women's Health Initiative Memory Study. J. Am. Geriatr. Soc. 59, 107–112. doi: 10.1111/j.1532-5415.2010.03219.x
Pubmed Abstract | Pubmed Full Text | CrossRef Full Text | Google Scholar
Kohlmeier, M., Salomon, A., Saupe, J., and Shearer, M. J. (1996). Transport of vitamin K to bone in humans. J. Nutr. 126(4 Suppl.), 1192S–1196S.
Laws, S. M., Clarnette, R. M., Taddei, K., Martins, G., Paton, A., Hallmayer, J., et al. (2002). APOEε 4 and APOE 491A polymorphisms in individuals with subjective memory loss. Mol. Psychiatry 7, 768–775. doi: 10.1038/sj.mp.4001083
Pubmed Abstract | Pubmed Full Text | CrossRef Full Text | Google Scholar
Levine, M. E., and Crimmins, E. M. (2012). Sarcopenic obesity and cognitive functioning: the mediating roles of insulin resistance and inflammation? Curr. Gerontol. Geriatr. Res. 2012:826398. doi: 10.1155/2012/826398
Pubmed Abstract | Pubmed Full Text | CrossRef Full Text | Google Scholar
Lieb, W., Beiser, A. S., Vasan, R. S., Tan, Z. S., Au, R., Harris, T. B., et al. (2009). Association of plasma leptin levels with incident Alzheimer disease and MRI measures of brain aging. JAMA 302, 2565–2572. doi: 10.1001/jama.2009.1836
Pubmed Abstract | Pubmed Full Text | CrossRef Full Text | Google Scholar
Luchsinger, J. A., and Mayeux, R. (2007). Adiposity and Alzheimer's disease. Curr. Alzheimer Res. 4, 127–134. doi: 10.2174/156720507780362100
Pubmed Abstract | Pubmed Full Text | CrossRef Full Text | Google Scholar
Luchsinger, J. A., Patel, B., Tang, M. X., Schupf, N., and Mayeux, R. (2007). Measures of adiposity and dementia risk in elderly persons. Arch. Neurol. 64, 392–398. doi: 10.1001/archneur.64.3.392
Pubmed Abstract | Pubmed Full Text | CrossRef Full Text | Google Scholar
Lui, L. Y., Stone, K., Cauley, J. A., Hillier, T., and Yaffe, K. (2003). Bone loss predicts subsequent cognitive decline in older women: the study of osteoporotic fractures. J. Am. Geriatr. Soc. 51, 38–43. doi: 10.1034/j.1601-5215.2002.51007.x
Pubmed Abstract | Pubmed Full Text | CrossRef Full Text | Google Scholar
Mahley, R. W., Nathan, B. P., and Pitas, R. E. (1996). Apolipoprotein, E. Structure, function, and possible roles in Alzheimer's disease. Ann. N.Y. Acad. Sci. 777, 139–145. doi: 10.1111/j.1749-6632.1996.tb34412.x
Pubmed Abstract | Pubmed Full Text | CrossRef Full Text | Google Scholar
Masters, C. L., Multhaup, G., Simms, G., Pottgiesser, J., Martins, R. N., and Beyreuther, K. (1985). Neuronal origin of a cerebral amyloid: neurofibrillary tangles of Alzheimer's disease contain the same protein as the amyloid of plaque cores and blood vessels. EMBO J. 4, 2757–2763.
Maylor, E. A., Reimers, S., Choi, J., Collaer, M. L., Peters, M., and Silverman, I. (2007). Gender and sexual orientation differences in cognition across adulthood: age is kinder to women than to men regardless of sexual orientation. Arch. Sex. Behav. 36, 235–249. doi: 10.1007/s10508-006-9155-y
Pubmed Abstract | Pubmed Full Text | CrossRef Full Text | Google Scholar
Norton, S., Matthews, F. E., Barnes, D. E., Yaffe, K., and Brayne, C. (2014). Potential for primary prevention of Alzheimer's disease: an analysis of population-based data. Lancet Neurol. 13, 788–794. doi: 10.1016/S1474-4422(14)70136-X
Pubmed Abstract | Pubmed Full Text | CrossRef Full Text | Google Scholar
Parhami, F., Garfinkel, A., and Demer, L. L. (2000). Role of lipids in osteoporosis. Arterioscler. Thromb. Vasc. Biol. 20, 2346–2348. doi: 10.1161/01.ATV.20.11.2346
Pubmed Abstract | Pubmed Full Text | CrossRef Full Text | Google Scholar
Parhami, F., Morrow, A. D., Balucan, J., Leitinger, N., Watson, A. D., Tintut, Y., et al. (1997). Lipid oxidation products have opposite effects on calcifying vascular cell and bone cell differentiation - A possible explanation for the paradox of arterial calcification in osteoporotic patients. Arterioscler. Thromb. Vasc. Biol. 17, 680–687. doi: 10.1161/01.ATV.17.4.680
Pubmed Abstract | Pubmed Full Text | CrossRef Full Text | Google Scholar
Price, P. A., June, H. H., Buckley, J. R., and Williamson, M. K. (2001). Osteoprotegerin inhibits artery calcification induced by warfarin and by vitamin D. Arterioscler. Thromb. Vasc. Biol. 21, 1610–1616. doi: 10.1161/hq1001.097102
Pubmed Abstract | Pubmed Full Text | CrossRef Full Text | Google Scholar
Prince, M., Bryce, R., Albanese, E., Wimo, A., Ribeiro, W., and Ferri, C. P. (2013). The global prevalence of dementia: a systematic review and metaanalysis. Alzheimers Dement. 9, 63–75.e2. doi: 10.1016/j.jalz.2012.11.007
Pubmed Abstract | Pubmed Full Text | CrossRef Full Text | Google Scholar
Qiu, C. X., Xu, W. L., Winblad, B., and Fratiglioni, L. (2010). Vascular risk profiles for dementia and Alzheimer's disease in very old people: a population-based longitudinal study. J. Alzheimers Dis. 20, 293–300. doi: 10.3233/JAD-2010-1361
Pubmed Abstract | Pubmed Full Text | CrossRef Full Text | Google Scholar
Roses, A. D. (1997). Apolipoprotein, E., a gene with complex biological interactions in the aging brain. Neurobiol Dis. 4, 170–185. doi: 10.1006/nbdi.1997.0161
Pubmed Abstract | Pubmed Full Text | CrossRef Full Text | Google Scholar
Roth, M., Huppert, F. A., Mountjoy, C. Q., and Tym, E. (1998). CAMDEX-R: The Cambridge Examination for Mental Disorders of the Elderly-Revised. Cambridge, UK: Cambridge University Press.
Rothman, M. S., Arciniegas, D. B., Filley, C. M., and Wierman, M. E. (2007). The neuroendocrine effects of traumatic brain injury. J. Neuropsychiatry Clin. Neurosci. 19, 363–372. doi: 10.1176/jnp.2007.19.4.363
Pubmed Abstract | Pubmed Full Text | CrossRef Full Text | Google Scholar
Sarkis, K. S., Martini, L. A., Szejnfeld, V. L., and Pinheiro, M. M. (2012). Low fatness, reduced fat intake and adequate plasmatic concentrations of LDL-cholesterol are associated with high bone mineral density in women: a cross-sectional study with control group. Lipids Health Dis. 11:37. doi: 10.1186/1476-511X-11-37
Pubmed Abstract | Pubmed Full Text | CrossRef Full Text | Google Scholar
Saunders, A. M., Strittmatter, W. J., Schmechel, D., George-Hyslop, P. H., Pericak-Vance, M. A., Joo, S. H., et al. (1993). Association of apolipoprotein E allele ε 4 with late-onset familial and sporadic Alzheimer's disease. Neurology 43, 1467–1472. doi: 10.1212/WNL.43.8.1467
Pubmed Abstract | Pubmed Full Text | CrossRef Full Text | Google Scholar
Schoofs, M. W. C. J., van der Klift, M., Hofman, A., van Duijn, C. M., Stricker, B. H. C., A. P., Pols, H., et al. (2004). ApoE gene polymorphisms, BMD, and fracture risk in elderly men and women: the Rotterdam Study. J. Bone Miner. Res. 19, 1490–1496. doi: 10.1359/JBMR.040605
Pubmed Abstract | Pubmed Full Text | CrossRef Full Text | Google Scholar
Sohrabi, H. R., Bates, K. A., Rodrigues, M., Taddei, K., Martins, G., Laws, S. M., et al. (2009). The relationship between memory complaints, perceived quality of life and mental health in apolipoprotein Eε 4 carriers and non-carriers. J. Alzheimers Dis. 17, 69–79. doi: 10.3233/JAD-2009-1018
Pubmed Abstract | Pubmed Full Text | CrossRef Full Text | Google Scholar
Takeda, S. (2009). Osteoporosis: a neuroskeletal disease? Int. J. Biochem. Cell Biol. 1, 455–459. doi: 10.1016/j.biocel.2008.08.002
Pubmed Abstract | Pubmed Full Text | CrossRef Full Text | Google Scholar
Tan, Z. S., Seshadri, S., Beiser, A., Zhang, Y., Felson, D., Hannan, M. T., et al. (2005). Bone mineral density and the risk of Alzheimer disease. Arch. Neurol. 62, 107–111. doi: 10.1001/archneur.62.1.107
Pubmed Abstract | Pubmed Full Text | CrossRef Full Text | Google Scholar
Twamley, E. W., Ropacki, S. A., and Bondi, M. W. (2006). Neuropsychological and neuroimaging changes in preclinical Alzheimer's disease. J. Int. Neuropsychol. Soc. 12, 707–735. doi: 10.1017/S1355617706060863
Pubmed Abstract | Pubmed Full Text | CrossRef Full Text | Google Scholar
Van Loan, M. D., and Mayclin, P. L. (1992). Body composition assessment: dual-energy X-ray absorptiometry (DEXA) compared to reference methods. Eur. J. Clin. Nutr. 46, 125–130.
Verdile, G., Fuller, S., Atwood, C. S., Laws, S. M., Gandy, S. E., and Martins, R. N. (2004). The role of beta amyloid in Alzheimer's disease: still a cause of everything or the only one who got caught? Pharmacol. Res. 50, 397–409. doi: 10.1016/j.phrs.2003.12.028
Pubmed Abstract | Pubmed Full Text | CrossRef Full Text | Google Scholar
Vickland, V., Morris, T., Draper, B., Low, L., and Brodaty, H. (2012). Modelling the Impact of Interventions to Delay the Onset of Dementia in Australia. A report for Alzheimer's Australia. Alzheimer's Australia.
Weisgraber, K. H. (1994). Apolipoprotein E: structure-function relationships. Adv. Protein Chem. 45, 249–302. doi: 10.1016/S0065-3233(08)60642-7
Pubmed Abstract | Pubmed Full Text | CrossRef Full Text | Google Scholar
White, K. G., and Ruske, A. C. (2002). Memory deficits in Alzheimer's disease: the encoding hypothesis and cholinergic function. Psychon. Bull. Rev. 9, 426–437. doi: 10.3758/BF03196301
Pubmed Abstract | Pubmed Full Text | CrossRef Full Text | Google Scholar
Whitmer, R. A., Gustafson, D. R., Barrett-Connor, E., Haan, M. N., Gunderson, E. P., and Yaffe, K. (2008). Central obesity and increased risk of dementia more than three decades later. Neurology 71, 1057–1064. doi: 10.1212/01.wnl.0000306313.89165.ef
Pubmed Abstract | Pubmed Full Text | CrossRef Full Text | Google Scholar
W.H.O. (1994). Assessment of Fracture Risk and its Application to Screening for Postmenopausal Osteoporosis. Report of a WHO Study Group. World Health Organization.
Wirths, O., Multhaup, G., and Bayer, T. A. (2004). A modified beta-amyloid hypothesis: intraneuronal accumulation of the beta-amyloid peptide–the first step of a fatal cascade. J. Neurochem. 91, 513–520. doi: 10.1111/j.1471-4159.2004.02737.x
Pubmed Abstract | Pubmed Full Text | CrossRef Full Text | Google Scholar
Wolf, P. A., Beiser, A., Elias, M. F., Au, R., Vasan, R. S., and Seshadri, S. (2007). Relation of obesity to cognitive function: importance of central obesity and synergistic influence of concomitant hypertension. The Framingham Heart Study. Curr. Alzheimer Res. 4, 111–116. doi: 10.2174/156720507780362263
Pubmed Abstract | Pubmed Full Text | CrossRef Full Text | Google Scholar
Yaffe, K., Browner, W., Cauley, J., Launer, L., and Harris, T. (1999). Association between bone mineral density and cognitive decline in older women. J. Am. Geriatr. Soc. 47, 1176–1182.
Yaffe, K., Sawaya, G., Lieberburg, I., and Grady, D. (1998). Estrogen therapy in postmenopausal women: effects on cognitive function and dementia. JAMA 279, 688–695. doi: 10.1001/jama.279.9.688
Pubmed Abstract | Pubmed Full Text | CrossRef Full Text | Google Scholar
Yang, R., and Barouch, L. A. (2007). Leptin signaling and obesity: cardiovascular consequences. Circ. Res. 101, 545–559. doi: 10.1161/CIRCRESAHA.107.156596
Pubmed Abstract | Pubmed Full Text | CrossRef Full Text | Google Scholar
Yesavage, J. A., Brink, T. L., Rose, T. L., Lum, O., Huang, V., Adey, M., et al. (1982). Development and validation of a geriatric depression screening scale: a preliminary report. J. Psychiatr. Res. 17, 37–49. doi: 10.1016/0022-3956(82)90033-4
Pubmed Abstract | Pubmed Full Text | CrossRef Full Text | Google Scholar
Yoon, D. H., Choi, S. H., Yu, J. H., Ha, J. H., Ryu, S. H., and Park, D. H. (2012). The relationship between visceral adiposity and cognitive performance in older adults. Age Ageing 41, 456–461. doi: 10.1093/ageing/afs018
Pubmed Abstract | Pubmed Full Text | CrossRef Full Text | Google Scholar
Zhang, Y., Seshadri, S., Ellison, R. C., Heeren, T., and Felson, D. T. (2001). Bone mineral density and verbal memory impairment: Third National Health and Nutrition Examination Survey. Am. J. Epidemiol. 154, 795–802. doi: 10.1093/aje/154.9.795
Pubmed Abstract | Pubmed Full Text | CrossRef Full Text | Google Scholar
Keywords: dual energy x-ray absorptiometry, cognition, apolipoprotein E, bone mineral density, episodic verbal memory, executive function, aging
Citation: Sohrabi HR, Bates KA, Weinborn M, Bucks RS, Rainey-Smith SR, Rodrigues MA, Bird SM, Brown BM, Beilby J, Howard M, Criddle A, Wraith M, Taddei K, Martins G, Paton A, Shah T, Dhaliwal SS, Mehta PD, Foster JK, Martins IJ, Lautenschlager NT, Mastaglia F, Laws SM and Martins RN (2015) Bone mineral density, adiposity, and cognitive functions. Front. Aging Neurosci. 7:16. doi: 10.3389/fnagi.2015.00016
Received: 18 November 2014; Accepted: 03 February 2015;
Published online: 18 February 2015.
Edited by:
Tania Álvarez Avellón, Universidad de Oviedo, SpainReviewed by:
Carsten Culmsee, University of Marburg, GermanyAurel Popa-wagner, Rostock Medical School, Germany
Copyright © 2015 Sohrabi, Bates, Weinborn, Bucks, Rainey-Smith, Rodrigues, Bird, Brown, Beilby, Howard, Criddle, Wraith, Taddei, Martins, Paton, Shah, Dhaliwal, Mehta, Foster, Martins, Lautenschlager, Mastaglia, Laws and Martins. This is an open-access article distributed under the terms of the Creative Commons Attribution License (CC BY). The use, distribution or reproduction in other forums is permitted, provided the original author(s) or licensor are credited and that the original publication in this journal is cited, in accordance with accepted academic practice. No use, distribution or reproduction is permitted which does not comply with these terms.
*Correspondence: Ralph N. Martins, School of Medical Sciences, Edith Cowan University, 270 Joondalup Dr, Joondalup, WA 6027, Australia e-mail: ralph.r.martins@gmail.com