- 1Division of Gastroenterology and Hepatology, Key Laboratory of Gastroenterology and Hepatology, Ministry of Health, Shanghai Institute of Digestive Disease, Renji Hospital, School of Medicine, Shanghai Jiao Tong University, Shanghai, China
- 2State Key Laboratory of Natural Medicines, Research Center of Biostatistics and Computational Pharmacy, China Pharmaceutical University, Nanjing, China
- 3Department of Urology, The First Affiliated Hospital of Anhui Medical University, Hefei, China
- 4Department of Liver Surgery, Renji Hospital, School of Medicine, Shanghai Jiao Tong University, Shanghai, China
It is currently difficult for pathologists to diagnose pancreatic cancer (PC) using biopsy specimens because samples may have been from an incorrect site or contain an insufficient amount of tissue. Thus, there is a need to develop a platform-independent molecular classifier that accurately distinguishes benign pancreatic lesions from PC. Here, we developed a robust qualitative messenger RNA signature based on within-sample relative expression orderings (REOs) of genes to discriminate both PC tissues and cancer-adjacent normal tissues from non-PC pancreatitis and healthy pancreatic tissues. A signature comprising 12 gene pairs and 17 genes was built in the training datasets and validated in microarray and RNA-sequencing datasets from biopsy samples and surgically resected samples. Analysis of 1,007 PC tissues and 257 non-tumor samples from nine databases indicated that the geometric mean of sensitivity and specificity was 96.7%, and the area under receiver operating characteristic curve was 0.978 (95% confidence interval, 0.947–0.994). For 20 specimens obtained from endoscopic biopsy, the signature had a diagnostic accuracy of 100%. The REO-based signature described here can aid in the molecular diagnosis of PC and may facilitate objective differentiation between benign and malignant pancreatic lesions.
Introduction
Pancreatic cancer (PC) is the fourth leading cause of cancer deaths in the United States and the sixth leading cause in China. Patients with PC have a 5-year survival rate of 8.5% in the United States and 7.2% in China (Siegel et al., 2019; Zhao et al., 2019). The diagnosis and treatment of pancreatic cancer remain challenging. Patients with early-stage PC are usually asymptomatic, and only about 10% of patients are diagnosed at an early stage (Singhi et al., 2019). Serum cancer antigen 19-9 (CA 19-9) is the only marker approved by the United States Food and Drug Administration for use in the routine management of PC. Imaging techniques, such as computed tomography (CT), magnetic resonance imaging (MRI), endoscopic ultrasonography (EUS), and endoscopic retrograde cholangiopancreatography (ERCP), can help in the diagnosis of PC. EUS is currently the most effective imaging method for diagnosis and is superior to CT and MRI (Costache et al., 2017). However, the accuracy of EUS for early detection of PC is still unsatisfactory, and it is often difficult to distinguish benign and malignant pancreatic lesions (Singhi et al., 2019).
Therefore, it is sometimes necessary to perform a tissue biopsy for the pathological diagnosis of PC. In clinical practice, more accurate and definitive pathological diagnoses can be made using biopsies from EUS-guided fine needle aspiration (EUS-FNA), and this method has an overall diagnostic accuracy of 91% (Banafea et al., 2016). However, biopsy samples may be collected from incorrect locations, and this can lead to false-negative results. Repeated EUS-FNA not only increases the diagnostic accuracy to 96.3% but also increases the risk of complications (Suzuki et al., 2013). Thus, it is vital to develop molecular signatures to complement the present histological methods for diagnosis of early PC, especially when the locations of biopsy samples are incorrect. Recent studies reported that within-sample relative expression orderings (REOs) of genes are insensitive to experimental batch effects and can provide qualitative transcriptional signatures that can be applied to samples at an individual level (Eddy et al., 2010; Wang et al., 2015). Within-sample REOs are also insensitive to variable proportions of tumor epithelial cells sampled from different tumor locations in the same patient (Cheng et al., 2017), RNA degradation during specimen storage and preparation (Chen et al., 2017), and amplification bias for minimum specimens, which can lead to failure of quantitative transcriptional signatures in clinical applications (Liu et al., 2017). Thus, within−sample REOs may provide a robust qualitative signature for the early diagnosis of PC.
In this study, based on the REOs of 12 gene pairs, we identified a qualitative transcriptional signature for the early diagnosis of PC. The signature can accurately discriminate both PC tissues and adjacent-normal tissues from normal pancreatic tissues and non−PC pancreatitis in both biopsy and surgical resection samples.
Materials and Methods
Data and Preprocessing
Transcriptional profiles of pancreatic tissues were retrieved from Gene Expression Omnibus (GEO), the Genotype-Tissue Expression (GTEx) project, and the Cancer Genome Atlas (TCGA) Data Portal1 and were measured by the Illumina, Affymetrix, or Agilent platforms. The training cohort consisted of 5 microarray datasets (GSE101462, GSE71989, GSE91035, E-MEXP-1121, and E-MTAB-1791), with 74 normal pancreatic tissues, 72 pancreatitis tissues, and 269 PC samples (Table 1). The tuning dataset (GSE41368) consisted of 6 normal pancreas tissues and 6 PC tissues, and the external validation cohort consisted of 9 microarray and RNA-sequencing datasets of 258 normal pancreatic samples and 1,051 tumor-related pancreatic lesions (GSE50827, GSE19650, GSE62165, GSE43288, GSE21501, GSE71729, E-MTAB-6134, GTEx, and TCGA).
Transcriptome HTSeq-counts data of the TCGA-Pancreatic Adenocarcinoma (PAAD) project were downloaded from the Genomic Data Commons using the R package “TCGAbiolink,” including 183 non-formalin-fixed and paraffin-embedded (FFPE) samples of primary pancreatic tumors. Ensembl ID for protein-coding messenger RNAs (mRNAs) was annotated to symbol name using GENCODE27. The number of fragments per kilobase of non-overlapped exons per million fragments mapped (FPKM) was calculated first and was then transformed into transcripts per kilobase million (TPM) values. All mRNAs with TPM values < 1 in more than 90% of the samples were considered to be noise and removed prior to downstream analysis. Data of the GTEx project were retrieved from the UCSC Xena browser2. For microarray datasets measured by Affymetrix platforms, the robust multiarray average (RMA) procedure was performed, with raw CEL files for background, using the R package “affy” for adjustment (Irizarry et al., 2003). For other platforms, the processed data were obtained from GEO and utilized for subsequent analyses.
Identification of Qualitative REO-Based PC Diagnosis Signature
For the purpose of this study, tumor samples were identified as “cancer” or “cancer-adjacent normal” because the transcriptional characteristics of apparently normal tissue that is adjacent to a tumor differs from healthy normal tissues (Aran et al., 2017), whereas non-tumor samples involve “healthy normal” or “pancreatitis.”
Data analysis consisted of several sequential steps (Figure 1). First, a pairwise gene or REO within a sample with genes i and j was assigned a value of “1” if gene “i” had higher expression and “0” if gene j had greater expression. A “reversal gene pair” (RGP) was defined by the presence of the same REO pattern in more than 85% of the tumor samples (“cancer” and “cancer-adjacent normal”) and a reversed pattern in more than 85% of the non-tumor samples (“healthy normal” and “pancreatitis”) in the training dataset. Then, RGPs were filtered using the tuning dataset to establish a candidate REO signature of PC. The rank difference for each RGP was computed for each sample as:
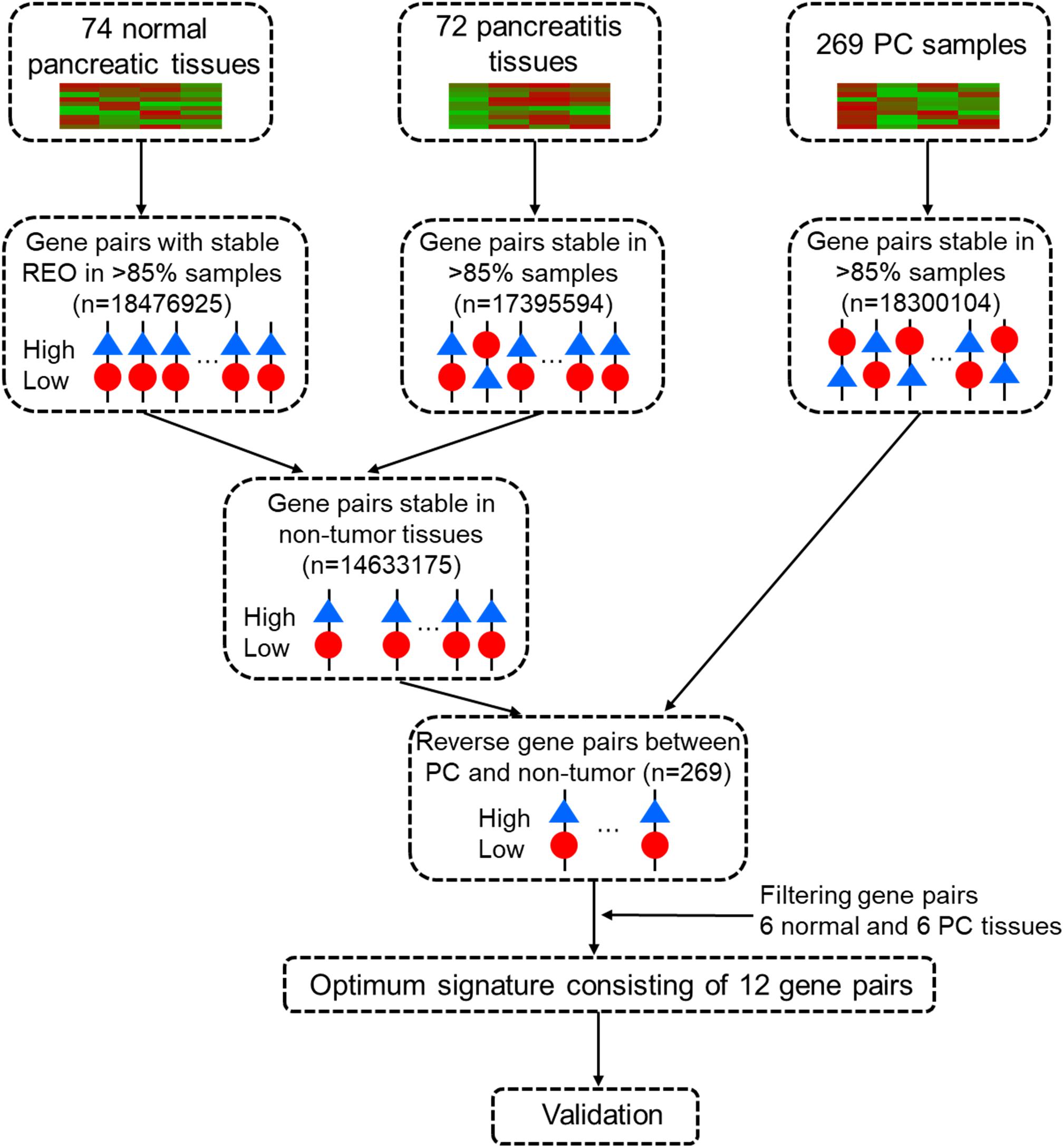
Figure 1. Workflow for identification and evaluation of a qualitative diagnostic signature of pancreatic cancer based on relative expression orderings (REOs).
where Ri and Rj represent the rank of gene i and j within a sample, and Rij represents the rank difference.
Then, Rij, the geometric mean of the mean value of Rij in tumor samples was used to assess the extent of reversal of the gene pair between tumor and non-tumor samples:
where is the mean value in tumor samples, and is the mean value in non-tumor samples. Ideally, the sign for each should be uniform in each sample type, X{X ∈ (T,N)}. However, in most cases, a sample in a specific X may have an with a different sign, and this can cause bias because of the absolute value operation. Therefore, an with the “wrong” sign was forced to zero before subsequent analysis. Thus, a higher value corresponds to a larger reversal of the REO of the gene pair between tumor and non-tumor samples.
The selected candidate REO signatures were then sorted in a descending order according to their values, and the RGP with the largest was set as the seed. Then, a forward selection procedure was used, with one RGP entered at a time, to evaluate the classification accuracy based on a voting rule. Thus, if more than half of the RGPs of a sample in a signature framework had an REO for the tumor, the sample was classified as “tumor”; otherwise, it was classified as “non-tumor”. This selection procedure eventually selected the minimum and optimal number of RGPs with the highest accuracy.
Performance Evaluation
All samples in the training, tuning, and validation datasets were first pooled together to assess the general predictive performance in different samples (tumor, cancer-adjacent normal, pancreatitis, and healthy normal) using receiver operating characteristic (ROC) analysis and calculation of the area under the curve (AUC). The accuracy was defined as the portion of correctly identified samples in the entire cohort, and the AUC, sensitivity, and specificity were calculated using the R package “pROC”. The diagnostic performance was further evaluated in each independent dataset by calculation of sensitivity and specificity. In this procedure, sensitivity refers to the proportion correctly identified true positives and specificity to the proportion of correctly identified true negatives. Sensitivity and specificity were recorded at the median cutoff of the voting threshold.
Statistical Analyses
All statistical analyses were performed using R software (version: 3.6.13). The data analysis source code is provided in the github: https://github.com/xlucpu/PCsig.
Results
Identification of the Qualitative Gene Pair Signature for PC
We first used the training cohort from a merger of five microarray datasets (GSE101462, GSE71989, GSE91035, E-MEXP-1121, and E-MTAB-1791) to identify common gene pairs with stable REOs in 74 normal pancreatic tissues (18,476,925 gene pairs) and 72 pancreatitis samples (17,395,594 gene pairs). Then, we identified 14,633,175 gene pairs with identical REO patterns in at least 85% of normal pancreas and pancreatitis samples as stable gene pairs of non-tumor samples. We also identified 18,300,104 gene pairs with stable REO patterns in at least 85% of the 269 PC samples in the training cohort. Next, we used these data to identify 269 RGPs between the non-tumor and tumor tissues (Supplementary Table S1). After a tuning procedure using 6 normal pancreas and 6 PC tissues, we selected 20 gene pairs with identical REO patterns in the testing dataset (GSE41368).
Then, we sorted the 20 RGPs into descending order based on the rank difference () between PC and non-tumor tissues (normal pancreas and pancreatitis) in the merged data from the training set and utilized the top−ranked k gene pairs for sample classification using majority vote rule. The results indicated that for k ranging from 1 to 20, the largest geometric mean of sensitivity and specificity (93.79%) occurred for k = 12 (Figure 2). We thus identified these 12 gene pairs (Table 2) as the transcriptional signature for discriminating tumor and non-tumor samples.
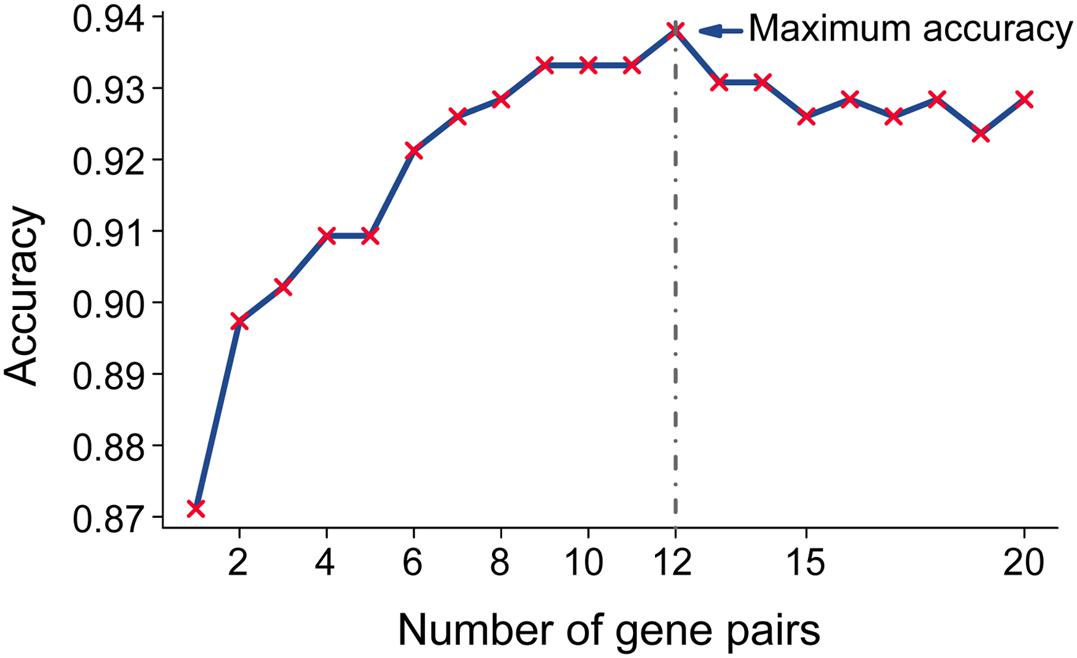
Figure 2. Accuracy of top-ranked gene pairs among the 20 reversal gene pairs (RGPs) in the training data. Twenty RGPs were sorted in a descending order according to the extent of reversal between tumor and non-tumor tissues in the training datasets. Twelve gene pairs provided the highest classification accuracy according to the “majority voting rule” and were used for the qualitative diagnostic signature.
Validation of the Diagnostic Signature in External Validation Datasets
Next, we assessed the performance of the 12-gene pair signature to discriminate PC (including cancer-adjacent tissue) from non-tumor samples. For the 1,007 PC tissues and 257 non-tumor samples from the 9 external validation databases, the geometric mean of sensitivity and specificity was 96.7% and the area under receiver operating characteristic curve was 0.978 (95% confidence interval, 0.947–0.994; Figure 3).
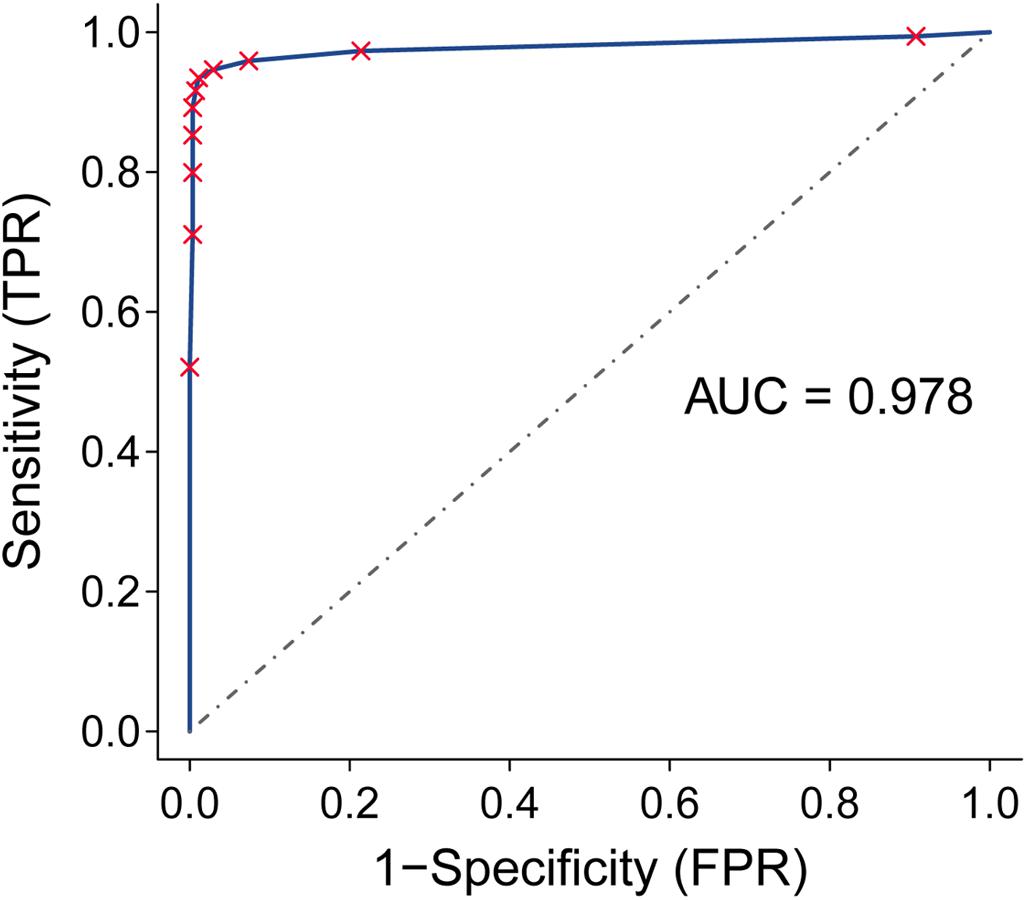
Figure 3. Receiver operating characteristic (ROC) analysis and area under the curve (AUC) for the qualitative diagnostic signature in nine validation datasets.
Notably, our analysis of 17 PC and 3 normal samples obtained from endoscopic biopsies (GSE43288) had a diagnostic accuracy of 100%. For normal pancreatic tissues obtained by autopsy (GTEx), our method correctly classified all 248 samples as normal pancreatic tissue. The other validation sets consisted of samples from surgically resected tissues. For data measured by microarray, our signature correctly identified 96.07% of 842 tumor tissues as tumor samples. The details of diagnostic performance for the transcriptional signature are shown in Table 3 and Supplementary Table S2.
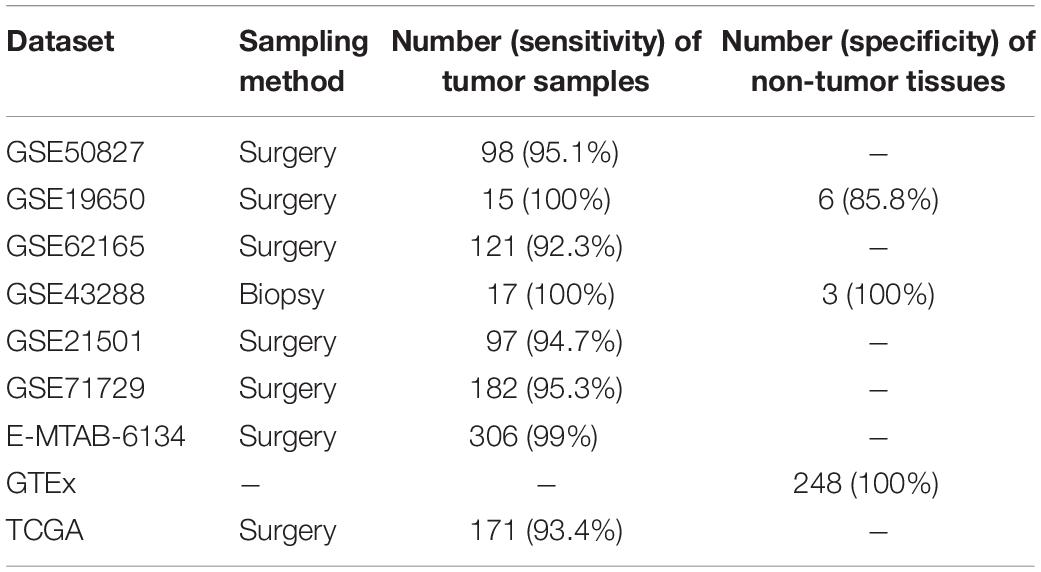
Table 3. Performance of the gene signature in the validation datasets (surgically resected samples and biopsy samples).
Discussion
PC is expected to become the leading cause of cancer-specific mortality in Western countries by 2030, and yet early diagnosis remains difficult (Rahib et al., 2014). It is therefore necessary to identify a molecular diagnostic signature to reduce the uncertainty of pathological diagnosis due to sample error. In this study, we developed and validated a qualitative REO-based signature consisting of 12 different gene pairs with 17 genes for the early and accurate molecular diagnosis of PC. The proposed transcriptional signature can discriminate malignant tissues and most PC-adjacent tissues from benign tissues. Because the signature is effective even when sample site was inaccurate due to imperfect biopsy procedures, this can help to prevent the need for a second procedure.
In contrast to previous studies that used a transcriptomic diagnostic signature based on complicated procedures of data normalization and parameter fitting (Klett et al., 2018; Long et al., 2019), we analyzed the relative expression of gene pairs, instead of the expression of single genes, to differentiate PC from benign lesions. Our transcriptional REO-based signature employed relative ranking of gene expression by identifying multiple gene pairs and can be used without confounding from the batch effect and use of different sequencing platforms (Eddy et al., 2010). Previous studies successfully used this approach in the molecular diagnosis of colorectal cancer, gastric cancer, and hepatocellular carcinoma (Ao et al., 2018; Guan et al., 2019; Yan et al., 2019). The recent developments of high-throughput sequencing technologies have accompanied dramatic decreases in price. Given the limited amount of tissue sampled from biopsies, it is more efficient to measure the expression of a set of genes as markers for aiding pathological diagnosis, molecular subtype classification (Danilova et al., 2019), and chemoresistance of PC as part of the approach of “whole genome sequencing for all” (Xuan et al., 2013; Shao et al., 2018).
Several genes in the transcriptional signature proposed here have established roles in the carcinogenesis of PC. For instance, laminin γ2-chain (LAMC2) is a well-known PC-related gene whose level is elevated in the circulation of PC patients (Katayama et al., 2005). This gene upregulates mesenchymal markers in the microenvironment by activating the Akt/NHE1 signaling pathway and thus mediates the invasion and metastasis of cancer cells (Wang et al., 2020). Cystatin 6 (CST6) is overexpressed in pancreatic ductal adenocarcinoma (PDAC) cells and can stimulate PDAC cell growth by reducing the activity of intracellular cathepsin B (Hosokawa et al., 2008). There is evidence that S100 calcium-binding protein P (S100P) can be used as a biomarker in duodenal fluid and fine needle aspiration biopsies for detection of PDAC, and this protein has a diagnostic sensitivity of 84.8% in biopsy samples (Matsunaga et al., 2017; Aksoy-Altinboga et al., 2018). S100P secretes matrix metalloproteinase 9 (MMP9) and regulates the invasion of PC cells into the lymphatic endothelial monolayer, thereby promoting tumor cell invasion and metastasis (Dakhel et al., 2014; Nakayama et al., 2019). Cadherin-3 (CDH3) regulates cell migration and tumor growth by interacting with cadherin-1 in PC and is upregulated during early-stage PC (Siret et al., 2018).
There were some limitations in our study. First, due to the limited number of biopsy tissues, we only used samples collected from surgery (not from biopsy) in the training set, and this may have led to selection bias. Second, our study design was retrospective, with genomic data derived from publicly available databases. Prospective clinical studies are needed to validate our findings.
In summary, we constructed and validated an REO-based signature consisting of 12 gene pairs and 17 genes that can aid in the early diagnosis of PC.
Data Availability Statement
All datasets generated for this study are included in the article/Supplementary Material.
Ethics Statement
Ethical review and approval was not required for the study on human participants in accordance with the local legislation and institutional requirements. The patients/participants provided their written informed consent to participate in this study.
Author Contributions
Y-JZ and C-JY obtained data from TCGA, GEO, and GTEx database, designed the study, and wrote the manuscript. Y-JZ, X-FL, J-LM, X-YW, Q-WW, Y-JG, and H-MC analyzed and interpreted the data. X-FL was responsible for the statistical analyses. X-BL and F-RY contributed to conception, design, and funding. All authors have been involved in revising and proofreading of the manuscript, and approved the manuscript.
Funding
This work was supported by the grants from the National Natural Science Foundation of China (General Program: 81772519), Excellent Youth Program of Shanghai Municipal Commission of Health and Family Planning (2018YQ29), the Program for Promoting Advanced Appropriate Technology of Shanghai Health Commission (2019SY003), and the “Double First-Class” University Project (CPU2018GY09).
Conflict of Interest
The authors declare that the research was conducted in the absence of any commercial or financial relationships that could be construed as a potential conflict of interest.
Supplementary Material
The Supplementary Material for this article can be found online at: https://www.frontiersin.org/articles/10.3389/fmolb.2020.569842/full#supplementary-material
Footnotes
- ^ https://tcga-data.nci.nih.gov/tcga/
- ^ https://xenabrowser.net/datapages/
- ^ http://www.r-project.org/
References
Aksoy-Altinboga, A., Baglan, T., Umudum, H., and Ceyhan, K. (2018). Diagnostic value of S100p, IMP3, Maspin, and pVHL in the differantial diagnosis of pancreatic ductal adenocarcinoma and normal/chronic pancreatitis in fine needle aspiration biopsy. J. Cytol. 35, 247–251. doi: 10.4103/joc.Joc_18_17
Ao, L., Zhang, Z., Guan, Q., Guo, Y., Guo, Y., Zhang, J., et al. (2018). A qualitative signature for early diagnosis of hepatocellular carcinoma based on relative expression orderings. Liver Int. 38, 1812–1819. doi: 10.1111/liv.13864
Aran, D., Camarda, R., Odegaard, J., Paik, H., Oskotsky, B., Krings, G., et al. (2017). Comprehensive analysis of normal adjacent to tumor transcriptomes. Nat. Commun. 8, 1077–1077. doi: 10.1038/s41467-017-01027-z
Banafea, O., Mghanga, F. P., Zhao, J., Zhao, R., and Zhu, L. (2016). Endoscopic ultrasonography with fine-needle aspiration for histological diagnosis of solid pancreatic masses: a meta-analysis of diagnostic accuracy studies. BMC Gastroenterol. 16:108. doi: 10.1186/s12876-016-0519-z
Chen, R., Guan, Q., Cheng, J., He, J., Liu, H., Cai, H., et al. (2017). Robust transcriptional tumor signatures applicable to both formalin-fixed paraffin-embedded and fresh-frozen samples. Oncotarget 8, 6652–6662. doi: 10.18632/oncotarget.14257
Cheng, J., Guo, Y., Gao, Q., Li, H., Yan, H., Li, M., et al. (2017). Circumvent the uncertainty in the applications of transcriptional signatures to tumor tissues sampled from different tumor sites. Oncotarget 8, 30265–30275. doi: 10.18632/oncotarget.15754
Costache, M. I., Costache, C. A., Dumitrescu, C. I., Tica, A. A., Popescu, M., Baluta, E. A., et al. (2017). Which is the best imaging method in pancreatic adenocarcinoma diagnosis and staging - CT. MRI or EUS? Curr. Health Sci. J. 43, 132–136. doi: 10.12865/chsj.43.02.05
Dakhel, S., Padilla, L., Adan, J., Masa, M., Martinez, J. M., Roque, L., et al. (2014). S100P antibody-mediated therapy as a new promising strategy for the treatment of pancreatic cancer. Oncogenesis 3:e92. doi: 10.1038/oncsis.2014.7
Danilova, L., Ho, W. J., Zhu, Q., Vithayathil, T. T., De Jesus-Acosta, A., Azad, N. S., et al. (2019). Programmed cell death ligand-1 (PD-L1) and CD8 expression profiling identify an immunologic subtype of pancreatic ductal adenocarcinomas with favorable survival. Cancer Immunol. Res. 7, 886–895. doi: 10.1158/2326-6066.cir-18-0822
Eddy, J. A., Sung, J., Geman, D., and Price, N. D. (2010). Relative expression analysis for molecular cancer diagnosis and prognosis. Technol. Cancer Res. Treat. 9, 149–159. doi: 10.1177/153303461000900204
Guan, Q., Zeng, Q., Yan, H., Xie, J., Cheng, J., Ao, L., et al. (2019). A qualitative transcriptional signature for the early diagnosis of colorectal cancer. Cancer Sci. 110, 3225–3234. doi: 10.1111/cas.14137
Hosokawa, M., Kashiwaya, K., Eguchi, H., Ohigashi, H., Ishikawa, O., Furihata, M., et al. (2008). Over-expression of cysteine proteinase inhibitor cystatin 6 promotes pancreatic cancer growth. Cancer Sci. 99, 1626–1632. doi: 10.1111/j.1349-7006.2008.00869.x
Irizarry, R. A., Bolstad, B. M., Collin, F., Cope, L. M., Hobbs, B., and Speed, T. P. (2003). Summaries of Affymetrix GeneChip probe level data. Nucleic Acids Res. 31:e15. doi: 10.1093/nar/gng015
Katayama, M., Funakoshi, A., Sumii, T., Sanzen, N., and Sekiguchi, K. (2005). Laminin gamma2-chain fragment circulating level increases in patients with metastatic pancreatic ductal cell adenocarcinomas. Cancer Lett. 225, 167–176. doi: 10.1016/j.canlet.2004.11.052
Klett, H., Fuellgraf, H., Levit-Zerdoun, E., Hussung, S., Kowar, S., Kusters, S., et al. (2018). Identification and validation of a diagnostic and prognostic multi-gene biomarker panel for pancreatic ductal adenocarcinoma. Front. Genet. 9:108. doi: 10.3389/fgene.2018.00108
Liu, H., Li, Y., He, J., Guan, Q., Chen, R., Yan, H., et al. (2017). Robust transcriptional signatures for low-input RNA samples based on relative expression orderings. BMC Genomics 18:913. doi: 10.1186/s12864-017-4280-7
Long, N. P., Jung, K. H., Anh, N. H., Yan, H. H., Nghi, T. D., Park, S., et al. (2019). An integrative data mining and omics-based translational model for the identification and validation of oncogenic biomarkers of pancreatic cancer. Cancers 11:155. doi: 10.3390/cancers11020155
Matsunaga, T., Ohtsuka, T., Asano, K., Kimura, H., Ohuchida, K., Kitada, H., et al. (2017). S100P in duodenal fluid is a useful diagnostic marker for pancreatic ductal adenocarcinoma. Pancreas 46, 1288–1295. doi: 10.1097/mpa.0000000000000940
Nakayama, H., Ohuchida, K., Yonenaga, A., Sagara, A., Ando, Y., Kibe, S., et al. (2019). S100P regulates the collective invasion of pancreatic cancer cells into the lymphatic endothelial monolayer. Int. J. Oncol. 55, 211–222. doi: 10.3892/ijo.2019.4812
Rahib, L., Smith, B. D., Aizenberg, R., Rosenzweig, A. B., Fleshman, J. M., and Matrisian, L. M. (2014). Projecting cancer incidence and deaths to 2030: the unexpected burden of thyroid, liver, and pancreas cancers in the United States. Cancer Res. 74, 2913–2921. doi: 10.1158/0008-5472.Can-14-0155
Shao, F., Huang, M., Meng, F., and Huang, Q. (2018). Circular RNA signature predicts gemcitabine resistance of pancreatic ductal adenocarcinoma. Front. Pharmacol. 9:584. doi: 10.3389/fphar.2018.00584
Siegel, R. L., Miller, K. D., and Jemal, A. (2019). Cancer statistics, 2019. CA Cancer J. Clin. 69, 7–34. doi: 10.3322/caac.21551
Singhi, A. D., Koay, E. J., Chari, S. T., and Maitra, A. (2019). Early detection of pancreatic cancer: opportunities and challenges. Gastroenterology 156, 2024–2040. doi: 10.1053/j.gastro.2019.01.259
Siret, C., Dobric, A., Martirosyan, A., Terciolo, C., Germain, S., Bonier, R., et al. (2018). Cadherin-1 and cadherin-3 cooperation determines the aggressiveness of pancreatic ductal adenocarcinoma. Br. J. Cancer 118, 546–557. doi: 10.1038/bjc.2017.411
Suzuki, R., Lee, J. H., Krishna, S. G., Ramireddy, S., Qiao, W., Weston, B., et al. (2013). Repeat endoscopic ultrasound-guided fine needle aspiration for solid pancreatic lesions at a tertiary referral center will alter the initial inconclusive result. J. Gastrointestin. Liver Dis. 22, 183–187.
Wang, H., Cai, J., Du, S., Wei, W., and Shen, X. (2020). LAMC2 modulates the acidity of microenvironments to promote invasion and migration of pancreatic cancer cells via regulating AKT-dependent NHE1 activity. Exp. Cell Res. 391:111984. doi: 10.1016/j.yexcr.2020.111984
Wang, H., Sun, Q., Zhao, W., Qi, L., Gu, Y., Li, P., et al. (2015). Individual-level analysis of differential expression of genes and pathways for personalized medicine. Bioinformatics 31, 62–68. doi: 10.1093/bioinformatics/btu522
Xuan, J., Yu, Y., Qing, T., Guo, L., and Shi, L. (2013). Next-generation sequencing in the clinic: promises and challenges. Cancer Lett. 340, 284–295. doi: 10.1016/j.canlet.2012.11.025
Yan, H., Li, M., Cao, L., Chen, H., Lai, H., Guan, Q., et al. (2019). A robust qualitative transcriptional signature for the correct pathological diagnosis of gastric cancer. J. Transl. Med. 17:63. doi: 10.1186/s12967-019-1816-4
Keywords: molecular signature, relative expression orderings, early diagnosis, pancreatic cancer, gene pairs
Citation: Zhou Y-J, Lu X-F, Meng J-L, Wang X-Y, Ruan X-J, Yang C-J, Wang Q-W, Chen H-M, Gao Y-J, Yan F-R and Li X-B (2020) Qualitative Transcriptional Signature for the Pathological Diagnosis of Pancreatic Cancer. Front. Mol. Biosci. 7:569842. doi: 10.3389/fmolb.2020.569842
Received: 05 June 2020; Accepted: 26 August 2020;
Published: 23 September 2020.
Edited by:
Kamran Ghaedi, University of Isfahan, IranReviewed by:
Milena Urbini, Romagnolo Scientific Institute for the Study and Treatment of Tumors (IRCCS), ItalyNavid Abedpoor, Royan Institute, Iran
Masoud Baghi, University of Isfahan, Iran
Copyright © 2020 Zhou, Lu, Meng, Wang, Ruan, Yang, Wang, Chen, Gao, Yan and Li. This is an open-access article distributed under the terms of the Creative Commons Attribution License (CC BY). The use, distribution or reproduction in other forums is permitted, provided the original author(s) and the copyright owner(s) are credited and that the original publication in this journal is cited, in accordance with accepted academic practice. No use, distribution or reproduction is permitted which does not comply with these terms.
*Correspondence: Xiao-Bo Li, lxb_1969@163.com
†These authors have contributed equally to this work