- 1School of Life Sciences, Beijing Institute of Technology, Beijing, China
- 2Department of Neurosurgery, Sanbo Brain Hospital, Capital Medical University, Beijing, China
Glioblastoma (GBM) is highly invasive and the deadliest brain tumor in adults. It is characterized by inter-tumor and intra-tumor heterogeneity, short patient survival, and lack of effective treatment. Prognosis and therapy selection is driven by molecular data from gene transcription, genetic alterations and DNA methylation. The four GBM molecular subtypes are proneural, neural, classical, and mesenchymal. More effective personalized therapy heavily depends on higher resolution molecular subtype signatures, combined with gene therapy, immunotherapy and organoid technology. In this review, we summarize the principal GBM molecular classifications that guide diagnosis, prognosis, and therapeutic recommendations.
Introduction
The World Health Organization (WHO) defines adult diffuse gliomas into grade II and grade III astrocytic tumors, grade II and III oligodendrogliomas, and grade IV glioblastomas (Louis et al., 2016). Glioblastoma (GBM) is grade IV, the most invasive and deadly glioma (Brennan et al., 2009; Szopa et al., 2017; Lee et al., 2018; Ghosh et al., 2018; Shergalis et al., 2018; Paolillo et al., 2018). It invades adjacent areas of the brain but rarely spreads outside the brain (Phillips et al., 2006). Clinical data show GBM has a poor prognosis, with less than 5% of patients surviving 5 years after diagnosis (Verhaak et al., 2010). Based on clinicopathologic features, GBM is defined as primary or secondary GBM (Ohgaki and Kleihues, 2013). Primary GBM starts as grade IV, with no evidence of lower grades, and is more aggressive and more likely to affect elderly patients. Secondary GBM develops from astrocytoma (Grade II or III glioma), grows slowly initially then gradually becomes aggressive (Ohgaki and Kleihues, 2007). The mechanism of GBM tumorigenesis is still unclear, many patients relapse due to ineffective treatment options. Notably, recurrent GBM is frequently accompanied by molecular alterations compared with the initial diagnosis (Li et al., 2015; van den Bent et al., 2015; Cioca et al., 2016; Neilsen et al., 2019; Schafer et al., 2019).
Histomorphology ambiguity and tumor heterogeneity pose challenges to GBM diagnosis, prognosis and treatment. Histologic diagnosis often varies among clinicians and limits diagnostic reproducibility. GBM histologically and genetically show significant inter-tumoral and intra-tumoral heterogeneity, differing mutations, and indistinct phenotypic and epigenetic states reflect genomic instability that leads to varying therapy choices and clinical outcomes (Homma et al., 2006; Marusyk and Polyak, 2010; Szerlip et al., 2012; Brennan et al., 2013). Molecular classification of GBM is a newer tool and a complement to the traditional pathology-based description (Verhaak et al., 2010; Brennan et al., 2013; Ceccarelli et al., 2016).
Molecular-based diagnosis, patient stratification, and personalized treatment are increasingly important. The ISN Haarlem recommends “hierarchical diagnosis with histological classification, WHO classification, and molecular information for comprehensive diagnosis” (Louis et al., 2014). In 2016, the WHO updated guidelines combining morphology and genetic variation, leading to a significant reorganization of the classification of several brain tumor entities, especially in gliomas (Louis et al., 2016). Two significant entities of 2016 WHO classification based on IDH (Isocitrate dehydrogenase) gene mutant status are IDH wild-type and IDH mutated GBM; patients whose full IDH evaluation cannot be assessed are classified as GBM NOS (not otherwise specified) (Louis et al., 2016).
Multi-omics studies from the landscape of GBM in the Cancer Genome Atlas Research Network (TCGA), the Chinese Glioma Genome Atlas (CGGA), and other databases, together reveal the complicated genetic profile of GBM (Cancer Genome Atlas Research Network, 2008; Brennan et al., 2013; Zhao Z. et al., 2020). These aberrant molecules, including 1p and 19q co-deletions (oligodendroglioma-specific), IDH gene mutations, PTEN (Phosphatase and tensin homolog) gene mutations, TP53 mutations, TERT (Telomerase reverse transcriptase) gene promoter mutations, ATRX (Alpha thalassemia/mental retardation syndrome X-linked) gene mutations, and EGFR (Epithelial growth factor receptor) gene amplification, are forcing clinicians to reconsider traditional GBM treatment (Mclendon et al., 2008; Brennan et al., 2013). GBM classification based on aberrant molecules shortens the time from diagnosis to treatment, and significantly improves accuracy and targeting.
In this paper, we summarize the process of GBM classification based on transcription levels, genetic alterations, and DNA methylation. We also describe the molecular characteristics of each category, and the relationship between different classification methods. Finally, we provide the current guiding strategy for diagnosis and treatment.
GBM Heterogeneity Identified by Transcription, Genetic Alteration, and DNA Methylation
Deciphering GBM heterogeneity and complexity is the key to understanding it’s progression and creating effective therapies. Some important and aberrant molecular events drive GBM malignant transformation, highlighting the importance of molecular classification. First, GBM has a wide variety of chromosomal changes, including amplification in chromosome 4 (Chr.4, PDGFRA), Chr.7 (EGFR; MET, hepatocyte growth factor receptor; CDK6, Cyclin-dependent kinase 6), Chr.12 (CDK4, Cyclin-dependent kinase 6; MDM2, Mouse double minute 2 homolog), and deletion in Chr.10 (PTEN). Notably, some GBM patients have simultaneous gain of Chr.19 and 20 (Brennan et al., 2013).
Second, the TCGA GBM project describes somatic genome changes based on multidimensional and comprehensive features that show significant mutations in GBM, including TP53 (34.4%), EGFR (32.6%), PTEN (32%), NF1 (Neurofibromin 1, 13.7%), PIK3CA (Phosphatidylinositol 4,5-bisphosphate 3-kinase catalytic subunit alpha isoform, 12%), PIK3R1 (Phosphatidylinositol 3-kinase regulatory subunit alpha, 11.7%), RB1 (Retinoblastoma-associated protein 1, 9.3%), SPTA1 (Spectrin alpha chain, erythrocytic 1, 9%), ATRX (6%), IDH1 (5.2%), KEL (Kell blood group glycoprotein, 5%), PDGFRA (Platelet-derived growth factor receptor A, 4.5%), and GABRA6 (Gamma-aminobutyric acid receptor subunit alpha-6, 4%) (Cancer Genome Atlas Research Network, 2008; Parsons et al., 2008; Verhaak et al., 2010; Brennan et al., 2013).
Lastly, DNA methylation is a key factor when measuring heterogeneity and stratification of GBM patients. Epigenetic modifications of GBM is related to biological characteristics and are considered therapeutic targets (Hegi et al., 2005; Etcheverry et al., 2010; Romani et al., 2018; Carella et al., 2020). DNA methylation states in GBM are correlated with survival, which has been extensively explored in recent years (Lofton-Day and Lesche, 2003; Hegi et al., 2005; Etcheverry et al., 2010; Christensen et al., 2011). GBM genome-wide methylation data show biologically distinct subtypes (Brennan et al., 2013). For example, DNA methylation of the MGMT (O6-Methyl guanine DNA methyltransferase) gene promoter occurs in 48.5% of GBM patients (174/359); MGMT is a known marker for treatment strategy (Parsons et al., 2008). Additionally, GBM patient data show other methylated genes, including GATA6 (GATA binding protein 6) (68.4%), CD81 (CD81 antigen) (46.1%), DR4 (Death receptor 4) (41.3%) and CASP8 (Caspase-8) (56.8%) (Skiriute et al., 2012). Interestingly, H. Noushmehr et al. found CpG island hypermethylation in a distinct subgroup of gliomas (G-CIMP), however only a small number of GBM patients with a positive prognosis belong to G-CIMP phenotype (Noushmehr et al., 2010).
Molecular-Based GBM Classification in Diagnosis and Prognosis Prediction
With the recent development of technology and classification algorithms, GBM is divided into different subtypes based on transcription profiles, genetic alterations, and DNA methylation. This allows targeted therapy based on molecular characteristics of subclasses. For example, clinicians can target the mesenchymal subtype from transcription subtypes in GBM via inhibition of diacylglycerol kinase alpha. In doing so, patients with MGMT methylation had a more robust response to temozolomide (Hegi et al., 2005; Taylor and Schiff, 2015; Olmez et al., 2017). The TCGA GBM project used a multi-platform analysis and comprehensively determined the genomic landscape to better understand the pathogenic and drug-resistant mechanism of GBM (Brennan et al., 2013). Here, we describe the classical classification, and analyze the differences among various GBM subtypes.
Transcription-Based Subtypes
GBM classification based on gene expression profiles initially used microarray technology, then large-scale high-throughput next-generation sequencing technology. The molecular map of GBM is shown in Figure 1A. The classification method proposed by Verhaak et al. has been widely used, includes four subtypes: Proneural, Neural, Classical and Mesenchymal (Verhaak et al., 2010).
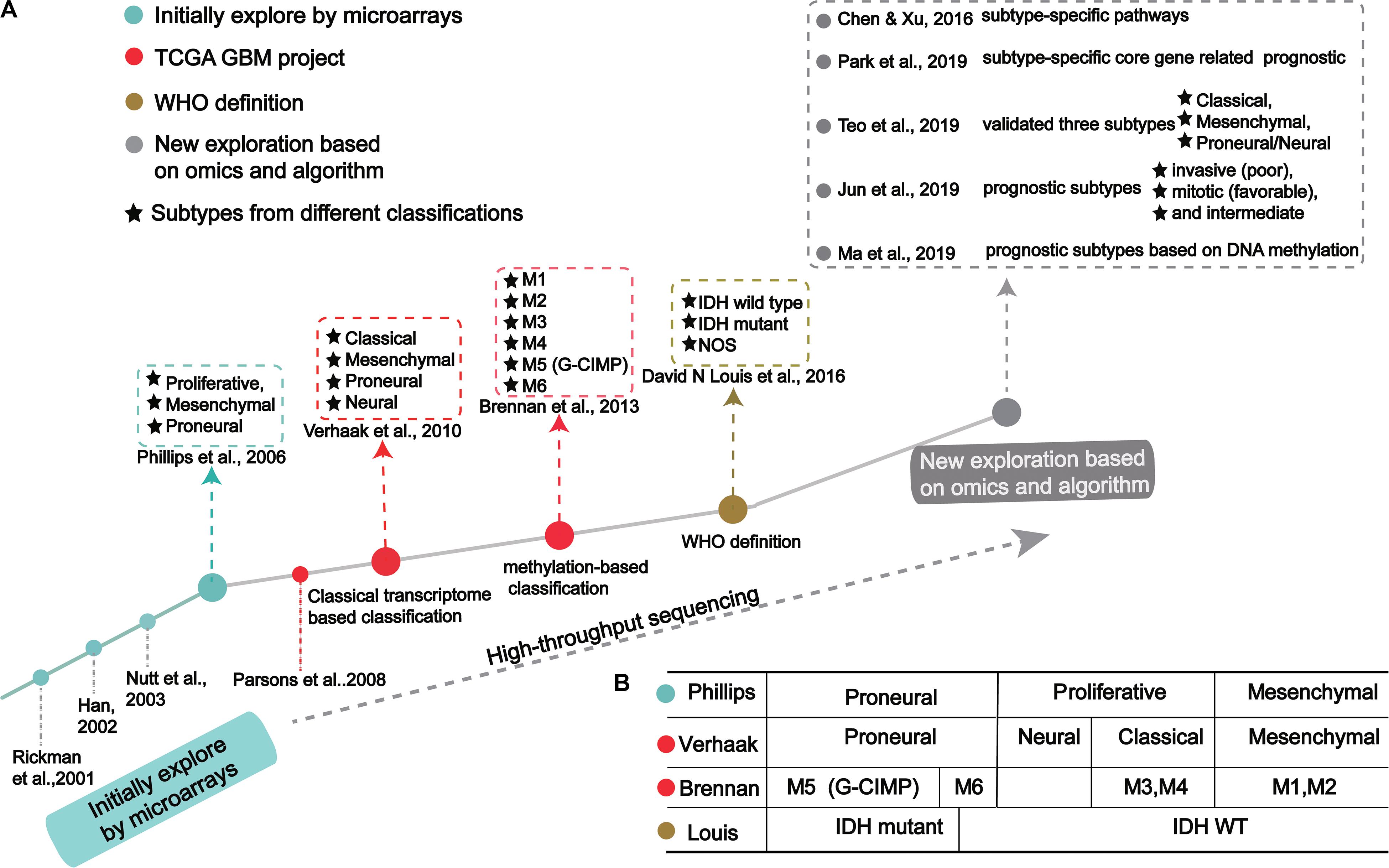
Figure 1. The process of molecular-based GBM classification: (A) GBM classification timeline and classical subtypes. (B) The relative overlap between subtypes from different classification methods.
Initial Exploration on the Transcription-Based Classification
In the 1990s, scientists acquired data from techniques like PCR, allele analysis, and first-generation sequencing to analyze gliomas. They found a variety of molecular markers of different types and grades, but the landscape was not clear (Sehgal, 1998). Indeed, tumor development is highly complex, involving multiple genetic and epigenetic changes. Through microarray investigations, genes associated with GBM were identified and used as biomarkers in early diagnosis, leading many researchers to begin exploration of molecular diagnosis, classification, and treatment (Figure 1A; Schena et al., 1995; Velculescu et al., 1995; Derisi et al., 1996; Dudoit et al., 2002; Irizarry et al., 2003; Hu et al., 2006). Rickman et al. found 360 distinct genes in GBM from pilocytic astrocytomas, including MDM2, IGFBP2 (Insulin-like growth factor-binding protein 2), CD44 (CD44 antigen), and CDK4 (Cyclin-dependent Kinase 4) (Rickman et al., 2001). Sallinen et al. found more than 200 gene expression alterations in GBM and demonstrated a strategy for high-throughput molecular genetic profiling of brain tumors (Sallinen et al., 2000). In addition, Nutt et al. found 14 GBMs and 7 anaplastic oligodendroglioma, diagnosed by pathology, were predicted using gene markers that accurately classified 18 samples (Nutt et al., 2003). The classification prediction model objectively and reliably classifies high-grade non-classical glial tumors (Nutt et al., 2003). Compared with pathological classification, this model reliably predicts the prognosis of atypical lesions more accurately.
In a groundbreaking study, Phillips et al. classified three GBM subtypes: Proneural, Proliferative and Mesenchymal (Figure 1 and Table 1; Phillips et al., 2006). Proneural subtypes are more common in young patients, less pathological compared with proliferative or interstitial GBM and have a better prognosis (Phillips et al., 2006). NCAM (Neural cell adhesion molecule), GABBR1 (Gamma-aminobutyric acid type B receptor subunit 1), and SNAP91 (Clathrin coat assembly protein AP180) are associated with neurons and are more similar to normal brain tissue and expression in proneural subtype (Phillips et al., 2006). The Proliferative subtype is similar to stem cells with significantly up-regulated markers of proliferation, including TOP2A (DNA topoisomerase II alpha) and PCNA (Proliferating cell nuclear antigen) (Phillips et al., 2006). In contrast, the Mesenchymal subtype displays overexpression of angiogenesis markers, including the endothelial marker PECAM1 (Platelet endothelial cell adhesion molecule) gene, VEGF (Vascular endothelial growth factor) gene, VEGFR1 (Vascular endothelial growth factor receptor 1) gene and VEGFR2 (Vascular endothelial growth factor receptor 2) gene, which shows mesenchymal and angiogenic characteristics (Phillips et al., 2006). Proliferative and Mesenchymal subtypes are characterized by activation of PI3K/AKT (Phosphoinositide 3-kinase/Protein kinase B) signaling, loss on Chr.10 (location of PTEN), gain on Chr.7 (location of EGFR), and poor prognosis with invasive growth and angiogenic pathways (Phillips et al., 2006). These three subtypes are reminiscent of the various stages of developmental neurogenesis, which provides the basis and perspective for the molecular classification of GBM.
Deep Analysis of Transcription-Based Classification
The above studies demonstrated tumors often cluster in groups that display heterogeneity, highlighting the weaknesses of conventional diagnosis. With the advent of large-scale, high-throughput, next-generation sequencing methods, and with algorithms in machine learning, complex tumor data is becoming more precise.
Verhaak et al. (2010) offered more in-depth research and treatment possibilities for GBM (Figure 1A) based on the four subtypes Proneural, Neural, Classical and Mesenchymal (Table 1). The Proneural subtype is found primarily in younger patients, characterized by high PDGFRA gene expression and frequent IDH1 mutation. Compared with the other three subtypes, the Proneural subtypes may have better survival rates. However, Proneural subtypes showed no significant difference from other subtypes in response to chemotherapy and radiotherapy (Colman et al., 2010). The Neural subtype has similar gene expression patterns compared with normal brain tissue and tends to be more responsive to radiation and chemotherapy. GBMs with neural markers like SYT1 (Synaptotagmin 1), SLC12A5 (Solute carrier family 12 members 5), GABRA1 (Gamma-aminobutyric acid type A receptor alpha1) and NEFL (Neurofilament light polypeptide), are classified as the Neural subtype. The Classical subtype shows aberrant changes, including Chr.7 amplification, Chr.10 loss, inactivation of the RB (Retinoblastoma-associated protein) pathway, and focal 9p21.3 homozygous deletion. In addition, Sonic hedgehog pathways (SMO, Smoothened homolog; GAS1, Growth arrest-specific protein 1; GLI2, Growth arrest-specific protein 2), Notch signaling pathways (NOTCH3, Neurogenic locus notch homolog protein 3; JAG1, Jagged1; LFNG, Lunatic fringe) and the neural precursor and stem cell marker NES are highly expressed in the Classical subtype. Importantly, patients with Classical subtype show a significant reduction in mortality with aggressive radiotherapy and chemotherapy. The Mesenchymal subtype is characterized by extensive necrosis and inflammation, upregulation of interstitial and angiogenesis genes, deletion of tumor suppressor genes P53, PTEN, and NF1, and high expression of genes in the tumor necrosis factor superfamily and the NF-κB pathway. Although responsive to aggressive radiotherapy and chemotherapy, the prognosis of Mesenchymal subtypes is the worst among all subtypes (Colman et al., 2010). Recently, Sharma et al. found that VEGF-A (Vascular endothelial growth factor A), VEGF-B (Vascular endothelial growth factor B), ANG1 (Angiopoietin 1) and ANG24 (Angiopoietin 24) genes are highly expressed in the Mesenchymal subtype (Sharma et al., 2017).
In 2017, Wang et al. (2017) proved that GBM tumor cells include Classical, Proneural, and Mesenschymal, and Neural subtype is non-tumor cells in the tumor microenvironment. They found the median survival of Mesenchymal, Classical, or Proneural are 11.5, 14.7, and 17.0 months, respectively. Wang’s classification is based on tumor cells rather than microenvironmental/non-malignant tumor cells in tumor entities.
Using cancer genome data from the TCGA GBM project and classification from Verhaak et al. (2010) and Park A. K. et al., 2019 identified subtype-specific prognostic core genes and further examined prognostic chromosome changes and mutations (Figure 1A). Specific prognostic core genes in Classical subtype exist in DNA repair, cell cycle, Janus kinase, and transcription activation factor (JAK-STAT) pathway. And, specific prognosis genes in Mesenchymal subtype are related to mesenchymal cell movement, PI3K/AKT pathway, Mitogen-activated protein kinase (MAPK) pathways, extracellular signal-regulated kinase (ERK) pathways, and Wnt pathways (Park A. K. et al., 2019). Notably, patients with Mesenchymal subtypes with PIK3R1 or PCLO (Protein piccolo) mutations show a poorer prognosis (Park A. K. et al., 2019). These results demonstrate specific molecular targets and biomarkers for each subtype of GBM.
Recent studies offer new insights into GBM classification based on transcription. Teo et al. validated three robust GBM-subtypes: Proneural/Neural, Classical, and Mesenchymal across six different datasets (Figure 1A; Verhaak et al., 2010; Teo et al., 2019). This was validated in subtype-specific patient-derived orthotopic xenograft (PDOX) mice; the Classical subtype showed no survival difference between radiotherapy and temozolomide monotherapy. A Proneural/Neural specific-PDOX model showed temozolomide significantly improved survival compared to radiotherapy. This points to better predictive clinical outcomes based on more precise patient selection in clinical trials.
Park J. et al. (2019) identified three subtypes related to prognosis prediction: Mitotic (favorable), Intermediate, and Invasive (poor) by analyzing and verifying four large-scale gene expression profiles (Figure 1A). These new GBM subtypes have different multi-omics features and biological phenotypes. Among GBM prognostic subtypes, the invasiveness in the Invasive subtype is significantly higher than the Mitotic subtype. Interestingly, the methylated MGMT gene promoter is correlated with the Mitotic subtype, indicating Mitotic subtype patients are more likely to respond to temozolomide (Park J. et al., 2019). This study suggests that treatment strategies should be based on prognostic subtypes. For example, patients in the Mitotic subtype can be treated with temozolomide, while patients in Invasive subtypes require therapeutic intervention for the aggressiveness of the GBM. Although the prognostic subtype is based only on transcription and survival time, genomic features such as pathogenic somatic variations of IDH1 and ATRX and DNA methylation are only present in Mitotic subtypes. Since these three subtypes suggest a prognosis for GBM, inhibition of target genes in different subtypes may improve patient survival. Further, these genes may have clinical value as prognostic biomarkers and new drug targets, while also leading to new pathological and etiological factors for the oncogenesis and development for GBM.
Genetic Alteration-Based Subtypes
In recent years, large-scale genomic studies have revealed many mutations in tumor suppressor genes and oncogenes, and significantly improved our understanding of GBM. Specifically, mutated IDH, PTEN and EGFR are related to patient survival and can be used as indicators of patient classification.
IDH-Wild Type and IDH-Mutation Type
The identification of the IDH mutation is an important contribution to the molecular pathology of GBM. In 2008, Parsons et al. (2008) found the IDH1 gene had a point mutation in a small number of glioblastoma samples. Subsequently, Yan et al. (2009) found that GBM patients with IDH1/IDH2 mutations had a higher survival rate than those without these mutations (Table 2). Many studies have shown that patients with IDH mutations are significantly different from those without IDH mutations in molecular and clinical characteristics, including prognosis (Ichimura et al., 2009; Nobusawa et al., 2009; Watanabe et al., 2009; Yan et al., 2009; Lu et al., 2012; Songtao et al., 2012; Stancheva et al., 2014; Mondesir et al., 2016). There are three IDH enzymes: IDH1, IDH2, and IDH3 (Yan et al., 2009). IDH1 is mainly cytoplasmic, while IDH2 and IDH3 are mostly present in the mitochondrial matrix. IDH is the central enzyme in the citric acid cycle and plays a vital role in oxidative stress resistance (Marko and Weil, 2013). The most common IDH1 mutation observed in gliomas is the point mutation at position 132 (R132H), which is regarded as a typical IDH1 mutation (Parsons et al., 2008).
In 2016, the WHO divided it into two: IDH mutation and IDH wild type (Figure 1A; Louis et al., 2016). IDH wild type GBM with poor survival is dominated by stellate cell differentiation, characterized by nuclear atypia, cell polymorphism, typical diffuse growth patterns, mitotic activity and microvascular proliferation and/or necrosis. There are three variants of IDH wild-type, including giant cell GBM, gliosarcoma and epithelial-like GBM (Ep-GBM) (Louis et al., 2016). Genetically, giant cell GBM lacks EGFR amplification and homozygous CDKN2A deletion and contains PTEN mutation and TP53 mutation (Meyer-Puttlitz et al., 1997). Patients with the giant cell GBM have outcomes similar to classical GBM. In gliosarcoma, TP53 mutations are rare, and EGFR amplification is also uncommon, and contains CDKN2A deletion (Lowder et al., 2019). The clinical outcome of gliosarcoma differs from classical GBM, but there are still conflicting and uncertain results from various studies. Ep-GBM, as a new variant of GBM, is more prevalent in children and young people, manifesting as superficial brain or mesencephalic masses, and often carries BRAF (Serine/threonine-protein kinase B-Raf) V600E mutations (Chapman et al., 2011; Kleinschmidtdemasters et al., 2013; Broniscer et al., 2014). Ep-GBM is based on the absence of INI1 expression, distinguishing it from similar epithelioid counterparts (Kleinschmidt-DeMasters et al., 2010). Additionally, Ep-GBM often lacks EGFR amplification and PTEN loss, but ODZ3 usually has hemizygous deletions (Alexandrescu et al., 2016).
Multiple studies have confirmed that IDH mutations have prognosis and predictive value (Yan et al., 2009; Beiko et al., 2014; Stancheva et al., 2014). Compared to GBM patients with wild-type IDH, IDH-mutant GBM patients had higher overall survival and were more responsive to temozolomide (Songtao et al., 2012). The inhibitor of IDH mutation, which has been applied in preclinical models, shows activity to retard glioma cell growth (Rohle et al., 2013).
Other Genetic Mutations
In IDH1 wild type GBM, the median survival rate of patients with CDK4/MDM2 co-amplification is 6.6 months after diagnosis, while the median survival rate of patients without an CDK4/MDM2 co-amplification is 12.7 months (Abedalthagafi et al., 2018). The TERT promoter mutation was recently identified as a sign of poor prognosis. It is enriched in elderly patients, with approximately 40% having grade II/III glioma, suggesting TERT’s correlation with shorter overall survival as a key pathological player and therapeutic target (Chamberlain and Sanson, 2015; Mosrati et al., 2015; Spiegl-Kreinecker et al., 2015; Yang et al., 2016; Yuan et al., 2016).
EGFR amplification is usually accompanied by EGFR mutation, the most frequent being EGFRvIII (Gan et al., 2009). Under normal physiological conditions, EGFR plays a central role in cell proliferation, differentiation and development. EGFR is located on the short arm of Chr.7 (7p12) and encodes a cell surface receptor tyrosine kinase (Hatanpaa et al., 2010). EGFRvIII is characterized by the absence of 267 amino acids in the extracellular domain, resulting in the inability of the receptor to bind to the ligand but with substitutive activity (Hatanpaa et al., 2010). EGFRvIII enhances the tumorigenic potential of GBM by activating and maintaining mitotic and anti-apoptotic signaling pathways, along with their impaired internalization and degradation (Gan et al., 2009). Some studies have found that EGFRvIII overexpression and EGFR amplification are associated with poor prognosis in young patients, and other data show EGFR overexpression is associated with poor prognosis in elderly patients (Shinojima et al., 2003; Srividya et al., 2010). But recently, Felsberg et al. found EGFRvIII and EGFR SNVs are not prognostic; Chen et al. showed that there is insufficient evidence for the presence of either EGFR amplification or EGFRvIII mutation has prognostic value in patients with GBM using meta-analysis (Chen et al., 2015; Felsberg et al., 2017). These results may be biased by the inherent variability in subtypes, therefore, the exploration of the relationship between EGFR and prognosis needs to be carried out in different subtypes. Notably, compared with patients with both TERT and EGFR gene mutations, the overall survival of TERT/EGFR wild-type patients (EGFR not amplified) is almost twice that of the former (Chamberlain and Sanson, 2015; Yang et al., 2016).
The PTEN protein catalyzes the dephosphorylation of 3’ phosphorylation of the inositol ring in PIP3 (phosphatidylinositol-3,4,5-trisphosphate) to produce PIP2 (phosphatidylinositol-4,5-bisphosphate). The dephosphorylation is critical because it inhibits the AKT signaling pathway. The PI3K/AKT pathway is normally dormant in differentiated and quiescent cells, but when activated, the cell cycle modulation leads to cancer. The deficiency of PTEN mainly plays the role of lipid phosphatase through the PI3K/AKT pathway (Endersby and Baker, 2008). Therefore, the loss of PTEN is associated with a more aggressive phenotype.
In addition to the genes above, other genetic mutations also drive GBM development. However, the mutation or deletion of a single gene may not serve to classify GBM independently. The combination of aberrant events related to survival may be a more effective classifier. Data suggest combination of two or three genes provide a robust classifier to diagnostic analysis for clinical applications (Kim et al., 2002). Classification driven by genetic mutation (single or consistent) is the basis for exploring GBM classification, and critical genetic targets can be used as the key for diagnosis, prognosis and treatment.
DNA Methylation-Based Subtypes
Epigenetic changes are common markers of human cancers, including GBM (Kim and Kim, 2008; Romani et al., 2018). DNA methylation is a core element of epigenetic alteration, an essential signaling tool for regulating genomic functions, and a key feature mediating tumorigenesis (Koch et al., 2018; Muhammad et al., 2018; Yamashita et al., 2018). DNA methylation can provide biomarkers for the early diagnosis and prognosis of cancer and provide a new method for further clinical applications (Lofton-Day and Lesche, 2003; Gustafsson et al., 2018; Kim et al., 2018; Li et al., 2018, 2019; Pérez et al., 2018. We know the methylation status of single genes corresponds to expression levels in GBM (Bell et al., 2018; Johannessen et al., 2018). MGMT promoter methylation is a prognostic factor for glioblastoma patients and has a significant correlation with worse survival rates (16.9 months vs. 12.7 months) (Brennan et al., 2013). Due to the diversity of GBM, a broader genome and expression profile is needed to gain insight into the potential response of treatment methods.
Brennan et al. used large-scale methylated sequencing data to classify GBM, divided into six categories based on the expression level of DNA methylation, including Cluster M1 to Cluster M6, in which Cluster M5 was G-CIMP subtype (Figure 1A; Brennan et al., 2013). Cluster M6 is relatively hypomethylated and has the majority of IDH1 wild type patients than the G-CIMP subtype. Cases of missense mutations or deletions in MLL (Histone-lysine N-methyltransferase 2A) genes or HDAC (Histone deacetylase) family genes were concentrated in Cluster M2 (Brennan et al., 2013). These results indicate that the classification based on DNA methylation makes GBM classification clearer.
Recently, Ma et al. (2019) identified specific prognostic subtypes based on DNA methylation status and identified 3 GBM methylation clusters (Cluster 1, Cluster 2, and Cluster 3), which have significantly different survival curves (Figure 1A). Among all clusters, Cluster 2 has the best prognosis. The methylation levels in each cluster are related to specific molecular characteristics. Compared with Cluster1 and Cluster2, Cluster 3 showed more TP53 mutations and deletion of wildtype IDH1 and 1p/19q. The genes corresponding to the promoter region of the CpG site annotation are related to the survival and biological processes in GBM. By focusing on the level of DNA methylations in patients with GBM, researchers eventually developed a new prediction panel for 10 CpGs. They are superior to other molecular indicators because these 10 CpG signals reflect the relationship between GBM intrinsic tumor subtypes (Kloosterhof et al., 2013; Paul et al., 2017; Yin et al., 2018). The study also found the enriched CpG sites in genes involved in neuronal differentiation and brain development, including KIFC3 (Kinesin-like protein), OC90 (Otoconin-90), CRB2 (DNA repair protein crb2), IGSF22 (Immunoglobulin superfamily member 22) and NR0B2 (Nuclear receptor subfamily 0 group B member 2) (Wu et al., 2010; Yu et al., 2013).
DNA methylation provides a framework for understanding GBM and guiding a therapeutic strategy. It has offered more molecular biomarkers for each subtype and suggested more targets for treatment. Methylation is a powerful complement to classification based on genetic alterations and transcription, making GBM classification more comprehensive.
The Relationship Among Transcription, Genetic Alterations and DNA Methylation Classifications
Early attempts to identify specific tumor subtypes generally focused only on gene expression patterns. But biological processes are not so simply regulated. Omics data have helped identify clusters of tumors with similar characteristics, including genotypic and epigenetic regulation. Many studies have found that molecular subtypes classified at different levels are related and overlapped, as exampled from the four transcription-based subtypes from Verhaak et al. (2010), six DNA methylation-based subtypes from Brennan et al. (2013) and IDH mutation-based subtypes (Figure 1B). Combined analysis with four transcriptome-based subtypes of TCGA, Cluster M1 and M2 are enriched in the Mesenchymal subtypes, Cluster M3 and M4 in the Classical subtype, Cluster G-CIMP in the Proneural subtype, and Cluster M6 is relatively hypomethylated, which belongs to the Proneural subtype (Verhaak et al., 2010). Notably, Cluster G-CIMP increases the likelihood of DNA methylation of MGMT (79% of patients with DNA methylation of MGMT in Cluster G-CIMP and 46% in non-G-CIMP). Interestingly, MGMT DNA methylation is a predicted biomarker of classical subtypes, but not other subtypes. In addition, C-CIMP is a unique and almost invariable feature of IDH1/2 mutant GBMs, and studies have shown that patients with this GBM subtype have a better prognosis (Noushmehr et al., 2010; Baysan et al., 2012). According to the characteristic of DNA methylation pattern causally related to IDH1/2 mutation status and better prognosis, the Proneural subtype is further subdivided into G-CIMP positive and negative groups (Noushmehr et al., 2010).
Molecular Subtype Migration in Recurrent GBM
Recently, some studies have shown that subtype migration and molecular changes occur in recurrent GBM, highlighting the need for further research (Wang et al., 2016). The recurrence of GBM is inevitable, although current standards of care for GBM patients include chemotherapy after surgical resection (Stupp et al., 2005). However, when GBM occurs, the tumor always recurs, and treatment options are limited. There is no standard care for patients with relapsed GBM because pathological and molecular features are lacking (Weller et al., 2012; Lukas and Mrugala, 2016). The progression-free survival of recurrent GBM is 2–4 months, and the survival of conventional chemotherapy after progression is 6–8 months (Gorlia et al., 2012).
Transcription-based molecular subtypes are also associated with tumor recurrence. For example, Wang et al. found that two-thirds of patients with primary GBM switched transcriptional subtype after recurrence. Importantly, the Mesenchymal subtype was the most stable primary GBM subtype (Wang et al., 2016). Therefore, further analysis of the molecular changes of recurrent GBM poses significant value in guiding treatment. van den Bent et al. showed that half of recurrent GBM patients lost EGFRvIII compared with the molecular expression of GBM at initial diagnosis (Table 3; van den Bent et al., 2015). Cioca et al. found recurrent GBM had lower EGFR expression than primary GBM in 10 cases, and only one case had increased expression on recurrence (Table 3; Cioca et al., 2016). The discrepancies of EGFR expression between primary GBM and recurrence suggest heterogeneity of GBMs is actively fluid. Neilsen et al. analyzed 10 pairs of matched primary and recurrent GBM through genomic changes, and the results indicate all matched tumor pairs showed differences. This study showed that EGFR mutation increased significantly in 3 cases, and the other three genes were generally changed in primary GBM and recurrent GBM, namely CDKN2A and CDKN2B deletion, and TERT mutation. Mutations that cause activation of the PI3K pathway are also common (Table 3; Neilsen et al., 2019). Kim et al. (2015) found that recurrent GBM had a hypermutant phenotype that initially occurred in the IDH1 mutant, suggesting IDH1 is associated with a hypermethylated phenotype, resulting in MGMT inhibition, making tumors more susceptible to mutagenesis by temozolomide.
Studies focused on recurrent GBM have shown molecular composition and molecular subtypes of tumors evolve in response to radiotherapy and targeted therapy, therefore molecular signatures guiding treatment protocols may improve patient survival (Campos et al., 2016). However, it is still challenging to develop new molecular therapies for recurrent GBM patients and personalized treatment.
Molecular Subtypes and Signatures Guiding Clinical Treatment
Subtype-Specific Molecular Guidance for the Selection of Targeted Drugs
Treatment corresponding to tumor subtypes is an effective strategy to avoid the obstacles caused by molecular heterogeneity (Collisson et al., 2011; Linnekamp et al., 2015; Zhao S. et al., 2020). Chen et al. analyzed the relationship between four subtypes distinguished by Verhaak (Figure 1A; Verhaak et al., 2010; Chen and Xu, 2016). The gene signatures in the Mesenchymal subtype is highly enriched in pathways associated with immune response, such as Hepatic Fibrosis/Hepatic Stellate Cell Activation, Coagulation System and IL-10 Signaling. The gene signatures in Proneural subtype are significantly enriched in pathways associated with cellular processes, such as Wnt/β-catenin Signaling and Cyclins and Cell Cycle Regulation. Signatures in the Neural subtype are significantly enriched in pathways associated with nervous system pathways and environmental information processing, such as nNOS Signaling in Skeletal Muscle Cells and cAMP-mediated signaling. Finally, the gene signatures in the Classical subtype are significantly enriched in pathways associated with the metabolism pathways, the nervous system and immune system, such as Fatty Acid Activation, CREB Signaling in Neurons, and PI3K Signaling in B Lymphocytes. They found the response to temozolomide in Classical and Mesenchymal subtypes was higher than that of neurotypes, and the Proneural subtype was lower than these three subtypes. They also developed a computational drug repurposing approach to predict GBM drugs based on the molecular subtypes. Protein kinase inhibitors, antipsychotics, and antidepressants have been identified as the most common drugs for all four subtypes. But in different subtypes, the ranking of drugs is different. In the Proneural subtype, antidepressants and antipsychotics were more effective. Anti-globulin inhibitors of the Mesenchymal subtype are involved in many immune system pathways and phenotypes. These results indicate that different molecular subtypes respond differently to drugs, and GBM subtype-specific therapies should be used.
Further evidence of molecularly guided treatment comes from Sandmann et al. that showed a 4.3 month increase in median survival with the addition of bevacizumab for IDH1 wild-type GBM in the proneural subgroup (Sandmann et al., 2015). The IDH1 R132H vaccine has been developed and shown promising results in animal models of IDH mutant glioblastomas (Schumacher et al., 2014; Dimitrov et al., 2015). These results demonstrate the necessity of diagnosing and developing personalized treatment plans according to IDH status.
Temozolomide is an oral alkylation agent. The main mechanism of temozolomide arrests the cell cycle at G2/M checkpoint, which leads to apoptosis of cancer cells (Alonso et al., 2007). The study showed the median survival was 12 months for patients receiving both temozolomide and radiation therapy and only 8 months for patients receiving radiation therapy alone (Alonso et al., 2007). However, in GBM, due to individual differences, the lack of MGMT methylation in some patients leads to the formation of temozolomide resistance. Herrlinger et al. found that for patients newly diagnosed, without MGMT methylation and with irinotecan/bevacizumab/radiation combination therapy had significantly prolonged mPFS (median progression – free survival) of 9.7 months. Temozolomide/radiation had significant mPFS of 5.9 months, an encouraging result that supports further investigation with this combination (Herrlinger et al., 2014). Therefore, before temozolomide treatment, it is advised to determine the methylation status of MGMT for most effective strategy.
Due to the heterogeneity of GBM, individualized treatment based on specific tumor subtype is clearly a more effective clinical strategy. Gene mutations in TP53, IDH-1 and PDGFR-A in Proneural subtype; mutations or amplification of EGFR gene in Classical subtype; NF-1 gene mutations in Mesenchymal subtype; and the expression of neural markers in Neural subtype are promising therapeutic targets. Several studies have shown that targeting these molecules improves treatment. For example, Sang et al. found the efficacy of SHP099, a potent, selective, and oral SHP-2 inhibitor for treating GBM with activated PDGFR-A signaling; and Liu et al. proved that the third-generation EGFR inhibitor osimertinib overcomes primary resistance by continuously blocking ERK signaling in GBM (Liu et al., 2019; Sang et al., 2019).
Due to subtype migration and molecular changes after recurrence, molecular evaluation of patients must be performed prior to chemotherapy. Patients undergoing surgical resection must undergo immunohistochemical studies to determine various predictors, such as MGMT methylation, to assist in treatment planning.
Individualized Treatment
Gene Therapy
Gene therapy aims to introduce genetic material into cells to compensate for abnormal genes or to make beneficial proteins. If a mutated gene causes a necessary protein loss, gene therapy can introduce a normal gene to supplement the protein’s function. Gene therapy is the delivery of a gene through a vector to a cell. Viruses are often used as vectors because they can deliver new genes by infecting cells. These viruses are modified so that when they are used in humans, they do not cause disease. Adenovirus (AAV) vectors have been used to inject directly into GBM cells in the brain to express tumor-killing genes. Crommentujin et al. demonstrated the AAV9 vector, which produces the anticancer agent sTRAIL, killed up to 60% of GBM cells in mouse models and transfected cell lines (Gray et al., 2011; Crommentuijn et al., 2016). AAV9 virus vector is an excellent choice because its serotype can cross the blood-brain barrier during intravenous administration (Gray et al., 2011). CRISPR gene editing also belongs to gene therapy. By combining Cas9 nuclease with synthetic guide RNA and introducing it into the cell, the cell genome can be accurately trimmed, allowing existing genes to be removed or new ones added (Hendel et al., 2015). Using gene therapy technology to repair and compensate the tumor suppressor gene mutation in each subtype of GBM patients, such as PTEN mutation in the Classical subtype, may improve the survival time of patients.
Immunotherapy
Immunotherapy offers the promise of a sustained antitumor immunity that is pathway independent and has the potential to amplify antigens to boost immune responses. Peptide vaccines, such as EGFRvIII found in Classical GBM subtypes, can trigger immunity to GBM tumor cells expressing EGFRvIII. In a phase II trial involving 18 patients, EGFRvIII patients showed an overall survival of 26 months, compared with only 15 months for the control group (Heimberger, 2005). The vaccine has a promising future in immunotherapy for GBM. Tumor-specific antigen vaccines require confirmation that the tumor expresses the targeted antigen. Thus, immunotherapy limits the scope of these vaccines and the population in which they can be used, so specific vaccines can be designed according to the expression of molecules in different subtypes.
Organoid Model
Glioblastoma organs can be an effective model for rapid testing of personalized treatment strategies. The models allow researchers to reconstruct key features of a patient’s diseased brain to help paint a clearer picture of the cancer and then allow researchers to explore the best ways to treat it. Researchers have successfully transplanted eight glioblastoma organoids (GMOs) samples into brains of adult mice, administering standard care and targeted therapy to GBOs, including clinical trial drugs and chimeric antigen receptor T (CAR-T) cell immunotherapy (Jacob et al., 2020). For each treatment, the researchers showed that organ-like responses were different, and the effect was linked to genetic mutations in the patient’s tumor. The model opens the possibility of future clinical trials that can personalize treatment based on how a patient’s tumor responds to different drugs. Notably, the researchers have observed the benefits of treating organ-like organs with CAR-T therapy in clinical trials for EGFRvIII mutations, a driver of the disease. In 6 cases of GBOs, the EGFRvIII mutation was shown to have a specific effect on patient GBOs, with increased CART cells and decreased EGFRvIII expression cells (Jacob et al., 2020). These results highlight the potential of using personalized approaches to detect and treat glioblastoma.
Conclusion
The unique and highly reproducible molecular changes discovered in recent years have begun to elucidate the diversity of GBM and contribute to the more effective classification of tumors. These studies provide insights into how to improve current treatment strategies. GBM genomics, transcription, and epigenetic features reveal critical molecular changes that may lead to pathologic disease progression. Large-scale analysis, like the TCGA project, confirm that GBM is a heterogenetic tumor at the molecular level that can be subdivided into different subtypes according to the molecular pathogenesis and biological entities of “driving factor” lesions. Although these comprehensive studies provide useful insights into the characteristics and classification of tumors, their limitations need to be considered when drawing conclusions. Some prognostic markers have appeared in these studies, and there is still a great need to identify true predictive markers to improve the treatment process of personalized care. In addition, the intra-tumoral heterogeneity of GBM needs to be further classified by single-cell sequencing technology to obtain a more complete and more precise inter-tumoral and intra-tumoral classification. We still need large-scale animal experiments and human clinical verification to improve treatment response and survival time among the different subtypes.
Through these molecular-level studies, we can further improve the molecular detection methods, guide the targeted therapy based on molecular classification, and form a set of accurate GBM molecular therapy manuals that can improve patient outcome.
Author Contributions
QX and PZ contributed to the conception and design of manuscript. PZ drafted the manuscript. QX provided useful comments and suggestions. LD and QX revised the manuscript. All authors reviewed and approved the final manuscript.
Funding
This work was supported by grants from the Beijing Natural Science Foundation (Z190018), the National Natural Science Foundation of China (81870123), the China Postdoctoral Science Foundation Grant (2018M641206), and the National Science Foundation for Young Scientists of China (81902545).
Conflict of Interest
The authors declare that the research was conducted in the absence of any commercial or financial relationships that could be construed as a potential conflict of interest.
References
Abedalthagafi, M., Barakeh, D., and Foshay, K. M. (2018). Immunogenetics of glioblastoma: the future of personalized patient management. NPJ Precis. Oncol. 2:27. doi: 10.1038/s41698-018-0070-1
Alexandrescu, S., Korshunov, A., Lai, S. H., Dabiri, S., Patil, S., Li, R., et al. (2016). Epithelioid glioblastomas and anaplastic epithelioid pleomorphic xanthoastrocytomas-same entity or first cousins? Brain Pathol. 26, 215–223. doi: 10.1111/bpa.12295
Alonso, M. M., Gomez-Manzano, C., Bekele, B. N., Yung, W. K. A., and Fueyo, J. (2007). Adenovirus-based strategies overcome temozolomide resistance by silencing the O6-Methylguanine-DNA methyltransferase promoter. Cancer Res. 67, 11499–11504. doi: 10.1158/0008-5472.can-07-5312
Baysan, M., Bozdag, S., Cam, M. C., Kotliarova, S., Ahn, S., Walling, J., et al. (2012). G-cimp status prediction of glioblastoma samples using mRNA expression data. PLoS One 7:e47839. doi: 10.1371/journal.pone.0047839
Beiko, J., Suki, D., Hess, K. R., Fox, B. D., Cheung, V. J., Cabral, M., et al. (2014). IDH1 mutant malignant astrocytomas are more amenable to surgical resection and have a survival benefit associated with maximal surgical resection. Neuro Oncol. 16, 81–91. doi: 10.1093/neuonc/not159
Bell, E. H., Zhang, P., Fisher, B. J., Macdonald, D. R., McElroy, J. P., Lesser, G. J., et al. (2018). Association of MGMT promoter methylation status with survival outcomes in patients with high-risk glioma treated with radiotherapy and temozolomide. Jama Oncol. 4:1405. doi: 10.1001/jamaoncol.2018.1977
Brennan, C., Momota, H., Hambardzumyan, D., Ozawa, T., Tandon, A., Pedraza, A., et al. (2009). Glioblastoma subclasses can be defined by activity among signal transduction pathways and associated genomic alterations. PLoS One 4:e7752. doi: 10.1371/journal.pone.0007752
Brennan, C. W., Verhaak, R. G., McKenna, A., Campos, B., Noushmehr, H., Salama, S. R., et al. (2013). The somatic genomic landscape of glioblastoma. Cell 155, 462–477. doi: 10.1016/j.cell.2013.09.034
Broniscer, A., Tatevossian, R. G., Sabin, N. D., Klimo, P., Dalton, J., Lee, R., et al. (2014). Clinical, radiological, histological and molecular characteristics of paediatric epithelioid glioblastoma. Neuropathol. Appl. Neurobiol. 40, 327–336. doi: 10.1111/nan.12093
Campos, B., Olsen, L. R., Urup, T., and Poulsen, H. S. (2016). A comprehensive profile of recurrent glioblastoma. Oncogene 35, 5819–5825. doi: 10.1038/onc.2016.85
Cancer Genome Atlas Research Network (2008). Comprehensive genomic characterization defines human glioblastoma genes and core pathways. Nature 455, 1061–1068. doi: 10.1038/nature07385
Carella, A., Tejedor, J. R., Garcia, M. G., Urdinguio, R. G., Bayon, G. F., Sierra, M., et al. (2020). Epigenetic downregulation of TET3 reduces genome-wide 5hmC levels and promotes glioblastoma tumorigenesis. Int. J. Cancer 146, 373–387. doi: 10.1002/ijc.32520
Ceccarelli, M., Barthel Floris, P., Malta Tathiane, M., Sabedot Thais, S., Salama Sofie, R., Murray Bradley, A., et al. (2016). Molecular profiling reveals biologically discrete subsets and pathways of progression in diffuse glioma. Cell 164, 550–563. doi: 10.1016/j.cell.2015.12.028
Chamberlain, M. C., and Sanson, M. (2015). Combined analysis of TERT, EGFR, and IDH status defines distinct prognostic glioblastoma classes. Neurology 84:2007. doi: 10.1212/wnl.0000000000001625
Chapman, P. B., Hauschild, A., Robert, C., Haanen, J. B. A. G., Ascierto, P. A., Larkin, J., et al. (2011). Improved survival with vemurafenib in melanoma with BRAF V600E mutation. N. Engl. J. Med. 364, 2507–2516.
Chen, J. R., Xu, H. Z., Yao, Y., and Qin, Z. Y. (2015). Prognostic value of epidermal growth factor receptor amplification and EGFRvIII in glioblastoma: meta-analysis. Acta Neurol. Scand. 132, 310–322. doi: 10.1111/ane.12401
Chen, Y., and Xu, R. (2016). Drug repurposing for glioblastoma based on molecular subtypes. J. Biomed. Inform. 64, 131–138. doi: 10.1016/j.jbi.2016.09.019
Christensen, B. C., Smith, A. A., Zheng, S., Koestler, D. C., Houseman, E. A., Marsit, C. J., et al. (2011). DNA methylation, isocitrate dehydrogenase mutation, and survival in glioma. J. Natl. Cancer Instit. 103, 143–153. doi: 10.1093/jnci/djq497
Cioca, A., Gheorghe-Emilian, O., Gisca, M., Morosanu, C., Marin, I., and Florian, S. (2016). Expression of EGFR in paired new and recurrent glioblastomas. Asian Pac. J. Cancer Prevent. APJCP 17, 4205–4208.
Collisson, E. A., Sadanandam, A., Olson, P., Gibb, W. J., Truitt, M., Gu, S., et al. (2011). Subtypes of pancreatic ductal adenocarcinoma and their differing responses to therapy. Nat. Med. 17, 500–503. doi: 10.1038/nm.2344
Colman, H., Zhang, L., Sulman, E. P., Mcdonald, J. M., Shooshtari, N. L., Rivera, A. L., et al. (2010). A multigene predictor of outcome in glioblastoma. Neuro Oncol. 12, 49–57. doi: 10.1093/neuonc/nop007
Crommentuijn, M. H. W., Kantar, R., Noske, D. P., Vandertop, W. P., Badr, C. E., Würdinger, T., et al. (2016). Systemically administered AAV9-sTRAIL combats invasive glioblastoma in a patient-derived orthotopic xenograft model. Mol. Ther Oncolyt. 3:16017. doi: 10.1038/mto.2016.17
Derisi, J. L., Penland, L., Brown, P. O., Bittner, M. L., Meltzer, P. S., Ray, M., et al. (1996). Use of a cDNA microarray to analyse gene expression patterns in human cancer. Nat. Genet. 14, 457–460. doi: 10.1038/ng1296-457
Dimitrov, L., Hong, C. S., Yang, C., Zhuang, Z., and Heiss, J. D. (2015). New developments in the pathogenesis and therapeutic targeting of the IDH1 mutation in glioma. Int. J. Med. Sci. 12, 201–213. doi: 10.7150/ijms.11047
Dudoit, S., Fridlyand, J., and Speed, T. P. (2002). Comparison of discrimination methods for the classification of tumors using gene expression data. J. Am. Stat. Assoc. 97, 77–87. doi: 10.1198/016214502753479248
Endersby, R., and Baker, S. J. (2008). PTEN signaling in brain: neuropathology and tumorigenesis PTEN signaling in brain: neuropathology and tumorigenesis. Oncogene 27, 5416–5430. doi: 10.1038/onc.2008.239
Etcheverry, A., Aubry, M., de Tayrac, M., Vauleon, E., Boniface, R., Guenot, F., et al. (2010). DNA methylation in glioblastoma: impact on gene expression and clinical outcome. BMC Genomics 11:701. doi: 10.1186/1471-2164-11-701
Felsberg, J., Hentschel, B., Kaulich, K., Gramatzki, D., Zacher, A., Malzkorn, B., et al. (2017). Epidermal growth factor receptor variant III (EGFRvIII) positivity in EGFR-amplified glioblastomas: prognostic role and comparison between primary and recurrent tumors. Clin. Cancer Res. 23, 6846–6855. doi: 10.1158/1078-0432.CCR-17-0890
Gan, H. K., Kaye, A. H., and Luwor, R. B. (2009). The EGFRvIII variant in glioblastoma multiforme. J. Clin. Neurosci. 16, 748–754. doi: 10.1016/j.jocn.2008.12.005
Ghosh, D., Nandi, S., and Bhattacharjee, S. (2018). Combination therapy to checkmate glioblastoma: clinical challenges and advances. Clin. Transl. Med. 7:33. doi: 10.1186/s40169-018-0211-8
Gorlia, T., Stupp, R., Brandes, A. A., Rampling, R., Fumoleau, P., Dittrich, C., et al. (2012). New prognostic factors and calculators for outcome prediction in patients with recurrent glioblastoma: a pooled analysis of EORTC Brain Tumour Group phase I and II clinical trials. Eur. J. Cancer 48, 1176–1184. doi: 10.1016/j.ejca.2012.02.004
Gray, S. J., Matagne, V., Bachaboina, L., Yadav, S., Ojeda, S. R., and Samulski, R. J. (2011). Preclinical differences of intravascular AAV9 delivery to neurons and glia: a comparative study of adult mice and nonhuman primates. Mol. Ther. 19, 1058–1069. doi: 10.1038/mt.2011.72
Gustafsson, J. R., Katsioudi, G., Degn, M., Ejlerskov, P., Issazadeh-Navikas, S., and Kornum, B. R. (2018). DNMT1 regulates expression of MHC class I in post-mitotic neurons. Mol. Brain 11:36. doi: 10.1186/s13041-018-0380-9
Hatanpaa, K. J., Burma, S., Zhao, D., and Habib, A. A. (2010). Epidermal growth factor receptor in glioma: signal transduction, neuropathology, imaging, and radioresistance. Neoplasia 12, 675–684. doi: 10.1593/neo.10688
Hegi, M. E., Diserens, A., Gorlia, T., Hamou, M., De Tribolet, N., Weller, M., et al. (2005). MGMT gene silencing and benefit from temozolomide in glioblastoma. N. Engl. J. Med. 352, 997–1003.
Heimberger, A. B. (2005). Prognostic effect of epidermal growth factor receptor and EGFRvIII in glioblastoma multiforme patients. Clin. Cancer Res. 11, 1462–1466. doi: 10.1158/1078-0432.ccr-04-1737
Hendel, A., Bak, R. O., Clark, J. T., Kennedy, A. B., Ryan, D. E., Roy, S., et al. (2015). Chemically modified guide RNAs enhance CRISPR-Cas genome editing in human primary cells. Nat. Biotechnol. 33, 985–989. doi: 10.1038/nbt.3290
Herrlinger, U., Schaefer, N., Steinbach, J. P., Weyerbrock, A., Hau, P., Goldbrunner, R., et al. (2014). Survival and quality of life in the randomized, multicenter GLARIUS trial investigating bevacizumab/irinotecan versus standard temozolomide in newly diagnosed, MGMT-non-methylated glioblastoma patients. J. Clin. Oncol. 32:2042. doi: 10.1200/jco.2014.32.15_suppl.2042
Homma, T., Fukushima, T., Vaccarella, S., Yonekawa, Y., Patre, P. L. D., Franceschi, S., et al. (2006). Correlation among pathology, genotype, and patient outcomes in glioblastoma. J. Neuropathol. Exp. Neurol. 65, 846–854. doi: 10.1097/01.jnen.0000235118.75182.94
Hu, Z., Fan, C., Oh, D. S., Marron, J. S., He, X., Qaqish, B. F., et al. (2006). The molecular portraits of breast tumors are conserved across microarray platforms. BMC Genomics 7:96. doi: 10.1186/1471-2164-7-96
Ichimura, K., Pearson, D. M., Kocialkowski, S., Backlund, L. M., Chan, R., Jones, D. T., et al. (2009). IDH1 mutations are present in the majority of common adult gliomas but rare in primary glioblastomas. Neuro Oncol. 11, 341–347. doi: 10.1215/15228517-2009-025
Irizarry, R. A., Bolstad, B. M., Collin, F., Cope, L., Hobbs, B., and Speed, T. P. (2003). Summaries of affymetrix geneChip probe level data. Nucleic Acids Res. 31:e15.
Jacob, F., Salinas, R. D., Zhang, D. Y., Nguyen, P. T. T., Schnoll, J. G., Wong, S. Z. H., et al. (2020). A patient-derived glioblastoma organoid model and biobank recapitulates inter- and intra-tumoral heterogeneity. Cell 180, 188.e22–204.e22. doi: 10.1016/j.cell.2019.11.036
Johannessen, L. E., Brandal, P., Myklebust, T. O. R. Å, Heim, S., Micci, F., and Panagopoulos, I. (2018). MGMT gene promoter methylation status – assessment of two pyrosequencing kits and three methylation-specific PCR methods for their predictive capacity in glioblastomas. Cancer Genomics Proteomics 15, 437–446. doi: 10.21873/cgp.20102
Kim, D., Lee, W., and Park, J. (2018). Promoter methylation of Wrap53α, an antisense transcript of p53, is associated with the poor prognosis of patients with non-small cell lung cancer. Oncol. Lett. 16, 5823–5828. doi: 10.3892/ol.2018.9404
Kim, J., Lee, I.-H., Cho Hee, J., Park, C.-K., Jung, Y.-S., Kim, Y., et al. (2015). Spatiotemporal evolution of the primary glioblastoma genome. Cancer Cell 28, 318–328. doi: 10.1016/j.ccell.2015.07.013
Kim, S., Dougherty, E. R., Shmulevich, I., Hess, K. R., Hamilton, S. R., Trent, J. M., et al. (2002). Identification of combination gene sets for glioma classification. Mol. Cancer Ther. 1, 1229–1236.
Kim, Y. K., and Kim, W.-J. (2008). Epigenetic markers as promising prognosticators for bladder cancer. Int. J. Urol. 16, 17–22. doi: 10.1111/j.1442-2042.2008.02143.x
Kleinschmidtdemasters, B. K., Aisner, D. L., Birks, D. K., and Foreman, N. K. (2013). Epithelioid GBMs show a high percentage of BRAF V600E mutation. Am. J. Surg. Pathol. 37, 685–698. doi: 10.1097/pas.0b013e31827f9c5e
Kleinschmidt-DeMasters, B. K., Alassiri, A. H., Birks, D. K., Newell, K. L., Moore, W., and Lillehei, K. O. (2010). Epithelioid versus rhabdoid glioblastomas are distinguished by monosomy 22 and immunohistochemical expression of INI-1 but not Claudin 6. Am. J. Surg. Pathol. 34, 341–354. doi: 10.1097/PAS.0b013e3181ce107b
Kloosterhof, N. K., de Rooi, J. J., Kros, M., Eilers, P. H. C., Smitt, P. A. E. S., van den Bent, M. J., et al. (2013). Molecular subtypes of glioma identified by genome-wide methylation profiling. Genes Chromosomes Cancer 52, 665–674. doi: 10.1002/gcc.22062
Koch, A., Joosten, S. C., Feng, Z., de Ruijter, T. C., Draht, M. X., Melotte, V., et al. (2018). Author correction: analysis of DNA methylation in cancer: location revisited. Nat. Rev. Clin. Oncol. 15:467. doi: 10.1038/s41571-018-0028-9
Lee, E., Yong, R. L., Paddison, P., and Zhu, J. (2018). Comparison of glioblastoma (GBM) molecular classification methods. Semin. Cancer Biol. 53, 201–211. doi: 10.1016/j.semcancer.2018.07.006
Li, R., Chen, X., You, Y., Wang, X., Liu, Y., Hu, Q., et al. (2015). Comprehensive portrait of recurrent glioblastoma multiforme in molecular and clinical characteristics. Oncotarget 6, 30968–30974. doi: 10.18632/oncotarget.5038
Li, Y., Gong, Y., He, S., Li, X., and Zhou, L. (2019). Downregulation of CLDN7 due to promoter hypermethylation is associated with human clear cell renal cell carcinoma progression and poor prognosis. Eur. Urol. Suppl. 18:e91. doi: 10.1016/s1569-9056(19)30069-7
Li, Z., Takenobu, H., Setyawati, A. N., Akita, N., Haruta, M., Satoh, S., et al. (2018). EZH2 regulates neuroblastoma cell differentiation via NTRK1 promoter epigenetic modifications. Oncogene 37, 2714–2727. doi: 10.1038/s41388-018-0133-3
Linnekamp, J. F., Wang, X., Medema, J. P., and Vermeulen, L. (2015). Colorectal cancer heterogeneity and targeted therapy: a case for molecular disease subtypes. Cancer Res. 75, 245–249. doi: 10.1158/0008-5472.can-14-2240
Liu, X., Chen, X., Shi, L., Shan, Q., Cao, Q., Yue, C., et al. (2019). The third-generation EGFR inhibitor AZD9291 overcomes primary resistance by continuously blocking ERK signaling in glioblastoma. J. Exp. Clin. Cancer Res. 38:219. doi: 10.1186/s13046-019-1235-7
Lofton-Day, C., and Lesche, R. (2003). DNA methylation markers in patients with gastrointestinal cancers. Digest. Dis. 21, 299–308. doi: 10.1159/000075352
Louis, D. N., Perry, A., Burger, P., Ellison, D. W., Reifenberger, G., von Deimling, A., et al. (2014). International society of neuropathology–haarlem consensus guidelines for nervous system tumor classification and grading. Brain Pathol. 24, 429–435. doi: 10.1111/bpa.12171
Louis, D. N., Perry, A., Reifenberger, G., Von Deimling, A., Figarellabranger, D., Cavenee, W. K., et al. (2016). The 2016 World Health Organization classification of tumors of the central nervous system: a summary. Acta Neuropathol. 131, 803–820. doi: 10.1007/s00401-016-1545-1
Lowder, L., Hauenstein, J., Woods, A., Chen, H. R., Rupji, M., Kowalski, J., et al. (2019). Gliosarcoma: distinct molecular pathways and genomic alterations identified by DNA copy number/SNP microarray analysis. J. Neuro Oncol. 143, 381–392. doi: 10.1007/s11060-019-03184-1
Lu, C., Ward, P. S., Kapoor, G. S., Rohle, D., Turcan, S., Abdelwahab, O., et al. (2012). IDH mutation impairs histone demethylation and results in a block to cell differentiation. Nature 483, 474–478. doi: 10.1038/nature10860
Lukas, R. V., and Mrugala, M. M. (2016). Pivotal therapeutic trials for infiltrating gliomas and how they affect clinical practice. Neuro Oncol. Pract. 4, 209–219. doi: 10.1093/nop/npw016
Ma, H., Zhao, C., Zhao, Z., Hu, L., Ye, F., Wang, H., et al. (2019). Specific glioblastoma multiforme prognostic-subtype distinctions based on DNA methylation patterns. Cancer Gene Therap 1–13. doi: 10.1038/s41417-019-0142-6
Marko, N. F., and Weil, R. J. (2013). The molecular biology of WHO Grade II gliomas. Neurosurg. Focus 34:E1. doi: 10.1155/2011/372509
Marusyk, A., and Polyak, K. (2010). Tumor heterogeneity: causes and consequences. Biochim. Biophys. Acta 1805, 105–117. doi: 10.1016/j.bbcan.2009.11.002
Mclendon, R. E., Friedman, A. H., Bigner, D. D., Van Meir, E. G., Brat, D. J., Mastrogianakis, G. M., et al. (2008). Comprehensive genomic characterization defines human glioblastoma genes and core pathways. Nature 455, 1061–1068. doi: 10.1038/nature07385
Meyer-Puttlitz, B., Hayashi, Y., Waha, A., Rollbrocker, B., Boström, J., Wiestler, O. D., et al. (1997). Molecular genetic analysis of giant cell glioblastomas. Am. J. Pathol. 151, 853–857.
Mondesir, J., Willekens, C., Touat, M., and de Botton, S. (2016). IDH1 and IDH2 mutations as novel therapeutic targets: current perspectives. J. Blood Med. 7, 171–180. doi: 10.2147/JBM.S70716
Mosrati, M., Malmström, A., Lysiak, M., Krysztofiak, A., Hallbeck, M., Milos, P., et al. (2015). TERT promoter mutations and polymorphisms as prognostic factors in primary glioblastoma. Oncotarget 6, 16663–16673. doi: 10.18632/oncotarget.4389
Muhammad, J. S., Khan, M. R., and Ghias, K. (2018). DNA methylation as an epigenetic regulator of gallbladder cancer: an overview. Int. J. Surg. 53, 178–183. doi: 10.1016/j.ijsu.2018.03.053
Neilsen, B. K., Sleightholm, R., McComb, R., Ramkissoon, S. H., Ross, J. S., Corona, R. J., et al. (2019). Comprehensive genetic alteration profiling in primary and recurrent glioblastoma. J. Neurooncol. 142, 111–118. doi: 10.1007/s11060-018-03070-2
Nobusawa, S., Watanabe, T., Kleihues, P., and Ohgaki, H. (2009). IDH1 mutations as molecular signature and predictive factor of secondary glioblastomas. Clin. Cancer Res. 15, 6002–6007. doi: 10.1158/1078-0432.CCR-09-0715
Noushmehr, H., Weisenberger, D. J., Diefes, K., Phillips, H. S., Pujara, K., Berman, B. P., et al. (2010). Identification of a CpG island methylator phenotype that defines a distinct subgroup of glioma. Cancer Cell 17, 510–522.
Nutt, C. L., Mani, D. R., Betensky, R. A., Tamayo, P., Cairncross, J. G., Ladd, C., et al. (2003). Gene expression-based classification of malignant gliomas correlates better with survival than histological classification. Cancer Res. 63, 1602–1607.
Ohgaki, H., and Kleihues, P. (2007). Genetic pathways to primary and secondary glioblastoma. Am. J. Pathol. 170, 1445–1453. doi: 10.2353/ajpath.2007.070011
Ohgaki, H., and Kleihues, P. (2013). The definition of primary and secondary glioblastoma. Clin. Cancer Res. 19, 764–772. doi: 10.1158/1078-0432.CCR-12-3002
Olmez, I., Love, S., Xiao, A., Manigat, L., Randolph, P., McKenna, B. D., et al. (2017). Targeting the mesenchymal subtype in glioblastoma and other cancers via inhibition of diacylglycerol kinase alpha. Neuro Oncol. 20, 192–202. doi: 10.1093/neuonc/nox119
Paolillo, M., Boselli, C., and Schinelli, S. (2018). Glioblastoma under siege: an overview of current therapeutic strategies. Brain Sci. 8:15. doi: 10.3390/brainsci8010015
Park, A. K., Kim, P., Ballester, L. Y., Esquenazi, Y., and Zhao, Z. (2019). Subtype-specific signaling pathways and genomic aberrations associated with prognosis of glioblastoma. Neuro Oncol. 21, 59–70. doi: 10.1093/neuonc/noy120
Park, J., Shim, J. K., Yoon, S. J., Kim, S. H., Chang, J. H., and Kang, S. G. (2019). Transcriptome profiling-based identification of prognostic subtypes and multi-omics signatures of glioblastoma. Sci. Rep. 9:10555. doi: 10.1038/s41598-019-47066-y
Parsons, D. W., Jones, S., Zhang, X., Lin, J., Leary, R. J., Angenendt, P., et al. (2008). An integrated genomic analysis of human glioblastoma multiforme. Science 321, 1807–1812.
Paul, Y., Mondal, B., Patil, V., and Somasundaram, K. (2017). DNA methylation signatures for 2016 WHO classification subtypes of diffuse gliomas. Clin. Epigenet. 9:32. doi: 10.1186/s13148-017-0331-9
Pérez, R. F., Tejedor, J. R., Bayón, G. F., Fernández, A. F., and Fraga, M. F. (2018). Distinct chromatin signatures of DNA hypomethylation in aging and cancer. Aging Cell 17:e12744. doi: 10.1111/acel.12744
Phillips, H. S., Kharbanda, S., Chen, R., Forrest, W. F., Soriano, R. H., Wu, T. D., et al. (2006). Molecular subclasses of high-grade glioma predict prognosis, delineate a pattern of disease progression, and resemble stages in neurogenesis. Cancer Cell 9, 157–173. doi: 10.1016/j.ccr.2006.02.019
Rickman, D. S., Bobek, M. P., Misek, D. E., Kuick, R., Blaivas, M., Kurnit, D. M., et al. (2001). Distinctive molecular profiles of high-grade and low-grade gliomas based on oligonucleotide microarray analysis. Cancer Res. 61, 6885–6891.
Rohle, D., Popovicimuller, J., Palaskas, N., Turcan, S., Grommes, C., Campos, C., et al. (2013). An inhibitor of mutant IDH1 delays growth and promotes differentiation of glioma cells. Science 340, 626–630. doi: 10.1126/science.1236062
Romani, M., Pistillo, M. P., and Banelli, B. (2018). Epigenetic targeting of glioblastoma. Front. Oncol. 8:448. doi: 10.3389/fonc.2018.00448
Sallinen, S.-L., Sallinen, P. K., Haapasalo, H. K., Helin, H. J., Helén, P. T., Schraml, P., et al. (2000). Identification of differentially expressed genes in human gliomas by DNA microarray and tissue chip techniques. Cancer Res. 60, 6617–6622.
Sandmann, T., Bourgon, R., Garcia, J., Li, C., Cloughesy, T., Chinot, O. L., et al. (2015). Patients With proneural glioblastoma may derive overall survival benefit from the addition of bevacizumab to first-line radiotherapy and temozolomide: retrospective analysis of the AVAglio trial. J. Clin. Oncol. 33, 2735–2744. doi: 10.1200/JCO.2015.61.5005
Sang, Y., Hou, Y., Cheng, R., Zheng, L., Alvarez, A., Hu, B., et al. (2019). Targeting PDGFRα-activated glioblastoma through specific inhibition of SHP-2–mediated signaling. Neuro Oncol. 21, 1423–1435. doi: 10.1093/neuonc/noz107
Schafer, N., Gielen, G. H., Rauschenbach, L., Kebir, S., Till, A., Reinartz, R., et al. (2019). Longitudinal heterogeneity in glioblastoma: moving targets in recurrent versus primary tumors. J. Transl. Med. 17:96. doi: 10.1186/s12967-019-1846-y
Schena, M., Shalon, D., Davis, R. W., and Brown, P. O. (1995). Quantitative monitoring of gene expression patterns with a complementary DNA microarray. Science 270, 467–470. doi: 10.1126/science.270.5235.467
Schumacher, T., Bunse, L., Pusch, S., Sahm, F., Wiestler, B., Quandt, J., et al. (2014). A vaccine targeting mutant IDH1 induces antitumour immunity. Nature 512, 324–327. doi: 10.1038/nature13387
Sehgal, A. (1998). Molecular changes during the genesis of human gliomas. Semin. Surg. Oncol. 14, 3–12. doi: 10.1002/(sici)1098-2388(199801/02)14:1<3::aid-ssu2<3.0.co;2-f
Sharma, A., Bendre, A., Mondal, A., Muzumdar, D., Goel, N., and Shiras, A. (2017). Angiogenic gene signature derived from subtype specific cell models segregate proneural and mesenchymal glioblastoma. Front. Oncol. 7:146. doi: 10.3389/fonc.2017.00146
Shergalis, A., Bankhead, A. III, Luesakul, U., Muangsin, N., and Neamati, N. (2018). Current challenges and opportunities in treating glioblastoma. Pharmacol. Rev. 70, 412–445. doi: 10.1124/pr.117.014944
Shinojima, N., Tada, K., Shiraishi, S., Kamiryo, T., Kochi, M., Nakamura, H., et al. (2003). Prognostic value of epidermal growth factor receptor in patients with glioblastoma multiforme. Cancer Res. 63, 6962–6970.
Skiriute, D., Vaitkiene, P., Saferis, V., Asmoniene, V., Skauminas, K., Deltuva, V. P., et al. (2012). MGMT, GATA6, CD81, DR4, and CASP8 gene promoter methylation in glioblastoma. BMC Cancer 12:218. doi: 10.1186/1471-2407-12-218
Songtao, Q., Lei, Y., Si, G., Yanqing, D., Huixia, H., Xuelin, Z., et al. (2012). IDH mutations predict longer survival and response to temozolomide in secondary glioblastoma. Cancer Sci. 103, 269–273. doi: 10.1111/j.1349-7006.2011.02134.x
Spiegl-Kreinecker, S., Lötsch, D., Ghanim, B., Pirker, C., Mohr, T., Laaber, M., et al. (2015). Prognostic quality of activating TERT promoter mutations in glioblastoma: interaction with the rs2853669 polymorphism and patient age at diagnosis. Neuro Oncol. 17, 1231–1240. doi: 10.1093/neuonc/nov010
Srividya, M. R., Thota, B., Arivazhagan, A., Thennarasu, K., Balasubramaniam, A., Chandramouli, B. A., et al. (2010). Age-dependent prognostic effects of EGFR/p53 alterations in glioblastoma: study on a prospective cohort of 140 uniformly treated adult patients. J. Clin. Pathol. 63, 687–691. doi: 10.1136/jcp.2009.074898
Stancheva, G., Goranova, T., Laleva, M., Kamenova, M., Mitkova, A., Velinov, N., et al. (2014). IDH1/IDH2 but Not TP53 mutations predict prognosis in bulgarian glioblastoma patients. BioMed. Res. Int. 2014:654727.
Stupp, R., Mason, W. P., Den Bent, M. J. V., Weller, M., Fisher, B., Taphoorn, M. J. B., et al. (2005). Radiotherapy plus concomitant and adjuvant temozolomide for glioblastoma. N. Engl. J. Med. 352, 987–996.
Szerlip, N., Pedraza, A., Chakravarty, D., Azim, M., Mcguire, J., Fang, Y., et al. (2012). Intratumoral heterogeneity of receptor tyrosine kinases EGFR and PDGFRA amplification in glioblastoma defines subpopulations with distinct growth factor response. Proc. Natl. Acad. Sci. U. S. A. 109, 3041–3046. doi: 10.1073/pnas.1114033109
Szopa, W., Burley, T. A., Kramer-Marek, G., and Kaspera, W. (2017). Diagnostic and therapeutic biomarkers in glioblastoma: current status and future perspectives. Biomed. Res. Int. 2017:8013575. doi: 10.1155/2017/8013575
Taylor, J., and Schiff, D. (2015). Treatment considerations for MGMT-unmethylated glioblastoma. Curr. Neurol. Neurosci. Rep. 15:507. doi: 10.1007/s11910-014-0507-z
Teo, W. Y., Sekar, K., Seshachalam, P., Shen, J., Chow, W. Y., Lau, C. C., et al. (2019). Relevance of a TCGA-derived glioblastoma subtype gene-classifier among patient populations. Sci. Rep. 9:7442. doi: 10.1038/s41598-019-43173-y
van den Bent, M. J., Gao, Y., Kerkhof, M., Kros, J. M., Gorlia, T., van Zwieten, K., et al. (2015). Changes in the EGFR amplification and EGFRvIII expression between paired primary and recurrent glioblastomas. Neuro Oncol. 17, 935–941. doi: 10.1093/neuonc/nov013
Velculescu, V. E., Zhang, L., Vogelstein, B., and Kinzler, K. W. (1995). Serial analysis of gene expression. Science 270, 484–487.
Verhaak, R. G., Hoadley, K. A., Purdom, E., Wang, V., Qi, Y., Wilkerson, M. D., et al. (2010). Integrated genomic analysis identifies clinically relevant subtypes of glioblastoma characterized by abnormalities in PDGFRA, IDH1, EGFR, and NF1. Cancer Cell 17, 98–110. doi: 10.1016/j.ccr.2009.12.020
Wang, J., Cazzato, E., Ladewig, E., Frattini, V., Rosenbloom, D. I. S., Zairis, S., et al. (2016). Clonal evolution of glioblastoma under therapy. Nat. Genet. 48, 768–776.
Wang, Q., Hu, B., Hu, X., Kim, H., Squatrito, M., Scarpace, L., et al. (2017). Tumor evolution of glioma-intrinsic gene expression subtypes associates with immunological changes in the microenvironment. Cancer Cell 32:152. doi: 10.1016/j.ccell.2017.06.003
Watanabe, T., Nobusawa, S., Kleihues, P., and Ohgaki, H. (2009). IDH1 mutations are early events in the development of astrocytomas and oligodendrogliomas. Am. J. Pathol. 174, 1149–1153. doi: 10.2353/ajpath.2009.080958
Weller, M., Cloughesy, T., Perry, J. R., and Wick, W. (2012). Standards of care for treatment of recurrent glioblastoma—are we there yet? Neuro Oncol. 15, 4–27. doi: 10.1093/neuonc/nos273
Wu, X., Rauch, T. A., Zhong, X., Bennett, W. P., Latif, F., Krex, D., et al. (2010). CpG island hypermethylation in human astrocytomas. Cancer Res. 70, 2718–2727. doi: 10.1158/0008-5472.can-09-3631
Yamashita, K., Hosoda, K., Nishizawa, N., Katoh, H., and Watanabe, M. (2018). Epigenetic biomarkers of promoter DNA methylation in the new era of cancer treatment. Cancer Sci. 109, 3695–3706. doi: 10.1111/cas.13812
Yan, H., Parsons, D. W., Jin, G., Mclendon, R. E., Rasheed, B. K. A., Yuan, W., et al. (2009). IDH1 and IDH2 mutations in gliomas. N. Engl. J. Med. 360, 765–773.
Yang, P., Cai, J., Yan, W., Zhang, W., Wang, Y., Chen, B., et al. (2016). Classification based on mutations of TERT promoter and IDH characterizes subtypes in grade II/III gliomas. Neuro Oncol. 18, 1099–1108. doi: 10.1093/neuonc/now021
Yin, A. A., Lu, N., Etcheverry, A., Aubry, M., Barnholtz-Sloan, J., Zhang, L. H., et al. (2018). A novel prognostic six-CpG signature in glioblastomas. CNS Neurosci. Ther. 24, 167–177. doi: 10.1111/cns.12786
Yu, Z.-Q., Zhang, B.-L., Ren, Q.-X., Wang, J.-C., Yu, R.-T., Qu, D.-W., et al. (2013). Changes in transcriptional factor binding capacity resulting from promoter region methylation induce aberrantly high GDNF expression in human glioma. Mol. Neurobiol. 48, 571–580. doi: 10.1007/s12035-013-8443-5
Yuan, Y., Qi, C., Maling, G., Xiang, W., Yanhui, L., Ruofei, L., et al. (2016). TERT mutation in glioma: frequency, prognosis and risk. J. Clin. Neurosci. 26, 57–62. doi: 10.1016/j.jocn.2015.05.066
Zhao, Z., Zhang, K., Wang, Q., Li, G., Zeng, F., Zhang, Y., et al. (2020). Chinese Glioma Genome Atlas (CGGA): a comprehensive resource with functional genomic data for chinese glioma patients. bioRxiv doi: 10.1101/2020.01.20.911982
Keywords: glioblastoma, molecular heterogeneity, transcription-based subtype, genetic alteration-based subtype, DNA methylation-based subtype, subtype-specific therapy
Citation: Zhang P, Xia Q, Liu L, Li S and Dong L (2020) Current Opinion on Molecular Characterization for GBM Classification in Guiding Clinical Diagnosis, Prognosis, and Therapy. Front. Mol. Biosci. 7:562798. doi: 10.3389/fmolb.2020.562798
Received: 18 May 2020; Accepted: 18 August 2020;
Published: 08 September 2020.
Edited by:
Cheng Zhang, Royal Institute of Technology, SwedenReviewed by:
Sahin Hanalioglu, Hacettepe University, TurkeyJorge Lima, University of Porto, Portugal
Copyright © 2020 Zhang, Xia, Liu, Li and Dong. This is an open-access article distributed under the terms of the Creative Commons Attribution License (CC BY). The use, distribution or reproduction in other forums is permitted, provided the original author(s) and the copyright owner(s) are credited and that the original publication in this journal is cited, in accordance with accepted academic practice. No use, distribution or reproduction is permitted which does not comply with these terms.
*Correspondence: Lei Dong, ldong@bit.edu.cn
†These authors share first authorship