- 1Cancer Research Program, Rajiv Gandhi Centre for Biotechnology, Thiruvananthapuram, India
- 2Manipal Academy of Higher Education (MAHE), Manipal, India
- 3Centre for BioSystems Science and Engineering, Indian Institute of Science, Bengaluru, India
Intratumoral heterogeneity is a major ongoing challenge in the effective therapeutic targeting of cancer. Accumulating evidence suggests that a fraction of cells within a tumor termed Cancer Stem Cells (CSCs) are primarily responsible for this diversity resulting in therapeutic resistance and metastasis. Adding to this complexity, recent studies have shown that there can be different subpopulations of CSCs with varying biochemical and biophysical traits resulting in varied dissemination and drug-resistance potential. Moreover, cancer cells can exhibit a high level of plasticity or the ability to dynamically switch between CSC and non-CSC states or among different subsets of CSCs. In addition, CSCs also display extensive metabolic plasticity. The molecular mechanisms underlying these different interconnected axes of plasticity has been under extensive investigation and the trans-differentiation process of Epithelial to Mesenchymal transition (EMT) has been identified as a major contributing factor. Besides genetic and epigenetic factors, CSC plasticity is also shaped by non-cell-autonomous effects such as the tumor microenvironment (TME). In this review, we discuss the latest developments in decoding mechanisms and implications of CSC plasticity in tumor progression at biochemical and biophysical levels, and the latest in silico approaches being taken for characterizing cancer cell plasticity. These efforts can help improve existing therapeutic approaches by taking into consideration the contribution of cellular plasticity/heterogeneity in enabling drug resistance.
Introduction
Heterogeneity in cancer biology has long been recognized and exploited in the clinical management of the disease (Rich, 2016). Intertumoral heterogeneity within breast cancer patients, for example, exhibiting different molecular subtypes based on immunohistochemical markers like Estrogen Receptor (ER) or Her2, has been the basis of successful targeted therapeutic approaches (Turashvili and Brogi, 2017; Januskeviciene and Petrikaite, 2019). The inbuilt cellular variation within a tumor has been shown to be an important driver for the emergence of therapy resistant clones which ultimately lead to recurrence and spread of the cancer cells resulting in patient mortality (Somasundaram et al., 2012; Dagogo-Jack and Shaw, 2018; Akgul et al., 2019).
The development of intratumoral diversity in tumor cells has been widely attributed to two contrasting processes (Shackleton et al., 2009; Prasetyanti and Medema, 2017). The clonal evolution theory takes into account the intrinsic differences between all cells based on genetic and epigenetic programs as well as the influence of the tumor microenvironment (TME). The fitter clones are selected for and contribute to the diversity of the tumor cell population (McGranahan and Swanton, 2017). The second model – Cancer Stem Cell (CSC) model – proposes that there are a subset of cells (termed CSCs) which are predisposed to drive the tumor progression, metastatic and therapeutic resistance of the entire tumor. In this hierarchical model, CSCs can differentiate into less self-renewing populations of non-CSCs which form the bulk of the tumor, in an analogous fashion to stem cell development (Vermeulen et al., 2012). More recently, the ability of cells to switch states via different programs such as Epithelial-Mesenchymal Transition (EMT) has given rise to the concept that non-CSCs can also convert to being a CSC. Thus, CSCs need not be always a priori defined; rather stemness can be thought of as a cell state can that be reversibly gained or lost. In other words, cellular plasticity can allow CSCs and non-CSCs to switch among one another (Chaffer et al., 2011; Marjanovic et al., 2013; Gupta et al., 2019). Moreover, different subsets of CSCs can lie on various points on the epithelial-mesenchymal axis and can possibly interconvert (Liu et al., 2014; Bocci et al., 2018; Bocci et al., 2019). Therefore, clonal evolution and CSC models are not necessarily mutually exclusive and the plasticity model ushers in more complexity to the manner in which heterogeneous cell populations can possibly arise within a tumor (Cabrera et al., 2015; Figure 1).
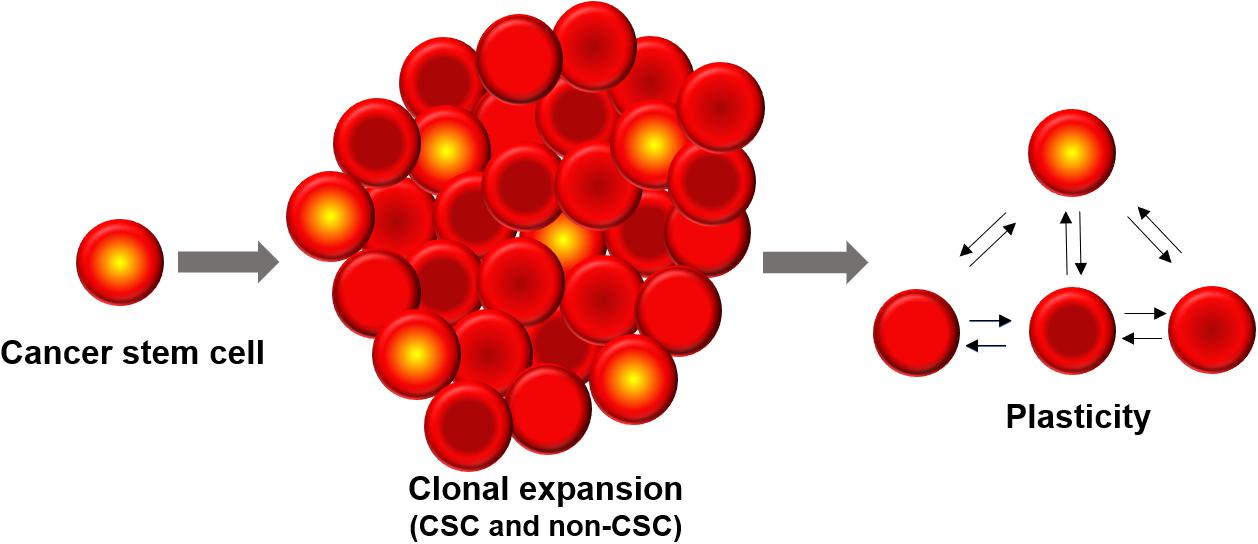
Figure 1. Cancer stem cells (CSCs) constitute a minor sub-population of tumor mass. Phenotypic plasticity can enable CSCs and non-CSCs to interconvert among one another, depending on cell-intrinsic (e.g., epigenetic) and cell-extrinsic (e.g., tumor microenvironment) features.
A direct consequence of interconverting or plastic cellular populations in a tumor is the rise of drug resistant and/or metastatic cells which are ultimately responsible for the mortality associated with cancer (Biddle et al., 2016; Doherty et al., 2016). The need of the hour is hence to understand the molecular underpinnings for CSC plasticity and to decode the impact of bidirectional nature of CSC plasticity on the clinical management of the disease.
CSC Heterogeneity and Plasticity in Tumor Progression
The concept that CSCs are dynamic populations and can undergo spontaneous state transitions has been strengthened by various studies (Chaffer et al., 2011, 2013; Gupta et al., 2011). In the study done by Chaffer et al. (2011), using basal-like breast cancer cells, non-stem cells were shown to spontaneously switch to stem-like cells in vitro and in vivo; this plasticity was later found to be regulated by ZEB1 (Chaffer et al., 2013) – a key regulator of EMT (Jia et al., 2017). CSC heterogeneity and plasticity has been observed in different cancers. Just like their non-cancerous counterparts, identification of CSCs has been mainly based on the expression of cell surface markers (Chen W. et al., 2016). However, even within a single tumor type, different markers can identify distinct CSCs which are phenotypically distinct and could vary from patient to patient depending on the genetic make-up of the tumor (Tang, 2012). For instance, in glioblastoma, multiple markers like CD133, CD44, A2B5, SSEA have been utilized for identifying the stem cell populations (Singh et al., 2004; Ogden et al., 2008; Son et al., 2009). However, the use of CD133 marker is controversial as CD133– cells have also been shown to form tumors in glioma and CD133+ cells could be derived from CD133– cells in vivo, implying the underlying plasticity (Wang et al., 2008). A recent study by Dirkse et al. (2019) found that in glioblastoma, the cell-membrane associated CSC markers such as CD133, A2B5, SSEA, and CD15 does not represent a clonal entity but a plastic state which can be adapted by most of the cells in response to varying conditions in the microenvironment. They also proposed that the enhanced tumorigenic potential of CSC-like state is a result of faster adaptation of the cells to the microenvironment.
The evidence for plasticity of CSC states comes from melanoma as well. A slow cycling population of melanoma CSC-like cells were identified using H3K4 demethylase JARID1B as a biomarker (Roesch et al., 2010). Intriguingly, the expression of this marker was dynamically regulated and JARID1B-negative cells could re-express the marker, thus indicating the dynamic nature of the stemness trait. Another seminal study done on melanoma supports the phenotypic plasticity model of CSCs. In this study, phenotypically distinct melanoma cells were shown to undergo reversible phenotypic changes in vivo and recapitulate the original tumor (Quintana et al., 2010). In breast cancer, different subsets of CSCs were identified based on ALDH1, CD44, and CD24; and the two subpopulations (epithelial-like ALDH1+, mesenchymal like CD44+/CD24–) were shown to be capable of inter converting among themselves as well as give rise to non-CSCs (Liu et al., 2014). Moreover, in breast cancer, CSCs and non-CSCs were shown to exhibit dynamic equilibrium maintained by cytokine-mediated crosstalk among these distinct populations (Iliopoulos et al., 2011). These results suggest that at least in some cancers, phenotypic plasticity is reversible and does not necessarily depend on genetic alterations (Jolly et al., 2018a).
Another compelling evidence for CSC plasticity in tumor progression comes from studies on colorectal cancer. LGR5, a Wnt target gene, is used as a marker for colorectal CSCs. Kobayashi et al. (2012) has established human colon cancer cell lines that express LGR5 and possess CSC properties. However, treatment with an anticancer drug resulted in the conversion of the LGR5+ cells into LGR5– cells; the absence of drug drove the transition back from LGR5– to LGR5+ cells, suggesting the inherent plasticity. Both of these cell types could reconstitute the tumor in vivo. Consistently, targeted ablation of Lgr5+ CSCs did not lead to tumor regression in vivo as the Lgr5– cells could give rise to Lgr5+ cells and sustained the tumor growth. But interestingly, the Lgr5– cells could not form liver metastases (de Sousa e Melo et al., 2017), suggesting that the contribution of CSCs in primary tumor formation and that in metastatic settings may be different. However, contrary to these results, a very recent study has shown that majority of the colorectal cancer metastases were seeded by Lgr5– cells. Interestingly, these cells could re-establish cellular hierarchy by giving rise to Lgr5+ cells and thereby reinforcing the concept of plasticity (Fumagalli et al., 2020). Therefore, the ability of CSCs and non-CSCs to switch among one another seems crucial both for the primary tumor and metastatic growth. More recently, some markers for metastatic CSCs have been identified across cancers (Celia-Terrassa and Jolly, 2019).
CSC plasticity has also been observed alongside vasculogenic mimicry (VM) – a hallmark process of cancer cell plasticity in which cancer cells transdifferentiate and acquire endothelial cell like characteristics (Fernandez-Cortes et al., 2019). In triple negative breast cancer, a CD133+ cell population with CSC-like traits was found to show the ability to form tube-like structures (Liu et al., 2013). In renal cell carcinoma, using immunohistochemistry analysis of patient samples, the expression of stem cell like markers CD133 and CD44 was found to correlate with VM and high CSC marker expression and VM correlated with poor survival (Zhang et al., 2013). Thus, CSCs may not only interconvert among their sub-groups, but also give rise to different kinds of non-CSC differentiated cells.
Mechanisms Controlling CSC Plasticity
CSC plasticity is controlled by both cell-intrinsic and cell-extrinsic factors (Poli et al., 2018). Several studies have implied the importance of key transcription factors such as OCT3/4, SOX2, NANOG and KLF4 in modulating the generation of CSCs and regulation of cellular plasticity (Gu et al., 2007; Ben-Porath et al., 2008; Liu et al., 2013; Eun et al., 2017). For example, the introduction of OCT3/4, NANOG and KLF4 retrovirally into human colon cancer cells resulted in enhanced CSC properties and the xenografts of these cells actually resembled the original human tumor tissue (Oshima et al., 2014). Similarly, in glioblastoma, Suva et al. (2014) identified a core set of neurodevelopmental transcription factors (POU3F2, SOX2, SALL2, and OLIG2) that were sufficient to reprogram differentiated glioblastoma cells to CSCs. Tumor suppressor transcription factors like p53, pTEN has also been associated with CSC plasticity (Cabrera et al., 2015; da Silva-Diz et al., 2018; Santoro et al., 2019). Loss of p53 lead to increased expression of Nestin and enable the dedifferentiation of hepatocytes and thereby contributes to cellular plasticity in liver carcinogenesis (Tschaharganeh et al., 2014). Similarly, combined loss of p53/pTEN in clonal prostate epithelial cells caused transformation of multipotent progenitors and lead to epithelial to mesenchymal transition (EMT) (Martin et al., 2011). Moreover, genetic mutations of oncogene like KRAS and tumor suppressor like APC is also linked to the generation of stem-like cells (Easwaran et al., 2014).
Many studies have pointed out various mechanisms of epigenetic regulation such as bivalent chromatin state, DNA methylation, histone modifications in mediating CSC plasticity (Poli et al., 2018). For example, in basal like breast cancer cells, Chaffer and colleagues observed that ZEB1 promoter of non-CSCs is maintained in a bivalent configuration and in response to TGFβ, the chromatin switches to an active state leading to the transcription of ZEB1, consequently converting non-CSCs to CSCs (Chaffer et al., 2013). On the other hand, loss of function of HOXC8, a homeobox gene, in non-tumorigenic mammary epithelial cells due to its promoter DNA hypermethylation has been shown to be associated with CSC pool expansion, increased self-renewal and a transformed phenotype (Shah et al., 2017). A histone modifier, enhancer of zeste homolog 2 (EZH2) is a core member of polycomb repressor complex 2 (PRC2) and mediates transcriptional repression of target genes via the trimethylation of lysine 27 of histone 3 (H3K27me3) (Gan et al., 2018). EZH2 is upregulated in many cancers and its enhanced expression is associated with invasion, migration and stemness (Yamaguchi and Hung, 2014). In breast cancer, overexpression of EZH2 can increase mammosphere formation and self-renewal ability in CSCs (Chang et al., 2011; Wen et al., 2017). In glioblastoma, loss of H3K27me3 can lead to aberrant activation of Wnt pathway which is required for tumorigenicity and CSC maintenance (Rheinbay et al., 2013). On the other hand, in pediatric glioblastomas, the mutations in histone variants H3.1 and H3.3 results in reduced activity of EZH2 and consequently reprograms toward a stem cell-like state (Lewis et al., 2013). These observations suggest that CSCs are capable of exploiting the reversible nature of epigenetic modifications to achieve their plastic nature (Wainwright and Scaffidi, 2017). However, this reversibility also putatively offers an attractive opportunity that needs to be harnessed for therapeutic targeting.
CSC Plasticity and EMT
EMT is a reversible, dynamic process which is critical during embryonic development and also aberrantly activated during various pathological processes like wound healing, fibrosis and cancer progression (Kalluri and Weinberg, 2009; Jolly et al., 2018d). EMT is characterized by the loss of apico-basal polarity, rearrangements in the cytoskeleton and the acquisition of mesenchymal gene expression signature (Kalluri and Weinberg, 2009). The activation of EMT program is associated with the acquisition of stem like characteristics and has been implicated in different cancers (Mani et al., 2008; Shibue and Weinberg, 2017; Singla et al., 2018; Dongre and Weinberg, 2019). Initial reports suggested that activation of an EMT program endowed cells with traits similar to CSCs, such as enhanced colony formation in vitro and enhanced tumorigenesis in vivo (Shibue and Weinberg, 2017). Recent studies have, however, presented a more nuanced understanding of the interconnection between EMT and CSCs. Cells that undergo a more extreme version of EMT can lose the stemness gained during the initiation of EMT; thus, cells in a hybrid epithelial/mesenchymal phenotype are much more likely to be stem-like as compared to those on either end of the spectrum – pure epithelial or pure mesenchymal (Bierie et al., 2017; Jolly et al., 2018b). A recent study by Kroger et al. has found that the acquisition of a hybrid phenotype is a critical for the maintenance of tumorigenicity of basal breast cancer cells. Based on CD104/CD44 cell surface antigen expression and by regulating the expression of transcription factors like Zeb1 and Snail, they isolated highly tumorigenic cell population residing stably in a hybrid E/M state. This hybrid E/M cell population showed enhanced stemness which was mediated by increased expression of Snail and Wnt signaling pathway (Kroger et al., 2019). Another interesting study by Pastushenko et al. (2018) looked at the spectrum of EMT states that exist in a tumor rather than the binary fixed state that was accepted for long. The hybrid E/M tumor cells were associated with differences in their transcriptional and epigenetic programs, metastatic potential and also the location within a tumor (Pastushenko et al., 2018). It would be interesting to further understand whether these different hybrid states also respond differently to cues like chemotherapeutic treatment leading to resistance and ultimately relapse in cancer patients.
The Effect of the Tumor Microenvironment on CSC Plasticity
Besides juxtacrine crosstalk among cancer cells and stromal cells, there are factors secreted by the different cell types that form complex interacting networks in a TME (Swartz et al., 2012; Quail and Joyce, 2013; Peltanova et al., 2019). Accumulating evidence suggests that such crosstalk can modulate stem-like behavior and phenotypic plasticity of cancer cells (Pattabiraman and Weinberg, 2014; Cabrera et al., 2015; Prasetyanti and Medema, 2017). Cancer associated fibroblasts (CAFs) are a major component of the TME and play a pivotal role in various aspects of tumor progression (Kwa et al., 2019). CAFs were found to modulate the CSC plasticity in hepatocellular carcinoma through c-Met/FRA1/HEY1 signaling (Lau et al., 2016), in pancreatic adenocarcinoma through FAK signaling (Begum et al., 2019) and in lung cancer by IGF-II/IGF1R signaling pathway (Chen et al., 2014). In a recent study, the extent of intracellular Notch1 signaling in mesenchymal stem cell-derived dermal fibroblasts was found to determine the ability of these cells to regulate melanoma aggressiveness, stemness and phenotypic plasticity (Du et al., 2019). Another key component in TME is the immune system which plays a crucial role in regulating CSC plasticity. In response to chemotherapy, macrophages can secrete factor like Oncostatin-M (OSM), an IL-6 family cytokine which in turn can activate the dedifferentiation of triple negative breast cancer cells into aggressive stem cells (Doherty et al., 2019) and this activation could be mediated through co-operative STAT3/SMAD3 signaling (Junk et al., 2017). OSM can also be secreted by cancer associated adipocytes which can also promote stemness (Wolfson et al., 2015). Similarly, a crosstalk between macrophages of various polarizations (M1, M2) can alter the composition of tumor cells in terms of epithelial vs. mesenchymal populations, thus modulating stemness (Li et al., 2019).
The physical and chemical composition of the microenvironment such as acidic pH, low oxygen and nutrient availability, rigidity and porosity of the ECM can also play an important role in regulating the cancer stem cell behavior (Hjelmeland et al., 2011; Nallanthighal et al., 2019; Prager et al., 2019). A classic example would be hypoxia which is a hallmark of tumor progression in solid tumors and is associated with metastasis, therapeutic resistance and poor survival (Lequeux et al., 2019). A hypoxic microenvironment is known to regulate various aspects of malignant progression including cellular plasticity. In Glioblastoma, hypoxia was found to promote self-renewal in non-stem cells by upregulating important factors like OCT4, NANOG, and cMYC (Heddleston et al., 2009). Also, the hypoxic microenvironment can select the fate of breast cancer stem cells (CSCs) in vivo (Kim et al., 2018). Using flow cytometry, hypoxic and non-hypoxic breast cancer cells were isolated from hypoxia sensing xenografts of MDA-MB-231 and MCF7 breast cancer cell lines. Hypoxic tumor cells showed enhanced CSC characteristics compared to non-hypoxic cells which is attributed to the PI3K/AKT signaling. Interestingly, this differential cell fate was observed only in tumor cells isolated from hypoxic TME in vivo and not in tumor cells treated by hypoxia in vitro alone (Kim et al., 2018).
These studies underscore the importance of the microenvironment in sculpting intra-tumoral heterogeneity and CSC plasticity and highlight the need to better understand the tumor-microenvironment crosstalk for the development of effective therapeutic strategies (Figure 2). However, it is still controversial whether the CSC heterogeneity arises as a consequence of the microenvironment exerted selection pressure or whether plasticity is an intrinsic, default feature of the cancer cells that enable to adapt to varying cues from the microenvironment (Poli et al., 2018; Dirkse et al., 2019). Recent evidence from the study on glioblastoma suggests that intrinsic plasticity of tumor cells enables them to stochastically transition between different states defined by distinct expression of cancer stem cell markers and adapt to the microenvironment. Although all cell subpopulations are capable of phenotypic adjustment, they vary in their speed of adaptation (Dirkse et al., 2019).
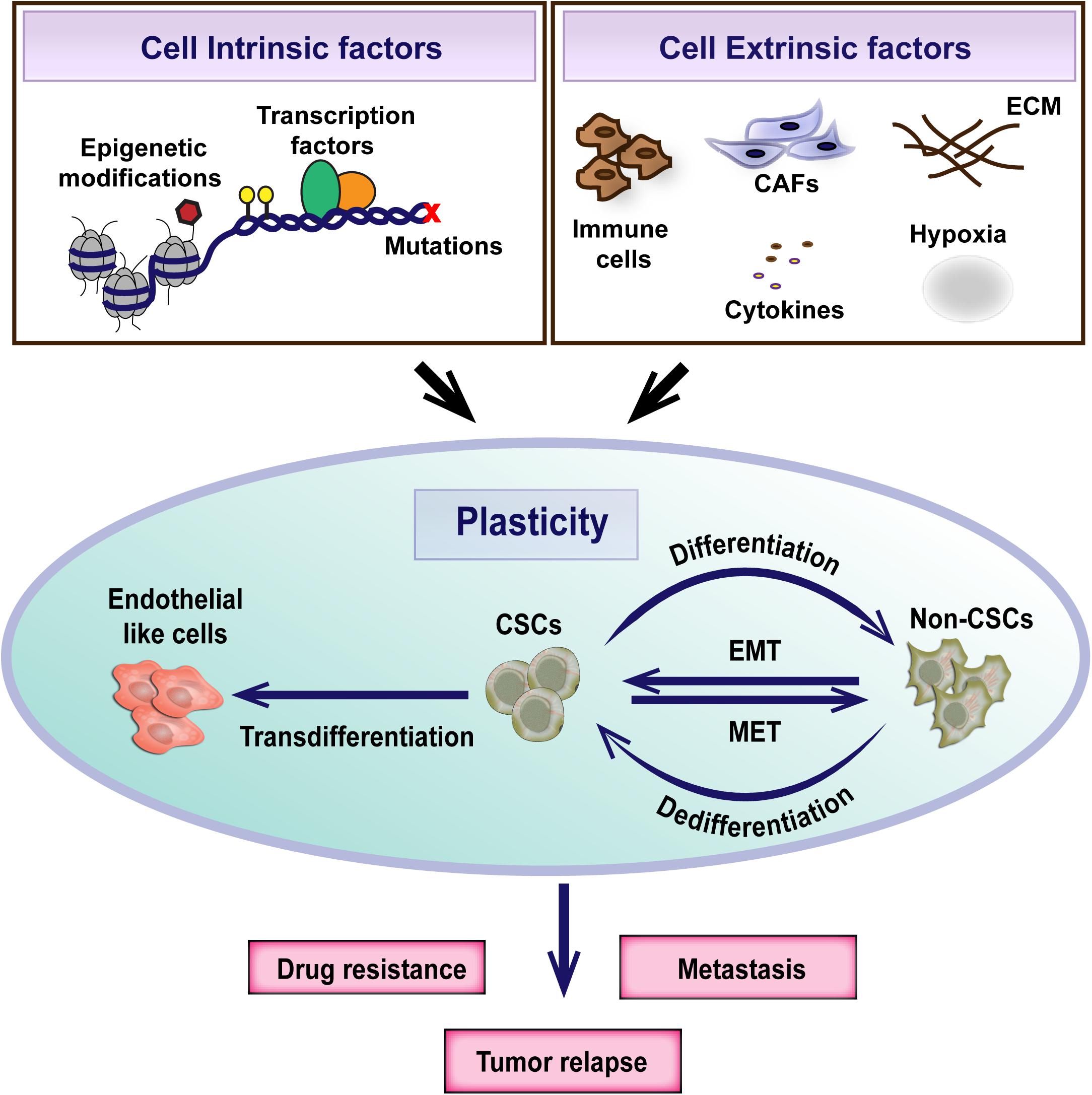
Figure 2. Cancer stem cell plasticity is the ability to dynamically switch between CSC and non-CSC states. It is a complex process regulated by both cell intrinsic and extrinsic factors. Plasticity plays an important role in the evolution of therapeutic resistance, tumor relapse and metastasis.
Biochemical Characterization of Cancer Stem Cells and Their Subsets
CSC plasticity can be instigated by various components in the microenvironment such as the secretion of cytokines and chemokines, communication with different stromal cell types and extracellular matrix and hypoxia (Agliano et al., 2017). Consequent activation of transcription factors and/or epigenetic modifications have been shown to mediate this interconversion (Cabrera et al., 2015). To understand the biology of CSC plasticity and the mechanisms underlying their functional phenotype with the aim of developing efficient treatment strategies, an essential requirement is the characterization and methods to selectively isolate the plastic CSC population from bulk tumors (Figure 3).
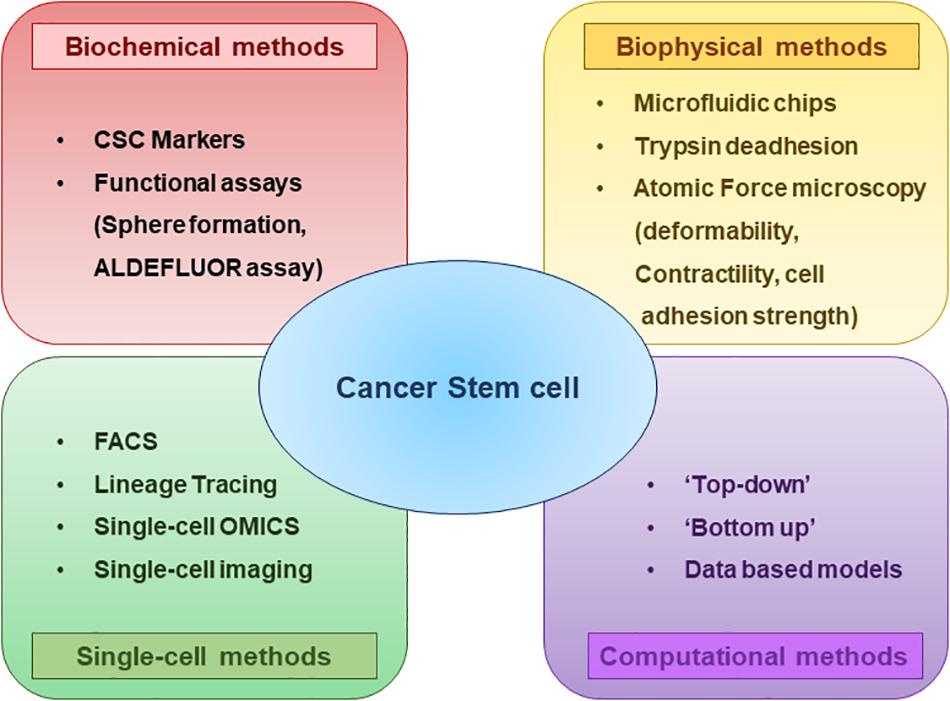
Figure 3. Methods to characterize CSCs and their subsets at a glance. Biochemical and biophysical characteristics of the CSCs can be strikingly different and this diversity can be understood by using multiple assays. Analyzing the properties of CSCs at Single-cell resolution enables to better comprehend the CSC plasticity. Different computational and mathematical models are also being used which helps to gain insights regarding the CSC diversity and plasticity.
One of the serious and longstanding challenges in studying CSCs is the determination of appropriate methodology for the isolation and characterization of CSCs (Agliano et al., 2017). One of the most widely applied method to identify CSCs is to sort the cells based on the expression of cell surface markers such as CD44, CD133, CD24, CD26, EPCAM, CD166 (Jaggupilli and Elkord, 2012; Chen et al., 2013) or based on enzymatic activity of intracellular proteins like ALDH1 (Pattabiraman and Weinberg, 2014; Table 1). However, even these markers are not universally expressed on all CSCs, limiting their use in few cancers (Jaggupilli and Elkord, 2012). To overcome this limitation, more than one markers are used together in several cancers (Agliano et al., 2017). Although multiple markers have been described, the lack of reliable and accurate markers remains to be a stumbling block in the identification of CSCs. Moreover, recent single cell transcriptome analyses revealed that many CSC markers could be co-expressed by a single cell at the same time (Patel et al., 2014; Eun et al., 2017; Table 2) and the expression of CSC markers could vary in vivo as a consequence of plasticity and adaptation to the microenvironment (Dirkse et al., 2019). These observations clearly highlight the heterogeneity of CSCs and inefficiency of the markers currently in use in distinguishing CSCs and non-CSCs. Therefore, combining marker-based isolation strategies with functional assays such as in vitro clonogenic and in vivo limiting dilution xenotransplantation assays are of paramount importance to validate the stemness trait of the cells (Dirkse et al., 2019; Prager et al., 2019; Table 2).
Biophysical Characterization of CSCs and Their Subsets
The properties of stem cells such as self-renewal and multipotency can be governed by intra-cellular and extracellular components constituting the stem cell niche (Morrison and Spradling, 2008). Similar to stem cells, properties of CSCs can be regulated by the TME to enhance their metastatic and tumor initiation capabilities (Aponte and Caicedo, 2017). CSCs, on the other hand, can also remodel ECM more strongly as compared to bulk cancer population (Srinivasan et al., 2017); thereby setting a complex feedback loop among the CSC and its niche. Biochemical constituents of such loops have been well-characterized earlier (Korkaya et al., 2011). Recent evidence suggests how biophysical cues such as matrix stiffness, cell contractility and cell-matrix adhesion strengths can regulate the tumor-initiating properties of CSCs. For instance, blocking ROCK (Rho-associated protein kinase) can inhibit cell contractility and invasion potential of breast CSCs (Srinivasan et al., 2017). At a biophysical level, TME is often characterized by increased stiffness due to ECM remodeling, increased compressive stress due to confined growth, enhanced interstitial pressure and an increased interstitial fluid flow (Zanotelli et al., 2018). Extrinsic mechanical forces exerted by ECM constituents can trigger biochemical changes inside cells such as cytoskeleton rearrangement, and changes in gene expression, protein-protein interactions and enzyme modifications, thus converging on various mechano-transduction and mechano-chemical axes (Ogden et al., 2008; Broders-Bondon et al., 2018; Roy Choudhury et al., 2019).
Cancer cells display variations in response to extrinsic biomechanical stimuli, leading to heterogeneity in their biophysical properties, which influences the overall nature of cells such as stemness and differentiation. Recent studies have shown that mechano-transduction cues greatly influences the generation and maintenance of CSCs and eventually metastasis (Chen and Kumar, 2017). In addition, mechanical properties such as deformability and adhesiveness are different for CSCs as compared to the bulk tumor population (Saliba et al., 2010; Zhang et al., 2012; Babahosseini et al., 2014; Chen J. et al., 2016). These advances have enabled attempts to isolate and identify CSCs based on biophysical marker using engineering techniques such as microfluidic devices (Saliba et al., 2010; Gossett et al., 2012; Zhang et al., 2012; Liu et al., 2015; Chen J. et al., 2016) in a label-free manner.
For instance, CSCs were enriched based on their adhesive traits using a microfluid chip having micro-channels coated with basement membrane extract. The cells entered into the chip driven by hydrodynamic forces. While highly adhesive cells were captured in micro-channels, less adhesive cells were collected from the outlet, which were shown to be enriched in CSCs, had greater motility and were resistant to chemotherapeutic drugs (Zhang et al., 2015). This study emphasizes the interconnections between EMT, stemness, and drug resistance (Jolly et al., 2019), and the use of microfluidics in investigating these associations (Nath et al., 2019). Similarly, another microfluidic device fitted with microbarriers was used to isolate cancer cells based on their deformability in vitro. The more deformable flexible phenotype was associated with expression of many genes involved in motility, metastasis and greater mammosphere formation efficiency (Zhang et al., 2012). Consistently, in vivo, deformability has been shown to be crucial for efficient extravasation of tumor-repopulating cells during metastasis as seen in zebrafish models (Chen J. et al., 2016; Figure 3).
Similar to biochemical heterogeneity observed within CSCs (Bocci et al., 2019; Moreira et al., 2019), CSCs can be biophysically strikingly different too (Table 2). In a recent study, Chen et al. (2019) showed the association of biophysical properties of CSCs and their ability to invade, migrate, and initiate tumors, using the SUM149 inflammatory breast cancer (IBC) stem cells. In this study, the authors sorted SUM149 CSCs based on the expression of aldehyde dehydrogenase enzyme (ALDH) and assayed biophysical properties of ALDH+ and ALDH– cells in terms of deformability, adhesion strength and contractility. ALDH+ cells displayed greater deformability, lower adhesion strength and reduced contractility relative to ALDH– cells, and resulted into enhanced functional phenotypes in vitro and greater tumor development in vivo. In addition, the authors isolated IBC cells based on their adhesive property using a microfluidic device and showed that the less adhesive cell population was ALDH– enriched, displayed enhanced in vitro invasion and migration as well as increased in vivo tumor development. Further, exogenous alteration of cell stiffness also resulted in changes in metastatic potential of these cells with less stiff cells showing greater invasion and migration (Chen et al., 2019). The results observed in this study corroborated well with earlier studies showing that cancer cells with greater deformability, lower adhesion strength and lower contractile force show enhanced metastatic potential (Swaminathan et al., 2011; Kraning-Rush et al., 2012; Byun et al., 2013; Sun et al., 2016; Bongiorno et al., 2018).
Therefore, with increasingly detailed characterization of biomechanical properties of various subpopulations of cancer cells and ECM, a “mechanosome” or “matrisome” signature may be helpful in identifying and isolating the most aggressive cancer cell subpopulations (Roy Choudhury et al., 2019).
Single-Cell Methods to Identify CSCs and Their Subsets
Much of our current understanding about CSCs comes from studies performed on bulk cancer cell populations. However, bulk analysis masks the underlying intra-tumor heterogeneity and does not inform much about rare cell subpopulations within the tumor (Navin, 2015; Bhatia et al., 2019). Therefore, it becomes extremely critical to study the cancer cells at a single-cell resolution to better comprehend the CSC heterogeneity and plasticity (Etzrodt et al., 2014). Flow cytometry and Fluorescence-Activated Cell Sorting (FACS) is a widely used method for the isolation and characterization of single CSCs (Greve et al., 2012; Etzrodt et al., 2014). Also, lineage tracing can be used to follow the fate of individual cells (Figure 3; McKenna and Gagnon, 2019).
A recent study integrated FACS analysis of CSC markers with functional assays such as proliferation, self-renewal and multipotency tests, and observed that glioblastoma stem cell heterogeneity results from tumor plasticity which is determined by the microenvironment cues (Dirkse et al., 2019). Lineage tracing methods have also been utilized by researchers to decipher the properties of CSCs and has huge potential in understanding the transition of cellular states. Using intra-vital in vivo lineage tracing method in a genetic mouse model of breast cancer, Zomer et al. (2013) demonstrated the existence of CSCs in unperturbed mammary tumor. They also found that CSC state is plastic and can be activated, lost or deactivated. In another study, lineage tracing and transcriptional analysis of Notch1 expressing cells of intestinal tumors has led to the identification of a previously uncharacterized and undifferentiated stem cell population that contribute to tumor progression and heterogeneity (Mourao et al., 2019).
The advent of single-cell omics approaches has revolutionized our knowledge on CSC biology. Single cell genomic and transcriptomic analyses have provided invaluable insights regarding intra-tumor heterogeneity and clonal evolution in different cancers (Patel et al., 2014; Eirew et al., 2015; Hou et al., 2016; Puram et al., 2017). In addition to discerning the dynamics of clonal evolution, single cell omics methods have also enabled the identification of transitioning CSCs and their contribution to drug resistance (Puram et al., 2018; Sharma et al., 2018). Considering the rarity of CSCs, combining single cell transcriptomics with enrichment strategies such as flow cytometry or sphere assays are capable of drastically improving the characterization of CSC (Akrap et al., 2016; Jonasson et al., 2019). Single cell multi-omics approaches involving obtaining information from multiple components within a single cell is also gaining interest, because it facilitates the assessment of genotype and phenotype relationship in regulating the individual cell states (Macaulay et al., 2017; Liu et al., 2019).
Nonetheless, most of the single-cell omics analyses does not preserve spatial information as it requires the cells to be isolated from their microenvironment (Yuan et al., 2017). Another limitation is that a snap-shot analysis is inadequate to evaluate the dynamic nature of cellular processes (Skylaki et al., 2016). Transcriptomic profiling of cells using fluorescence in situ hybridization or sequencing will enable the decoding of the spatial regulation of cellular heterogeneity at single-cell resolution (Lee et al., 2014; Chen et al., 2015; Suva and Tirosh, 2019). Single-cell RNA sequencing data can be mapped to spatial transcriptomic data using advanced computational methods (Satija et al., 2015; Edsgard et al., 2018). Using a newly developed high-throughput automated single cell image analysis (HASCIA), the spatio-temporal factors regulating glioblastoma stem cell state transitions has been recently investigated (Chumakova et al., 2019). Integrating the transcriptomic and spatial data can significantly improve the interpretation of the CSC plasticity (Satija et al., 2015; Yuan et al., 2017). A recent work coupling large scale single cell-resolution 3D imaging strategy, lineage tracing and RNA sequencing in pTEN/Trp53 deficient mice models, observed extensive molecular heterogeneity and clonal plasticity within tumors and found that EMT is not a rare event within the tumors (Rios et al., 2019). Live single-cell imaging techniques are also being developed which overcomes the limitation of static snap-shot analyses in studying the temporal regulation of cellular state changes (Fumagalli et al., 2019). The number of genes analyzed by such studies are much less than snap-shot studies and the lack of specialized tools and computational methods for handling the large amount of data generated through such studies is a major challenge (Skylaki et al., 2016).
With emerging evidence about the potential of single-cell analysis in understanding the biology of CSCs, development of newer tools and analysis methods and integrative approach are required for better comprehending the cell state transitions and improved therapeutic strategies.
Computational Methods to Identify CSCs
With the deluge of preclinical and clinical data being generated at a high-dimensional level, computational approaches to extract meaningful information and generate testable hypotheses are becoming more common (Suhail et al., 2019). Various “top–down” and “bottom-up” computational methods provide a framework to unravel novel insights into various aspects of the dynamics of cancer progression such as role of intra-tumoral heterogeneity, dynamics of EMT, CSCs and its role in metastases, evolutionary dynamics of cancer initiation and progression, prediction of treatment response and therapy resistance (Figure 3). While “top–down” methods use high-dimensional data and apply an inferential metric to identify patterns through machine learning and/or network reconstruction, the “bottom-up” approaches aim to elucidate the emergent dynamics of a phenomenon based on its mechanism-based description through mathematical modeling. Both approaches can be synergistically used to predict and/or interpret cellular behavior (Altrock et al., 2015; Jolly et al., 2017). Mechanism-based, i.e., “bottom-up,” mathematical models have been useful in understanding the dynamics of complex regulatory networks that modulate cancer stem cell behavior such as stem cell state transitions and dedifferentiation (Sehl and Wicha, 2018). Recent studies using mathematical models have predicted that cells in one or more hybrid E/M phenotypes are associated more with stemness as compared to cells in purely mesenchymal or purely epithelial (Jolly et al., 2014). These predictions have since been validated in vitro and in vivo (Grosse-Wilde et al., 2015; Bierie et al., 2017; Pastushenko et al., 2018; Kroger et al., 2019) and has been supported by clinical data (Jolly et al., 2019). How are the pathways of EMT and stemness interconnected with each other? These questions can be addressed by investigating in silico the coupling between core regulatory circuits of EMT, CSCs and other connected signaling pathways such as Notch signaling; this model predicted that altering the coupling strength between EMT and CSC networks and/or modulating Notch signaling can change the position of “stemness window” on the “EMT axis,” thus generating various subsets of CSCs in terms of EMT phenotypes (Bocci et al., 2018). Such CSC heterogeneity has been extensively seen across cancers (Liu et al., 2014; Giraddi et al., 2018; Zheng et al., 2018). A common unifying principle that has emerged upon investigating the regulatory networks underlying EMT, CSCs and related traits such as drug resistance has been the role of interconnected feedback loops in enabling multiple phenotypes (i.e., heterogeneity) and the ability to switch among them (i.e., plasticity) (Mooney et al., 2017; Tripathi et al., 2017; Jolly et al., 2018c). Phenotypic plasticity can abet the generation and maintenance of phenotypic heterogeneity (Celia-Terrassa et al., 2018; Hari et al., 2019); thus, breaking these feedback loops can be thought of as a novel potential therapeutic strategy to restrict phenotypic plasticity and/or heterogeneity (Celia-Terrassa et al., 2018; Hari et al., 2019).
“Data-based” models have also been valuable in decoding CSC signatures. A stemness index was derived using one-class logistic regression and observed to be higher in the metastatic breast cancer cells compared with primary tumors (Malta et al., 2018), suggesting the possibility of a signature specific to metastatic CSCs. Similar logistic regression models have been used to quantify the extent of EMT (George et al., 2017) that has revealed the heterogeneity of EMT phenotypes in various CSCs and their subsets (Bocci et al., 2018), hence strengthening the insights from “mechanism-based” or “bottom-up” models.
Another set of questions where mathematical models have been useful is estimating the fraction of CSCs in a tumor. Many studies have used population-level models to understand the difference in growth kinetics of CSCs and non-CSCs, and used that to offer a potential mechanistic underpinning of “tumor growth paradox,” i.e., accelerated tumor growth with increased cell death (Hillen et al., 2013). Typically, CSC represent a minor cell population within a tumor, major population being the non-CSCs which compete with the CSCs for space and resources. While induction of cell death results into death of bulk of non-CSCs which facilitates increase in CSC division, ultimately resulting into expansion of CSC population and increase in tumor progression (Hillen et al., 2013). In contrary, another study showed that the CSC population within a tumor is homeostatically maintained such that reducing CSC population below a threshold triggers extensive phenotypic switching of non-CSC to CSC population (Sellerio et al., 2015). Thus, while the dynamics and mechanisms of CSC generation, plasticity and maintenance remain to be comprehensively understood (Enderling, 2015), integrating these different modeling approaches with one another and with experimental and clinical data shall contribute to revealing this complex behavior at an intracellular and at a population level. Such an improved dynamic understanding can help identify optimal treatment strategies to reduce tumor burden, such as a combination of radiation and differentiation therapies (Bachman and Hillen, 2013), or a sequential treatment of drugs to tackle the de novo generation of CSCs (Gupta et al., 2011) and their functional attributes (Goldman et al., 2015).
Spatial Organization of CSCs
Spatial heterogeneity is a fundamental feature of TME that contains diverse cell types (Yuan, 2016; Yuan et al., 2017). One canonical representation of spatial heterogeneity is the co-occurrence of vascular and hypoxic regions, as observed in solid tumors (Alfarouk et al., 2013). This heterogeneity can alter cellular phenotypes, for instance, glioblastoma cells in hypoxic regions have been shown to over-express epidermal growth factor receptor (EGFR) while those over-expressing platelet-derived growth factor receptor (PDGFRA) were enriched in vascular regions (Little et al., 2012). Similarly, hypoxic TME induced by anti-angiogenic agents can increase breast CSCs (Conley et al., 2012). Hypoxia can be acute, chronic or cyclic (intermittent), each with its unique effects on tumor progression (Saxena and Jolly, 2019). Thus, spatial heterogeneity of TME can give rise to differential spatial organization of cancer sub-populations within a tumor.
Varying levels of nuclear β-catenin expression was observed in different sub-populations of well-differentiated colorectal cancer, suggested to be regulated by TME (Brabletz et al., 2001). Cells in the invasive front of the primary tumor as well as metastases expressed high levels of nuclear β-catenin, and lacked the expression of membranous E-cadherin, indicative of an EMT. On the other hand, centrally located cells in the primary tumor and metastases showed cytoplasmic β-catenin and membranous E-cadherin expression, perhaps due to a mesenchymal-epithelial transition (MET) (Brabletz et al., 2001). Spatial heterogeneity with respect to EMT has been reported since in primary tumor (Jung et al., 2001; Schmalhofer et al., 2009; Bronsert et al., 2014; Liu et al., 2014; Grigore et al., 2016). Consistently, CD24–CD44+ mesenchymal breast CSCs were found in the invasive edge of the tumor, while the more epithelial or hybrid E/M CSCs, identified by ALDH1+, were localized in the interior regions of the tumor (Liu et al., 2014). Put together, these observations beg the question of what mechanisms might underlie such patterning. Recent efforts including a mathematical modeling analysis revealed that in the presence of a gradient of TGF-β (EMT inducing) signal, cell-cell communication among tumor cells mediated via Notch-Jagged signaling can recapitulate the experimentally observed spatial organization of CSCs sub-populations with varying EMT phenotypes (Bocci et al., 2019). In vitro knock down of JAG1 in SUM149 human breast cancer cells significantly reduced their tumor organoid formation, confirming the role of Notch-Jagged signaling in tumor progression (Bocci et al., 2019). Future studies can focus on gaining a understanding of other interconnected aspects of heterogeneity in TME.
Spatial heterogeneity of tumors can be used as a predictor of cancer prognosis and treatment response across different cancer types (Yuan, 2016). For example, colorectal cancer patients with high density of tumor infiltrating lymphocytes responded to anti-cancer therapy (Gong et al., 2018). Spatial heterogeneity can also significantly impact the time to occurrence in cancer cells exposed to continuous as well as adaptive therapies (Gallaher et al., 2018). Thus, the spatiotemporal dynamics of phenotypic changes induced by TME can be pivotal in aggravating aspects of tumor progression.
Metabolic Plasticity of CSCs
Ever since Otto Warburg’s observation that cancer cells, unlike normal cells preferentially rely on glycolysis for energy production even under aerobic conditions, referred to as aerobic glycolysis or Warburg effect (Warburg et al., 1927), metabolic adaptation of cancer has been under extensive investigation (Liberti and Locasale, 2016; Peiris-Pages et al., 2016). To meet the varying metabolic demands set by the microenvironment during the course of tumor progression and to survive, cancer cells must dynamically rewire their metabolic phenotype and has been recognized as a hallmark feature of cancer (Hanahan and Weinberg, 2011). This ability to adapt is critical for tumor growth, metastasis and response to therapy (Dupuy et al., 2015; Luo and Wicha, 2015). Metabolic plasticity, also contributes to the tumor heterogeneity (Lehuede et al., 2016; De Francesco et al., 2018). Emerging evidence suggest that CSCs display extensive metabolic plasticity and can reprogramme their metabolism in a context dependent manner in response to the cues from the microenvironment (Albini et al., 2015; Sancho et al., 2016). Also, non-CSCs can acquire a stem-like character through changing the metabolic phenotype. This acquired stemness by altering metabolism is an emerging hallmark of cancer, known as “metabostemness” and contributes to the CSC plasticity (Menendez and Alarcon, 2014; De Francesco et al., 2018). There is no consensus regarding the metabolic phenotype of CSCs (Peiris-Pages et al., 2016). Although many reports show that CSCs are more glycolytic than the other differentiated cells, several other conflicting reports suggest that they prefer mitochondrial oxidative phosphorylation (OXPHOS). For example, CSC from the breast, lung, and colon cancers have been found to show higher glycolytic activity than the other cells (Ciavardelli et al., 2014; Liu et al., 2014). On the other hand, CSCs from pancreatic cancer and leukemia depend on OXPHOS for energy production (Skrtic et al., 2011; Lonardo et al., 2013; Sancho et al., 2015). Also, studies have shown that CSCs could switch from one metabolic state to another in response to various challenges microenvironment like pH, hypoxia and nutritional status (Singh et al., 2004; Sancho et al., 2015; Chae and Kim, 2018). This apparent discrepancy in these observations could be due to multiple reasons. One reason could be the intrinsic differences between the metabolic phenotype of different cancer types from which they were derived (Kim and DeBerardinis, 2019). Another major reason could be the metabolic plasticity of CSCs, existence of multiple CSC subsets, each of which may have an increased proclivity to exhibit a particular metabolic phenotype, reminiscent of observations for varying degrees of stemness observed along the spectrum of EMT states (Gammon et al., 2013; Peiris-Pages et al., 2016; Bocci et al., 2018). The inherent limitation associated with the use of distinct markers/techniques for the isolation and characterization of CSCs in these studies can be another confounding factor (Sancho et al., 2016; Snyder et al., 2018; Martano et al., 2019). Also, the preference of metabolic states relies heavily on the microenvironmental conditions, differences in the nutrient availability at the primary and metastatic sites (Dupuy et al., 2015; Pascual et al., 2018) and the co-operation (Pavlides et al., 2009) or competition with the stromal cells (Chang et al., 2015). The cause for the differences in the metabolic states are also often attributed to alterations in the mitochondrial mass, mitochondrial dynamics, biogenesis and mitochondrial DNA content (Guha et al., 2014; De Luca et al., 2015; Farnie et al., 2015; De Francesco et al., 2018). Metabolic reprogramming can be orchestrated by a wide array of signaling pathways (Ito and Suda, 2014; Papa et al., 2019). For example, overexpression WNT1/FGF3 signaling in MCF7 resulted in increased stemness by increased mitochondrial mass and thereby increasing the mitochondrial respiration (Lamb et al., 2015). Thus, various different cell-intrinsic and cell-extrinsic factors may modulate the “metabostemness.”
In addition to switching between glycolytic and OXPHOS phenotype, CSCs have also been shown to coopt these two pathways and exist in a hybrid metabolic state as suggested by recent studies (Yu et al., 2017). So, it is imperative to use drugs that block both glycolysis and OXPHOS to target CSC plasticity and has been found to inhibit tumor growth and metastasis (Cheong et al., 2011; Peiris-Pages et al., 2016). For instance, combining fasting induced hypoglycemia (to reduce glycolysis) with metformin, an OXPHOS inhibitor has impaired the metabolic plasticity of cancer cells by regulating PP2A-GSK3β-MCL-1 Axis (Elgendy et al., 2019).
Although most studies have focused on the glucose metabolism, the involvement of other metabolic pathways like lipid and amino acid metabolism still needs to be investigated further. A better understanding of the metabolic phenotypes and plasticity of CSC is required for the effective elimination of these cells.
Discussion
The issue of phenotypic plasticity presents a clear and present danger in the treatment of cancer patients. Accumulating evidence suggests that CSCs consist of different sub-populations that can interconvert among different states through intracellular and intercellular regulatory networks. Over expression of one or more transcription factors or activating trans-differentiation processes such as EMT and metabolic alterations can drive the switch among CSCs and non-CSCs as well as between different subsets of CSCs. These adaptive strategies adopted by cells must be taken into account while devising new therapeutic strategies in the clinic in order to target all populations effectively.
The challenges to addressing this issue are multifold. The ability to identify the plastic CSC population using markers has its inherent problems which are further confounded by each individual patient’s unique biochemical and biomechanical signatures. The general consensus is that drug resistance is achieved through a transition to a slow cycling state which is reversible once the stress is removed (De Angelis et al., 2019). Understanding how the cells switch from a slow cycling state and reenter the proliferative phase of the cell cycle will be key to targeting this population which contributes to minimal residual disease.
Using latest developments in computational and experimental methods will allow us to map the different states of tumor cells within multiple locations in a tumor, thus enabling a more comprehensive view of the genetic and non-genetic heterogeneity that exists within a cancer. Correlating such intratumoral heterogeneity with cellular phenotypes will be key to devising better therapeutic options in patients to ablate the tumor cells stably within a patient.
Author Contributions
RN and MJ designed the review. AT, KS, RM, MJ and RN wrote, read, and approved of the manuscript.
Funding
This work was supported by Ramanujan Fellowship awarded to RN (SB/S2/RJN/182/2014) and to MJ (SB/S2/RJN-049/2018) provided by Science and Engineering Research Board (SERB), Department of Science and Technology (DST), Government of India. AT was supported by CSIR (Council of Scientific Industrial Research) – Junior research fellowship.
Conflict of Interest
The authors declare that the research was conducted in the absence of any commercial or financial relationships that could be construed as a potential conflict of interest.
References
Agliano, A., Calvo, A., and Box, C. (2017). The challenge of targeting cancer stem cells to halt metastasis. Semin. Cancer Biol. 44, 25–42. doi: 10.1016/j.semcancer.2017.03.003
Akgul, S., Patch, A. M., D’Souza, R. C. J., Mukhopadhyay, P., Nones, K., Kempe, S., et al. (2019). Intratumoural heterogeneity underlies distinct therapy responses and treatment resistance in glioblastoma. Cancers (Basel) 11:190. doi: 10.3390/cancers11020190
Akrap, N., Andersson, D., Bom, E., Gregersson, P., Stahlberg, A., and Landberg, G. (2016). Identification of distinct breast cancer stem cell populations based on single-cell analyses of functionally enriched stem and progenitor pools. Stem Cell Rep. 6, 121–136. doi: 10.1016/j.stemcr.2015.12.006
Albini, A., Bruno, A., Gallo, C., Pajardi, G., Noonan, D. M., and Dallaglio, K. (2015). Cancer stem cells and the tumor microenvironment: interplay in tumor heterogeneity. Connect. Tissue Res. 56, 414–425. doi: 10.3109/03008207.2015.1066780
Alfarouk, K. O., Ibrahim, M. E., Gatenby, R. A., and Brown, J. S. (2013). Riparian ecosystems in human cancers. Evol. Appl. 6, 46–53. doi: 10.1111/eva.12015
Al-Hajj, M., Wicha, M. S., Benito-Hernandez, A., Morrison, S. J., and Clarke, M. F. (2003). Prospective identification of tumorigenic breast cancer cells. Proc. Natl. Acad. Sci. U.S.A. 100, 3983–3988.
Altrock, P. M., Liu, L. L., and Michor, F. (2015). The mathematics of cancer: integrating quantitative models. Nat. Rev. Cancer 15, 730–745. doi: 10.1038/nrc4029
Aponte, P. M., and Caicedo, A. (2017). Stemness in cancer: stem cells, cancer stem cells, and their microenvironment. Stem Cells Int. 2017:5619472.
Babahosseini, H., Ketene, A. N., Schmelz, E. M., Roberts, P. C., and Agah, M. (2014). Biomechanical profile of cancer stem-like/tumor-initiating cells derived from a progressive ovarian cancer model. Nanomedicine 10, 1013–1019.
Bachman, J. W., and Hillen, T. (2013). Mathematical optimization of the combination of radiation and differentiation therapies for cancer. Front. Oncol. 3:52. doi: 10.3389/fonc.2013.00052
Begum, A., McMillan, R. H., Chang, Y. T., Penchev, V. R., Rajeshkumar, N. V., Maitra, A., et al. (2019). Direct interactions with cancer-associated fibroblasts lead to enhanced pancreatic cancer stem cell function. Pancreas 48, 329–334. doi: 10.1097/mpa.0000000000001249
Bekaii-Saab, T., and El-Rayes, B. (2017). Identifying and targeting cancer stem cells in the treatment of gastric cancer. Cancer 123, 1303–1312. doi: 10.1002/cncr.30538
Ben-Porath, I., Thomson, M. W., Carey, V. J., Ge, R., Bell, G. W., Regev, A., et al. (2008). An embryonic stem cell-like gene expression signature in poorly differentiated aggressive human tumors. Nat. Genet. 40, 499–507. doi: 10.1038/ng.127
Bhatia, S., Monkman, J., Blick, T., Pinto, C., Waltham, M., Nagaraj, S. H., et al. (2019). Interrogation of phenotypic plasticity between epithelial and mesenchymal states in breast cancer. J. Clin. Med. 8:893. doi: 10.3390/jcm8060893
Biddle, A., Gammon, L., Liang, X., Costea, D. E., and Mackenzie, I. C. (2016). Phenotypic plasticity determines cancer stem cell therapeutic resistance in oral squamous cell carcinoma. EBioMedicine 4, 138–145. doi: 10.1016/j.ebiom.2016.01.007
Bierie, B., Pierce, S. E., Kroeger, C., Stover, D. G., Pattabiraman, D. R., Thiru, P., et al. (2017). Integrin-beta4 identifies cancer stem cell-enriched populations of partially mesenchymal carcinoma cells. Proc. Natl. Acad. Sci. U.S.A. 114, E2337–E2346.
Bocci, F., Gearhart-Serna, L., Boareto, M., Ribeiro, M., Ben-Jacob, E., Devi, G. R., et al. (2019). Toward understanding cancer stem cell heterogeneity in the tumor microenvironment. Proc. Natl. Acad. Sci. U.S.A. 116, 148–157. doi: 10.1073/pnas.1815345116
Bocci, F., Jolly, M. K., George, J. T., Levine, H., and Onuchic, J. N. (2018). A mechanism-based computational model to capture the interconnections among epithelial-mesenchymal transition, cancer stem cells and Notch-Jagged signaling. Oncotarget 9, 29906–29920.
Bongiorno, T., Gura, J., Talwar, P., Chambers, D., Young, K. M., Arafat, D., et al. (2018). Biophysical subsets of embryonic stem cells display distinct phenotypic and morphological signatures. PLoS One 13:e0192631. doi: 10.1371/journal.pone.0192631
Brabletz, T., Jung, A., Reu, S., Porzner, M., Hlubek, F., Kunz-Schughart, L. A., et al. (2001). Variable beta-catenin expression in colorectal cancers indicates tumor progression driven by the tumor environment. Proc. Natl. Acad. Sci. U.S.A. 98, 10356–10361. doi: 10.1073/pnas.171610498
Broders-Bondon, F., Nguyen, T. H., Ho-Bouldoires, M., Fernandez-Sanchez, E., and Farge, E. (2018). Mechanotransduction in tumor progression: the dark side of the force. J. Cell Biol. 217, 1571–1587. doi: 10.1083/jcb.201701039
Bronsert, P., Enderle-Ammour, K., Bader, M., Timme, S., Kuehs, M., Csanadi, A., et al. (2014). Cancer cell invasion and EMT marker expression: a three-dimensional study of the human cancer-host interface. J. Pathol. 234, 410–422. doi: 10.1002/path.4416
Bussolati, B., Bruno, S., Grange, C., Ferrando, U., and Camussi, G. (2008). Identification of a tumor-initiating stem cell population in human renal carcinomas. FASEB J. 22, 3696–3705. doi: 10.1096/fj.08-102590
Byun, S., Son, S., Amodei, D., Cermak, N., Shaw, J., Kang, J. H., et al. (2013). Characterizing deformability and surface friction of cancer cells. Proc. Natl. Acad. Sci. U.S.A. 110, 7580–7585. doi: 10.1073/pnas.1218806110
Cabrera, M. C., Hollingsworth, R. E., and Hurt, E. M. (2015). Cancer stem cell plasticity and tumor hierarchy. World J. Stem Cells 7, 27–36.
Celia-Terrassa, T., Bastian, C., Liu, D. D., Ell, B., Aiello, N. M., Wei, Y., et al. (2018). Hysteresis control of epithelial-mesenchymal transition dynamics conveys a distinct program with enhanced metastatic ability. Nat. Commun. 9:5005.
Celia-Terrassa, T., and Jolly, M. K. (2019). Cancer stem cells and epithelial-to-mesenchymal transition in cancer metastasis. Cold Spring Harb. Perspect. Med. doi: 10.1101/cshperspect.a036905
Chae, Y. C., and Kim, J. H. (2018). Cancer stem cell metabolism: target for cancer therapy. BMB Rep. 51, 319–326. doi: 10.5483/bmbrep.2018.51.7.112
Chaffer, C. L., Brueckmann, I., Scheel, C., Kaestli, A. J., Wiggins, P. A., Rodrigues, L. O., et al. (2011). Normal and neoplastic nonstem cells can spontaneously convert to a stem-like state. Proc. Natl. Acad. Sci. U.S.A. 108, 7950–7955. doi: 10.1073/pnas.1102454108
Chaffer, C. L., Marjanovic, N. D., Lee, T., Bell, G., Kleer, C. G., Reinhardt, F., et al. (2013). Poised chromatin at the ZEB1 promoter enables breast cancer cell plasticity and enhances tumorigenicity. Cell 154, 61–74. doi: 10.1016/j.cell.2013.06.005
Chang, C. H., Qiu, J., O’Sullivan, D., Buck, M. D., Noguchi, T., Curtis, J. D., et al. (2015). Metabolic competition in the tumor microenvironment is a driver of cancer progression. Cell 162, 1229–1241. doi: 10.1016/j.cell.2015.08.016
Chang, C. J., Yang, J. Y., Xia, W., Chen, C. T., Xie, X., Chao, C. H., et al. (2011). EZH2 promotes expansion of breast tumor initiating cells through activation of RAF1-beta-catenin signaling. Cancer Cell 19, 86–100. doi: 10.1016/j.ccr.2010.10.035
Chen, J., and Kumar, S. (2017). Biophysical regulation of cancer stem/initiating cells: implications for disease mechanisms and translation. Curr. Opin. Biomed. Eng. 1, 87–95. doi: 10.1016/j.cobme.2017.02.006
Chen, J., Zhou, W., Jia, Q., Chen, J., Zhang, S., Yao, W., et al. (2016). Efficient extravasation of tumor-repopulating cells depends on cell deformability. Sci. Rep. 6:19304.
Chen, K., Huang, Y. H., and Chen, J. L. (2013). Understanding and targeting cancer stem cells: therapeutic implications and challenges. Acta Pharmacol. Sin. 34, 732–740. doi: 10.1038/aps.2013.27
Chen, K. H., Boettiger, A. N., Moffitt, J. R., Wang, S., and Zhuang, X. (2015). RNA imaging. Spatially resolved, highly multiplexed RNA profiling in single cells. Science 348:aaa6090. doi: 10.1126/science.aaa6090
Chen, W., Allen, S. G., Qian, W., Peng, Z., Han, S., Li, X., et al. (2019). Biophysical phenotyping and modulation of ALDH+ inflammatory breast cancer stem-like cells. Small 15:e1802891.
Chen, W., Dong, J., Haiech, J., Kilhoffer, M. C., and Zeniou, M. (2016). Cancer stem cell quiescence and plasticity as major challenges in cancer therapy. Stem Cells Int. 2016:1740936.
Chen, W. J., Ho, C. C., Chang, Y. L., Chen, H. Y., Lin, C. A., Ling, T. Y., et al. (2014). Cancer-associated fibroblasts regulate the plasticity of lung cancer stemness via paracrine signalling. Nat. Commun. 5:3472.
Cheong, J. H., Park, E. S., Liang, J., Dennison, J. B., Tsavachidou, D., Nguyen-Charles, C., et al. (2011). Dual inhibition of tumor energy pathway by 2-deoxyglucose and metformin is effective against a broad spectrum of preclinical cancer models. Mol. Cancer Ther. 10, 2350–2362. doi: 10.1158/1535-7163.mct-11-0497
Chumakova, A. P., Hitomi, M., Sulman, E. P., and Lathia, J. D. (2019). High-throughput Automated single-cell imaging analysis reveals dynamics of glioblastoma stem cell population during state transition. Cytometry A 95, 290–301. doi: 10.1002/cyto.a.23728
Ciavardelli, D., Rossi, C., Barcaroli, D., Volpe, S., Consalvo, A., Zucchelli, M., et al. (2014). Breast cancer stem cells rely on fermentative glycolysis and are sensitive to 2-deoxyglucose treatment. Cell Death Dis. 5:e1336. doi: 10.1038/cddis.2014.285
Collins, A. T., Berry, P. A., Hyde, C., Stower, M. J., and Maitland, N. J. (2005). Prospective identification of tumorigenic prostate cancer stem cells. Cancer Res. 65, 10946–10951. doi: 10.1158/0008-5472.can-05-2018
Conley, S. J., Gheordunescu, E., Kakarala, P., Newman, B., Korkaya, H., Heath, A. N., et al. (2012). Antiangiogenic agents increase breast cancer stem cells via the generation of tumor hypoxia. Proc. Natl. Acad. Sci. U.S.A. 109, 2784–2789. doi: 10.1073/pnas.1018866109
da Silva-Diz, V., Lorenzo-Sanz, L., Bernat-Peguera, A., Lopez-Cerda, M., and Munoz, P. (2018). Cancer cell plasticity: impact on tumor progression and therapy response. Semin. Cancer Biol. 53, 48–58. doi: 10.1016/j.semcancer.2018.08.009
Dagogo-Jack, I., and Shaw, A. T. (2018). Tumour heterogeneity and resistance to cancer therapies. Nat. Rev. Clin. Oncol. 15, 81–94. doi: 10.1038/nrclinonc.2017.166
De Angelis, M. L., Francescangeli, F., La Torre, F., and Zeuner, A. (2019). Stem cell plasticity and dormancy in the development of cancer therapy resistance. Front. Oncol. 9:626. doi: 10.3389/fonc.2019.00626
De Francesco, E. M., Sotgia, F., and Lisanti, M. P. (2018). Cancer stem cells (CSCs): metabolic strategies for their identification and eradication. Biochem. J. 475, 1611–1634. doi: 10.1042/bcj20170164
De Luca, A., Fiorillo, M., Peiris-Pages, M., Ozsvari, B., Smith, D. L., Sanchez-Alvarez, R., et al. (2015). Mitochondrial biogenesis is required for the anchorage-independent survival and propagation of stem-like cancer cells. Oncotarget 6, 14777–14795.
de Sousa e Melo, F., Kurtova, A. V., Harnoss, J. M., Kljavin, N., Hoeck, J. D., Hung, J., et al. (2017). A distinct role for Lgr5(+) stem cells in primary and metastatic colon cancer. Nature 543, 676–680. doi: 10.1038/nature21713
Di Carlo, C., Brandi, J., and Cecconi, D. (2018). Pancreatic cancer stem cells: perspectives on potential therapeutic approaches of pancreatic ductal adenocarcinoma. World J. Stem Cells 10, 172–182. doi: 10.4252/wjsc.v10.i11.172
Dirkse, A., Golebiewska, A., Buder, T., Nazarov, P. V., Muller, A., Poovathingal, S., et al. (2019). Stem cell-associated heterogeneity in glioblastoma results from intrinsic tumor plasticity shaped by the microenvironment. Nat. Commun. 10:1787.
Doherty, M. R., Parvani, J. G., Tamagno, I., Junk, D. J., Bryson, B. L., Cheon, H. J., et al. (2019). The opposing effects of interferon-beta and oncostatin-M as regulators of cancer stem cell plasticity in triple-negative breast cancer. Breast Cancer Res. 21:54.
Doherty, M. R., Smigiel, J. M., Junk, D. J., and Jackson, M. W. (2016). Cancer stem cell plasticity drives therapeutic resistance. Cancers (Basel) 8:8. doi: 10.3390/cancers8010008
Dongre, A., and Weinberg, R. A. (2019). New insights into the mechanisms of epithelial-mesenchymal transition and implications for cancer. Nat. Rev. Mol. Cell. Biol. 20, 69–84. doi: 10.1038/s41580-018-0080-4
Du, Y., Shao, H., Moller, M., Prokupets, R., Tse, Y. T., and Liu, Z. J. (2019). Intracellular Notch1 signaling in cancer-associated fibroblasts dictates the plasticity and stemness of melanoma stem/initiating cells. Stem Cells 37, 865–875. doi: 10.1002/stem.3013
Dupuy, F., Tabaries, S., Andrzejewski, S., Dong, Z., Blagih, J., Annis, M. G., et al. (2015). PDK1-Dependent metabolic reprogramming dictates metastatic potential in breast cancer. Cell Metab. 22, 577–589. doi: 10.1016/j.cmet.2015.08.007
Easwaran, H., Tsai, H. C., and Baylin, S. B. (2014). Cancer epigenetics: tumor heterogeneity, plasticity of stem-like states, and drug resistance. Mol. Cell 54, 716–727. doi: 10.1016/j.molcel.2014.05.015
Edsgard, D., Johnsson, P., and Sandberg, R. (2018). Identification of spatial expression trends in single-cell gene expression data. Nat. Methods 15, 339–342. doi: 10.1038/nmeth.4634
Eirew, P., Steif, A., Khattra, J., Ha, G., Yap, D., Farahani, H., et al. (2015). Dynamics of genomic clones in breast cancer patient xenografts at single-cell resolution. Nature 518, 422–426.
Elgendy, M., Ciro, M., Hosseini, A., Weiszmann, J., Mazzarella, L., Ferrari, E., et al. (2019). Combination of hypoglycemia and metformin impairs tumor metabolic plasticity and growth by modulating the PP2A-GSK3beta-MCL-1 Axis. Cancer Cell 35, 798–815.e5. doi: 10.1016/j.ccell.2019.03.007
Enderling, H. (2015). Cancer stem cells: small subpopulation or evolving fraction? Integr. Biol. (Camb) 7, 14–23. doi: 10.1039/c4ib00191e
Etzrodt, M., Endele, M., and Schroeder, T. (2014). Quantitative single-cell approaches to stem cell research. Cell Stem Cell 15, 546–558. doi: 10.1016/j.stem.2014.10.015
Eun, K., Ham, S. W., and Kim, H. (2017). Cancer stem cell heterogeneity: origin and new perspectives on CSC targeting. BMB Rep. 50, 117–125. doi: 10.5483/bmbrep.2017.50.3.222
Fang, D., Nguyen, T. K., Leishear, K., Finko, R., Kulp, A. N., Hotz, S., et al. (2005). A tumorigenic subpopulation with stem cell properties in melanomas. Cancer Res. 65, 9328–9337. doi: 10.1158/0008-5472.can-05-1343
Farnie, G., Sotgia, F., and Lisanti, M. P. (2015). High mitochondrial mass identifies a sub-population of stem-like cancer cells that are chemo-resistant. Oncotarget 6, 30472–30486.
Fernandez-Cortes, M., Delgado-Bellido, D., and Oliver, F. J. (2019). Vasculogenic mimicry: become an endothelial cell but not so much. Front. Oncol. 9:803. doi: 10.3389/fonc.2019.00803
Fumagalli, A., Bruens, L., Scheele, C., and van Rheenen, J. (2019). Capturing stem cell behavior using intravital and live cell microscopy. Cold Spring Harb. Perspect. Biol. doi: 10.1101/cshperspect.a035949
Fumagalli, A., Oost, K. C., Kester, L., Morgner, J., Bornes, L., Bruens, L., et al. (2020). Plasticity of Lgr5-negative cancer cells drives metastasis in colorectal cancer. Cell Stem Cell 26, 569–578.e7.
Gallaher, J. A., Enriquez-Navas, P. M., Luddy, K. A., Gatenby, R. A., and Anderson, A. R. A. (2018). Spatial heterogeneity and evolutionary dynamics modulate time to recurrence in continuous and adaptive cancer therapies. Cancer Res. 78, 2127–2139. doi: 10.1158/0008-5472.can-17-2649
Gammon, L., Biddle, A., Heywood, H. K., Johannessen, A. C., and Mackenzie, I. C. (2013). Sub-sets of cancer stem cells differ intrinsically in their patterns of oxygen metabolism. PLoS One 8:e62493. doi: 10.1371/journal.pone.0062493
Gan, L., Yang, Y., Li, Q., Feng, Y., Liu, T., and Guo, W. (2018). Epigenetic regulation of cancer progression by EZH2: from biological insights to therapeutic potential. Biomark Res. 6:10.
George, J. T., Jolly, M. K., Xu, S., Somarelli, J. A., and Levine, H. (2017). Survival outcomes in cancer patients predicted by a partial EMT gene expression scoring metric. Cancer Res. 77, 6415–6428. doi: 10.1158/0008-5472.can-16-3521
Giannone, G., Attademo, L., Scotto, G., Genta, S., Ghisoni, E., Tuninetti, V., et al. (2019). Endometrial cancer stem cells: role, characterization and therapeutic implications. Cancers (Basel) 11:1820. doi: 10.3390/cancers11111820
Giraddi, R. R., Chung, C. Y., Heinz, R. E., Balcioglu, O., Novotny, M., Trejo, C. L., et al. (2018). Single-cell transcriptomes distinguish stem cell state changes and lineage specification programs in early mammary gland development. Cell Rep. 24, 1653–1666.e7. doi: 10.1016/j.celrep.2018.07.025
Goldman, A., Majumder, B., Dhawan, A., Ravi, S., Goldman, D., Kohandel, M., et al. (2015). Temporally sequenced anticancer drugs overcome adaptive resistance by targeting a vulnerable chemotherapy-induced phenotypic transition. Nat. Commun. 6:6139.
Gong, C., Anders, R. A., Zhu, Q., Taube, J. M., Green, B., Cheng, W., et al. (2018). Quantitative characterization of CD8+ T cell clustering and spatial heterogeneity in solid tumors. Front. Oncol. 8:649. doi: 10.3389/fonc.2018.00649
Gossett, D. R., Tse, H. T., Lee, S. A., Ying, Y., Lindgren, A. G., Yang, O. O., et al. (2012). Hydrodynamic stretching of single cells for large population mechanical phenotyping. Proc. Natl. Acad. Sci. U.S.A. 109, 7630–7635. doi: 10.1073/pnas.1200107109
Greve, B., Kelsch, R., Spaniol, K., Eich, H. T., and Gotte, M. (2012). Flow cytometry in cancer stem cell analysis and separation. Cytometry A 81, 284–293. doi: 10.1002/cyto.a.22022
Grigore, A. D., Jolly, M. K., Jia, D., Farach-Carson, M. C., and Levine, H. (2016). Tumor budding: the name is EMT. Partial EMT. J. Clin. Med. 5:51. doi: 10.3390/jcm5050051
Grosse-Wilde, A., d’Herouel, A., McIntosh, E., Ertaylan, G., Skupin, A., Kuestner, R. E., et al. (2015). Stemness of the hybrid epithelial/mesenchymal state in breast cancer and its association with poor survival. PLoS One 10:e0126522. doi: 10.1371/journal.pone.0126522
Gu, G., Yuan, J., Wills, M., and Kasper, S. (2007). Prostate cancer cells with stem cell characteristics reconstitute the original human tumor in vivo. Cancer Res 67, 4807–4815. doi: 10.1158/0008-5472.can-06-4608
Guha, M., Srinivasan, S., Ruthel, G., Kashina, A. K., Carstens, R. P., Mendoza, A., et al. (2014). Mitochondrial retrograde signaling induces epithelial-mesenchymal transition and generates breast cancer stem cells. Oncogene 33, 5238–5250. doi: 10.1038/onc.2013.467
Gupta, P. B., Fillmore, C. M., Jiang, G., Shapira, S. D., Tao, K., Kuperwasser, C., et al. (2011). Stochastic state transitions give rise to phenotypic equilibrium in populations of cancer cells. Cell 146, 633–644. doi: 10.1016/j.cell.2011.07.026
Gupta, P. B., Pastushenko, I., Skibinski, A., Blanpain, C., and Kuperwasser, C. (2019). Phenotypic plasticity: driver of cancer initiation, progression, and therapy resistance. Cell Stem Cell 24, 65–78. doi: 10.1016/j.stem.2018.11.011
Hanahan, D., and Weinberg, R. A. (2011). Hallmarks of cancer: the next generation. Cell 144, 646–674. doi: 10.1016/j.cell.2011.02.013
Hari, K., Sabuwala, B., Subramani, B. V., La Porta, C. A. M., Zapperi, S., Font-Clos, F., et al. (2019). Identifying inhibitors of epithelial-mesenchymal plasticity using a network topology based approach. bioRxiv [Preprint]. doi: 10.1101/854307
Heddleston, J. M., Li, Z., McLendon, R. E., Hjelmeland, A. B., and Rich, J. N. (2009). The hypoxic microenvironment maintains glioblastoma stem cells and promotes reprogramming towards a cancer stem cell phenotype. Cell Cycle 8, 3274–3284. doi: 10.4161/cc.8.20.9701
Hillen, T., Enderling, H., and Hahnfeldt, P. (2013). The tumor growth paradox and immune system-mediated selection for cancer stem cells. Bull. Math. Biol. 75, 161–184. doi: 10.1007/s11538-012-9798-x
Hjelmeland, A. B., Wu, Q., Heddleston, J. M., Choudhary, G. S., MacSwords, J., Lathia, J. D., et al. (2011). Acidic stress promotes a glioma stem cell phenotype. Cell Death Differ. 18, 829–840. doi: 10.1038/cdd.2010.150
Hou, Y., Guo, H., Cao, C., Li, X., Hu, B., Zhu, P., et al. (2016). Single-cell triple omics sequencing reveals genetic, epigenetic, and transcriptomic heterogeneity in hepatocellular carcinomas. Cell Res. 26, 304–319. doi: 10.1038/cr.2016.23
Iliopoulos, D., Hirsch, H. A., Wang, G., and Struhl, K. (2011). Inducible formation of breast cancer stem cells and their dynamic equilibrium with non-stem cancer cells via IL6 secretion. Proc. Natl. Acad. Sci. U.S.A. 108, 1397–1402. doi: 10.1073/pnas.1018898108
Ito, K., and Suda, T. (2014). Metabolic requirements for the maintenance of self-renewing stem cells. Nat. Rev. Mol. Cell. Biol. 15, 243–256. doi: 10.1038/nrm3772
Jaggupilli, A., and Elkord, E. (2012). Significance of CD44 and CD24 as cancer stem cell markers: an enduring ambiguity. Clin. Dev. Immunol. 2012:708036.
Januskeviciene, I., and Petrikaite, V. (2019). Heterogeneity of breast cancer: the importance of interaction between different tumor cell populations. Life Sci. 239:117009. doi: 10.1016/j.lfs.2019.117009
Jia, D., Jolly, M. K., Tripathi, S. C., Den Hollander, P., Huang, B., Lu, M., et al. (2017). Distinguishing mechanisms underlying EMT tristability. Cancer Converg. 1:2.
Jolly, M. K., Huang, B., Lu, M., Mani, S. A., Levine, H., and Ben-Jacob, E. (2014). Towards elucidating the connection between epithelial-mesenchymal transitions and stemness. J. R. Soc. Interface 11:20140962. doi: 10.1098/rsif.2014.0962
Jolly, M. K., Kulkarni, P., Weninger, K., Orban, J., and Levine, H. (2018a). Phenotypic plasticity, bet-hedging, and androgen independence in prostate cancer: role of non-genetic heterogeneity. Front. Oncol. 8:50. doi: 10.3389/fonc.2018.00050
Jolly, M. K., Mani, S. A., and Levine, H. (2018b). Hybrid epithelial/mesenchymal phenotype(s): the ‘fittest’ for metastasis? Biochim. Biophys. Acta Rev. Cancer 1870, 151–157. doi: 10.1016/j.bbcan.2018.07.001
Jolly, M. K., Preca, B. T., Tripathi, S. C., Jia, D., George, J. T., Hanash, S. M., et al. (2018c). Interconnected feedback loops among ESRP1, HAS2, and CD44 regulate epithelial-mesenchymal plasticity in cancer. APL Bioeng. 2:031908. doi: 10.1063/1.5024874
Jolly, M. K., Somarelli, J. A., Sheth, M., Biddle, A., Tripathi, S. C., Armstrong, A. J., et al. (2019). Hybrid epithelial/mesenchymal phenotypes promote metastasis and therapy resistance across carcinomas. Pharmacol. Ther. 194, 161–184. doi: 10.1016/j.pharmthera.2018.09.007
Jolly, M. K., Tripathi, S. C., Somarelli, J. A., Hanash, S. M., and Levine, H. (2017). Epithelial/mesenchymal plasticity: how have quantitative mathematical models helped improve our understanding? Mol. Oncol. 11, 739–754. doi: 10.1002/1878-0261.12084
Jolly, M. K., Ward, C., Eapen, M. S., Myers, S., Hallgren, O., Levine, H., et al. (2018d). Epithelial-mesenchymal transition, a spectrum of states: role in lung development, homeostasis, and disease. Dev. Dyn. 247, 346–358. doi: 10.1002/dvdy.24541
Jonasson, E., Ghannoum, S., Persson, E., Karlsson, J., Kroneis, T., Larsson, E., et al. (2019). Identification of breast cancer stem cell related genes using functional cellular assays combined with single-cell RNA sequencing in MDA-MB-231 cells. Front. Genet. 10:500. doi: 10.3389/fgene.2019.00500
Jung, A., Schrauder, M., Oswald, U., Knoll, C., Sellberg, P., Palmqvist, R., et al. (2001). The invasion front of human colorectal adenocarcinomas shows co-localization of nuclear beta-catenin, cyclin D1, and p16INK4A and is a region of low proliferation. Am. J. Pathol. 159, 1613–1617. doi: 10.1016/s0002-9440(10)63007-6
Junk, D. J., Bryson, B. L., Smigiel, J. M., Parameswaran, N., Bartel, C. A., and Jackson, M. W. (2017). Oncostatin M promotes cancer cell plasticity through cooperative STAT3-SMAD3 signaling. Oncogene 36, 4001–4013. doi: 10.1038/onc.2017.33
Kalluri, R., and Weinberg, R. A. (2009). The basics of epithelial-mesenchymal transition. J Clin Invest 119, 1420–1428. doi: 10.1172/jci39104
Kim, H., Lin, Q., Glazer, P. M., and Yun, Z. (2018). The hypoxic tumor microenvironment in vivo selects the cancer stem cell fate of breast cancer cells. Breast Cancer Res. 20:16.
Kim, J., and DeBerardinis, R. J. (2019). Mechanisms and implications of metabolic heterogeneity in cancer. Cell Metab. 30, 434–446. doi: 10.1016/j.cmet.2019.08.013
Kobayashi, S., Yamada-Okabe, H., Suzuki, M., Natori, O., Kato, A., Matsubara, K., et al. (2012). LGR5-positive colon cancer stem cells interconvert with drug-resistant LGR5-negative cells and are capable of tumor reconstitution. Stem Cells 30, 2631–2644. doi: 10.1002/stem.1257
Korkaya, H., Liu, S., and Wicha, M. S. (2011). Breast cancer stem cells, cytokine networks, and the tumor microenvironment. J. Clin. Invest. 121, 3804–3809.
Kraning-Rush, C. M., Califano, J. P., and Reinhart-King, C. A. (2012). Cellular traction stresses increase with increasing metastatic potential. PLoS One 7:e32572. doi: 10.1371/journal.pone.0032572
Kroger, C., Afeyan, A., Mraz, J., Eaton, E. N., Reinhardt, F., Khodor, Y. L., et al. (2019). Acquisition of a hybrid E/M state is essential for tumorigenicity of basal breast cancer cells. Proc. Natl. Acad. Sci. U.S.A. 116, 7353–7362. doi: 10.1073/pnas.1812876116
Kumar, D., Gorain, M., Kundu, G., and Kundu, G. C. (2017). Therapeutic implications of cellular and molecular biology of cancer stem cells in melanoma. Mol. Cancer 16:7.
Kwa, M. Q., Herum, K. M., and Brakebusch, C. (2019). Cancer-associated fibroblasts: how do they contribute to metastasis? Clin. Exp. Metastasis 36, 71–86.
Lamb, R., Bonuccelli, G., Ozsvari, B., Peiris-Pages, M., Fiorillo, M., Smith, D. L., et al. (2015). Mitochondrial mass, a new metabolic biomarker for stem-like cancer cells: understanding WNT/FGF-driven anabolic signaling. Oncotarget 6, 30453–30471.
Lapidot, T., Sirard, C., Vormoor, J., Murdoch, B., Hoang, T., Caceres-Cortes, J., et al. (1994). A cell initiating human acute myeloid leukaemia after transplantation into SCID mice. Nature 367, 645–648. doi: 10.1038/367645a0
Lau, E. Y., Lo, J., Cheng, B. Y., Ma, M. K., Lee, J. M., Ng, J. K., et al. (2016). Cancer-associated fibroblasts regulate tumor-initiating cell plasticity in hepatocellular carcinoma through c-Met/FRA1/HEY1 signaling. Cell Rep. 15, 1175–1189. doi: 10.1016/j.celrep.2016.04.019
Lee, J. H., Daugharthy, E. R., Scheiman, J., Kalhor, R., Yang, J. L., Ferrante, T. C., et al. (2014). Highly multiplexed subcellular RNA sequencing in situ. Science 343, 1360–1363. doi: 10.1126/science.1250212
Lehuede, C., Dupuy, F., Rabinovitch, R., Jones, R. G., and Siegel, P. M. (2016). Metabolic plasticity as a determinant of tumor growth and metastasis. Cancer Res. 76, 5201–5208. doi: 10.1158/0008-5472.can-16-0266
Lequeux, A., Noman, M. Z., Xiao, M., Sauvage, D., Van Moer, K., and Viry, E. (2019). Impact of hypoxic tumor microenvironment and tumor cell plasticity on the expression of immune checkpoints. Cancer Lett. 458, 13–20. doi: 10.1016/j.canlet.2019.05.021
Leung, E. L., Fiscus, R. R., Tung, J. W., Tin, V. P., Cheng, L. C., Sihoe, A. D., et al. (2010). Non-small cell lung cancer cells expressing CD44 are enriched for stem cell-like properties. PLoS One 5:e14062. doi: 10.1371/journal.pone.0014062
Lewis, P. W., Muller, M. M., Koletsky, M. S., Cordero, F., Lin, S., Banaszynski, L. A., et al. (2013). Inhibition of PRC2 activity by a gain-of-function H3 mutation found in pediatric glioblastoma. Science 340, 857–861. doi: 10.1126/science.1232245
Li, C., Heidt, D. G., Dalerba, P., Burant, C. F., Zhang, L., Adsay, V., et al. (2007). Identification of pancreatic cancer stem cells. Cancer Res. 67, 1030–1037.
Li, X., Jolly, M. K., George, J. T., Pienta, K. J., and Levine, H. (2019). Computational modeling of the crosstalk between macrophage polarization and tumor cell plasticity in the tumor microenvironment. Front. Oncol. 9:10. doi: 10.3389/fonc.2019.00010
Liberti, M. V., and Locasale, J. W. (2016). The warburg effect: how does it benefit cancer cells? Trends Biochem. Sci. 41, 211–218. doi: 10.1016/j.tibs.2015.12.001
Little, S. E., Popov, S., Jury, A., Bax, D. A., Doey, L., Al-Sarraj, S., et al. (2012). Receptor tyrosine kinase genes amplified in glioblastoma exhibit a mutual exclusivity in variable proportions reflective of individual tumor heterogeneity. Cancer Res. 72, 1614–1620. doi: 10.1158/0008-5472.can-11-4069
Liu, A., Yu, X., and Liu, S. (2013). Pluripotency transcription factors and cancer stem cells: small genes make a big difference. Chin. J. Cancer 32, 483–487.
Liu, L., Liu, C., Quintero, A., Wu, L., Yuan, Y., Wang, M., et al. (2019). Deconvolution of single-cell multi-omics layers reveals regulatory heterogeneity. Nat. Commun. 10:470.
Liu, P. P., Liao, J., Tang, Z. J., Wu, W. J., Yang, J., Zeng, Z. L., et al. (2014). Metabolic regulation of cancer cell side population by glucose through activation of the Akt pathway. Cell Death Differ. 21, 124–135. doi: 10.1038/cdd.2013.131
Liu, S., Cong, Y., Wang, D., Sun, Y., Deng, L., Liu, Y., et al. (2014). Breast cancer stem cells transition between epithelial and mesenchymal states reflective of their normal counterparts. Stem Cell Rep. 2, 78–91. doi: 10.1016/j.stemcr.2013.11.009
Liu, T. J., Sun, B. C., Zhao, X. L., Zhao, X. M., Sun, T., Gu, Q., et al. (2013). CD133+ cells with cancer stem cell characteristics associates with vasculogenic mimicry in triple-negative breast cancer. Oncogene 32, 544–553. doi: 10.1038/onc.2012.85
Liu, Z., Lee, Y., Jang, J., Li, Y., Han, X., Yokoi, K., et al. (2015). Microfluidic cytometric analysis of cancer cell transportability and invasiveness. Sci. Rep. 5:14272.
Lonardo, E., Cioffi, M., Sancho, P., Sanchez-Ripoll, Y., Trabulo, S. M., Dorado, J., et al. (2013). Metformin targets the metabolic achilles heel of human pancreatic cancer stem cells. PLoS One 8:e76518. doi: 10.1371/journal.pone.0076518
Luo, M., and Wicha, M. S. (2015). Metabolic plasticity of cancer stem cells. Oncotarget 6, 35141–35142.
Macaulay, I. C., Ponting, C. P., and Voet, T. (2017). Single-cell multiomics: multiple measurements from single cells. Trends Genet 33, 155–168. doi: 10.1016/j.tig.2016.12.003
Maiuthed, A., Chantarawong, W., and Chanvorachote, P. (2018). Lung cancer stem cells and cancer stem cell-targeting natural compounds. Anticancer Res. 38, 3797–3809. doi: 10.21873/anticanres.12663
Malta, T. M., Sokolov, A., Gentles, A. J., Burzykowski, T., Poisson, L., Weinstein, J. N., et al. (2018). Machine learning identifies stemness features associated with oncogenic dedifferentiation. Cell 173, 338–354.e5.
Mani, S. A., Guo, W., Liao, M. J., Eaton, E. N., Ayyanan, A., Zhou, A. Y., et al. (2008). The epithelial-mesenchymal transition generates cells with properties of stem cells. Cell 133, 704–715.
Marjanovic, N. D., Weinberg, R. A., and Chaffer, C. L. (2013). Cell plasticity and heterogeneity in cancer. Clin. Chem. 59, 168–179. doi: 10.1373/clinchem.2012.184655
Martano, G., Borroni, E. M., Lopci, E., Cattaneo, M. G., Mattioli, M., Bachi, A., et al. (2019). Metabolism of stem and progenitor cells: proper methods to answer specific questions. Front. Mol. Neurosci. 12:151. doi: 10.3389/fnmol.2019.00151
Martin, P., Liu, Y. N., Pierce, R., Abou-Kheir, W., Casey, O., Seng, V., et al. (2011). Prostate epithelial Pten/TP53 loss leads to transformation of multipotential progenitors and epithelial to mesenchymal transition. Am. J. Pathol. 179, 422–435. doi: 10.1016/j.ajpath.2011.03.035
McGranahan, N., and Swanton, C. (2017). Clonal heterogeneity and tumor evolution: past, present, and the future. Cell 168, 613–628. doi: 10.1016/j.cell.2017.01.018
McKenna, A., and Gagnon, J. A. (2019). Recording development with single cell dynamic lineage tracing. Development 146:dev169730. doi: 10.1242/dev.169730
Menendez, J. A., and Alarcon, T. (2014). Metabostemness: a new cancer hallmark. Front. Oncol. 4:262. doi: 10.3389/fonc.2014.00262
Mooney, S. M., Talebian, V., Jolly, M. K., Jia, D., Gromala, M., Levine, H., et al. (2017). The GRHL2/ZEB feedback loop-a key axis in the regulation of EMT in breast cancer. J. Cell Biochem. 118, 2559–2570. doi: 10.1002/jcb.25974
Moreira, M. P., Brayner, F. A., Alves, L. C., Cassali, G. D., and Silva, L. M. (2019). Phenotypic, structural, and ultrastructural analysis of triple-negative breast cancer cell lines and breast cancer stem cell subpopulation. Eur. Biophys. J. 48, 673–684. doi: 10.1007/s00249-019-01393-0
Morrison, S. J., and Spradling, A. C. (2008). Stem cells and niches: mechanisms that promote stem cell maintenance throughout life. Cell 132, 598–611. doi: 10.1016/j.cell.2008.01.038
Mourao, L., Jacquemin, G., Huyghe, M., Nawrocki, W. J., Menssouri, N., Servant, N., et al. (2019). Lineage tracing of Notch1-expressing cells in intestinal tumours reveals a distinct population of cancer stem cells. Sci. Rep. 9:888.
Nallanthighal, S., Heiserman, J. P., and Cheon, D. J. (2019). The role of the extracellular matrix in cancer stemness. Front. Cell Dev. Biol. 7:86. doi: 10.3389/fcell.2019.00086
Nath, B., Bidkar, A. P., Kumar, V., Dalal, A., Jolly, M. K., Ghosh, S. S., et al. (2019). Deciphering hydrodynamic and drug-resistant behaviors of metastatic EMT breast cancer cells moving in a constricted microcapillary. J. Clin. Med. 8:1194. doi: 10.3390/jcm8081194
Navin, N. E. (2015). The first five years of single-cell cancer genomics and beyond. Genome Res. 25, 1499–1507. doi: 10.1101/gr.191098.115
Ogden, A. T., Waziri, A. E., Lochhead, R. A., Fusco, D., Lopez, K., Ellis, J. A., et al. (2008). Identification of A2B5+CD133- tumor-initiating cells in adult human gliomas. Neurosurgery 62, 505–514.
Oshima, N., Yamada, Y., Nagayama, S., Kawada, K., Hasegawa, S., Okabe, H., et al. (2014). Induction of cancer stem cell properties in colon cancer cells by defined factors. PLoS One 9:e101735. doi: 10.1371/journal.pone.0101735
Papa, S., Choy, P. M., and Bubici, C. (2019). The ERK and JNK pathways in the regulation of metabolic reprogramming. Oncogene 38, 2223–2240. doi: 10.1038/s41388-018-0582-8
Pascual, G., Dominguez, D., and Benitah, S. A. (2018). The contributions of cancer cell metabolism to metastasis. Dis. Model. Mech. 11:dmm032920. doi: 10.1242/dmm.032920
Pastushenko, I., Brisebarre, A., Sifrim, A., Fioramonti, M., Revenco, T., Boumahdi, S., et al. (2018). Identification of the tumour transition states occurring during EMT. Nature 556, 463–468. doi: 10.1038/s41586-018-0040-3
Patel, A. P., Tirosh, I., Trombetta, J. J., Shalek, A. K., Gillespie, S. M., Wakimoto, H., et al. (2014). Single-cell RNA-seq highlights intratumoral heterogeneity in primary glioblastoma. Science 344, 1396–1401. doi: 10.1126/science.1254257
Pattabiraman, D. R., and Weinberg, R. A. (2014). Tackling the cancer stem cells – what challenges do they pose? Nat. Rev. Drug Discov. 13, 497–512. doi: 10.1038/nrd4253
Pavlides, S., Whitaker-Menezes, D., Castello-Cros, R., Flomenberg, N., Witkiewicz, A. K., Frank, P. G., et al. (2009). The reverse warburg effect: aerobic glycolysis in cancer associated fibroblasts and the tumor stroma. Cell Cycle 8, 3984–4001. doi: 10.4161/cc.8.23.10238
Peired, A. J., Sisti, A., and Romagnani, P. (2016). Renal cancer stem cells: characterization and targeted therapies. Stem Cells Int. 2016:8342625.
Peiris-Pages, M., Martinez-Outschoorn, U. E., Pestell, R. G., Sotgia, F., and Lisanti, M. P. (2016). Cancer stem cell metabolism. Breast Cancer Res. 18:55.
Peitzsch, C., Nathansen, J., Schniewind, S. I., Schwarz, F., and Dubrovska, A. (2019). Cancer stem cells in head and neck squamous cell carcinoma: identification, characterization and clinical implications. Cancers (Basel) 11:616. doi: 10.3390/cancers11050616
Peltanova, B., Raudenska, M., and Masarik, M. (2019). Effect of tumor microenvironment on pathogenesis of the head and neck squamous cell carcinoma: a systematic review. Mol. Cancer 18:63.
Poli, V., Fagnocchi, L., and Zippo, A. (2018). Tumorigenic cell reprogramming and cancer plasticity: interplay between signaling, microenvironment, and epigenetics. Stem Cells Int. 2018:4598195.
Prager, B. C., Xie, Q., Bao, S., and Rich, J. N. (2019). Cancer stem cells: the architects of the tumor ecosystem. Cell Stem Cell 24, 41–53. doi: 10.1016/j.stem.2018.12.009
Prasetyanti, P. R., and Medema, J. P. (2017). Intra-tumor heterogeneity from a cancer stem cell perspective. Mol. Cancer 16:41.
Prince, M. E., Sivanandan, R., Kaczorowski, A., Wolf, G. T., Kaplan, M. J., and Dalerba, P. (2007). Identification of a subpopulation of cells with cancer stem cell properties in head and neck squamous cell carcinoma. Proc. Natl. Acad. Sci. U.S.A. 104, 973–978. doi: 10.1073/pnas.0610117104
Puram, S. V., Parikh, A. S., and Tirosh, I. (2018). Single cell RNA-seq highlights a role for a partial EMT in head and neck cancer. Mol. Cell Oncol. 5:e1448244.
Puram, S. V., Tirosh, I., Parikh, A. S., Patel, A. P., Yizhak, K., Gillespie, S., et al. (2017). Single-cell transcriptomic analysis of primary and metastatic tumor ecosystems in head and neck cancer. Cell 171, 1611–1624.e24. doi: 10.1016/j.cell.2017.10.044
Qiu, Y., Wang, L., Zhong, X., Li, L., Chen, F., Xiao, L., et al. (2019). A multiple breast cancer stem cell model to predict recurrence of T1-3, N0 Breast Cancer. BMC Cancer 19:729. doi: 10.1186/s12885-019-5941-5
Quail, D. F., and Joyce, J. A. (2013). Microenvironmental regulation of tumor progression and metastasis. Nat. Med. 19, 1423–1437. doi: 10.1038/nm.3394
Quintana, E., Shackleton, M., Foster, H. R., Fullen, D. R., Sabel, M. S., Johnson, T. M., et al. (2010). Phenotypic heterogeneity among tumorigenic melanoma cells from patients that is reversible and not hierarchically organized. Cancer Cell 18, 510–523. doi: 10.1016/j.ccr.2010.10.012
Rheinbay, E., Suva, M. L., Gillespie, S. M., Wakimoto, H., Patel, A. P., Shahid, M., et al. (2013). An aberrant transcription factor network essential for Wnt signaling and stem cell maintenance in glioblastoma. Cell Rep. 3, 1567–1579. doi: 10.1016/j.celrep.2013.04.021
Ricci-Vitiani, L., Lombardi, D. G., Pilozzi, E., Biffoni, M., Todaro, M., Peschle, C., et al. (2007). Identification and expansion of human colon-cancer-initiating cells. Nature 445, 111–115. doi: 10.1038/nature05384
Rich, J. N. (2016). Cancer stem cells: understanding tumor hierarchy and heterogeneity. Medicine (Baltimore) 95(1 Suppl. 1), S2–S7.
Rios, A. C., Capaldo, B. D., Vaillant, F., Pal, B., van Ineveld, R., Dawson, C. A., et al. (2019). Intraclonal plasticity in mammary tumors revealed through large-scale single-cell resolution 3d imaging. Cancer Cell 35, 618–632.e6. doi: 10.1016/j.ccell.2019.02.010
Roesch, A., Fukunaga-Kalabis, M., Schmidt, E. C., Zabierowski, S. E., Brafford, P. A., Vultur, A., et al. (2010). A temporarily distinct subpopulation of slow-cycling melanoma cells is required for continuous tumor growth. Cell 141, 583–594. doi: 10.1016/j.cell.2010.04.020
Roy, L., and Cowden Dahl, K. D. (2018). Can stemness and chemoresistance be therapeutically targeted via signaling pathways in ovarian cancer? Cancers (Basel) 10:241. doi: 10.3390/cancers10080241
Roy Choudhury, A., Gupta, S., Chaturvedi, P. K., Kumar, N., and Pandey, D. (2019). Mechanobiology of cancer stem cells and their niche. Cancer Microenviron. 12, 17–27. doi: 10.1007/s12307-019-00222-4
Saliba, A. E., Saias, L., Psychari, E., Minc, N., Simon, D., Bidard, F. C., et al. (2010). Microfluidic sorting and multimodal typing of cancer cells in self-assembled magnetic arrays. Proc. Natl. Acad. Sci. U.S.A. 107, 14524–14529. doi: 10.1073/pnas.1001515107
Sancho, P., Barneda, D., and Heeschen, C. (2016). Hallmarks of cancer stem cell metabolism. Br. J. Cancer 114, 1305–1312. doi: 10.1038/bjc.2016.152
Sancho, P., Burgos-Ramos, E., Tavera, A., Bou Kheir, T., Jagust, P., Schoenhals, M., et al. (2015). MYC/PGC-1alpha balance determines the metabolic phenotype and plasticity of pancreatic cancer stem cells. Cell Metab. 22, 590–605. doi: 10.1016/j.cmet.2015.08.015
Santoro, A., Vlachou, T., Luzi, L., Melloni, G., Mazzarella, L., D’Elia, E., et al. (2019). p53 loss in breast cancer leads to Myc activation, increased cell plasticity, and expression of a mitotic signature with prognostic Value. Cell Rep. 26, 624–638.e8. doi: 10.1016/j.celrep.2018.12.071
Satija, R., Farrell, J. A., Gennert, D., Schier, A. F., and Regev, A. (2015). Spatial reconstruction of single-cell gene expression data. Nat. Biotechnol. 33, 495–502. doi: 10.1038/nbt.3192
Saxena, K., and Jolly, M. K. (2019). Acute vs. chronic vs. cyclic hypoxia: their differential dynamics, molecular mechanisms, and effects on tumor progression. Biomolecules 9:339. doi: 10.3390/biom9080339
Schmalhofer, O., Brabletz, S., and Brabletz, T. (2009). E-cadherin, beta-catenin, and ZEB1 in malignant progression of cancer. Cancer Metastasis Rev. 28, 151–166. doi: 10.1007/s10555-008-9179-y
Sehl, M. E., and Wicha, M. S. (2018). Modeling of interactions between cancer stem cells and their microenvironment: predicting clinical response. Methods Mol. Biol. 1711, 333–349. doi: 10.1007/978-1-4939-7493-1_16
Sellerio, A. L., Ciusani, E., Ben-Moshe, N. B., Coco, S., Piccinini, A., Myers, C. R., et al. (2015). Overshoot during phenotypic switching of cancer cell populations. Sci. Rep. 5:15464.
Shackleton, M., Quintana, E., Fearon, E. R., and Morrison, S. J. (2009). Heterogeneity in cancer: cancer stem cells versus clonal evolution. Cell 138, 822–829. doi: 10.1016/j.cell.2009.08.017
Shah, M., Cardenas, R., Wang, B., Persson, J., Mongan, N. P., Grabowska, A., et al. (2017). HOXC8 regulates self-renewal, differentiation and transformation of breast cancer stem cells. Mol. Cancer 16:38.
Sharma, A., Cao, E. Y., Kumar, V., Zhang, X., Leong, H. S., Wong, A. M. L., et al. (2018). Longitudinal single-cell RNA sequencing of patient-derived primary cells reveals drug-induced infidelity in stem cell hierarchy. Nat. Commun. 9:4931.
Shibue, T., and Weinberg, R. A. (2017). EMT, CSCs, and drug resistance: the mechanistic link and clinical implications. Nat. Rev. Clin. Oncol. 14, 611–629. doi: 10.1038/nrclinonc.2017.44
Singh, S. K., Hawkins, C., Clarke, D., Squire, J. A., Bayani, J., Hide, T., et al. (2004). Identification of human brain tumour initiating cells. Nature 432, 396–401.
Singla, M., Kumar, A., Bal, A., Sarkar, S., and Bhattacharyya, S. (2018). Epithelial to mesenchymal transition induces stem cell like phenotype in renal cell carcinoma cells. Cancer Cell Int. 18:57.
Skrtic, M., Sriskanthadevan, S., Jhas, B., Gebbia, M., Wang, X., Wang, Z., et al. (2011). Inhibition of mitochondrial translation as a therapeutic strategy for human acute myeloid leukemia. Cancer Cell 20, 674–688.
Skvortsov, S., Skvortsova, I. I., Tang, D. G., and Dubrovska, A. (2018). Concise review: prostate cancer stem cells: current understanding. Stem Cells 36, 1457–1474. doi: 10.1002/stem.2859
Skylaki, S., Hilsenbeck, O., and Schroeder, T. (2016). Challenges in long-term imaging and quantification of single-cell dynamics. Nat. Biotechnol. 34, 1137–1144. doi: 10.1038/nbt.3713
Snyder, V., Reed-Newman, T. C., Arnold, L., Thomas, S. M., and Anant, S. (2018). Cancer stem cell metabolism and potential therapeutic targets. Front. Oncol. 8:203. doi: 10.3389/fonc.2018.00203
Somasundaram, R., Villanueva, J., and Herlyn, M. (2012). Intratumoral heterogeneity as a therapy resistance mechanism: role of melanoma subpopulations. Adv. Pharmacol. 65, 335–359. doi: 10.1016/b978-0-12-397927-8.00011-7
Son, M. J., Woolard, K., Nam, D. H., Lee, J., and Fine, H. A. (2009). SSEA-1 is an enrichment marker for tumor-initiating cells in human glioblastoma. Cell Stem Cell 4, 440–452. doi: 10.1016/j.stem.2009.03.003
Srinivasan, S., Ashok, V., Mohanty, S., Das, A., Das, S., Kumar, S., et al. (2017). Blockade of Rho-associated protein kinase (ROCK) inhibits the contractility and invasion potential of cancer stem like cells. Oncotarget 8, 21418–21428.
Suhail, Y., Cain, M. P., Vanaja, K., Kurywchak, P. A., Levchenko, A., Kalluri, R., et al. (2019). Systems biology of cancer metastasis. Cell Syst. 9, 109–127.
Sun, J., Luo, Q., Liu, L., Zhang, B., Shi, Y., Ju, Y., et al. (2016). Biomechanical profile of cancer stem-like cells derived from MHCC97H cell lines. J. Biomech. 49, 45–52. doi: 10.1016/j.jbiomech.2015.11.007
Suva, M. L., Rheinbay, E., Gillespie, S. M., Patel, A. P., Wakimoto, H., Rabkin, S. D., et al. (2014). Reconstructing and reprogramming the tumor-propagating potential of glioblastoma stem-like cells. Cell 157, 580–594. doi: 10.1016/j.cell.2014.02.030
Suva, M. L., and Tirosh, I. (2019). Single-cell RNA sequencing in cancer: lessons learned and emerging challenges. Mol. Cell 75, 7–12. doi: 10.1016/j.molcel.2019.05.003
Swaminathan, V., Mythreye, K., O’Brien, E. T., Berchuck, A., Blobe, G. C., and Superfine, R. (2011). Mechanical stiffness grades metastatic potential in patient tumor cells and in cancer cell lines. Cancer Res. 71, 5075–5080. doi: 10.1158/0008-5472.can-11-0247
Swartz, M. A., Iida, N., Roberts, E. W., Sangaletti, S., Wong, M. H., Yull, F. E., et al. (2012). Tumor microenvironment complexity: emerging roles in cancer therapy. Cancer Res. 72, 2473–2480. doi: 10.1158/0008-5472.can-12-0122
Tang, D. G. (2012). Understanding cancer stem cell heterogeneity and plasticity. Cell Res. 22, 457–472. doi: 10.1038/cr.2012.13
Terris, B., Cavard, C., and Perret, C. (2010). EpCAM, a new marker for cancer stem cells in hepatocellular carcinoma. J. Hepatol. 52, 280–281. doi: 10.1016/j.jhep.2009.10.026
Tripathi, S. C., Fahrmann, J. F., Celiktas, M., Aguilar, M., Marini, K. D., Jolly, M. K., et al. (2017). MCAM mediates chemoresistance in small-cell lung cancer via the PI3K/AKT/SOX2 signaling pathway. Cancer Res. 77, 4414–4425. doi: 10.1158/0008-5472.can-16-2874
Tschaharganeh, D. F., Xue, W., Calvisi, D. F., Evert, M., Michurina, T. V., Dow, L. E., et al. (2014). p53-dependent Nestin regulation links tumor suppression to cellular plasticity in liver cancer. Cell 158, 579–592. doi: 10.1016/j.cell.2014.05.051
Turashvili, G., and Brogi, E. (2017). Tumor heterogeneity in breast cancer. Front. Med. (Lausanne) 4:227. doi: 10.3389/fmed.2017.00227
Vermeulen, L., de Sousa e Melo, F., Richel, D. J., and Medema, J. P. (2012). The developing cancer stem-cell model: clinical challenges and opportunities. Lancet Oncol. 13, e83–e89. doi: 10.1016/s1470-2045(11)70257-1
Wainwright, E. N., and Scaffidi, P. (2017). Epigenetics and cancer stem cells: unleashing, hijacking, and restricting cellular plasticity. Trends Cancer 3, 372–386. doi: 10.1016/j.trecan.2017.04.004
Wang, J., Sakariassen, P. O., Tsinkalovsky, O., Immervoll, H., Boe, S. O., Svendsen, A., et al. (2008). CD133 negative glioma cells form tumors in nude rats and give rise to CD133 positive cells. Int. J. Cancer 122, 761–768. doi: 10.1002/ijc.23130
Wang, K., and Sun, D. (2018). Cancer stem cells of hepatocellular carcinoma. Oncotarget 9, 23306–23314.
Wang, X., Huang, S., and Chen, J. L. (2017). Understanding of leukemic stem cells and their clinical implications. Mol. Cancer 16:2.
Warburg, O., Wind, F., and Negelein, E. (1927). The metabolism of tumors in the body. J. Gen. Physiol. 8, 519–530. doi: 10.1085/jgp.8.6.519
Wen, Y., Cai, J., Hou, Y., Huang, Z., and Wang, Z. (2017). Role of EZH2 in cancer stem cells: from biological insight to a therapeutic target. Oncotarget 8, 37974–37990.
Wolfson, B., Eades, G., and Zhou, Q. (2015). Adipocyte activation of cancer stem cell signaling in breast cancer. World J. Biol. Chem. 6, 39–47.
Yamaguchi, H., and Hung, M. C. (2014). Regulation and role of EZH2 in cancer. Cancer Res. Treat. 46, 209–222. doi: 10.4143/crt.2014.46.3.209
Yang, Y. C., Wang, S. W., Hung, H. Y., Chang, C. C., Wu, C., Huang, Y. L., et al. (2007). Isolation and characterization of human gastric cell lines with stem cell phenotypes. J. Gastroenterol. Hepatol. 22, 1460–1468.
Yu, L., Lu, M., Jia, D., Ma, J., Ben-Jacob, E., Levine, H., et al. (2017). Modeling the genetic regulation of cancer metabolism: interplay between glycolysis and oxidative phosphorylation. Cancer Res. 77, 1564–1574. doi: 10.1158/0008-5472.can-16-2074
Yuan, G. C., Cai, L., Elowitz, M., Enver, T., Fan, G., Guo, G., et al. (2017). Challenges and emerging directions in single-cell analysis. Genome Biol. 18:84.
Yuan, Y. (2016). Spatial heterogeneity in the tumor microenvironment. Cold Spring Harb. Perspect. Med. 6:a026583. doi: 10.1101/cshperspect.a026583
Zanotelli, M. R., Goldblatt, Z. E., Miller, J. P., Bordeleau, F., Li, J., VanderBurgh, J. A., et al. (2018). Regulation of ATP utilization during metastatic cell migration by collagen architecture. Mol. Biol. Cell 29, 1–9. doi: 10.1091/mbc.e17-01-0041
Zhang, S., Balch, C., Chan, M. W., Lai, H. C., Matei, D., Schilder, J. M., et al. (2008). Identification and characterization of ovarian cancer-initiating cells from primary human tumors. Cancer Res. 68, 4311–4320. doi: 10.1158/0008-5472.can-08-0364
Zhang, W., Kai, K., Choi, D. S., Iwamoto, T., Nguyen, Y. H., Wong, H., et al. (2012). Microfluidics separation reveals the stem-cell-like deformability of tumor-initiating cells. Proc. Natl. Acad. Sci. U.S.A. 109, 18707–18712. doi: 10.1073/pnas.1209893109
Zhang, Y., Sun, B., Zhao, X., Liu, Z., Wang, X., Yao, X., et al. (2013). Clinical significances and prognostic value of cancer stem-like cells markers and vasculogenic mimicry in renal cell carcinoma. J. Surg. Oncol. 108, 414–419. doi: 10.1002/jso.23402
Zhang, Y., Wu, M., Han, X., Wang, P., and Qin, L. (2015). High-throughput, label-free isolation of cancer stem cells on the basis of cell adhesion capacity. Angew. Chem. Int. Ed. Engl. 54, 10838–10842. doi: 10.1002/anie.201505294
Zheng, H., Pomyen, Y., Hernandez, M. O., Li, C., Livak, F., Tang, W., et al. (2018). Single-cell analysis reveals cancer stem cell heterogeneity in hepatocellular carcinoma. Hepatology 68, 127–140. doi: 10.1002/hep.29778
Zhou, Y., Xia, L., Wang, H., Oyang, L., Su, M., Liu, Q., et al. (2018). Cancer stem cells in progression of colorectal cancer. Oncotarget 9, 33403–33415.
Keywords: cancer stem cells, plasticity, epithelial-mesenchymal transition, metastasis, microenvironment, metabolic plasticity
Citation: Thankamony AP, Saxena K, Murali R, Jolly MK and Nair R (2020) Cancer Stem Cell Plasticity – A Deadly Deal. Front. Mol. Biosci. 7:79. doi: 10.3389/fmolb.2020.00079
Received: 07 January 2020; Accepted: 06 April 2020;
Published: 30 April 2020.
Edited by:
Yong Teng, Augusta University, United StatesReviewed by:
Aamir Ahmad, Mitchell Cancer Institute, United StatesMonica Venere, The Ohio State University, United States
Copyright © 2020 Thankamony, Saxena, Murali, Jolly and Nair. This is an open-access article distributed under the terms of the Creative Commons Attribution License (CC BY). The use, distribution or reproduction in other forums is permitted, provided the original author(s) and the copyright owner(s) are credited and that the original publication in this journal is cited, in accordance with accepted academic practice. No use, distribution or reproduction is permitted which does not comply with these terms.
*Correspondence: Radhika Nair, cmFkaGlrYW5haXJAcmdjYi5yZXMuaW4=; Mohit Kumar Jolly; bWtqb2xseUBpaXNjLmFjLmlu
†These authors share first authorship