- 1Instituto de Biotecnología, Universidad Nacional Autónoma de México, Cuernavaca, Mexico
- 2CONACYT-Laboratorio de Biotecnología Acuícola, Instituto de Investigaciones Agropecuarias y Forestales, Universidad Michoacana de San Nicolás de Hidalgo, Morelia, Mexico
- 3Centro de Investigación Sobre Enfermedades Infecciosas, Departamento de Bioinformática en Enfermedades Infecciosas, Instituto Nacional de Salud Pública, Cuernavaca, Mexico
- 4Laboratorio de Inmunología Molecular y Biotoxinas, Departamento de Innovación Biomedica, CICESE, Ensenada, Mexico
The Gulf of Mexico (GoM) is a particular environment that is continuously exposed to hydrocarbon compounds that may influence the microbial community composition. We carried out a metagenomic assessment of the bacterial community to get an overall view of this geographical zone. We analyzed both taxonomic and metabolic markers profiles to explain how the indigenous GoM microorganims participate in the biogeochemical cycling. Two geographically distant regions in the GoM, one in the north-west (NW) and one in the south-east (SE) of the GoM were analyzed and showed differences in their microbial composition and metabolic potential. These differences provide evidence the delicate equilibrium that sustains microbial communities and biogeochemical cycles. Based on the taxonomy and gene groups, the NW are more oxic sediments than SE ones, which have anaerobic conditions. Both water and sediments show the expected sulfur, nitrogen, and hydrocarbon metabolism genes, with particularly high diversity of the hydrocarbon-degrading ones. Accordingly, many of the assigned genera were associated with hydrocarbon degradation processes, Nitrospira and Sva0081 were the most abundant in sediments, while Vibrio, Alteromonas, and Alcanivorax were mostly detected in water samples. This basal-state analysis presents the GoM as a potential source of aerobic and anaerobic hydrocarbon degradation genes important for the ecological dynamics of hydrocarbons and the potential use for water and sediment bioremediation processes.
Introduction
The Gulf of Mexico (GoM) is a basin with one of the most significant oil reservoirs in the world. There are few microbial diversity studies in Mexican waters of the GoM (Lizarrága-Partida, 1986; Rosano-Hernández et al., 2012; Sanchez-Soto et al., 2018; Ramírez et al., 2020) in contrast to an extensive effort along the northeastern coast, particularly related to the Deep Water Horizon oil spill (Kostka et al., 2011; Liu et al., 2012; Mason et al., 2014). Worldwide, oceans’ microbiology has been studied principally throughout the water-column and hot-spots (i.e., seeps, hydrothermal vents, oil discharge sites, etc.). There are very few metagenomic studies from typical “desert-like” sediments, as the latest endeavors to cover all the oceans (Zinger et al., 2011; Bienhold et al., 2016), and whole Earth (Thompson et al., 2017).
The way to study, analyze, interpret, and present microbial diversity data is still quite complex. With the popularization of metagenomics with new generation sequencing techniques, hundreds of studies are carried out yearly, and several methods to report microbial diversity are currently in use. Computational strategies are being developed to try to disentangle microbial richness and functional data, the most used nowadays is statistical correlation analyses. However, network-based modeling methods and the integration of several distinct approaches, could permit a more comprehensive understanding of microbial ecology (Chong and Xia, 2017). The analysis or simulation of functional traits linked directly to microbial richness has seldom been done. Commonly, microbial taxon structure (microbial community structure) and functional traits or interactions (functional dynamics) are analyzed independently. One of the first efforts to integrate richness and function is shown in Delmont et al. (2018); where the importance of Planctomycetes within the essential nitrogen-fixing microbial population through both a phylogenetic and a functional network analysis was demonstrated.
Biogeochemical cycles in sediments, water and air are influenced by microbial metabolism. Also, climate, currents, rivers, faults, hydrocarbon emanations, among other natural processes, and pollution or other anthropogenic activities, change or disrupt microbial profiles and therefore their role in the cycles. The GoM is known for having different depositional environments in which the accumulation of sediments ranges widely (Davis, 2017). In many of these locations, microorganisms reduce sulfate ions to hydrogen sulfide, which is an essential energy source for many free living and symbiotic organisms. The sedimentary depositional process known as diagenesis is the source of organic matter that is oxidized through the sulfate reduction process in sediments (Kasten and Jørgensen, 2000).
Genes involved in dissimilatory sulfate reduction (DSR) such as sat (sulfate adenylyltransferase), aprA (adenylylsulfate reductase), and dsrAB [dissimilatory (bi) sulfate reductase], a key enzyme in the biogeochemical sulfur cycle, have been used as marker genes for phylogenetic affiliation of the different taxonomic groups that participate in the sulfur cycle. Although sulfate oxidation lacks a preserved metabolic pathway like DSR, soxB (S-sulfosulfanyl-L-cysteine sulfohydrolase) has been used as an excellent functional gene marker for sulfur oxidation in different studies (Meyer and Kuever, 2007a; Hügler et al., 2010; Jaffer et al., 2019), and aprA and dsrA have previously been used as functional maker genes for sulfate-reducing but also sulfur-oxidizing microorganisms (Meyer and Kuever, 2007b).
Markers for nitrate reduction, denitrification and dissimilatory nitrate reduction to ammonium (DNRA) have been used to study the community diversity of environmental samples genes (Braker et al., 2000). narG gene codes for membrane-bound nitrate reductase, napA gene for periplasmic nitrate reductase, both catalyzing the reduction of nitrate to nitrite (Moreno-Vivián et al., 1999). nirS, nirK (haem-cytochrome cd-1 type and copper-containing type nitrite reductases), norB (nitric oxide reductase) and nosZ (nitrous oxide reductase), are four markers that have been used to detect communities of denitrifying bacteria (Braker et al., 1998; Smith et al., 2007). nrfA gene has been used as a marker for nitrite reductase in DNRA, catalyzing the six-electron reduction of nitrite to ammonium (Stach et al., 2000; Klotz et al., 2008; Kern et al., 2011), coupled to oxidation of electron donors, such as organic matter, ferrous iron, hydrogen, sulfide, and methane (Burgin and Hamilton, 2007). Anammox bacteria extract energy by producing molecular nitrogen from ammonium and nitric oxide. Previous studies searching for environmental diversity of anammox bacteria employed the genes hzsA coding for hydrazine synthase (Harhangi et al., 2012) and the gene hdh or hdo coding for hydrazine dehydrogenase (Kong et al., 2013).
GoM is exposed to a continuous hydrocarbon flux through natural seeps, independently of oil-spills, therefore, its bacterial community must be adapted to oil and degradation processes. Oil from natural leaks concentrate has been regionally concentrated in the GoM, and 68, 25, 7, and <1% of the total has been detected in the NW, SW, NE, and SE gulf regions, respectively (Macdonald et al., 2015). Hydrocarbon degraders are active and blooming whenever an oil-spill occurs, then hydrocarbon degradation genes must be present within indigenous bacterial communities. The first step, in both aerobic and anaerobic hydrocarbon degradation, involves chemical activation, and subsequently, the compound is channeled into central metabolism (Fuchs et al., 2011; Lueders and Von Netzer, 2014). To explore hydrocarbon degradation gene families associated to the activation in aerobic degradation of alkanes have been used as are the alkane hydroxylases alkB and CYP153 (Nie et al., 2014), and for aerobic degradation of aromatic compounds the Rieske non-heme iron dependent oxygenases (Duarte et al., 2014). For the anaerobic degradation of hydrocarbons bssA benzyl succinate like synthases (Callaghan et al., 2010) between others have been used as markers.
We have previously reported the microbial diversity found in southwestern GoM sediments (Godoy-Lozano et al., 2018). In this study, we continue to investigate this geographical zone, in particular the Campeche Knolls, and also the Perdido Fold Belt region off the coast of Tamaulipas (NW). In these two areas, natural oil seeps have been detected (Macdonald et al., 2015) and particular oil-adapted bacterial communities are expected. We carried out a metagenomic assessment of the bacterial community in water column and sediment, and searched for metabolic marker genes of carbon, nitrogen, sulfur and hydrocarbon metabolism to set these GoM regions in a biogeochemical context.
Materials and Methods
Sample Collection and Processing
Water and sediments from 19 locations at the GoM were collected in March 2016. Two longitudinal transects corresponding to the Perdido region, and two latitudinal transects corresponding to a southern region in Campeche Knolls (Figure 1 and Supplementary Table S2) were sampled. Seawater was collected using a rosette with 20-l Niskin bottles at four depths: maximum fluorescence (MAX), minimum oxygen zone (MIN), 1,000 m depth at the Antarctic Intermediate Water (AAIW), that assures a good comparison because it is a well-oxygenated water, and bottom water (DEEP), that varied between 550 and 3,200 m depth. A total of 57 water samples were collected and analyzed: 14 MAX, 17 MIN, 9 of AAIW (1,000 m), and 17 DEEP samples. Each seawater 100 L sample was concentrated to approximately 3 L with a tangential filtration system (KrosFlo; mean/rating: mPES7100 kD; surface area: 2.55 m2). From this volume, 100 mL were stored at 4°C for filtration through a Sterivex filter or passed through a 0.22 μm polycarbonate membrane (Merck Millipore). Immediately afterward, the filters were stored in liquid N2. Sediment was brought to the ship surface with a box corer, and then with a manual tube core, a sample of the first 10 cm was collected (SED). An extra sample (E03) was taken in a pre-expedition in September 2015. All samples were kept frozen at −20°C until processed.
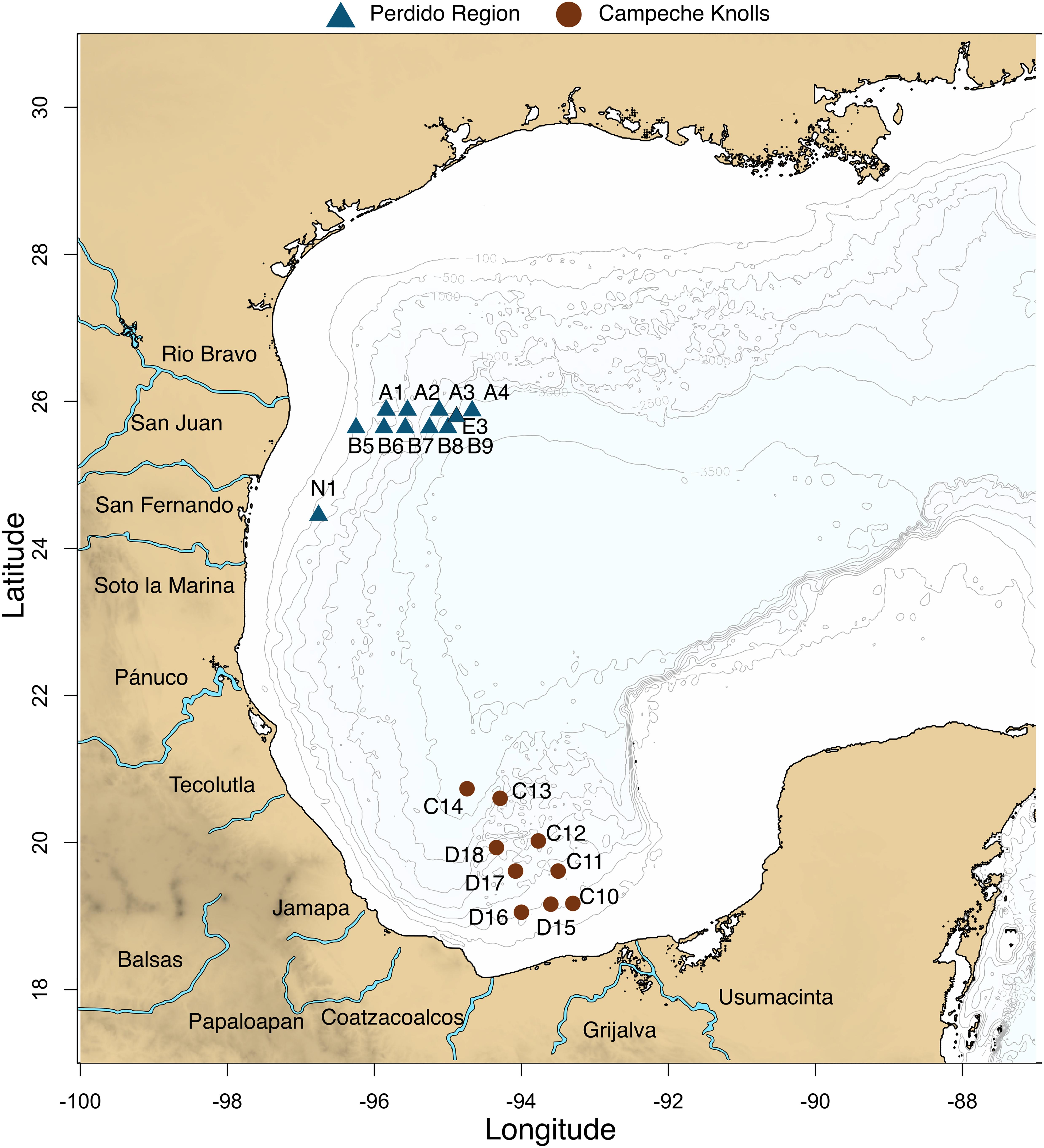
Figure 1. Twenty locations were sampled in Mexican waters from the Gulf of Mexico. Blue triangles represent Perdido region and brown circles Campeche Knolls. Nineteen samples were collected in March 2016. An extra sample (E03) in September 2015.
Physicochemical Parameters
Oxygen, fluorescence, temperature and salinity were measured along the water column with a CTD (SBE-9) coupled to the rosette, and sampling was determined based on these parameters. Oxygen values were between 7.07 and 3.6 mg/L at the Perdido Fold Belt zone, and from 6.9 to 3.5 mg/L in the Campeche Knolls area. The lowest oxygen levels detected were between 320 and 450 m depth (MIN samples). Fluorescence values were between 0.07 and 1.22, being the highest between 50 m and 70 m depth (MAX samples). Temperatures were between 21.7 to 4.3°C and salinity between 34.9 and 36.3 in the Perdido zone and 24.86 to 4.38°C and 34.9 to 36.1 salinity in the Campeche area (Supplementary Table S1). Due to the lack of instrumentation, parameters of the sediments were not measured, we can only extrapolate from measurements performed in Godoy-Lozano et al. (2018) sampling.
Metagenomic DNA Extraction and Sequencing
This study is immersed in a major Mexican project which included studying the diversity of the Gulf of Mexico1. Our goal here was to describe and study the bacterial community. Our choice would have been to cover all sites with shotgun analysis, however, we could not, due to economic constraints and therefore we covered all sites with amplicon metagenomics. Although primers to amplify both bacteria and archaea or simply primers to get the most archaea (Klindworth et al., 2013) are available, we standardized the use of primers throughout the CIGOM consortium, as well as DNA extraction.
DNA extraction was performed from Sterivex or Millipore water samples filters using the Power Water DNA isolation kit (MO BIO-QIAGEN), or from 0.5 g of surface sediment, using the PowerSoil DNA Isolation Kit (MO BIO-QIAGEN). Extracted metagenomic DNA was used to prepare libraries for Illumina sequencing of variable V3–V4 regions from 16S rRNA taxonomic marker gene. Amplification of the V3–V4 region was carried out with primer pair S-D-Bact-0341-b-S-17 and S-D-Bact-0785-a-A-21 (Klindworth et al., 2013), and PCR conditions were 98°-3 min/98°-3 s/50°-1.5 min/72°-1 min/72°-10 min, for 25 cycles with Phusion Polymerase (Thermo Scientific). For library preparation, the Nextera XT DNA Library Prep Kit (Illumina) protocol was used following the Illumina’s protocol. Libraries sequencing was accomplished in an Illumina MiSeq platform with a paired-end reads configuration of 300 cycles by side. Amplicon raw reads are available at the SRA site under project id PRJNA609564 (SAMN14259149-SAMN14259166, SAMN14255300-SAMN14255357), and PRJNA380945 (SAMN06649846).
Additionally, water and sediment samples with the better DNA extraction yield (D18_MAX, A04_MIN, A04_AAIW, A04_SED, E03_SED, D18_SED) were used to perform whole metagenome shotgun sequencing. These libraries were prepared using the TruSeq DNA PCR-Free HT Library Prep (Illumina) kit. Sequencing of paired-end reads was carried out in an Illumina NextSeq500 platform with a configuration of 75 or 150 cycles by side (see Supplementary Table S2. Sample stats, “WMS” sheet). Metagenomic reads were also submitted to SRA under the projects PRJNA609564 (SAMN14329496, SAMN14329497, and SAMN14329491-SAMN14329493) and PRJNA380945 (SAMN06649846).
Bioinformatic Analysis: Amplicon Profiles and Taxonomic Assignment, and Whole Metagenome Shotgun (WMS) Data
16S rRNA amplicon samples were sequenced to obtain a yield of 1-5 e + 05 paired-end reads, from which, the overlapping reads ratio was ∼70–94% (see Supplementary Table S2) using the Flash v1.2.7 software (Magoč and Salzberg, 2011). Merged reads were used for both OTUs clustering and taxonomic annotation analysis. For taxonomic annotation the extended fragments were denoised using DADA2 algorithm with the R Package dada2 (Callahan et al., 2016). Afterward, ASVs (amplicon sequence variants) reads were annotated for taxonomy using the Sklearn tool within the QIIME 2 pipeline (Bolyen et al., 2019) against the SILVA 132 SSU database (Quast et al., 2013).
The extended fragments were clustered in OTUs at 97% identity (OTUs0.03) using VSEARCH tool v2.4.3 (Rognes et al., 2016). To discard artificial diversity, we filtered chimeric sequences, and clusters containing one sequence were discarded. Sampling effort was calculated as well as the Chao1 and Shannon alpha diversity indices with R Phyloseq library (Mcmurdie and Holmes, 2013). Finally, the filtered matrix was normalized using the metagenomeSeq v1.16.0 method (Mcmurdie and Holmes, 2014), and a beta diversity distance matrix was calculated using the Bray–Curtis index.
To perform the metabolic potential prediction of samples sequenced by WMS, we assembled genomic fragments from reads using the IDBA-UD v1.1.1 (Peng et al., 2010) metagenomic assembler. Thereafter, we predicted the coding DNA sequences (CDS) from contigs by MetaGeneMark v3.26 (Zhu et al., 2010), and annotated the translated amino acidic chains by blastp 2.2.31+ (Camacho et al., 2009) against the Swissprot database included in the Trinotate v3.0.1 annotation suite (Bryant et al., 2017). Uniprot annotations were retrieved from the Trinotate output. Gene abundance was estimated as coverage through mapping reads to contigs using BWA v0.7.12-r1039 (Li and Durbin, 2009) and coverageBed function from bedtools v2.25.0 (Quinlan and Hall, 2010).
Networks Analysis
We built a network using the assigned KO number and taxonomic information of the closest Uniprot hit (e-value cutoff 1e-5) from the Trinotate annotation report. Nodes in the network represent either a KO or a genus. Edges represent a found KO-genus pair and edge-width is proportional to the log2 of total gene counts found for that pair. We included selected genes (KO numbers) that are markers for photosynthesis and for hydrocarbon degradation, sulfur metabolism, nitrogen metabolism, and methane metabolism pathways in the final network. Networks were generated with the Cytoscape software v.3.6.1 (Shannon et al., 2003). The network heterogeneity values calculated with Cytoscape are included, as they show the topological feature of complex networks with the degree of interactions. A higher value means a more complex network.
Metabolic Markers Search
WMS analysis revealed important and interesting archaeal genes, thus we decided to include them in the analyses. We searched for homologous sequences of genes related to sulfur (sat, aprA, dsrAB, soxB), nitrogen (nrfA, nosZ, norB, nirS, nirK, nifH, narG, napA, nxrB, hzsA, hdh, hao, amoA), methane (mcrA, mer, fmd, ftr, mch, mtd, mtrA) and hydrocarbon (acsB, alkB, bssA-like, Fe-Fe_Hydrogenases, Ni-Fe_Hydrogenases, Rieske_superfamily genes, rplC and rplE) metabolism using GraftM v0.11.1 (Boyd et al., 2018) through the metagenome assemblies, using an e-value cutoff of 1e-30. The packages of the genes sat, mer, nosZ, nirK, nifH, narG, napA, napA, nxrB, hao, acsB, Fe-Fe_Hydrogenases, Ni-Fe_Hydrogenases, rplC and rplE were retrieved from http://github.com/geronimp/graftm_gpkgs/tree/master/7 (Boyd et al., 2018) through the metagenome assemblies, using an e-value cutoff of 1e-30. The gene packages of aprA, soxB, fmd, ftr, mch, mtd, mtrA, nrfA, norB, nirS, hzsA, and hdh were constructed collecting sequences from Uniprot (Uniprot Consortium, 2018) and using their corresponding NCBI taxonomy. Finally, gene packages of the following genes were manually built based on previous studies as reference for taxonomic annotation: dsrAB (Müller et al., 2015), alkB (Nie et al., 2014), bssA-like (Acosta-González et al., 2013), Rieske_superfamily genes (Duarte et al., 2014), and amoA which included ammonia-oxidizing archaea (AOA) and ammonia-oxidizing bacteria (AOB) markers, plus pmoA (Khadka et al., 2018).
Gene package files constructed in this study can be found at https://github.com/garciafertson/mmf1_gpkgs.git. After homologous sequence identification with GraftM, we calculated the relative abundance for the identified genes in relation to the total number of predicted genes in the sample; thus we extracted each identified sequence and added the sequencing depth value divided by the total number of genes in the metagenome assembly times the metagenome’s average sequencing depth: gene abundance = [sum(detected_gen_sequencing_depth)]/(total_ number_of_genes∗total_sequencing_depth).
Results
Community Distribution by Habitats and Alpha Diversity of Perdido Fold Belt (NW) and Campeche Knolls (SE)
In order to analyze alpha diversity of the different sampling sites, both OTUs0.03 and ASVs were used. The total number of observed OTUs0.03 from both Perdido and Campeche sampling sites varies between 1,589 to 6,170 in the case of the water column samples and from 6,099 to 33,740 in sediment samples. These observed OTUs0.03 represent ∼96% of the maximum OTUs0.03 expected according to the Chao1 index. The Good’s coverage values for these samples are in the range of 0.9096 and 0.9979. We used both ASVs and OTUs to analyze diversity and sample dispersion, however we are aware of the 16S rRNA gene intragenomic heterogeneity which could be reflected in both analysis, with ASVs analysis overestimating, and OTUs clustering at 97% underestimating diversity. However, both calculations were very similar, indicating that both analyses are correct for V3–V4 variable regions. Shannon index for both analyses indicate that sediment samples have greater diversity than water samples, as expected. Within the water samples, the MIN and DEEP samples have higher bacterial diversity than MAX and AAIW (see Supplementary Table S2 for sequencing yields and alpha diversity indices statistics). We generated a Bray–Curtis distance matrix and plotted an NMDS to observe differences in diversity between samples (Figure 2). Sediment samples segregate from the water samples into a single, separated cluster. Among water samples, aphotic layers form a mixed group that includes the MIN, AAIW and DEEP-water layers, and the samples from the MAX layer, form an independent photic cluster (Figure 2A). According to a pairwise ADONIS test, photic and aphotic layers form independent groups that are homogeneous regardless of the geographic origin of the samples (Figure 2B). With respect to sediments we observe a tendency to cluster by water column depth (>800 m or >800 m) independently of their geographical origin, except for sample D18 that does not cluster with any of both groups (Figure 2C).
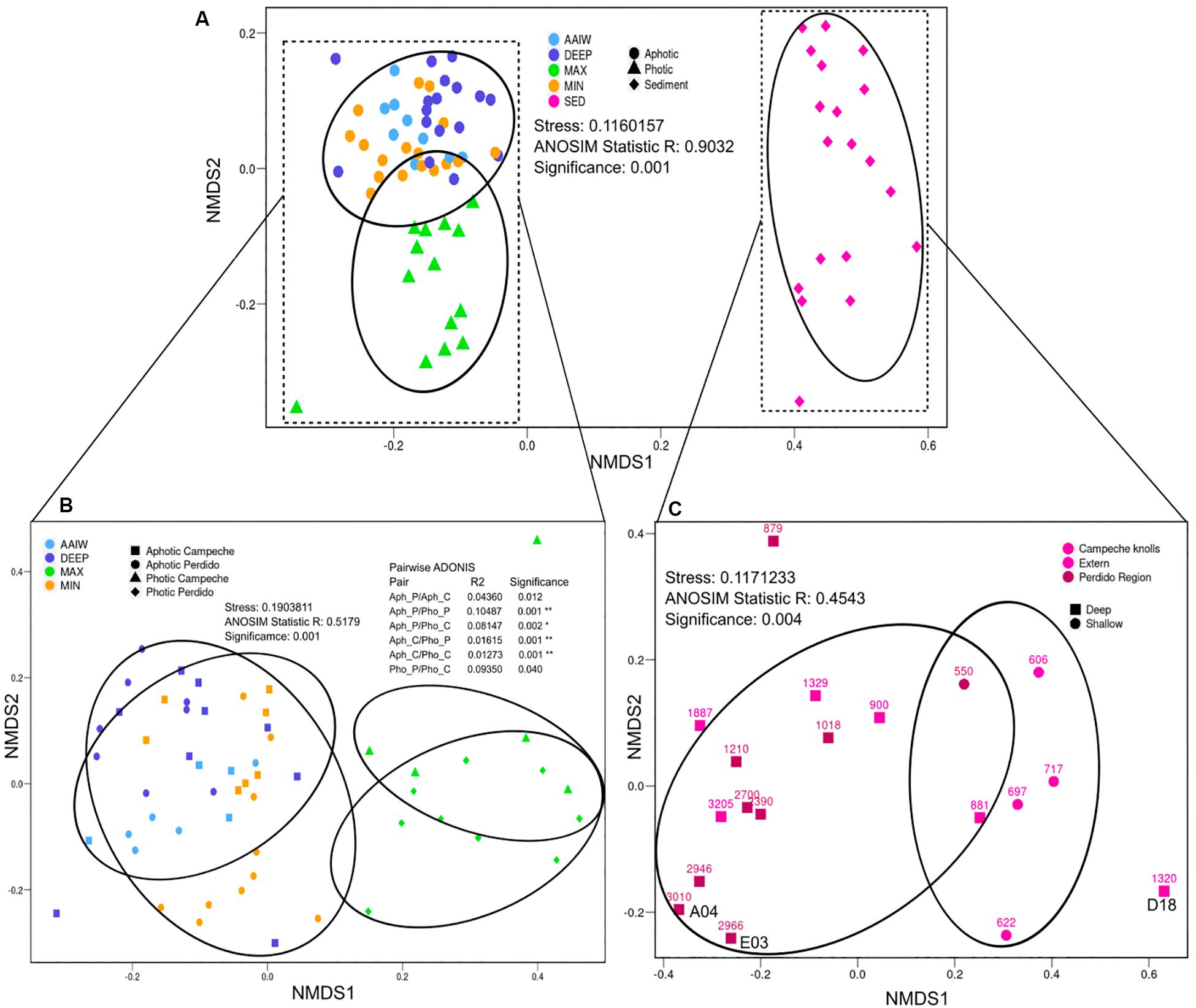
Figure 2. Beta diversity NMDS. Non-metric Multidimensional Scaling plot (NMDS) depicting Bray–Curtis dissimilarity distance for ASVs diversity. (A) All samples where labeled according to the sample type (color) and category (shape). (B) Water where samples labeled according to the sample type (color), and geographical origin with photic or aphotic category (shape). (C) Sediment where samples labeled according to their geographical region (color) and depth (shape), where shallow groups correspond to lower than 800 m. Ellipses represent the area enclosing the group at 90% of confidence. Analysis of ANOSIM and pairwise ADONIS between groups is shown to demonstrate group segregation.
Microbial Community Composition of Water Column and Sediments of Perdido Fold Belt (NW) and Campeche Knolls (SE)
Water Column
The bacterial community composition is structured by depth as each layer has a characteristic diversity (Figure 3). In the maximum fluorescence layer (MAX), Oxyphotobacteria reaches between 8–42% of the relative community abundance, as expected, and with respect to most abundant classes Alphaproteobacteria reach 21–49%, Gammaproteobacteria 4–24%, Acidimicrobiia 4–18% and Bacteroidia 0.7–6.4% (Figure 3). In addition to the well-known genus Synechococcus that reaches up to 25%, abundant genera related to potential hydrocarbon degrading bacteria (PHDB) such as Pseudomonas spp. (6%), Alcanivorax (5.4%), and Alteromonas (4%) among other less abundant genera, are observed.
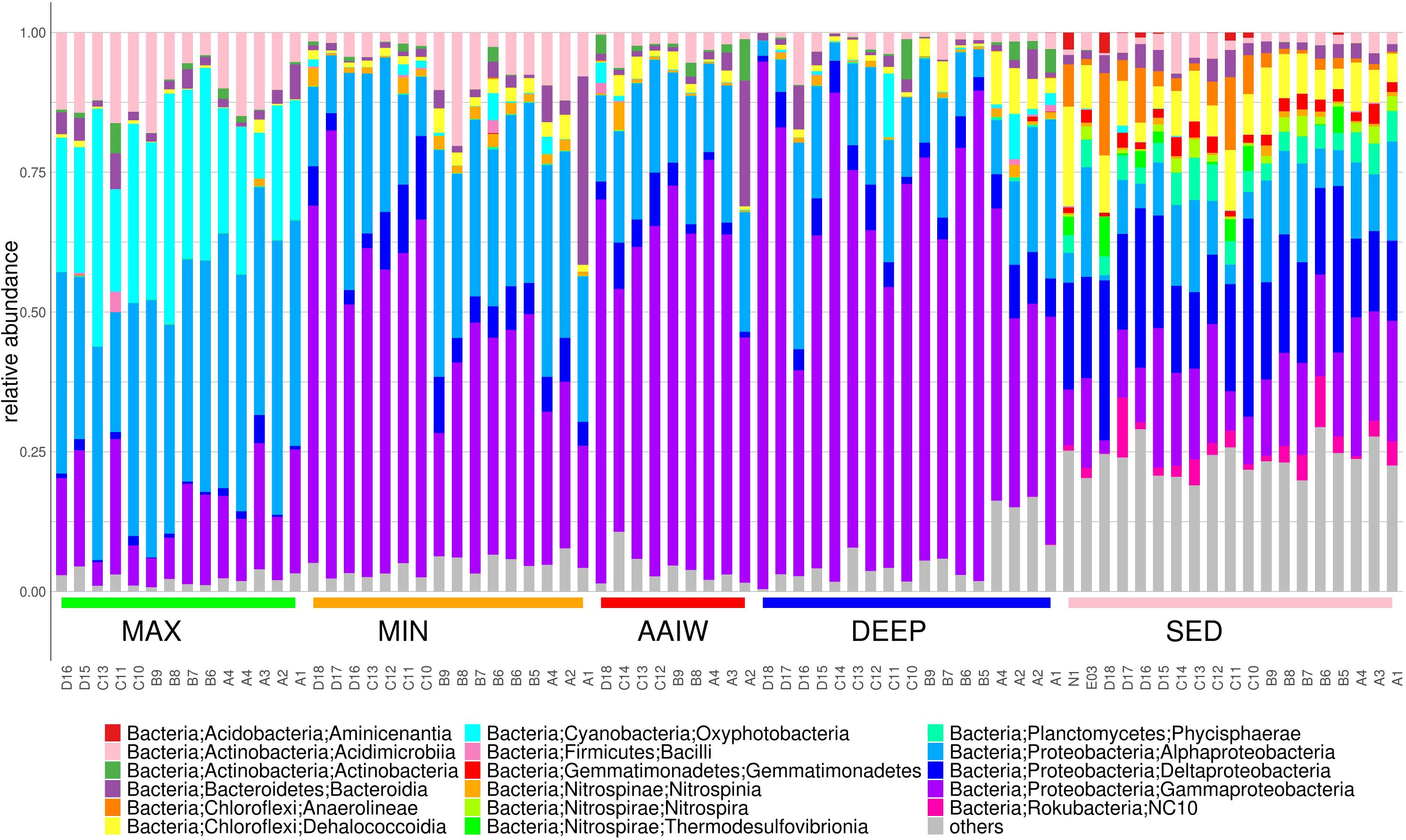
Figure 3. The bar plot displays relative abundances for the 16S rRNA amplicon taxonomic annotations at the Class level. Classes with relative frequencies smaller than 3.5% in every sample were collapsed into the others category.
The next well-separated layer is MIN (between −253 and −456 m depth), where oxygen levels were 0.5 mg/L lower than in the rest of the whole water column; we observed that Alphaproteobacteria decrease 10–40% while Gammaproteobacteria increase substantially (21–80%) in the aphotic layers concerning MAX layer and sediments. PHDB are still abundant, at over 1%, as shown by Halomonas spp. (5-17%), and Alcanivorax spp. (0.1–12%). Other abundant genera at this level are Sva0996 marine group that reaches up to 20%, Methylophaga 11%, Roseovarius 10%, Leeuwenhoekiella 32%, Alteromonas 27%, and Synechococcus CladeIb 11%.
In the next deeper layer, at −1000 m depth (AAIW), Alphaproteobacteria decrease even more (15–24%) and Betaproteobacteria are not detected. Halomonas (1–48%), Alcanivorax (0.1–4.3%) Methylophaga 12% and Sva0996 marine group 6% increase substantially as well as Vibrio that reaches 54%. Contrary, Alteromonas decrease to 1–13% and Leeuwenhoekiella to 22%. Gammaproteobacteria continue to increase and top up 43 and 75% in the DEEP layer.
Sediments
Bacterial communities in sediment samples are dominated by different proportions of Deltaproteobacteria (12–35%), Gammaproteobacteria (2–24%), Alphaproteobacteria (1–20%) and Dehalococcoidia (0.3–17%) (Figure 3). We found that the Deltaproteobacteria class is more predominant in deep sediments. Also genera related to sulfur metabolism and hydrocarbon degraders as Wb1-A12 (0.1–10), Urania-1B-19 (1–6.8), Nitrospira (0.3–3.5%) Sva0081 sediment group (0–4.8). New reclassified NC10 class 0.1–10% and Woeseia (0.7–6.8%) are also present (Du et al., 2016; Bacosa et al., 2018).
Linking Taxonomy With Metabolism Through Networks
We performed a network analysis to link taxonomy with function (Figure 4). The network heterogeneity values for each shotgun network were: D18MAX = 1.102, A04MIL = 1.302, A04SED = 1.627, D18SED = 1.581, E03SED = 1.430. Bacterial or gene “hubs” are shown in the networks. Each hub should be showing a key-role player in the environment (Banerjee et al., 2018). We discarded genes that are redundant in the metabolism, or that take part in many different metabolic pathways. Some genes and some taxa become a hub, either as an “essential metabolic marker gene” or a “keystone taxon,” respectively. Pseudomonas and Acinetobacter are detected as bacterial keystone players in all layers. Synechococcus and Prochlorococcus are keystone players in the MAX layer, as expected. Marker gene K00958 (sulfate adenylyltransferase), a sulfate metabolism gene, is present in all layers as an essential hub in sediments. The K02567 module, a marker for denitrification, is also present as a hub in sediments A04 and D18. The D18 sediment (Campeche region) seems to be more metabolically diverse than A04 and E03, showing more genes for alkanes degradation (K00656, K06281, K06282, K07540), and genes for methanogenesis and methane metabolism (K00441, K00577, K0584, K03390, K08264) (Supplementary Table S3). The K01563 gene (haloalkane dehalogenase) is a hub in all three sediments. Desulfovibrio is found in all networks, representing a sulfate-reducing bacterial module, and this genus is related to the sulfur metabolism marker genes K00394-K00395 (aprA-B, the bidirectional enzyme transforming sulfite to adenylyl sulfate). Bacillus and Escherichia might represent heterotrophic bacteria, and are associated with respiration and nitrate reduction genes (nitrate reductase narG – K00370). Acinetobacter and Pseudomonas represent the PHDB, and the genes associated belong to the hydrocarbon degradation pathways: K01607 (4-carboxymuconolactone decarboxylase PcaC), K01055 (3-oxoadipate enol-lactonase-PcaD) (Supplementary Table S3).
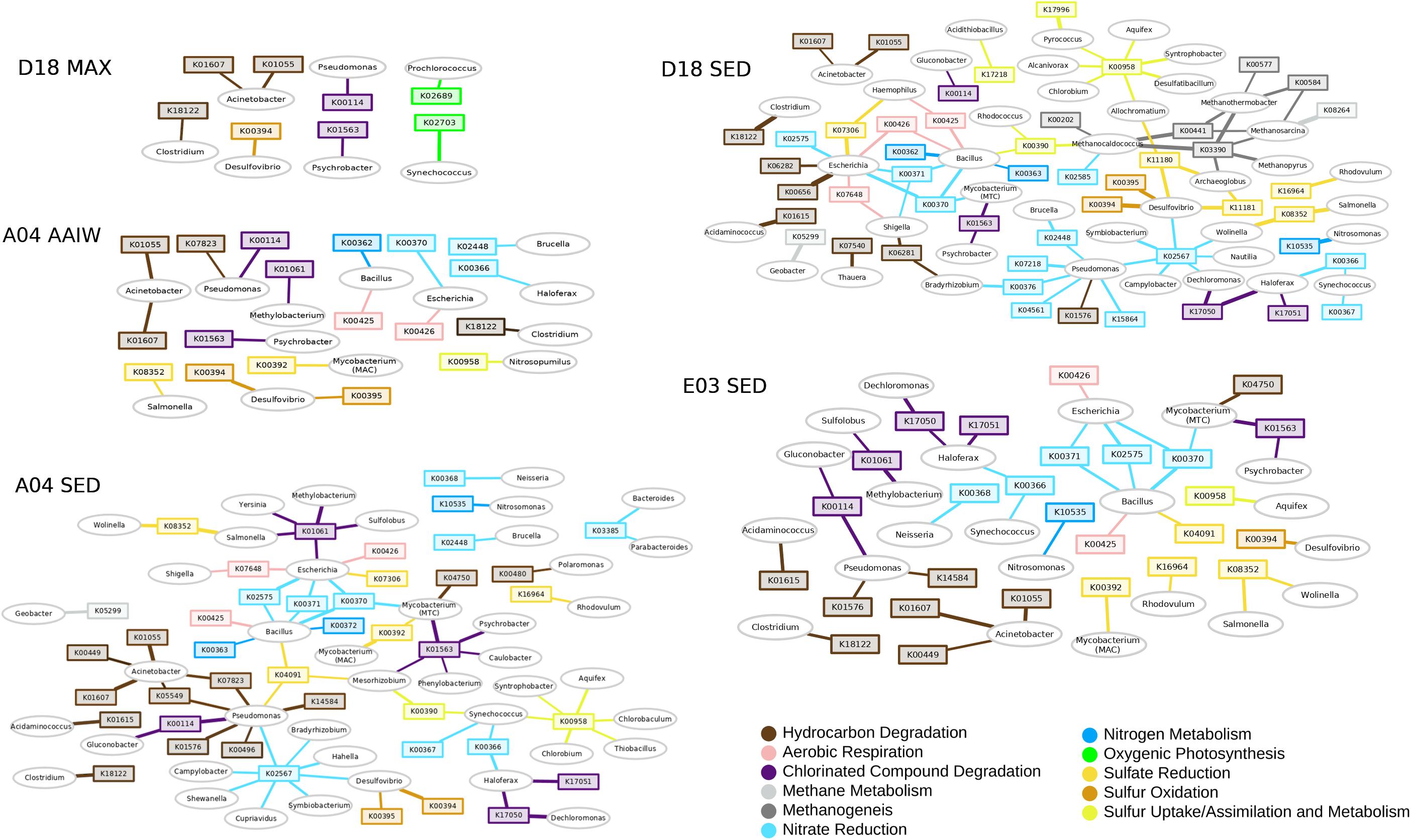
Figure 4. KO and genus networks. Ovals represent genera, squares represent KOs. Edge width is proportional to the logarithm of total counts; only edges and nodes with a total count above 10 are displayed for image clarity. Methane metabolism is shown in light gray and methanogenesis in gray; sulfur oxidation in ocre, sulfate reduction in yellow and sulfur uptake/assimilation in lime; nitrate reduction/denitrification in sky blue and nitrogen metabolism in blue; alkanes/hydrocarbon degradation in dark brown, aromatics degradation in brown and chlorinated compound degradation in purple; oxygenic photosynthesis in green and aerobic respiration in pink (the KO list is included in Supplementary Table S3).
Marker Genes Involved in Anaerobic Metabolic Processes in Sediments
Sulfur Metabolism
The sat, aprA, and dsrA genes, involved in DSR, were detected in the three sediment metagenomes. Their abundance as well as their associated taxonomy show a clear difference between D18 and E03 and A04; genes associated to Deltaproteobacteria (mostly dsrAB) are more abundant in D18 (it shows up as K11181 only in D18 in Figure 4) in comparison to E03 and A04 which show fewer Deltaproteobacteria- and more Alphaproteobacteria-associated genes (Figure 5). We also analyzed sulfur oxidation marker soxB and D18 sediment is clearly different again as indicated by the absence of Alphaproteobacteria-associated genes. We found sat and aprA homologous sequences in all sediment samples with some differences in the number of associated homologs and the taxonomy between samples; E03 and A04 metagenomes are similar, both having a high proportion of sequences associated to Alphaproteobacteria and almost no sequences belonging to Deltaproteobacteria in contrast to D18 where there is a high amount of Deltaproteobacteria and no Alphaproteobacteria-associated genes (Supplementary Figure S1).
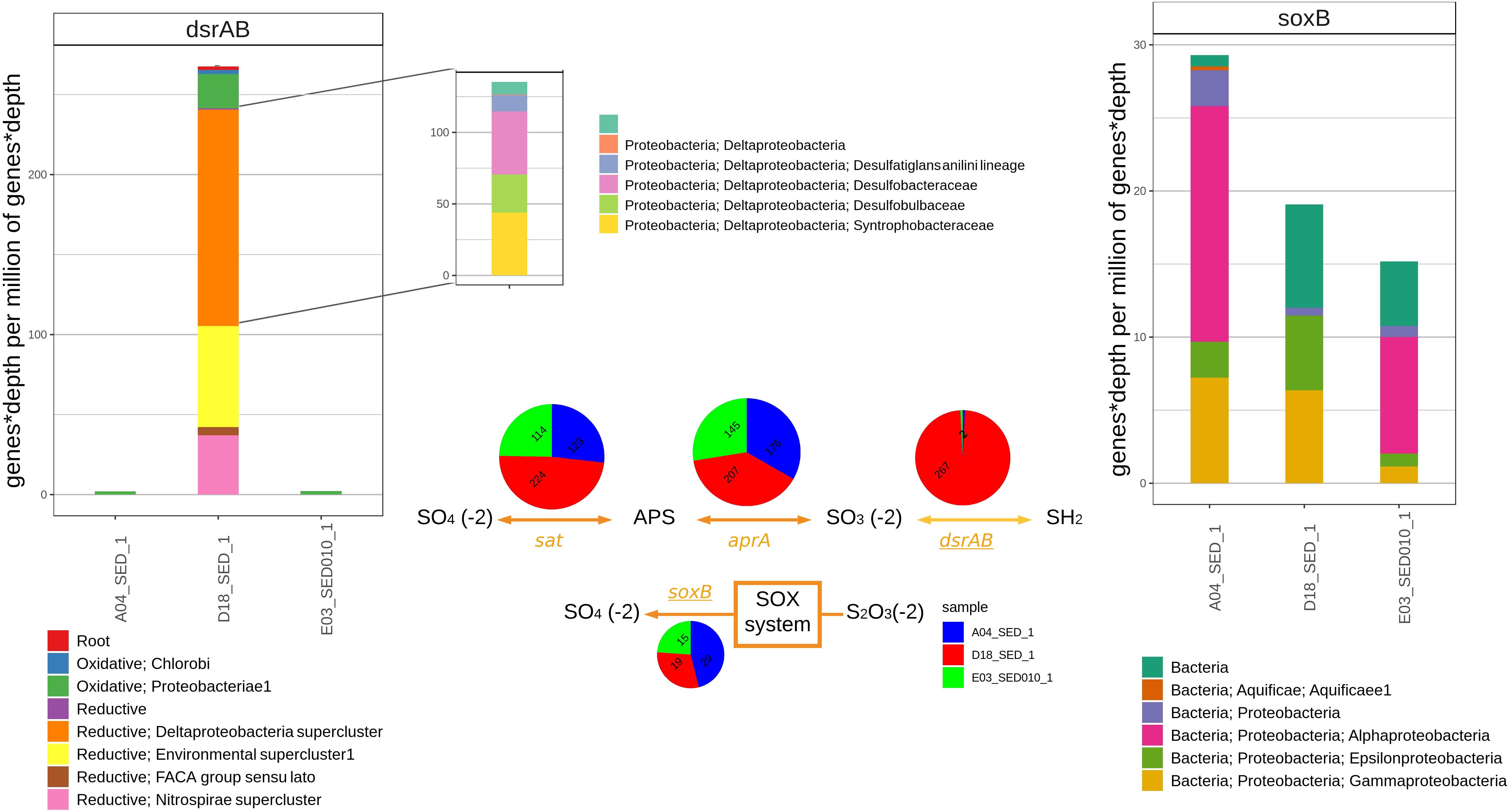
Figure 5. The bar graph on the left displays the abundance of genes with sequence homology to dsrAB in shotgun sediment assemblies from A04SED, D18SED, and E03SED samples and their most likely taxonomic classification according to the classification proposed by Müller et al. (2015). Center of the figure shows the relative abundance of genes aprA, sat, dsrAB (in the DSR pathway) and soxB (thiosulfate oxidation). The bar graph on the right displays the relative abundance of genes with sequence homology to soxB in sediment metagenomic assemblies.
We constructed a graftM package using the manually curated database of Müller et al. (2015). This phylogeny can identify dsrAB from archaea or bacteria and also what group they correspond to reductive bacteria, oxidative bacteria and reductive archaea. Sequences related to dsrAB show the greatest contrast in the number of associated homologs and taxonomic groups between sediment samples. The A04 and E03 samples possess a low abundance of reads (Figure 6, top left), and all of them are related to the oxidative Gammaproteobacteria oxidative groups. For the D18 sediment, the majority of associated homologs correspond to the reductive clusters (89%). The biggest cluster belongs to Deltaproteobacteria (51%) followed by the environmental supercluster (30%), that is composed of two lineages known as sulfate reducing families with no cultivated groups (Müller et al., 2015). The most significant difference between sediments is shown in the relative abundance of dsrAB gene. Almost no homologs were found for A04 and E03, while in D18, most homologs correspond to the reductive type metabolism and their taxonomy shows a predominance of the Deltaproteobacteria-supercluster of Desulfobacteraceae (45%), Desulfobulbaceae (26%), Desulfatiglans anili lineage (16%) and Syntrophobacteraceae (12%) families (Figure 6, top left). Sequences related to soxB were found in all three sediment samples (relative abundance numbers of predicted genes for soxB in A04SED sample, 29; for E03SED sample, 15; and for D18SED sample, 19). However, sequences assigned to Alphaproteobacteria only appeared in A04 and E03 sediment samples (Figure 6, top right).
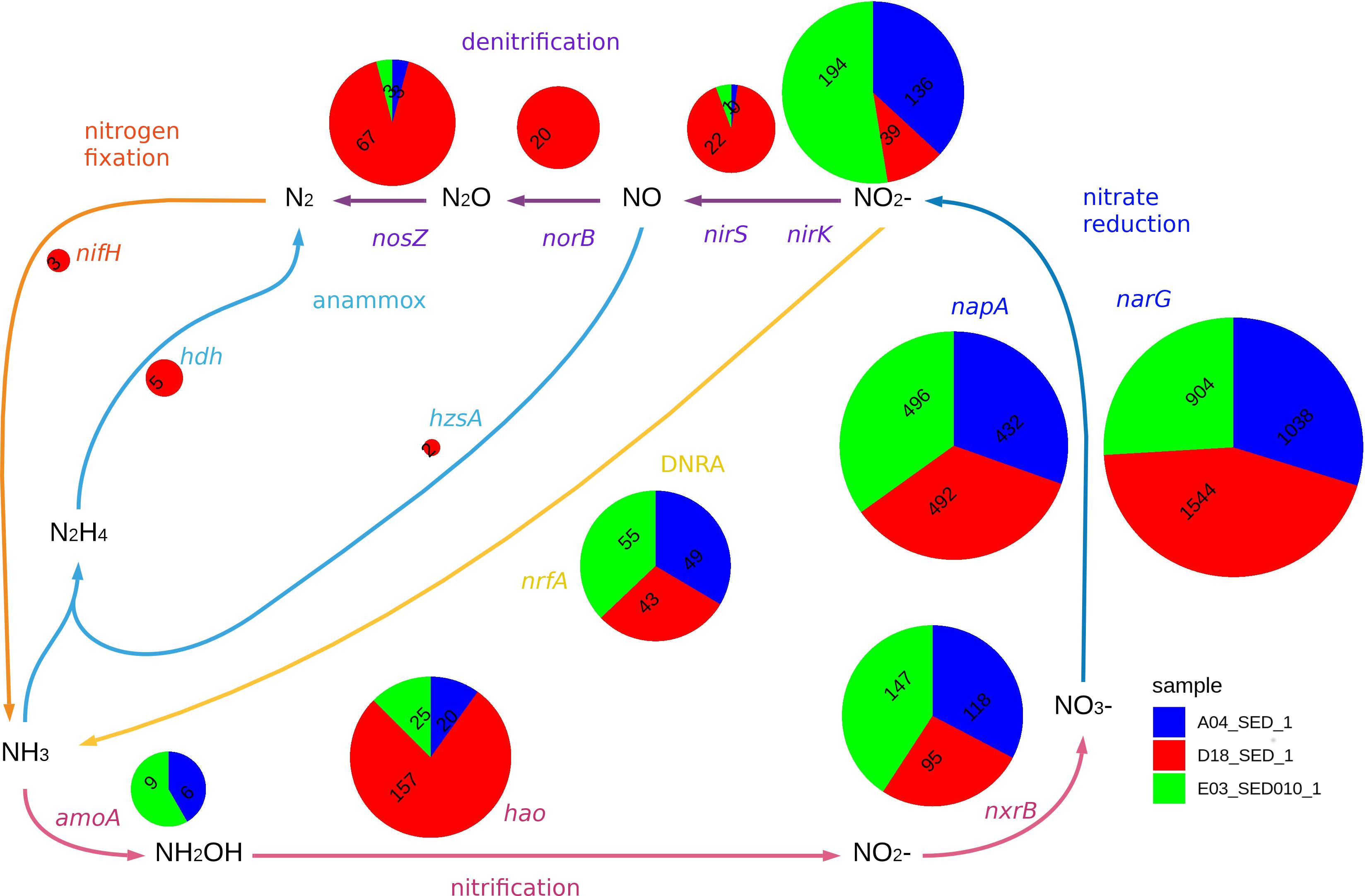
Figure 6. The figure displays the relative abundance of genes with homology marker genes in nitrate reduction (narG, napA) and related pathways (nirS, nirK, nrfA, norB, nosZ, hzsA, hdh, amoA, hao, nxrB, nifH). Each pie chart radius is proportional to the logarithm of the sum of relative abundances for the three samples. DNRA stands for Dissimilatory Nitrate Reduction to Ammonia. The microbial nitrogen cycle scheme was based on (Simon and Klotz, 2013). Classification for each gene is included in Supplementary Figure S2.
Nitrogen Metabolism
We searched for sequences homologous to genes involved in nitrogen fixation, nitrification, nitrate reduction, denitrification, DNRA, and anammox in all three sediment samples. However, we only identified homologs of anammox and nitrogen fixation pathways genes in the D18 sample (Figure 6), and conversely, amoA homologs (i.e., amoA of AOA and AOB, and pmoA) only in A04 and E03 sediment samples, all of them related to archaeal sequences (Supplementary Figure S2); hao homologs (nitrification marker) were found in the three sediment samples, although the majority were classified as distant homologs (Supplementary Figure S2). Both A04 and E03 sediments display a higher abundance of nxrB homologous sequences while D18 sediment samples showed more related to the narH gene.
We found homologous sequences to narG and napA, the typical nitrate reduction markers, and nrfA, a homolog of dnrA, in the three sediment samples. Sequences affiliated with Deltaproteobacteria stand out in the D18 sample. Meanwhile, sequences related to Alpha- and Gammaproteobacteria were more prevalent in samples from Perdido (A04 and E03). We found nirK related sequences (a denitrification marker) in all sediment samples. However, a significant proportion was present in A04 and E03. We also observed sequences related to the experimentally characterized nirK group only in those samples. A higher number of sequences related to nirS were detected in the D18 sample, and were associated to Betaproteobacteria and Gammaproteobacteria. norB related sequences were only found in the D18 sediment sample (from Betaproteobacteria and Gammaproteobacteria). Previous reports have shown the existence of two nosZ clades: TAT-dependent or typical Z-type NosZ protein, and Sec-dependent or atypical NosZ. TAT-dependent is commonly found in Alpha, Beta and, Gammaproteobacteria capable of complete denitrification, while Sec-dependent are present on a broader group of organisms with diverse nitrogen metabolism (Sanford et al., 2012; Jones et al., 2013). We only identified Sec-dependent nosZ in A04 and E03, however, we found both Sec-dependent and TAT-dependent homologous sequences in D18 (Supplementary Figure S2).
The gene marker for nitrogen fixation, nifH, was only found in D18 (nifH sequences related to cluster IV), and the remaining sequences could not be associated to any previously characterized nifH clusters. The anammox (anaerobic ammonium oxidation) process was detected only in the D18 sediment sample, with a small proportion of sequences related to hzsA and hdh of Planctomycetes, suggesting the possible presence of anammox bacteria in D18 sediment sample in low abundance.
Methanogenesis, Anaerobic Methane Oxidation and Wood–Ljungdhal Pathway
The best marker gene for methanogenesis and anaerobic oxidation of methane (AOM) is mcrA wich codes for the alpha subunit of methyl-coenzyme M reductase, and is used for the initial oxidation of methane or the final reduction step releasing methane in reverse methanogenesis (Friedrich, 2005). As we did not find any mcrA sequences, we looked for genes involved in the canonical hydrogenotrophic methanogenesis and reverse methanogenesis (Timmers et al., 2017): fmd (formylmethanofuran dehydrogenase), ftr (formylmethanofuran-tetrahydromethanopterin N-formyl transferase), mch (methenyltetrahydromethanopterin cyclo hydrolase), mtd (methylenetetrahydrofolate dehydrogenase), mer (5,10-methylenetetrahydromethanopterin reductase) and mtr (tetrahydromethanopterin S-methyltransferase).
A small number of sequences from the methanogenesis/AOM pathway (fmd, ftr, mch, mer, mtd, and mtrA) was found in the D18 sediment sample, most of those gene sequences were related to archaea (Figure 7), which included the Bathyarchaeota and the Thorarchaeota groups, that were not found in A04 nor in E03 sediments. In the A04 and E03 samples, we found some homologous sequences to the fmd, ftr and mch genes related to candidate NC10 division bacteria and better related to the Wood–Ljungdahl pathway (WLP).
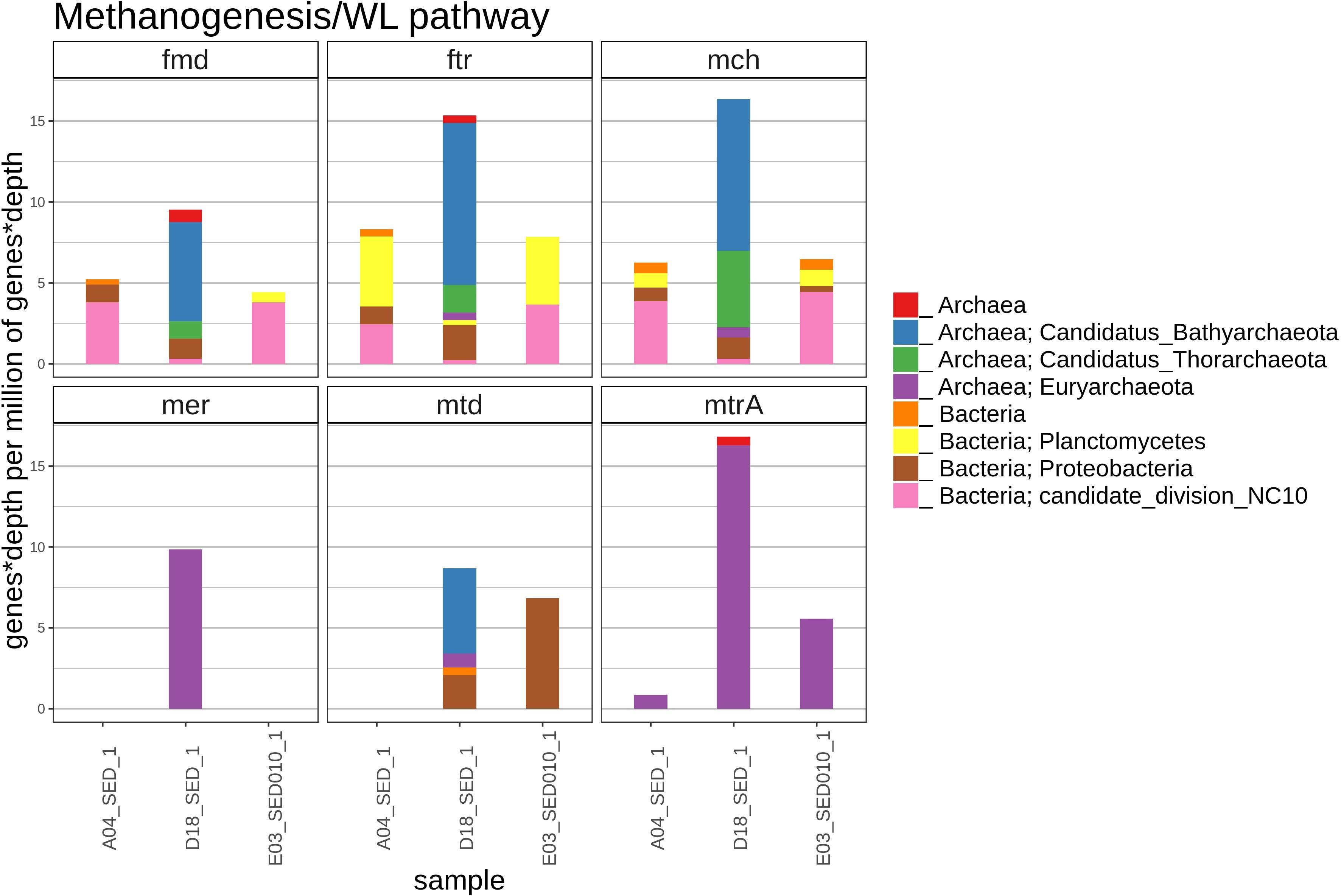
Figure 7. The figure shows the relative abundance (rate represented as number detected genes per million of genes in the metagenomic assembly) of genes with homology to genes fmd (formylmethanofuran dehydrogenase), ftr (formylmethanofuran:H 4 MPT formyltransferase), mch (methenyl-H 4 MPT cyclohydrolase), mer (methylene-H 4 MPT reductase), mtd (methylene-H 4 MPT dehydrogenase), mtrA (N 5-methyltetrahydromethanopterin: coenzyme M methyltransferase), in the methanogenesis/AOM/achaeal WL pathway in each sediment sample, and their most likely taxonomic classification according to NCBI taxonomy.
Aerobic and Anaerobic Hydrocarbon Degradation in Sediment and Water Samples
We found sequences related to aerobic hydrocarbon degradation in the water column samples. The highest diversity of detected alkB homologous sequences comes from D18MAX and A04AAIW. Sediment samples A04 and E03 and A04MIN water sample showed a lower sequence diversity (Figure 8 and Supplementary Figure S3).
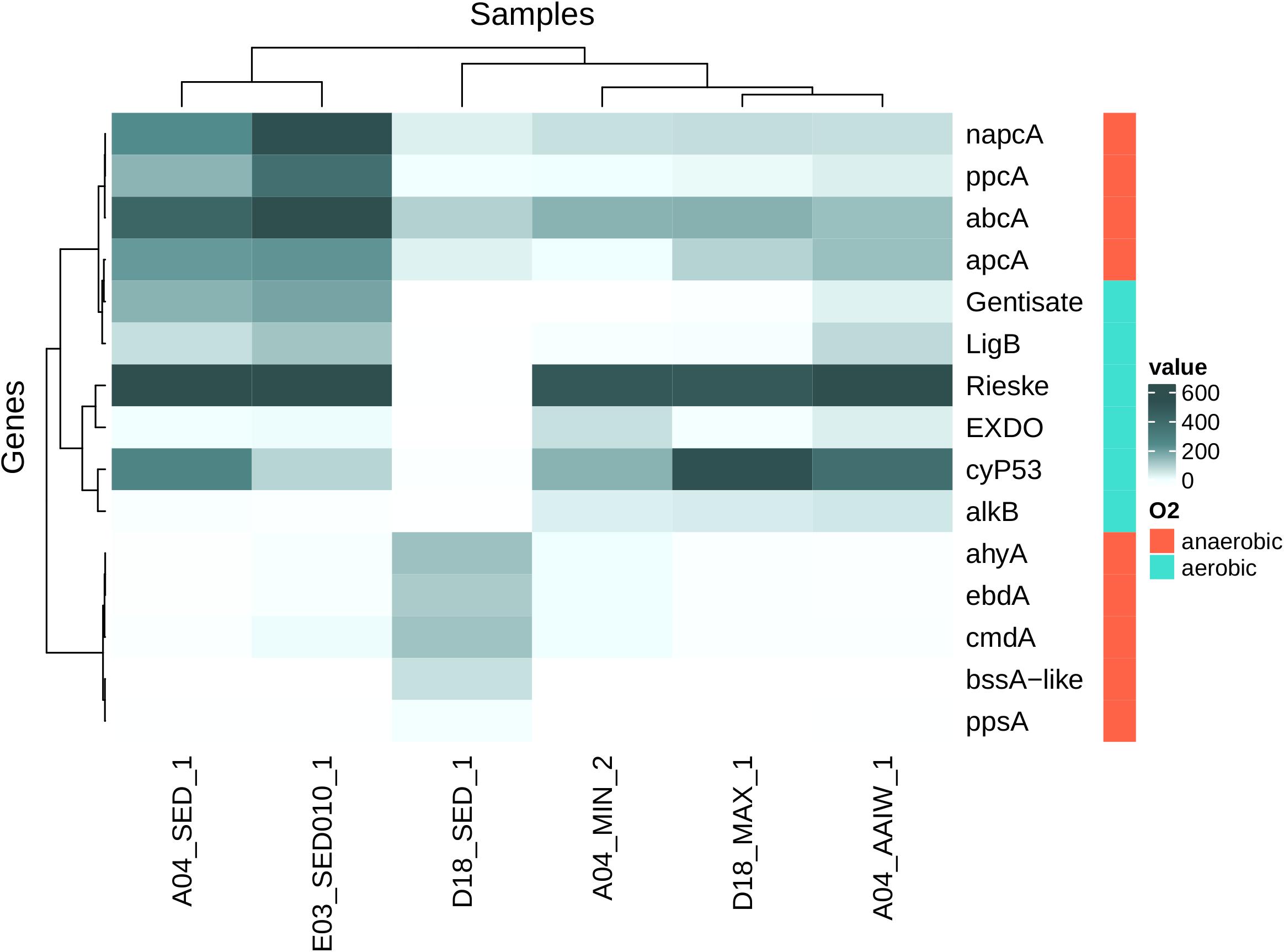
Figure 8. Heatmap shows homologous genes (number of genes per million of genes in metagenomic assemblies) to genes related with hydrocarbon activation step for posterior degradation. Rieske (Rieske oxygenases), cytP53 (cytochrome P53), napcA (naphthalene carboxylase), abcA (Putative Benzene Carboxylase), ppcA (Phenylphosphate Carboxylase), apcA (Acetophenone Carboxylase), LigB (protocatechuate dioxygenase), Gentisate (Cupin superfamily), ppsA (Phenylphosphate Synthase), bssA-like (Benzyl-Succinate Synthase like), ahyA (Alkane C2-Methylene hydroxylase), ebdA (Ethylbenzene Dehydrogenase), cmdA (p-Cymene Dehydrogenase), EXDO (Extradiol Dioxygenases, monocyclic bicyclic and miscellaneous substrates), alkB (Alkane Hydroxylase) (here gene sequencing depth was not considered). Samples and genes were sorted using hierarchical clustering.
Sequences associated with anaerobic hydrocarbon degradation were found in the D18 sediment sample. On the other hand, we found sequences related to aerobic hydrocarbon degradation and only carboxylases related to anaerobic degradation in the A04 and E03 samples. The bssA-like related sequences were detected only in the D18 sediment sample. Some sequences were closely related to assA, but a large fraction does not associate with any of the described families from the bssA-like family, apparently forming new taxonomic groups (Supplementary Figure S3).
We detected sequences homologous to the Rieske oxygenase family in all but the D18 sediment samples, the detected sequences encompass almost every class in the known diversity of this family (Duarte et al., 2014). In addition, many PHDB genera were identified in all the analyzed samples. This was based on genera previously identified in the literature to be associated with metabolic pathways involved in hydrocarbon degradation and related compounds (Ramírez et al., 2020). The abundance of PHDB is evident in the sites studied. In water samples we found 34 genera reported as hydrocarbon degrading bacteria while in sediment samples 39 were present.
Discussion
Bacterial Community Throughout the Water Column
It is well known that Alphaproteobacteria are very diverse, therefore their metabolism is also very varied. However, this group tends to dominate in mesophilic environments and decrease in harsher situations; we do observe the same behavior in our water column taxonomic assignment, where Alphaproteobacteria decrease trough depth (Figure 3). On the other hand, we found Gammaproteobacteria as the most abundant class in the aphotic layer. This group of bacteria plays an important ecological role in xenobiotic degradation, as the majority of the obligate hydrocarbon degrader bacteria belong to this class such as Alcanivorax, Alteromonas, Cycloclasticus, and Pseudomonas (Yakimov et al., 2007). All of them were identified in the aphotic layers with an average abundance range of 0.01–5.0%. We observed a particular enrichment of Alcanivorax 23%, Alteromonas 26.8%, and Vibrio 64% in A04 DEEP, D18 MIN and C10 DEEP respectively. These findings suggest that bacteria of the water column are actively degrading hydrocarbons, especially those from the higher depths, perhaps maintaining the equilibrium of the GoM hydrocarbon levels.
Bacterial Community Throughout Sediments
Anthropogenic inputs are detected in both NW and SE regions, increasing organic matter inputs. It has previously been reported that the presence of diverse hydrocarbons in NW GoM is due to various factors, especially to the organic material discharged by the Laguna Madre, Bravo, Soto La Marina and Pánuco rivers, which contribute to the complex mixture of organic compounds that accumulate in the marine sediments (Macdonald et al., 2015; Aguilera et al., 2019). Bacterial taxonomic profiles only showed significant differences for deep and shallow sediments and it was not possible to see the differences between NW and SE regions that the whole-metagenome data showed. However, we are aware that D18 sample is not enough to characterize a complete region, and even more after the variations that we have seen in SE GoM (Godoy-Lozano et al., 2018), where we identified changes in the bacterial community possibly due to perturbations generated by anthropogenic oil activities. Sanchez-Soto et al. (2018), reported a similar bacterial community from the Perdido Fold Belt as in this work. In both reports we observe several coincidences at the Class, Order, and even at Genus level. However, some of the taxonomic assignments vary due to the use of different databases.
Linking Taxonomy and Metabolism
Due to the technical and amplification biases of amplicon and shotgun metagenomics, we do not expect a correlation between the taxonomic results from both methods, therefore, we report amplicon data for our taxonomy analysis and we use WMS data for networks and metabolism. Networks could be built as WMS gave us a closest hit for each sequence for both genus and functional gene. However, we are aware that in fact each genus and each gene represent a whole group of similar bacteria or genes. Heterogeneity values determined in the networks show that sediments present a more complex community than water (Figure 4), suggesting a complex coordination-like dynamic drives the population in this habitat, as indicated in a recent network interpretation study (Pinheiro and Hartmann, 2017). In contrast, traits are more independent in the water column resulting in a less functionally redundant system, with specialized bacteria as Synechococcus and Prochlorococcus in the MAX layer. The recurrent presence of Acinetobacter and Pseudomonas in all layers as taxonomical keystones, and K01563 and K02567 as metabolic hubs, supports the presumption that bacteria adapted to hydrocarbons and xenobiotics are dominant in these sites. Nevertheless, networks also reflect the nitrogen, sulfur, and carbon pathways that are central in the biogeochemical cycles, and the functional redundancy that exists in sediments.
Marker Genes for Anaerobic Mineralization of Organic Matter
Marine sediments can be divided into three zones from top to bottom: oxic, suboxic, and reduced. Aerobic respiration and nitrification take place in the oxic zone, sulfate reduction and denitrification occur in the anoxic zone. Dissimilatory sulfate reduction takes place in the top part of the reduced zone, and below all, carbon dioxide reduction (methanogenesis) is carried out. The potential for denitrification solely found in A04 and E03 suggests that our samples of the 10 centimeters below the surface (cmbs) only reached the oxic and suboxic zones; this is consistent for deep samples, where we expect deeper oxygen penetration depths (Kristensen, 2000). On the other hand, in the same 10 cmbs of the D18 sediment sample, dissimilatory sulfate reduction potential and denitrification stand out, which along with the presence of hao and absence of amoA, suggest a thin oxic zone above the anoxic zone, as would be expected for shallower sediment samples (Kristensen, 2000). It would seem that natural hydrocarbon emanations could be playing an important role as accessible organic matter that depletes the oxygen rapidly, although we cannot yet rule out the involvement of other sources of organic matter such as river’s sewage, dead fauna or fecal pellets.
Sulfur Metabolisms (DSR and Sulfur Oxidation)
The dsrAB gene has been previously detected in Alphaproteobacteria, Betaproteobacteria, Gammaproteobacteria, Deltaproteobacteria, and Chlorobi using reverse dissimilatory sulfite reduction pathway (RDSR) (Loy et al., 2009; Swan et al., 2011; Sheik et al., 2014; Callbeck et al., 2018; Koch and Dahl, 2018). The homologous genes related to dissimilatory sulfate reduction (sat, aprA, and dsrAB) suggest the presence of a sulfate reduction population in the D18 sediment associated to Deltaproteobacteria; on the other hand, homologs of the same genes and of soxB suggest the presence of Alphaproteobacteria sulfur oxidizing populations in the A04 and E03 sediment samples.
The relative abundance of sat and aprA homologous genes is similar; however, their associated taxonomy has a main difference, in D18 sediments seem to be coming from a Deltaproteobacteria population and in A04 and E03 from the Alphaproteobacteria group (Supplementary Figure S1). The bacterial community in D18 sediments has the typical Deltaproteobacteria members of anoxic marine sediments shown to have an important role in acetate assimilation and carbon cycling (Jørgensen, 1982). These communities appear to be predominantly composed by Desulfobulbaceae, Desulfobacteraceae, and Syntrophobacteraceae (Figure 6). The latest have a diverse metabolism that allows them to grow with or without sulfate (Kuever, 2014; Wörner and Pester, 2019). Desulfobulbaceae also appears to have an essential role in acetate consumption, while Desulfobacteraceae remains dominant in the two groups (Dyksma et al., 2018). Interestingly, organisms from these families have been previously shown to anaerobically degrade hydrocarbons (Stagars et al., 2016; Davidova et al., 2018), to carry out acetate assimilation (Dyksma et al., 2018) and to be syntrophic partners of methanogenic and methanotrophic archaea (Mcglynn et al., 2015; Oberding and Gieg, 2016). A new yet unresolved pathway for sulfate reduction has been proposed for a syntrophic microbial consortium that mediated the AOM coupled to sulfate reduction and polysulfide disproportionation (Milucka et al., 2012). Remarkably, sulfate was reduced by an unknown mechanism in the archaeal partner resulting in the formation of disulfide and not by the deltaproteobacterial partner that harbors the canonical DsrAB-based pathway (Müller et al., 2015).
The dominance of the Alphaproteobacteria population increases within the soxB sulfur oxidation marker gene in the A04 and E03 sediments, followed by Epsilonproteobacteria and Gammaproteobacteria, all these groups have been reported to have species related to sulfur oxidation bacteria (SOB) (Yamamoto and Takai, 2011). Sediments from D18 show an absence of Alphaproteobacteria, and only Gamma and Epsilon were found with this marker gene. Marine microorganisms from Epsilonproteobacteria class are known to use the SOX system for sulfur oxidation, and Gammaproteobacteria use the reverse sulfate reduction (RDSR) apart from the sox system (Yamamoto and Takai, 2011), it has been suggested that they constitute 40–70% of the autotrophic sulfur oxidizers (Lenk et al., 2011; Boschker et al., 2014; Jørgensen et al., 2019). The high abundance of Alphaproteobacteria in the sediments from A04 and E03 is uncommon as there are few reports of them being the dominating group of sulfur oxidizers. Further studies are required to determine if the abundance of this group is due to the type of sediment and associated with abiotic variables.
Methane Metabolism and Wood–Ljungdahl Pathway (WLP)
Methane metabolism in the ocean is of particular interest because of its global warming potential, 23 times that of carbon dioxide (Brown et al., 2014). Also oceans act as a sink for atmospheric methane, and contribute to global methane emissions by 1–4% (Karl et al., 2008). We decided to look for methane metabolism genes in our sediments, given the importance of methane and the numerous oil and gas seeps of diverse origin in the GoM (Kennicutt, 2017). Methane related metagenomic sequences represent a small proportion of the total sequences, we do not rule out the possibility of mcrA absence due to incomplete sequencing. With respect to the other explored genes, we could detect only methanogenesis genes in D18 sediment and some carbon fixation genes of the WLP in E03 and A04 sediments. The relation between WLP and methanogenesis is considered to be an important and ancient energy generation and carbon fixation metabolism, where these two metabolisms share some homology and gene conservation between archaea and bacteria (Borrel et al., 2016).
The presence of anaerobic archaeal communities with energetic coupling types that have not yet been completely explored, where reducing power comes from oxidation of organic compounds using enzymes related to WLP and methanogenic pathways is likely in our D18 sediment sample. All of the identified methanogenic organisms belong exclusively to three phyla of the archaeal domain: the well-known Euryarchaeota (Methanomicrobiales), the recently discovered Bathyarchaeota (former Crenoarchaeota group) (Evans et al., 2015) and the very recent Verstraetearchaeota phylum (Berghuis et al., 2019). We detected the known WLP genes carried by NC10 bacteria and Planctomycetia (Chistoserdova et al., 2004; Adam et al., 2019; Kalyuzhnaya et al., 2019). WLP is commonly found in acetogenic bacteria, archaea and recently has been found in non-methanogenic and non-methylotrophic archaea and bacteria, where its function remains unclear in the absence of methyl-coenzyme M reductase complex (MCR) and N5-Methyltetrahydromethanopterin:coenzyme M methyltransferase complex (MTR) complexes (Borrel et al., 2016; Chistoserdova, 2016); it has been suggested that it could be involved in other processes than methanogenesis like extra H2 production during fermentation or formaldehyde detoxification (Adam et al., 2019). Recently, methanogenesis has been linked to alkane degradation through a divergent MCR within some Euryarchaeota capable of degrading ethane (Chen et al., 2019; Laso-Pérez et al., 2019; Wang et al., 2020), which opens the possibility of having methanogens degrading hydrocarbons with MCRs not detected by this study.
Dissimilatory Nitrate Reduction and Adjacent Pathways Related to the Nitrogen Cycle
Dissimilatory nitrate reduction is a key step in the nitrogen cycle, as nitrate is the second preferred electron acceptor after oxygen, useful for metabolism in anaerobic conditions (Hensen et al., 2006). We identified nitrate reduction potential in the three sediment samples, having a high relative abundance and diversity of both nitrate reductases we searched for, this is also consistent with the wide distribution of nitrate reduction in nature (Kraft et al., 2011).
Nitrogen in nitrite produced by dissimilatory nitrate reduction can go back to ammonium (DNRA) or escape as dinitrogen gas (denitrification or anammox), which depends on the environmental conditions and the genomic composition of the community (Kraft et al., 2011). These enzymatic steps can also be coupled to electron transport and energy conservation processes (Simon and Klotz, 2013). The abundance of nrfA homologs (gene marker for DNRA) indicates the potential to return nitrite into ammonium in all samples. Nitrite reductases that catalyze the initial step for nitrogen loss (nirK and nirS) are also present in the three samples, notably nirS is more abundant in D18 and nirK dominates in A04 and E03. The abundance patterns and associated populations for norB and nosZ, suggest a complete denitrification potential in the D18 sample. Nitrate reduction generally occurs when oxygen is depleted in the environment. The presence of nirS, norB, nosZ (denitrification pathway) in D18 sediment, complements the findings that this sediment is more anoxic.
We found the marker genes for anammox only in the D18 sample, and in low abundances. So far, the proposed pathways for anammox start with nitrite reduction into nitric oxide by the nirS enzyme (Kartal et al., 2011). Annammox has been exclusively found in five genera belonging to the phylum Planctomycetes (Kartal and Keltjens, 2016) but no sequence found here could be directly associated with any of those five genera. Also, the nifH homologous sequences we found were not related to any characterized nitrogen fixing clusters (Chien and Zinder, 1994; Dang et al., 2009).
Nitrification transforms ammonium into nitrate in the presence of oxygen (Kuypers et al., 2018). The amoA (ammonia monooxygenase subunit A) and hao (hydroxylamine oxidoreductase) genes have been previously used to assess the environmental diversity of aerobic ammonium oxidizing microorganisms (Rotthauwe et al., 1997; Schmid et al., 2008; Alves et al., 2018). We only detected amoA related sequences in A04 and E03, suggesting that a community of aerobic archaea performing ammonium oxidation is present only there and not in D18.
Homologous sequences to the hao and nxrB genes are present in the three sediment samples, however, their homology to other genes in the nitrogen cycle hinders the direct interpretation of the results for these two steps, for example a large portion of nxrB homologs in D18 sediment sample is more similar to respiratory nitrate reductase (narH), that encodes a subunit of the NAR complex involved in nitrate reduction (Simon and Klotz, 2013).
An accurate interpretation is hard to achieve, as the nitrogen cycle and the enzymes involved are a complex system, as Simon and Klotz (2013) have analyzed. The role enzymes play depends upon both environmental conditions and the organism in question. Many enzymes show homology between different steps in the cycle, some enzymes display promiscuous activity, and most enzymes belong to protein complexes. Therefore, the query enzymes we are taking here, give us only an overview of the metabolic potential for nitrate reduction and related steps.
Hydrocarbon Degradation in Sediment and Water Samples
We expected the presence of diverse sequences related to hydrocarbon degradation as previous studies in the water and sediments in the GoM have shown the presence of PHDB (Godoy-Lozano et al., 2018; Moreno-Ulloa et al., 2019; Ramírez et al., 2020). It is important to note that some of the most abundant bacterial genera reported in this work are related to the biodegradation of contaminants and support the idea that microbial communities in deep-sea sediments of GoM have the potential to metabolize a variety of organic compounds, including oil, crude oil and its derivatives.
We found sequences associated to anaerobic hydrocarbon degradation in the D18 sediment sample, sequences related to both anaerobic and aerobic degradation in A04 and E03. Finally, water samples contain primarily sequences related to aerobic degradation. The diversity of sequences suggests microorganisms have the intrinsic potential to degrade a wide range of hydrocarbon compounds, as might be the case in an oil spill event. alkB genes are associated with the degradation of C10-C28 alkanes (Van Beilen and Funhoff, 2007; Nie et al., 2014; Muriel-Millán et al., 2019), and we detected the majority of these sequences in water samples. The high diversity of Rieske oxygenases in water samples and in the A04 and E03 sediments, indicates there is potential for degradation of diverse aromatic compounds such as benzoate, benzene, toluene, phthalate, naphthalene or biphenyl (Duarte et al., 2014).
The AlkB and Rieske oxygenases enzymes are active under aerobic conditions, but hydrocarbon degradation can also occur under anaerobic conditions. The D18 sediment sample shows a high diversity of bssA-like sequences, which are involved in hydrocarbon (both aromatic and aliphatic compounds) anaerobic degradation activation step through fumarate addition (Acosta-González et al., 2013) and have been reported in sulfate reducing bacteria and nitrate reducing bacteria (Kropp et al., 2000; Rabus et al., 2001). Other studies have reported the presence of bssA-like sequences in bacterial consortia capable of growing using hydrocarbon compounds under anaerobic conditions (Callaghan et al., 2010).
Conclusion
The microbial diversity and structure of the bacterial communities in water samples and surface sediments of the NW and SE region of the GoM revealed differences between these two geographical zones particularly in sediments. The high relative abundance of sulfate reduction, denitrification marker-genes and a high numbers of anaerobic genes and bacterial genera shown in networks and also in our drawn metabolic pathways leads us to conclude that our SE GoM sample is a more oxygen depleted sediment with the presence of an anaerobic bacterial community, explained perhaps by the high organic matter deposits in seafloor coming from both, river discharge and hydrocarbons. The abundance of putative hydrocarbon degrading bacteria and hydrocarbon degradation genes in both studied areas could be indicative of impacted zones caused by the presence of hydrocarbons in the environment that could influence the equilibrium of biogeochemical cycles. Our findings contribute to understanding the role of indigenous GoM bacterial taxa in biogeochemical cycles and hydrocarbon-degrading metabolism that would have a potential role in bioremediation strategies for oil-spills in water and marine sediments.
Data Availability Statement
The datasets presented in this study can be found in online repositories. The names of the repository/repositories and accession number(s) can be found in the article/ Supplementary Material.
Author Contributions
KJ and LS conceived and designed the experiments. LR, FG-G, AM-S, EG-L, and AE-Z analyzed and interpreted the data. LR, FG-G, AM-S, KJ, and LS wrote the manuscript and prepared the figures and tables. AL-N managed the L4-CIGOM consortium resources and guaranteed their availability to perform the experiments and analysis. LP-L coordinated the IBT-L4-CIGOM group. EM, RG-R, AL, AS-F, and LP-L contributed to the improvement of the project and reviewed the final version of the manuscript. All the authors contributed to the article and approved the submitted version.
Funding
The research funded by the National Council of Science and Technology of Mexico – Mexican Ministry of Energy –Hydrocarbon Trust, project 201441. This is a contribution of the Gulf of Mexico Research Consortium (CIGoM).
Conflict of Interest
The authors declare that the research was conducted in the absence of any commercial or financial relationships that could be construed as a potential conflict of interest.
Acknowledgments
We thank Cristina Domínguez-Mendoza, Silvia Hinojosa-Alvarez, L. Felipe Muriel, Wendy Hinojosa, and Jose Luis Rodríguez for their technical support in the laboratory. We also thank the crew of the R/V Justo Sierra who participated in the research cruises and the “Laboratorio Nacional de Buques Oceanográficos” (LANAB/O). Alejandro Estradas, Diana Ramirez, and Emmanuel Alvizo for technical support during sampling. We are grateful to “Unidad de Secuenciación Masiva y Bioinformática” at the Instituto de Biotecnología/UNAM for sequencing services and Alejandro Abdala Asbun for database support.
Supplementary Material
The Supplementary Material for this article can be found online at: https://www.frontiersin.org/articles/10.3389/fmicb.2020.01825/full#supplementary-material
Footnotes
References
Acosta-González, A., Rosselló-Móra, R., and Marqués, S. (2013). Diversity of benzylsuccinate synthase-like (bssA) genes in hydrocarbon-polluted marine sediments suggests substrate-dependent clustering. Appl. Environ. Microbiol. 79, 3667–3676. doi: 10.1128/aem.03934-12
Adam, P. S., Borrel, G., and Gribaldo, S. (2019). An archaeal origin of the Wood–Ljungdahl H4MPT branch and the emergence of bacterial methylotrophy. Nat. Microbiol. 4, 2155–2163. doi: 10.1038/s41564-019-0534-2
Aguilera, C., Leija, A., Torres, M., and Mendoza, R. (2019). Assessment of environmental quality in the tamaulipas laguna madre, gulf of mexico, by integrated biomarker response using the cross-barred venus clam chione elevata. Water Air Soil Pollut. 230:27.
Alves, R. J., Eloy, B. Q. M., Urich, T., Von Haeseler, A., and Schleper, C. (2018). Unifying the global phylogeny and environmental distribution of ammonia-oxidising archaea based on amoa genes. Nat. Commun. 9:1517.
Angel, R., Nepel, M., Panhölzl, C., Schmidt, H., Herbold, C. W., Eichorst, S. A., et al. (2018). Evaluation of primers targeting the diazotroph functional gene and development of NifMAP–A bioinformatics pipeline for analyzing nifH amplicon data. Front. Microbiol. 9:703. doi: 10.3389/fmicb.2018.00703
Bacosa, H., Erdner, D., Rosenheim, B., Shetty, P., Seitz, K., Baker, B., et al. (2018). Hydrocarbon degradation and response of seafloor sediment bacterial community in the northern gulf of mexico to light louisiana sweet crude oil. ISME J. 12:1.
Bae, H. S., Huang, L., White, J. R., Wang, J., Delaune, R. D., and Ogram, A. (2018). Response of microbial populations regulating nutrient biogeochemical cycles to oiling of coastal saltmarshes from the Deepwater Horizon oil spill. Environ. Pollut. 241, 136–147. doi: 10.1016/j.envpol.2018.05.033
Banerjee, S., Schlaeppi, K., and Marcel Van der Heijden, G. A. (2018). Keystone taxa as drivers of microbiome structure and functioning. Nat. Rev. Microbiol. 16, 567–576. doi: 10.1038/s41579-018-0024-1
Berghuis, B. A., Yu, F. B., Schulz, F., Blainey, P. C., Woyke, T., and Quake, S. R. (2019). Hydrogenotrophic methanogenesis in archaeal phylum Verstraetearchaeota reveals the shared ancestry of all methanogens. Proc. Natl. Acad. Sci. U.S.A. 116, 5037–5044. doi: 10.1073/pnas.1815631116
Bienhold, C., Zinger, L., Boetius, A., and Ramette, A. (2016). Diversity and biogeography of bathyal and abyssal seafloor bacteria. PLoS One 11:e0148016. doi: 10.1371/journal.pone.0148016
Bolyen, E., Rideout, J. R., Matthew, R., Nicholas, A. D., Bokulich, C. C., Abnet, G. A., et al. (2019). Reproducible, interactive, scalable and extensible microbiome data science using QIIME 2. Nat. Biotechnol. 37, 852–857.
Borrel, G., Adam, P. S., and Gribaldo, S. (2016). Methanogenesis and the wood–ljungdahl pathway: an ancient. Versatile, and fragile association. Genome Biol. Evol. 8, 1706–1711. doi: 10.1093/gbe/evw114
Boschker, H. T. S., Vasquez-Cardenas, D., Bolhuis, H., Moerdijk-Poortvliet, T. W. C., and Moodley, L. (2014). Chemoautotrophic carbon fixation rates and active bacterial communities in intertidal marine sediments. PLoS One 9:e101443. doi: 10.1371/journal.pone.0101443
Boyd, J. A., Woodcroft, B. J., and Tyson, G. W. (2018). GraftM: a tool for scalable, phylogenetically informed classification of genes within metagenomes. Nucleic Acids Res. 46:e59. doi: 10.1093/nar/gky174
Braker, G., Fesefeldt, A., and Witzel, K. P. (1998). Development of PCR primer systems for amplification of nitrite reductase genes (nirK and nirS) to detect denitrifying bacteria in environmental samples. Appl. Environ. Microbiol. 64, 3769–3775. doi: 10.1128/aem.64.10.3769-3775.1998
Braker, G., and Tiedje, J. M. (2003). Nitric oxide reductase (norB) genes from pure cultures and environmental samples. Appl. Environ. Microbiol. 69, 3476–3483. doi: 10.1128/aem.69.6.3476-3483.2003
Braker, G., Zhou, J., Wu, L., Devol, A. H., and Tiedje, J. M. (2000). Nitrite reductase genes (nirK and nirS) as functional markers to investigate diversity of denitrifying bacteria in pacific northwest marine sediment communities. Appl. Environ. Microbiol. 66, 2096–2104. doi: 10.1128/aem.66.5.2096-2104.2000
Brown, I. J., Torres, R., and Rees, A. P. (2014). The origin of sub-surface source waters define the sea-air flux of methane in the Mauritanian Upwelling. NW Africa. Dynam. Atmosph. Oceans 67, 39–46. doi: 10.1016/j.dynatmoce.2014.06.001
Bryant, D. M., Johnson, K., DiTommaso, T., Tickle, T., Brian, M., Payzin-Dogru, C. D., et al. (2017). A tissue-mapped axolotl de novo transcriptome enables identification of limb regeneration factors. Cell Rep. 18, 762–776. doi: 10.1016/j.celrep.2016.12.063
Burgin, A. J., and Hamilton, S. K. (2007). Have we overemphasized the role of denitrification in aquatic ecosystems? A review of nitrate removal pathways. Front. Ecol. Environ. 5:89–96. doi: 10.1890/1540-9295(2007)5[89:hwotro]2.0.co;2
Callaghan, A. V., Davidova, I. A., Savage-Ashlock, K., Parisi, V. A., Gieg, L. M., Suflita, J. M., et al. (2010). Diversity of Benzyl- and alkylsuccinate synthase genes in hydrocarbon-impacted environments and enrichment cultures. Environ. Sci. Technol. 44, 7287–7294. doi: 10.1021/es1002023
Callahan, B. J., McMurdie, P. J., Rosen, M. J., Han, A. W., Amy, J. A. J., and Holmes, S. P. (2016). DADA2: high-resolution sample inference from illumina amplicon data. Nat. Methods 13, 581–583. doi: 10.1038/nmeth.3869
Callbeck, C. M., Lavik, G., Ferdelman, T. G., Fuchs, B., Gruber-Vodicka, H. R., Hach, P. F., et al. (2018). Oxygen minimum zone cryptic sulfur cycling sustained by offshore transport of key sulfur oxidizing bacteria. Nat. Commun. 9, 1–11.
Camacho, C., Coulouris, G., Avagyan, V., Ma, N., Papadopoulos, J., Bealer, K., et al. (2009). BLAST+: architecture and applications. BMC Bioinformatics 10:421. doi: 10.1186/1471-2105-10-421
Chen, S., Musat, N., Lechtenfeld, O., Paschke, H., Schmidt, M., Said, N., et al. (2019). Anaerobic oxidation of ethane by archaea from a marine hydrocarbon seep. Nature 568, 108–111. doi: 10.1038/s41586-019-1063-0
Chien, Y. T., and Zinder, S. H. (1994). Cloning, DNA sequencing, and characterization of a nifD-homologous gene from the archaeon Methanosarcina barkeri 227 which resembles nifD1 from the eubacterium Clostridium pasteurianum. J. Bacteriol. 176, 6590–6598. doi: 10.1128/jb.176.21.6590-6598.1994
Chistoserdova, L. (2016). Wide distribution of genes for tetrahydromethanopterin/methanofuran-linked C1 transfer reactions argues for their presence in the common ancestor of bacteria and archaea. Front. Microbiol. 7:1425. doi: 10.3389/fmicb.2016.01425
Chistoserdova, L., Jenkins, C., Kalyuzhnaya, M. G., Marx, C. J., Lapidus, A., Vorholt, J. A., et al. (2004). The enigmatic planctomycetes may hold a key to the origins of methanogenesis and methylotrophy. Mol. Biol. Evol. 21, 1234–1241. doi: 10.1093/molbev/msh113
Chong, J., and Xia, J. (2017). Computational approaches for integrative analysis of the metabolome and microbiome. Metabolites 7:62. doi: 10.3390/metabo7040062
Dang, H., Luan, X., Zhao, J., and Li, J. (2009). Diverse and novel nifh and nifh-like gene sequences in the deep-sea methane seep sediments of the okhotsk sea. Appl. Environ. Microbiol. 75, 2238–2245. doi: 10.1128/aem.02556-08
Davidova, I. A., Marks, C. R., and Suflita, J. M. (2018). Anaerobic Hydrocarbon-Degrading Deltaproteobacteria. Manhattan, NY: Springer International Publishing, 1–38.
Delmont, T. O., Quince, C., Shaiber, A., Esen, ÖC., Lee, S. T., Rappé, M. S., et al. (2018). Nitrogen-fixing populations of Planctomycetes and Proteobacteria are abundant in surface ocean metagenomes. Nat. Microbiol. 3, 804–813. doi: 10.1038/s41564-018-0176-9
Du, Z.-J., Wang, Z.-J., Zhao, J.-X., and Chen, G.-J. (2016). Woeseia oceani gen. nov., sp. nov., a chemoheterotrophic member of the order chromatiales, and proposal of woeseiaceae fam. nov. Int. J. Syst. Evol. Microbiol. 66, 107–112. doi: 10.1099/ijsem.0.000683
Duarte, M., Jauregui, R., Vilchez-Vargas, R., Junca, H., and Pieper, D. H. (2014). AromaDeg, a novel database for phylogenomics of aerobic bacterial degradation of aromatics. Database 2014, 1–12.
Dyksma, S., Lenk, S., Sawicka, J. E., and Mußmann, M. (2018). Uncultured Gammaproteobacteria and desulfobacteraceae account for major acetate assimilation in a coastal marine sediment. Front. Microbiol. 9:3124. doi: 10.3389/fmicb.2018.03124
Evans, P. N., Parks, D. H., Chadwick, G. L., Robbins, S. J., Orphan, V. J., Golding, S. D., et al. (2015). Methane metabolism in the archaeal phylum bathyarchaeota revealed by genome-centric metagenomics. Science 350, 434–438. doi: 10.1126/science.aac7745
Friedrich, M. W. (2005). Methyl−Coenzyme M reductase genes: unique functional markers for methanogenic and anaerobic methane−oxidizing archaea. Environ. Microbiol. 397, 428–442. doi: 10.1016/s0076-6879(05)97026-2
Fuchs, G., Boll, M., and Heider, J. (2011). Microbial degradation of aromatic compounds- From one strategy to four. Nat. Rev. Microbiol. 9, 803–816. doi: 10.1038/nrmicro2652
Godoy-Lozano, E. E., Escobar-Zepeda, A., Raggi, L., Merino, E., Gutierrez-Rios, R. M., Juarez, K., et al. (2018). Bacterial diversity and the geochemical landscape in the southwestern Gulf of Mexico. Front. Microbiol. 9:2528. doi: 10.3389/fmicb.2018.02528
Harhangi, H. R., Le Roy, M., Van Alen, T., Hu, B. L., Groen, J., Kartal, B., et al. (2012). Hydrazine synthase, a unique phylomarker with which to study the presence and biodiversity of anammox bacteria. Appl. Environ. Microbiol. 78, 752–758. doi: 10.1128/aem.07113-11
Hensen, C., Zabel, M., and Schulz, H. N. (2006). Benthic Cycling of Oxygen, Nitrogen and Phosphorus. Berlin: Springer, 207–240.
Hügler, M., Gärtner, A., and Imhoff, J. F. (2010). Functional genes as markers for sulfur cycling and CO2 fixation in microbial communities of hydrothermal vents of the Logatchev field. FEMS Microbiol. Ecol. 73, 526–537.
Jaffer, Y. D., Purushothaman, C. S., Sanath, K. H., Irfan, A. B., Gireesh-Babu, P., Ganie, P. A., et al. (2019). A combined approach of 16S rRNA and a functional marker gene, soxB to reveal the diversity of sulphur-oxidising bacteria in thermal springs. Arch. Microbiol. 201, 951–967. doi: 10.1007/s00203-019-01666-4
Jones, C. M., Graf, D. R. H., Bru, D., Philippot, L., and Hallin, S. (2013). The unaccounted yet abundant nitrous oxide-reducing microbial community: a potential nitrous oxide sink. ISME J. 7, 417–426. doi: 10.1038/ismej.2012.125
Jørgensen, B. B. (1982). Mineralization of organic matter in the sea bed—the role of sulphate reduction. Nature 296, 643–645. doi: 10.1038/296643a0
Jørgensen, B. B., Findlay, A. J., and Pellerin, A. (2019). The biogeochemical sulfur cycle of marine sediments. Front. Microbiol. 10:1–27.
Kalyuzhnaya, M. G., Gomez, O. A., and Murrell, J. C. (2019). The Methane-Oxidizing Bacteria (Methanotrophs). Manhattan, NY: Springer International Publishing, 1–34.
Karl, D. M., Beversdorf, L., Björkman, K. M., Church, M. J., Martinez, A., and Delong, E. F. (2008). Aerobic production of methane in the sea. Nat. Geosci. 1, 473–478. doi: 10.1038/ngeo234
Kartal, B., and Keltjens, J. T. (2016). Anammox biochemistry: a tale of heme c proteins. Trends Biochem. Sci. 41, 998–1011. doi: 10.1016/j.tibs.2016.08.015
Kartal, B., Maalcke, W. J., De Almeida, N. M., Cirpus, I., Gloerich, J., Geerts, W., et al. (2011). Molecular mechanism of anaerobic ammonium oxidation. Nature 479, 127–130.
Kasten, S., Jørgensen, B. B. (2000). “Sulfate reduction in marine sediments,” in Marine Geochemistry, eds Schulz, H. D., and Zabel, M. (Berlin: Springer Berlin Heidelberg), 263–281.
Kern, M., Klotz, M. G., and Simon, J. (2011). The Wolinella succinogenes mcc gene cluster encodes an unconventional respiratory sulphite reduction system. Mol. Microbiol. 82, 1515–1530. doi: 10.1111/j.1365-2958.2011.07906.x
Khadka, R., Clothier, L., Wang, L., Lim, C. K., Klotz, M. G., and Dunfield, P. F. (2018). Evolutionary history of copper membrane monooxygenases. Front. Microbiol. 9:2493.
Klindworth, A., Pruesse, E., Schweer, T., Peplies, J., Quast, C., Horn, M., et al. (2013). Evaluation of general 16S ribosomal RNA gene PCR primers for classical and next-generation sequencing-based diversity studies. Nucleic Acids Res. 41, 1–11.
Klotz, M. G., Schmid, M. C., Strous, M., Op Den, C. H. J. M., Jetten, M. S. M., and Hooper, A. B. (2008). Evolution of an octahaem cytochrome c protein family that is key to aerobic and anaerobic ammonia oxidation by bacteria. Environ. Microbiol. 10, 3150–3163. doi: 10.1111/j.1462-2920.2008.01733.x
Knittel, K., and Boetius, A. (2009). Anaerobic oxidation of methane: progress with an unknown process. Ann. Rev. Microbiol. 63, 311–334. doi: 10.1146/annurev.micro.61.080706.093130
Koch, T., and Dahl, C. (2018). A novel bacterial sulfur oxidation pathway provides a new link between the cycles of organic and inorganic sulfur compounds. ISME J. 12, 2479–2491. doi: 10.1038/s41396-018-0209-7
Kong, L., Jing, H., Kataoka, T., Buchwald, C., and Liu, H. (2013). Diversity and spatial distribution of hydrazine oxidoreductase (hzo) gene in the oxygen minimum zone off Costa Rica. PLoS One 8:e78275. doi: 10.1371/journal.pone.0078275
Kostka, J. E., Prakash, O., Overholt, W. A., Green, S. J., Freyer, G., Canion, A., et al. (2011). Hydrocarbon-degrading bacteria and the bacterial community response in gulf of mexico beach sands impacted by the deepwater horizon oil spill. Appl. Environ. Microbiol. 77:7962. doi: 10.1128/AEM.05402-11
Kraft, B., Strous, M., and Tegetmeyer, H. E. (2011). Microbial nitrate respiration–Genes, enzymes and environmental distribution. J. Biotechnol. 155, 104–117. doi: 10.1016/j.jbiotec.2010.12.025
Kristensen, E. (2000). Organic matter diagenesis at the oxic/anoxic interface in coastal marine sediments, with emphasis on the role of burrowing animals. Hydrobiologia 426, 1–24. doi: 10.1007/978-94-011-4148-2_1
Kropp, K. G., Davidova, I. A., and Suflita, J. M. (2000). Anaerobic oxidation of n-dodecane by an addition reaction in a sulfate-reducing bacterial enrichment culture. Appl. Environ. Microbiol. 66, 5393–5398. doi: 10.1128/aem.66.12.5393-5398.2000
Kuypers, M. M. M., Marchant, H. K., and Kartal, B. (2018). The microbial nitrogen-cycling network. Nat. Rev. Microbiol. 16, 263–276. doi: 10.1038/nrmicro.2018.9
Laso-Pérez, R., Hahn, C., Van Vliet, D., Tegetmeyer, H., Schubotz, F., Smit, N. T., et al. (2019). Anaerobic degradation of non. methane alkanes by “Candidatus methanoliparia” in hydrocarbon seeps of the gulf of mexico. mBio 10:e01 814-19.
Lenk, S., Arnds, J., Zerjatke, K., Musat, N., Amann, R., and Mußmann, M. (2011). Novel groups of Gammaproteobacteria catalyse sulfur oxidation and carbon fixation in a coastal, intertidal sediment. Environ. Microbiol. 13, 758–774. doi: 10.1111/j.1462-2920.2010.02380.x
Li, H., and Durbin, R. (2009). Fast and accurate short read alignment with burrows–wheeler transform. Bioinformatics 25, 1754–1760. doi: 10.1093/bioinformatics/btp324
Liu, Z., Liu, J., Zhu, Q., and Wu, W. (2012). The weathering of oil after the Deepwater Horizon Oil spill: insights from the chemical composition of the oil from the sea surface, salt marshes and sediments. Environ. Res. Lett. 7:e035302.
Lizarrága-Partida, M. L. (1986). Bacteriology of the southern gulf of mexico and yucatan channel area. Cien. Mar. 12, 21–34. doi: 10.7773/cm.v12i2.511
Loy, A., Duller, S., Baranyi, C., Mußmann, M., Ott, J., Sharon, I., et al. (2009). Reverse dissimilatory sulfite reductase as phylogenetic marker for a subgroup of sulfur-oxidizing prokaryotes. Environ. Microbiol. 11, 289–299. doi: 10.1111/j.1462-2920.2008.01760.x
Lueders, T., and Von Netzer, F. (2014). Primers: Functional Genes for Anaerobic Hydrocarbon Degrading Microbes, ed. T. K. N. B. Mcgenity (Berlin: Springer), 39–55.
Macdonald, I. R., Garcia-Pineda, O., Beet, A., Daneshgar Asl, S., Feng, L., Graettinger, G., et al. (2015). Natural and unnatural oil slicks in the Gulf of Mexico. J. Geophys. Res. 120, 8364–8380.
Magoč, T., and Salzberg, S. L. (2011). FLASH: fast length adjustment of short reads to improve genome assemblies. Bioinformatics 27, 2957–2963. doi: 10.1093/bioinformatics/btr507
Mason, O. U., Scott, N. M., Gonzalez, A., Robbins-Pianka, A., Bælum, J., Kimbrel, J., et al. (2014). Metagenomics reveals sediment microbial community response to deepwater horizon oil spill. ISME J. 8, 1464–1475. doi: 10.1038/ismej.2013.254
Mcglynn, S. E., Chadwick, G. L., Kempes, C. P., and Orphan, V. J. (2015). Single cell activity reveals direct electron transfer in methanotrophic consortia. Nature 526, 531–535. doi: 10.1038/nature15512
Mcmurdie, P. J., and Holmes, S. (2013). Phyloseq: an R package for reproducible interactive analysis and graphics of microbiome census data. PLoS One 8:e61217. doi: 10.1371/journal.pone.0061217
Mcmurdie, P. J., and Holmes, S. (2014). Waste not, want not: why rarefying microbiome data is inadmissible. PLoS Comput. Biol. 10:e1003531. doi: 10.1371/journal.pcbi.1003531
Meyer, B., and Kuever, J. (2007a). Molecular analysis of the distribution and phylogeny of dissimilatory adenosine-5’-phosphosulfate reductase-encoding genes (aprBA) among sulfur-oxidizing prokaryotes. Microbiology 153, 3478–3498. doi: 10.1099/mic.0.2007/008250-0
Meyer, B., and Kuever, J. (2007b). Phylogeny of the alpha and beta subunits of the dissimilatory adenosine-5’-phosphosulfate (APS) reductase from sulfate-reducing prokaryotes–origin and evolution of the dissimilatory sulfate-reduction pathway. Microbiology 153, 2026–2044. doi: 10.1099/mic.0.2006/003152-0
Milucka, J., Ferdelman, T. G., Polerecky, L., Franzke, D., Wegener, G., Schmid, M., et al. (2012). Zero-valent sulphur is a key intermediate in marine methane oxidation. Nature 491, 541–546. doi: 10.1038/nature11656
Moreno-Ulloa, A., Diaz, V. S., Tejeda-Mora, J. A., Macias, C. M. I., Díaz, C. F., Guerrero, A., et al. (2019). Metabolic and metagenomic profiling of hydrocarbon-degrading microorganisms obtained from the deep biosphere of the Gulf of México. bioRxiv doi: 10.1101/606806
Moreno-Vivián, C., Cabello, P., Martínez-Luque, M., Blasco, R., and Castillo, F. (1999). Prokaryotic nitrate reduction: molecular properties and functional distinction among bacterial nitrate reductases. J. Bacteriol. 181, 6573–6584. doi: 10.1128/jb.181.21.6573-6584.1999
Müller, A. L., Kjeldsen, K. U., Rattei, T., Pester, M., and Loy, A. (2015). Phylogenetic and environmental diversity of DsrAB-type dissimilatory (bi)sulfite reductases. ISME J. 9, 1152–1165. doi: 10.1038/ismej.2014.208
Muriel-Millán, L. F., Rodríguez-Mejía, J. L., Godoy-Lozano, E. E., Rivera-Gómez, N., Gutierrez-Rios, R. M., Morales-Guzmán, D., et al. (2019). Functional and genomic characterization of a Pseudomonas Aeruginosa strain isolated from the southwestern gulf of mexico reveals an enhanced adaptation for long-chain alkane degradation. Front. Mar. Sci. 6:1–15.
Nie, Y., Chi, C. Q., Fang, H., Liang, J. L., Lu, S. L., Lai, G. L., et al. (2014). Diverse alkane hydroxylase genes in microorganisms and environments. Sci. Rep. 4, 1–11.
Oberding, L., and Gieg, L. (2016). Metagenomic analyses reveal that energy transfer gene abundances can predict the syntrophic potential of environmental microbial communities. Microorganisms 4, 5–5.
Parks, D. H., Rinke, C., Chuvochina, M., Chaumeil, P. A., Woodcroft, B. J., Evans, P. N., et al. (2017). Recovery of nearly 8,000 metagenome-assembled genomes substantially expands the tree of life. Nat. Microbiol. 2, 1533–1542. doi: 10.1038/s41564-017-0012-7
Peng, Y., Leung, H. C. M., Yiu, S. M., and Chin, F. Y. L. (2010). IDBA–a practical iterative de bruijn graph de novo assembler BT. Res. Comput. Mol. Biol. 10, 426–440. doi: 10.1007/978-3-642-12683-3_28
Pinheiro, F. L., and Hartmann, D. (2017). Intermediate levels of network heterogeneity provide the best evolutionary outcomes. Sci. Rep. 7:15242.
Quast, C., Pruesse, E., Yilmaz, P., Gerken, J., Schweer, T., Yarza, P., et al. (2013). The SILVA ribosomal RNA gene database project: improved data processing and web-based tools. Nucleic Acids Res. 41, D590–D596.
Quinlan, A. R., and Hall, I. M. (2010). BEDTools: a flexible suite of utilities for comparing genomic features. Bioinformatics 26, 841–842. doi: 10.1093/bioinformatics/btq033
Rabus, R., Wilkes, H., Behrends, A., Armstroff, A., Fischer, T., Pierik, A. J., et al. (2001). Anaerobic initial reaction of n-alkanes in a denitrifying bacterium: evidence for (1-Methylpentyl)succinate as initial product and for involvement of an organic radical in n-hexane metabolism. J. Bacteriol. 183, 1707–1715. doi: 10.1128/jb.183.5.1707-1715.2001
Ramírez, D., Vega-Alvarado, L., Taboada, B., Estradas-Romero, A., Soto, L., and Juárez, K. (2020). Bacterial diversity in surface sediments from the continental shelf and slope of the north west gulf of mexico and the presence of hydrocarbon degrading bacteria. Mar. Pollut Bull. 150:110590. doi: 10.1016/j.marpolbul.2019.110590
Raymond, J., Siefert, J. L., Staples, C. R., and Blankenship, R. E. (2004). The natural history of nitrogen fixation. Mol. Biol. Evol. 21, 541–554.
Rodriguez, L. M., Will, A., Hagan, O. C., Huettel, M., Kostka, J. E., and Konstantinidis, K. T. (2015). Microbial community successional patterns in beach sands impacted by the deepwater horizon oil spill. ISME J. 9, 1928–1940. doi: 10.1038/ismej.2015.5
Rognes, T., Flouri, T., Nichols, B., Quince, C., and Mahé, F. (2016). VSEARCH: a versatile open source tool for metagenomics. PeerJ 2016, 1–22.
Rosano-Hernández, M. C., Ramírez-Saad, H., and Fernández-Linares, L. (2012). Petroleum-influenced beach sediments of the campeche bank, mexico: diversity and bacterial community structure assessment. J. Environ. Manag. 95, S325–S331.
Rotthauwe, J. H., Witzel, K. P., and Liesack, W. (1997). The ammonia monooxygenase structural gene amoa as a functional marker: molecular fine-scale analysis of natural ammonia-oxidizing populations. Appl. Environ. Microbiol. 63, 4704–4712. doi: 10.1128/aem.63.12.4704-4712.1997
Sanchez-Soto, M. F., Cerqueda-Garcia, D., Montero-Munoz, J. L., Aguirre-Macedo, M. L., and Garcia-Maldonado, J. Q. (2018). Assessment of the bacterial community structure in shallow and deep sediments of the perdido fold belt region in the gulf of mexico. PeerJ 6:e5583. doi: 10.7717/peerj.5583
Sanford, R. A., Wagner, D. D., Wu, Q., Chee-Sanford, J. C., Thomas, S. H., Cruz-García, C., et al. (2012). Unexpected nondenitrifier nitrous oxide reductase gene diversity and abundance in soils. Proc. Natl. Acad. Sci. U.S.A. 109, 19709–19714. doi: 10.1073/pnas.1211238109
Schmid, M. C., Hooper, A. B., Klotz, M. G., Woebken, D., Lam, P., Kuypers, M. M. M., et al. (2008). Environmental detection of octahaem cytochrome c hydroxylamine/hydrazine oxidoreductase genes of aerobic and anaerobic ammonium-oxidizing bacteria. Environ. Microbiol. 10, 3140–3149. doi: 10.1111/j.1462-2920.2008.01732.x
Shannon, P., Markiel, A., Ozier, O., Baliga, N. S., Wang, J. T., Ramage, D., et al. (2003). Cytoscape: a software environment for integrated models of biomolecular interaction networks. Genome Res. 13, 2498–2504. doi: 10.1101/gr.1239303
Sheik, C. S., Jain, S., and Dick, G. J. (2014). Metabolic flexibility of enigmatic SAR324 revealed through metagenomics and metatranscriptomics. Environ. Microbiol. 16, 304–317. doi: 10.1111/1462-2920.12165
Simon, J., and Klotz, M. G. (2013). Diversity and evolution of bioenergetic systems involved in microbial nitrogen compound transformations. Biochim. Biophys. Acta Bioenerge. 1827, 114–135. doi: 10.1016/j.bbabio.2012.07.005
Smith, C. J., Nedwell, D. B., Dong, L. F., and Osborn, A. M. (2007). Diversity and abundance of nitrate reductase genes (narG and napA), nitrite reductase genes (nirS and nrfA), and their transcripts in estuarine sediments. Appl. Environ. Microbiol. 73, 3612–3622. doi: 10.1128/aem.02894-06
Stach, P., Einsle, O., Schumacher, W., Kurun, E., and Kroneck, M. H. (2000). Bacterial cytochrome c nitrite reductase: new structural and functional aspects. J. Inorg. Biochem. 79, 381–385. doi: 10.1016/s0162-0134(99)00248-2
Stagars, M. H., Emil Ruff, S., Amann, R., and Knittel, K. (2016). High diversity of anaerobic alkane-degrading microbial communities in marine seep sediments based on (1-methylalkyl)succinate synthase genes. Front. Microbiol. 6, 1–17.
Swan, B. K., Martinez-Garcia, M., Preston, C. M., Sczyrba, A., Woyke, T., Lamy, D., et al. (2011). Potential for chemolithoautotrophy among ubiquitous bacteria lineages in the dark ocean. Science 333, 1296–1300. doi: 10.1126/science.1203690
Thompson, L. R., Sanders, J. G., Mcdonald, D., Amir, A., Ladau, J., Locey, K. J., et al. (2017). A communal catalogue reveals Earth’s multiscale microbial diversity. Nature 551, 457–463.
Timmers, P. H. A., Welte, C. U., Jasper, J. K., Plugge, C. M., Mike, S. M. J., and Stams, A. J. M. (2017). Reverse methanogenesis and respiration in methanotrophic archaea. Archaea 2017:1654237.
Tully, B. J., Graham, E. D., and Heidelberg, J. F. (2018). The reconstruction of 2,631 draft metagenome-assembled genomes from the global oceans. Sci. Data 5, 1–8.
Uniprot Consortium (2018). UniProt: a worldwide hub of protein knowledge. Nucleic Acids Res. 47, D506–D515. doi: 10.1093/nar/gky1049
Van Beilen, J. B., and Funhoff, E. G. (2007). Alkane hydroxylases involved in microbial alkane degradation. Appl. Microbiol. Biotechnol. 74, 13–21. doi: 10.1007/s00253-006-0748-0
Vigneron, A., Alsop, E. B., Cruaud, P., Philibert, G., King, B., Baksmaty, L., et al. (2019). Contrasting pathways for anaerobic methane oxidation in gulf of mexico cold seep sediments. mSystems 4:e00091-18.
Wang, Y., Wegener, G., Ruff, S., and Wang, F. (2020). Methyl/alkyl−coenzyme M reductase−based anaerobic alkane oxidation in archaea. Environ. Microbiol. 20:15057. doi: 10.1111/1462-2920.15057
Wood, D. E., and Salzberg, S. L. (2014). Kraken: Ultrafast metagenomic sequence classification using exact alignments. Genome Biol. 15:46.
Wörner, S., and Pester, M. (2019). The active sulfate-reducing microbial community in littoral sediment of oligotrophic lake constance. Front. Microbiol. 10:247. doi: 10.3389/fmicb.2019.00247
Yakimov, M. M., Timmis, K. N., and Golyshin, P. N. (2007). Obligate oil-degrading marine bacteria. Curr. Opin. Biotechnol. 18, 257–266. doi: 10.1016/j.copbio.2007.04.006
Yamamoto, M., and Takai, K. (2011). Sulfur metabolisms in epsilon-and gamma-proteobacteria in deep-sea hydrothermal fields. Front. Microbiol. 2, 1–8.
Zhu, W., Lomsadze, A., and Borodovsky, M. (2010). Ab initio gene identification in metagenomic sequences. Nucleic Acids Res. 38:e132. doi: 10.1093/nar/gkq275
Keywords: Gulf of Mexico, bacterial community structure, metagenome, metabolic potential, hydrocarbon degradation
Citation: Raggi L, García-Guevara F, Godoy-Lozano EE, Martínez-Santana A, Escobar-Zepeda A, Gutierrez-Rios RM, Loza A, Merino E, Sanchez-Flores A, Licea-Navarro A, Pardo-Lopez L, Segovia L and Juarez K (2020) Metagenomic Profiling and Microbial Metabolic Potential of Perdido Fold Belt (NW) and Campeche Knolls (SE) in the Gulf of Mexico. Front. Microbiol. 11:1825. doi: 10.3389/fmicb.2020.01825
Received: 14 May 2020; Accepted: 10 July 2020;
Published: 11 August 2020.
Edited by:
Tony Gutierrez, Heriot-Watt University, United KingdomReviewed by:
Anirban Chakraborty, University of Calgary, CanadaJennifer Pratscher, The Lyell Centre, United Kingdom
Copyright © 2020 Raggi, García-Guevara, Godoy-Lozano, Martínez-Santana, Escobar-Zepeda, Gutierrez-Rios, Loza, Merino, Sanchez-Flores, Licea-Navarro, Pardo-Lopez, Segovia and Juarez. This is an open-access article distributed under the terms of the Creative Commons Attribution License (CC BY). The use, distribution or reproduction in other forums is permitted, provided the original author(s) and the copyright owner(s) are credited and that the original publication in this journal is cited, in accordance with accepted academic practice. No use, distribution or reproduction is permitted which does not comply with these terms.
*Correspondence: Lorenzo Segovia, lorenzo@ibt.unam.mx; Katy Juarez, katy@ibt.unam.mx
†These authors have contributed equally to this work