- Instituto Español de Oceanografía (IEO), Centre Oceanogràfic de les Balears, Ecosystem Oceanography Group (GRECO), Palma, Spain
Surface microbial communities are exposed to seasonally changing environmental conditions, resulting in recurring patterns of community composition. However, knowledge on temporal dynamics of open ocean microbial communities remains scarce. Seasonal patterns and associations of taxa and oligotypes from surface and chlorophyll maximum layers in the western Mediterranean Sea were studied over a 2-year period. Summer stratification versus winter mixing governed not only the prokaryotic community composition and diversity but also the temporal dynamics and co-occurrence association networks of oligotypes. Flavobacteriales, Rhodobacterales, SAR11, SAR86, and Synechococcales oligotypes exhibited contrasting seasonal dynamics, and consequently, specific microbial assemblages and potential inter-oligotype connections characterized the different seasons. In addition, oligotypes composition and dynamics differed between surface and deep chlorophyll maximum (DCM) prokaryotic communities, indicating depth-related environmental gradients as a major factor affecting association networks between closely related taxa. Taken together, the seasonal and depth specialization of oligotypes suggest temporal dynamics of community composition and metabolism, influencing ecosystem function and global biogeochemical cycles. Moreover, our results indicate highly specific associations between microbes, pointing to keystone ecotypes and fine-tuning of the microbes realized niche.
Introduction
Marine microbes are crucial players of ocean element cycles and modulate global biogeochemical processes (Azam and Malfatti, 2007; Fuhrman, 2009; Moran, 2015). Autotrophic prokaryotes account for 8–19% of primary production in the sunlit oligotrophic ocean regions (Rousseaux and Gregg, 2013), contributing significantly to the export of carbon and energy that fuels the dark ocean communities (Johnson and Lin, 2009; Guidi et al., 2016). Prokaryotic communities transfer energy to other trophic levels through grazing (Pernthaler, 2005; Vaque et al., 2014), viral lysis (Suttle, 2007; Chen et al., 2019), and organic matter decomposition and remineralization (Kujawinski, 2011; Buchan et al., 2014).
Marine surface microbial communities show remarkable seasonal dynamics, driven by environmental seasonality patterns (Fuhrman et al., 2015; Bunse and Pinhassi, 2017). Temperature, light intensity, nutrient availability, water mixing, and stratification are seasonally related factors that regulate microbial community dynamics (Morris et al., 2005; Malmstrom et al., 2010; Gilbert et al., 2012; Sintes et al., 2013; Pearman et al., 2017), originating recurrent patterns in community composition and functionality (Giovannoni and Vergin, 2012; Teeling et al., 2012; Fuhrman et al., 2015). Growth rates and activity of specific prokaryotic taxa are also strongly influenced by seasonal fluctuations (Alonso-Sáez and Gasol, 2007; Hugoni et al., 2013).
Additionally, community structure is influenced by microbial interactions (Faust and Raes, 2012). A plethora of organism interactions have been evidenced that alter community metabolism and function (Azam and Malfatti, 2007; Fuhrman, 2009; Teira et al., 2019). Interactions can result in positive, negative, or neutral impact on the organisms involved, including mutualism, commensalism, parasitism or predation, and amensalism (Faust and Raes, 2012). Quorum sensing is a microbe interaction mechanism through chemical communication involved in different processes of marine biogeochemical cycles, trophic interactions and biofilm formation and functioning (Hmelo, 2017). The release of extracellular products or metabolites derived from microorganism’s metabolism may also result in microbe-microbe interactions (Li et al., 2015; Reintjes et al., 2019). Free amino acids and other organic compounds released by phytoplankton and zooplankton are a significant energy and carbon source for marine prokaryotes (Williams et al., 2013; Buchan et al., 2014; Clifford et al., 2019). Alteromonadaceae release exo-enzymes to hydrolyze complex compounds into simpler molecules, which can potentially be assimilated by other microbes such as SAR11 (Reintjes et al., 2019). Symbiosis between cyanobacteria and eukaryotic algae has also been reported (Martínez-Pérez et al., 2016). Interactions may also be negative for one or both microbes involved, e.g., competition for limiting resources (Teira et al., 2019), predation (Pernthaler, 2005; Banning et al., 2010) or release of inhibitory compounds (Long and Azam, 2001). Alteromonadales and Vibrionales, either free-living or surface-attached, produce and release inhibitory compounds, strongly reducing Bacteroidetes growth (Long and Azam, 2001). However, interactions between microbes are challenging to resolve.
Correlation-based network-analysis (Liu et al., 2019) is receiving increased attention as a tool to advance our knowledge of connectivity between microbial taxa. Studies based on operational taxonomic units (OTUs) have revealed seasonal cycles and co-occurrence patterns among ammonia-oxidizing archaea, SAR11, SAR86, Cyanobacteria, Actinobacteria, and Flavobacteria microbial OTUs and other common groups (Beman et al., 2011; Chow et al., 2013), outlining potential ecological networks. Nevertheless, the association (positive or negative) between microbes can be caused by the interplay between organisms but also by a similar response of these organisms to the environmental conditions, not involving direct microbe interactions.
In addition, the ecological significance of taxa, specially at high taxonomic ranks, is still debated (Philippot et al., 2010). A recent approach developed to resolve closely related groups of ecological significance is oligotyping analysis (Eren et al., 2013). This analysis identifies varying positions in nucleotide (Eren et al., 2013) or protein (Sintes et al., 2016) sequences of specific taxonomic groups, generating oligotypes and revealing different ecological patterns within the specific taxon (i.e., ecotypes). Seasonal recurring dynamics have been observed between SAR11 ecotypes, related to winter physical mixing and stratification of the water column (Treusch et al., 2009; Vergin et al., 2013a; Salter et al., 2015). Abundant and rare archaeal ecotypes have also shown seasonal patterns, exhibiting temporal fluctuations in abundance and activity (Hugoni et al., 2013). Seasonal changes in light, temperature, and water mixing drive temporal patterns of depth-differentiated Prochlorococcus ecotypes (Malmstrom et al., 2010). Information on temporal dynamics of ecotypes is essential to determine ecological niches and fine-scale temporal community dynamics, extending our knowledge on microbial interactions and ecosystem functioning. However, studies on ecotype seasonality, specially of open ocean communities, are still scarce.
The aims of the study were to (i) further characterize seasonal and spatial dynamics of prokaryotic communities and oligotypes of abundant taxa from surface and chlorophyll maximum layers in the open western Mediterranean Sea and (ii) assess inter-oligotype connections. Oligotyping analysis was performed on 16S ribosomal RNA (rRNA) gene sequences and correlation-based networks were obtained. The results provide new insights into the temporal niche partitioning and associations of prokaryotic phylotypes.
Materials and Methods
Study Site and Sampling
The sampling was carried out seasonally from February 2016 to December 2017 at five stations in the western Mediterranean Sea during the RADMED cruises. Campaigns in February, April, July, and October 2016 and February, June, and November 2017 were, hereinafter, named Feb16, Apr16, Jul16, Oct16, Feb17, Jun17, and Nov17, respectively. Campaigns were grouped as follows, in order to discuss the seasonal patterns: Feb16 and Feb17 campaigns are referred to as winter, Apr16 as spring, Jul16 and Jun17 as summer, and Oct16 and Nov17 campaigns as autumn. Specific sampling dates for each station are detailed in Supplementary Table S1. The stations were located in different regions of the basin: north Balearic sub-basin (A), Mallorca channel (B), south Balearic sub-basin (C), north Algerian sub-basin (D), and south Algerian sub-basin (E) (Supplementary Figure S1).
Seawater from the upper 200 m was collected using Niskin bottles mounted on a SBE911 conductivity-temperature-depth (CTD) rosette sampler equipped with oxygen (SBE43), Chlorophyll-a (Chl-a) fluorescence (SeaPoint Fluorometer) and photosynthetically active radiance (PAR and SPAR Biospherical) sensors. The thermocline was defined as the layer at the base of the surface mixed layer in which the temperature vertical gradient was more pronounced. Water samples for inorganic nutrients, Chl-a, picophytoplankton abundances, and total prokaryotic abundance were collected at 0, 25, 50, 75, 100, and 200 m and at the deep chlorophyll maximum (DCM) depth. The prokaryotic community composition was assessed at 0 m and DCM depth.
Inorganic Nutrients and Chlorophyll-a
Twelve milliliter of seawater for dissolved inorganic nutrients was collected and stored frozen at −20°C until further analysis at the home lab. Nitrate (NO3−), nitrite (NO2−), phosphate (PO43−), and silicate (SiO42−) concentrations were analyzed with a QuAAtro gas segmented continuous flow analyzer (SEAL analytical) following standard methods (Murphy and Riley, 1962; Strickland and Parsons, 1968; Grasshoff et al., 1983). Detection limits were 0.023, 0.01, 0.007, and 0.030 μM for nitrate + nitrite, nitrite, phosphate, and silicate, respectively.
Chl-a concentration was measured spectrophotometrically after filtering 1 L of seawater onto GF/F Whatman glass fiber filters. Chl-a from microbes retained on the filters was extracted in cold acetone (90%) for 24 h and analyzed with a turner-designs 10AU fluorometer. Phaeopigments concentration was analyzed after adding two drops of 1.2 N HCl. The measurements prior to and after acidification were used to calculate concentration of Chl-a and phaeopigments, respectively (Holm-Hansen et al., 1965; Lorenzen, 1967).
Picophytoplankton and Total Prokaryotic Abundances
Microbial abundances were measured by flow cytometry. Seawater (1.5 ml) was fixed with glutaraldehyde (0.1% final concentration) for 10 min, frozen in liquid nitrogen and stored at −80°C until further analysis. Prior to enumeration, fluorescent beads (Fluospheres polystyrene 1.0 μm, Molecular probes) were added to all samples as internal standard.
Picophytoplankton groups were counted on a FACSAria II flow cytometer (BD Biosciences). Prochlorococcus, Synechococcus, and picoeukaryotes were differentiated based on their side scatter versus red fluorescence and orange fluorescence versus red fluorescence signals (Marie et al., 2000). Cytogram plots were used to draw a gate for each group with the BD FACSDiva software. Flow rate was measured daily.
Total prokaryotes were enumerated on an ACCURI C6 flow cytometer (BD Biosciences), after staining with SYBR Green I (Sigma-Aldrich, 1× final concentration) for 10 min in the dark. High nucleic acid (HNA) and low nucleic acid (LNA) content cells were separated based on their side scatter versus green fluorescence signals (Brussaard, 2004). Gating on cytogram plots was adjusted for each sample.
Prokaryotic Community Composition
Four liters of seawater were filtered onto polycarbonate filters (0.2 μm pore size, 47 mm diameter, Whatman, Nucleopore), immediately frozen in liquid nitrogen and stored at −80°C. At the lab, filters were thawed, cut into small pieces and incubated 45 min at 37°C with lysis buffer containing lysozyme. Subsequently, filter pieces were incubated 1 h at 55°C with proteinase K. Zirconium beads were added and the lysate was subjected to beat-beating for 10 min, followed by 30 min incubation at 70°C. Subsequently, DNA was extracted successively with phenol (pH8), phenol-chloroform-isoamylalcohol (25:24:1), and chloroform. DNA was precipitated with two volumes of cold ethanol and 0.02 volumes of 5 M NaCl at −20°C overnight. DNA was pelleted by centrifugation (25 min, 21,000 × g), washed with cold ethanol (70°C), resuspended in sterile DNAse-RNAse free water and stored frozen at −80°C until further analysis.
16S rRNA gene of Archaea and Bacteria was amplified using primers 515F-Y (5'-GTGYCAGCMGCCGCGGTAA) and 926R (5'-CCGYCAATTYMTTTRAGTTT) (Parada et al., 2016). PCR cycling was performed following Parada et al. (2016). PCR products were purified using the Qiaquick PCR purification kit (Qiagen, Hilden) according to manufacturer’s instructions, and checked on a 2% agarose gel. Sequencing was performed with Illumina MiSeq 2 × 250 bp and analyzed using QIIME21. Quality check, denoise, and dereplication of paired-end sequence reads were performed using DADA2 algorithm (Callahan et al., 2016) implemented in QIIME2. Sequences with ambiguities were removed (maxN = 0) and the length of forward and reverse reads was truncated to 245 and 230 bp, respectively. After filtering, a total number of 547,992 sequences were retained for further analyses, ranging from 4,707 to 13,709 sequences per sample. The obtained amplicon sequence variants (ASVs) were aligned with multiple alignment using fast Fourier transform (MAFFT) (Katoh and Standley, 2013) and a phylogenetic tree was built with Fasttree (Price et al., 2010). The ASVs table, rarefied to 4,707 sequences, was used to calculate Shannon’s diversity, Pielou’s evenness, phylogenetic diversity indexes, and unweighted UniFrac distances. Taxonomic assignment was obtained based on the SILVA database (release 132). 16S rRNA raw sequences were deposited in the NCBI database, accession number PRJNA612168.
Oligotyping
Oligotyping analysis was performed on the most abundant phylotypes at the order level (>3% relative abundance in the photic layer), including main representatives of the phyla/classes of both Archaea and Bacteria: SAR11, Synechococcales, Chloroplast, Flavobacteriales, Marine Group II, Rhodobacterales, SAR86, Actinomarinales, and Alteromonadales, following the pipeline described in http://oligotyping.org. Oligotyping identifies closely related taxa consistently differing in nucleotide composition at specific positions (Eren et al., 2013), helping resolve ecologically significant populations. We complemented ASVs with oligotyping analysis in order to group ASVs based on an information-theoretic approach instead of arbitrary sequence identity thresholds and to infer ecologically meaningful groups (i.e., ecotypes). Between 7 and 14 entropy positions were used to assess the oligotypes of the abovementioned phylotypes (see Table 1). Oligotypes that occurred in more than 0.5% of reads (a = 0.5) were considered.
Statistical Analyses
Analysis of variance (ANOVA) was used to assess statistically significant differences in environmental and abundance data. The “aov” function of the “vegan” package of R with a significance level of p < 0.05 was used. Distance-based redundancy analysis (db-RDA) was used to evaluate the prokaryotic community variation based on unweighted UniFrac distances. Only non-colinear variables were selected as explanatory variables based on the variance inflation factor (VIF, Zuur et al., 2009) analysis, i.e., temperature, salinity, nitrate, nitrite, phosphate, Chl-a, phaeopigments, station, season, depth, and year. PAR was not included in the analysis due to limited data availability (18 depth profiles out of a total of 31 were available; missing PAR values were due to night measurements or sensor malfunction). The explained variation and the significance of the explanatory variables were assessed using “dbrda” and “anova.cca” functions of the “vegan” package of R. Variation partitioning was applied to the db-RDA model to discern the variability explained by environmental, spatial, or temporal variables using “varpart” function of the “vegan” package of R. Environmental variables included temperature, salinity, and inorganic nutrients concentrations; spatial variables included depth and station; and temporal variables included season and year. Prokaryotic community was further analyzed using db-RDA analysis for surface and DCM communities separately. Due to collinearity, silicate and Chl-a were excluded from the surface model and nitrate was excluded from the DCM model. RDA was used to relate oligotypes composition with environmental parameters. Same explanatory variables selected for the db-RDA prokaryotic community model were used. Pearson correlation was used to reveal connectivity of oligotypes for surface and DCM communities separately based on their relative abundance. Significantly, correlated oligotypes (p < 0.05) were visualized in a network using the Cytoscape open-source platform (Shannon et al., 2003). Subsequently, the resulting networks were referred to as association networks, as they include both positive (co-occurrence, co-presence) and negative correlations (non-coexistence or exclusion).
Results
Physicochemical and Biological Temporal and Spatial Variability
Temperature ranged between 13 and 15°C throughout the water column in winter and increased to 16–17°C at surface in spring. During the summer stratification, surface temperature reached 23–26°C and remained at 13–15°C below the thermocline (>50 m). The thermocline was disrupted in autumn, with temperature ranging between 18–22 and 13–22°C at surface and below 50 m, respectively (Supplementary Figure S2A). Available PAR data did not show significant differences between seasons at surface (0–10 m), ranging between 4.2 and 79.5%. However, PAR reached 5.4 ± 1.6% (mean ± SE) at the DCM depth in winter and 1.9 ± 0.5% in the other seasons (Supplementary Figure S2E).
No significant differences in inorganic nutrient concentrations were found between winter surface and DCM waters, ranging 0–3.07, 0–0.20, 0.01–0.14, and 0.59–2.26 μM for nitrate, nitrite, phosphate, and silicate, respectively. Surface nutrient concentrations were significantly lower than DCM concentrations in spring, summer, and autumn, varying 0–0.05, 0–0.13, 0–0.04, and 0.37–1.15 μM for nitrate, nitrite, phosphate and silicate, respectively, at surface, and between 0.01–3.13, 0.01–0.27, 0–0.10, and 0.53–2.06 μM for nitrate, nitrite, phosphate, and silicate, respectively, at DCM (Supplementary Figure S3).
Water column maximum Chl-a fluorescence values were observed at increasing depths from winter to summer (Supplementary Figure S2D). Chl-a concentration ranged between 0.34 and 1.07 mg m−3 in winter and peaked at 0–30 m. In spring, Chl-a ranged between 0.23 and 1.55 mg m−3 with maximum at 37–40 m. Subsequently, Chl-a varied between 0.42 and 1.34 mg m−3 and its maximum deepened to 58–100 m in summer, rising up to 47–58 m depth in autumn with concentrations ranging between 0.20 and 1.07 mg m−3 (Supplementary Figure S2D).
Seasonal Patterns of Microbial Communities
Seasonality of picophytoplankton groups (Figure 1) was statistically significant when depth layer was considered (Season*Depth, p < 0.001, ANOVA). The geographic location (station) was not significantly related to abundance variations (p > 0.05, ANOVA). Synechococcus was more abundant at the upper 25 m in winter (Feb16 and Feb17) and autumn (Oct16 and Nov17) (2.67 ± 0.21 × 104 cells ml−1, mean ± SE) compared to deeper layers (Figure 1A; Supplementary Figure S4). Synechococcus surface abundance (0–25 m) decreased to 0.86 ± 0.08 × 104 cells ml−1 in summer (Jul16 and Jun17), concurrently increasing to 1.96 ± 0.36 × 104 cells ml−1 at 50–75 m (Supplementary Figure S4). Prochlorococcus were always more abundant below 50 m than at upper layers, and increased significantly at DCM-75 m in summer (2.30 ± 0.43 × 104 cells ml−1; Figure 1B; Supplementary Figure S4). Picoeukaryotes abundance peaked at surface and DCM during winter (4.99 ± 0.60 × 103 cells ml−1) and at DCM in spring (Apr16, 5.78 ± 1.59 × 103 cells ml−1), exhibiting lower abundances at other depths (on average 1.38 ± 0.19 × 103 cells ml−1) and seasons (on average 1.84 ± 0.30 × 103 cells ml−1) (Figure 1C; Supplementary Figure S4).
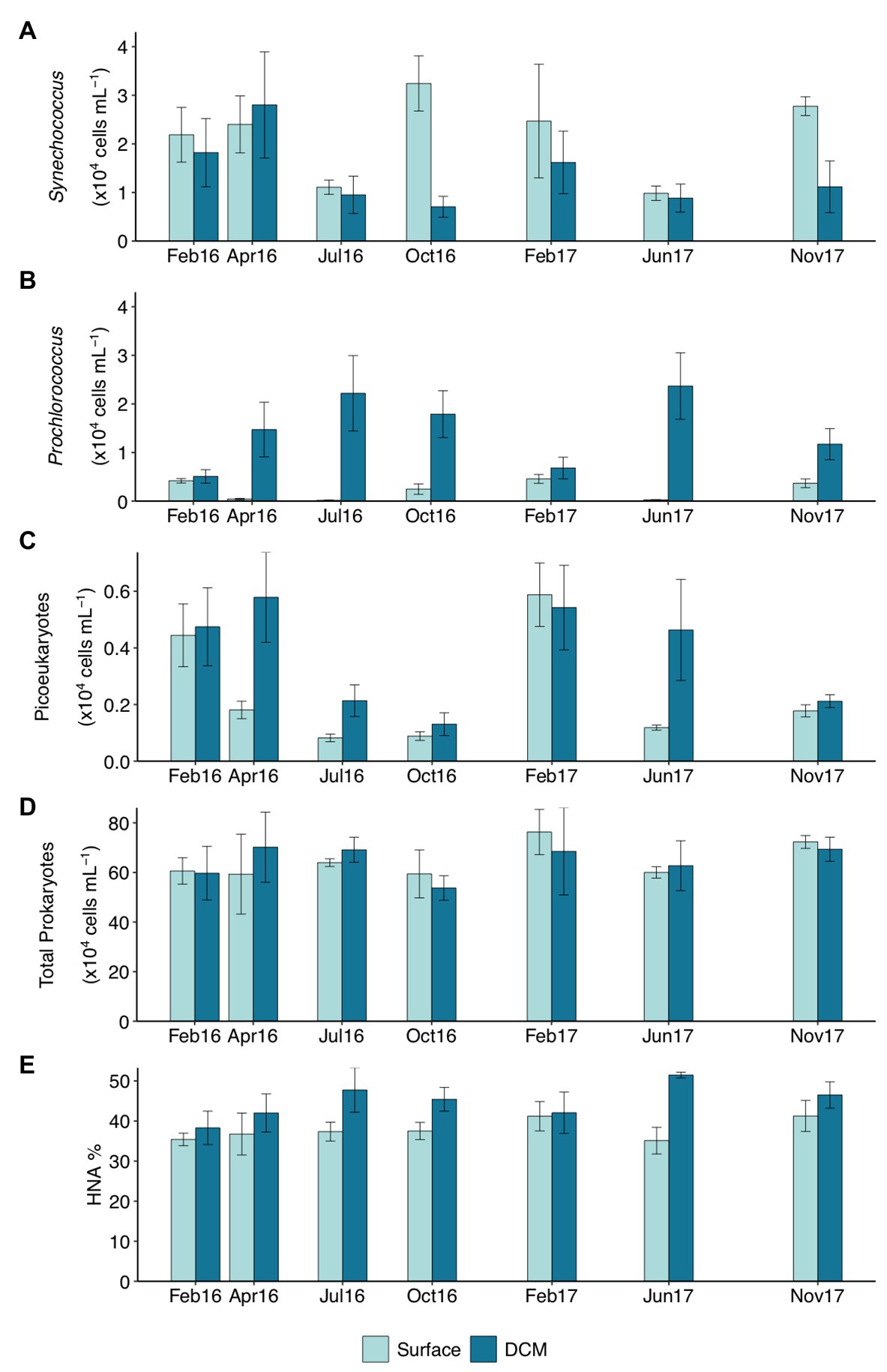
Figure 1. Abundance (×104 cells ml−1) of (A) Synechococcus, (B) Prochlorococcus, (C) picoeukaryotes, (D) total prokaryotes, and (E) percentage of high nucleic acid (HNA) cells throughout the studied period in surface and deep chlorophyll maximum (DCM) waters. Notice different scales for C–E. Bar height indicates the corresponding average value of all the stations at the specific depth and campaign, vertical lines indicate the standard error. Feb16 and Feb17 correspond to winter season, Apr16 to spring, Jul16 and Jun17 to summer, and Oct16 and Nov17 to autumn. DCM, deep chlorophyll maximum.
Total prokaryotic abundance decreased with depth, ranging between 6.40 ± 0.27 × 105 cells ml−1 at surface and 2.21 ± 0.16 × 105 cells ml−1 at 200 m. However, prokaryotes abundance increased in summer up to 9.84 × 105 cells ml−1 at 50 and 75 m (Supplementary Figure S4). Relative abundance of HNA cells increased with depth from 37.61 ± 1.16% HNA at surface to 49.67 ± 1.52% HNA at 200 m (Supplementary Figure S4 and Supplementary Table S1). Surface HNA relative abundance was significantly different from DCM in summer (Figure 1E).
Prokaryotic Community Composition
16S rRNA gene sequence analysis revealed a total of 6,727 ASVs. Fourteen percent of ASVs were shared between surface and DCM communities during the winter mixing period, decreasing to 10% in spring and to 4.3% in summer (Supplementary Figure S5). Subsequently, shared ASVs between surface and DCM increased to 11% in autumn (Supplementary Figure S5). Higher Shannon diversity index and evenness were observed in DCM than in surface communities (p < 0.01, ANOVA; Supplementary Figure S6). However, only surface communities showed significant seasonal diversity dynamics. Shannon index of surface prokaryotic communities peaked in winter and was minimum in summer (p < 0.05, ANOVA; Supplementary Figure S6).
Clustering of the communities according to depth (p < 0.05) and season (p < 0.01) was noticeable based on unweighted UniFrac distances (Figure 2). The first two RDA axes were significant (p = 0.001), explaining 40.8% of the constrained variation (Figure 2; Supplementary Table S2). Surface and DCM communities clustered together in winter and differed in other seasons, more pronouncedly in summer (Figure 2). Temperature and inorganic nutrients concentrations were significantly correlated to the first db-RDA axis (p < 0.01), whereas the sampling year (p < 0.05) correlated with the second db-RDA axis (Figure 2; Supplementary Table S2). Variation partitioning supported a main role of environmental variables on community composition, accounting for 67% of the explained variability, 33 and 17% of which was shared with spatial and temporal variability, respectively (Supplementary Figure S7). Community composition variability explained uniquely by environmental, temporal (season and year), and spatial (station and depth) variables separately accounted for 17, 17, and 4%, respectively (Supplementary Figure S7). The db-RDA analyses of surface and DCM communities separately indicated that temperature and season influenced significantly prokaryotic community structure throughout the photic zone (p < 0.01; Supplementary Figure S8 and Supplementary Table S3). Nitrite significantly affected surface communities (p < 0.05), while silicate (p < 0.05) and Chl-a (p < 0.01) were significant for DCM communities (Supplementary Figure S8 and Supplementary Table S3).
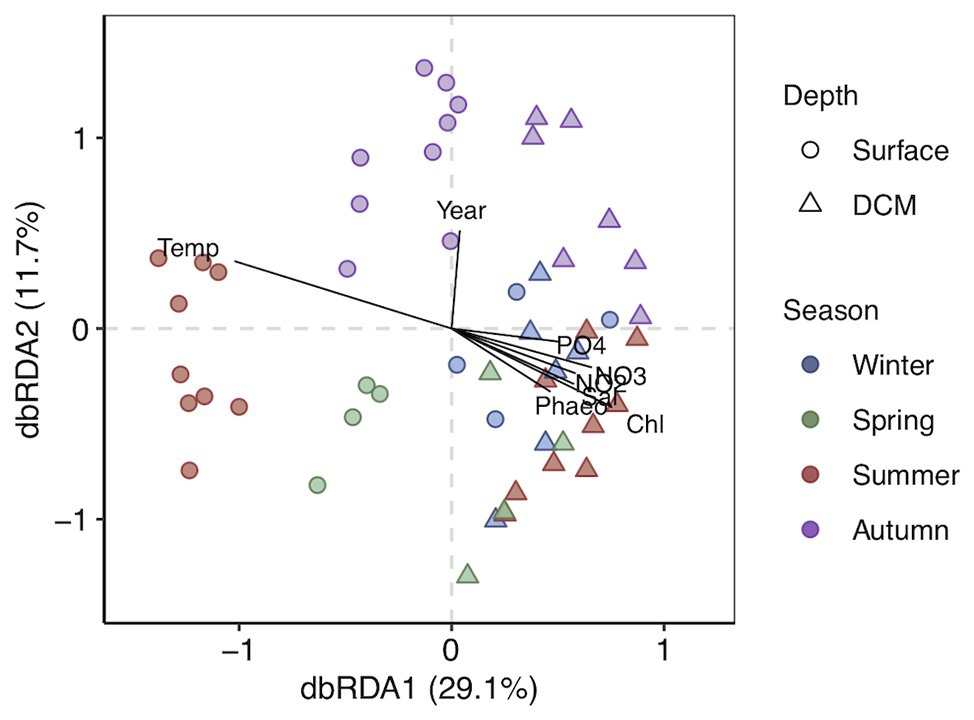
Figure 2. Distance-based redundancy analysis (db-RDA) based on unweighted UniFrac distances with constraint variables. The percentage of variance explained is shown for each axis. Vectors represent the constrained continuous variables used in the RDA model. Strength of relative correlations with RDA axes is indicated by the length and direction of vectors. Shapes indicate depth layer and colors indicate seasons.
The photic zone prokaryotic communities were dominated by SAR11 Clade I, Flavobacteriaceae, and Cyanobiaceae, contributing 14.8% (±0.8), 12% (±0.6), and 6.7% (±0.6) at surface and 10.7% (±0.5), 10.4% (±0.9), and 4.8% (±0.3) at DCM [mean (±SE)], respectively (Figure 3). Chloroplast families contributed on average 7.4% (±1.4) at surface and 7.8% (±1.2) at DCM communities, increasing in winter to 17.7% (±4.8) and 11.1% (±2.8) at surface and DCM, respectively. SAR86 families also contributed considerably to photic zone communities on average 6.4% (±0.5) at surface and 4.1% (±0.3) at DCM. SAR116 (Puniceispirillales) were more abundant at surface (6.3 ± 0.5%) than at DCM (2.1 ± 0.2%) waters, peaking during spring and summer. Nitrosopumilales and Marine Group II families contributed more to DCM communities, 3.9% (±0.4) and 4.2% (±0.4), respectively, than to surface communities, contributing 0.5% (±0.2) and 1.5% (±0.4) on average, respectively (Figure 3).
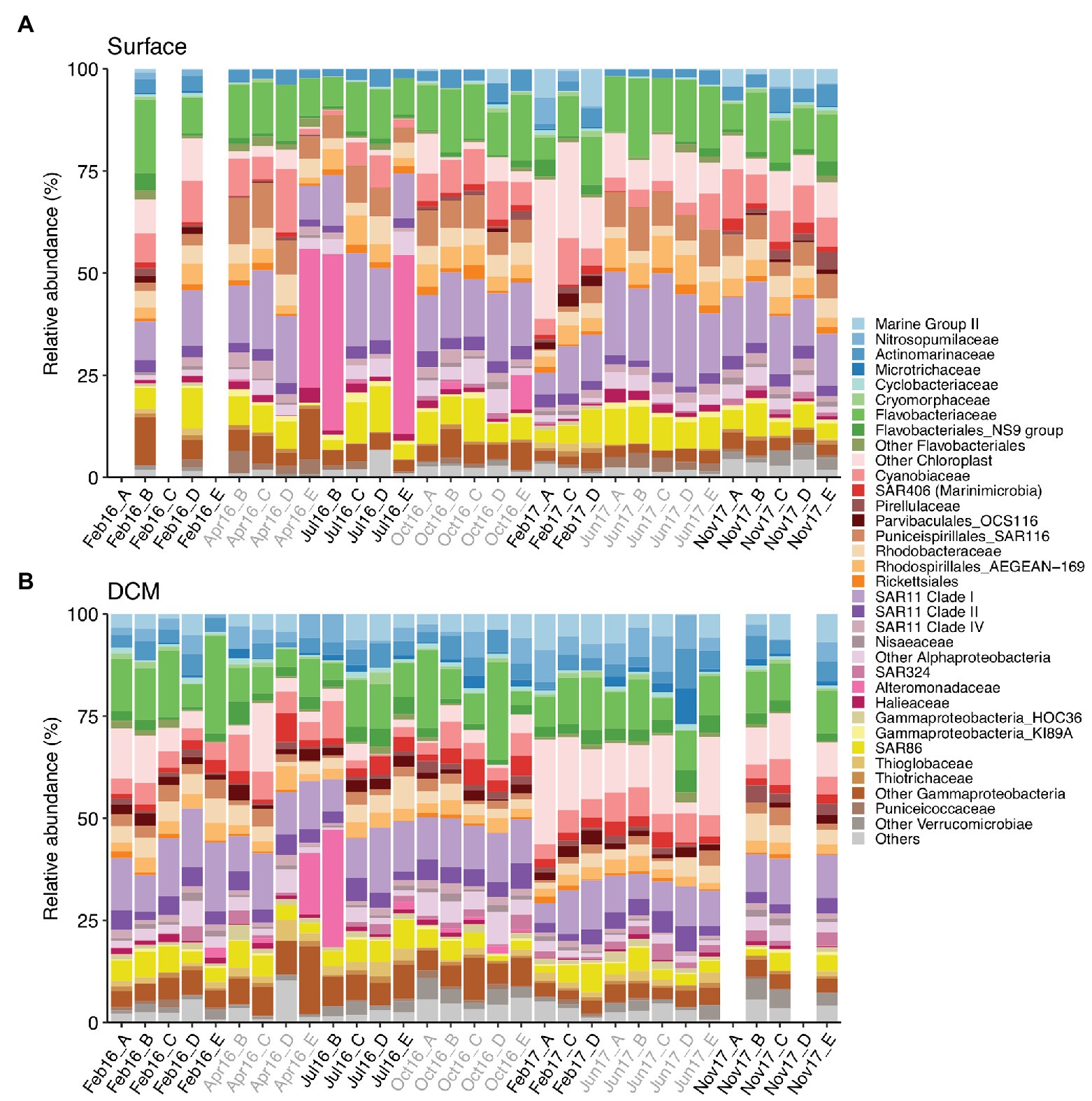
Figure 3. Prokaryotic community composition at family level at (A) surface and (B) DCM from February 2016 to November 2017. Samples are labeled according to cruise and station (Cruise_Station). Phylotypes contributing ≤0.6% are combined in “Others” group. Legend indicates order_family levels. Cruise correspondence to seasons as in Figure 1. DCM, deep chlorophyll maximum.
Alteromonadaceae comprised on average 0.4% (±0.2) of the photic zone community. However, Alteromonadaceae increased drastically its contribution to definite spring-summer communities from surface and DCM, accounting for 15–43.7% of the community (Figure 3). Other family phylotypes considerably contributing to photic zone communities were Rhodobacteraceae (4.1 ± 0.2% at surface and 3.7 ± 0.3% at DCM), AEGEAN-169 group from Rhodospirillales (4.4 ± 0.3% at surface and 2.9 ± 0.2% at DCM), Actinomarinaceae (3.1 ± 0.2% at surface and 3.8 ± 0.3% at DCM), SAR11 Clade II (2.7 ± 0.1% at surface and 3.8 ± 0.2% at DCM), and Clade IV (2 ± 0.1% at surface and 1.3 ± 0.1% at DCM). OCS116 from Parvibaculales exhibited pronounced seasonal variation at surface (2.1 ± 0.3% in winter and 0.2 ± 0.1% in other seasons), whereas steady abundances at DCM were obtained throughout the study (1.9 ± 0.1%) (Figure 3).
Oligotypes Composition
Oligotyping analysis revealed between 22 and 103 distinct oligotypes for the selected phylotypes examined (Table 1). Oligotypes composition of all phylotypes was remarkably different between surface and DCM (Figure 4; Supplementary Figure S9).
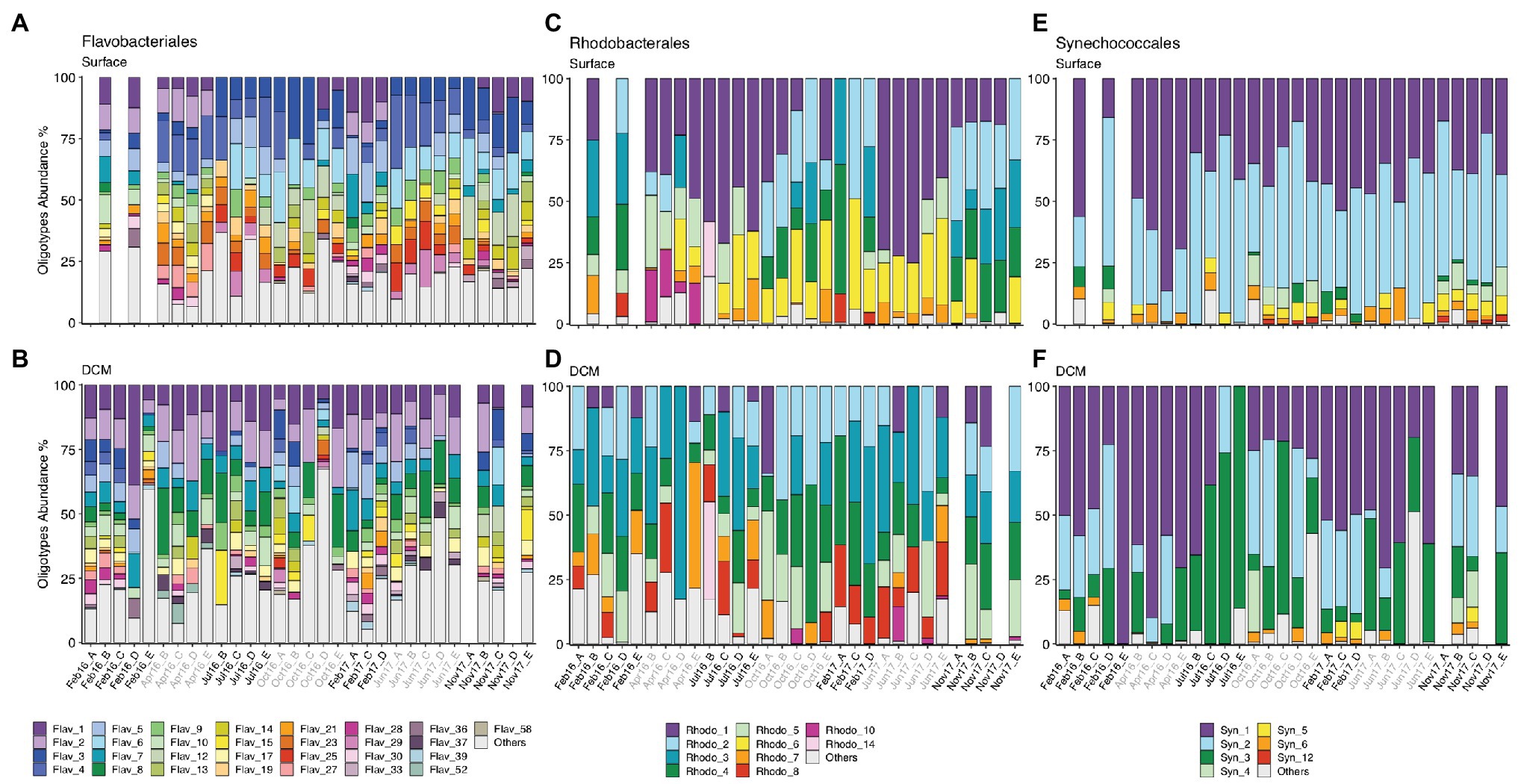
Figure 4. (A,B) Flavobacteriales, (C,D) Rhodobacterales, and (E,F) Synechococcales oligotype composition from surface and DCM from February 2016 to November 2017. Samples are labeled according to cruise and station (Cruise_Station). Cruise correspondence to seasons as in Figure 1. Legends indicate phylotype_oligotype number for each phylotype. Oligotypes that show no temporal pattern are combined in “Others” group. Missing bars indicate missing samples. DCM, deep chlorophyll maximum.
Flavobacteriales, Rhodobacterales, SAR11, Synechococcales, SAR86, and Actinomarinales exhibited seasonal patterns at surface communities, evidenced by appearance or disappearance of specific oligotypes as well as changes in relative abundance of the most abundant oligotypes in different seasons (Figure 4; Supplementary Figure S9). Flavobacteriales oligotypes 1 and 2 disappeared of surface samples during summer and autumn (Jul16, Oct16, Jun17, and Nov17), when stratification prevailed, while the relative abundance of other oligotypes increased (Figure 4A). Similarly, some surface Rhodobacterales oligotypes (e.g., oligotypes 2 and 4) retreated during summer stratification (Figure 4C). Synechococcales oligotypes composition varied seasonally at surface and DCM, linked to changes in low (e.g., oligos 3, 4, 5, 6, and 12) and high abundance oligotypes (e.g., oligos 1, 2, and 3), respectively (Figures 4E,F). SAR86 oligotypes 4, 5, and 8 increased in spring and summer (Apr16, Jul16, and Jun16), while oligotype 6 developed in autumn (Oct16 and Nov17) (Supplementary Figure S9E). Seasonal dynamics of Actino-marinales oligotypes composition was less pronounced than the previously mentioned groups, however, specific oligotypes developed in autumn (oligotypes 12–15; Supplementary Figure S9G). No clear temporal patterns were observed for Marine Group II, Chloroplast, or Alteromonadales oligotypes (Supplementary Figure S9).
The variability of oligotypes composition related to environmental parameters was tested with RDA (Supplementary Figure S10 and Supplementary Table S4). The first RDA axis was significant (p < 0.01) for the models of all groups, and the second axis was also significant (p < 0.01) for all the group models except Alteromonadales and Marine Group II (Supplementary Table S4). Depth (surface vs. DCM) and season significantly explained Flavobacteriales, Rhodobacterales, Synechococcales, SAR11, SAR86, Actinomarinales, and Marine Group II oligotypes composition. Surface and DCM oligotypes composition grouped together in winter and clustered separately in summer except for Alteromonadales oligotypes composition (Supplementary Figure S10). Besides, nitrate concentration significantly explained Flavobacteriales, SAR11, and SAR86 oligotypes composition and phosphate concentration contributed to explain the variability of Rhodobacterales, Synechococcales, SAR11, and Marine Group II oligotypes composition. Nitrite, Chl-a, and phaeopigments also significantly explained the variability of SAR11 oligotypes composition. The location of the samples (station) significantly accounted for Synechococcales, SAR86 and Alteromonadales oligotypes composition changes but not for the oligotypes composition of the other groups (Supplementary Figure S10 and Supplementary Table S4).
Network Association Analysis of Oligotypes
Relative abundances of phylotypes Rhodobacterales and SAR86 and of Marine Group II and Chloroplast at order level significantly correlated both at surface and DCM (p < 0.05, ANOVA; Supplementary Figure S11). Relative abundances of the other orders analyzed were only significantly correlated at surface, e.g., Synechococcales and Rhodobacterales, SAR11 and SAR86 or Rhodobacterales and SAR11 (p < 0.05, ANOVA; Supplementary Figure S11A).
Association networks of oligotypes from all the phylotypes analyzed showed different connectivity patterns for surface and DCM (Figure 5; Supplementary Figure S12). Association network from surface oligotypes exhibited lower interconnectivity (Figure 5) than DCM oligotypes (Supplementary Figure S12). Separated assemblies in surface communities were comprised mostly by low abundance oligotypes concurrently occurring in one or few discrete samples, which resulted in high correlation coefficients (Figure 5).
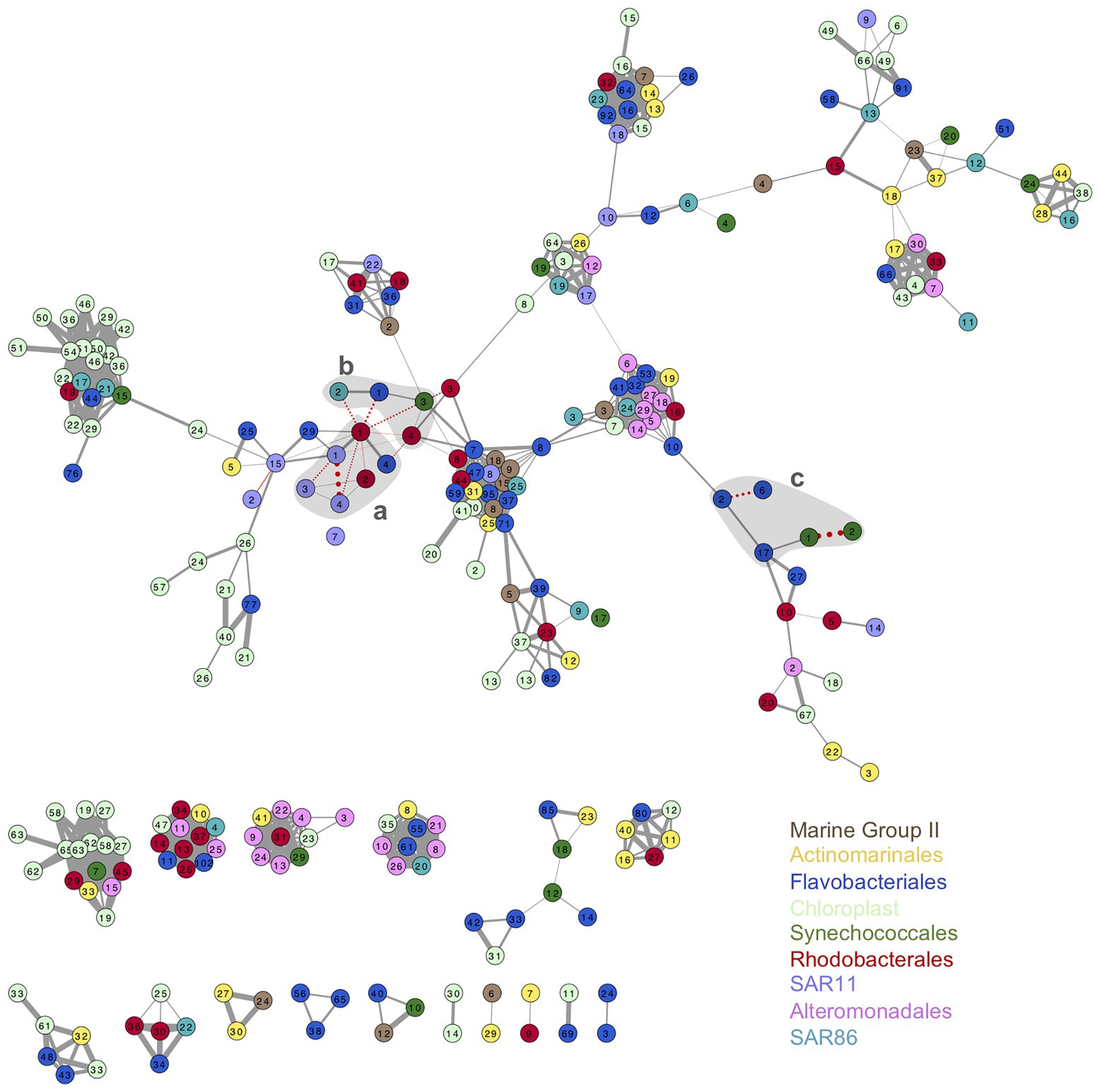
Figure 5. Association network of surface oligotypes. Each node represents one oligotype, node colors indicate the nine phylotypes studied (see legend). Width of edges connecting nodes is drawn proportionally to the Pearson correlation value between the two oligotypes. Solid gray edges indicate positive correlations and pointed red edges indicate negative correlations. Only significant correlations are shown (p < 0.05). Shaded gray areas labeled a, b, and c enclose the association of oligotypes represented in Figure 6 and Supplementary Figure S13.
As example of seasonal dynamics of connected oligotypes within and between phylotypes, we selected a set of abundant oligotypes from surface communities surrounded by gray areas in Figure 5. The two most abundant oligotypes from Rhodobacterales and Synechococcales (oligotypes 1 vs. 2) exhibited opposed seasonal patterns at surface waters (Figures 6A,C). Rhodobacterales oligotype 1 dominated in spring and summer while oligotype 2 increased in winter and autumn (Figure 6A). Synechococcales oligotype 1 dominated in winter and spring and oligotype 2 dominated in summer and autumn (Figure 6C). However, although also negatively correlated, oligotypes 1 and 2 from Rhodobacterales and Synechococcales did not show the same temporal patterns at DCM (Supplementary Figures S13A,C). Similarly, SAR11 oligotype 1 exhibited an opposed seasonal pattern to that of oligotypes 3 and 4 (Figure 6A), whereas Flavobacteriales oligotype 6 displayed opposed seasonal dynamics to oligotypes 2 and 17 at surface waters (Figure 6C). However, neither SAR11 nor Flavobacteriales oligotypes showed clear seasonal trends at the DCM (Supplementary Figure S13).
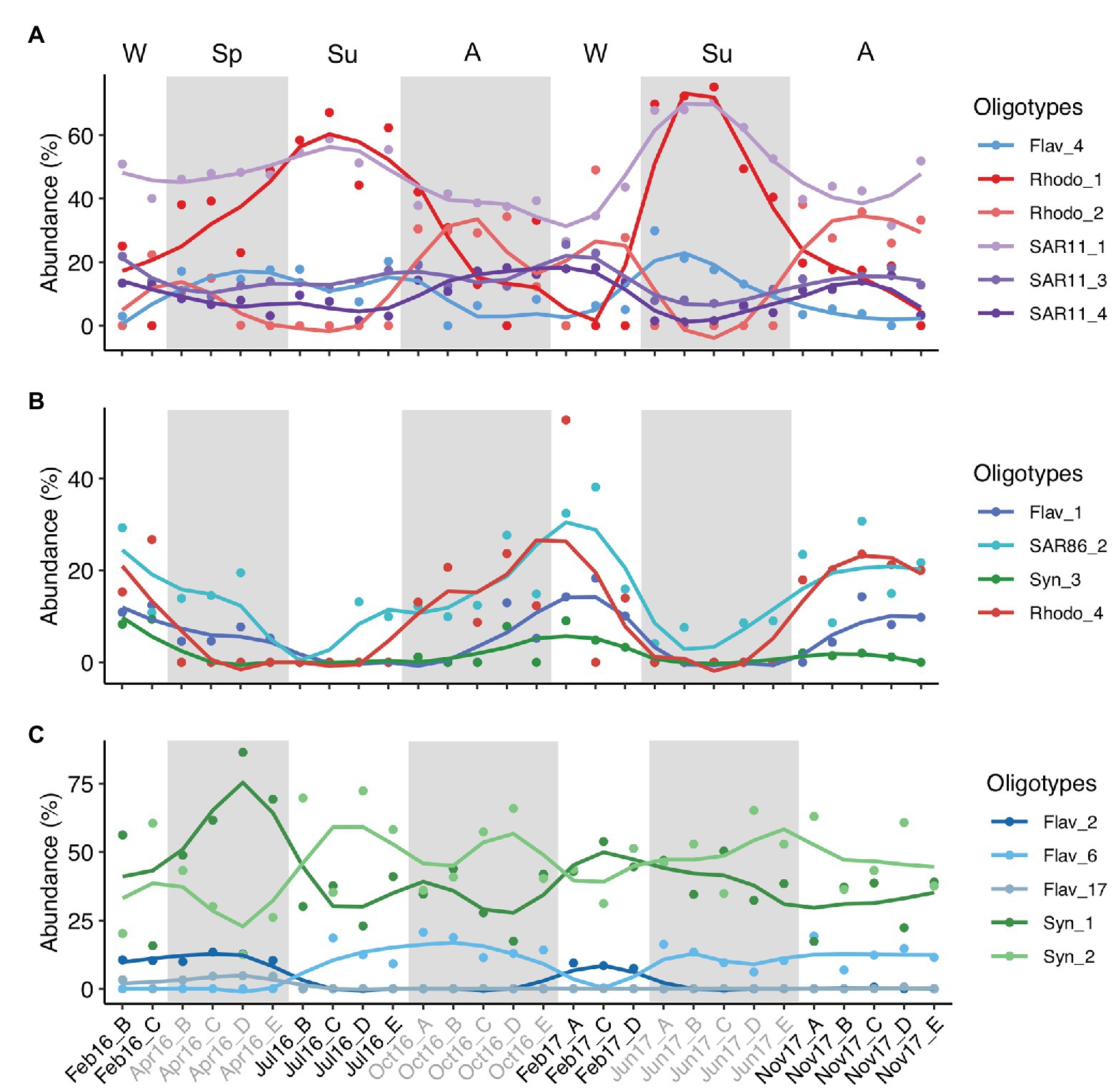
Figure 6. Temporal variation of three groups of connected abundant oligotypes. The three groups of oligotypes (A–C) correspond to those enclosed in shaded gray areas in surface network of Figure 5. Y-axis indicates the relative abundance of the oligotypes analyzed in this study. Lines are loess smoothed (smoothing function to facilitate trends visualization) and dots indicate the data values. Samples are labeled according to cruise and station (Cruise_Station). Alternate light/shaded areas indicate changing seasons: W (winter), Sp (spring), Su (summer), and A (autumn). Oligotypes are labeled according to phylotype_oligotype number. Flav, Flavobacteriales; Rhodo, Rhodobacterales; Syn, Synechococcales.
Inter-phylotype connection of abundant oligotypes was noticeable at surface waters, e.g., Flavobacteriales oligotype 1, SAR86 oligotype 2, Synechococcales oligotype 3, and Rhodobacterales oligotype 4 relative abundances decreased during summer and increased in winter and autumn (Figure 6B). However, these oligotypes showed different temporal patterns at DCM communities (Supplementary Figure S13B). Marine Group II, Chloroplast, Alteromonadales, and Actinomarinales oligotypes co-occurred with other low-abundant oligotypes in the association networks (Figure 5; Supplementary Figure S13).
Discussion
Seasonal and Spatial Dynamics of Prokaryotic Communities in the Western Mediterranean Sea
The upper-ocean prokaryotic communities were strongly shaped by winter water column mixing and summer stratification in agreement with previous findings in the open ocean and coastal waters (Treusch et al., 2009; Gilbert et al., 2012; Fuhrman et al., 2015; García et al., 2015; Salter et al., 2015). Noticeably, surface prokaryotic communities are subjected to more pronounced seasonal variability than DCM communities. The different seasonal trends between surface and DCM are driven by the different variability in seawater temperature between the two depths, as well as the nutrient depletion in summer surface waters associated to thermal stratification (Pinhassi et al., 2006; D’Ortenzio and Prieur, 2012). Accordingly, surface communities markedly differed from DCM communities during summer stratification, separated by the thermocline structure, whereas during the winter mixing, surface and DCM communities were more similar and shared a higher proportion of ASVs (Figure 2; Supplementary Figure S5; Morris et al., 2005; García et al., 2015; Salter et al., 2015). Hence, irradiance intensity, primarily governing surface ocean temperature, and nutrient availability are key factors shaping the seasonal and depth-related trends of microbial communities in marine systems, even in ocean regions with mild climatic variability, such as the North Pacific Subtropical Gyre (Bryant et al., 2016; Mende et al., 2017).
The seasonal variability of the epipelagic Mediterranean Sea microbial communities was prominent for the autotrophs Synechococcus and Prochlorococcus. Depth distribution patterns of these two phototrophs are strongly influenced by irradiance levels and light stress responsiveness (Mella-Flores et al., 2012). The relative abundance of Synechococcus and Prochlorococcus decreased at the highly irradiated surface waters and the two taxa sheltered below the thermocline in summer. This finding supports the negative effect of light and radiation overexposure on the growth of these phototrophs, Prochlorococcus being more sensitive to excess radiation (Mella-Flores et al., 2012). The temporal and depth variability of these two picophytoplankters contrasts with other findings in the oligotrophic Atlantic and Pacific oceans. Prochlorococcus dominates picophytoplankton abundance in the photic zone at the Bermuda Atlantic Time-Series Study (BATS) site, except during the spring bloom mixing period, when similar abundances of Prochlorococcus and Synechococcus occur (DuRand et al., 2001). At the North Pacific Subtropical Gyre (station ALOHA), Prochlorococcus dominates year-round without remarkable seasonal changes (Bryant et al., 2016). Considering that Synechococcus and Prochlorococcus dominate the photosynthetic community in the oligotrophic Mediterranean Sea (Mena et al., 2019), the results suggest an important role of these groups in the strong primary production seasonality of the open ocean Mediterranean Sea (Marty and Chiavérini, 2002), with reportedly higher new primary production at the DCM during summer stratification and in the upper layers during mixing conditions (Estrada, 1996; Pedrós-Alió et al., 1999). Based on ocean color and a phytoplankton class-specific bio-optical model, Uitz et al. (2012) estimated an annual contribution of picophytoplankton of ~31% in the western basin of the Mediterranean Sea, smaller than nanophytoplankton (~48%) and larger than microphytoplankton (~21%). However, contribution of picophytoplankton to primary production changes according to local environmental conditions (Magazzu and Decembrini, 1995) and seasonally (Charles et al., 2005). Further research on the temporal and spatial activity of these two autotrophs and the comparison to the activity rates of eukaryotic phototrophs are needed to complete our view of the carbon cycle in the upper Mediterranean Sea.
Similarly to the cyanobacteria groups, uncultured Alphaproteo-bacteria OCS116 and Marine Group II group revealed similar contributions at surface and DCM during the mixed period, which persisted at the DCM throughout the study, in agreement with previous findings on seasonal trends of these phylotypes (Morris et al., 2005; Treusch et al., 2009; Hugoni et al., 2013; Haro-Moreno et al., 2018). OCS116 had been described to concurrently bloom with phytoplankton in spring (Morris et al., 2005; Treusch et al., 2009); however, no positive correlation to Chl-a was found in this study.
Contrary to the previous groups, SAR116 clade members thrived in the stratified surface waters in agreement with their association with warmer temperatures and water stratification (Morris et al., 2005; Giovannoni and Vergin, 2012; Alonso-Sáez et al., 2015; Bryant et al., 2016). SAR116 clade members harbor a diverse metabolic potential, including dimethylsulfoniopropionate degradation, oxidation of one-carbon compounds, potential tolerance to high irradiance levels, and their own light-dependent proteorhodopsin (Oh et al., 2010), suggesting an important role in the carbon dynamics of the oligotrophic Mediterranean Sea during the stratified period. Other groups show no apparent seasonal variations, such as orders Flavobacteriales or Rhodobacterales (Figure 3; Supplementary Figure S11), abundant photic groups frequently associated with phytoplankton blooms (Buchan et al., 2014). Also, no significant temporal patterns for SAR11 and SAR86 clades were determined in our study (Figure 3; Supplementary Figure S11), in contrast to previous studies in the Sargasso Sea (Morris et al., 2005; Treusch et al., 2009) and NW Mediterranean Sea (Salter et al., 2015). However, these groups harbor a large intra-diversity and consist of numerous ecotypes adapted to different environmental conditions (Vergin et al., 2013a; Hoarfrost et al., 2019), as discussed further on.
Seasonality of Oligotypes Within Particular Phylotypes
The function and dynamics of an ecosystem is contingent on the assembly of taxa playing ecologically distinct roles (ecotypes) and on their interactions with other taxa and with the environment (Koeppel et al., 2008). A recent approach to determine the presence and dynamics of ecotypes within closely related taxa is oligotyping (Eren et al., 2013). Indeed, Flavobacteriales, Rhodobacterales, SAR11, and SAR86 showed seasonal dynamics only at oligotype level in this study (Supplementary Figure S10) but not at order level (Figure 3; Supplementary Figure S11). Although the main differences were observed between winter and summer, i.e., under well-defined mixed and stratified conditions (Salter et al., 2015), there were particular oligotypes preferentially associated to spring (e.g., Rhodobacterales oligotype 10; Figure 4C) or autumn conditions (e.g., Flavobacteriales, Synechococcales, SAR86, and Actinomarinales oligotypes; Figures 4A,E; Supplementary Figure S9). SAR86 clade, significant contributor to the surface ocean communities (Dupont et al., 2012; this study), showed notable seasonal variability in oligotype composition and a diversity decrease during winter at surface (Supplementary Figure S9E).
The temporal patterns of oligotypes suggest niche specialization. Ecotypes differentiation can be originated by spatial and temporal environmental gradients. Temperature and light-level induce depth differentiation of Prochlorococcus ecotypes (Zwirglmaier et al., 2008). The absence of specific oligotypes at surface during the stratified period (e.g., oligotypes 3 and 4 of Synechococcales; Figure 4E) could be explained by their sensitivity to light-stress and/or to low nutrient concentrations. Indeed, phosphate concentrations significantly explained the variability of Synechococcales oligotypes composition, suggesting that phosphate availability selects for distinct surface oligotypes during the stratification period, in accordance with the general phosphate limitation of the Mediterranean Sea (Pasqueron de Fommervault et al., 2015). Dissolved organic matter (DOM) is the largest reservoir of reduced carbon in the ocean (Hansell et al., 2009), supporting heterotrophic microbes throughout the water column (Kujawinski, 2011). However, DOM is composed of a very diverse and complex mixture of compounds, with different chemical and physical properties (Nagata, 2008), providing a heterogeneity of potential niches for heterotrophs. Fine-tuning of the metabolic capacities of closely-related microbes to the use of these diverse set of substrates helps explain the seasonality and spatial distribution of ecotypes within specific taxa (Carlson et al., 2009; Hehemann et al., 2016). Temporally recurring biotic and abiotic conditions, e.g., phytoplankton blooms and their associated production, atmospheric deposition (Koçak et al., 2010), or quantity and quality of organic carbon inputs (Pasqual et al., 2015), induce seasonal variations in availability of specific sets of DOM compounds for surface heterotrophic prokaryotic communities, supporting the recurring seasonal patterns of closely related taxa determined in coastal marine systems (Ward et al., 2017; Chafee et al., 2018) and in open Mediterranean sea waters (e.g., oligotypes 1 and 2 of Flavobacteriales or oligotypes 2, 3, and 4 of Rhodobacterales in this study).
Nevertheless, not all examined taxa exhibited clear seasonality of oligotypes. Presumably, the low abundance of sequences assigned to Marine Group II at surface and to Chloroplast in the first year of study hindered the detection of oligotypes and their potential temporal patterns. During the second year of study, Chloroplast sequences were more abundant, and seasonal differences in oligotypes diversity and composition during winter, summer, and autumn were determined. However, further studies will be needed to support recurring seasonal patterns for this group. The copiotrophic lifestyle of Alteromonadales resulted in a conspicuous feast and famine response (Vergin et al., 2013b). Their low abundance (or undetectability) in most samples interfered with oligotyping analysis, hindering the description of oligotypes composition, and therefore preventing to determine temporal variations. However, the pronounced increase in the total abundance of Alteromonadales in particular samples coincided with a notable increase of oligotypes diversity, indicating microdiversity of the blooming Alteromonadales. Albeit challenging, further studies focusing on disentangle the influence of environmental variability, substrate specialization, and predation dynamics on the differentiation of ecotypes are essential to understand microdiversity patterns and their influence in the metabolic activity of microbial communities (Alonso-Sáez and Gasol, 2007; Teira et al., 2019).
Temporal Co-occurrence of Particular Phylotypes
Microbes interact in various ways with positive, negative, or neutral effects for one or both organisms implicated (Faust and Raes, 2012). Consequently, inter-organism interactions contribute to determine the realized niche of a specific organism and shape ecosystem function with profound effects on the biogeochemical ocean cycles (Strom, 2008). Oligotypes of different taxa showed temporal co-occurrence or exclusion patterns, however, the inter-oligotypes associations differed noticeably between surface and DCM communities, suggesting an influence of environmental conditions on the microbe-interactions and dynamics between the two depth layers (Cui et al., 2019) and the fine-tuning of the realized niche of closely-related taxa.
Surface communities are exposed to more variable conditions than DCM communities. During summer, surface Mediterranean prokaryotic communities are exposed to unfavorable conditions, including nutrient depletion and light stress (Ruiz-González et al., 2012; Tanhua et al., 2013). Microbes with an oligotrophic lifestyle have an advantage in nutrient poor environments; however, streamlining of their genomes induces the lack of essential metabolic pathways to synthesize key metabolites (Giovannoni et al., 2014) or to remove toxic or reactive molecules (e.g., Morris et al., 2011). Consequently, streamlined microorganisms depend on co-occurring microbes (Morris et al., 2011; Peura et al., 2015), successively resulting in the expansion of their realized niches and habitat ranges (Ma et al., 2018). Accordingly, free-living bacteria, generally harboring oligotrophic lifestyles, exhibit higher connectivity to other organisms than typically copiotrophic particle-attached bacteria (Milici et al., 2016).
The positive correlation between the total abundances of Rhodobacterales and SAR86 observed in this study suggests that the simpler compounds released by Rhodobacterales during the algal-derived organic matter degradation can be subsequently used by SAR86 (Dupont et al., 2012). Correlation between Rhodobacterales and SAR11 at surface was found not only at the order level but it was also substantiated by the seasonal correlation of particular surface ocean Rhodobacterales and SAR11 oligotypes. Oligotype 1 of both lineages increased during summer while oligotype 2 of Rhodobacterales and oligotypes 3 and 4 of SAR11 dominated during autumn-winter (Figure 6A). Rhodobacterales members perform key metabolic pathways involved in phytoplankton-derived organic matter processing (Buchan et al., 2014), resulting in the production and release of derived compounds which can be used by SAR11 members (Reintjes et al., 2019). Rhodobacterales have been described as a remarkably metabolically versatile group based on the genomic analysis of different isolates (Newton et al., 2010), supporting contrasting specific linkages between Rhodobacterales and SAR11 oligotypes in different seasons. Network associations have to be interpreted with caution (Faust and Raes, 2012), and the good agreement between the temporal dynamics of these specific phylotypes indicates either specialized interactions or similar ecological niche (e.g., utilization of similar substrates, similar effect of environmental factors, and/or interactions with other organisms such as predation/parasitism). Furthermore, our findings are based on a relatively low temporal sampling frequency (i.e., once per season) over approximately 2 years. Higher sampling resolution and extended time series studies should improve the robustness of the results and provide further support to the conclusions.
The seasonal dynamics of oligotypes also supports that the seed bank theory (Lennon and Jones, 2011) and the Baas Becking tenet (De Wit and Bouvier, 2006) applies to ecotypes within specific microbial taxa. Whereas Synechococcales oligotype 1 was dominant at DCM year-round with few exceptions and at surface from winter to spring, oligotype 2 dominated at surface in summer, indicating a better fitness of the latter oligotype under the summer environmental conditions (Figure 6; Supplementary Figure S13). Flavobacteriales oligotype 6 is a clear example of low abundance oligotype blooming when conditions are favorable in summer and autumn (Figure 6C).
Taken together, our results indicate a major effect of the seasonal stratification on prokaryotic community composition and diversity in the sunlit temperate open ocean. Noticeably, the temporal dynamics and association networks strongly responded to stratification conditions at a fine-scale phylogeny level, with implications for the future ocean function under the predicted increase of surface ocean temperatures and stratification associated to climate change. Oligotype dynamics within a specific taxon and connectivity patterns to other taxa oligotypes suggests temporal niche partitioning and fine-tuning of the realized niche of ecotypes, highlighting the importance of examining communities beyond common phylogenetically assigned taxa (Louca et al., 2016), and contributing to explain the plankton paradox. Further analysis on the metabolic functions of the different ecotypes using omic approaches will help elucidate how these oligotypes are specifically linked, and how their predicted dynamics in the framework of climate change might affect the global element cycles.
Data Availability Statement
The datasets presented in this study can be found in online repositories. The names of the repository/repositories and accession number(s) can be found in the article/Supplementary Material.
Author Contributions
CM, PR, RB, and ES conceived and planned the work. CM, RS, MM, and ES carried out the sampling and laboratory work. CM analyzed the data. CM and ES wrote the first draft of the manuscript. All authors contributed to the article and approved the submitted version.
Funding
This work was supported by the projects RADMED-TRES (2015-2019) funded by the Spanish Institute of Oceanography and ATHAPOC (CTM2014-54374-R) funded by the Spanish Ministry of Economy and Competitiveness. CM was supported by pre-doctoral FPI fellowship from Conselleria d’Innovació, Recerca i Turisme of the regional Government of the Balearic Islands, co-financed by the European Social Fund as part of the FSE 2014-2020 operational program.
Conflict of Interest
The authors declare that the research was conducted in the absence of any commercial or financial relationships that could be construed as a potential conflict of interest.
Acknowledgments
We acknowledge the captain and crew members of R/V Francisco de Paula Navarro and R/V Ramón Margalef and to the entire RADMED team for their help and support. Laboratory facilities and technical support by the Microbial Oceanography Lab at the University of Vienna and the Interdisciplinary Ecology group and Marine Ecology and Systematics group from the University of the Balearic Islands are greatly appreciated.
Supplementary Material
The Supplementary Material for this article can be found online at: https://www.frontiersin.org/articles/10.3389/fmicb.2020.01749/full#supplementary-material.
Footnotes
References
Alonso-Sáez, L., Díaz-Pérez, L., and Morán, X. A. G. (2015). The hidden seasonality of the rare biosphere in coastal marine bacterioplankton. Environ. Microbiol. 17, 3766–3780. doi: 10.1111/1462-2920.12801
Alonso-Sáez, L., and Gasol, J. M. (2007). Seasonal variations in the contributions of different bacterial groups to the uptake of low-molecular-weight compounds in Northwestern Mediterranean coastal waters. Appl. Environ. Microbiol. 73, 3528–3535. doi: 10.1128/AEM.02627-06
Azam, F., and Malfatti, F. (2007). Microbial structuring of marine ecosystems. Nat. Rev. Microbiol. 5, 782–791. doi: 10.1038/nrmicro1747
Banning, E. C., Casciotti, K. L., and Kujawinski, E. B. (2010). Novel strains isolated from a coastal aquifer suggest a predatory role for flavobacteria. FEMS Microbiol. Ecol. 73, 254–270. doi: 10.1111/j.1574-6941.2010.00897.x
Beman, J. M., Steele, J. A., and Fuhrman, J. A. (2011). Co-occurrence patterns for abundant marine archaeal and bacterial lineages in the deep chlorophyll maximum of coastal California. ISME J. 5, 1077–1085. doi: 10.1038/ismej.2010.204
Brussaard, C. P. D. (2004). Optimization of procedures for counting viruses by flow cytometry. Appl. Environ. Microbiol. 70, 1506–1513. doi: 10.1128/AEM.70.3.1506-1513.2004
Bryant, J. A., Aylward, F. O., Eppley, J. M., Karl, D. M., Church, M. J., and DeLong, E. F. (2016). Wind and sunlight shape microbial diversity in surface waters of the North Pacific subtropical gyre. ISME J. 10, 1308–1322. doi: 10.1038/ismej.2015.221
Buchan, A., Lecleir, G. R., Gulvik, C. A., and González, J. M. (2014). Master recyclers: features and functions of bacteria associated with phytoplankton blooms. Nat. Rev. Microbiol. 12, 686–698. doi: 10.1038/nrmicro3326
Bunse, C., and Pinhassi, J. (2017). Marine bacterioplankton seasonal succession dynamics. Trends Microbiol. 25, 494–505. doi: 10.1016/j.tim.2016.12.013
Callahan, B. J., McMurdie, P. J., Rosen, M. J., Han, A. W., Johnson, A. J. A., and Holmes, S. P. (2016). Dada2: high-resolution sample inference from illumina amplicon data. Nat. Methods 13, 581–583. doi: 10.1038/nmeth.3869
Carlson, C. A., Morris, R., Parsons, R., Treusch, A. H., Giovannoni, S. J., and Vergin, K. (2009). Seasonal dynamics of SAR11 populations in the euphotic and mesopelagic zones of the Northwestern Sargasso Sea. ISME J. 3, 283–295. doi: 10.1038/ismej.2008.117
Chafee, M., Fernàndez-Guerra, A., Buttigieg, P. L., Gerdts, G., Eren, A. M., Teeling, H., et al. (2018). Recurrent patterns of microdiversity in a temperate coastal marine environment. ISME J. 12, 237–252. doi: 10.1038/ismej.2017.165
Charles, F., Lantoine, F., Brugel, S., Chrétiennot-Dinet, M. J., Quiroga, I., and Rivière, B. (2005). Seasonal survey of the phytoplankton biomass, composition and production in a littoral NW Mediterranean site, with special emphasis on the picoplanktonic contribution. Estuar. Coast. Shelf Sci. 65, 199–212. doi: 10.1016/j.ecss.2005.06.006
Chen, X., Ma, R., Yang, Y., Jiao, N., and Zhang, R. (2019). Viral regulation on bacterial community impacted by lysis-lysogeny switch: a microcosm experiment in eutrophic coastal waters. Front. Microbiol. 10:1763. doi: 10.3389/fmicb.2019.01763
Chow, C. T., Sachdeva, R., Cram, J. A., Steele, J. A., Needham, D. M., Patel, A., et al. (2013). Temporal variability and coherence of euphotic zone bacterial communities over a decade in the Southern California bight. ISME J. 7, 2259–2273. doi: 10.1038/ismej.2013.122
Clifford, E. L., Varela, M. M., De Corte, D., Bode, A., Ortiz, V., Herndl, G. J., et al. (2019). Taurine is a major carbon and energy source for marine prokaryotes in the North Atlantic Ocean off the Iberian Peninsula. Microb. Ecol. 78, 299–312. doi: 10.1007/s00248-019-01320-y
Cui, Y., Chun, S. J., Baek, S. H., Lee, M., Kim, Y., Lee, H. G., et al. (2019). The water depth-dependent co-occurrence patterns of marine bacteria in shallow and dynamic southern coast, Korea. Sci. Rep. 9, 1–13. doi: 10.1038/s41598-019-45512-5
De Wit, R., and Bouvier, T. (2006). “Everything is everywhere, but, the environment selects”; what did Baas Becking and Beijerinck really say? Environ. Microbiol. 8, 755–758. doi: 10.1111/j.1462-2920.2006.01017.x
D’Ortenzio, F., and Prieur, L. (2012). The upper mixed layer. ed. N. Stambler (Hauppauge, NY: Nova Science Publishers, Inc.).
Dupont, C. L., Rusch, D. B., Yooseph, S., Lombardo, M. J., Alexander Richter, R., Valas, R., et al. (2012). Genomic insights to SAR86, an abundant and uncultivated marine bacterial lineage. ISME J. 6, 1186–1199. doi: 10.1038/ismej.2011.189
DuRand, M. D., Olson, R. J., and Chisholm, S. W. (2001). Phytoplankton population dynamics at the Bermuda Atlantic time-series station in the Sargasso Sea. Deep-Sea Res. II 48, 1983–2003. doi: 10.1016/S0967-0645(02)00025-5
Eren, A. M., Maignien, L., Sul, W. J., Murphy, L. G., Grim, S. L., Morrison, H. G., et al. (2013). Oligotyping: differentiating between closely related microbial taxa using 16S rRNA gene data. Methods Ecol. Evol. 4, 1111–1119. doi: 10.1111/2041-210X.12114
Faust, K., and Raes, J. (2012). Microbial interactions: from networks to models. Nat. Rev. Microbiol. 10, 538–550. doi: 10.1038/nrmicro2832
Fuhrman, J. A. (2009). Microbial community structure and its functional implications. Nature 459, 193–199. doi: 10.1038/nature08058
Fuhrman, J. A., Cram, J. A., and Needham, D. M. (2015). Marine microbial community dynamics and their ecological interpretation. Nat. Rev. Microbiol. 13, 133–146. doi: 10.1038/nrmicro3417
García, F. C., Alonso-Sáez, L., Morán, X. A. G., and López-Urrutia, Á. (2015). Seasonality in molecular and cytometric diversity of marine bacterioplankton: the re-shuffling of bacterial taxa by vertical mixing. Environ. Microbiol. 17, 4133–4142. doi: 10.1111/1462-2920.12984
Gilbert, J. A., Steele, J. A., Caporaso, J. G., Steinbrück, L., Reeder, J., Temperton, B., et al. (2012). Defining seasonal marine microbial community dynamics. ISME J. 6, 298–308. doi: 10.1038/ismej.2011.107
Giovannoni, S. J., Cameron Thrash, J., and Temperton, B. (2014). Implications of streamlining theory for microbial ecology. ISME J. 8, 1553–1565. doi: 10.1038/ismej.2014.60
Giovannoni, S. J., and Vergin, K. L. (2012). Seasonality in ocean microbial communities. Science 335, 671–676. doi: 10.1126/science.1198078
Grasshoff, K., Ehrhardt, M., and Kremling, K. (1983). Methods of seawater analysis. Weinheim: Verlag Chemie GmbH.
Guidi, L., Chaffron, S., Bittner, L., Eveillard, D., Larhlimi, A., Roux, S., et al. (2016). Plankton networks driving carbon export in the oligotrophic ocean. Nature 532, 465–470. doi: 10.1038/nature16942
Hansell, D. A., Carlson, C. A., Repeta, D. J., and Schlitzer, R. (2009). Dissolved organic matter in the ocean: a controversy stimulates new insights. Oceanography 22, 202–211. doi: 10.5670/oceanog.2009.109
Haro-Moreno, J. M., López-Pérez, M., de la Torre, J. R., Picazo, A., Camacho, A., and Rodriguez-Valera, F. (2018). Fine metagenomic profile of the Mediterranean stratified and mixed water columns revealed by assembly and recruitment. Microbiome 6, 1–19. doi: 10.1186/s40168-018-0513-5
Hehemann, J. H., Arevalo, P., Datta, M. S., Yu, X., Corzett, C. H., Henschel, A., et al. (2016). Adaptive radiation by waves of gene transfer leads to fine-scale resource partitioning in marine microbes. Nat. Commun. 7:12860. doi: 10.1038/ncomms12860
Hmelo, L. R. (2017). Quorum sensing in marine microbial environments. Annu. Rev. Mar. Sci. 9, 257–281. doi: 10.1146/annurev-marine-010816-060656
Hoarfrost, A., Nayfach, S., Ladau, J., Yooseph, S., Arnosti, C., Dupont, C. L., et al. (2019). Global ecotypes in the ubiquitous marine clade SAR86. ISME J. 14, 178–188. doi: 10.1038/s41396-019-0516-7
Holm-Hansen, O., Lorenzen, C. J., Holmes, R. W., and Strickland, J. D. H. (1965). Fluorometric determination of chlorophyll. ICES J. Mar. Sci. 30, 3–15.
Hugoni, M., Taib, N., Debroas, D., Domaizon, I., Dufournel, I. J., Bronner, G., et al. (2013). Structure of the rare archaeal biosphere and seasonal dynamics of active ecotypes in surface coastal waters. Proc. Natl. Acad. Sci. U. S. A. 110, 1–6. doi: 10.1073/pnas.1216863110
Johnson, Z. I., and Lin, Y. (2009). Prochlorococcus: approved for export. Proc. Natl. Acad. Sci. U. S. A. 106, 10400–10401. doi: 10.1073/pnas.0905187106
Katoh, K., and Standley, D. M. (2013). MAFFT multiple sequence aligment software version 7: improvements in performance and usability. Mol. Biol. Evol. 30, 772–780. doi: 10.1093/molbev/mst010
Koçak, M., Kubilay, N., Tuǧrul, S., and Mihalopoulos, N. (2010). Atmospheric nutrient inputs to the Northern Levantine basin from a long-term observation: sources and comparison with riverine inputs. Biogeosciences 7, 4037–4050. doi: 10.5194/bg-7-4037-2010
Koeppel, A., Perry, E. B., Sikorski, J., Krizanc, D., Warner, A., Ward, D. M., et al. (2008). Identifying the fundamental units of bacterial diversity: a paradigm shift to incorporate ecology into bacterial systematics. Proc. Natl. Acad. Sci. U. S. A. 105, 2504–2509. doi: 10.1073/pnas.0712205105
Kujawinski, E. B. (2011). The impact of microbial metabolism on marine dissolved organic matter. Annu. Rev. Mar. Sci. 3, 567–599. doi: 10.1146/annurev-marine-120308-081003
Lennon, J. T., and Jones, S. E. (2011). Microbial seed banks: the ecological and evolutionary implications of dormancy. Nat. Rev. Microbiol. 9, 119–130. doi: 10.1038/nrmicro2504
Li, M., Baker, B. J., Anantharaman, K., Jain, S., Breier, J. A., and Dick, G. J. (2015). Genomic and transcriptomic evidence for scavenging of diverse organic compounds by widespread deep-sea archaea. Nat. Commun. 6:8933. doi: 10.1038/ncomms9933
Liu, J., Meng, Z., Liu, X., and Zhang, X. -H. (2019). Microbial assembly, interaction, functioning, activity and diversification: a review derived from community compositional data. Mar. Life Sci. Tech. 1, 112–128. doi: 10.1007/s42995-019-00004-3
Long, R. A., and Azam, F. (2001). Antagonistic interactions among marine pelagic bacteria. Appl. Environ. Microbiol. 67, 4975–4983. doi: 10.1128/AEM.67.11.4975-4983.2001
Lorenzen, C. (1967). Determination of chlorophyll and pheo-pigments: spectrophotometric equations. Limnol. Oceanogr. 12, 343–346. doi: 10.4319/lo.1967.12.2.0343
Louca, S., Parfrey, L., and Doebeli, M. (2016). Decoupling function and taxonomy in the global ocean microbiome. Science 353, 1272–1277. doi: 10.1126/science.aaf4507
Ma, L., Calfee, B. C., Morris, J. J., Johnson, Z. I., and Zinser, E. R. (2018). Degradation of hydrogen peroxide at the ocean’s surface: the influence of the microbial community on the realized thermal niche of Prochlorococcus. ISME J. 12, 473–484. doi: 10.1038/ismej.2017.182
Magazzu, G., and Decembrini, F. (1995). Primary production, biomass and abundance of phototrophic picoplankton in the Mediterranean Sea – a review. Aquat. Microb. Ecol. 9, 97–104. doi: 10.3354/ame009097
Malmstrom, R. R., Coe, A., Kettler, G. C., Martiny, A. C., Frias-Lopez, J., Zinser, E. R., et al. (2010). Temporal dynamics of Prochlorococcus ecotypes in the Atlantic and Pacific oceans. ISME J. 4, 1252–1264. doi: 10.1038/ismej.2010.60
Marie, D., Simon, N., Guillou, L., Partensky, F., and Vaulot, D. (2000). In living color: Protocols in flow cytometry and cell sorting. Heidelberg, Germany: Springer-Verlag Berlin Heidelberg.
Martínez-Pérez, C., Mohr, W., Löscher, C. R., Dekaezemacker, J., Littmann, S., Yilmaz, P., et al. (2016). The small unicellular diazotrophic symbiont, UCYN-A, is a key player in the marine nitrogen cycle. Nat. Microbiol. 1:16163. doi: 10.1038/nmicrobiol.2016.163
Marty, J. C., and Chiavérini, J. (2002). Seasonal and interannual variations in phytoplankton production at DYFAMED time-series station, Northwestern Mediterranean Sea. Deep-Sea Res. II Top. Stud. Oceanogr. 49, 2017–2030. doi: 10.1016/S0967-0645(02)00025-5
Mella-Flores, D., Six, C., Ratin, M., Partensky, F., Boutte, C., Le Corguillé, G., et al. (2012). Prochlorococcus and Synechococcus have evolved different adaptive mechanisms to cope with light and uv stress. Front. Microbiol. 3:285. doi: 10.3389/fmicb.2012.00285
Mena, C., Reglero, P., Hidalgo, M., Sintes, E., Santiago, R., Martín, M., et al. (2019). Phytoplankton community structure is driven by stratification in the oligotrophic Mediterranean Sea. Front. Microbiol. 10:1698. doi: 10.3389/fmicb.2019.01698
Mende, D. R., Bryant, J. A., Aylward, F. O., Eppley, J. M., Nielsen, T., Karl, D. M., et al. (2017). Environmental drivers of a microbial genomic transition zone in the ocean’s interior. Nat. Microbiol. 2, 1367–1373. doi: 10.1038/s41564-017-0008-3
Milici, M., Deng, Z. L., Tomasch, J., Decelle, J., Wos-Oxley, M. L., Wang, H., et al. (2016). Co-occurrence analysis of microbial taxa in the Atlantic Ocean reveals high connectivity in the free-living bacterioplankton. Front. Microbiol. 7:649. doi: 10.3389/fmicb.2016.00649
Morris, J. J., Johnson, Z. I., Szul, M. J., Keller, M., and Zinser, E. R. (2011). Dependence of the cyanobacterium Prochlorococcus on hydrogen peroxide scavenging microbes for growth at the ocean’s surface. PLoS One 6:e16805. doi: 10.1371/journal.pone.0016805
Morris, R. M., Vergin, K. L., Cho, J. C., Rappé, M. S., Carlson, C. A., and Giovannoni, S. J. (2005). Temporal and spatial response of bacterioplankton lineages to annual convective overturn at the Bermuda Atlantic time-series study site. Limnol. Oceanogr. 50, 1687–1696. doi: 10.4319/lo.2005.50.5.1687
Murphy, J., and Riley, J. P. (1962). A modified single solution method for the determination of phosphate in natural waters. Anal. Chim. Acta 27, 31–36. doi: 10.1016/S0003-2670(00)88444-5
Nagata, T. (2008). “Organic matter–bacteria interactions in seawater” in Microbial ecology of the oceans. ed. D. L. Kirchman (Hoboken, New Jersey: John Wiley & Sons, Inc.), 207–241.
Newton, R. J., Griffin, L. E., Bowles, K. M., Meile, C., Gifford, S., Givens, C. E., et al. (2010). Genome characteristics of a generalist marine bacterial lineage. ISME J. 4, 784–798. doi: 10.1038/ismej.2009.150
Oh, H. M., Kwon, K. K., Kang, I., Kang, S. G., Lee, J. H., Kim, S. J., et al. (2010). Complete genome sequence of “Candidatus puniceispirillum marinum” IMCC1322, a representative of the SAR116 clade in the Alphaproteobacteria. J. Bacteriol. 192, 3240–3241. doi: 10.1128/JB.00347-10
Parada, A. E., Needham, D. M., and Fuhrman, J. A. (2016). Every base matters: assessing small subunit rRNA primers for marine microbiomes with mock communities, time series and global field samples. Environ. Microbiol. 18, 1403–1414. doi: 10.1111/1462-2920.13023
Pasqual, C., Calafat, A., Lopez-Fernandez, P., and Pusceddu, A. (2015). Organic carbon inputs to the sea bottom of the Mallorca continental slope. J. Mar. Syst. 148, 142–151. doi: 10.1016/j.jmarsys.2015.02.006
Pasqueron de Fommervault, O., Migon, C., D’Ortenzio, F., Ribera d’Alcalà, M., and Coppola, L. (2015). Temporal variability of nutrient concentrations in the Northwestern Mediterranean Sea (DYFAMED time-series station). Deep-Sea Res. I 100, 1–12. doi: 10.1016/j.dsr.2015.02.006
Pearman, J. K., Ellis, J., Irigoien, X., Sarma, Y. V. B., Jones, B. H., and Carvalho, S. (2017). Microbial planktonic communities in the Red Sea: high levels of spatial and temporal variability shaped by nutrient availability and turbulence. Sci. Rep. 7, 1–15. doi: 10.1038/s41598-017-06928-z
Pedrós-Alió, C., Calderón-Paz, J., Guixa-Boixereu, N., Estrada, M., and Gasol, J. M. (1999). Bacterioplankton and phytoplankton biomass and production during summer stratification in the Northwestern Mediterranean Sea. Deep-Sea Res. I Oceanogr. Res. Pap. 46, 985–1019. doi: 10.1016/S0967-0637(98)00106-X
Pernthaler, J. (2005). Predation on prokaryotes in the water column and its ecological implications. Nat. Rev. Microbiol. 3, 537–546. doi: 10.1038/nrmicro1180
Peura, S., Bertilsson, S., Jones, R. I., and Eiler, A. (2015). Resistant microbial cooccurrence patterns inferred by network topology. Appl. Environ. Microbiol. 81, 2090–2097. doi: 10.1128/AEM.03660-14
Philippot, L., Andersson, S. G. E., Battin, T. J., Prosser, J. I., Schimel, J. P., Whitman, W. B., et al. (2010). The ecological coherence of high bacterial taxonomic ranks. Nat. Rev. Microbiol. 8, 523–529. doi: 10.1038/nrmicro2367
Pinhassi, J., Gómez-Consarnau, L., Alonso-Sáez, L., Sala, M. M., Vidal, M., Pedrós-Alió, C., et al. (2006). Seasonal changes in bacterioplankton nutrient limitation and their effects on bacterial community composition in the NW Mediterranean Sea. Aquat. Microb. Ecol. 44, 241–252. doi: 10.3354/ame044241
Price, M., Dehal, P., and Arkin, A. (2010). Fasttree 2-approximately maximum-likelihood trees for large alignments. PLoS One 5:e9490. doi: 10.1371/journal.pone.0009490
Reintjes, G., Arnosti, C., Fuchs, B., and Amann, R. (2019). Selfish, sharing and scavenging bacteria in the Atlantic Ocean: a biogeographical study of bacterial substrate utilisation. ISME J. 13, 1119–1132. doi: 10.1038/s41396-018-0326-3
Rousseaux, C. S., and Gregg, W. W. (2013). Interannual variation in phytoplankton primary production at a global scale. Remote Sens. 6, 1–19. doi: 10.3390/rs6010001
Ruiz-González, C., Lefort, T., Galí, M., Montserrat Sala, M., Sommaruga, R., Simó, R., et al. (2012). Seasonal patterns in the sunlight sensitivity of bacterioplankton from Mediterranean surface coastal waters. FEMS Microbiol. Ecol. 79, 661–674. doi: 10.1111/j.1574-6941.2011.01247.x
Salter, I., Galand, P. E., Fagervold, S. K., Lebaron, P., Obernosterer, I., Oliver, M. J., et al. (2015). Seasonal dynamics of active SAR11 ecotypes in the oligotrophic Northwest Mediterranean Sea. ISME J. 9, 347–360. doi: 10.1038/ismej.2014.129
Shannon, P., Markiel, A., Ozier, O., Baliga, N., Wang, J., Ramage, D., et al. (2003). Cytoscape: a software environment for integrated models of biomolecular interaction networks. Genome Res. 13, 2498–2504. doi: 10.1101/gr.1239303
Sintes, E., De Corte, D., Haberleitner, E., and Herndl, G. J. (2016). Geographic distribution of archaeal ammonia oxidizing ecotypes in the Atlantic Ocean. Front. Microbiol. 7:77. doi: 10.3389/fmicb.2016.00077
Sintes, E., Witte, H., Stodderegger, K., Steiner, P., and Herndl, G. J. (2013). Temporal dynamics in the free-living bacterial community composition in the coastal North Sea. FEMS Microbiol. Ecol. 83, 413–424. doi: 10.1111/1574-6941.12003
Strickland, J. D. H., and Parsons, T. R. (1968). A practical handbook of seawater analysis. 2nd Edn. Ottawa: Fisheries Research Board of Canada.
Strom, S. L. (2008). Microbial ecology of ocean biogeochemistry: a community perspective. Science 218, 2007–2009. doi: 10.1126/science.1153527
Suttle, C. A. (2007). Marine viruses – major players in the global ecosystem. Nat. Rev. Microbiol. 5, 801–812. doi: 10.1038/nrmicro1750
Tanhua, T., Hainbucher, D., Schroeder, K., Cardin, V., Álvarez, M., and Civitarese, G. (2013). The Mediterranean Sea system: a review and an introduction to the special issue. Ocean Sci. 9, 789–803. doi: 10.5194/os-9-789-2013
Teeling, H., Fuchs, B. M., Becher, D., Klockow, C., Gardebrecht, A., Bennke, C. M., et al. (2012). Substrate-controlled succession of marine bacterioplankton populations induced by a phytoplankton bloom. Science 336, 608–611. doi: 10.1126/science.1218344
Teira, E., Logares, R., Gutiérrez-Barral, A., Ferrera, I., Varela, M. M., Morán, X. A. G., et al. (2019). Impact of grazing, resource availability and light on prokaryotic growth and diversity in the oligotrophic surface global ocean. Environ. Microbiol. 21, 1482–1496. doi: 10.1111/1462-2920.14581
Treusch, A. H., Vergin, K. L., Finlay, L. A., Donatz, M. G., Burton, R. M., Carlson, C. A., et al. (2009). Seasonality and vertical structure of microbial communities in an ocean gyre. ISME J. 3, 1148–1163. doi: 10.1038/ismej.2009.60
Uitz, J., Stramski, D., Gentili, B., D’Ortenzio, F., and Claustre, H. (2012). Estimates of phytoplankton class-specific and total primary production in the Mediterranean Sea from satellite ocean color observations. Glob. Biogeochem. Cycles 26, 1–10. doi: 10.1029/2011GB004055
Vaque, D., Alonso-Saez, L., Aristegui, J., Agusti, S., Duarte, C. M., Montserrat Sala, M., et al. (2014). Bacterial production and losses to predators along an open ocean productivity gradient in the subtropical North East Atlantic Ocean. J. Plankton Res. 36, 198–213. doi: 10.1093/plankt/fbt085
Vergin, K. L., Beszteri, B., Monier, A., Cameron Thrash, J., Temperton, B., Treusch, A. H., et al. (2013a). High-resolution SAR11 ecotype dynamics at the Bermuda Atlantic time-series study site by phylogenetic placement of pyrosequences. ISME J. 7, 1322–1332. doi: 10.1038/ismej.2013.32
Vergin, K. L., Done, B., Carlson, C. A., and Giovannoni, S. J. (2013b). Spatiotemporal distributions of rare bacterioplankton populations indicate adaptive strategies in the oligotrophic ocean. Aquat. Microb. Ecol. 71, 1–13. doi: 10.3354/ame01661
Ward, C. S., Yung, C. M., Davis, K. M., Blinebry, S. K., Williams, T. C., Johnson, Z. I., et al. (2017). Annual community patterns are driven by seasonal switching between closely related marine bacteria. ISME J. 11, 1412–1422. doi: 10.1038/ismej.2017.4
Williams, T. J., Wilkins, D., Long, E., Evans, F., Demaere, M. Z., Raftery, M. J., et al. (2013). The role of planktonic Flavobacteria in processing algal organic matter in coastal East Antarctica revealed using metagenomics and metaproteomics. Environ. Microbiol. 15, 1302–1317. doi: 10.1111/1462-2920.12017
Zuur, A. F., Ieno, E. N., Walker, N. J., Saveliev, A. A., and Smith, G. (2009). Mixed effects models and extensions in ecology with R. New York, NY: Springer.
Zwirglmaier, K., Jardillier, L., Ostrowski, M., Mazard, S., Garczarek, L., Vaulot, D., et al. (2008). Global phylogeography of marine Synechococcus and Prochlorococcus reveals a distinct partitioning of lineages among oceanic biomes. Environ. Microbiol. 10, 147–161. doi: 10.1111/j.1462-2920.2007.01440.x
Keywords: microbial communities, marine prokaryotes, oligotyping, seasonality, 16S ribosomal RNA gene, open ocean, Mediterranean Sea, association networks
Citation: Mena C, Reglero P, Balbín R, Martín M, Santiago R and Sintes E (2020) Seasonal Niche Partitioning of Surface Temperate Open Ocean Prokaryotic Communities. Front. Microbiol. 11:1749. doi: 10.3389/fmicb.2020.01749
Edited by:
Alison Buchan, The University of Tennessee, Knoxville, United StatesReviewed by:
Frank O’Neill Aylward, Virginia Tech, United StatesIan Salter, Alfred Wegener Institute Helmholtz Centre for Polar and Marine Research (AWI), Germany
Copyright © 2020 Mena, Reglero, Balbín, Martín, Santiago and Sintes. This is an open-access article distributed under the terms of the Creative Commons Attribution License (CC BY). The use, distribution or reproduction in other forums is permitted, provided the original author(s) and the copyright owner(s) are credited and that the original publication in this journal is cited, in accordance with accepted academic practice. No use, distribution or reproduction is permitted which does not comply with these terms.
*Correspondence: Catalina Mena, c.mena.oliver@gmail.com