- 1Department of Food Sciences, Fundamental and Applied Research for Animal and Health, Faculty of Veterinary Medicine, University of Liège, Liège, Belgium
- 2Quality Partner sa, Liège, Belgium
The aim of this study was to obtain the growth parameters of specific spoilage micro-organisms previously isolated in minced pork (MP) samples and to develop a three-spoilage species interaction model under different storage conditions. Naturally contaminated samples were used to validate this approach by considering the effect of the food microbiota. Three groups of bacteria were inoculated on irradiated samples, in mono- and in co-culture experiments (n = 1152): Brochothrix thermosphacta, Leuconostoc gelidum, and Pseudomonas spp. (Pseudomonas fluorescens and Pseudomonas fragi). Samples were stored in two food packaging [food wrap and modified atmosphere packaging (CO2 30%/O2 70%)] at three isothermal conditions (4, 8, and 12°C). Analysis was carried out by using both 16S rRNA gene amplicon sequencing and classical microbiology in order to estimate bacterial counts during the storage period. Growth parameters were obtained by fitting primary (Baranyi) and secondary (square root) models. The food packaging shows the highest impact on bacterial growth rates, which in turn have the strongest influence on the shelf life of food products. Based on these results, a three-spoilage species interaction model was developed by using the modified Jameson-effect model and the Lotka Volterra (prey–predator) model. The modified Jameson-effect model showed slightly better performances, with 40–86% out of the observed counts falling into the Acceptable Simulation Zone (ASZ). It only concerns 14–48% for the prey–predator approach. These results can be explained by the fact that the dynamics of experimental and validation datasets seems to follow a Jameson behavior. On the other hand, the Lotka Volterra model is based on complex interaction factors, which are included in highly variable intervals. More datasets are probably needed to obtained reliable factors, and so better model fittings, especially for three- or more-spoilage species interaction models. Further studies are also needed to better understand the interaction of spoilage bacteria between them and in the presence of natural microbiota.
Introduction
During production and distribution steps, spoilage of meat and meat products may occur, rendering them unacceptable for human food consumption. Spoilage is mainly caused by microbial growth, which triggers alterations in the sensorial qualities of the product, with off-odor and off-flavor, discoloration, texture changes, etc. (Kreyenschmidt et al., 2010; Dalcanton et al., 2013; Pinter et al., 2014; Cauchie et al., 2017; Den Besten et al., 2017; Torngren et al., 2018). It is well known that the initial bacterial counts on meat and meat products is highly variable (Benson et al., 2014), but several studies have established that only a dominant fraction of the microbiota, designated as specific spoilage organisms (SSOs), contributes to spoilage (Nychas et al., 2008; Kreyenschmidt et al., 2010; Pennacchia et al., 2011; Benson et al., 2014; Zotta et al., 2019). In this context, predictive microbiology can be a helpful tool because the prediction of microbial growth, especially SSOs, enables food industries to optimize their production and storage managements, and thus reduce their economic losses (Kreyenschmidt et al., 2010; Fakruddin et al., 2012; Li et al., 2017; Tamplin, 2018).
During the last years, several models have been developed to predict the growth of SSOs in meat and meat products (Liu et al., 2006; Mataragas et al., 2006; Koutsoumanis, 2009; Kreyenschmidt et al., 2010; Dalcanton et al., 2013; Mejlholm and Dalgaard, 2013). But the majority of the developed models are based on the growth of two bacterial species in a food matrix (Vereecken et al., 2000; Giuffrida et al., 2007), most often to study the interaction between spoilage and pathogenic bacteria (Lebert et al., 2000; Mejlholm and Dalgaard, 2007; Giuffrida et al., 2009; Cornu et al., 2011; Ye et al., 2014; Correia Peres Costa et al., 2019; Pedrozo et al., 2019). Moreover, these models often describe the growth of the SSOs depending on the storage temperature (Dominguez and Schaffner, 2007; Gospavic et al., 2008; Kreyenschmidt et al., 2010; Psomas et al., 2011; Longhi et al., 2013; Antunes-Rohling et al., 2019) or the packaging conditions (Devlieghere et al., 1999; Chaix et al., 2015; Guillard et al., 2016; Couvert et al., 2019; Kapetanakou et al., 2019), but do not always consider the interaction of these storage conditions for the growth of spoilage bacteria (Rosso et al., 1995; Augustin and Carlier, 2000; Le Marc et al., 2002; Pinon et al., 2004; Dalcanton et al., 2018; Kakagianni et al., 2018; Nyhan et al., 2018; Correia Peres Costa et al., 2019).
As mentioned by Correia Peres Costa et al. (2019): “interaction models are usually intended to quantify how much the growth of one population is reduced by the growth of other populations.” In this context, two model approaches are generally used to describe the microbial interaction: (i) those based on the modified Jameson-effect phenomenon (Jameson, 1962; Cornu et al., 2011; Ye et al., 2014; Cauchie et al., 2017; Correia Peres Costa et al., 2019), and (ii) those based on the predator-prey models (Lotka Volterra equation) (Dens et al., 1999; Berlow et al., 2004; Powell et al., 2004; Giuffrida et al., 2007; Mounier et al., 2008; Cornu et al., 2011; Ye et al., 2014; Correia Peres Costa et al., 2019).
As described by Cornu et al. (2011), the Jameson-effect model assumes that: “(i) many microbial interactions in foods limit the maximum population density, without any significant effect on the lag time, and (ii) the growth of the minority population is only partly inhibited after the majority population count has reached its stationary phase [maximum critical population, MCP, expressed in log colony forming units (CFU)/g].” The modified Jameson-effect model makes the hypothesis that there is one single inhibition function for both populations; hence, both populations are similarly inhibited by the same limiting resource, the same waste products, and/or by change in pH (Cornu et al., 2011). Recently, Quinto et al. (2018) have developed a three-strain model based on the modified Jameson-effect equation for inoculated spoilage and pathogenic bacteria in a reconstituted sterile skimmed milk. This study considers the effect of two bacteria, Pseudomonas fluorescens and Listeria innocua, on the bacterial growth of Listeria monocytogenes. But the effect of the natural food microbiota on the growth of specific spoilage bacteria needs to be studied (Rouger et al., 2017) in order to predict bacterial growth resulting from several interactions between three or more spoilage species (Ye et al., 2014). This approach needs to be studied.
The Lotka Volterra model can be considered as a prey-predator model that includes competition for a common substrate (Cornu et al., 2011). As cited by Chauvet et al. (2002), the Lotka Volterra model for a three-species food chain approach can be considered as: “the lowest-level prey x is preyed upon by a mid-level species y, which, in turn, is preyed upon by a top-level predator z.” However, this hypothesis cannot always be applied in food matrix. Indeed, the growth of a bacterium (BA) presents simultaneously with other bacteria in a food matrix (BB and BC) can be affected by three different ways: (i) BA growth with a reduced growth rate after that BB and BC reach their maximal population densities (Nmax, expressed in log CFU/g), (ii) BA stops growing when BB and BC reach their Nmax, and (iii) BA declines when BB and BC reach their Nmax (Cauchie et al., 2017; Correia Peres Costa et al., 2019). It could be so interesting to develop a Lotka Volterra model for a three-species approach, by considering the effect of the natural food microbiota for the growth of specific spoilage bacteria. Also, this approach is, to the best knowledge of the authors, not available in the literature.
Based on these, the objectives of the present study were (i) to obtain the growth parameters of three specific spoilage micro-organisms previously isolated in minced pork (MP) samples, according to different storage conditions, (ii) to develop a three-spoilage species interaction model based on available models, under food wrap and modified atmosphere packaging, at isothermal conditions, and (iii) to validate this approach with naturally contaminated food samples stored under different storage conditions.
Materials and Methods
Sampling
Fresh MP samples were obtained from a local Belgian manufacturer at the day of the production, corresponding to the day of slaughtering. MP samples were packed by the manufacturer in a polypropylene tray under cling film (high film permeability).
According to the recipe, MP is composed of 100% pork mince (70% lean, 30% fat), no salt, no spices, no additives, no eggs, and no sugar are added.
At the day of the production, the water activity of the product was 0.98 ± 0.02 and the pH value was 5.80 ± 0.05 (n = 12). pH of the homogenized samples (5 g in 45 mL of KCl) was measured with a pH meter (Knick 765 Calimatic, Allemagne). The water activity was measured for homogenized samples on the basis of the relative humidity measurement of the air balance in the micro enclosure at 25 ± 0.4°C (Thermoconstanter TH200, Novasina, Switzerland).
Food samples were then stored at −20°C and irradiated by gamma irradiation (17.5 ± 0.4 kGy) at the same temperature (Sterigenics, Fleurus, Belgium) to limit the adverse effects of irradiation at this dose (Kim et al., 2002; Ham et al., 2017; Wang et al., 2018).
Bacterial Strains
As described in the study of Cauchie et al. (2019), three specific spoilage micro-organisms were previously isolated from different batches of naturally contaminated Belgian MP samples at the end of their use-by date. Samples were stored under two packaging (under air and modified atmosphere—30% CO2–70% O2) and three temperature conditions (4, 8, and 12°C). These predominant strains, represented more than 50% of the natural microbiota, were identified by 16S rRNA sequencing and used for experiments: Brochothrix thermosphacta (MM008), Leuconostoc gelidum (MM045) Pseudomonas spp. (P. fluorescens MM026 and Pseudomonas fragi MM014). P. fluorescens and P. fragi were used together because experiments were carried out in an exploratory approach to the proposed method, thus wishing to consider a wide diversity of Pseudomonas species most frequently found in MP.
Brochothrix thermosphacta MM008, L. gelidum (MM045), P. fragi MM014, and P. fluorescens MM026 were stored at −80°C in nutrient broth with 30% glycerol as a cryoprotective agent. Before use, strains were transferred from the −80°C culture collection to Brain Heart Infusion (BHI) broth for 48 h at 22°C. The bacterial suspensions were incubated overnight at 4°C before inoculation at stationary phase (7.00 log CFU/mL).
Inoculation Experiments
The three selected bacteria suspensions were inoculated on irradiated MP samples (1% v/w), in triplicate, for mono-culture and co-culture experiments with the objective to reach an average concentration of 3.0 log CFU/g (on the product).
Mono-culture experiments were performed by inoculation of individual bacterial strains: B. thermosphacta MM008, Pseudomonas spp. (P. fluorescens MM026, P. fragi MM014, 1:1 ratio), and L. gelidum MM045.
Co-culture experiments were performed by inoculation of a mix containing B. thermosphacta MM008, Pseudomonas spp. (P. fragi MM0014 and P. fluorescens MM0026, 1:1 ratio), and L. gelidum MM045 (1:1:1 ratio).
Non-inoculated control samples were homogenized, in triplicate, by adding the same quantity of sterile water only.
After inoculation, MP samples were mixed in a Kenwood mixer for 2 min in speed 2 (Kenwood, Mechelen, Belgium).
Inoculated and non-inoculated MP samples were then packed (50 g) in two different types of non-sterile packaging. The first packaging was a high barrier tray (187 × 137 × 36, polyester 10 μm, homo-polymer polypropylene 50 μm, NutriPack, France) under modified atmosphere (MAP, CO2 30%/O2 70% ± 0.1%) (Olympia V/G, Technovac, Italy) using packaging wrap (PP/EVOH/PP) with random gas measurements (CheckMate 3, Dansensor, France). The second packaging concerns a weak barrier tray (175 × 135 × 22, polystyrene) under food wrap packing (FW) using cling film (Clinofilm).
In this study, MP samples were stored during a 13-days shelf life at isothermal temperature: (i) 4°C (±1°C), (ii) 8°C (±1°C), (iii) and 12°C (±1°C), in climatic chambers (Sanyo MIR 254) (288 samples for four experiments, n = 1152 samples) (Supplementary Figure S1). A storage time of 13 days was defined in this study in order to obtain a sufficient number of points for modeling, allowing us to predict all the growth phases.
The codes used for each experiment, depending on the inoculated bacteria and storage conditions, are listed in Table 1.
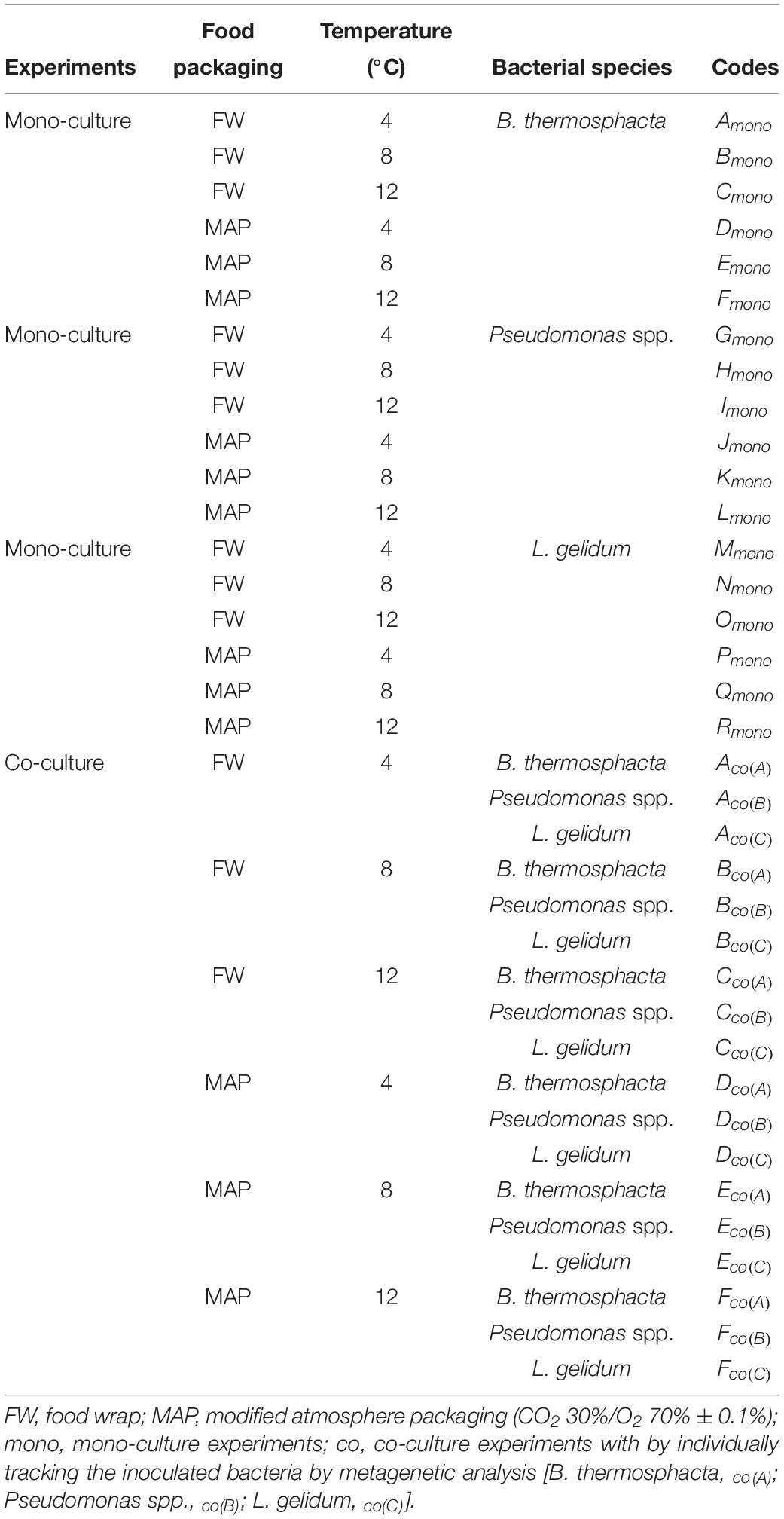
Table 1. List of the codes used for the experiments, depending on the inoculated bacteria and storage conditions.
pH and Gas Composition Measurements
At the first and the last day of storage, pH of the homogenized samples (5 g in 45 mL of KCl) was measured with a pH meter (Knick 765 Calimatic, Allemagne).
Oxygen and carbon oxygen concentrations of samples stored in modified atmosphere packaging were monitored daily (CheckMate 3, Dansesor, France).
Non-parametric statistical tests were used to compare the pH values and the gas measurements between samples. All tests were considered as significant for a p-value < 0.05.
Plate Count Enumeration
Twenty-five grams of product were put into a Stomacher bag with a mesh screen liner (80 μm pore size) (Biomérieux, Basingstoke, England, ref 80015) under aseptic conditions. Buffered peptone water (BPW, 10 g/L peptone, 5 g/L sodium chloride, #3564684, Bio-Rad, Marnes-la-Coquette, France) (225 mL) was automatically added to each bag (Dilumat, Biomérieux, Belgium) and the samples were homogenized for 2 min in a Stomacher (Bagmixer, Interscience, France). From this primary suspension, decimal dilutions in maximum recovery diluent (1.0 g/L peptone, 8.5 g/L sodium chloride, #CM0733, Oxoid, Hampshire, England) were prepared for microbiological analysis, and 0.1 mL aliquots of the appropriate dilutions were plated onto media for each analysis (Spiral plater, DW Scientific, England).
Total viable counts (TVCs) for the aerobic psychrophilic microbiota were enumerated on plate count agar (PCA agar, #3544475, Bio-Rad, Marnes-la-Coquette, France) after 72 h at 22°C (model 1535 incubator, Shel Lab, Sheldon Manufacturing, Inc., United States).
Plate counts were performed for mono- and co-culture experiments, and transformed in decimal logarithmic values. Samples for both experiments were enumerated at the first day of inoculation (day 0) and daily until the last day of storage (day 13). None specific agar media were used in co-culture experiments to separately enumerate the three inoculated species. Non-inoculated control samples were analyzed at day 0 and at day 13.
Using R software (R Core Team, 2019), an analysis of covariance (ANCOVA) was performed to evaluate the effect of the storage conditions on plate counts (FactoMineR package, Le et al., 2008). All tests were considered as significant for a p-value < 0.05.
16S rDNA Metagenetic Approach
A 16S rDNA metagenetic approach was used for mono- and co-culture experiments.
In mono-culture experiments, metagenetic analysis were performed at the first day of inoculation (day 0) and at the last day of storage (day 13) for samples stored at 4°C.
In co-culture experiments, samples were analyzed at day 0 and daily until day 13. The results were then correlated with plate counts in order to obtain estimate bacterial abundance over storage (see section “16S rDNA Data Analysis and Bacterial Abundance”).
No 16S rDNA metagenetic analysis was performed for non-inoculated control samples.
DNA Extraction and 16S rDNA Amplicon Sequencing
Bacterial DNA was extracted from each primary suspension, previously stored at –80°C, using the DNEasy Blood and Tissue kit (QIAGEN Benelux BV, Antwerp, Belgium) following the manufacturer’s recommendations. The resulting DNA extracts were eluted in DNAse/RNAse free water and their concentration and purity were evaluated by means of optical density using the NanoDrop ND-1000 spectrophotometer (Isogen, St-Pieters-Leeuw, Belgium). DNA samples were stored at –20°C until used for 16S rDNA amplicon sequencing.
PCR-amplification of the V1–V3 region of the 16S rDNA library preparation was performed with the following primers (with Illumina overhand adapters), forward (5′-GAGAGTTTGA TYMTGGCTCAG-3′) and reverse (5′-ACCGCGGCTGCTGG CAC-3′). Each PCR product was purified with the Agencourt AMPure XP beads kit (Beckman Coulter; Pasadena, CA, United States) and submitted to a second PCR round for indexing, using the Nextera XT index primers 1 and 2. Thermocycling conditions consisted of a denaturation step of 4 min at 94°C, followed by 25 cycles of denaturation (15 s at 94°C), annealing (45 s at 56°C), and extension (60 s at 72°C), with a final elongation step (8 min at 72°C). These amplifications were performed on an EP Mastercycler Gradient System device (Eppendorf, Hamburg, Germany). The PCR products of approximately 650 nucleotides were run on 1% agarose gel electrophoresis and the DNA fragments were plugged out and purified using a Wizard SV PCR purification kit (Promega Benelux, Leiden, Netherlands). After purification, PCR products were quantified using the Quanti-IT PicoGreen (ThermoFisher Scientific, Waltham, MA, United States) and diluted to 10 ng/μL. A final quantification, by quantitative (q)PCR, of each sample in the library was performed using the KAPA SYBR® FAST quantitative PCR (qPCR) Kit (KapaBiosystems, Wilmington, MA, United States) before normalization, pooling, and sequencing on a MiSeq sequencer using V3 reagents (Illumina, San Diego, CA, United States).
Bioinformatics Analysis
The 16S rRNA gene sequence reads were processed with MOTHUR. The quality of all sequence reads was denoised using the Pyronoise algorithm implemented in MOTHUR. The sequences were checked for the presence of chimeric amplification using ChimeraSlayer (developed by the Broad Institute1). The obtained read sets were compared to a reference dataset of aligned sequences of the corresponding region derived from the SILVA database of full-length rRNA gene sequences2 (version v1.2.11) implemented in MOTHUR. The final reads were clustered into operational taxonomic units (OTUs), using the nearest neighbor algorithm using MOTHUR with a 0.03 distance unit cutoff. A taxonomic identity was attributed to each OTU by comparison to the SILVA database, using an 80% homogeneity cutoff. As MOTHUR is not dedicated to the taxonomic assignment beyond the genus level, all unique sequences for each OTU were compared to the SILVA dataset 111, using a BLASTN algorithm. For each OTU, a consensus detailed taxonomic identification was given based upon the identity (<1% mismatch with the aligned sequence) and the metadata associated with the best hit (validated bacterial species or not).
16S rDNA Data Analysis and Bacterial Abundance
A correcting factor for 16S rDNA gene copy numbers was applied for any taxon i (Eq. 1).
Where Ai is the real abundance of 16S genes from the taxon in the sample, Nk is the number of reads for the taxon in the sample k, and Ci is determined by the genomic 16S copy number of that taxon. To obtain each gene copy number, Ribosomal RNA Database (rrnDB) (Stoddard et al., 2015) and EzBioCloud database (Yoon et al., 2017) were used.
Then, to compare the relative abundance of OTUs, the number of reads of each taxon was normalized as described by Chaillou et al. (2015). Reads counts of each taxon i in the sample k were divided by a sample-specific scaling factor (Si) (Eq. 2) (Fougy et al., 2016; Rouger et al., 2018):
Where Nri is the normalized number of reads for the taxon in the sample, Ai is the real abundance of 16S rRNA genes from that taxon obtained with a correcting factor for 16S rRNA gene copy numbers, and Sk is the normalization factor associated with sample k.
The sample-specific scaling factor was calculated by (Eq. 3):
Where Sk is the sample-specific scaling factor associated with sample k, Tk is the number of total reads in the sample k, and me is the median value of total reads for all the samples of the dataset. Reads counts of all samples were then transformed into a percentage of each OTU.
For co-culture experiments, the percentage of each OTUs was finally converted as a proportion of the TVC, obtained by classical microbiological analysis, in order to estimate counts for each species [in log10 CFU/g, and expressed as mean ± standard deviation (SD)] (Eq. 4), as described by Cauchie et al. (2017).
Where Cbacterial species is the estimated abundance concentration in the sample (log CFU/g), Ctotal microbiota is the bacterial concentration per samples in the PCA analysis (log CFU/g), and Preads of bacterial species is the proportion of reads for the bacterial species per sample in the metagenetic analysis (expressed in% of the total number reads in the sample).
All biosample raw reads were deposited at the National Center for Biotechnology Information (NCBI) and are available under de BioProject ID PRJNA590608. The raw data supporting the conclusions of this article will be made available by EC to any qualified researcher.
Approach Used to Develop the Interaction Model
As proposed by Correia Peres Costa et al. (2019), a step-wise approach (Figure 1) was followed to develop interaction models simulating the growth of specific spoilage micro-organisms.
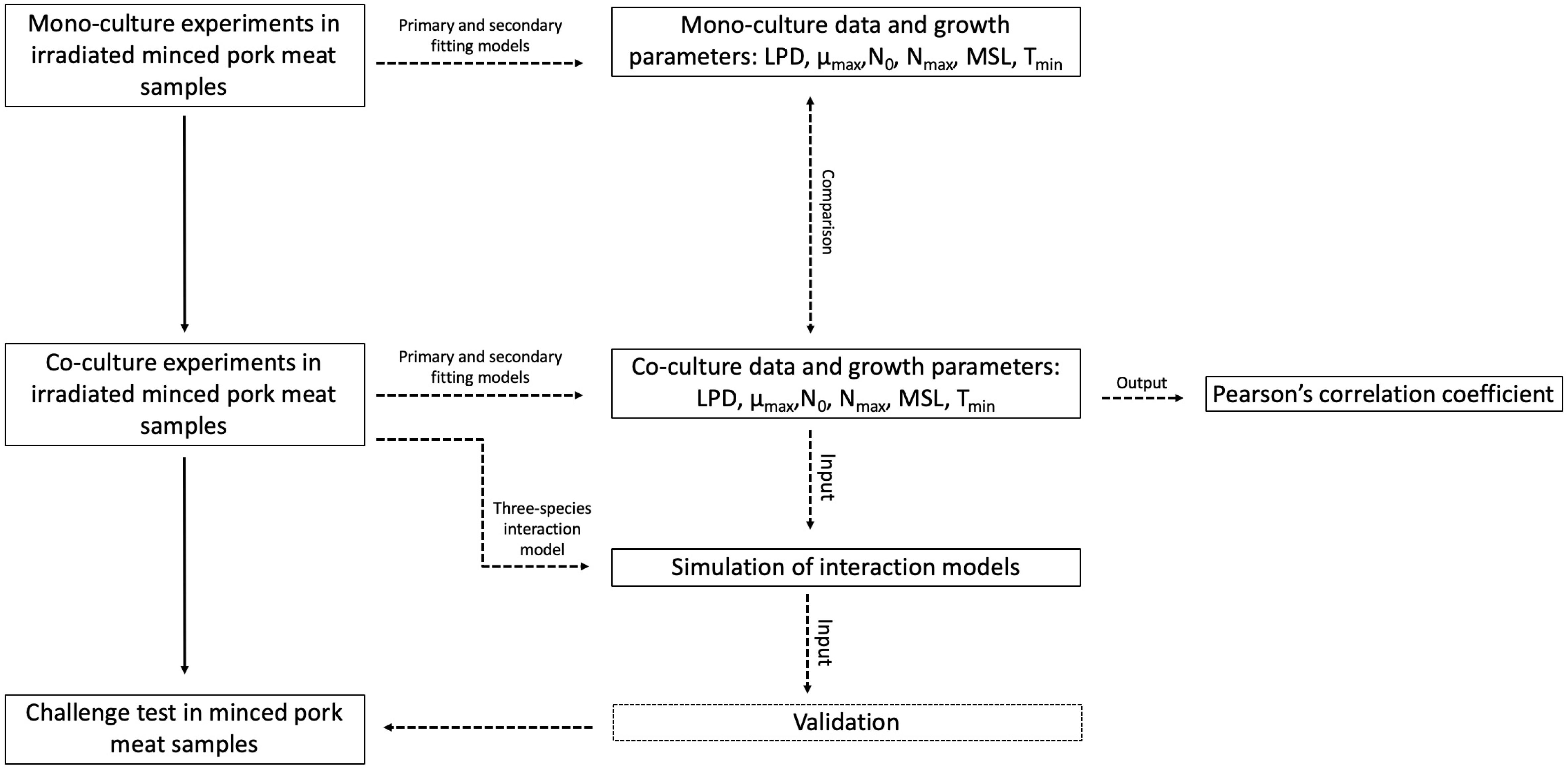
Figure 1. Schematic overview of the step-wise method used for the development of a three-spoilage species interaction model.
First, primary and secondary models were performed on mono-culture experiments to obtain the kinetic parameters (section “Primary and Secondary Model for the Fitting of Experimental Data”): lag phase duration (LPD, hours), maximum specific growth rate (μmax, 1/hours), initial and maximal population densities (N0 and Nmax, respectively, log CFU/g), theoretical minimal temperature of growth (Tmin,°C), growth rate obtained at the reference temperature of 20°C (μref, 1/hours), and minimal shelf life (MSL). The MSL is the time for the plate counts reaching approximatively 7.0 log CFU/g (expressed as Spoilage value according to the scientific literature, Sval).
Second, the same approach was applied for co-culture experiments in order to obtain the growth parameters (section “Primary and Secondary Model for the Fitting of Experimental Data”), and to compare them with those on mono-culture experiments (section “Correlations Between Growth Parameters”). The Pearson’s correlation coefficient was also used to choose the highest influencing growth parameters on the microbial shelf life of MP samples (section “Correlations Between Growth Parameters”).
Third, all of these results were used to estimate competitions parameters in interaction models for a three-species approach, based on the modified Jameson-effect model and Lotka Volterra model (section “Modeling Microbial Interactions for B. thermosphacta, Pseudomonas spp., and L. gelidum”).
Finally, validation of growth and interaction parameters obtained by the three-species models was performed with naturally contaminated MP samples stored under different conditions (section “Model Validation”).
Primary and Secondary Model for the Fitting of Experimental Data
The primary model of Baranyi and Roberts (1994) (Eq. 5) was fitted to the experiment dataset obtained for mono- and co-culture experiments. Experimental dataset is obtained by plate counts in mono-culture, and by estimate abundance based on metagenetic results in co-culture. All the data from the three replicates were modeled.
Based on primary fitting, the growth kinetic parameters were obtained.
Where Nt the bacterial population at any time t (log CFU/g); Nmax and N0, the maximum and initial population level, respectively (log CFU/g); μmax, the maximum specific growth rate (1/hour); and At, an adjustment function to define the LPD (Eq. 6).
Where h0 is simply a transformation of the initial conditions.
All fittings were performed using the nlsMicrobio package (function: baranyi, Baty and Delignette-Muller, 2013) from the open source R software (R Core Team, 2019).
The adequacy of the primary models to describe the experimental data was observed by using the root-mean-square error of the residuals (RrMSE, SD of the residuals) (Eq. 7) and the coefficient of multiple determination (R2, the fraction of the square of the deviations of the observed values about their mean explained by the equation fitted to the experimental data) (Eq. 8).
Where RSS, the residual sum of square; DF, the degrees of freedom; n, the number of data points; s, the number of parameters of the model; xi0, the observed values; and xif, the fitted values.
Where n, the total number of data points; mean, the average value from all observed values.
A reparameterized version of the square root secondary model (Ratkowsky et al., 1982) (Eq. 9) was then used in R (R Core Team, 2019) to assess the effects of temperature on the growth rates.
Where μref is the reference growth rate obtained at Tref = 20°C (1/hours), T is the temperature (°C), and Tmin is the minimal temperature for growth (°C) found in the scientific literature for the studied bacterial species: −3.36°C for B. thermosphacta (Leroi et al., 2012); −5.00°C for Pseudomonas spp. (Rashid et al., 2001); and + 1.00°C for L. gelidum (Kim et al., 2000).
For comparison, Tmin values were also estimated by the Rosso primary model (Rosso et al., 1995) and the square root model (Ratkowsky et al., 1983) (Eq. 10).
Where μmax is the maximal growth rate (1/hours), b is a constant parameter obtained by linear regression, T is the temperature (°C), and Tmin is the minimal temperature for growth (°C).
For secondary models, the coefficient of multiple determination (R2) and the goodness of fit (GoF, root-meat-square error of the model, analogous to the accuracy factor) were used (Eq. 11).
Extracts of the code in R for primary and secondary fittings are given in Supplementary Material (R-commands 1).
Correlations Between Growth Parameters
An analysis of covariance was performed to evaluate if the maximal bacterial growth rates (μmax) were significantly different between the two food packaging. All tests were considered as significant for a p-value of < 0.05. Extracts of the code in R for ANCOVA analysis are given in Supplementary Material (R-commands 2).
Using R software (R Core Team, 2019), correlations between the minimal shelf life (MSL) and the growth parameters (μmax, LPD, N0, Nmax) were obtained by the Pearson’s correlation coefficient (r) in mono-culture and co-culture experiments (Liu et al., 2006; Miks-Krajnik et al., 2016). High correlations were considered when |r| > 0.7000 (Miks-Krajnik et al., 2016). The best influencing growth parameter on the microbial shelf life was chosen according to the Pearson’s correlations coefficient.
Then, a reduction ratio (α) was calculated to quantify the interaction effect on μmax by inoculated bacteria in co-culture experiments (Eq. 12) (Correia Peres Costa et al., 2019).
Where α is the reduction ratio; pco and pmono are the growth parameters obtained in co-culture and mono-culture experiments, respectively.
Modeling Microbial Interactions for B. thermosphacta, Pseudomonas spp., and L. gelidum
Two well-known interactions models for two-species were modified to predict the simultaneous growth of the three-inoculated spoilage bacteria in irradiated MP samples: the modified Jameson-effect model and the Lotka Volterra model (Cornu et al., 2011; Correia Peres Costa et al., 2019).
As presented by Cornu et al. (2011) and Quinto et al. (2018), a modified generic primary growth model can be written as Eq. 13.
Where is the relative or instantaneous growth rate of the microorganism, Nt is the bacterial concentration at time t (log CFU/g), μmax is the maximum growth rate (1/h), α(t) is an adjustment function, and f(t) is an inhibition function, defined as Eqs 14 and 15:
Where LPD is the lag phase duration (hours) and Nmax is the maximal population density (log CFU/g).
Based on Eq. 13, an alternative deceleration function can be added for modeling the interaction of two bacterial species (Jameson-effect model) (Eq. 16) (Mejlholm and Dalgaard, 2007; Cornu et al., 2011).
Where N is the cell concentration (log CFU/g) at time t (h), μmax is the maximum specific growth rate (1/h), and Nmax is the maximum population density (log CFU/g).
In the modified Jameson-effect model, the deceleration function can be replaced by Eq. 17 (Mejlholm and Dalgaard, 2007; Cornu et al., 2011; Quinto et al., 2018; Cadavez et al., 2019).
Where Nt is the bacterial concentration at time t (log CFU/g), Nmax(t) is the maximal population density (log CFU/g), and NMCP(t) is maximum critical population (log CFU/g) that the bacterium should be reached to inhibit the growth of the other populations. MCP is inferior to its own maximum population density (Nmax) (Cornu et al., 2011; Correia Peres Costa et al., 2019).
Using R software (R Core Team, 2019), the modified Jameson-effect model (Eq. 17) was applied on mono-culture experiment data with the functions of Baranyi, Buchanan and without-lag (package nlsMicrobio, Baty and Delignette-Muller, 2013). The function without lag shown the best fitting in all cases (Supplementary Table S1). This model was then selected in the rest of the study, by using the growth parameters obtained on co-culture experiments. Extracts of the code in R for the modified Jameson-effect models for two species are given in Supplementary Material (R-commands 3).
For a three-species mixed culture model, Quinto et al. (2018) recently proposed a modification of the logistic deceleration model (Eq. 18).
Where NA(t), NB(t), and NC(t) are the cell concentration of microorganism A, B, or C in co-culture at time t; Nmaxtot is the maximal total population density (including all species present) and consequently the overall carrying capacity of the system from the three-species co-cultured.
However, this study only considers the effect of P. fluorescens and L. innocua on the bacterial growth of L. monocytogenes.
In our study, the aim of co-culture experiments was to consider the global effect of three inoculated bacterial species and the bacterial interaction on each other.
According to this, the modified Jameson-effect model was re-defined for a three-species model that was used in this study (Eq. 19).
Where N is the cell concentration (log CFU/g) at time t (h), μmax is the maximum specific growth rate (1/h), α(t) is an adjustment function, and NMCP is the maximum critical population of each bacterium (log CFU/g).
Extracts of the code in R for the three-species modified Jameson-effect models are given in Supplementary Material (R-commands 4).
In the two-species model based on the Lotka Volterra equation, the deceleration function can be replaced by Eq. 20 (Cornu et al., 2011), which includes empirical parameters reflecting the degree of interaction between microbial species (FAB and FBA) (Liu et al., 2006; Cornu et al., 2011; Cadavez et al., 2019; Correia Peres Costa et al., 2019).
Where the parameters FAB and FBA are the coefficients of interaction measuring the effects of one species on the other.
Using R software (R Core Team, 2019), the Lotka Volterra model (Eq. 20) was also re-defined for a three-species interaction model, represented by Eq. 21.
Where N is the cell concentration (log CFU/g) at time t (h), μmax is the maximum specific growth rate (1/h), α(t) is an adjustment function, FA,B,C are the coefficient of interaction measuring the effects of one species on the others, and Nmax is the maximum population density (log CFU/g).
Extracts of the code in R for the three-species Lotka Volterra models are given in Supplementary Material (R-commands 5).
Comparison of the two models was assessed by root-mean-square error (RMSE) and coefficient of determination (R2) (Correia Peres Costa et al., 2019), as previously described in the section above (Section 2.7.1.).
Model Validation
Validation of the developed three-species interaction models was performed using a new dataset of experimental data.
Fresh MP samples were obtained from a local Belgian manufacturer at the day of the production, corresponding to the day of slaughtering. MP samples were packed by the manufacturer in a polypropylene tray under cling film. Samples have the same composition as described above.
Samples were not irradiated and not inoculated in order to follow the dynamics of the natural food microbiota. MP samples were also packed (50 g) in two different packaging, in triplicate.
The first packaging was a tray (187 × 137 × 36, polyester 10 μm, homo-polymer polypropylene 50 μm, NutriPack, France) under modified atmosphere (MAP, CO2 30%/O2 70% ± 0.1%) (Olympia V/G, Technovac, Italy) using packaging wrap (PP/EVOH/PP) with random gas measurements (CheckMate 3, Dansensor, France). The second packaging consisted in a tray (175 × 135 × 22, polystyrene) under FW using cling film (Clinofilm).
In this study, MP samples were stored during a 13 days shelf life at isothermal temperature: (i) 4°C (±1°C), (ii) 8°C (±1°C), (iii) and 12°C (±1°C), in climatic chambers (Sanyo MIR 254).
Samples (n = 288) were then analyzed at the first day of inoculation (day 0) and daily until the last day of storage (day 13). Analyses were performed by classical plate counts and 16S rDNA metagenetics, as methods previously described in the sections above (sections “16S rDNA Metagenetic Approach” and “Approach Used to Develop the Interaction Model”), in order to estimate bacterial counts over the storage.
The performance of the developed interaction models was evaluated by the acceptable simulation zone (ASZ) approach. Model performance is considered acceptable when at least 70% of the observed log counts values are within the ASZ, defined as ± 0.5 log-units from the simulated concentration in log units (Correia Peres Costa et al., 2019).
Results
16S rDNA Metagenetic Results
Despite of the inability of differentiation between viable and non-viable cells by the culture-independent DNA-based methods used, high level (>95%) of relative abundance for each inoculated bacterium was observed for mono-culture experiments (Supplementary Figure S2).
The relative abundance results for co-culture experiments (expressed in%) at genus levels (>1%) are represented in cumulated histograms for all samples in FW (Figure 2) and MAP (Figure 3). These data including the relative abundance of sequences are also summarized in Supplementary Table S2.
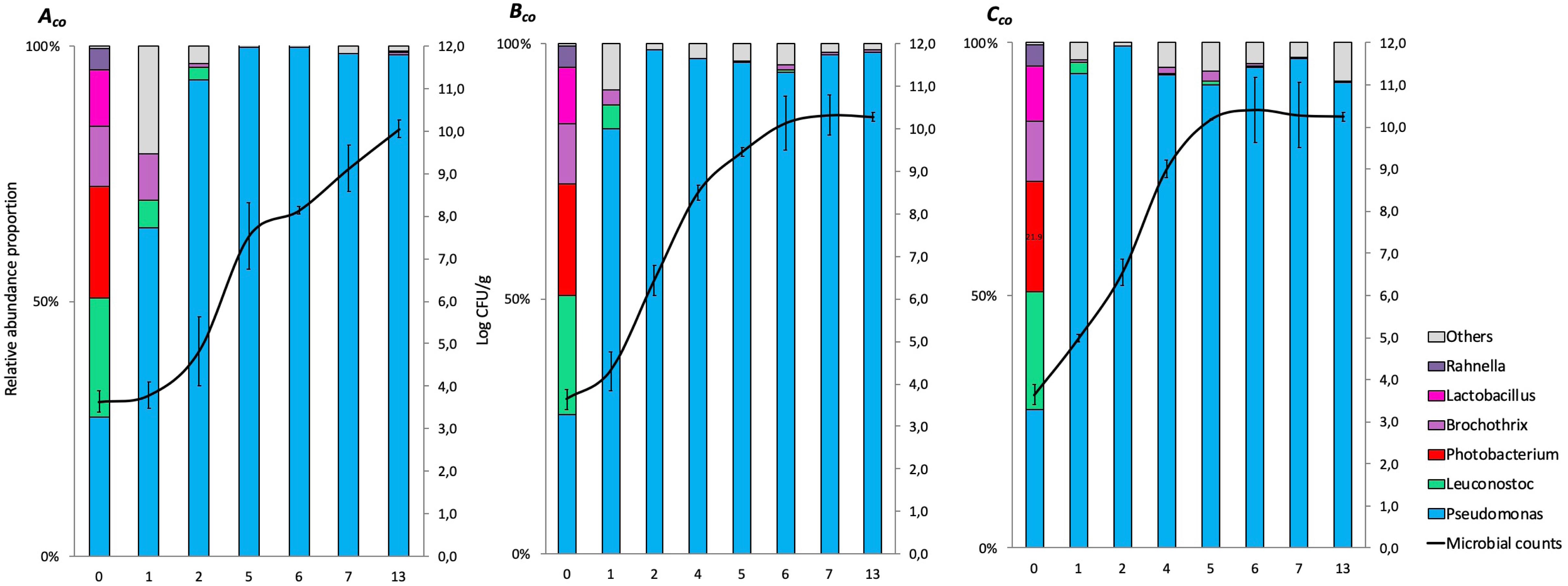
Figure 2. Cumulated histograms of the relative abundance (%) of taxa and the dynamics of the bacterial community identified by metagenetics at genus levels in co-culture experiment during storage in food wrap (Aco, at 4°C; Bco, at 8°C; Cco, at 12°C). At genus levels, the taxa representing < 1% in relative abundance were merged in the category of “Others.” The solid represents the plate counts (means and standard deviation of the three replicates).
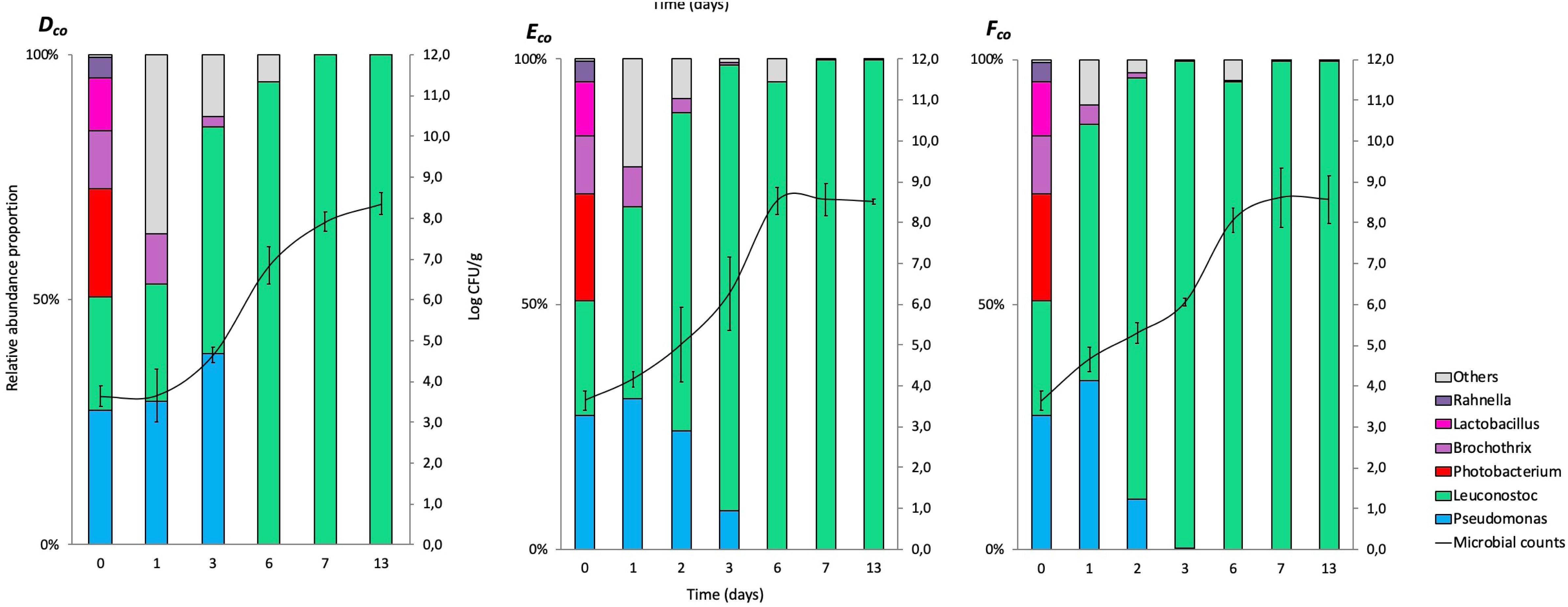
Figure 3. Cumulated histograms of the relative abundance (%) of taxa and the dynamics of the bacterial community identified by metagenetics at genus levels in co-culture experiment during storage in modified atmosphere packaging (Dco, at 4°C; Eco, at 8°C; Fco, at 12°C). At genus levels, the taxa representing < 1% in relative abundance were merged in the category of “Others.” The solid represents the plate counts (means and standard deviation of the three replicates).
The taxa representing < 1% in relative abundance were merged in the category of “Others.” “Others” are mainly composed by the genera Aeromonas, Arthrobacter, Bacteroides, Carnobacterium, Chryseobacterium, Enterococcus, Flavobacterium, Kurthia, Lactobacillus, Lactococcus, Mannheimia, Massilia, Micrococcus, Moraxella, Myroides, Ottowia, Peptococcus, Photobacterium, Porphyromonas, Propionibacterium, Rothia, Serratia, and Staphylococcus. Full data on taxa found in high (>1%) and low (<1%) frequencies will be made available by EC to any qualified researcher.
At day 0, small differences between the distribution of read percentages for the three inoculated bacteria are observed (11.8, 27.4, and 23.3% for Brochothrix, Pseudomonas, and Leuconostoc, respectively).
At day 3 in FW, Brochothrix became under the detection limit. At this same time, Pseudomonas became the most represented genus (>90%), and remained during the 13 days of storage.
In MAP, Leuconostoc and Pseudomonas were equally distributed during the first days of storage, but Leuconostoc became the most represented genus (>90%) after 3 days and until the end of storage.
Plate Counts and Estimated Abundance
In mono-culture experiments, plate counts for B. thermosphacta, Pseudomonas spp., and L. gelidum increased during the shelf life with increasing the temperature (Table 2).
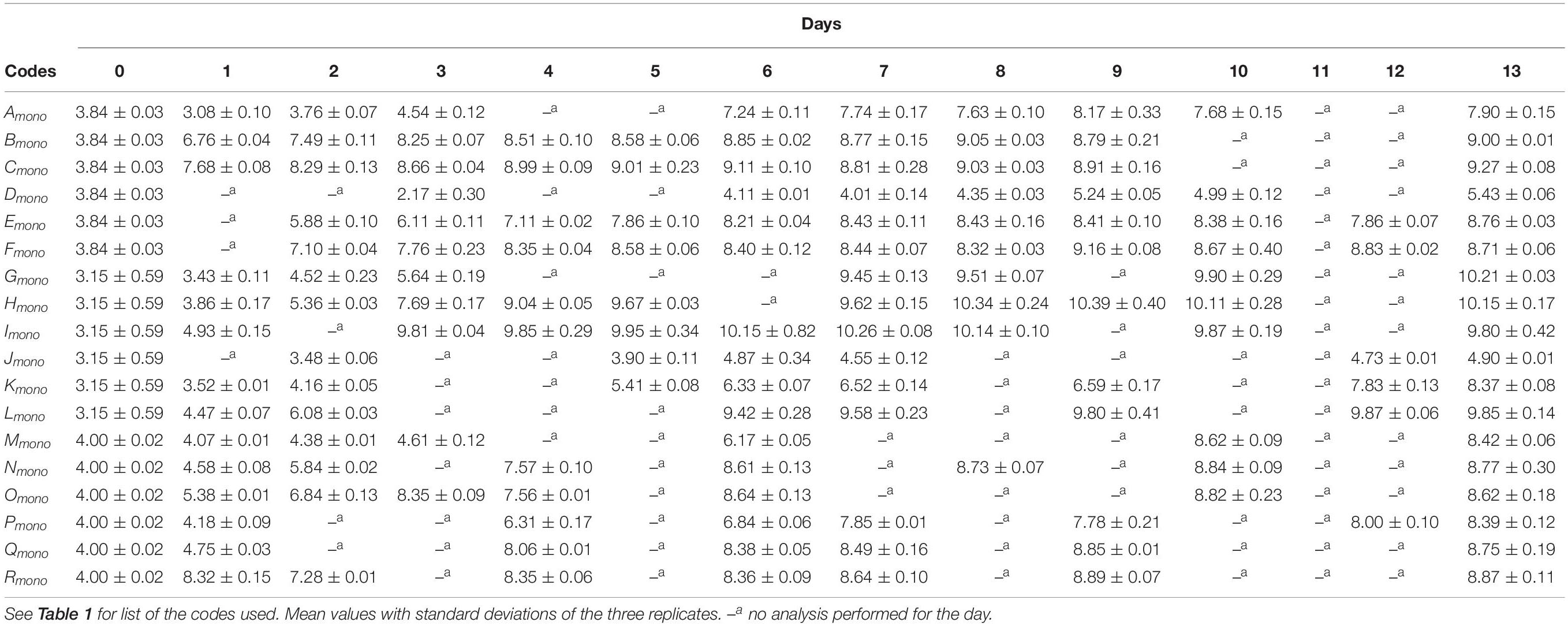
Table 2. Microbiological counts (log CFU/g) for mono-culture experiments in minced pork samples stored during 13-days shelf life, at constant temperature, in food wrap (FW) and modified atmosphere packaging (MAP, CO2 30%/O2 70% ± 0.1%).
At the end of the shelf life, the bacterial count was higher than 7.0 log CFU/g, except for some samples stored in MAP. During the storage, a high growth rate and a more rapidly reached stationary phase were also correlated to FW and the highest storage temperatures.
No bacterial growth was observed on PCA for the control samples (limit detection < 3.0 log CFU/g) (data not shown in this paper).
For co-culture experiments, the metagenetic data were combined with the plate counts results in order to obtain estimated bacterial counts (Table 3).
As previously observed, estimate counts increased during the shelf life with increasing the temperature. At the end of the shelf life, the bacterial count was over 7.0 log CFU/g, except for B. thermosphacta and Pseudomonas spp. stored in MAP. During the storage, the same growth profiles as mono-culture experiments were observed.
pH and Gas Measurements
A significant increase of pH is observed for MP samples inoculated by Pseudomonas spp. (7.54 ± 0.76, n = 5, p-value = 0.01) compared to the control samples (5.79 ± 0.05, n = 10).
In co-culture experiments, pH values at the end of the shelf life were not different to control samples (5.87 ± 0.02, n = 5) (Supplementary Figure S3).
A relatively stable concentration of carbon dioxide was observed in MAP at the end of the shelf life. Except for MP samples inoculated with Pseudomonas spp., which reached a higher significant carbon dioxide value (100.0 ± 0.1%) at 12°C (Supplementary Figure S4).
Microbial Growth Parameters
Results of the primary and secondary model fittings for mono- and co-culture experiments are shown in Tables 4, 5. Growth parameters from mono-culture experiments are based on plate counts, and those from co-culture experiments are based on estimate abundance (obtained by the association of metagenetic and plate counts results).
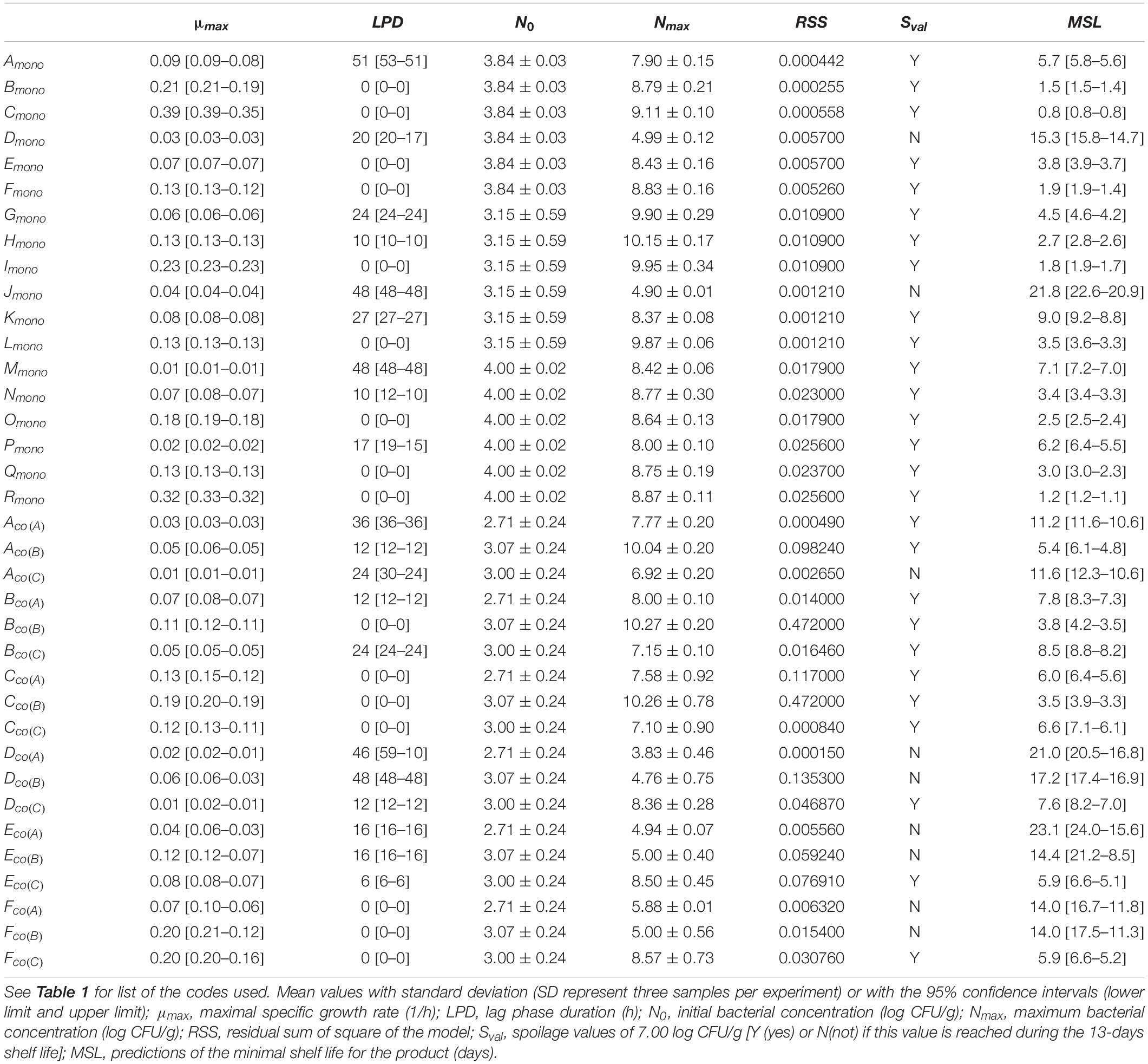
Table 4. Observed kinetic parameters of mono- and co-culture experiments, calculated by Baranyi equation without interactions.
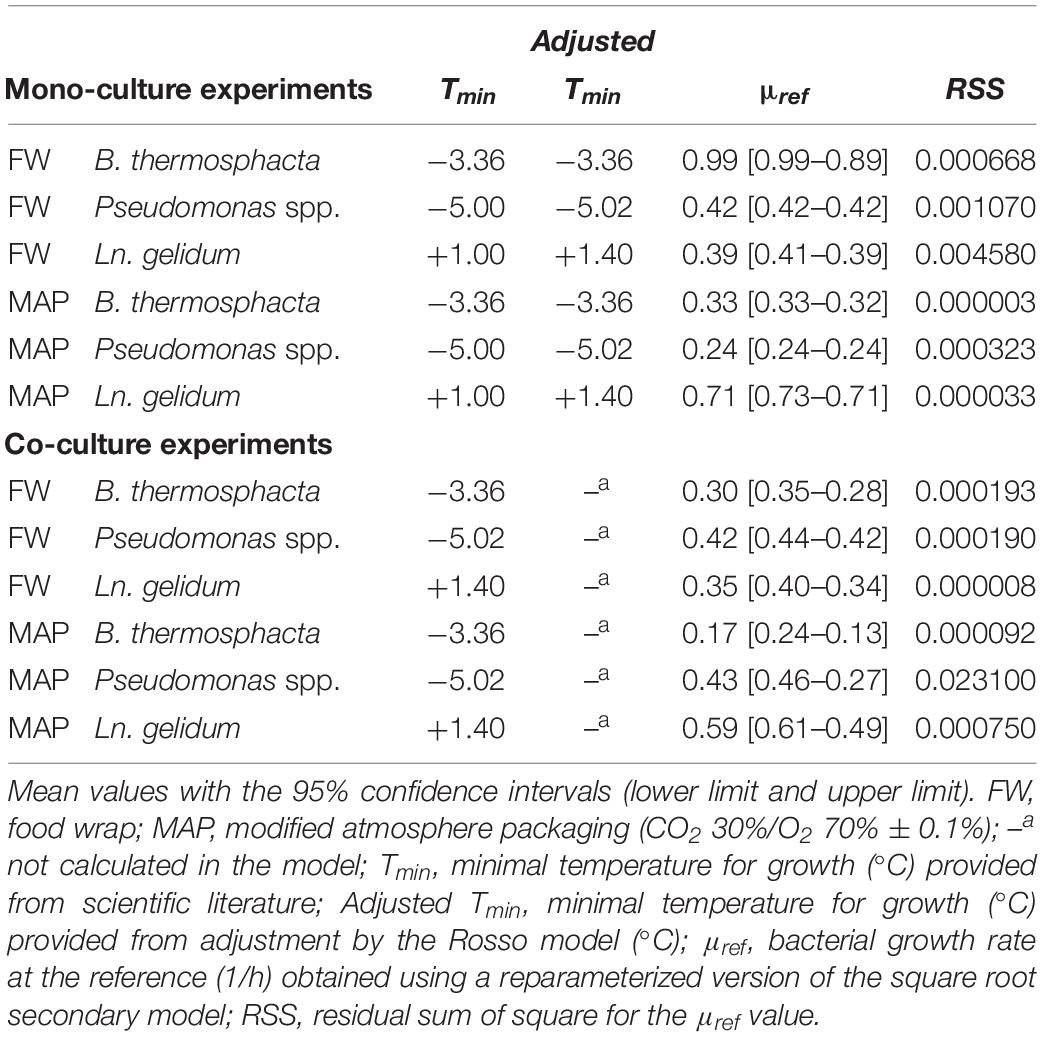
Table 5. Estimation of the secondary parameters obtained by the square root model without interactions.
Good fit indexes were obtained in all cases (Supplementary Tables S3, S4).
Growth parameters showed different dynamic changes depending on storage temperature: a high storage temperature is correlated to a high growth rate during exponential phase and a lower lag-time. These growth parameters are also higher in FW than in MAP.
The MSL value is more rapidly reached in FW, except for L. gelidum.
Moreover, the Sval was never reached in MAP for MP samples inoculated by Pseudomonas spp. and B. thermosphacta during the 13-days shelf-life at 4°C.
Based on these results, the evolution of μmax between a large range of temperature (from −6 to +25°C) in FW and MAP was performed for mono- and co-culture experiments (Figure 4).
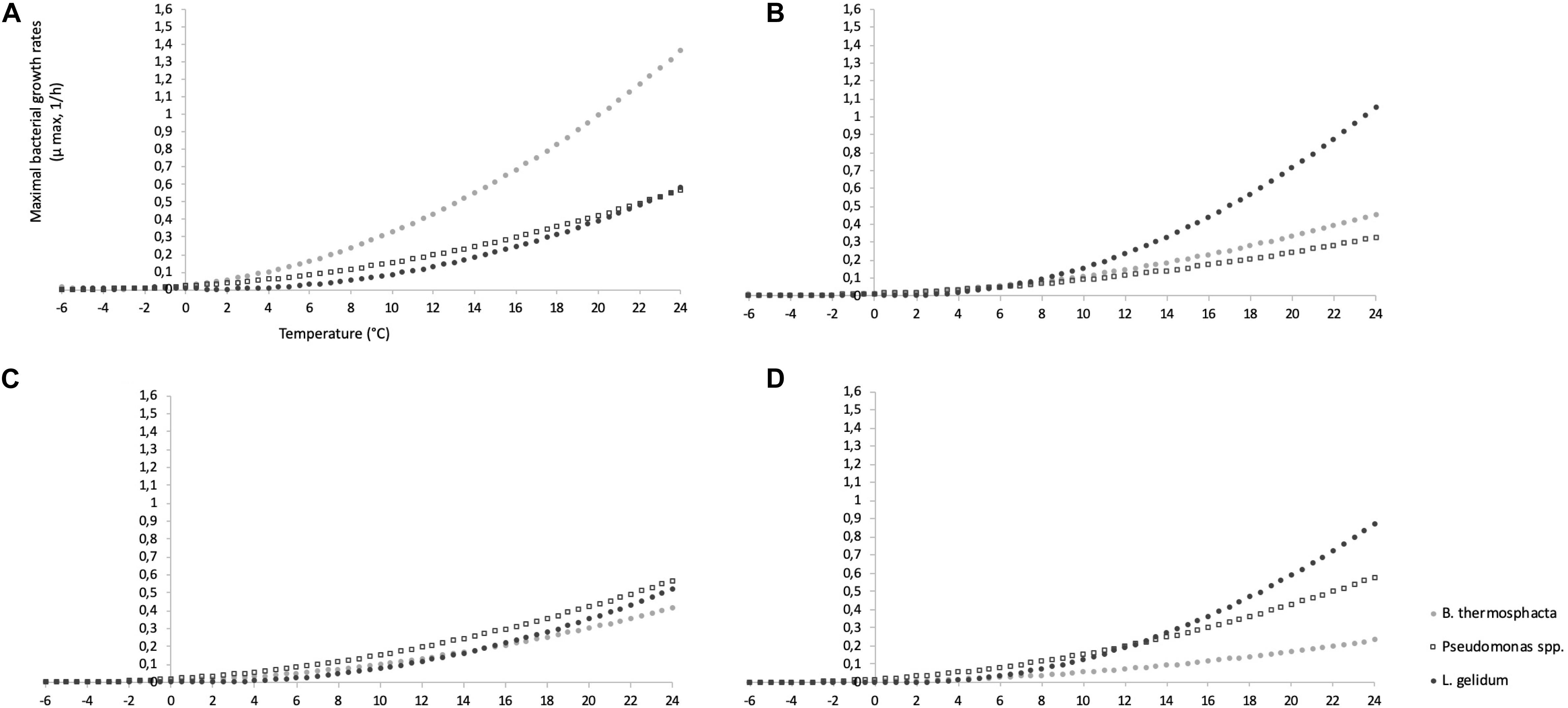
Figure 4. Evolution of μmax between a large range of temperature (from −6 to + 25°C) for mono-culture experiments in FW (A) and MAP (B), and for co-culture experiments in FW (C) and MAP (D).
It can be clearly observed that L. gelidum had a highest growth rate in MAP, while it concerns B. thermosphacta in FW in mono-culture experiments. B. thermosphacta had the lowest one in co-culture experiments.
Correlations Between Growth Parameters Obtained in Mono- and Co-culture Experiments
Correlations between growth parameters of B. thermosphacta, Pseudomonas spp., and L. gelidum for mono-culture and co-culture experiments are presented in Table 6.
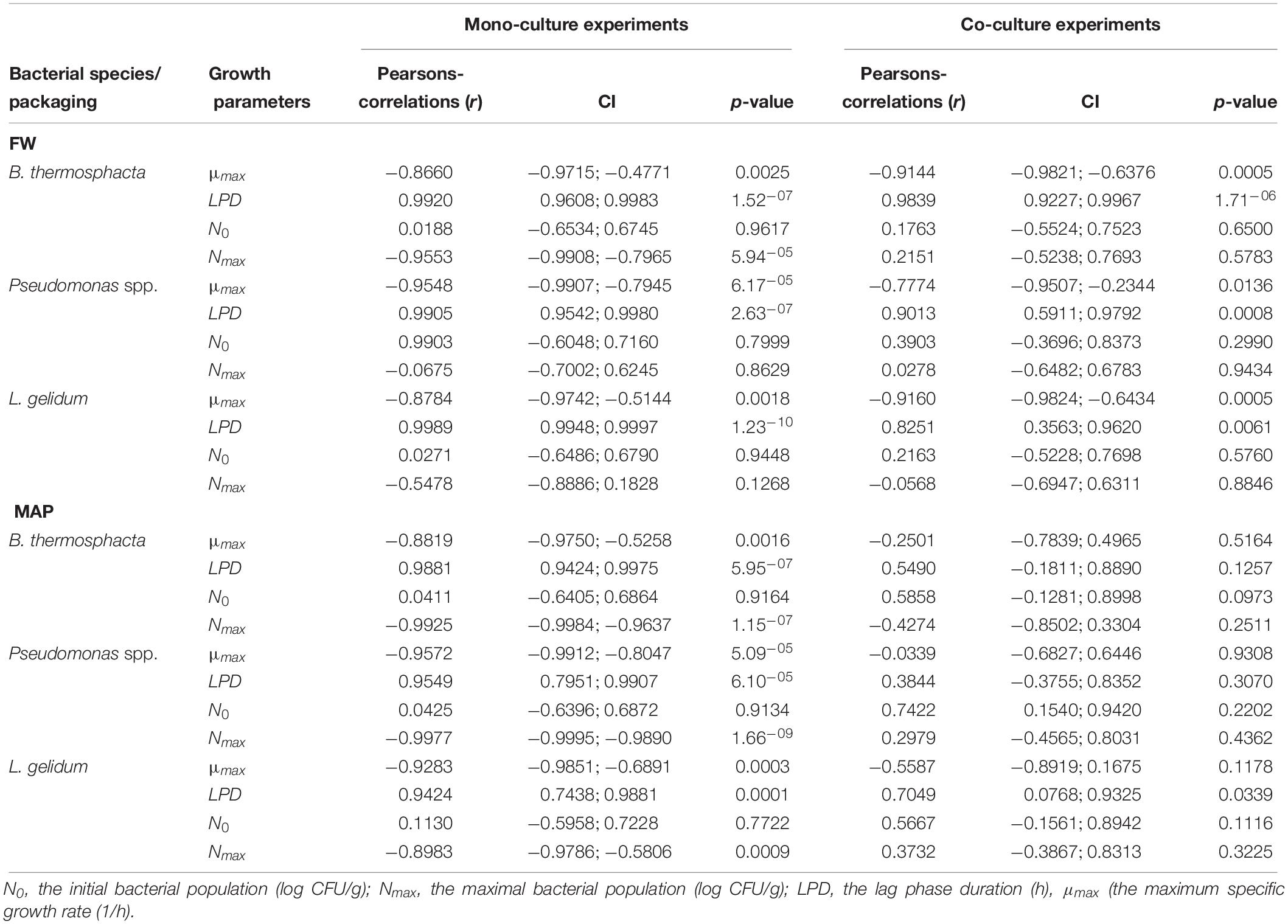
Table 6. Correlations between growth parameters and the minimal shelf life (MSL) for mono-culture and co-culture experiments.
It can be observed that the maximum specific growth rate (μmax) of micro-organisms was negatively correlated with microbial shelf life. The correlation was higher in mono-culture (−0.8660 to −0.9572) than in co-culture experiments (−0.0339 to −0.9160).
Lag phase duration (LPD) of all micro-organisms showed good correlation. High correlations of μmax and LPD were observed in FW for co-culture experiments.
N0 showed little correlations than the two others parameters, except for mono-culture of Pseudomonas spp. stored in FW.
Moreover, no obvious correlation has been shown between Nmax with shelf life for co-cultures experiments.
In conclusion, the results showed in our study that the microbial shelf life of MP samples is mainly correlated with μmax and LPD than by Nmax and N0. Even if the correlations are lower for experiments carried out in co-culture under MAP.
It was also showed that μmax seems to be mainly influenced by the food packaging (Table 7), and by the interaction of the storage conditions applied in this study (packaging and temperature). These results were confirmed by the study of the reduction ratio α (Figure 5). B. thermosphacta and L. gelidum presented a higher reduction in FW. But an increase was observed for Pseudomonas spp. in MAP. Indeed, μmax of Pseudomonas spp. was 0.04, 0.08, and 0.13, at 4, 8, and 12°C, respectively, in mono-culture experiments. While the parameter was gradually increasing to 0.06 (α = −50.0%), 0.12 (α = −50.0%), and 0.20 (α = −53.8%), at 4, 8, and 12°C, respectively, in co-culture experiments. However, Nmax values of this bacterium were lesser in co-culture than in mono-culture experiments.
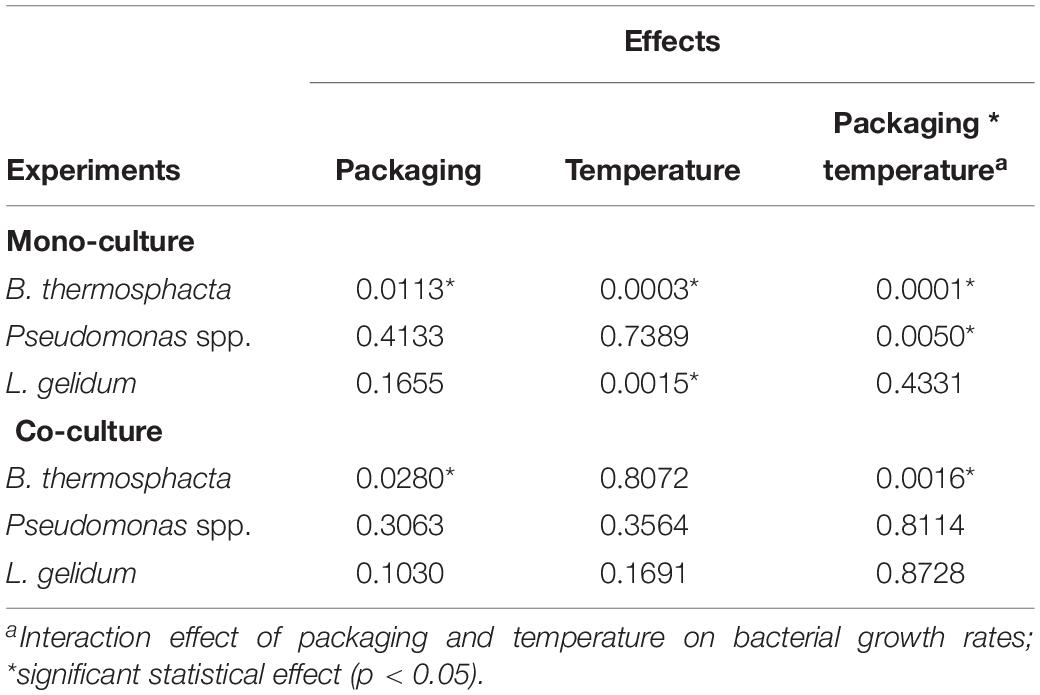
Table 7. Effect of food storage conditions on the maximal bacterial growth rates (μmax, 1/h) for mono- and co-cultures experiments (analysis of covariance, ANCOVA).
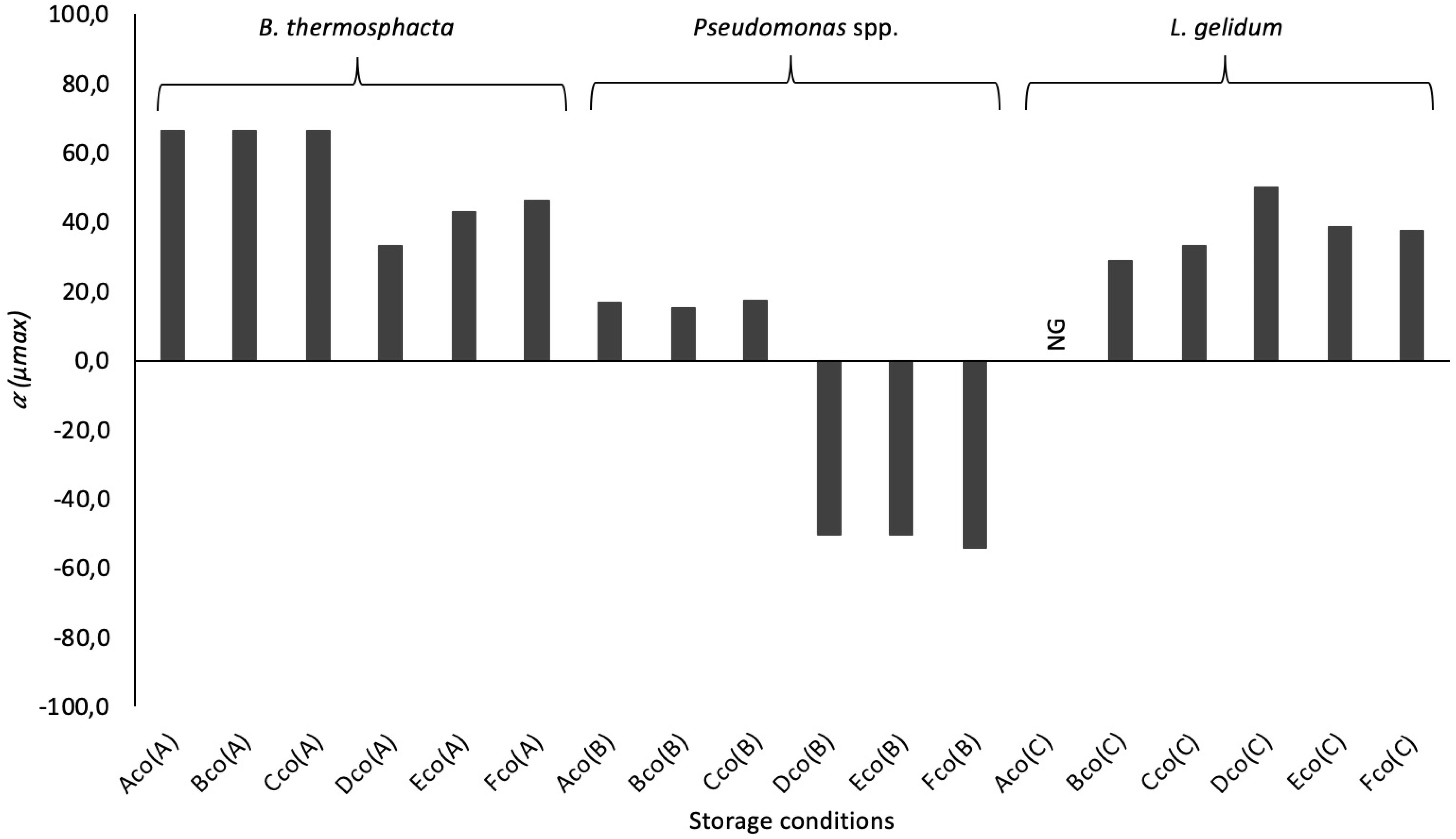
Figure 5. Reduction ratio (α), in%, of the parameters μmax for B. thermosphacta, Pseudomonas spp., and L. gelidum in co-culture experiments at different storage conditions (see Table 1 for legend). The negative bars represent an increase in co-culture for the specific parameters. No growth of bacteria (NG) was only observed for Ln. gelidum in MAP at 4°C.
Three-Species Interaction Models and Validation Step
Estimated growth parameters and goodness-of-fit indexes for the two developed interaction models are available in Table 8.
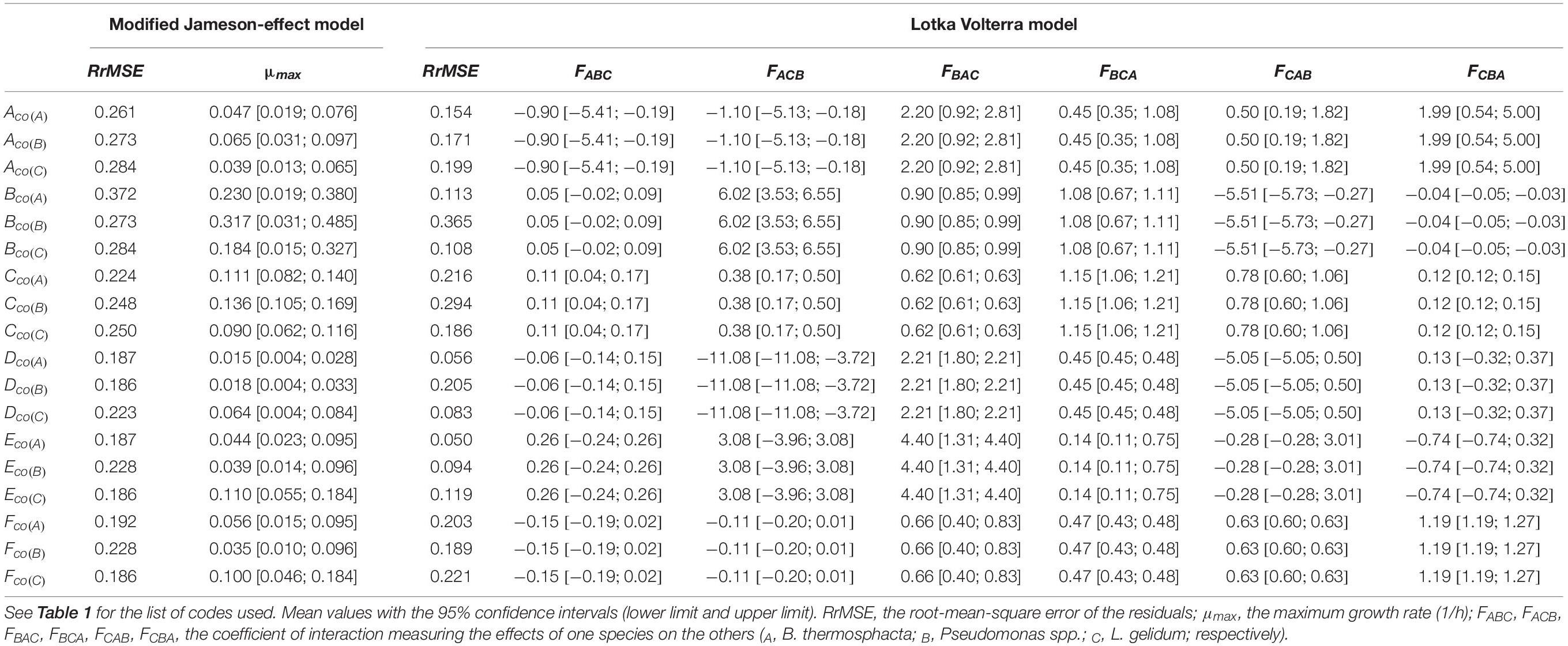
Table 8. Estimated growth parameters of the three-species modified Jameson-effect and Lotka Volterra models, with goodness-of-fit indexes.
The Lotka Volterra model showed lower RrMSE values but the interaction factors are sometimes included in high intervals.
Simulations provided by the predictive models based on the modified Jameson-effect model and the Lotka Volterra equations are represented in Figures 6, 7.
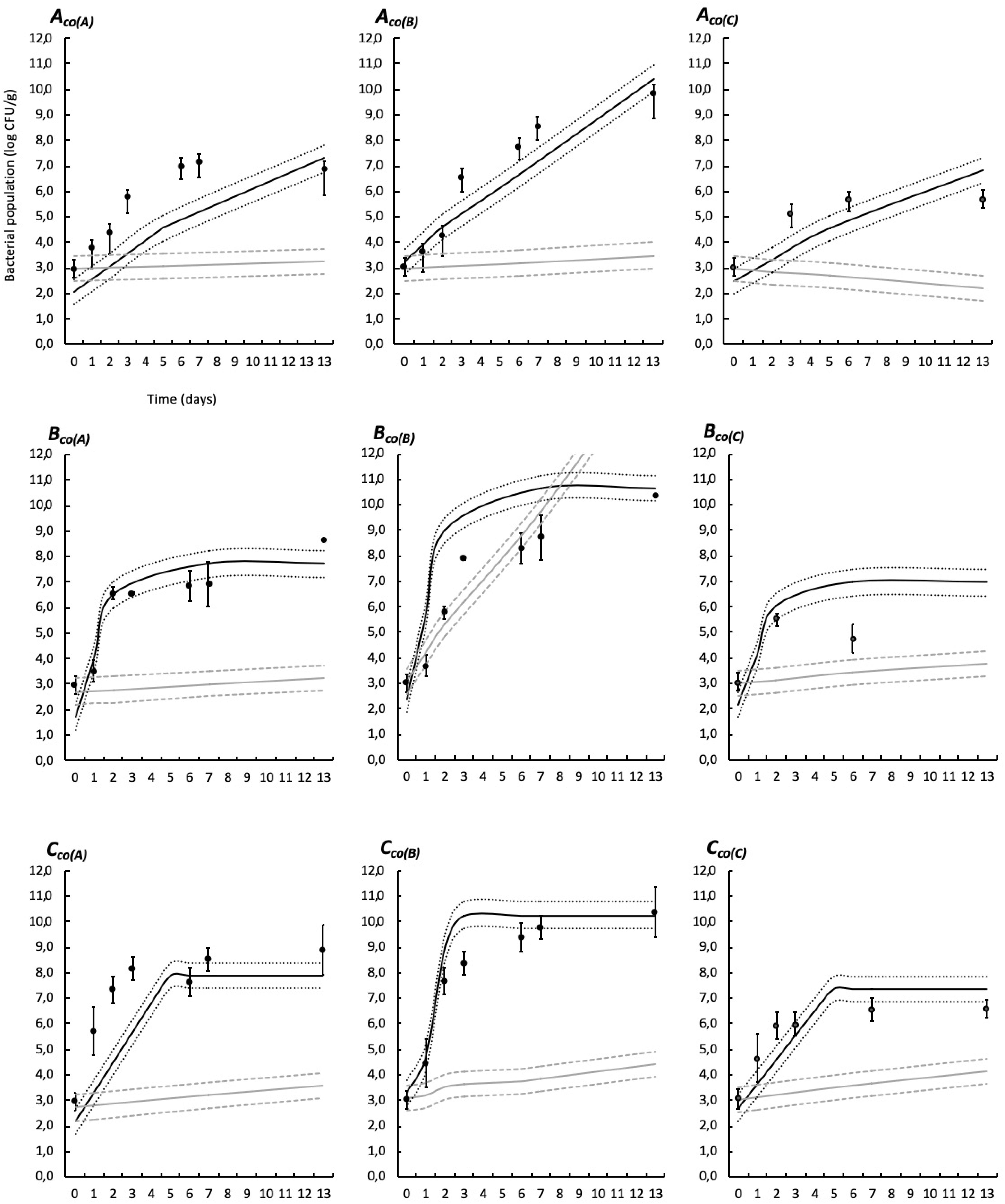
Figure 6. Experimental observed data (validation dataset, means, and standard deviation of the three replicates) and simulations provided by the predictive models based on the modified Jameson-effect equation and on the Lotka Volterra equation in food wrap. See Table 1 for list of the codes used. Black solid lines represent the Jameson-effect model and gray solid lines represent the Lotka Volterra model. Dashed and dotted lines represent the acceptable simulation zone (ASZ) used to compare observations versus predictions of the interaction models.
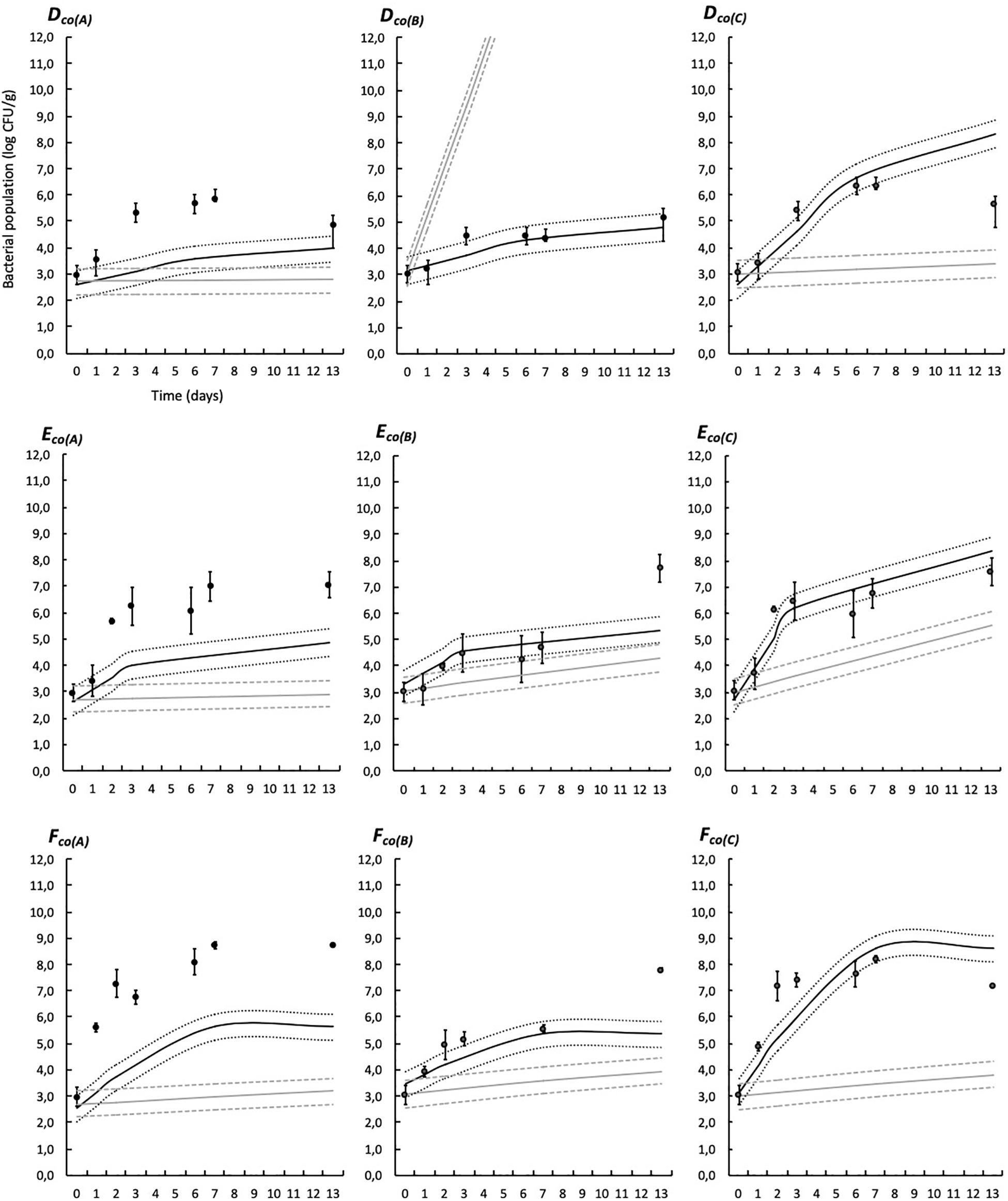
Figure 7. Experimental observed data (validation dataset, means, and standard deviation of the three replicates) and simulations provided by the predictive models based on the modified Jameson-effect equation and on the Lotka Volterra equation in modified atmosphere packaging. See Table 1 for list of the codes used. Black solid lines represent the Jameson-effect model and gray solid lines represent the Lotka Volterra model. Dashed and dotted lines represent the acceptable simulation zone (ASZ) used to compare observations versus predictions of the interaction models.
The modified Jameson-effect model showed the best model performance (ASZ), with a mean of 63 ± 23%, while the Lotka Volterra model showed lesser percentages [31 ± 17% (n = 18)]. Eight simulated models based on the equation of the modified Jameson-effect model can be considered as acceptable, because at least 70% of the observed log counts values are within the ASZ.
Validation Dataset
As previously described, plate counts in validation dataset increased during the shelf life with increasing the temperature (Supplementary Figures S5, S6).
At the end of the shelf life, the natural logarithm of the bacterial count was over 7.0 log CFU/g.
During the storage, a high growth rate and a more rapidly reached stationary phase are also correlated to FW and the highest storage temperatures.
No bacterial growth was observed on PCA for the control samples (limit detection < 3.00 log CFU/g) (data not shown in this paper).
The relative abundance results obtained by metagenetic analysis (expressed in%) at species levels (>1%) are represented in cumulated histograms for validation dataset in Supplementary Material for FW (Supplementary Table S5) and MAP (Supplementary Table S6). The metagenetic data were then combined with the plate counts results in order to obtain estimated bacterial counts (Supplementary Table S7).
At day 0, the distribution of read percentages shows high values (>90%) of Photobacterium spp., Photobacterium kishitanii and Photobacterium illiopiscarium.
In FW, Pseudomonas spp. reached higher values at day 3, and became the most represented bacteria until the end of the shelf-life (>90%). B. thermosphacta reached lesser values, with 3.22% at the end of the shelf-life. L. gelidum was always under the detection limit. These results are in accordance with those obtained in co-culture experiments.
In MAP, Photobacterium spp. was the most represented genus (>90%) during storage. However, low levels of B. thermosphacta and L. gelidum were observed at 8 and 12°C. Pseudomonas spp. was always under the detection limit. These results are different from those obtained in co-culture experiments.
Moreover, pH value of the validation dataset at the end of the shelf-life was statistically different to control samples (7.06 ± 0.80, n = 7, p-value = 0.01).
At the same time, the concentration of carbon dioxide also showed higher values than control samples (35.5 ± 1.64, 56.7 ± 2.17, and 96.7 ± 5.57, at 4, 8, and 12°C, respectively).
Discussion
The present study aimed to obtain the growth parameters of three specific spoilage micro-organisms previously isolated in MP samples, and to develop a three-spoilage species interaction model under different storage conditions. B. thermosphacta, Pseudomonas spp., and L. gelidum were previously isolated as predominant strains (>50% reads) from different batches of Belgian MP samples at the end of their use-by-date (Cauchie et al., 2019). Considered as the main representative spoilage species in meat and meat products (Koort et al., 2005; Liu et al., 2006; Nychas et al., 2008; Pennacchia et al., 2009, 2011; Andritsos et al., 2012; De Filippis et al., 2013; Casaburi et al., 2014; Stoops et al., 2015; Zhao et al., 2015; Mann et al., 2016; Stellato et al., 2016; Del Blanco et al., 2017; Geeraerts et al., 2017; Raimondi et al., 2018; Li et al., 2019; Mansur et al., 2019; Peruzy et al., 2019), these bacteria were inoculated on irradiated MP samples, in mono- and in co-culture experiments.
However, the selection of dominant and non-dominant species in inoculation experiments could have been more interesting in order to better represent the natural contamination of MP, and thus to better model the impact of sub-dominant microbiota. Indeed, others taxa were also present in MP samples but in lesser abundance, even if they are considered as dominant taxa in several studies: Photobacterium spp. (Ast et al., 2007; Bjornsdottir-Butler et al., 2016; Moretro et al., 2016; Nieminen et al., 2016; Kuuliala et al., 2018; Fogarty et al., 2019; Jääskeläinen et al., 2019) and Lactobacillus spp. (especially Lactobacillus algidus) (Kato et al., 2000; Fadda et al., 2010; Doulgeraki et al., 2012; Dalcanton et al., 2013; Nieminen et al., 2015; Pothakos et al., 2015; Alvarez-Sieiro et al., 2016; Woraprayote et al., 2016; Stefanovic et al., 2017). According to this, they were not included in models of this study, as all others non-dominant microbiota. Moreover, P. fluorescens and P. fragi were used together in experiments. The objective of this study was to offer an exploratory approach to the proposed method by following the common genus formed by the two species mentioned. So, it would have been interesting to inoculate MP samples with both species in different batches, as behavior of these species is different according to the storage conditions.
The inputs of models were provided from culture-dependent and culture-independent analysis performed on inoculation experiments. The association of both techniques allows us to obtain estimate abundance during storage in co-culture experiments. Although we acknowledge that the plate count method is not able to assess all the microbial populations in presence, the combination of these two methods was previously validated by a qPCR approach (Cauchie et al., 2017). This approach was also used in others studies (Chaillou et al., 2015; Delhalle et al., 2016). Fougy et al. (2016) also showed that this conversion can be used to obtain an extrapolated estimation of the bacterial concentration, and may be used in food industries. But comparison of these results with counts on selective media would also be interesting to study in the future. Moreover, even if this method overestimates the bacterial concentration, it could be beneficial in a worst-case risk assumption for food industries (Crotta et al., 2016; Membré and Boué, 2018).
In this study, models show relatively good fitting indexes (RrMSE and R2). Good performances (ASZ) in the three-species interaction approach were also obtained, especially with the modified Jameson-effect model.
The growth parameters of the three specific spoilage micro-organisms were obtained for mono- and co-culture experiments by fittings primary and secondary models (Tables 4, 5). The food packaging shows the highest impact on bacterial growth rates (μmax), which in turn have the strongest influence on the shelf life of food products (Simpson and Carevic, 2004; Stoops et al., 2015; Guillard et al., 2016; Saraiva et al., 2016; Couvert et al., 2017). In accordance with Liu et al. (2006), N0 showed a little correlation with the microbial shelf life in mono- and co-culture experiments, indicated that the storage outcome of food seems to be not completely determined by the initial microbial counts. Moreover, no obvious correlation has been shown between Nmax and shelf life in co-cultures experiments. This can be explained by the fact that meat shelf life is determined primarily by the metabolic patterns of the spoilage microbiota, rather than by total counts of bacteria (Liu et al., 2006). However, it can be observed that the parameters obtained in single culture were quite different from those in co-culture, especially for Pseudomonas spp. and B. thermosphacta. In FW, B. thermosphacta grew faster on mono-culture, but this behavior was not detected in co-culture. On the opposite, Pseudomonas spp. became the dominant bacteria in FW in the presence of the two others micro-organisms. These differences between mono- and co-culture inoculations have already been observed by Hibbing et al. (2010) and Quinto et al. (2018).
On the other hand, observations in co-culture experiments showed that the suppression of the two other bacteria occurred when the dominant one reached its MCP. This result reveals a potential Jameson effect between populations, rather than a prey-predator trend. According to these, differences between mono- and co-cultures experiments could maybe be explained by two hypotheses: (i) a non-specific interaction involving the Jameson effect, where growth inhibition is the result from a depletion in nutrient bioavailability and toxicity increase when the dominant bacteria reaches NMCP; and (ii) a specific interaction due to the modification of the food matrix where bacteria are growing (i.e., catabolism of carbon sources, the production of by products such as carbon dioxide and acids, …) (Bruce et al., 2017; Quinto et al., 2018; Correia Peres Costa et al., 2019; Kumariya et al., 2019). Nadell et al. (2016) have mentioned that P. fluorescens can produces extracellular matrix materials to give them an advantage over competitors. Quorum sensing could also be related to this inhibition by the dominant bacteria, by exchanging information to synchronize bacterial behavior in mixed-culture (Ng and Bassler, 2009; Dubey and Ben-Yehuda, 2011; Quinto et al., 2018).
The development of a three-spoilage species interaction model was then performed using two models: the modified Jameson-effect and the Lotka Volterra (Figures 6, 7). The modified Jameson-effect model showed slightly better fits than the Lotka Volterra equation, with 40–86% out of the observed counts falling into the ASZ, indicating a satisfactory model performance. It only concerns 14–48% for the prey-predator approach. These results can be explained by the fact that the dynamics of experimental and validation datasets seems to follow a Jameson behavior, because the minority bacteria decelerate when the majority one reaches the MCP (Cornu et al., 2011). Moreover, the modified Jameson-effect equation is considering growth parameters (μmax, tMCP, and N0) for modeling (Eq. 19). These parameters are obtained by primary and secondary fittings, and are relatively reliable in our study due to the numbers of samples analyzed. On the other hand, the Lotka Volterra model is based on complex interaction factors (Eq. 21) which are obtained by linear regression. Due to the high variability of interactions that can be simulated, particularly in three or more species models, these interaction factors must necessarily be as accurate as possible. In this study, interaction factors are included in highly variable intervals (Table 8), with some variations observed according to the temperature (Moller et al., 2013; Mejlholm and Dalgaard, 2015; Correia Peres Costa et al., 2019). More datasets are probably needed to obtained reliable factors. Also, the Lotka Volterra model could be modified for a more realistic approach by considering the effect of other influencing factors (e.g., environmental conditions such as several storage and packaging conditions, bacteriocin production, etc.) (Powell et al., 2004; Baka et al., 2014).
More inoculation experiments are so needed to develop better predictive models, especially for a three- or more-spoilage species interaction approach. And also, to better understand the dynamics of spoilage bacteria toward each other and in the presence of natural microbiota. As mentioned by Quinto et al. (2018): “it is well known that a spoilage microorganism can either stimulate, inhibit, or have no effect on the growth of the pathogenic species.” So, it could be interesting to study interactions between spoilage microorganisms, with production of metabolites or other substances as interaction factors. It would also be interesting to investigate co-culture experiments with two species. Moreover, metabolites production by each of the inoculated bacteria, as inputs interacting models, will be studied in another scientific publication.
Finally, naturally contaminated samples were used to validate the developed models by considering the effect of the food microbiota. Differences with co-culture experiments were obtained: a predominance of Photobacterium spp. (>90% of reads) was observed in MAP (Supplementary Figure S5). It could be interesting to take also into account this bacterium for modeling interactions. The addition of this bacterium could possibly improve the reliability of predictions, particularly for the Lotka Volterra model. Moreover, Photobacterium spp. is not well recovered on PCA at 22°C (Dalgaard et al., 1997; Hilgarth et al., 2018). According to this, improving cultivation methods for this bacterium is important to obtain more reliable results. Further studies are so needed to develop more realistic interacting predictive models, especially in a three- or more-spoilage species interaction approach, and to develop new food preservation process.
Conclusion
New omics technologies, such as metagenetics and metabolomics, are important to characterize and to follow the dynamics of bacterial microbiota and metabolites in complex food matrices. New generations of predictive models will probably need to be developed, by considering the results provided by these techniques. These models will provide a better understanding of the interactions between microorganisms and food, and micro-organisms between them.
Data Availability Statement
All biosample raw reads were deposited at the National Center for Biotechnology Information (NCBI) and are available under de BioProject ID PRJNA590608.
Author Contributions
EC did the experiments, interpreted the results, and wrote the manuscript. LD and GB performed the experiments, supervised analyses, and revisited the manuscript. BT, PF, FF, and GD were involved in the design of the study and provide help for interpretation of the results. AT and SB participated to the experiments. NK participated to the interpretation of the results and revisited of the manuscript. All authors read and approved the final manuscript.
Conflict of Interest
SB and PF was employed by Quality Partner sa.
The remaining authors declare that the research was conducted in the absence of any commercial or financial relationships that could be construed as a potential conflict of interest.
Acknowledgments
The authors thank the producers who provided the minced pork samples. This research did not receive any specific grant from funding agencies in the public, commercial, or not-for-profit sectors.
Supplementary Material
The Supplementary Material for this article can be found online at: https://www.frontiersin.org/articles/10.3389/fmicb.2020.00639/full#supplementary-material
Footnotes
References
Alvarez-Sieiro, P., Montalban-Lopez, M., Mu, D., and Kuipers, O. P. (2016). Bacteriocins of lactic acid bacteria: extending the family. Appl. Microbiol. Biotechnol. 100, 2939–2951. doi: 10.1007/s00253-016-7343-9
Andritsos, N. D., Mataragas, M., Mavrou, E., Stamatiou, A., and Drosinos, E. H. (2012). The microbiological condition of minced pork prepared at retail stores in Athens, Greece. Meat Sci. 91, 486–489. doi: 10.1016/j.meatsci.2012.02.036
Antunes-Rohling, A., Artaiz, A., Calero, S., Halaihel, N., Guillén, S., Raso, J., et al. (2019). Modeling microbial growth in modified-atmosphere-packaed hake (Merluccius merluccius) fillets stored at different temperatures. Food Res. Int. 122, 506–516. doi: 10.1016/j.foodres.2019.05.018
Ast, J. C., Cleewerck, I., Engelbeen, K., Urbanczyk, H., Thompson, F. L., De Vos, P., et al. (2007). Photobacterium kishitanii sp. nov., a luminous marine bacterium stmbiotic with deep-sea fishes. Int. J. Syst. Evol. Microbiol. 57, 2073–2078. doi: 10.1099/ijs.0.65153-0
Augustin, J.-C., and Carlier, V. (2000). Modelling the growth rate of Listeria monocytogenes with a multiplicative type model including interactions between environmental factors. Int. J. Food Microbiol. 56, 53–70. doi: 10.1016/S0168-1605(00)00224-5
Baka, M., Noriega, E., Mertens, L., Van Derlinden, E., and Van Impe, J. F. M. (2014). Protective role of indigenous Leuconostoc carnosum against Listeria monocytogenes on vacuum packed Frankfurter sausages at suboptimal temperatures. Food Res. Int. 66, 197–206. doi: 10.1016/j.foodres.2014.08.011
Baranyi, J., and Roberts, T. A. (1994). A dynamic approach to predicting bacterial growth in food. Int. J. Food Microbiol. 23, 277–294. doi: 10.1016/0168-1605(94)90157-0
Baty, F., and Delignette-Muller, M.-L. (2013). nlsMicrobio: Data Sets and Nonlinear Regression Models Dedicated to Predictive Microbiology. R Package Version 0.0-1. Available at: https://CRAN.R-project.org/package=nlsMicrobio (accessed December 5, 2018).
Benson, A. K., David, J. R. D., Gilbreth, S. E., Smith, G., Nietfeldt, H., Legge, R., et al. (2014). Microbial successions are associated with changes in chemical profiles of a model refrigerated fresh pork sausage during an 80-day shelf life study. Appl. Environ. Microbiol. 80, 5178–5194. doi: 10.1128/AEM.00774-14
Berlow, E. L., Neutel, A.-M., Cohen, J. E., De Ruiter, P. C., Ebenman, B., Emmerson, M., et al. (2004). Interaction strengths in food webs: issues and opportunities. J. Anim. Ecol. 73, 585–598. doi: 10.1111/j.0021-8790.2004.00833.x
Bjornsdottir-Butler, K., McCarthy, S. A., Dunlap, P. V., and Benner, J. A. (2016). Photobacterium angustum and Photobacterium kishitanii, psychrotrophic high-level histamine-producing bacteria indigenous to tuna. Appl. Environ. Microbiol. 82, 2167–2176. doi: 10.1128/AEM.02833-15
Bruce, J. B., West, S. A., and Griffin, A. S. (2017). Bacteriocins and the assembly of natural Pseudomonas fluorescens populations. J. Evol. Biol. 30, 352–360. doi: 10.1111/jeb.13010
Cadavez, V. A. P., Campagnollo, F. B., Silva, R. A., Duffner, C. M., Schaffner, D. W., Sant’Ana, A. S., et al. (2019). A comparison of dynamic tertiary and competition models for describing the fate of Listeria monocytogenes in Minas fresh cheese during refrigerated storage. Food Microbiol. 79, 48–60. doi: 10.1016/j.fm.2018.11.004
Casaburi, A., Piombino, P., Nychas, G.-J., Villani, F., and Ercolini, D. (2014). Bacterial populations and the volatilome associated to meat spoilage. Food Microbiol. 45, 83–102. doi: 10.1016/j.fm.2014.02.002
Cauchie, E., Delhalle, L., Taminiau, B., Tahiri, A., Korsak, N., Burteau, S., et al. (2019). Assessment of spoilage bacterial communities in food wrap and modified atmospheres-packed minced pork meat samples by 16S rDNA metagenetic analysis. Front. Microbiol. 10:3074. doi: 10.3389/fmicb.2019.03074
Cauchie, E., Gand, M., Kergourlay, G., Taminiau, B., Delhalle, L., Korsak, N., et al. (2017). The use of 16 rRNA gene metagenetic monitoring of refrigerated food products for understanding the kinetic of microbial subpopulations at different storage temperatures: the example of white pudding. Int. J. Food Microbiol. 247, 70–78. doi: 10.1016/j.ijfoodmicro.2016.10.012
Chaillou, S., Chaulot-Talmon, A., Caekebeke, H., Cardinal, M., Christieans, S., Denis, C., et al. (2015). Origin and ecological selection of core and food-specific bacterial communities associated with meat and seafood spoilage. Int. Soc. Microb. Ecol. J. 9, 1105–1118. doi: 10.1038/ismej.2014.202
Chaix, E., Broyart, B., Couvert, O., Guillaume, C., Gontard, N., and Guillard, V. (2015). Mechanistic model coupling gas exchange dynamics and Listeria monocytogenes growth in modified atmosphere packaging of non respiring food. Food Microbiol. 51, 192–205. doi: 10.1016/j.fm.2015.05.017
Chauvet, E., Paulet, J. E., Previte, J. P., and Walls, Z. (2002). A lotka-volterra three-species food chain. Math. Mag. 75, 243–255. doi: 10.2307/3219158
Cornu, M., Billoir, E., Bergis, H., Beaufort, A., and Zuliani, V. (2011). Modeling microbial competition in food: application to the behavior of Listeria monocytogenes and lactic acid flora in pork meat products. Food Microbiol. 28, 639–647. doi: 10.1016/j.fm.2010.08.007
Correia Peres Costa, J. C., Bover-Cid, S., Bolivar, A., Zurera, G., and Pérez-Rodriguez, F. (2019). Modelling the interaction of the sakacin-producing Lactobacillus sakei CTC494 and Listeria monocytogenes in filleted gilthead sea bream (Sparus aurata) under modified atmosphere packaging at isothermal and non-isothermal conditions. Int. J. Food Microbiol. 297, 72–84. doi: 10.1016/j.ijfoodmicro.2019.03.002
Couvert, O., Divanac’h, M.-L., Lochardet, A., Thuault, D., and Huchet, V. (2019). Modelling the effect of oxygen concentration on bacterial growth rates. Food Microbiol. 77, 21–25. doi: 10.1016/j.fm.2018.08.005
Couvert, O., Guégan, S., Hézard, B., Huchet, V., Lintz, A., Thuault, D., et al. (2017). Modeling carbon dioxide effect in a controlled atmosphere and its interactions with temperature and pH on the growth of L. monocytogenes and P. fluorescens. Food Microbiol. 68, 89–96. doi: 10.1016/j.fm.2017.07.003
Crotta, M., Paterlini, F., Rizzi, R., and Guitian, J. (2016). Consumers’ behavior in quantitative microbial risk assessment for pathogens in raw milk: incorporation of the likelihood of consumption as a function of storage time and temperature. J. Dairy Sci. 99, 1029–1038. doi: 10.3168/jds.2015-10175
Dalcanton, F., Carrasco, E., Pérez-Rodriguez, F., Posada-Izquierdo, G. D., de Aragao, G. M. F., and Garcia-Gimeno, R. M. (2018). Modeling the combined effects of temperature, pH, and sodium chloride and sodium lactate concentrations on the growth rate of Lactobacillus plantarum ATCC 8014. J. Food Qual. 5, 1–10. doi: 10.1155/2018/1726761
Dalcanton, F., Pérez-Rodriguez, F., Posada-Izquierdo, G. D., de Aragao, G. M. F., and Garcia-Gimeno, R. M. (2013). Modelling growth of Lactobacillus plantarum and shelf life of vacuum-packaged cooked chopped pork at different temperatures. Int. J. Food Sci. Technol. 48, 2580–2587. doi: 10.1111/ijfs.12252
Dalgaard, P., Mejholm, O., Christiansen, T. J., and Huss, H. H. (1997). Importance of Photobacterium phosphoreum in relation to spoilage of modified atmosphere-packed fish products. Lett. Appl. Microbiol. 24, 373–378. doi: 10.1046/j.1472-765X.1997.00152.x
De Filippis, F., Pennacchia, C., Di Pasqua, R., Fiore, A., Fogliano, V., Villani, F., et al. (2013). Decarboxylase gene expression and cadaverine and putrescine production by Serratia proteamaculans in vitro and in beef. Int. J. Food Microbiol. 165, 332–338. doi: 10.1016/j.ijfoodmicro.2013.05.021
Del Blanco, A., Caro, I., Quinto, E. J., and Mateo, J. (2017). Quality changes in refrigerated stored minced pork wrapped with plastic cling film and the effect of glucose supplementation. Meat Sci. 126, 55–62. doi: 10.1016/j.meatsci.2016.12.007
Delhalle, L., Korsak, N., Taminiau, B., Nezer, C., Burteau, S., Delcenserie, V., et al. (2016). Exploring the bacterial diversity of Belgian steak tartare using metagenetics and quantitative real-time PCR analysis. J. Food Prot. 79, 220–229. doi: 10.4315/0362-028X.JFP-15-185
Den Besten, H. M. W., Aryani, D. C., Metselaar, K. I., and Zwietering, M. H. (2017). Microbial variability in growth and heat resistance of a pathogen and a spoiler: all variabilities are equal but some are more equal than others. Int. J. Food Microbiol. 240, 24–31. doi: 10.1016/j.ijfoodmicro.2016.04.025
Dens, E. J., Vereecken, K. M., and Van Impe, J. F. (1999). A prototype model structure for mixed microbial populations in homogeneous food products. J. Theor. Biol. 201, 159–170. doi: 10.1006/jtbi.1999.1021
Devlieghere, F., Van Belle, B., and Debevere, J. (1999). Shelf life of modified atmosphere packed cooked meat products: a predictive model. Int. J. Food Microbiol. 46, 57–70. doi: 10.1016/S0168-1605(98)00175-5
Dominguez, S. A., and Schaffner, D. W. (2007). Development and validation of a mathematical model to describe the growth of Pseudomonas spp. in raw poultry stored under aerobic conditions. Int. J. Food Microbiol. 120, 287–295. doi: 10.1016/j.ijfoodmicro.2007.09.005
Doulgeraki, A. I., Ercolini, D., Villani, F., and Nychas, G.-J. E. (2012). Spoilage microbiota associated to the storage of raw meat in different conditions. Int. J. Food Microbiol. 157, 130–141. doi: 10.1016/j.ijfoodmicro.2012.05.020
Dubey, G. P., and Ben-Yehuda, S. (2011). Intercellular nanotubes mediate bacterial communication. Cell 144, 590–600. doi: 10.1016/j.cell.2011.01.015
Fadda, S., Lopez, C., and Vignolo, G. (2010). Role of lactic acid bacteria during meat conditioning and fermentation: peptides generated as sensorial and hygienic biomarkers. Meat Sci. 86, 66–79. doi: 10.1016/j.meatsci.2010.04.023
Fakruddin, M., Mazumder, R. M., and Mannan, K. S. B. (2012). Predictive microbiology: modeling microbial responses in food. Ceylon J. Sci. Biol. Sci. 40, 121–131. doi: 10.4038/cjsbs.v4i2.3928
Fogarty, C., Whyte, P., Brunton, N., Lyng, J., Smyth, C., Fagan, J., et al. (2019). Spoilage indicator bacteria in farmed Atlantic salmon (Salmo salar) stored on ice for 10 days. Food Microbiol. 77, 38–42. doi: 10.1016/j.fm.2018.08.001
Fougy, L., Desmonts, M.-H., Coeuret, G., Fassel, C., Hamon, E., Hézard, B., et al. (2016). Reducing salt in raw pork sausages increases spoilage and correlates with reduced bacterial diversity. Appl. Environ. Microbiol. 82, 3928–3940. doi: 10.1128/AEM.00323-16
Geeraerts, W., Pothakos, V., De Vuyst, L., and Leroy, F. (2017). Diversity of the dominant bacterial species on sliced cooked pork products at expiration date in the Belgian retail. Food Microbiol. 65, 236–243. doi: 10.1016/j.fm.2017.03.007
Giuffrida, A., Valenti, D., Ziino, G., Spagnolo, B., and Panebianco, A. (2009). A stochastic interspecific competition model to predict the behaviour of Listeria monocytogenes in the fermentation process of a traditional Sicilian salami. Eur. Food Res. Technol. 228, 767–775. doi: 10.1007/s00217-008-0988-6
Giuffrida, A., Ziino, G., Valenti, D., Donato, G., and Panebianco, A. (2007). Application of an interspecific competition model to predict the growth of Aeromonas hydrophila on fish surfaces during refrigerated storage. Arch. Lebensmittelhyg. 58, 136–141. doi: 10.2377/0003-925X-58-136
Gospavic, R., Kreyenschmidt, J., Bruckner, S., Popov, V., and Haque, N. (2008). Mathematical modelling for predicting the growth of Pseudomonas spp. in poultry under variable temperature conditions. Int. J. Food Microbiol. 127, 290–297. doi: 10.1016/j.ijfoodmicro.2008.07.022
Guillard, V., Couvert, O., Stahl, V., Hanin, A., Denis, C., Huchet, V., et al. (2016). Validation of a predictive model coupling gas transfer and microbial growth in fresh food packed under modified atmosphere. Food Microbiol. 58, 43–55. doi: 10.1016/j.fm.2016.03.011
Ham, Y.-K., Kim, H.-W., Hwang, K.-E., Song, D.-H., Kim, Y.-J., Choi, Y. S., et al. (2017). Effects of irradiation source and dose level on quality characteristics of processed meat products. Radiat. Phys. Chem. 130, 259–264. doi: 10.1016/j.radphyschem.2016.09.010
Hibbing, M. E., Fuqua, C., Parsek, M. R., and Peterson, S. B. (2010). Bacterial competition: surviving and thriving in the microbial jungle. Nat. Rev. Microbiol. 8, 15–25. doi: 10.1038/nrmicro2259
Hilgarth, M., Fuertes, S., Ehrmann, M., and Vogel, R. F. (2018). Photobacterium carnosum sp. nov., isolated from spoiled modified atmosphere packaged poultry meat. Syst. Appl. Microbiol. 41, 44–50. doi: 10.1016/j.syapm.2017.11.002
Jääskeläinen, E., Jakobsen, L. M. A., Hultman, J., Eggers, N., Bertram, H. C., and Björkroth, J. (2019). Metabolomics and bacterial diversity of packaged yellowfin tuna (Thunnus albacares) and salmon (Salmo salar) show fish species-specific spoilage development during chilled storage. Int. J. Food Microbiol. 293, 44–52. doi: 10.1016/j.ijfoodmicro.2018.12.021
Jameson, J. E. (1962). A discussion of the dynamics of Salmonella enrichment. J. Hyg. 60, 193–207. doi: 10.1017/s0022172400039462
Kakagianni, M., Kalantzi, K., Beetsiotis, E., Ghikas, D., Lianou, A., and Koutsoumanis, K. P. (2018). Development and validation of predictive models for the effect of storage temperature and pH on the growth boundaries and kinetics of Alicyclobacillus acidoterrestris ATTC 49025 in fruit drinks. Food Microbiol. 74, 40–49. doi: 10.1016/j.fm.2018.02.019
Kapetanakou, A. E., Taoukis, P., and Skandamis, P. N. (2019). Model development for microbial spoilage of package fresh-cut salad products using temperature and in-package CO2 levels as predictor variables. LWT – Food Sci. Technol. 113:108285. doi: 10.1016/j.lwt.2019.108285
Kato, Y., Sakala, R. M., Hayashidani, H., Kiuchi, A., Kaneuchi, C., and Ogawa, M. (2000). Lactobacillus algidus sp. nov., a psychrophilic lactic acid bacterium isolated from vacuum-packaged refrigerated beef. Int. J. Syst. Envol. Microbiol. 50, 1143–1149. doi: 10.1099/00207713-50-3-1143
Kim, B.-J., Lee, H.-J., Park, S.-Y., Kim, J., and Han, H.-U. (2000). Identification and characterization of Leuconostoc gelidum, isolated from Kimchi, a fermented cabbage product. J. Microbiol. 38, 132–136.
Kim, Y. H., Nam, K. C., and Ahn, D. U. (2002). Volatile profiles, lipid oxidation and sensory characteristics of irradiated meat from different animal species. Meat Sci. 61, 257–265. doi: 10/1016/S0309-1740(01)00191-7
Koort, J., Murros, A., Coenye, T., Eerola, S., Vandamme, P., Sukura, A., et al. (2005). Lactobacillus oligofermentans sp. nov., associated with spoilage of modified-atmosphere-packaged poultry products. Appl. Environ. Microbiol. 71, 4400–4406. doi: 10.1128/AEM.71.8.4400-4406.2005
Koutsoumanis, K. (2009). Modeling food spoilage in microbial risk assessment. J. Food Prot. 72, 425–427. doi: 10.4315/0362-028X-72.2.425
Kreyenschmidt, J., Hubner, A., Beierle, E., Chonsch, L., Sherer, A., and Petersen, B. (2010). Determination of the shelf life of sliced cooked ham based on the growth of lactic acid bacteria in different steps of the chain. J. Appl. Microbiol. 108, 510–520. doi: 10.1111/j.1365-2672.2009.04451.x
Kumariya, R., Garsa, A. K., Rajput, Y. S., Sood, S. K., and Akhtar, N. (2019). Bacteriocins: classification, synthesis, mechanism of action and resistance development in food spoilage causing bacteria. Microb. Pathog. 128, 171–177. doi: 10.1016/j.micpath.2019.01.002
Kuuliala, L., Al Hage, Y., Ioannidis, A.-G., Sader, M., Kerckgof, F.-M., Vanderroost, M., et al. (2018). Microbiological, chemical and sensory spoilage analysis of raw Atlantic cod (Gadus morhua) stored under modified atmospheres. Food Microbiol. 70, 232–244. doi: 10.1016/j.fm.2017.10.011
Le, S., Josse, J., and Husson, F. (2008). FactoMineR: an r package for multivariate analysis. J. Stat. Softw. 25, 1–18. doi: 10.18637/jss.v025.i01
Le Marc, Y., Huchet, V., Bourgeois, C. M., Guyonnet, J. P., Mafart, P., and Thuault, D. (2002). Modelling the growth kinetics of Listeria as a function of temperature, pH and organic acid concentration. Int. J. Food Microbiol. 73, 219–237. doi: 10.1016/S0168-1605(01)00640-7
Lebert, I., Robles-Olvera, V., and Lebert, A. (2000). Application of polynomial models to predict growth of miwed cultures of Pseudomonas spp. and Listeria in meat. Int. J. Food Microbiol. 61, 27–39. doi: 10.1016/S0168-1605(00)00359-7
Leroi, F., Fall, P. A., Pilet, M.-F., Chevalier, F., and Baron, R. (2012). Influence of temperature, pH and NaCl concentration on the maximal growth rate of Brochothrix thermosphacta and a bioprotective bacteria Lactococcus piscium CNCM I-4031. Food Microbiol. 31, 222–228. doi: 10.1016/j.fm.2012.02.014
Li, L., Cepeda, J., Subbiah, J., Froning, G., Juneja, V. K., and Thippareddi, H. (2017). Dynamic predictive model for growth of Salmonella spp. in scrambled egg mix. Food Microbiol. 64, 39–46. doi: 10.1016/j.fm.2016.12.007
Li, N., Zhang, Y., Wu, Q., Gu, Q., Chen, M., Zhang, Y., et al. (2019). High-throughput sequencing analysis of bacterial community composition and quality characteristics in refrigerated pork during storage. Food Microbiol. 83, 86–94. doi: 10.1016/j.fm.2019.04.013
Liu, F., Yang, R.-Q., and Li, Y.-F. (2006). Correlations between growth parameters of spoilage micro-organisms and shelf-life of pork stored under air and modified atmosphere at -2, 4 and 10°C. Food Microbiol. 23, 578–583. doi: 10.1016/j.fm.2005.10.002
Longhi, D. A., Dalcanton, F., Falcao de Arageo, G. M., Carciofi, B. A. M., and Laurindo, J. B. (2013). Assessing the prediction ability of different mathematical models for the growth of Lactobacillus plantarum under non-isothermal conditions. J. Theor. Biol. 335, 88–96. doi: 10.1016/j.jtbi.2013.06.030
Mann, E., Wetzels, S. U., Pinior, B., Metzler-Zebeli, B. U., Wagner, M., and Schmitz-Esser, S. (2016). Psychrophile spoilers dominate the bacterial microbiome in musculature samples of slaughter pigs. Meat Sci. 117, 36–40. doi: 10.1016/j.meatsci.2016.02.034
Mansur, A. R., Song, E.-J., Cho, Y.-S., Nam, Y.-D., Choi, Y.-S., Kim, D.-O., et al. (2019). Comparative evaluation of spoilage-related bacterial diversity and metabolite profiles in chilled beef stored under air and vacuum packaging. Food Microbiol. 77, 166–172. doi: 10.1016/j.fm.2018.09.006
Mataragas, M., Drosinos, E. H., Vaidanis, A., and Metaxopoulos, I. (2006). Development of a predictive model for spoilge of cooked cured meat products and its validation under constant and dynamic temperature storage conditions. J. Food Sci. 71, 157–167. doi: 10.1111/j.1750-3841.2006.00058.x
Mejlholm, O., and Dalgaard, P. (2007). Modeling and predicting the growth of lactic acid bacteria in lightly preserved seafood and their inhibiting effect on Listeria monocytogenes. J. Food Prot. 70, 2485–2497. doi: 10.4315/0362-028x-70.11.2485
Mejlholm, O., and Dalgaard, P. (2013). Development and validation of an extensive growth and growth boundary model for psychrotolerant Lactobacillus spp. in seafood and meat products. Int. J. Food Microbiol. 167, 244–260. doi: 10.1016/j.ijfoodmicro.2013.09.013
Mejlholm, O., and Dalgaard, P. (2015). Modelling and predicting the simultaneous growth of Listeria monocytogenes and psychrotolerant lactic acid bacteria in processed seafood and mayonnaise-based seafood salads. Food Microbiol. 46, 1–4. doi: 10.1016/j.fm.2014.07.005
Membré, J.-M., and Boué, G. (2018). Quantitative microbiological risk assessment in food industry: theory and practical application. Food Res. Int. 106, 1132–1139. doi: 10.1016/j.foodres.2017.11.025
Miks-Krajnik, M., Yoon, Y.-J., Ukuku, D. O., and Yuk, H.-G. (2016). Identification and quantification of volatile chemical spoilage indexes associated with bacterial growth dynamics in aerobically stored chicken. J. Food Sci. 81, 2006–2013. doi: 10.1111/1750-3841.13371
Moller, C. O. A., Ilg, Y., Aabo, S., Christensen, B. B., Dalgaard, P., and Hansen, T. B. (2013). Effect of natural microbiota on growth of Salmonella spp. in fresh pork – a predictive microbiology approach. Food Microbiol. 34, 284–295. doi: 10.1016/j.fm.2012.10.010
Moretro, T., Moen, B., Heir, E., Hansen, A. A., and Langsrud, S. (2016). Contamination of salmon fillets and processing plants with spoilage bacteria. Int. J. Food Microbiol. 237, 98–108. doi: 10.1016/j.ijfoodmicro.2016.08.016
Mounier, J., Monnet, C., Vallaeys, T., Arditi, R., Sarthou, A.-S., Hélias, A., et al. (2008). Microbial interaction within a cheese microbial community. Appl. Environ. Microbiol. 74, 172–181. doi: 10.1128/AEM.01338-07
Nadell, C. D., Drescher, K., and Foster, K. R. (2016). Spatial structure, cooperation and competition in biofilms. Nature 14, 589–600. doi: 10.1038/nrmicro2016.84
Ng, W.-L., and Bassler, B. L. (2009). Bacterial quorum-sensing network architectures. Annu. Rev. Genet. 43, 197–222. doi: 10.1146/annurev-gnet-102108-13404
Nieminen, T. T., Dalgaard, P., and Björkroth, J. (2016). Volatile organic compounds and Photobacterium phosphoreum associated with spoilage of modified-atmosphere-packaged raw pork. Int. J. Food Microbiol. 218, 86–95. doi: 10.1016/j.ijfoodmicro.2015.11.003
Nieminen, T. T., Nummela, M., and Björkroth, J. (2015). Packaging gas selects lactic acid bacterial communities on raw pork. J. Appl. Microbiol. 119, 1310–1316. doi: 10.1111/jam.12890
Nychas, G. J. E., Skandamis, P. N., Tassou, C. C., and Koutsoumanis, K. P. (2008). Meat spoilage during distribution. Meat Sci. 78, 77–89. doi: 10.1016/j.meatsci.2007.06.020
Nyhan, L., Begley, M., Mutel, A., Qu, Y., Johnson, N., and Callanan, M. (2018). Predicting the combinatorial effects of water activity, pH and organic acids on Listeria growth in media and complex food matrices. Food Microbiol. 74, 75–85. doi: 10.1016/j.fm.2018.03.002
Pedrozo, H. A., Dallagnol, A. M., Vignolo, G. M., Pucciarelli, A. B., and Schvezov, C. E. (2019). Mechanistically inspired kinetic approach to describe interactions during co-culture growth of Carnobacterium maltaromaticum and Listeria monocytogenes. J. Food Sci. 84, 2592–2602. doi: 10.1111/1750-3841.14754
Pennacchia, C., Ercolini, D., and Villani, F. (2009). Development of a real-time PCA assay for the specific detection of Brochothrix thermosphacta in fresh and spoiled raw meat. Int. J. Food Microbiol. 134, 230–236. doi: 10.1016/j.ijfoodmicro.2009.07.005
Pennacchia, C., Ercolini, D., and Villani, F. (2011). Spoilage-related microbiota associated with chilled beef stored in air or vacuum pack. Food Microbiol. 28, 84–93. doi: 10.1016/j.fm.2010.08.010
Peruzy, M. F., Murru, N., Yu, Z., Cnockaert, M., Joossens, M., Proroga, Y. T. R., et al. (2019). Determination of the microbiological contamination in minced pork by culture dependent and 16S amplicon sequencing analysis. Int. J. Food Microbiol. 290, 27–35. doi: 10.1016/j.ijfoodmicro.2018.09.025
Pinon, A., Zwietering, M., Perrier, L., Membré, J.-M., Leporq, B., Mettler, E., et al. (2004). Development and validation of experimental protocols for use of cardinal models for prediction of microorganism growth in food products. Appl. Environ Microbiol. 70, 1081–1087. doi: 10.1128/AEM.70.2.1081-1087.2004
Pinter, M. D., Harter, T., McCarthy, M. J., and Augustine, M. P. (2014). Towards usind NMR to screen for spoiled tomatoes stored in 1,000L, septically sealed, metal-lined totes. Sensors 14, 4167–4176. doi: 10.3390/s14034167
Pothakos, V., Devlieghere, F., Villani, F., Björkroth, J., and Ercolini, D. (2015). Lactic acid bacteria and their controversial role in fresh meat spoilage. Meat Sci. 109, 66–74. doi: 10.1016/j.meatsci.2015.04.014
Powell, M., Schosser, W., and Ebel, E. (2004). Considering the complexity of microbial community dynamics in food safety risk assessment. Int. J. Food Microbiol. 90, 171–179. doi: 10.1016/S0168-1605(03)00106-5
Psomas, A. N., Nychas, G.-J., Haroutounian, S. A., and Skandamis, P. N. (2011). Development and validation of a tertiary simulation model for predicting the growth of the food microorganisms under dynamic and static temperature conditions. Comput. Electron. Agric. 76, 119–129. doi: 10.1016/j.compag.2011.01.013
Quinto, E. J., Marin, J. M., Caro, I., Mateo, J., and Schaffner, D. W. (2018). Bayesian modeling of two- and three-species bacterial competition in milk. Food Res. Int. 105, 952–961. doi: 10.1016/j.foodres.2017.12.033
R Core Team (2019). R: A Language and Environment for Statistical Computing. Vienna: R Foundation for Statistical Computing.
Raimondi, S., Nappi, M. R., Sirangelo, T. M., Leonardi, A., Amaretti, A., Ulrici, A., et al. (2018). Bacterial community of industrial raw sausage packaged in modified atmosphere throughout the shelf life. Int. J. Food Microbiol. 280, 78–86. doi: 10.1016/j.ijfoodmicro.2018.04.041
Rashid, N., Shimada, Y., Ezaki, S., Atomi, H., and Imanaka, T. (2001). Low-temperature lipase from psychrotrophic Pseudomonas sp. strain KB700A. Appl. Environ. Microbiol. 67, 4064–4069. doi: 10.1128/AEM.67.9.4064-4069.2001
Ratkowsky, D. A., Lowry, R. K., McMeekin, T. A., Stokes, A. N., and Chandler, R. E. (1983). Model for bacterial culture growth rate throughout the entire biokinetic temperature range. J. Bacteriol. 154, 1222–1226. doi: 10.1128/jb.154.3.1222-1226.1983
Ratkowsky, D. A., Olley, J., McMeekin, T. A., and Ball, A. (1982). Relationship between temperature and growth rate of bacterial cultures. J. Bacteriol. 149, 1–5. doi: 10.1128/jb.149.1.1-5.1982
Rosso, L., Lobry, J. R., Bajard, S., and Flandrois, J. P. (1995). Convenient model to describe the combined effects of temperature and pH on microbial growth. Appl. Environ. Microbiol. 61, 610–616. doi: 10.1128/aem.61.2.610-616.1995
Rouger, A., Moriceau, N., Prévost, H., Remenant, B., and Zagorec, M. (2018). Diversity of bacterial communities in French chicken cuts stored under modified atmosphere packaging. Food Microbiol. 70, 7–16. doi: 10.1016/j.fm.2017.08.013
Rouger, A., Remenant, B., Prévost, H., and Zagorec, M. (2017). A method to isolate bacterial communities and characterize ecosystems from food products: validation and utilization in as a reproductible chicken meat model. Int. J. Food Microbiol. 247, 38–47. doi: 10.1016/j.ijfoodmicro.2016.04.028
Saraiva, C., Fontes, M. C., Patarata, L., Martins, C., Cadavez, V., and Gonzalas-Barron, U. (2016). Modelling the kinetics of Listeria monocytogenes in refrigerated fresh beef under different packaging atmospheres. Food Sci. Technol. 66, 664–671. doi: 10.1016/j.lwt.2015.11.026
Simpson, R., and Carevic, E. (2004). Designing a modified atmosphere packaging system for foodservice portions on nonrespiring foods: optimal gas mixture and food/headspace ratio. Foodserv. Res. Int. 14, 257–272. doi: 10.1111/j.1745-4506.2004.tb00194.x
Stefanovic, E., Fitzgerald, G., and McAulliffe, O. (2017). Advances in the genomics and metabolomics of dairy lactobacilli: a review. Food Microbiol. 61, 33–49. doi: 10.1016/j.fm.2016.08.009
Stellato, G., La Storia, A., De Filippis, F., Borriello, G., Villani, F., and Ercoloni, D. (2016). Overlap of spoilage-associated microbiota between meat and the meat processing environment in small-scale and large-scale retail distributions. Appl. Environ. Microbiol. 82, 4045–4054. doi: 10.1128/AEM.00793-16
Stoddard, S. F., Smith, B. J., Hein, R., Roller, B. R. K., and Schmidt, T. M. (2015). rrnDB: improved tools for interpreting rRNA gene abundance in bacteria and archaea and a new foundation for future development. Nucleic. Acids Res. 43, 593–598. doi: 10.1093/nar/gky1201
Stoops, J., Ruyters, S., Busschaert, P., Spaepen, R., Verreth, C., Claes, J., et al. (2015). Bacterial community dynamics during colt storage of minced meat packaged under modified atmosphere and supplemented with different preservatives. Food Microbiol. 48, 192–199. doi: 10.1016/j.fm.2014.12.012
Tamplin, M. L. (2018). Integrating predictive models and sensors to manage food stability in supply chains. Food Microbiol. 75, 90–94. doi: 10.1016/j.fm.2017.12.001
Torngren, M. A., Darré, M., Gunvig, A., and Bardenshtein, A. (2018). Case studies of packaging and processing solutions to improve meat quality and safety. Meat Sci. 144, 149–158. doi: 10.1016/j.meatsci.2018.06.018
Vereecken, K. M., Dens, E. J., and Van Impe, J. F. (2000). Predictive modeling of mixed microbial populations in food products: evaluation of two-species models. J. Theor. Biol. 205, 53–72. doi: 10.1006/jtbi.2000.2046
Wang, X. B., Wang, C. N., Zhang, Y. C., Liu, T. T., Lv, J. P., Shen, X., et al. (2018). Effects of gamma radiation on microbial, psychichemical, and structural properties of whey protein model system. J. Dairy Sci. 101, 4879–4890. doi: 10.3168/jds.2017-14085
Woraprayote, W., Malila, Y., Sorapukdee, S., Swetwiwathana, A., Benjakul, S., and Visessanguan, W. (2016). Bacteriocins from lactic acid bacteria and their applications in meat and meat products. Meat Sci. 120, 118–132. doi: 10.1016/j.meatsci.2016.04.004
Ye, K., Wang, H., Jiang, Y., Xinglian, X., Cao, J., and Zhou, G. (2014). Development of interspecific competition models for the growth of Listeria monocytogenes and Lactobacillus on vacuum-packaged chilled pork by quantitative real-time PCR. Food Res. Int. 64, 626–633. doi: 10.1016/j.foodres.2014.07.017
Yoon, S.-H., Ha, S.-M., Kwon, S., Lim, J., Kim, Y., Seo, H., et al. (2017). Introducing EzBioCloud: a taxonomically unite database of 16 rRNA gene sequences and whole-genome assemblies. Int. J. Syst. Evol. Microbiol. 67, 1613–1617. doi: 10.1099/ijsem.0.001755
Zhao, F., Zhou, G., Ye, K., Wang, S., Xu, X., and Li, C. (2015). Microbial changes in vacuum-packed chilled pork during storage. Meat Sci. 100, 145–149. doi: 10.1016/j.meatsci.2014.10.004
Keywords: predictive microbiology, growth parameters, interaction models, Brochothrix thermosphacta, Pseudomonas spp., Leuconostoc gelidum, Jameson-effect model, Lotka Volterra model
Citation: Cauchie E, Delhalle L, Baré G, Tahiri A, Taminiau B, Korsak N, Burteau S, Fall PA, Farnir F and Daube G (2020) Modeling the Growth and Interaction Between Brochothrix thermosphacta, Pseudomonas spp., and Leuconostoc gelidum in Minced Pork Samples. Front. Microbiol. 11:639. doi: 10.3389/fmicb.2020.00639
Received: 18 November 2019; Accepted: 20 March 2020;
Published: 09 April 2020.
Edited by:
Eugenia Bezirtzoglou, Democritus University of Thrace, GreeceReviewed by:
Mark Tamplin, University of Tasmania, AustraliaMarija Boskovic, University of Belgrade, Serbia
Qian Yun-Fang, Shanghai Ocean University, China
Per Johansson, University of Helsinki, Finland
Copyright © 2020 Cauchie, Delhalle, Baré, Tahiri, Taminiau, Korsak, Burteau, Fall, Farnir and Daube. This is an open-access article distributed under the terms of the Creative Commons Attribution License (CC BY). The use, distribution or reproduction in other forums is permitted, provided the original author(s) and the copyright owner(s) are credited and that the original publication in this journal is cited, in accordance with accepted academic practice. No use, distribution or reproduction is permitted which does not comply with these terms.
*Correspondence: Emilie Cauchie, ZWNhdWNoaWVAdWxpZWdlLmJl