- 1Department of Epidemiology, Geisel School of Medicine at Dartmouth, Hanover, NH, United States
- 2Institute for Molecular Medicine Finland, University of Helsinki, Helsinki, Finland
- 3Division of Neonatology, Department of Pediatrics, Children’s Hospital at Dartmouth, Lebanon, NH, United States
- 4Department of Community and Family Medicine, Geisel School of Medicine at Dartmouth, Hanover, NH, United States
- 5Josephine Bay Paul Center, Marine Biological Laboratory, Woods Hole, MA, United States
- 6Department of Biomedical Data Science, Geisel School of Medicine at Dartmouth, Hanover, NH, United States
- 7Department of Molecular and Systems Biology, Geisel School of Medicine at Dartmouth, Hanover, NH, United States
The process of breastfeeding exposes infants to bioactive substances including a diversity of bacteria from breast milk as well as maternal skin. Knowledge of the character of and variation in these microbial communities, as well as the factors that influence them, is limited. We aimed to identify profiles of breastfeeding-associated microbial communities and their association with maternal and infant factors. Bilateral milk samples were collected from women in the New Hampshire Birth Cohort Study at approximately 6 weeks postpartum without sterilization of the skin in order to capture the infant-relevant exposure. We sequenced the V4–V5 hypervariable region of the bacterial 16S rRNA gene in 155 human milk samples. We used unsupervised clustering (partitioning around medoids) to identify microbial profiles in milk samples, and multinomial logistic regression to test their relation with maternal and infant variables. Associations between alpha diversity and maternal and infant factors were tested with linear models. Four breastfeeding microbiome types (BMTs) were identified, which differed in alpha diversity and in Streptococcus, Staphylococcus, Acinetobacter, and Pseudomonas abundances. Higher maternal pre-pregnancy BMI was associated with increased odds of belonging to BMT1 [OR (95% CI) = 1.13 (1.02, 1.24)] or BMT3 [OR (95% CI) = 1.12 (1.01, 1.25)] compared to BMT2. Independently, increased gestational weight gain was related to reduced odds of membership in BMT1 [OR (95% CI) = 0.66 (0.44, 1.00) per 10 pounds]. Alpha diversity was positively associated with gestational weight gain and negatively associated with postpartum sample collection week. There were no statistically significant associations of breastfeeding microbiota with delivery mode. Our results indicate that the breastfeeding microbiome partitions into four profiles and that its composition and diversity is associated with measures of maternal weight.
Introduction
Through breastfeeding, infants are exposed to a diversity of bacteria from both maternal skin and milk (Pannaraj et al., 2017). Human milk is comprised of numerous bioactive substances including immune (Goldman et al., 1982; Hawkes et al., 1999; Admyre et al., 2007) and nutritive factors (López-López et al., 2002; Locascio et al., 2007) in addition to microbial communities. A distinct set of bacterial communities inhabits the breast skin, and both breast milk and skin-associated microbes are transferred to infants through the process of breastfeeding (Solis et al., 2010; Murphy et al., 2017; Pannaraj et al., 2017). The process of bacterial colonization of the gut in infancy is a key modulator of immune development (Grönlund et al., 2000; Mazmanian et al., 2005; Chichlowski et al., 2012) that may alter risk of early- and later-life diseases (Kalliomäki et al., 2008; Giongo et al., 2011; Arrieta et al., 2015). Breastfed infants have a reduced risk of diseases including otitis media, lower respiratory infections, gastroenteritis, atopy, cancers, and autoimmune disease, among others (Ip et al., 2007). The microbiota present in breast milk affect the symptomaticity of rotavirus infection in infants; infants with a symptomatic rotavirus infection consumed breast milk with lower levels of Streptococcus and Staphylococcus and higher levels of Enterobacter and Klebsiella compared to infants either negative for rotavirus or with an asymptomatic infection (Ramani et al., 2018). A more comprehensive understanding of factors influencing the microbiota present in breast milk and their contribution to the beneficial effects of breastfeeding has broad potential to influence public health.
Species of Bifidobacterium, Streptococcus, Staphylococcus, Lactobacillus, Enterococcus, and Clostridia have been observed in human milk, including butyrate-producing microbes (Martín et al., 2009; Cabrera-Rubio et al., 2012; Jost et al., 2013; Khodayar-Pardo et al., 2014). Butyrate is a short chain fatty acid of microbial metabolic origin that is known to be important for the integrity of the gut epithelial barrier and is protective against many intestinal and systemic diseases (Canani et al., 2011). Preliminary small studies using 16S rRNA gene sequencing and metagenomics approaches to assess breast milk microbial composition have reported that Streptococcus, Staphylococcus, Pseudomonas, Bacteroides, Acinetobacter, and others comprise the core breast milk microbiome, whereas Bifidobacterium and Lactobacillus abundances are comparatively low (Hunt et al., 2011; Jost et al., 2013; Ward et al., 2013; Jiménez et al., 2015; Sakwinska et al., 2016; Urbaniak et al., 2016; Murphy et al., 2017). Clusters of breast milk microbiota have been reported, often defined by the abundance of Staphylococcus, Streptococcus, and Pseudomonas, Enterobacteriaceae, and other rarer bacteria (Li et al., 2017; Biagi et al., 2018; Moossavi et al., 2019b).
The microbial composition of breast milk appears to be unique to each individual (Martín et al., 2007) and changes in early lactation, with bacterial counts rising with lactation stage (Khodayar-Pardo et al., 2014) and diversity increasing in the first 3 months postpartum (Simpson et al., 2018; Browne et al., 2019); however, bacterial diversity may be higher in colostrum compared to transition or mature milk (Cabrera-Rubio et al., 2012). Later in lactation, represented microbes appear stable within an individual in a single breast over a 4-week period of lactation (Hunt et al., 2011).
Previously, the breast milk microbiome composition has most commonly been associated with maternal and infant factors including maternal weight variables, delivery mode, and gestational age at delivery (Cabrera-Rubio et al., 2012; Collado et al., 2012; Khodayar-Pardo et al., 2014; Davé et al., 2016). Overweight women and those who gain excessive weight in pregnancy have been observed to harbor higher Lactobacillus and Staphylococcus, and lower Bifidobacterium counts in two prior studies (Cabrera-Rubio et al., 2012; Collado et al., 2012). In addition, maternal pre-pregnancy BMI also has been negatively associated with Streptococcus abundance, positively associated with overall microbial diversity (Davé et al., 2016) and diversity of Firmicutes, and negatively associated with the diversity of Proteobacteria (Moossavi et al., 2019b). In a small study of 18 Finnish women, milk from mothers who delivered by elective cesarean section had differential abundance of a number of microbes compared to those who delivered by non-elective cesarean section or vaginal delivery (Cabrera-Rubio et al., 2012). Further, in a study of 32 Spanish women, cesarean delivery was associated with increased bacterial counts in colostrum and transitional milk, and reduced likelihood of Bifidobacterium presence (Khodayar-Pardo et al., 2014). Grouping by both delivery mode and intrapartum antibiotic exposure shows that subjects cluster primarily by delivery mode, although both factors significantly associate with milk microbiome profile (Hermansson et al., 2019). Prophylactic intrapartum antibiotic exposure negatively associated with Bifidobacterium levels (Padilha et al., 2019b), yet is associated with increased microbial diversity and richness in breast milk (Hermansson et al., 2019). Additionally, women who delivered prematurely show lower abundance of Bifidobacterium (Khodayar-Pardo et al., 2014). However, other studies have not observed associations of delivery mode or gestational age with breast milk microbiota (Sakwinska et al., 2016; Urbaniak et al., 2016; Pannaraj et al., 2017; Ojo-Okunola et al., 2019). Relatively small sample size or unmeasured confounding factors in prior work may account for inconsistent results across studies and indicates the need for further investigation in larger studies.
Other important factors to the human milk microbiome have been identified, including the method of feeding, likely due to microbes from the infant oral cavity being transferred to the breast during feeding or microbes derived from the breast pump contaminating pumped milk. In a study of preterm infant–mother pairs, the microbial composition of breast milk changed after infants began direct feeding by latching (Biagi et al., 2018). Further, milk samples from mothers who sometimes fed infants indirectly via pumped milk show decreased alpha diversity, reduced prevalence of Bifidobacterium, and increased levels of Enterobacteriaceae and Pseudomonadaceae (Moossavi et al., 2019b). Additionally, ethnicity and geographical location (Kumar et al., 2016; Ojo-Okunola et al., 2019), diet (Padilha et al., 2019a), social network size of mother–infant pairs (Meehan et al., 2018), human milk oligosaccharides and other metabolites (Gómez-Gallego et al., 2018; Moossavi et al., 2019a), as well as maternal secretor status (Cabrera-Rubio et al., 2019) have been shown to affect microbial populations in breast milk.
To date, preliminary literature examining the microbiota associated with breastfeeding has been limited by study size, has largely focused on the abundance of specific microbes, and has given an incomplete picture of infants’ microbial exposure during breastfeeding due to sterile milk collection protocols. As part of a large pregnancy cohort study, we had the opportunity to characterize the “breastfeeding microbiome,” or the microbial communities that infants are exposed to during feeding. We used milk collected from a relatively racially homogeneous population of women in New Hampshire who provided milk samples around 6 weeks postpartum, without sterilization of the skin prior to collection in order to capture the full range of bacteria infants are exposed to during feeding. Using sequencing of the V4–V5 hypervariable region of the bacterial 16S rRNA gene, we sought to identify associations of hypothesized factors with the breastfeeding microbiota, including maternal pre-pregnancy BMI, gestational weight gain, delivery mode, and gestational age.
Materials and Methods
Study Population
Study subjects were from the New Hampshire Birth Cohort Study (NHBCS). Eligible participants for this birth cohort are pregnant women between the ages of 18 and 45 years who report using a private well for their home water source and are receiving prenatal care in clinics in New Hampshire, United States, as previously described (Gilbert-Diamond et al., 2011; Farzan et al., 2013). Subjects were recruited between approximately 24 and 28 weeks of gestation at routine prenatal visits. The Center for the Protection of Human Subjects at Dartmouth gave institutional review board approval, and all methods were performed according to guidelines. All subjects gave written informed consent for participation for themselves and their infants.
Telephone interviews conducted every 4 months ascertained breastfeeding and infant formula supplementation, as well as maternal medication use such as antibiotics. Mothers reporting any infant formula supplementation were considered to be combination feeding, while those reporting only breastfeeding were considered to be exclusively breastfeeding. Delivery mode, including elective vs. unplanned or emergent cesarean section, and peripartum antibiotic exposure were determined from maternal delivery records, while maternal ever smoking, race, education, relationship status, and parity were measured via a self-administered questionnaire upon study entry. Pre-pregnancy height and weight were determined either by questionnaire or from the medical record, if self-reported measures were unavailable, and were used to compute pre-pregnancy BMI. Gestational age was determined from prenatal medical records, estimated from date of last menstrual period and ultrasound. Weight gain during pregnancy and gestational diabetes was ascertained in a self-administered postpartum questionnaire.
Sample Collection and DNA Extraction
Breast milk samples were collected at home by study participants from unsterilized bilateral breasts, with separate study-provided sterile collection bottles used for milk from each breast. To capture a representative portrait of infant exposure during breastfeeding we did not use a sterile collection protocol. Subjects provided a minimum of 18 mL and up to 80 mL of milk from each breast, with a median of 35 mL per breast. Samples were stored in the refrigerator at participants homes for up to approximately 1 day, brought in cold packs to the postpartum follow-up appointment (between 3.7 and 12 weeks after delivery), and immediately chilled. Samples were processed within 24 h of receipt. Before sample processing, the milk was mixed by inverting the collection tubes 10 times. 3 mL of milk from each breast were combined, centrifuged at 3396 rcf for 90 min at 4°C. Fat and supernatant were removed, and 150 μL of PBS was added to and mixed with the cell pellet. The cell suspension was transferred to a 1.5 mL tube and frozen at −20°C. The sample was thawed at room temperature, and microbial DNA was extracted using the Zymo DNA extraction kit (Zymo Research) and was quantified using the OD260/280 Nanodrop.
Targeted 16S rRNA Sequencing
Using established methods (Huse et al., 2014; Newton et al., 2015), we sequenced the V4-V5 hypervariable region of the bacterial 16S rRNA gene at the Marine Biological Laboratory (MBL) in Woods Hole, MA, United States. Fusion primers were used to generate 16S rDNA V4–V5 amplicons from purified genomic DNA samples. Multiplexing of 96 samples per lane was possible due to the use of forward primers containing one of eight five-nucleotide barcodes between the Illumina-specific bridge and sequencing primer regions and the 16S-specific region and a single reverse primer containing one of 12 Illumina indices. Amplifications in triplicate with one negative control were used for internal quality control at the MBL in addition to positive and extraction controls prepared with samples at Dartmouth. The amplicon pool was quantified using qPCR (Kapa Biosystems). One Illumina MiSeq 500 cycle paired end run was used to sequence each pool of 96 libraries. Illumina MiSeq Reporter and a custom python script were used to demultiplex and divide datasets. Reads were not detected from extraction controls.
Microbiome Profiling
Microbial population profiles were identified using full-length amplicon sequences for the V4–V5 hypervariable regions of the 16S rRNA gene. Forward and reverse reads were joined based on sequence overlap (Eren et al., 2013). Primer sequences were removed, and sequences with any ambiguous nucleotides were discarded. The vsearch program was implemented to remove both known chimeras in comparison to the RDP classifier training reference and de novo (Rognes et al., 2016).
Data Processing and Analysis
Using QIIME version 1.9.1 (Caporaso et al., 2010b) we identified open reference operational taxonomic units (OTUs) at 97% similarity using the UCLUST algorithm (Edgar, 2010). Bacterial taxonomy was assigned using PyNAST alignment (Caporaso et al., 2010a) to the Greengenes reference database (Desantis et al., 2006; Mcdonald et al., 2012; Werner et al., 2012), and FastTree was used to construct the phylogenetic tree (Price et al., 2010). All statistical analyses were conducted in R version 3.3.0 (R Core Team, 2016).
Of 163 human milk samples collected at the 6-week time point and sequenced by September 24, 2016, 3 samples with less than 1000 sequence reads, 3 samples that were collected after 12 weeks postpartum, and 2 subjects with improbable weight gain values were excluded, resulting in a sample size of 155.
We calculated generalized UniFrac (GUniFrac) distances between paired samples using the OTU table, rarefied to the minimum sequencing depth (1863 reads), and midpoint rooted phylogenetic tree (Chen et al., 2012) to characterize the beta diversity of samples. Alpha diversity, a measure of the number and evenness of microbes in a sample, was characterized using Simpson’s diversity index, calculated using the diversity function in the R package vegan (Oksanen et al., 2015). Since Simpson’s diversity is bounded by 0 and 1, we logit transformed the values for analyses. We identified clusters of microbiota measured in human milk, “breastfeeding microbiome types (BMTs)” using an unsupervised clustering method, partitioning around medoids (PAM), on GUniFrac distances, as others have found this algorithm to perform well for microbiome data (Arumugam et al., 2011; Wu et al., 2011). We chose the optimal number of clusters (k = 4) by assessing within-cluster sum of squares for k of 2 through 10 using the elbow method, where the k at which the sum of squares begins to decrease less rapidly (the bend in the “elbow”) is considered optimal. Additionally, we examined average silhouette width (a measure of a sample’s similarity to its own versus another cluster), and observed separation of samples along the first and second principal coordinates by abundances of select bacterial taxa (Streptococcus, Staphylococcus, Acinetobacter, and Pseudomonas) and alpha diversity respectively. The mean silhouette width was greatest for k = 2, but the within cluster sum of squares continued to decrease until k = 5. This information, together with the observations related to cluster separation along the first axis by Streptococcus and Staphylococcus abundance, and along the second axis by alpha diversity, suggested k = 4 to be a biologically meaningful number of clusters. We compared alpha diversity between clusters using ANOVA and calculated 95% confidence intervals for pairwise comparisons using Tukey’s Honest Significant Difference. Differences in microbial community structure between clusters was tested using the permutational multivariate analysis of variance (PERMANOVA) method on GUniFrac distances with 10,000 permutations using the adonis function in the R package vegan (Oksanen et al., 2015). We tested for differences in the relative abundance of the top 25 most abundant (ranked by the median relative abundance) microbial taxa using the Kruskal–Wallis rank sum test, and Dunn’s test with FDR p-value correction to identify pairwise differences between BMTs. The Kruskal–Wallis rank sum test or Fisher’s exact test was used to test univariate associations between each variable and the BMT. Pairwise differences by BMT in maternal pre-pregnancy BMI and gestational weight gain were determined with Dunn’s test with FDR p-value correction. Multinomial logistic regression was used to test multivariate associations of covariates with cluster membership where the largest cluster (BMT2) was the reference. We calculated the predicted probabilities for each sample’s cluster membership, and plotted these values as a function of significant variables.
We used linear regression to test the associations between each variable and alpha diversity in a univariate and multivariate manner. Pairwise differences in alpha diversity by delivery mode were determined using Tukey’s Honest Significant Difference. To assess the relation between variables of interest and microbial community structure, we used the PERMANOVA method on GUniFrac distances with 10,000 permutations as specified previously.
For adjusted analyses, we limited our subjects to those without missing data for any variable with p-value < 0.10 in univariate analyses (n = 123). We performed sensitivity analyses limiting to women known to not have taken antibiotics in the postpartum period (up to 4-months postpartum, n = 92). We compared baseline characteristics between the overall study population and the subsets used for adjusted and sensitivity analyses (Supplementary Table S1).
Results
Subject Characteristics
Subject characteristics are presented in Table 1. Among study subjects, 94.8% were white, 81.3% were married, and average age was 32.4 years at study enrollment. Subjects were slightly overweight, with an average pre-pregnancy BMI of 26.0 and 41.3% gained more than the recommended amount of weight during pregnancy according to the Institute of Medicine guidelines (Institute of Medicine (US) and National Research Council (US) Committee to Reexamine Iom Pregnancy Weight Guidelines, 2009). Briefly, the recommended weight gain depends on pre-pregnancy BMI; for a singleton pregnancy, underweight, normal weight, overweight, and obese women should gain between 28–40, 25–35, 15–25, and 11–20 pounds, respectively. Parity ranged from 1 to 5. Gestational diabetes was rare, affecting only 5.2% of women, while peripartum antibiotic exposure was common with 45.8% of women given antibiotics before, during, or immediately after labor. Antibiotic exposure occurred in around 15% of subjects prenatally, and 11% within the first 4 months postpartum. Most deliveries were full term (average 39.2 weeks), but ranged from 30.0 to 42.4 weeks. The majority of women (70.3%) were exclusively breastfeeding at the time of sample collection around 6-weeks postpartum.
Most Abundant Breast Milk Taxa and Breast Milk Microbiome Types
High-throughput sequencing of the bacterial 16S rRNA gene V4–V5 hypervariable region yielded an average of ∼75,000 sequence reads per sample and a median of 46,366 reads per sample, with a range from 1,863 to 1,234,419 (Supplementary Figure S1). To ensure the largest possible sample size, we rarefied to the minimum sequencing depth, with reads mapping to 4,108 OTUs. The most highly abundant taxon was Acinetobacter, accounting for 14.3% of sequence reads per sample on average, followed by Streptococcus (13.7%), Pseudomonas (11.3%), Staphylococcus (11.0%), and Enterobacteriaceae (8.23%). Bacteroides and Bifidobacterium, well-studied microbes in the context of the infant gut, were present at 1.07 and 0.651% respectively.
Using partitioning around medoids, we identified four clusters of human milk microbiota, which we refer to as BMTs (Figure 1A). In principal coordinates analysis, PC 1 was positively related to abundance of Acinetobacter and Pseudomonas (Figure 1C), and negatively related to Streptococcus and Staphylococcus abundance (Figure 1B), while PC 2 was negatively related to Simpson’s diversity (Figure 1D). BMT1 was characterized by a high abundance of Streptococcus and Staphylococcus combined (>50% of a sample on average). Staphylococcus was highest and Enterobacteriaceae and Pseudomonas were lowest in BMT1 compared to all other clusters (Figures 2C,D). In BMT2 Streptococcus was the most abundant taxon, but samples were overall more diverse than those in BMT1, with other bacterial taxa comprising a large portion of microbiota, such as Caulobacteraceae with a median relative abundance of over 5%, and Phenylobacterium and Oxalobacteraceae with median relative abundances over 2% (Table 2); additionally, Bifidobacterium was most abundant in BMT2 compared to all other clusters (Figure 2V). Both BMT1 and BMT2 had higher Bacteroides levels than BMT3 and BMT4 (Figure 2Q). Acinetobacter was predominant in BMTs 3 and 4, with BMT3 also showing high median abundances of Enterobacteriaceae and Pseudomonas of around 8 and 4%, respectively. BMT4 was the least diverse cluster (Figure 1F) and was comprised predominantly of Acinetobacter, followed by Pseudomonas (Table 2). Simpson’s diversity index was highest in BMT2, and lowest in BMT4 (Figure 1E); all clusters had significantly different alpha diversity except for BMT1 and BMT3 (Figure 1F). Overall microbial community structure was significantly different according to BMT cluster membership (Figure 1G, GUniFrac PERMANOVA p-value = 1.0 × 10–4).
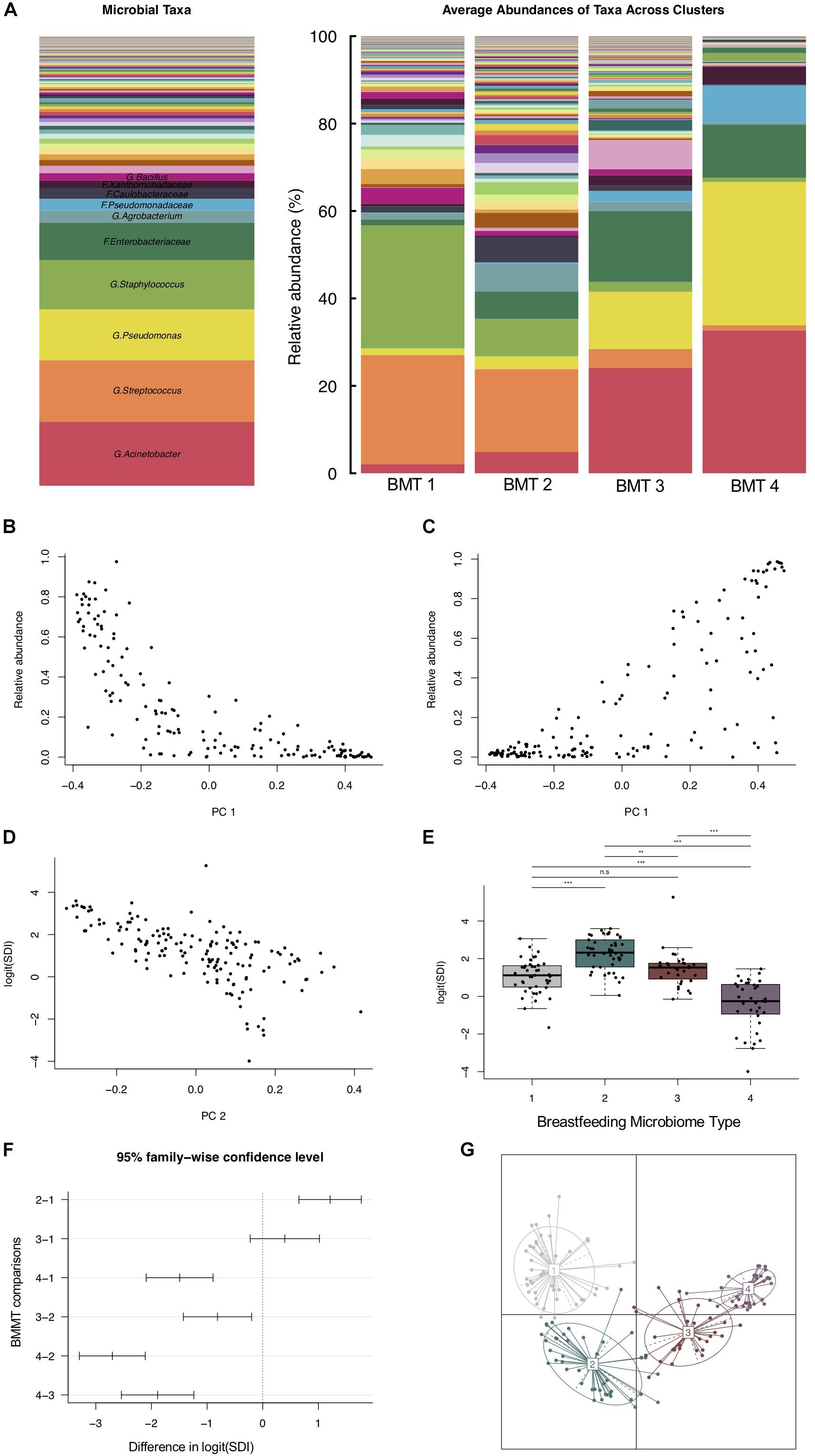
Figure 1. Breastfeeding microbiome types (BMTs) differ in diversity and taxonomic abundances. (A) Barplot showing the relative abundance of microbial taxa overall and on average within each of the four breastfeeding microbiome types [BMT1 (n = 43), BMT2 (n = 46), BMT3 (n = 31), BMT4 (n = 35)]. (B) The y-axis represents the combined relative abundance of Streptococcus + Staphylococcus in breast milk samples as a function of PC 1. (C) The y-axis represents the combined relative abundance of Pseudomonas + Acinetobacter in breast milk samples as a function of PC 1. (D) Microbial alpha diversity (Simpson’s diversity index) as a function of PC 2. (E) Boxplot showing microbial alpha diversity for each of the four BMTs, with significance of pairwise differences determined by ANOVA and Tukey’s HSD. Significance levels are n.s., not significant, ∗p-value < 0.05, ∗∗p-value < 0.01, ∗∗∗p-value < 0.001. (F) 95% confidence interval for pairwise differences in alpha diversity between BMTs calculated using ANOVA and Tukey’s HSD. (G) Principal coordinate analysis plot colored by BMT, indicated by the number label at the cluster centroid. Each point represents one subject, and lines indicate the distance from the cluster centroid.
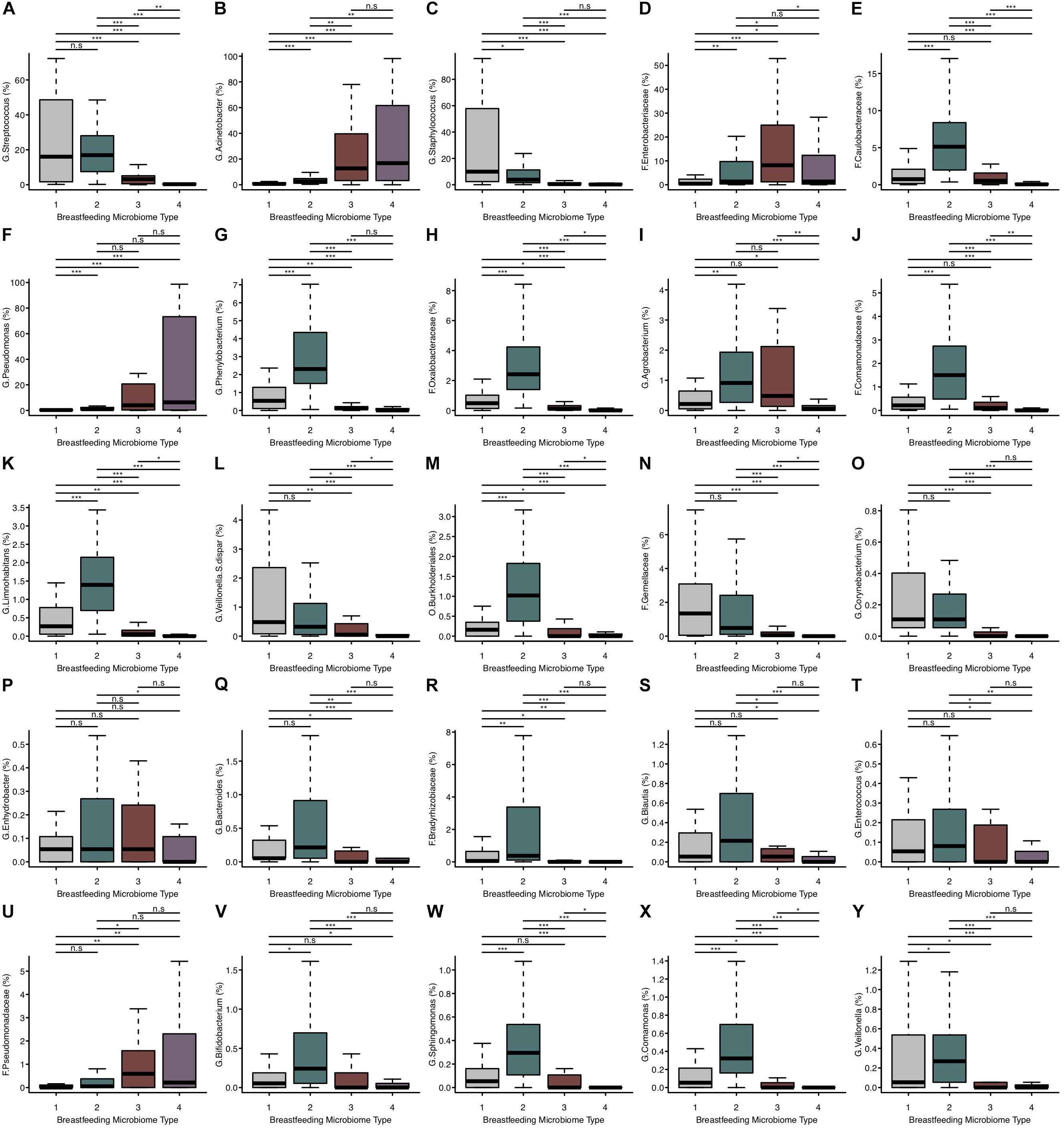
Figure 2. The relative abundance of microbial taxa differs between BMTs. (A–Y) Boxplots showing the relative abundance of the top 25 most abundant microbial taxa in breast milk for each BMT. Significance was determined by the Kruskal-Wallis rank-sum test followed by Dunn’s test with FDR correction. Significance levels are n.s., not significant, ∗p-value < 0.05, ∗∗p-value < 0.01, ∗∗∗p-value < 0.001.
There were both similarities and differences in the relative abundances of microbial taxa between different BMTs (Figures 2A–Y). For example, BMT1 and BMT2 had similar relative abundances of Streptococcus (Figure 2A) and Bacteroides (Figure 2Q), yet differed in abundances of Staphylococcus (Figure 2C), Enterobacteriaceae (Figure 2D), Bifidobacterium (Figure 2V) and many other taxa. Similarly, BMT3 and BMT4 had similar levels of Acinetobacter (Figure 2B) and Pseudomonas (Figure 2F), but differed by Streptococcus (Figure 2A), Caulobacteraceae (Figure 2E), and other taxa. Abundances of most taxa were different when comparing BMT1 to BMT3 and BMT4, except for a few taxa including Caulobacteraceae (BMT3, Figure 2E), and Enhydrobacter (BMT4, Figure 2P). Likewise, BMT2 was mostly different from BMT3 and BMT4, except for in the abundance of a few taxa. The relative abundances of some taxa, including Oxalobacteraceae, Limnohabitans, and Burkholderiales, were significantly different between all BMTs (Figures 2H,K,M).
In univariate analyses, maternal pre-pregnancy BMI was associated with cluster membership (Kruskal–Wallis rank sum test p-value = 0.042, n = 138), primarily between BMT2 vs. BMT3 (Dunn’s test p-value < 0.05) and BMT2 vs. BMT4 (Dunn’s test p-value < 0.05). Weight gain during pregnancy was marginally associated with cluster membership overall (Kruskal–Wallis rank sum test p-value = 0.050, n = 138), but significant differences were detected for BMT1 vs. BMT2 (Dunn’s test p-value < 0.05) and BMT1 vs. BMT3 (Dunn’s test p-value < 0.05, Figure 3 and Table 3). In addition to maternal BMI and weight gain, univariate multinomial logistic regression provided evidence that parity and postpartum week at sample collection were related to cluster membership (Supplementary Table S2). To test for associations between covariates and BMTs we fit multinomial logistic regression models adjusted for maternal BMI, weight gain during pregnancy, parity, and postpartum week at sample collection with BMT2 as the reference cluster (n = 123, Supplementary Table S2). For each unit increase in maternal BMI we observed an increased odds of membership in BMT1 compared to BMT2 [OR (95% CI) = 1.13 (1.02, 1.24)] and BMT3 compared to BMT2 [OR (95% CI) = 1.12 (1.01, 1.25), Figure 3C]. Additionally, increasing maternal weight gain (per 10 pounds) was associated with decreased probability of belonging to BMT1 versus BMT2 [OR (95% CI) = 0.66 (0.44, 1.00)] (Figure 3D), and there was an increased probability membership in BMT3 versus BMT2 with increasing parity [OR (95% CI) = 1.57 (0.81, 3.04)] and of membership in BMT4 versus BMT2 with increasing sample collection week [OR (95% CI) = 1.72 (1.00, 2.95)], but these associations did not reach statistical significance in the adjusted model.
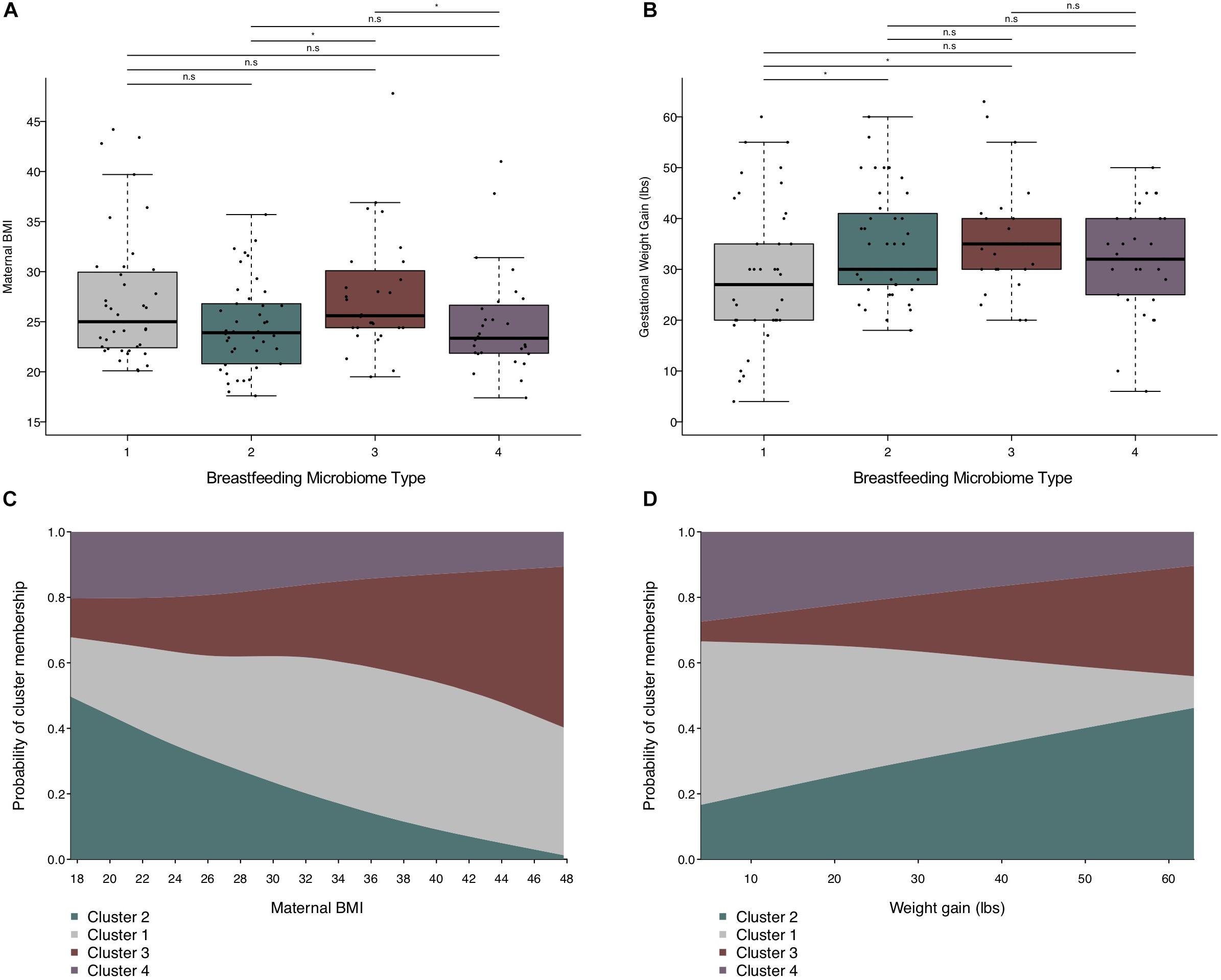
Figure 3. Maternal weight measures are related to BMT. (A) Boxplot showing the distribution of maternal pre-pregnancy BMI by BMT. (B) Boxplot of the distribution of gestational weight gain by BMT. Significance was determined by the Kruskal–Wallis rank-sum test followed by Dunn’s test with FDR correction. Significance levels are n.s., not significant, ∗p-value < 0.05. (C) Predicted probability plot of BMT membership by pre-pregnancy BMI from multinomial logistic regression models (n = 123), where the proportion of each block along the y-axis represents the probability of cluster membership for a given pre-pregnancy BMI. The model was adjusted for sample collection week, weight gain during pregnancy (per 10 lbs.), and parity. Cluster 2 is the reference group. (D) Predicted probability plot of BMT membership by weight gain during pregnancy from multinomial logistic regression models (n = 123), where the proportion of each block along the y-axis represents the probability of cluster membership for a given gestational weight gain. The model was adjusted for sample collection week, pre-pregnancy BMI, and parity. Cluster 2 is the reference group.
Characteristics Related to Microbial Alpha Diversity
Univariate models showed a negative association between postpartum week at sample collection and breastfeeding microbiota alpha diversity, and a positive association of weight gain during pregnancy with alpha diversity (Figures 4A,B and Supplementary Table S3). Additionally, maternal antibiotic exposure by 4-months postpartum was positively associated with microbial alpha diversity (Figure 4C and Supplementary Table S3), despite potential misclassification of exposure since we do not know if milk collection happened before or after antibiotic usage. Prenatal antibiotic exposure was not associated with breastfeeding microbiota diversity (Supplementary Table S3). In adjusted models, postpartum week at sample collection was associated with decreased alpha diversity (Simpson’s diversity, β = −0.33, p-value = 0.0039), and weight gain during pregnancy (per each 10 pounds gained) was associated with increased alpha diversity (Simpson’s diversity, β = 0.23, p-value = 0.022; Table 4). Restricting the analysis to women known to have not taken antibiotics before 4-months postpartum did not qualitatively change these results (Table 4). We found no clear association between alpha diversity of breastfeeding microbiota and delivery mode (Figure 4D and Supplementary Table S3), or between alpha diversity and women who experienced labor prior to delivery versus those that did not (data not shown).
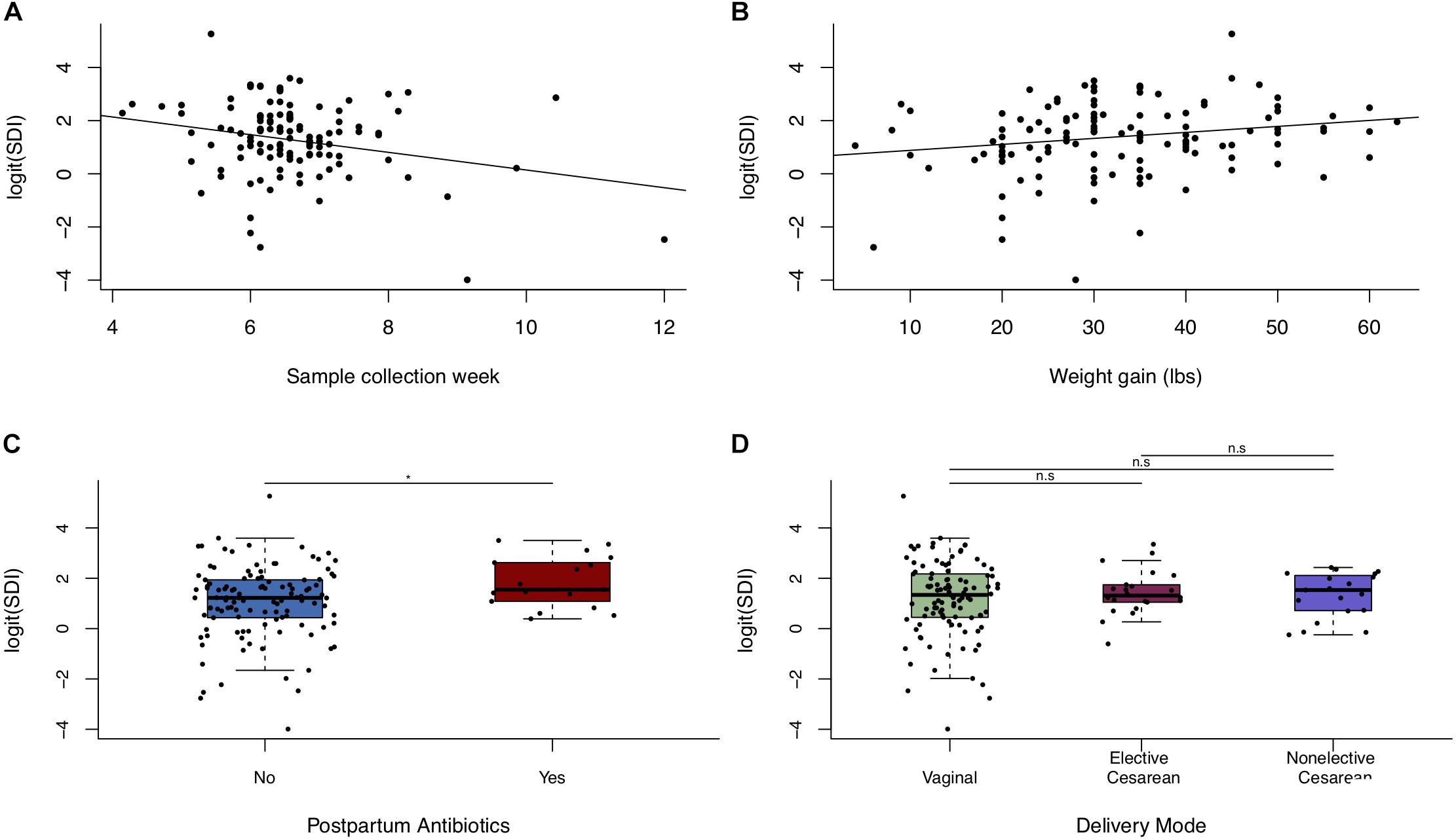
Figure 4. Alpha diversity of breastfeeding microbiota in relation to maternal weight and delivery mode. Scatter plots (n = 123) of logit(SDI) vs. (A) postpartum week at sample collection week and (B) weight gain during pregnancy. (C) Boxplot of logit(SDI) vs. postpartum antibiotic exposure. (D) Boxplot of logit(SDI) vs. delivery mode (n = 123). Significance was determined by ANOVA followed by Tukey’s HSD. Significance levels are n.s., not significant, ∗p-value < 0.05.
Characteristics Related to Microbial Community Structure
Postpartum week at sample collection, maternal weight gain, and parity were marginally associated with microbial community structure of breastfeeding microbiota in PERMANOVA analysis on generalized UniFrac distances in both univariate models and those adjusted for sample collection age, maternal weight gain, and parity (Supplementary Table S4). Differences were not observed with the community structure of breastfeeding microbiota by delivery mode or by antibiotic exposure at any time. The association with maternal weight gain was significant in sensitivity analysis restricting to women known not to have received antibiotics by 4 months postpartum (GUniFrac PERMANOVA, p-value = 0.036).
Discussion
We collected milk from women enrolled in a large molecular epidemiology birth cohort at approximately 6 weeks postpartum and measured the microbial composition using targeted sequencing of the 16S rRNA gene to evaluate physiological and environmental factors associated with the microbiota infants consume during feeding. Overall, the bacterial taxa we identified in our study are consistent with what has been found in the limited number of previous studies on human milk using sequencing of the bacterial 16S rRNA gene, with the most abundant bacteria on average belonging to the genera Acinetobacter, Streptococcus, Pseudomonas, and Staphylococcus. Unsupervised clustering revealed four main patterns of microbiota that have not previously been reported, denoted here as BMTs. While the average abundance of these four microbes are similar across all samples, two clusters each were characterized by higher abundances of Streptococcus and Staphylococcus, or Pseudomonas and Acinetobacter with differences in alpha diversity and the abundances of numerous other taxa further differentiating these clusters. The clusters we identified share both similarities and differences with the breast milk microbiome clusters identified in Chinese (Li et al., 2017), Italian (Biagi et al., 2018), and Canadian (Moossavi et al., 2019b) mothers, suggesting that some patterns of microbial populations in human milk may be similar amongst geographically and racially distinct populations.
Bacteria belonging to the genera Acinetobacter and Pseudomonas are often thought of as pathogens contributing to nosocomial infections (Fazeli et al., 2012; Custovic et al., 2014). However, given that over 40% of subjects belong to clusters characterized by high levels of these two microbes, we suggest their presence in breast milk is unlikely to be pathogenic. In fact, skin colonization of Acinetobacter has been associated with protection from allergies (Hanski et al., 2012) through dendritic cell programing (Debarry et al., 2010), a benefit that could possibly be transferred to the infant. In our cohort, 100% of infants with detectable Acinetobacter in their stool have mothers with detectable Acinetobacter in their milk, with an average relative abundance of 0.01% on average in the infant gut at 6-weeks of age. However, with targeted 16S rRNA sequencing data, we cannot confirm that the sequencing reads originate from the same strain in paired maternal milk and infant stool. Breast milk microbiota, including Acinetobacter, may contribute to the infant gut microbiome through multiple mechanisms in addition to direct colonization of the infant gut, for example through interactions with other microbes in the infant gut.
In the first BMT, Firmicutes were particularly prominent, a phylum associated with overweight and obesity (Koliada et al., 2017). This is interesting given that membership in the first cluster was associated with increased maternal pre-pregnancy BMI in our study. Studies suggest that maternal weight influences child weight status, potentially through alterations to infant gut microbiota (Ajslev et al., 2011; Mueller et al., 2016) in addition to genetics (Pietiläinen et al., 1999). Future studies may help elucidate whether infants of mothers belonging to this first cluster are at an increased risk for overweight.
We found a positive relationship between weight gain during pregnancy and bacterial alpha diversity of breastfeeding microbiota, as well as associations with cluster membership and microbial community structure. To our knowledge, this question has not previously been investigated in a cohort of this size. The maternal gut microbiome is thought to be a source of human milk microbiota (Jost et al., 2014), though only a small number of bacteria are shared between the maternal gut and breast milk (Avershina et al., 2018), and maternal intestinal microbiota have been found to correlate with weight gain during pregnancy (Santacruz et al., 2010). There is evidence that the maternal intestinal microbiome transforms in late pregnancy to promote insulin insensitivity, inflammation, and weight gain, and that this shift varies widely between women (Koren et al., 2012). It is possible that the higher alpha diversity of breastfeeding microbiota in women who gain more weight during pregnancy could result from this shift in maternal intestinal microbiota, perhaps due to changes in intestinal permeability. However, further studies are needed to test this hypothesis. Although maternal pre-pregnancy BMI was related to cluster membership in our study, we did not find an association with other measures of the microbiome. There was not strong evidence of a relation between parity and breastfeeding microbiota, or of infant gestational age, prenatal antibiotics, and peripartum antibiotics, although we found evidence that postpartum antibiotics relate with increased microbial diversity in breast milk despite potential misclassification of subjects. This suggests that the effect of antibiotics may be transient as is supported by existing studies (Padilha et al., 2019b), and that the effect of peripartum antibiotics may only be observable in the postpartum period before when the samples in this study were collected. Additional investigation will be necessary to understand these questions.
Delivery mode was not related to the breastfeeding microbiota in our study. Differences by delivery mode were observed in a study of milk samples from 80 women in four countries on three continents, but the effect was primarily among the subgroup of 20 Spanish women (Kumar et al., 2016). Consistent with our findings, a recent study of human milk from 60 Chinese women across three time points and two collection techniques (Sakwinska et al., 2016) and one studying 109 American mothers (Pannaraj et al., 2017) did not observe an association of cesarean section delivery with microbial populations in human milk. Other studies suggesting an influence of delivery mode on human milk microbiota (Cabrera-Rubio et al., 2012; Khodayar-Pardo et al., 2014; Cabrera-Rubio et al., 2016) were very small, with 32 subjects or fewer. Given the inter-individual variability of human milk microbiota, the addition of only a few subjects could potentially shift the results when the sample size is small, or the limited ability to adjust for confounding factors may account for varying findings between previous studies. Alternatively, differences in human milk microbiota by delivery mode may exist only early in the lactation period, dissipating by the time of sample collection in our study.
Many samples in our study contained Acinetobacter. This may be partially attributable to the collection method that did not involve sterilization of the breast skin prior to collection using a breast pump (Sakwinska et al., 2016), which we chose in order to have a clearer understanding of infants’ actual exposures. However, many subjects had very low abundances of this microbe despite the same collection method, so factors other than this likely contribute to its presence. Breastfeeding is not an aseptic process, and the microbial flora inhabiting the mother’s skin that infants are exposed to during feeding also play an important role in microbial colonization of the infant gut (Pannaraj et al., 2017). Non-aseptic human milk collection is thus preferable for assessing the relation between human milk and the infant gut microbiome, particularly since there is clear variation of these microbes between women. It is also possible that differences in both the detection of certain bacterial taxa as well as in reported associations between breast milk microbiota and factors of interest occur due to differences in the microbial sequencing method used. The reported 16S rRNA gene regions sequenced in reported studies varies widely, including V4–V5 used here, V1–V3 (Cabrera-Rubio et al., 2019), V1–V2 (Cabrera-Rubio et al., 2012), and commonly V4 (Kumar et al., 2016; Moossavi et al., 2019b; Ojo-Okunola et al., 2019) and others. In breast milk specifically, sequencing of the V1-V2 region does not detect Lactobacillus and Acinetobacter, V4 does not detect Listeria, V5-V6 does not detect Staphylococcus and Veillonella, and V9 does not detect Streptococcus (Meyer et al., 2019).
A strength of our study is its large sample size, which enabled us to adjust for potential confounding factors. Further, our well-defined, racially homogeneous study population supports the internal validity of our study. For instance, all of the subjects in our study resided in the same geographical region, and nearly all were white. Nonetheless, the generalizability of our findings to other populations requires independent validation. A high proportion of subjects in our study received peripartum antibiotics either as prophylaxis for cesarean delivery or for Group B Streptococcus colonization and peripartum prophylaxis, or for presumed intrapartum infection, which may differ in other populations. Our measure of maternal pre-pregnancy BMI is based on self-reported height and weight; at an average of 26.5 was lower than the U.S. average for women in 2015–2016 of 29.6 (Fryar et al., 2018). Given that 69% of subjects in our study completed college or postgraduate school (Emeny et al., 2019) and the association of high educational attainment with lower BMI (Hermann et al., 2011), it is not unexpected that BMI in our source population is lower than the U.S. national average. However, some subjects may have mis-reported their weight or height. Further, a pre-pregnancy measurement may not be the most relevant time of measurement for breastfeeding, which is occurs after delivery. Additionally, we were unable to adjust for maternal antibiotic usage that may have occurred in the approximately 6 week interval between delivery and sample collection due to lack of detail regarding the timing of exposure, but sensitivity analyses excluding individuals who may have received antibiotics in the first 4 months following delivery yielded consistent estimates.
Conclusion
In a prospective pregnancy cohort study of the general population, we found that breastfeeding microbiota follow four general patterns characterized by high or low alpha diversity as well as differential abundances of Streptococcus, Staphylococcus, Acinetobacter, and Pseudomonas. We found that these patterns may be shaped by maternal weight, including both pre-pregnancy BMI and weight gain during pregnancy, independently. Future studies are necessary to determine the extent to which these microbial populations can be altered and the implications of milk microbiota to infants’ health, including the development of the infant intestinal microbiome.
Data Availability Statement
The 16S rRNA gene sequences analyzed in this study are available through the National Center for Biotechnology Information (NCBI) Sequence Read Archive: http://www.ncbi.nlm.nih.gov/sra (Accession number PRJNA296814).
Ethics Statement
The studies involving human participants were reviewed and approved by the Center for the Protection of Human Subjects at Dartmouth. The participants provided their written informed consent to participate in this study.
Author Contributions
MK and JM designed the research. SL performed statistical analyses and wrote the manuscript. SL, AH, BC, MK, and JM interpreted the results. HM provided technical and methodological support from the Marine Biological Laboratory. BC has primary responsibility for the final content. All authors reviewed the manuscript, offered critical feedback, and approved the final version.
Funding
This study was supported by funding from the National Institutes of Health (Grants NIGMS P20GM104416, NIEHS P01ES022832, NIEHS P42ES007373, NLM K01LM011985, NLM R01LM012723, NCI R01CA216265, and NIDCR R01DE022772), the US Environmental Protection Agency (RD83544201), and the Rosaline Borison Memorial Fund.
Conflict of Interest
The authors declare that the research was conducted in the absence of any commercial or financial relationships that could be construed as a potential conflict of interest.
Acknowledgments
We would like to thank Dr. Emily Baker as well as the study staff and participants in the New Hampshire Birth Cohort Study, without which this research would not be possible. The content of this manuscript has been included in the doctoral thesis of SL (Lundgren, 2019).
Supplementary Material
The Supplementary Material for this article can be found online at: https://www.frontiersin.org/articles/10.3389/fmicb.2019.02886/full#supplementary-material
References
Admyre, C., Johansson, S. M., Qazi, K. R., Filen, J. J., Lahesmaa, R., Norman, M., et al. (2007). Exosomes with immune modulatory features are present in human breast milk. J. Immunol. 179, 1969–1978. doi: 10.4049/jimmunol.179.3.1969
Ajslev, T. A., Andersen, C. S., Gamborg, M., Sorensen, T. I., and Jess, T. (2011). Childhood overweight after establishment of the gut microbiota: the role of delivery mode, pre-pregnancy weight and early administration of antibiotics. Int. J. Obes. 35, 522–529. doi: 10.1038/ijo.2011.27
Arrieta, M. C., Stiemsma, L. T., Dimitriu, P. A., Thorson, L., Russell, S., Yurist-Doutsch, S., et al. (2015). Early infancy microbial and metabolic alterations affect risk of childhood asthma. Sci. Transl. Med. 7:307ra152. doi: 10.1126/scitranslmed.aab2271
Arumugam, M., Raes, J., Pelletier, E., Le Paslier, D., Yamada, T., Mende, D. R., et al. (2011). Enterotypes of the human gut microbiome. Nature 473, 174–180. doi: 10.1038/nature09944
Avershina, E., Angell, I., Simpson, M., Storrø, O., Øien, T., Johnsen, R., et al. (2018). Low maternal microbiota sharing across gut, breast milk and vagina, as revealed by 16S rRNA gene and reduced metagenomic sequencing. Genes 9:231. doi: 10.3390/genes9050231
Biagi, E., Aceti, A., Quercia, S., Beghetti, I., Rampelli, S., Turroni, S., et al. (2018). Microbial community dynamics in mother’s milk and infant’s mouth and gut in moderately preterm infants. Front. Microbiol. 9:2512. doi: 10.3389/fmicb.2018.02512
Browne, P. D., Aparicio, M., Alba, C., Hechler, C., Beijers, R., Rodríguez, J. M., et al. (2019). Human milk microbiome and maternal postnatal psychosocial distress. Front. Microbiol. 10:2333. doi: 10.3389/fmicb.2019.02333
Cabrera-Rubio, R., Collado, M. C., Laitinen, K., Salminen, S., Isolauri, E., and Mira, A. (2012). The human milk microbiome changes over lactation and is shaped by maternal weight and mode of delivery. Am. J. Clin. Nutr. 96, 544–551. doi: 10.3945/ajcn.112.037382
Cabrera-Rubio, R., Kunz, C., Rudloff, S., García-Mantrana, I., Crehuá-Gaudiza, E., Martínez-Costa, C., et al. (2019). Association of maternal secretor status and human milk oligosaccharides with milk microbiota: an observational pilot study. J. Pediatr. Gastroenterol. Nutr. 68, 256–263. doi: 10.1097/MPG.0000000000002216
Cabrera-Rubio, R., Mira-Pascual, L., Mira, A., and Collado, M. C. (2016). Impact of mode of delivery on the milk microbiota composition of healthy women. J. Dev. Orig. Health Dis. 7, 54–60. doi: 10.1017/S2040174415001397
Canani, R. B., Costanzo, M. D., Leone, L., Pedata, M., Meli, R., and Calignano, A. (2011). Potential beneficial effects of butyrate in intestinal and extraintestinal diseases. World J. Gastroenterol. 17, 1519–1528. doi: 10.3748/wjg.v17.i12
Caporaso, J. G., Bittinger, K., Bushman, F. D., Desantis, T. Z., Andersen, G. L., and Knight, R. (2010a). PyNAST: a flexible tool for aligning sequences to a template alignment. Bioinformatics 26, 266–267. doi: 10.1093/bioinformatics/btp636
Caporaso, J. G., Kuczynski, J., Stombaugh, J., Bittinger, K., Bushman, F. D., Costello, E. K., et al. (2010b). QIIME allows analysis of high-throughput community sequencing data. Nat. Methods 7, 335–336.
Chen, J., Bittinger, K., Charlson, E. S., Hoffmann, C., Lewis, J., Wu, G. D., et al. (2012). Associating microbiome composition with environmental covariates using generalized UniFrac distances. Bioinformatics 28, 2106–2113. doi: 10.1093/bioinformatics/bts342
Chichlowski, M., De Lartigue, G., German, J. B., Raybould, H. E., and Mills, D. A. (2012). Bifidobacteria isolated from infants and cultured on human milk oligosaccharides affect intestinal epithelial function. J. Pediatr. Gastroenterol. Nutr. 55, 321–327. doi: 10.1097/MPG.0b013e31824fb899
Collado, M. C., Laitinen, K., Salminen, S., and Isolauri, E. (2012). Maternal weight and excessive weight gain during pregnancy modify the immunomodulatory potential of breast milk. Pediatr. Res. 72, 77–85. doi: 10.1038/pr.2012.42
Custovic, A., Smajlovic, J., Tihic, N., Hadzic, S., Ahmetagic, S., and Hadzagic, H. (2014). Epidemiological monitoring of nosocomial infections caused by Acinetobacter baumannii. Med. Arch. 68, 402–406. doi: 10.5455/medarh.2014.68.402-406
Davé, V., Street, K., Francis, S., Bradman, A., Riley, L., Eskenazi, B., et al. (2016). Bacterial microbiome of breast milk and child saliva from low-income Mexican-American women and children. Pediatr. Res. 79, 846–854. doi: 10.1038/pr.2016.9
Debarry, J., Hanuszkiewicz, A., Stein, K., Holst, O., and Heine, H. (2010). The allergy-protective properties of Acinetobacter lwoffii F78 are imparted by its lipopolysaccharide. Allergy 65, 690–697. doi: 10.1111/j.1398-9995.2009.02253.x
Desantis, T. Z., Hugenholtz, P., Larsen, N., Rojas, M., Brodie, E. L., Keller, K., et al. (2006). Greengenes, a chimera-checked 16S rRNA gene database and workbench compatible with ARB. Appl. Environ. Microbiol. 72, 5069–5072. doi: 10.1128/aem.03006-05
Edgar, R. C. (2010). Search and clustering orders of magnitude faster than BLAST. Bioinformatics 26, 2460–2461. doi: 10.1093/bioinformatics/btq461
Emeny, R. T., Korrick, S. A., Li, Z., Nadeau, K., Madan, J., Jackson, B., et al. (2019). Prenatal exposure to mercury in relation to infant infections and respiratory symptoms in the new hampshire birth cohort study. Environ. Res. 171, 523–529. doi: 10.1016/j.envres.2019.01.026
Eren, A. M., Vineis, J. H., Morrison, H. G., and Sogin, M. L. (2013). A filtering method to generate high quality short reads using illumina paired-end technology. PLoS One 8:e66643. doi: 10.1371/journal.pone.0066643
Farzan, S. F., Korrick, S., Li, Z., Enelow, R., Gandolfi, A. J., Madan, J., et al. (2013). In utero arsenic exposure and infant infection in a United States cohort: a prospective study. Environ. Res. 126, 24–30. doi: 10.1016/j.envres.2013.05.001
Fazeli, H., Akbari, R., Moghim, S., Narimani, T., Arabestani, M. R., and Ghoddousi, A. R. (2012). Pseudomonas aeruginosa infections in patients, hospital means, and personnel’s specimens. J. Res. Med. Sci. 17, 332–337.
Fryar, C. D., Kruszan-Moran, D., Gu, Q., and Ogden, C. L. (2018). Mean body weight, weight, waist circumference, and body mass index among adults: United States, 1999–2000 through 2015–2016. Nat. Health Stat. Rep. 122, 1–16.
Gilbert-Diamond, D., Cottingham, K. L., Gruber, J. F., Punshon, T., Sayarath, V., Gandolfi, A. J., et al. (2011). Rice consumption contributes to arsenic exposure in US women. Proc. Natl. Acad. Sci. U.S.A. 108, 20656–20660. doi: 10.1073/pnas.1109127108
Giongo, A., Gano, K. A., Crabb, D. B., Mukherjee, N., Novelo, L. L., Casella, G., et al. (2011). Toward defining the autoimmune microbiome for type 1 diabetes. ISME J. 5, 82–91. doi: 10.1038/ismej.2010.92
Goldman, A. S., Garza, C., Nichols, B. L., and Goldblum, R. M. (1982). Immunologic factors in human milk during the first year of lactation. J. Pediatr. 100, 563–567. doi: 10.1016/s0022-3476(82)80753-1
Gómez-Gallego, C., Morales, J., Monleón, D., Du Toit, E., Kumar, H., Linderborg, K., et al. (2018). Human breast milk NMR metabolomic profile across specific geographical locations and its association with the milk microbiota. Nutrients 10:1355. doi: 10.3390/nu10101355
Grönlund, M. M., Arvilommi, H., Kero, P., Lehtonen, O. P., and Isolauri, E. (2000). Importance of intestinal colonisation in the maturation of humoral immunity in early infancy: a prospective follow up study of healthy infants aged 0-6 months. Arch. Dis. Child Fetal Neonatal. 83, F186–F192.
Hanski, I., Von Hertzen, L., Fyhrquist, N., Koskinen, K., Torppa, K., Laatikainen, T., et al. (2012). Environmental biodiversity, human microbiota, and allergy are interrelated. Proc. Natl. Acad Sci. U.S.A. 109, 8334–8339. doi: 10.1073/pnas.1205624109
Hawkes, J. S., Bryan, D. L., James, M. J., and Gibson, R. A. (1999). Cytokines (IL-1beta, IL-6, TNF-alpha, TGF-beta1, and TGF-beta2) and prostaglandin E2 in human milk during the first three months postpartum. Pediatr. Res. 46, 194–199. doi: 10.1203/00006450-199908000-00012
Hermann, S., Rohrmann, S., Linseisen, J., May, A. M., Kunst, A., Besson, H., et al. (2011). The association of education with body mass index and waist circumference in the EPIC-PANACEA study. BMC Publ. Health 11:169. doi: 10.1186/1471-2458-11-169
Hermansson, H., Kumar, H., Collado, M. C., Salminen, S., Isolauri, E., and Rautava, S. (2019). Breast milk microbiota is shaped by mode of delivery and intrapartum antibiotic exposure. Front. Nutr. 6:4. doi: 10.3389/fnut.2019.00004
Hunt, K. M., Foster, J. A., Forney, L. J., Schutte, U. M., Beck, D. L., Abdo, Z., et al. (2011). Characterization of the diversity and temporal stability of bacterial communities in human milk. PLoS One 6:e21313. doi: 10.1371/journal.pone.0021313
Huse, S. M., Young, V. B., Morrison, H. G., Antonopoulos, D. A., Kwon, J., Dalal, S., et al. (2014). Comparison of brush and biopsy sampling methods of the ileal pouch for assessment of mucosa-associated microbiota of human subjects. Microbiome 2:5. doi: 10.1186/2049-2618-2-5
Institute of Medicine (US) and National Research Council (US) Committee to Reexamine Iom Pregnancy Weight Guidelines, (2009). Weight Gain During Pregnancy: Reexamining the Guidelines. Washington, DC: National Academies Press.
Ip, S., Chung, M., Raman, G., Chew, P., Magula, N., Devine, D., et al. (2007). Breastfeeding and maternal and infant health outcomes in developed countries. Evid. Rep. Technol. Assess. 153, 1–186.
Jiménez, E., De Andrés, J., Manrique, M., Pareja-Tobes, P., Tobes, R., Martínez-Blanch, J. F., et al. (2015). Metagenomic analysis of milk of healthy and mastitis-suffering women. J. Hum. Lact. 31, 406–415. doi: 10.1177/0890334415585078
Jost, T., Lacroix, C., Braegger, C., and Chassard, C. (2013). Assessment of bacterial diversity in breast milk using culture-dependent and culture-independent approaches. Br. J. Nutr. 110, 1253–1262. doi: 10.1017/S0007114513000597
Jost, T., Lacroix, C., Braegger, C. P., Rochat, F., and Chassard, C. (2014). Vertical mother-neonate transfer of maternal gut bacteria via breastfeeding. Environ. Microbiol. 16, 2891–2904. doi: 10.1111/1462-2920.12238
Kalliomäki, M., Collado, M. C., Salminen, S., and Isolauri, E. (2008). Early differences in fecal microbiota composition in children may predict overweight. Am. J. Clin. Nutr. 87, 534–538. doi: 10.1093/ajcn/87.3.534
Khodayar-Pardo, P., Mira-Pascual, L., Collado, M. C., and Martínez-Costa, C. (2014). Impact of lactation stage, gestational age and mode of delivery on breast milk microbiota. J. Perinatol. 34, 599–605. doi: 10.1038/jp.2014.47
Koliada, A., Syzenko, G., Moseiko, V., Budovska, L., Puchkov, K., Perederiy, V., et al. (2017). Association between body mass index and Firmicutes/Bacteroidetes ratio in an adult Ukrainian population. BMC Microbiol. 17:120. doi: 10.1186/s12866-017-1027-1
Koren, O., Goodrich, J. K., Cullender, T. C., Spor, A., Laitinen, K., Bäckhed, H. K., et al. (2012). Host remodeling of the gut microbiome and metabolic changes during pregnancy. Cell 150, 470–480. doi: 10.1016/j.cell.2012.07.008
Kumar, H., Du Toit, E., Kulkarni, A., Aakko, J., Linderborg, K. M., Zhang, Y., et al. (2016). Distinct patterns in human milk microbiota and fatty acid profiles across specific geographic locations. Front. Microbiol. 7:1619. doi: 10.3389/fmicb.2016.01619
Li, S. W., Watanabe, K., Hsu, C. C., Chao, S. H., Yang, Z. H., Lin, Y. J., et al. (2017). Bacterial composition and diversity in breast milk samples from mothers living in Taiwan and Mainland China. Front. Microbiol. 8:965. doi: 10.3389/fmicb.2017.00965
Locascio, R. G., Ninonuevo, M. R., Freeman, S. L., Sela, D. A., Grimm, R., Lebrilla, C. B., et al. (2007). Glycoprofiling of bifidobacterial consumption of human milk oligosaccharides demonstrates strain specific, preferential consumption of small chain glycans secreted in early human lactation. J. Agric. Food Chem. 55, 8914–8919. doi: 10.1021/jf0710480
López-López, A., López-Sabater, M. C., Campoy-Folgoso, C., Rivero-Urgell, M., and Castellote-Bargalló, A. I. (2002). Fatty acid and sn-2 fatty acid composition in human milk from Granada (Spain) and in infant formulas. Eur. J. Clin. Nutr. 56, 1242–1254. doi: 10.1038/sj.ejcn.1601470
Lundgren, S. N. (2019). Influences of Maternal Diet and the Human Milk Microbiome on the Developing Infant Gut Microbiome. Doctoral Dissertation, Hanover, NH: Dartmouth College.
Martín, R., Heilig, H. G., Zoetendal, E. G., Jiménez, E., Fernández, L., Smidt, H., et al. (2007). Cultivation-independent assessment of the bacterial diversity of breast milk among healthy women. Res. Microbiol. 158, 31–37. doi: 10.1016/j.resmic.2006.11.004
Martín, R., Jiménez, E., Heilig, H., Fernández, L., Marín, M. L., Zoetendal, E. G., et al. (2009). Isolation of bifidobacteria from breast milk and assessment of the bifidobacterial population by PCR-denaturing gradient gel electrophoresis and quantitative real-time PCR. Appl. Environ. Microbiol. 75, 965–969. doi: 10.1128/AEM.02063-08
Mazmanian, S. K., Liu, C. H., Tzianabos, A. O., and Kasper, D. L. (2005). An immunomodulatory molecule of symbiotic bacteria directs maturation of the host immune system. Cell 122, 107–118. doi: 10.1016/j.cell.2005.05.007
Mcdonald, D., Price, M. N., Goodrich, J., Nawrocki, E. P., Desantis, T. Z., Probst, A., et al. (2012). An improved Greengenes taxonomy with explicit ranks for ecological and evolutionary analyses of bacteria and archaea. ISME J. 6, 610–618. doi: 10.1038/ismej.2011.139
Meehan, C. L., Lackey, K. A., Hagen, E. H., Williams, J. E., Roulette, J., Helfrecht, C., et al. (2018). Social networks, cooperative breeding, and the human milk microbiome. Am. J. Hum. Biol. 30:e23131. doi: 10.1002/ajhb.23131
Meyer, K. M., Pace, R. M., Mohammad, M., Haymond, M., and Aagaard, K. M. (2019). 941: composition of the breast milk microbiome is influenced by the method of 16S-amplicon sequencing used. Am. J. Obs. Gynecol. 220, S607–S608.
Moossavi, S., Atakora, F., Miliku, K., Sepehri, S., Robertson, B., Duan, Q. L., et al. (2019a). Integrated analysis of human milk microbiota with oligosaccharides and fatty acids in the CHILD Cohort. Front. Nutr. 6:58. doi: 10.3389/fnut.2019.00058
Moossavi, S., Sepehri, S., Robertson, B., Bode, L., Goruk, S., Field, C. J., et al. (2019b). Composition and variation of the human milk microbiota are influenced by maternal and early-life factors. Cell Host Microb. 25, 324–335. doi: 10.1016/j.chom.2019.01.011
Mueller, N. T., Shin, H., Pizoni, A., Werlang, I. C., Matte, U., Goldani, M. Z., et al. (2016). Birth mode-dependent association between pre-pregnancy maternal weight status and the neonatal intestinal microbiome. Sci. Rep. 6:23133. doi: 10.1038/srep23133
Murphy, K., Curley, D., O’callaghan, T. F., O’shea, C. A., Dempsey, E. M., O’toole, P. W., et al. (2017). The Composition of human milk and infant faecal microbiota over the first three months of life: a pilot study. Sci. Rep. 7:40597. doi: 10.1038/srep40597
Newton, R. J., Mclellan, S. L., Dila, D. K., Vineis, J. H., Morrison, H. G., Eren, A. M., et al. (2015). Sewage reflects the microbiomes of human populations. mBio 6:e02574. doi: 10.1128/mBio.02574-14
Ojo-Okunola, A., Claassen-Weitz, S., Mwaikono, K. S., Gardner-Lubbe, S., Stein, D. J., Zar, H. J., et al. (2019). Influence of socio-economic and psychosocial profiles on the human breast milk bacteriome of South African Women. Nutrients 11:1390. doi: 10.3390/nu11061390
Oksanen, J., Blanchet, F. G., Kindt, R., Legendre, P., Minchin, P. R., O’hara, R., et al. (2015). Package ‘Vegan’. Community ecology Package, Version, 2.2–1.
Padilha, M., Danneskiold-Samsøe, N. B., Brejnrod, A., Hoffmann, C., Cabral, V. P., Iaucci, J. D. M., et al. (2019a). The human milk microbiota is modulated by maternal diet. Microorganisms 7, 502. doi: 10.3390/microorganisms7110502
Padilha, M., Iaucci, J., Cabral, V. P., Diniz, E., Taddei, C. R., and Saad, S. M. I. (2019b). Maternal antibiotic prophylaxis affects Bifidobacterium spp. counts in the human milk, during the first week after delivery. Benef. Microb. 10, 155–163. doi: 10.3920/BM2018.0046
Pannaraj, P. S., Li, F., Cerini, C., Bender, J. M., Yang, S., Rollie, A., et al. (2017). Association between breast milk bacterial communities and establishment and development of the infant gut microbiome. JAMA Pediatr. 171, 647–654. doi: 10.1001/jamapediatrics.2017.0378
Pietiläinen, K. H., Kaprio, J., Rissanen, A., Winter, T., Rimpelä, A., Viken, R., et al. (1999). Distribution and heritability of BMI in Finnish adolescents aged 16 y and 17 y: a study of 4884 twins and 2509 singletons. Int. J. Obes. 23:107. doi: 10.1038/sj.ijo.0800767
Price, M. N., Dehal, P. S., and Arkin, A. P. (2010). FastTree 2–approximately maximum-likelihood trees for large alignments. PLoS One 5:e9490. doi: 10.1371/journal.pone.0009490
R Core Team (2016). R: A Language and Environment for Statistical Computing. Vienna: Foundation for Statistical Computing.
Ramani, S., Stewart, C. J., Laucirica, D. R., Ajami, N. J., Robertson, B., Autran, C. A., et al. (2018). Human milk oligosaccharides, milk microbiome and infant gut microbiome modulate neonatal rotavirus infection. Nat. Commun. 9:5010. doi: 10.1038/s41467-018-07476-4
Rognes, T., Flouri, T., Nichols, B., Quince, C., and Mahe, F. (2016). VSEARCH: a versatile open source tool for metagenomics. PeerJ 4:e2584. doi: 10.7717/peerj.2584
Sakwinska, O., Moine, D., Delley, M., Combremont, S., Rezzonico, E., Descombes, P., et al. (2016). Microbiota in breast milk of chinese lactating mothers. PLoS One 11:e0160856. doi: 10.1371/journal.pone.0160856
Santacruz, A., Collado, M. C., García-Valdés, L., Segura, M. T., Martín-Lagos, J. A., Anjos, T., et al. (2010). Gut microbiota composition is associated with body weight, weight gain and biochemical parameters in pregnant women. Br. J. Nutr. 104, 83–92. doi: 10.1017/S0007114510000176
Simpson, M. R., Avershina, E., Storrø, O., Johnsen, R., Rudi, K., and Øien, T. (2018). Breastfeeding-associated microbiota in human milk following supplementation with Lactobacillus rhamnosus GG, Lactobacillus acidophilus La-5, and Bifidobacterium animalis ssp. lactis Bb-12. J. Dairy Sci. 101, 889–899. doi: 10.3168/jds.2017-13411
Solis, G., De Los Reyes-Gavilan, C. G., Fernández, N., Margolles, A., and Gueimonde, M. (2010). Establishment and development of lactic acid bacteria and bifidobacteria microbiota in breast-milk and the infant gut. Anaerobe 16, 307–310. doi: 10.1016/j.anaerobe.2010.02.004
Urbaniak, C., Angelini, M., Gloor, G. B., and Reid, G. (2016). Human milk microbiota profiles in relation to birthing method, gestation and infant gender. Microbiome 4:1. doi: 10.1186/s40168-015-0145-y
Ward, T. L., Hosid, S., Ioshikhes, I., and Altosaar, I. (2013). Human milk metagenome: a functional capacity analysis. BMC Microbiol. 13:116. doi: 10.1186/1471-2180-13-116
Werner, J. J., Koren, O., Hugenholtz, P., Desantis, T. Z., Walters, W. A., Caporaso, J. G., et al. (2012). Impact of training sets on classification of high-throughput bacterial 16s rRNA gene surveys. ISME J. 6, 94–103. doi: 10.1038/ismej.2011.82
Keywords: human milk, microbiome, BMI, gestational weight gain, 16S rRNA gene sequencing
Citation: Lundgren SN, Madan JC, Karagas MR, Morrison HG, Hoen AG and Christensen BC (2019) Microbial Communities in Human Milk Relate to Measures of Maternal Weight. Front. Microbiol. 10:2886. doi: 10.3389/fmicb.2019.02886
Received: 17 September 2019; Accepted: 29 November 2019;
Published: 20 December 2019.
Edited by:
Maria Carmen Collado, Institute of Agrochemistry and Food Technology (IATA), SpainReviewed by:
Carlos Gómez-Gallego, University of Eastern Finland, FinlandHein Min Tun, The University of Hong Kong, Hong Kong
Copyright © 2019 Lundgren, Madan, Karagas, Morrison, Hoen and Christensen. This is an open-access article distributed under the terms of the Creative Commons Attribution License (CC BY). The use, distribution or reproduction in other forums is permitted, provided the original author(s) and the copyright owner(s) are credited and that the original publication in this journal is cited, in accordance with accepted academic practice. No use, distribution or reproduction is permitted which does not comply with these terms.
*Correspondence: Brock C. Christensen, QnJvY2suQy5DaHJpc3RlbnNlbkBkYXJ0bW91dGguZWR1