- 1Center for Conservation and Research of Endangered Wildlife, Cincinnati Zoo & Botanical Garden, Cincinnati, OH, United States
- 2Department of Medicine, School of Medicine, Stanford University, Stanford, CA, United States
- 3Department of Microbiology and Immunology, School of Medicine, Stanford University, Stanford, CA, United States
- 4Division of Pathology and Laboratory Medicine, Cincinnati Children’s Hospital Medical Center, Cincinnati, OH, United States
- 5Infectious Diseases Section, VA Palo Alto Health Care System, Palo Alto, CA, United States
- 6Division of Biostatistics and Epidemiology, Cincinnati Children’s Hospital Medical Center, Cincinnati, OH, United States
- 7Department of Pediatrics, College of Medicine, University of Cincinnati, Cincinnati, OH, United States
Iron overload disorder (IOD) affects many wildlife species cared for ex situ. Two of the four rhinoceros species in human care, Sumatran rhinoceros (Dicerorhinus sumatrensis) and black rhinoceros (Diceros bicornis), are susceptible, whereas the other two, white rhinoceros (Ceratotherium simum) and greater one-horned (GOH) rhinoceros (Rhinoceros unicornis), are relatively resistant to IOD. Complex interrelationships exist between mammalian hosts, their indigenous gut microbiota, metabolome, physical condition, and iron availability. The goal of this study was to gain insight into these relationships within the family Rhinocerotidae. Specific objectives were to (1) characterize the gut microbiome and metabolome of four rhinoceros species; (2) compare the microbiome and metabolome of IOD-susceptible and IOD-resistant rhinoceros species; and (3) identify variation in the microbiome and metabolome associated with compromised health or disease in IOD-susceptible rhinoceroses. Fecal samples were collected from 31 rhinoceroses (Sumatran rhinoceros, n = 3; black rhinoceros, n = 6; GOH rhinoceros, n = 9; white rhinoceros, n = 13) located at five facilities, and matched fecal aliquots were processed for microbiome and metabolome analyses using 16S rRNA gene sequencing and nuclear magnetic resonance spectroscopy, respectively. Despite the phylogenetic disparity and dissimilar zoo diets of the hosts, the structure of the fecal microbiota of the two IOD-susceptible rhinoceros species were more closely related to each other than to those of the two IOD-resistant species (Bray–Curtis dissimilarity; IOD-susceptible vs. IOD-resistant p-value < 0.001). In addition, IOD-susceptible rhinoceroses exhibited less microbial diversity than their IOD-resistant relatives (Shannon diversity; p-value < 0.001) which could have health implications. Of note, the black rhinoceros was distinct among the four rhinoceros species with the most divergent fecal metabolome; interestingly, it contained higher concentrations of short chain fatty acids. Neither age nor sex were associated with differences in microbial community composition (p = 0.253 and 0.488, respectively) or fecal metabolomic profile (p = 0.634 and 0.332, respectively). Differences in the distal gut microbiomes between IOD-resistant and IOD-susceptible rhinoceroses support hypotheses that gut microbes play a role in host iron acquisition, and further studies and experiments to test these hypotheses are warranted.
Introduction
Iron overload disease has been identified in a wide range of wildlife species maintained ex situ including birds, tapirs, primates, bats, dolphins, pinnipeds, rodents, rhinoceroses, and lagomorphs (reviewed by Clauss and Paglia, 2012). In the rhinoceros, the term iron overload disorder (IOD) was adopted in 2012 to describe the condition of excess body iron identified post-mortem in the form of excessive organ tissue hemosiderosis in this taxon, and to catalyze efforts to better understand the frequency of progression to disease-states and its role in predisposing individuals to unrelated diseases (Dennis et al., 2012). IOD affects two of the four rhinoceros species maintained in zoos, the black rhinoceros (Diceros bicornis) (Olias et al., 2012; Paglia and Tsu, 2012) and the Sumatran rhinoceros (Dicerorhinus sumatrensis) (Paglia and Tsu, 2012; Roth et al., 2017). Both species are browsers in the wild with diets consisting primarily of shrubs, bushes, leaves, twigs, tree branches, and bark (Hall-Martin et al., 1982; Van Strien, 1986). In contrast, wild white rhinoceros (Ceratotherium simum) and greater one-horned (GOH) rhinoceros (Rhinoceros unicornis) are grazers with grasses making up 100% and over 85% of their diets, respectively (Shrader et al., 2006; Hazarika and Saikia, 2012), and IOD is only rarely reported in these species (Ball and Mueller, 2011; Olias et al., 2012). Because the food of browsers is more difficult to mimic in zoos than that of grazers, suboptimal browser diets have always been suspected to be responsible for IOD in the two IOD-susceptible rhinoceros species. For certain mammalian species, there is some evidence that maintaining a low iron diet offers a practical way to prevent disease progression (Clauss and Paglia, 2012). However, if caretakers follow recommended nutritional guidelines, rhinoceros diets already are inherently low in bioavailable iron so there is limited ability for further reduction. Moreover, dietary iron may not be the primary cause of disease progression. The physiological sequelae resulting from dietary and life-style differences experienced by zoo animals vs. those in the wild may also lead to IOD (Figure 1). For example, black rhinoceroses maintained in zoos have elevated biomarkers of inflammation and decreased insulin sensitivity relative to wild counterparts, suggesting metabolic disturbance (Schook et al., 2015). The analogous condition in obese humans is associated with hyperferritinemia, metabolic changes and even hemosiderosis in some cases (Aigner et al., 2015; Datz et al., 2017).
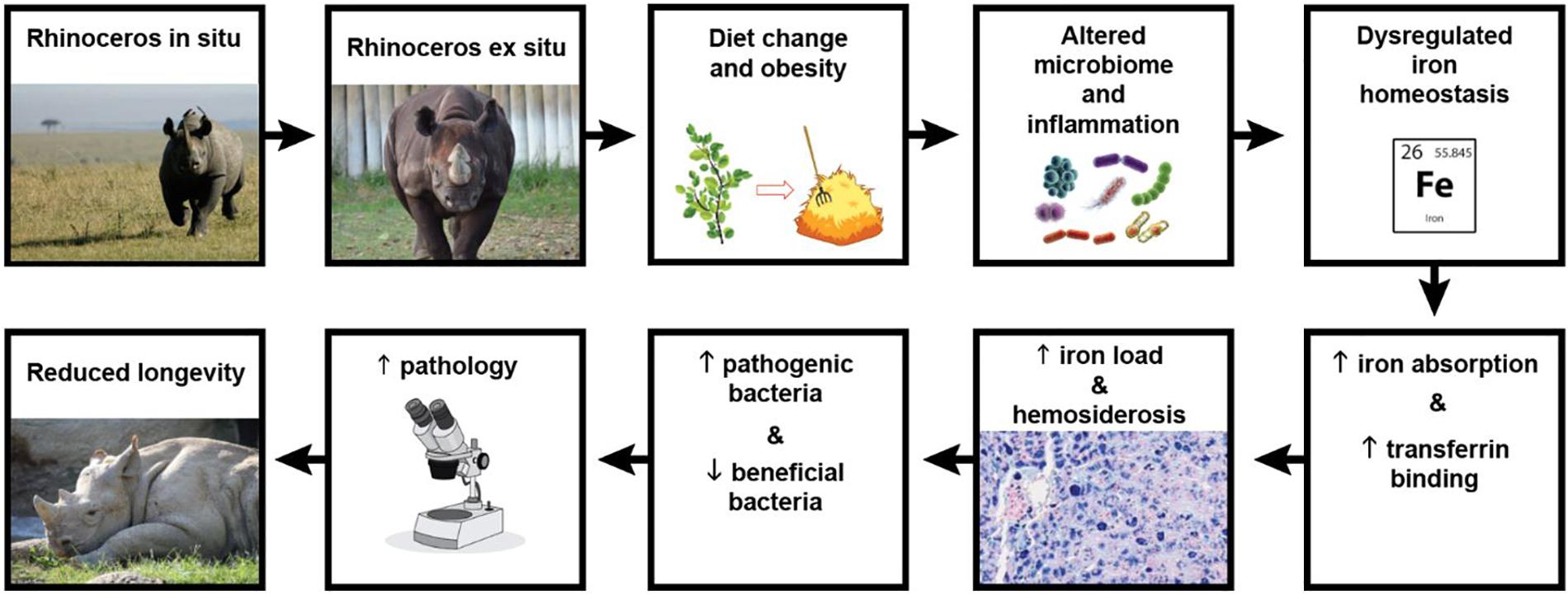
Figure 1. Schematic of the study’s suggested hypothetical model. When black rhinoceroses are captured, their diet changes from browse to hay, altering their natural microbiome and subsequent nutrient absorption. Additionally, the high nutrient diet and lack of exercise lead to over conditioning, obesity, inflammation, and metabolic disturbance which results in dysregulated iron homeostasis. Transferrin binding sites become saturated with iron which is also increasingly stored in organ tissues (hemosiderosis). Microbial competition in an iron-rich environment alters gut colonization in favor of pathogenic lineages, while more beneficial populations decrease. The resulting microbial and metabolic disturbances lead to greater disease susceptibility, health syndromes and pathologies, ultimately reducing the population’s median longevity. (Photo credits following the flow of the diagram: Renee Fulconis, Cincinnati Zoo & Botanical Garden; Hailee Butler, Cincinnati Zoo & Botanical Garden.)
High iron stores are thought to be associated with heightened infectious disease susceptibility in black rhinoceroses, however, the mechanism remains obscure (Dennis et al., 2012). Although iron stores in the form of hemosiderosis are noted in many tissues of most black rhinoceroses at necropsy (Olias et al., 2012; Paglia and Tsu, 2012), hemochromatosis is seldom reported as the cause of death in this species (Dennis et al., 2007; Olias et al., 2012; Roth et al., 2019). A compilation of United States zoo mortality data over a 16-year period ending in 2007, revealed that 22% of black rhinoceros deaths were due to infectious disease including salmonellosis and tuberculosis (Roth et al., 2009), both of which are more severe in an environment with increased iron availability (Gobin and Horwitz, 1996; Kortman et al., 2012). Over the past decade, 18% of reported black rhinoceros deaths were due to infectious or liver disease (Roth et al., 2019). Both contribute to low median longevity (19 years) experienced by this species when compared to the GOH rhinoceros and white rhinoceros (>30 years) (Survival Statistics Library, 2019). In contrast, gastrointestinal tract disease is more prevalent in white rhinoceroses, and infectious disease is rare (Roth et al., 2019).
To advance our understanding of IOD in the rhinoceros, it is important to clarify the complex relationship between the host, its indigenous microbial populations and iron availability. The mammalian gut is colonized at birth by bacteria which play an integral role in host nutrition, metabolic regulation and immunology (reviewed in Gerritsen et al., 2011). Most of these bacteria require iron for survival, function and proliferation (Andrews et al., 2003). To maintain the delicate balance of a healthy gut microbiota without excessive pathogen proliferation, the body limits the availability of iron to microbes by ensuring free iron is rapidly and tightly bound by host proteins. The gut microbiota competes for iron, and therefore iron availability directly affects population structure (Tompkins et al., 2001; Werner et al., 2011; Dostal et al., 2014). Iron also can enhance microbial virulence (Kortman et al., 2012). In contrast, iron-independent taxa are largely beneficial barrier microbes like those found in probiotics (Weinberg, 1997; Morelli, 2000; Madden and Hunter, 2002) and are out-competed in an iron-rich environment. These interdependencies explain why iron supplementation during an infection often exacerbates the problem (Griffiths, 1999; Zimmermann et al., 2010). In fact, there have been several studies on humans and mice linking the effects of iron overload, iron supplementation and iron insufficiency on the gut microbiome to the physiological health of the individual (Kelly et al., 1987; Tompkins et al., 2001; Zimmermann et al., 2010).
It is unknown if there are changes in the microbiota associated with IOD in rhinoceroses, and if so, whether these changes are a contributor to, or sequelum of, the disease. Since the gut microbiota of rodents and humans has been shown to affect absorption of nutrients, including iron (Reddy et al., 1972; González et al., 2017) and iron-related proteins (Deschemin et al., 2016), it is possible that dysbiosis could directly affect iron availability. Alternatively, microbial community shifts could help explain IOD etiology if they are characterized by the proliferation of pathogens, or loss of beneficial taxa.
The gut microbiota produces metabolites which also play a vital role in organismal health (Flint et al., 2015). For example, short chain fatty acids (SCFAs) produced by microbes from non-digestible carbohydrates are believed to have anti-inflammatory and anti-carcinogenic activity (Hosseini et al., 2011; Vinolo et al., 2011). They also tend to be elevated in the fecal metabolome of obese humans (Patil et al., 2012; Ríos-Covián et al., 2016). It has already been demonstrated that the serum metabolic profile of Sumatran rhinoceroses with symptomatic hemochromatosis differs from that of healthy individuals (Watanabe et al., 2016). However, it is not known if such changes occur as a precursor to or an effect of illness. Serum metabolites may be largely host-generated, whereas the by-products of microbial populations in the gut are better represented in the fecal metabolome (Karu et al., 2018). It is therefore of interest to characterize the fecal metabolome of rhinoceroses to determine if the byproducts of the gut microbiome change in association with IOD.
To shed some light on these complex relationships, the goal of this study was to compare the gut microbiome and metabolome of IOD-susceptible and IOD-resistant rhinoceroses cared for in zoos. The hypothesis was that the microbiome and metabolome of the two IOD-susceptible rhinoceros species would differ from those of the two IOD-resistant rhinoceros species. Specific objectives were to: (1) characterize the gut microbiome and metabolome of four rhinoceros species, (2) compare the microbiome and metabolome of IOD-susceptible to IOD-resistant rhinoceros species, and (3) identify microbiota and metabolomic differences associated with compromised health or disease in IOD-susceptible rhinoceroses.
Materials and Methods
Ethics Statement
The Institutional Animal Care and Use Committee of the Cincinnati Zoo & Botanical Garden deemed that, in accordance with the United States Animal Welfare Act, the Public Health Service Policy on Humane Care and Use of Laboratory Animals, and the Office of Laboratory Animal Welfare, this study did not require review and approval. All rhino fecal samples were collected non-invasively from the ground during routine animal husbandry procedures. No animal activities were involved.
Sample Collections
The targeted sample size for this study was six fecal samples from each rhinoceros species, and within each species, a minimum of three males and three females were to be included, except Sumatran rhinoceroses since only three individuals were available in the United States. All five collaborating facilities maintained their rhinos under intensively managed conditions during the sampling period. Animal care staff provided the daily diets and water, visually examined each rhino for health and/or injury, cleaned the enclosures and monitored environmental conditions both indoors and outdoors, giving rhinoceroses access to these habitats according to standard husbandry protocols. Staff at the holding facilities were asked to sample from fresh fecal boluses that were either found in individual rhino enclosures during the morning cleaning routine or collected shortly after an individual rhino was observed defecating. Boluses were broken open and sampled from the middle using a sterile scoop to avoid any environmental contamination. Fecal samples were only to be collected from clinically healthy rhinoceroses that were not being treated with any medication.
Fecal samples were collected from black rhinoceroses (n = 6; three female and three male), white rhinoceroses (n = 13; eight female and five male), GOH rhinoceroses (n = 9; five female and four male), and Sumatran rhinoceroses (n = 3; two male and one female). Rhinoceros ages ranged from 2.5 to 39.5 years. Samples were stored frozen (−20°C) and sliced into two aliquots each exceeding 0.5 g in weight. One set of samples was processed for 16S rRNA gene sequencing and the other set of matched samples was dried by lyophilization prior to metabolomic analysis. Two samples, one each from a GOH rhinoceros and white rhinoceros, were omitted from the final analyses of the 16S rRNA sequencing data due to a high proportion of soil bacteria reads suggesting excessive soil contamination in the sample and failed amplification attempts, respectively. The final list of samples included in the statistical analyses is shown in Table 1.
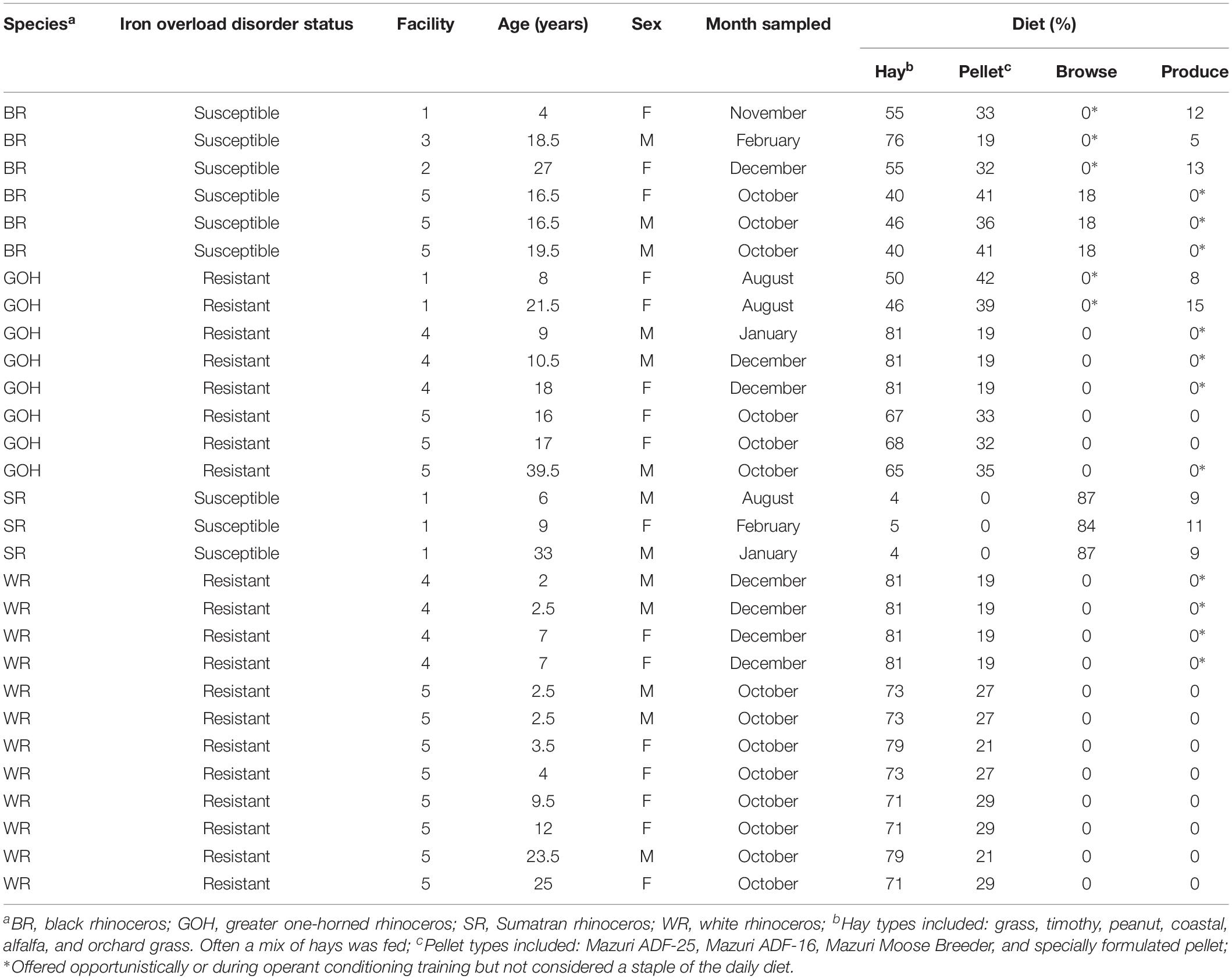
Table 1. Details about the rhinoceroses involved in the study and the general diet composition fed during the sample collection period.
DNA Extraction
Epicentre Catch-All foam swabs (Illumina, San Francisco, CA, United States) were moistened in sterile water and used to swab aliquots of each fecal sample until the swab tips were visibly stained with feces, yet free of excess debris (such as plant material in the feces). Swab tips were immediately placed into 2-ml screwcap vials (Sarstedt, Numbrecht, Germany) for DNA extraction. All specimens were extracted in one batch using the QIAamp DNA mini kit (Qiagen, Valencia, CA, United States; tissue protocol), according to instructions by the manufacturer, and eluted in 200 μl AE buffer. A tube without fecal material was processed in parallel to serve as a negative extraction control.
PCR Amplification
The V4 hypervariable region of the 16S rRNA gene was amplified by PCR using barcoded universal fusion primers 515 forward (5′-GTGYCAGCMGCCGCGGTAA-3′) and 806 reverse (5′-GGACTACNVGGGTWTCTAAT-3′). The 25-μL PCR reactions were carried out in 96-well plates and run in triplicate using 0.4 μM concentrations of each commercially synthesized primer, 2.5× Hot MasterMix (5 PRIME) and 1 μL of each DNA template. As a negative control, a PCR reaction was also carried out in parallel without DNA template. Thermal cycling conditions consisted of an initial denaturing step of 94°C for 2 min, followed by 30 cycles of 95°C for 30 s, 53°C for 30 s, and 65°C for 30 s, with a final extension step of 65°C for 8 min. Triplicates were pooled and purified using the UltraClean 96 PCR Cleanup Kit per the manufacturer’s protocol (Polz and Cavanaugh, 1998). The Quant-iT High-Sensitivity dsDNA Assay Kit (Invitrogen) was then used to quantify the DNA concentrations of each well (mean = 7.47 ng/ul, range = 0.02–12.7 ng/ul). The amplicons were then pooled in a single tube at equimolar ratios using a liquid handler. This amplicon pool was concentrated by ethanol precipitation, resuspended in 100 uL of molecular biology-grade water (Life Technologies) and gel purified and recovered using a QIAquick Gel Extraction Kit (Qiagen). The Quant-iT PicoGreen dsDNA Assay Kit (Invitrogen) and a Tapestation 2000 instrument (Agilent) were used to quantify the final DNA concentration (16 ng/uL) of the purified amplicon pool. The Agilent Bioanalyzer was used to determine the average size of the amplicon library (389 bp). PCR products and negative controls were sequenced (paired end sequencing, 2 × 250 nucleotides) on a HiSeq 2500 instrument (Illumina) at the University of Illinois, Urbana–Champaign, IL, United States.
16S rRNA Sequence Read Processing
Primer sequences were removed using cutadapt v1.16 (Martin, 2011). Read processing and error correction was performed using the open-source R package DADA2 v1.8 (Callahan et al., 2016a). Quality filtering, error modeling, dereplication, denoising, and merging of paired end reads were conducted using the default parameters. Truncation of forward and reverse reads was performed at 240 and 220 nucleotides, respectively based on visual inspection of the Illumina quality scores. Reads were removed if an Illumina quality score was less than or equal to two, the maximum expected error rate for the forward or reverse read exceeded two, or the forward or reverse read contained an ambiguous base. The sample inference function (dada) was run using the default specification and each sample processed independently precluding the calling of singleton ASVs. Forward and reverse reads were merged after error correction to form an amplicon sequence variant (ASV) table. Chimera removal was implemented using the consensus method provided by the DADA2 function removeBimeraDenovo. Taxonomic classification was performed using the DADA2 formatted RDP v16 reference files. The assignSpecies and assignTaxonomy functions were used to combine exact genus- and species-level matching with an R implementation of the RDP Naive Bayesian Classifier to facilitate optimal assignment. Sequence alignment was performed using the default settings of the AlignSeqs function in the DECIPHER package v2.8.1 (Wright, 2016). A de novo phylogenetic tree was generated using the phanghorn package v2.4 (Schliep, 2011) to first construct a neighbor-joining tree and subsequent maximum likelihood tree with the neighbor-joining tree as the starting point as recommended by Callahan et al. (2016b). Outgroup rooting was accomplished by selecting the longest individual branch. Phyloseq v1.24 (McMurdie and Holmes, 2013) was used to integrate the sample metadata, ASV table, phylogenetic tree, and taxonomic assignments for statistical analyses. A total of 65 and 111 reads and nine ASVs were retained in the negative control samples, respectively. Four of these ASVs were also observed in rhino specimens including an abundant ASV (ASV1) which was classified to the genus Ruminococcus. The other three ASVs were all Proteobacteria and classified as Escherichia (ASV2832), Acinetobacter (ASV3207), and Vampirovibrio (3986) organisms and were detected in ten, four, and six rhino samples, respectively. All four ASVs were retained in downstream analyses as we could not rule them out as true members of the rhino microbiota.
Sample Preparation for Metabolomics Analyses
All dried fecal test samples were weighed (95–162 mg) and transferred into 2 mL tubes containing 2.8 mm ceramic beads (VWR) prior to processing. On the day of processing, 1.5 mL of cold PBS was added to each tube and the samples were homogenized for 30 s at 5000 rpm using Minilys (Bertin Technologies) Samples were then transferred into 2 mL tubes without beads and centrifuged at 10,000 × gn at 4°C for 20 min. Supernatants were filtered at 12,000 × gn for 60 min at 4°C using pre-washed 3 kDa spin filters (NANOSEP 3K, Pall Life Sciences). The nuclear magnetic resonance (NMR) buffer containing 100 mM phosphate buffer in D2O, pH 7.3, and 1.0 mM TMSP (3-Trimethylsilyl 2,2,3,3-d4 propionate) was added to 540 uL of fecal filtrate. The final sample volume was 600 uL and final TMSP concentration in each sample was 0.1 mM.
NMR Spectroscopy Data
The experiments were conducted using 550 μL samples in 103.5 mm × 5 mm NMR tubes (Bruker Analytik, Rheinstetten, Germany). One-dimensional 1H-NOESY NMR spectra were acquired on a Bruker Avance II 600 MHz spectrometer. All data were collected at a calibrated temperature of 298K using the noesygppr1d pulse sequence in the Bruker pulse sequence library. Experiments were run with 4 dummy scans and 256 acquisition scans with an acquisition time of 3.4 s and a relaxation delay of 3.0 s. The NOESY mixing time was 6 ms. The spectral width was 12 ppm, and 64K real data points were collected. All free induction decays were subjected to an exponential line-broadening of 0.3 Hz. Upon Fourier transformation, each spectrum was manually phased, baseline corrected, and referenced to the internal standard TMSP at 0.0 ppm using Topspin 3.5 software (Bruker). Two-dimensional data, 1H–1H total correlation spectroscopy (TOCSY) and 1H–13C heteronuclear single quantum coherence (HSQC), were collected for metabolite assignment on representative samples.
Metabolomics Data Analysis
Metabolites were assigned by comparing the chemical shifts with reference spectra found in databases, such as the Human Metabolome Database (Wishart et al., 2018), and Chenomx® NMR Suite profiling software (Chenomx Inc., version 8.1). A total of 36 metabolites were assigned and quantified using Chenomx software based on the internal standards. Prior to statistical analysis, normalization by dry sample weights and mean-centered scaling were applied.
Statistical Analysis
Means with standard deviations, frequencies, and percentages were used to describe the rhinoceros characteristics and habitats. Proportions were used to quantify the fraction of total reads mapped to each taxonomic level from phylum to species. Heat trees as generated by the metacoder v0.3.2 package (Foster et al., 2017) were used to visualize the number of reads mapping to taxonomic levels from kingdom to genus. Stacked bar charts were used to describe the relative abundance of major bacterial phyla for each rhinoceros species. Kruskal–Wallis or Wilcoxon rank-sum tests were used to test for differences in Shannon diversity according to rhinoceros species, sex, facility and hemosiderosis risk. Spearman correlations were used to assess the correlation between Shannon diversity and age. Principal components analysis (PCA) was conducted on the raw counts after variance stabilizing transformation as implemented in DESeq2 v1.24 (Love et al., 2014). PCA was also conducted on the raw counts after a centered log-ratio transformation (Aitchison distance) and principal coordinates analysis (PCoA) performed on the Bray–Curtis dissimilarity matrix and weighted and unweighted UniFrac distances after subsampling to the lowest observed read depth (45,074 reads) to assess the robustness of the beta-diversity ordinations to choices in the normalization procedure and distance measure. Differences in beta-diversity were tested by permutational multivariate analysis of variance (PERMANOVA) as implemented by the Adonis function in vegan v2.5.5 (Oksanen et al., 2019) and differences in beta dispersion tested using the betadisper and permutest functions at the default settings. Differentially abundant ASVs in IOD-susceptible vs. IOD-resistant rhinoceros species were identified using moderated negative binomial regression as implemented by DESeq2 after filtering to include ASVs detected in at least half of all samples. Facility was included as a covariate in all models and ASVs with a Benjamini–Hochberg false discovery rate (FDR) corrected p-value of <0.1 reported. Wilcoxon rank-sum tests were performed on the subsampled taxa as a sensitivity analysis for differential abundance.
Principal components analysis was performed on the normalized metabolite concentrations to assess the clustering of stool specimens based on rhinoceros facility or species. PERMANOVA was used to test for differences in the overall metabolite concentrations. Linear regression was used to test for differences in metabolite concentrations between IOD-susceptible and IOD-resistant rhinoceros species. Rhinoceros facility was included as a covariate and metabolites with a FDR p-value of <0.1 reported. Correlations between bacterial families and fecal metabolites according to IOD susceptibility status and within the black rhinoceros species were visualized using heatmaps. ASVs were aggregated to the family level and the top 30 families seen in at least three samples in both IOD-susceptible and black rhinoceros species selected. Heatmaps were generated using the ComplexHeatmap v2.0.0 package (Gu, 2016). All analyses were conducted using R v3.6.0 (R Core Team, 2019) including the tidyverse (version: 1.2.1; Wickham, 2017), phyloseq (version: 1.28.0; McMurdie and Holmes, 2013), vegan (version: 2.5.5; Oksanen et al., 2019), microbiome (version: 1.6.0; Lahti, 2018), DESeq2 (version: 1.24.0; Love et al., 2014), ComplexHeatmap (version: 2.0.0; Gu, 2016), circlize (version: 0.4.6; Gu, 2014), Metacoder (version: 0.3.2; Foster et al., 2017), and Cowplot (version: 1.0.0; Wilke, 2019) packages.
Data Deposition
The 16S rRNA data sets generated in this study were deposited in the NCBI BioProject database under accession number PRJNA558964.
Results
Microbiota of IOD-Susceptible Rhinoceros Species Cluster Together and Are Less Diverse Than Those of IOD-Resistant Rhinoceros Species
A total of 2,303 ASVs, representing 18 phyla, were detected across all rhinoceros species. Firmicutes represented the highest relative abundance (range; 66.3–51.0%) followed by Bacteroidetes (39.8–23.4%) (Figure 2A). Also present in all species, but at much lower abundance, were Verrucomicrobia (7.6–1.9%), Spirochetes (3.1–1.1%), Actinobacteria (1.04–0.03%), and Fibrobacteres (2.14–0.19%). The mean log-ratio of Firmicutes to Bacteroidetes was 0.41 ± 0.61 for IOD-susceptible and 0.98 ± 0.47 for IOD-resistant species (p = 0.011). Although 97–98% of all reads were successfully mapped to a bacterial phylum for white, black, and GOH rhinoceroses, only 88% of Sumatran rhinoceros reads were mapped to a phyla (Supplementary Table 1A) due to a few unidentified ASVs with high read counts. Subsequent BLAST analysis against the NCBI database showed an abundant ASV had >90% coverage and 80% identity to numerous Clostridium sequences, whereas another abundant ASV was found to have <80% identity to all alignments. In general, however, the majority of ASVs mapped to the family level and above were found across rhinoceros species (Supplementary Table 1B), including those affiliated with the orders Bacteroidales and Clostridiales and the Families Ruminococcaceae, Spirochaetaceae, and Coriobacteriaceae. Heat trees showing the number of ASV mapped to taxonomic ranks are provided as Supplementary Figure 1. Despite the higher level taxonomic similarities in microbial orders and families, the presence of specific ASVs differed across rhinoceros species. Supplementary Figure 2 highlights differences in the presence/absence of ASVs for selected subsections in many parts the phylogenic tree. For example, many ASVs mapping to Coriobacteriaceae, Prevotellaceae, and Clostridium XlVa were not detected in IOD-susceptible rhinos.
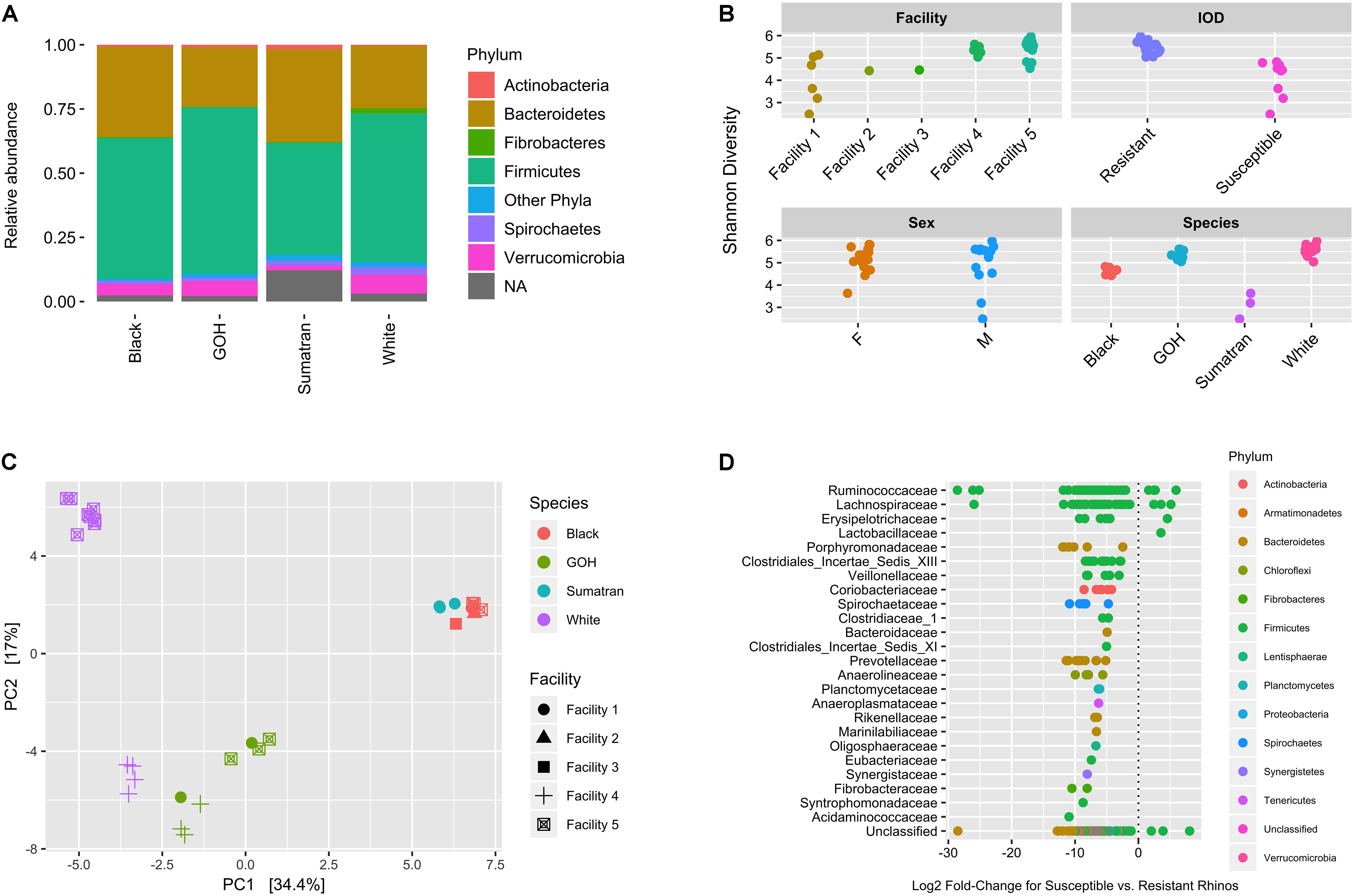
Figure 2. Microbial community composition, diversity, and differential abundance. (A) Fecal community relative abundance at the phylum level according to rhino species. (B) Shannon diversity according to facility, IOD risk, sex, and species. (C) Principal coordinates analysis performed on the Bray–Curtis dissimilarity metric. (D) Differentially abundant core ASVs in IOD susceptible vs. resistant rhinos. Log2 fold-changes shown for ASVs with a BH-FDR corrected p-value < 0.1 (356 of 446 core taxa). Each dot reflects a distinct ASV. ASVs are presented by bacterial family on the y-axis and colored by phylum. ASVs with log2 fold-change values greater than zero are more abundant in IOD-susceptible rhino species. ASVs with log2 fold-change values less than zero are more abundant in IOD-resistant rhino species.
Shannon diversity within rhinoceros fecal samples differed according to facility (p = 0.007), rhinoceros species (p < 0.001) and IOD susceptibility (p < 0.001; Figure 2B). Age was inversely associated with Shannon diversity (Spearman rho = −0.45, p = 0.015), but no difference was observed for sex (p = 0.914). PCoA performed on the Bray–Curtis dissimilarity matrix indicated clustering of samples based on rhinoceros species and facility, and IOD-susceptibility (Figure 2C). Rhinoceros species explained the greatest amount of variance (R2 = 0.49; p < 0.001) followed by facility (R2 = 0.17; p < 0.001; Supplementary Table 2), whereas age and sex were not significant (R2 = 0.02; p = 0.253 and R2 = 0.01; p = 0.488, respectively). Ordinations performed after variance stabilizing or centered log-ratio (i.e., Aitchison distance) transformation produced similar clustering (Supplementary Figure 3). Clustering based on weighted and unweighted UniFrac distances was less pronounced for IOD-susceptible, and more pronounced for IOD-resistant species, suggesting importance for the relative abundances of microbial taxa in these rhinoceros species. However, samples from GOH and White rhinoceroses generally separated from those collected from Sumatran and Black rhinoceroses along the first axis regardless of approach.
A large proportion (356/446) of abundant ASVs differed (with FDR p-values < 0.1) between IOD-susceptible and -resistant groups (Figure 2D). Almost all of these ASVs were more abundant in IOD-resistant rhinoceroses, consistent with the greater Shannon diversity in these species. In fact, with the exception of a few ASVs mapping to Firmicutes, many of these ASVs were not consistently detected or were much less abundant in IOD-susceptible rhinos. Similar results were obtained with the Wilcoxon rank-sum test (Supplementary Table 3) performed on the subsampled data (392/446 abundant ASVs with FDR p-value < 0.1) and when testing for differential abundance according to rhino species via DESeq2 (441/446 abundant ASVs with FDR p-value < 0.1; 81% of these ASVs overlapped with those detected for IOD status; Supplementary Table 4).
The Black Rhinoceros Metabolome Is Distinct Among the Rhinoceros Species Containing Higher SCFA Concentrations
A total of 36 polar metabolites were identified and quantified in the fecal samples. PCoA was used to project the relative relationships of overall metabolic profiles among samples from each of the species and facilities (Figure 3A). Both factors significantly affected the metabolic profiles (PERMANOVA p-values < 0.001). In contrast, rhinoceros age and sex were not significant (p = 0.634 and 0.332, respectively). A total of 15 out of 36 analyzed metabolites differed in abundance (with FDR corrected p-value < 0.1) between susceptible and resistant groups. These metabolites were further analyzed among host species and the results presented in boxplot format (Figure 3B) to better identify how the variance was distributed among and within the species. The black rhinoceros stood out as the primary source of variation with a higher abundance of SCFAs (2-hydroxybutyrate, butyrate, isobutyrate, isovalerate, and propionate) compared to the other three species. In general, samples from the Sumatran rhinoceroses fell in line with those from the GOH and white rhinoceroses, but were relatively impoverished for many amino acids (aspartate, glutamate, isoleucine, valine, and tyrosine). With the exception of two individuals, white rhinoceroses were unique in exhibiting a high abundance of lactate compared to the other rhinoceros species. Interestingly, the white rhinoceros data for isobutyrate and some of the amino acids (aspartate, glutamate, valine, and tyrosine) formed high and low concentration groups based on their facility origin (Facility 4 = high concentration; Facility 5 = low concentration). These metabolites were likely strong drivers of the significant facility effect in the statistical model as shown in PCoA scores plot.
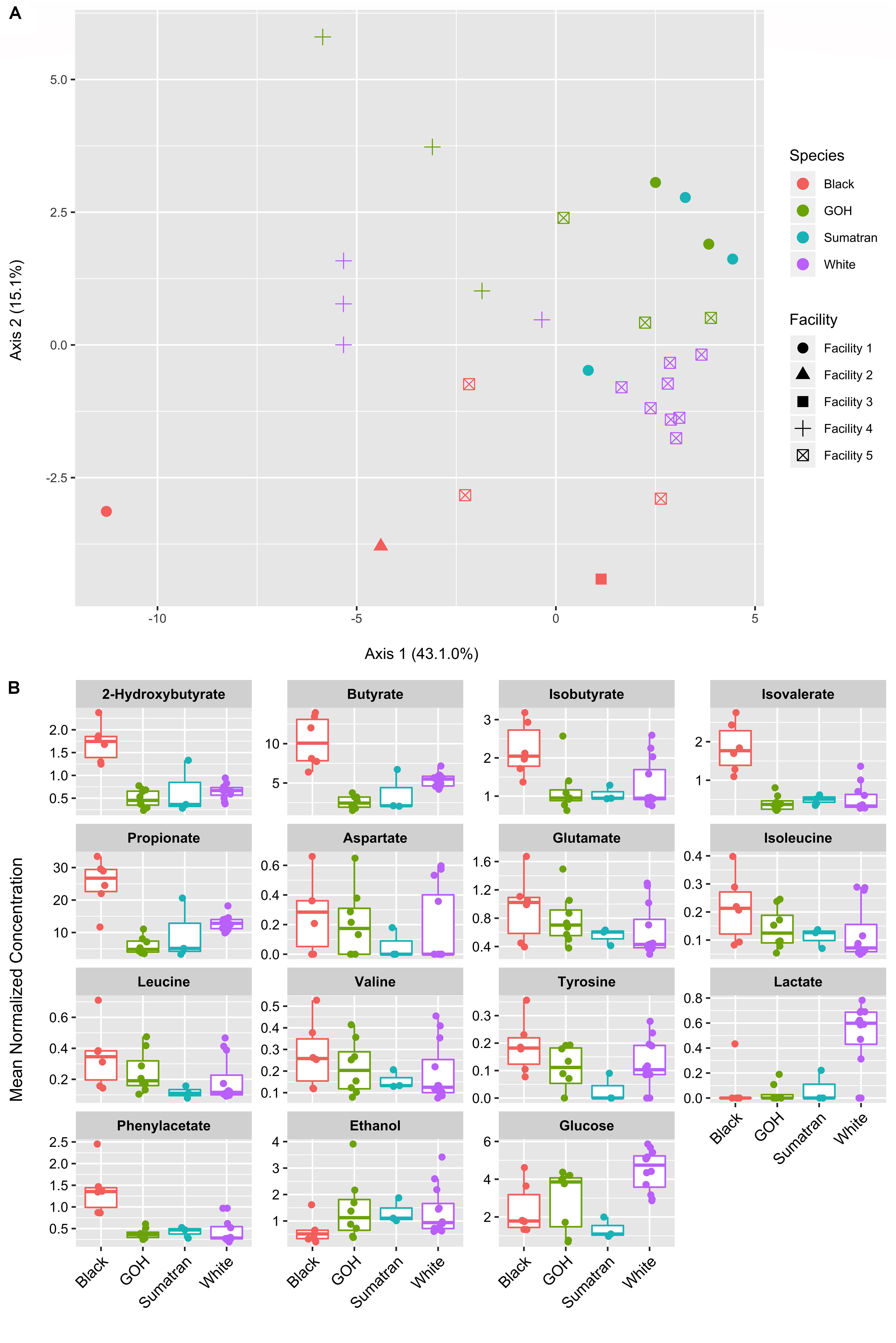
Figure 3. Fecal metabolite ordination and differential abundance. (A) Principal components analysis of 36 fecal metabolites. (B) Boxplots of differentially abundant fecal metabolites (15 of 36) according to rhino species. Plots shown where the FDR corrected p-value < 0.1 for IOD-susceptible vs. IOD-resistant rhinos.
Heatmaps displaying the Spearman correlations between fecal bacterial families and metabolites were initially developed for IOD-resistant and IOD-susceptible species separately, and correlation pattern differences were noted between these two groups (Figure 4A). However, the metabolome signature of the black rhinoceros was distinct among the four species, and combining results from the few Sumatran rhinoceros samples with those of the black rhinoceros samples obscured the extent to which black rhinoceroses differed from their counterparts. Therefore, heatmaps were produced to demonstrate the differences between black rhinoceros and the white/GOH rhinoceroses (IOD-resistant group; Figure 4B).
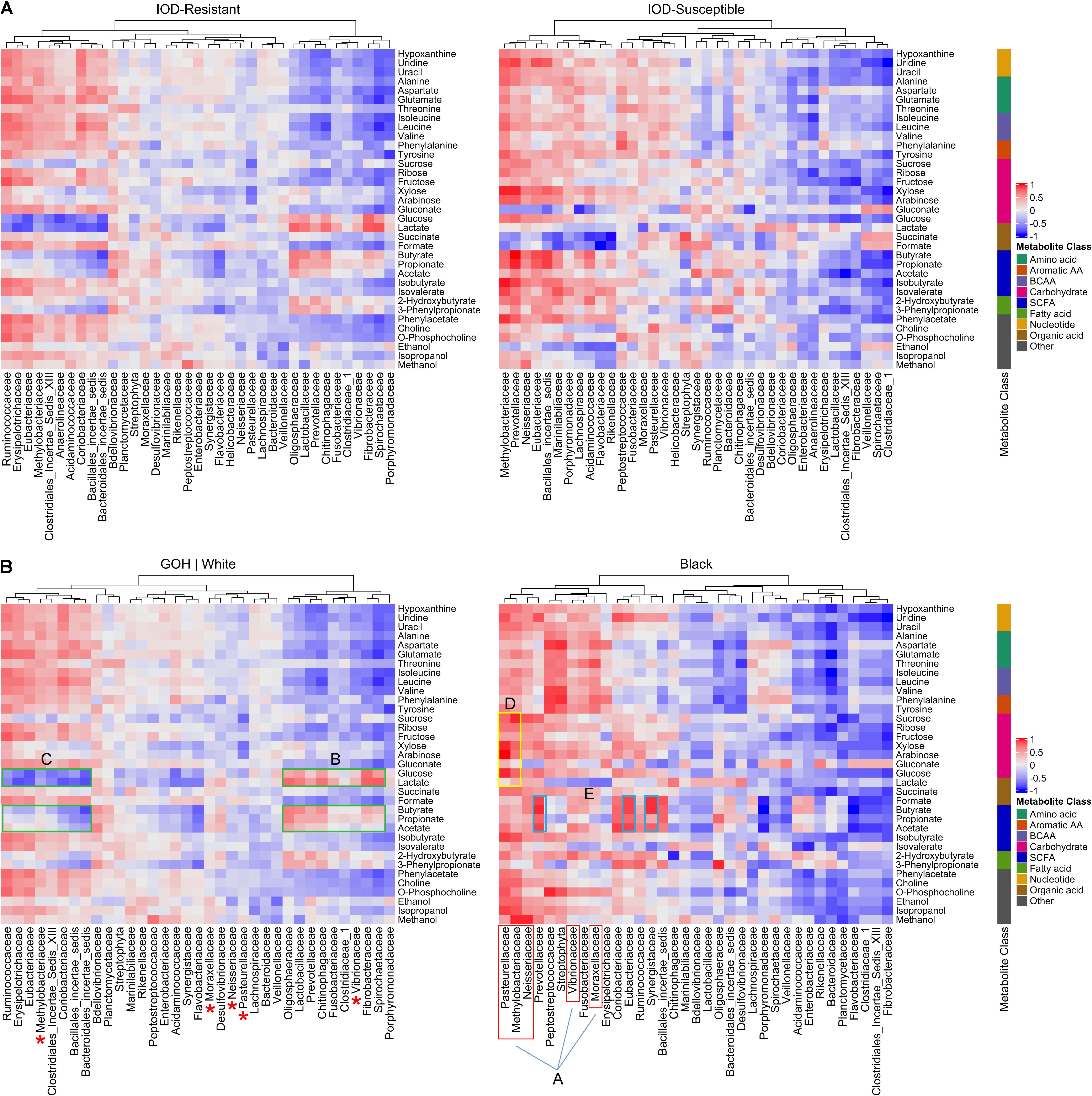
Figure 4. Heatmap showing the Spearman correlation between fecal bacterial families and metabolites according to IOD susceptibility status (A). Heatmaps exhibiting the Spearman correlation between fecal bacterial families and metabolites in IOD-susceptible black rhinoceroses and the IOD-resistant white rhinoceroses and GOH rhinoceroses (B). A-red, proteobacteria in black rhinoceroses positively correlated with most metabolites. Red asterisks indicate proteobacteria families demonstrating little to no correlation with any metabolites in IOD-resistant rhinoceroses. In IOD-resistant species: B-green, bacterial families positively correlated with glycolytic metabolites and SCFAs; C-green, families negatively correlated with sugars and SCFAs. In black rhinoceroses: D-yellow, proteobacteria exhibiting strong positive correlations with sugars; E-blue, bacterial families exhibiting the highest correlations to SCFAs.
The differences in correlations between microbial families and metabolite abundances strongly suggested that different microbial populations influenced the gut metabolomic environment in each of the rhinoceros groups. For example, several families belonging to the Proteobacteria phylum, such as Vibrionaceae, Moraxellaceae, and Neisseriaceae were positively correlated with many of the metabolites detected in black rhinoceroses (A, red box). Furthermore, Pasteurellaceae and Methylobacteriaceae exhibited strong positive correlations with all sugar metabolites (e.g., glucose, xylose, and arabinose; D, yellow box). The families exhibiting the highest correlations to SCFAs included Prevotellaceae, Eubacteriaceae, and Synergistaceae, well-known SCFA producers (Ríos-Covián et al., 2016; E, blue boxes). In contrast, many of these Proteobacteria families demonstrated little to no correlation with any metabolites in IOD-resistant rhinoceroses (red ∗).
In IOD-resistant species, strong correlation patterns revealed links between the bacterial families and metabolic processes. The bacterial families positively correlated with glycolytic metabolites (glucose and lactate), such as Bacteroidetes, Spirochaetaceae, and Fibrobacteraceae, also demonstrated positive correlations with microbial metabolites such as the SCFAs (B, green boxes). In contrast, members of Clostridiales and Firmicutes were negatively correlated with both sugars and SCFAs (C, green boxes).
Discussion
In this study, the gut microbiomes and metabolomes among four of the five extant rhinoceros species were characterized and compared, and an association was identified between gut microbiome and IOD susceptibility. Within the two distantly related, IOD-susceptible rhinoceros species, our findings demonstrated shared features of the distal gut microbiota, differences with their two IOD-resistant counterparts and divergence in metabolite profiles.
Previous large wildlife studies that included a few rhinoceros samples demonstrated that host phylogeny and diet influenced bacterial diversity, and the most abundant phyla in most mammals were Firmicutes and Bacteroidetes (Ley et al., 2008; Youngblut et al., 2019). Therefore, the dominance of these two phyla members in the gut microbiota of rhinoceroses across several North American zoos was expected and in accordance with reports of rhinoceros microbiome in China (Bian et al., 2013), Europe (Antwis et al., 2019), San Diego (Williams et al., 2019), South Africa and Texas (Gibson et al., 2019).
The Sumatran rhinoceros is the closest living relative of the wooly rhinoceros (Willerslev et al., 2009), but the analysis of a single wooly rhinoceros gut revealed very small numbers of Bacteroidetes (0.2% of reads) with Firmicutes dominating at 68% followed by Proteobacteria (19.2%) (Mardanov et al., 2012). The latter was not identified in appreciable abundance in the Sumatran rhinoceros. In contrast, Proteobacteria were more abundant in female black rhinoceroses in European zoos, particularly in two post-partem samples (Antwis et al., 2019) and in wild black rhinoceroses in South Africa (Gibson et al., 2019).
Interestingly, the two IOD-susceptible species, the Sumatran and black rhinoceroses, contained higher proportions of Bacteroidetes than the IOD-resistant GOH and white rhinoceroses. Similarly, Ley et al. (2008) reported a higher proportion of Bacteroidetes in the black rhinoceros compared to the GOH rhinoceros. Previous studies have reported a shift favoring Bacteroidetes in the Firmicutes:Bacteroidetes ratio associated with several factors including colitis (Costa et al., 2012), increased dietary fiber (De Filippo et al., 2010), and obesity (Larsen et al., 2010; Schwiertz et al., 2010). However, results are mixed regarding the latter (Ley et al., 2006; Guo et al., 2008; Turnbaugh et al., 2008; Turnbaugh and Gordon, 2009; Sze and Schloss, 2016) suggesting no strong association exists. Bacteroidetes are known for producing enzymes that degrade dietary polymers such as plant cell wall compounds which otherwise would pass through the gut undigested (Thomas et al., 2011). Given the tree branches, bark, bushes and shrubs preferred by browsers over more protein-rich grasses consumed by grazers, a shift toward greater Bacteroidetes populations may reflect the mutually beneficial relationship between the host and its gut microbiota that evolved over time.
There have been many reports of a shift in microbial populations with pathogenic microbes increasing in the presence of iron or iron supplementation (Zimmermann et al., 2010; Jaeggi et al., 2015; Manzo and Bhatt, 2015; La Carpia et al., 2016). If the same occurs in rhinoceroses susceptible to IOD in response to elevated body iron load, it could help substantiate the suspected link between IOD and increased disease susceptibility in the black rhinoceros. Unfortunately, the inability to classify a high proportion of reads to the species, genus or even family level, precluded that goal. Unclassified sequences at deeper taxonomic levels are common with 16S rRNA sequencing due to the inability to discriminate between closely related taxa over the amplicon spanning region and incomplete reference databases. Most gut microbiome research has focused on rodents and humans, but microbial populations in wildlife, and even horses, are largely uncharacterized much beyond the phyla level (Daly et al., 2001; Ley et al., 2008; Proudman et al., 2015), with the exception of a recent study (Youngblut et al., 2019). A previous paper reported 15–42 and 58–85% unclassified bacteria at the family and genus levels, respectively, across five white rhinoceroses (Bian et al., 2013), and more recent publications on rhinoceros microbiota also reported high proportions of unmapped reads (Gibson et al., 2019; Williams et al., 2019). Similarly, we report 26–52 and 62–78% unclassified reads within family and genus, respectively, across all four rhinoceros species. Therefore, it could be misleading to compare mapped microbial populations among rhinoceros species at deeper taxonomic levels since a high proportion of unclassified reads may not map to available reference sequences.
Our data demonstrated clustering of microbiotas based on host IOD-susceptibility. Despite phylogeny being one of the main drivers of gut microbiome (Ley et al., 2008; Youngblut et al., 2019), the white rhinoceros and black rhinoceros microbiotas were not closely associated. Instead, the IOD-susceptible Sumatran rhinoceros and black rhinoceros clustered together even though they are more distantly related with non-overlapping historic distributions on two different continents (Africa and Asia1). Additionally, the two IOD-resistant, grazing GOH and white rhinoceros species were more closely aligned with each other than with their respective continental counterparts. Furthermore, gut microbiota diversity is desirable for overall organismal health (Le Chatelier et al., 2013), and the Sumatran rhinoceros and black rhinoceros harbored less microbial diversity than the GOH rhinoceros and white rhinoceros. Considering that the former are browsers that habitually consume a more diversified, high fiber diet than their grazing cousins, the opposite result was anticipated. Although black rhinoceroses and Sumatran rhinoceroses are strict browsers in the wild, in a zoo setting, black rhinoceroses do consume a high proportion of hay in their daily diet, much like their grazing counterparts, whereas the captive Sumatran rhinoceros’s diet primarily consists of browse (Table 1; Dierenfeld et al., 2000). Regardless of these significant dietary differences between zoo-maintained black rhinoceroses and Sumatran rhinoceroses, their microbiota profiles suggested that something other than phylogeny and diet influences gut microbial communities within the Rhinocerotidae family. Surprisingly, a study comparing the microbiotas of wild and captive wildlife reported increased alpha diversity in zoo-managed black rhinoceroses and white rhinoceroses compared to their wild counterparts (McKenzie et al., 2017). However, the difference may be explained by location and uneven sampling size. Wild samples were collected from rhinos in a single reserve and only included one black rhinoceros, whereas captive rhino samples were from a mix of United States and European zoos and included six black rhinoceroses. In contrast, a recent study reported higher alpha diversity in wild black rhinoceroses in South Africa compared to black rhinoceroses maintained at two facilities in Texas (Gibson et al., 2019).
Although facility/location can impact the microbiota, we could not fully determine the independent contribution of facility to differences in alpha- or beta-diversity due to imbalanced species contribution across facilities and inconsistent findings. For example, Facility 4 did not contribute samples from any IOD-susceptible individuals. However, black rhinoceroses in our study came from four different facilities with varied diets (Table 1) and still clustered together with the Sumatran rhinoceroses. Similarly, the GOH rhinoceroses were housed at three facilities and they also clustered together. These data suggest that facility did not significantly influence the microbiota in these two rhinoceros species. In contrast, white rhinoceroses separated by location. Regardless, host species was the predominant factor influencing variation in microbiota structure, whereas neither age nor sex significantly affected clustering.
The gut microbiota contributes substantially to the fecal metabolome (Martin et al., 2007; Thomas et al., 2011; Flint et al., 2015), especially in herbivores that rely on their microbial populations to breakdown plant cell membranes and convert complex carbohydrates to more readily digestible compounds. Given the close relationship between the gut microbiotas of Sumatran rhinoceroses and black rhinoceroses, a similar relationship of their fecal metabolomes was expected. However, in this study, the black rhinoceros had a distinct fecal metabolome in comparison with the other three species. In contrast, the Sumatran rhinoceros metabolome was more closely associated with those of the GOH rhinoceros and white rhinoceros. Williams et al. (2019) also reported similar metabolite composition between GOH and white rhinoceroses despite differences in gut microbial composition.
Short chain fatty acids (acetate, isobutyrate, isovalerate, propionate, and butyrate) are microbial fermentation products of the large intestine (Cummings, 1984) and an important energy source for the host. Our data suggested that the black rhinoceros fecal metabolome has higher levels of SCFAs than the other three rhinoceros species. These findings are intriguing since SCFAs, especially butyrate and propionate, are implicated as important mediators of obesity and metabolic syndromes (reviewed by Ríos-Covián et al., 2016). Several studies have demonstrated that total fecal SCFA concentrations increase with obesity and its associated metabolic disturbances in humans, and decrease with anti-obesity treatment (Schwiertz et al., 2010; Patil et al., 2012; Fernandes et al., 2014; Rahat-Rozenbloom et al., 2014; Ríos-Covián et al., 2016). Schook et al. (2015) reported that black rhinoceroses in zoos exhibit elevated inflammatory markers (tumor necrosis factor alpha, serum amyloid A and insulin), similar to those documented in obese humans. They suggested that metabolic disturbance could be the primary disrupter of black rhinoceros health, and that iron storage may be a secondary response. Additionally, serum ferritin, an acute phase protein, is extraordinarily high in some black rhinoceroses that are not sick from IOD (Wojtusik and Roth, 2018). Hyperferritinemia occurs in obese humans in the presence or absence of excess body iron load, and it is now known that up to one third of non-alcoholic fatty liver disease (NAFLD) patients have elevated iron levels, a condition termed “dysmetabolic iron overload syndrome” (Aigner et al., 2015). SCFAs are essential for maintaining intestinal epithelium physiology by modulating the response to inflammatory/infectious stimuli (Corrêa-Oliveira et al., 2016). Given the previously reported evidence for unhealthy inflammatory and/or metabolic states in the black rhinoceros, it is possible the elevated fecal SCFAs reported in this study are a response to those conditions.
Despite its similarity with black rhinoceroses in gut microbiota taxa composition, the Sumatran rhinoceros produced SCFA concentrations similar to those of the GOH rhinoceros and white rhinoceros. Although both black rhinoceroses and Sumatran rhinoceroses are susceptible to IOD, Sumatran rhinoceroses have presented with classic hemochromatosis in the absence of other disease states, whereas black rhinoceroses typically die of another primary cause and hemosiderosis is noted post-mortem (Dennis et al., 2007; Olias et al., 2012; Paglia and Tsu, 2012). Furthermore, serum ferritin concentrations in healthy black rhinoceroses can be significantly higher than those measured in Sumatran rhinoceroses that die of hemochromatosis (Roth et al., 2017; Wojtusik and Roth, 2018), Not only do these observations and data reveal the inadequacy of serum ferritin as a biomarker for IOD progression in the rhinoceros, they also suggest that the iron overload condition of these two species may differ in origin, etiology and/or impact.
All but two white rhinoceroses exhibited significantly elevated lactate concentrations compared to the other three rhinoceros species. Gut lactate accumulation can be detrimental, leading to colitis in humans (Vernia et al., 1988; Hove et al., 1994); lactate levels have been used as a measure of colic severity in horses (Latson et al., 2010). Host intestinal absorption of butyrate is preferred to lactate, thus bacterial cross-feeding that reduces intestinal lactate and increases butyrate or propionate production can benefit the host (Flint et al., 2015). Given that gastrointestinal tract ailments are one of the top health concerns and causes of death in zoo-maintained white rhinoceroses (Roth et al., 2019) and are also strong indicators of poor adaptation to translocation procedures (Miller et al., 2016), further research is warranted in identifying differences among rhinoceroses in microbial populations that lead to lactate production and conversion.
Finally, fecal ethanol levels were significantly lower in the black rhinoceros compared to the other three species. An increase in ethanol production has been linked to obesity and NAFLD in humans and mice (Cope et al., 2000; Raman et al., 2013). Therefore, this finding does not support the hypothesis that black rhinoceroses are suffering from metabolic disturbances associated with obesity. However, “normal” fecal metabolite values for individual species are highly variable, so it is possible that ethanol is high compared to that in wild black rhinoceroses.
Whereas this study has many strengths, there are also limitations. First, the sample size and unequal distribution of rhinoceros species across facilities limited our ability to disentangle the effect of facility from rhinoceros species for several analyses. Second, the time of the year in which stool specimens were collected was not standardized. While we saw little systematic difference in microbial composition or diversity according to season (Supplementary Figure 4), we cannot rule out more modest effects.
In conclusion, we found intriguing differences in gut microbial community composition and diversity in IOD-susceptible vs. IOD-resistant rhinoceros species despite the phylogenetic disparity within each group. The IOD-susceptible rhinoceroses exhibited less microbial diversity, which could have health implications. These findings provide the first insight into a relationship that clearly warrants further investigation given the strong association between microbiome and IOD susceptibility in this study. Furthermore, the black rhinoceros metabolome was distinct among the four rhinoceros species, a finding congruent with evidence of metabolic disturbance in serum profiles reported in zoo-housed black rhinoceroses (Schook et al., 2015). Such metabolic changes could be associated with hemosiderosis and the high susceptibility to infectious disease not observed in any of the other rhinoceros species in human care.
Data Availability Statement
The 16S rRNA data sets generated in this study were deposited in the NCBI BioProject database under accession number PRJNA558964.
Author Contributions
TR conceived the study, acquired the samples, and drafted the manuscript. NO performed bioinformatic analyses, interpreted the data, produced figures, and contributed to the writing of the manuscript. MW-C performed all metabolomics experiments and contributed to the data interpretation, figure production, and writing of the manuscript. AS and EB conducted the microbiome experiments and contributed to the data interpretation and writing of the manuscript. DR contributed to the data interpretation, and writing and editing of the manuscript. LR-R contributed to the writing and editing of the manuscript.
Conflict of Interest
The authors declare that the research was conducted in the absence of any commercial or financial relationships that could be construed as a potential conflict of interest.
Acknowledgments
The authors thank the animal care staff at the following facilities for collaborating in this study by providing fecal samples from their rhinos: Cincinnati Zoo & Botanical Garden, Milwaukee County Zoo, Pittsburgh Zoo & PPG Aquarium, the Wilds Conservation Center, and White Oak Conservation Foundation. The authors are also grateful to Stuart Bauer (Cincinnati Children’s Hospital Medical Center), Elizabeth Donelan (CREW, Cincinnati Zoo & Botanical Garden), and Natasha Dudek (Stanford University) for their help in processing the samples for the analyses.
Supplementary Material
The Supplementary Material for this article can be found online at: https://www.frontiersin.org/articles/10.3389/fmicb.2019.02291/full#supplementary-material
Footnotes
References
Aigner, E., Weiss, G., and Datz, C. (2015). Dysregulation of iron and copper homeostasis in nonalcoholic fatty liver. World J. Hepatol. 7, 177–188. doi: 10.4254/wjh.v7.i2.177
Andrews, S. C., Robinson, A. K., and Rodríguez-Quiñones, F. (2003). Bacterial iron homeostasis. FEMS Microbiol. Rev. 27, 215–237. doi: 10.1016/s0168-6445(03)00055-x
Antwis, R. E., Edwards, K. L., Unwin, B., Walker, S. L., and Shultz, S. (2019). Rare gut microbiota associated with breeding success, hormone metabolites and ovarian cycle phase in the critically endangered eastern black rhino. Microbiome 7:27. doi: 10.1186/s40168-019-0639-0
Ball, R. L., and Mueller, S. (2011). “Hemosiderosis and clinical findings consistent with black rhino syndromes in greater one horned rhinoceros,” in Proceedings of the 2011 International Elephant and Rhino Conservation and Research Symposium, (Rotterdam), 1283–1308.
Bian, G., Ma, L., Su, Y., and Zhu, W. (2013). The microbial community in the feces of the white rhinoceros (Ceratotherium simum) as determined by barcoded pyrosequencing analysis. PLoS One 8:e70103. doi: 10.1371/journal.pone.0070103
Callahan, B. J., McMurdie, P. J., Rosen, M. J., Han, A. W., Johnson, A. J., and Holmes, S. P. (2016a). DADA2: high-resolution sample inference from Illumina amplicon data. Nat. Methods 13, 581–583. doi: 10.1038/nmeth.3869
Callahan, B. J., Sankaran, K., Fukuyama, J. A., McMurdie, P. J., and Holmes, S. P. (2016b). Bioconductor workflow for microbiome data analysis: from raw reads to community analyses. Version 2. F1000Res 5, 1492. doi: 10.12688/f1000research.8986.2
Clauss, M., and Paglia, D. E. (2012). Iron storage disorders in captive wild mammals: the comparative evidence. J. Zoo Wildl. Med. 43, S6–S18.
Cope, K., Risby, T., and Diehl, A. M. (2000). Increased gastrointestinal ethanol production in obese mice: implications for fatty liver disease pathogenesis. Gastroent 119, 1340–1347. doi: 10.1053/gast.2000.19267
Corrêa-Oliveira, R., Fachi, J. L., Vieira, A., Sato, F. T., and Vinolo, M. A. (2016). Regulation of immune cell function by short-chain fatty acids. Clin. Transl. Immunol. 5:e73. doi: 10.1038/cti.2016.17
Costa, M. C., Arroyo, L. G., Allen-Vercoe, E., Henry, S. R., Kim, P. T., Sturgeon, A., et al. (2012). Comparison of the fecal microbiota of healthy horses and horses with colitis by high throughput sequencing of the V3-V5 region of the 16S rRNA gene. PLoS One 7:e41484. doi: 10.1371/journal.pone.0041484
Cummings, J. H. (1984). Colonic absorption: the importance of short chain fatty acids in man. Scand. J. Gastroent. Suppl. 93, 89–99.
Daly, K., Stewart, C. S., Flint, H. J., and Shirazi-Beechey, S. P. (2001). Bacterial diversity within the equine large intestine as revealed by molecular analysis of cloned 16S rRNA genes. FEMS Microbiol. Ecol. 38, 141–152.
Datz, C., Müller, E., and Aigner, E. (2017). Iron overload and non-alcoholic fatty liver disease. Min. Endocrinol. 42, 173–183.
De Filippo, C., Cavalieri, D., Di Paola, M., Ramazzotti, M., Poullet, J. B., Massart, S., et al. (2010). Impact of diet in shaping gut microbiota revealed by a comparative study in children from Europe and rural Africa. Proc. Natl. Acad. Sci. U.S.A. 107, 14691–14696. doi: 10.1073/pnas.1005963107
Dennis, P., Ellis, S., Mellen, J., Lee, P., Olea-Popelka, F., Petric, A., et al. (2012). IOD in rhinos - epidemiology group report: report from the epidemiology working group of the international workshop on iron overload disorder in browsing rhinoceros (February 2011). J. Zoo Wildl. Med. 43, S114–S116.
Dennis, P. M., Funk, A., Rajala-Schultz, P. J., Blumer, E. S., Miller, R. E., Wittum, T. E., et al. (2007). A review of some of the health issues of captive black rhinoceroses (Diceros bicornis). J. Zoo Wildl. Med. 38, 509–517. doi: 10.1638/ms05-012.1
Deschemin, J. C., Noordine, M. L., Remot, A., Willemetz, A., Afif, C., Canonne-Hergaux, F., et al. (2016). The microbiota shifts the iron sensing of intestinal cells. FASEB J. 30, 252–261. doi: 10.1096/fj.15-276840
Dierenfeld, E. S., Wildman, R. E. C., and Romo, S. (2000). Feed intake, diet utilization, and composition of browses consumed by the Sumatran rhino (Dicerorhinus sumatrensis) in a North American zoo. Zoo Biol. 19, 169–180. doi: 10.1002/1098-2361(2000)19:3<169::aid-zoo1>3.0.co;2-d
Dostal, A., Baumgartner, J., Riesen, N., Chassard, C., Smuts, C. M., Zimmermann, M., et al. (2014). Effects of iron supplementation on dominant bacterial groups in the gut, faecal SCFA and gut inflammation: a randomised, placebo-controlled intervention trial in South African children. Brit. J. Nutr. 112, 547–556. doi: 10.1017/S0007114514001160
Fernandes, J., Su, W., Rahat-Rozenbloom, S., Wolever, T. M., and Comelli, E. M. (2014). Adiposity, gut microbiota and faecal short chain fatty acids are linked in adult humans. Nutr. Diabetes 4:e121. doi: 10.1038/nutd.2014.23
Flint, H. J., Duncan, S. H., Scott, K. P., and Louis, P. (2015). Links between diet, gut microbiota composition and gut metabolism. Proc. Nutr. Soc. 74, 13–22. doi: 10.1017/s0029665114001463
Foster, Z., Sharpton, T., and Grünwald, N. (2017). Metacoder: an R package for visualization and manipulation of community taxonomic diversity data. PLoS Comput. Biol. 13:e1005404. doi: 10.1371/journal.pcbi
Gerritsen, J., Smidt, H., Rijkers, G. T., and de Vos, W. M. (2011). Intestinal microbiota in human health and disease: the impact of probiotics. Genes Nutr. 6, 209–240. doi: 10.1007/s12263-011-0229-7
Gibson, K. M., Nguyen, B. N., Neumann, L. M., Miller, M., Buss, P., Daniels, S., et al. (2019). Gut microbiome differences between wild and captive black rhinoceros - implications for rhino health. Sci. Rep. 3:7570. doi: 10.1038/s41598-019-43875-3
Gobin, J., and Horwitz, M. A. (1996). Exochelins of Mycobaterium tuberculosis remove iron from human iron-binding proteins and donate iron to mycobactins in the M. tuberculosis cell wall. J. Exp. Med. 83, s1527–s1532.
González, A., Gálvez, N., Martin, J., Reyes, F., Pérez-Victoria, I., and Dominguez-Vera, J. M. (2017). Identification of the key excreted molecule by Lactobacillus fermentum related to host iron absorption. Food Chem. 228, 374–380. doi: 10.1016/j.foodchem.2017.02.008
Griffiths, E. (1999). “Iron in biological systems,” in Iron and Infection: Molecular, Physiological and Clinical Aspects, 2nd Edn, eds J. J. Bullen, and E. Griffiths, (Chester: John Wiley & Sons), 1–26.
Gu, Z. (2014). circlize implements and enhances circular visualization in R. Bioinformatics 30, 2811–2812. doi: 10.1093/bioinformatics/btu393
Gu, Z. (2016). Complex heatmaps reveal patterns and correlations in multidimensional genomic data. Bioinformatics 32, 2847–2849. doi: 10.1093/bioinformatics/btw313
Guo, X., Xia, X., Tang, R., Zhou, J., and Wang, K. (2008). Development of a real-time PCR method for firmicutes and bacteroidetes in faeces and its application to quantify intestinal population of obese and lean pigs. Lett. Appl. Microbiol. 47, 367–373. doi: 10.1111/j.1472-765X.2008.02408.x
Hall-Martin, A. J., Erasmus, T., and Botha, B. P. (1982). Seasonal variation of diet and faeces composition of black rhinoceros Diceros bicornis in the Addo Elephant National Park. Koedoe 25, 63–82.
Hazarika, B. C., and Saikia, P. K. (2012). Food habit and feeding patterns of great Indian one-horned rhinoceros (Rhinoceros unicornis) in Rajiv Gandhi Orang National Park, Assam, India. Int. Schol. Res. Net. 2012:259695. doi: 10.5402/2012/259695
Hosseini, E., Gootaert, C., Verstraete, W., and Van de Wiele, T. (2011). Propionate as a health-promoting microbial metabolite in the human gut. Nutr. Rev. 5, 245–258. doi: 10.1111/j.1753-4887.2011.00388.x
Hove, H., Nordgaard-Andersen, I., and Brobech Mosrtensen, P. (1994). Faecal DL-lactate concentration in 100 gastrointestinal patients. Scand. J. Gastroenterol. 29, 255–259. doi: 10.3109/00365529409090473
Jaeggi, T., Kortman, G. A. M., Moretti, D., Chassard, C., Holding, P., Dostal, A., et al. (2015). Iron fortification adversely affects the gut microbiome, increases pathogen abundance and induces intestinal inflammation in Kenyan infants. Gut 64, 731–742. doi: 10.1136/gutjnl-2014-307720
Karu, N., Deng, L., Slae, M., Guo, A. C., Sajed, T., Huynh, H., et al. (2018). A review on human fecal metabolomics: methods, applications and the human fecal metabolome Database. Anal. Chim. Acta 1030, 1–24. doi: 10.1016/j.aca.2018.05.031
Kelly, D. A., Price, E., Jani, B., Wright, V., Rossiter, M., and Walker-Smith, J. A. (1987). Yersinia enterocolits in iron overload. J. Pediatric Gastroenterol. Nutr. 6, 643–645. doi: 10.1097/00005176-198707000-00027
Kortman, G. A. M., Boleij, A., Swinkels, D. W., and Tjalsma, H. (2012). Iron availability increases the pathogenic potential of Salmonella typhimurium and other enteric pathogens at the intestinal epithelial interface. PLoS One 7:e29968. doi: 10.1371/journal.pone.0029968
La Carpia, F., Wojczyk, B., Rebbaa, A., Tang, A., and Hod, E. A. (2016). Chronic transfusion and iron overload modify the mouse gut microbiome. Blood 128:200.
Lahti, L. (2018). Microbiome R Package. Available at: http://microbiome.github.io/microbiome/ (accessed June 8, 2018).
Larsen, N., Vogensen, F. K., van den Berg, F. W. J., Nielson, D. S., Andreasen, A. S., Pedersen, B. K., et al. (2010). Gut microbiota in human adults with type 2 diabetes differs from non-diabetic adults. PLoS One 5:e9085. doi: 10.1371/journal.pone.0009085
Latson, K. M., Nieto, J. E., Beldomenica, P. M., and Snyder, J. R. (2010). Evaluation of peritoneal fluid lactate as a marker of intestinal ischemia in equine colic. Equine Vet. J. 37, 342–346. doi: 10.2746/0425164054529319
Le Chatelier, E., Nielsen, T., Qin, U., Prifti, E., Hildebrand, F., Falony, G., et al. (2013). Richness of the human gut microbiome correlates with metabolic markers. Nature 500, 541–546. doi: 10.1038/nature12506
Ley, R. E., Hamady, M., Lozupone, C., Turnbaugh, P., Ramey, R. R., Bircher, J. S., et al. (2008). Evolution of mammals and their gut microbes. Science 320, 1647–1651. doi: 10.1126/science.1155725
Ley, R. E., Turnbaugh, P. J., Klein, S., and Gordon, J. I. (2006). Microbial ecology: human gut microbes associated with obesity. Nature 44, 1022–1023.
Love, M. I., Huber, W., and Anders, S. (2014). Moderated estimation of fold change and dispersion for RNA-seq data with DESeq2. Genome Biol. 15:550.
Madden, J. A. J., and Hunter, J. O. (2002). A review of the role of the gut microflora in irritable bowel syndrome and the effects of probiotics. Brit. J. Nutr. 88(Suppl.1), S67–S72.
Manzo, V. E., and Bhatt, A. S. (2015). The human microbiome in hematopoiesis and hematologic disorders. Blood 126, 311–318. doi: 10.1182/blood-2015-04-574392
Mardanov, V., Bulygina, E. S., Nedoluzhko, A. V., Kadnikov, V. V., Beletskii, A. V., Tsygankova, S. V., et al. (2012). Molecular analysis of the intestinal microbiome composition of mammoth and woolly rhinoceros. Dokl. Biochem. Biophys. 445, 203–206. doi: 10.1134/S1607672912040060
Martin, F. J., Dumas, M. E., Wang, Y., Legido-Quigley, C., Yap, I. K., Tang, H., et al. (2007). A top-down systems biology view of microbiome-mammalian metabolic interactions in a mouse model. Mol. Syst. Biol. 3:112. doi: 10.1038/msb4100153
Martin, M. (2011). Cutadapt removes adapter sequences from high-throughput sequencing reads. EMBnet J. 17, 10–12.
McKenzie, V. J., Song, S. J., Delsuc, F., Prest, T. L., Oliverio, A. M., Korpita, T. M., et al. (2017). The effects of captivity on the mammalian gut microbiome. Integ. Comp. Biol. 57, 690–704. doi: 10.1093/icb/icx090
McMurdie, P. J., and Holmes, S. (2013). Phyloseq: an R package for reproducible sinteractive analysis and graphics of microbiome census data. PLoS One 8:e61217. doi: 10.1371/journal.pone.0061217
Miller, M., Kriger, M., Olea-Popelka, F., and Buss, P. (2016). A scoring system to improve decision making and outcomes in the adaptation of recently captured white rhinoceroses (Ceratotherium simum) to captivity. J. Wildl. Dis. 52, S78–S85. doi: 10.7589/52.2S.S85
Morelli, L. (2000). In vitro selection of probiotic lactobacilli: a critical appraisal. Curr. Issue Intestinal Microbiol. 1, 59–67.
Oksanen, J., Blanchet, F. G., Friendly, M., Kindt, R., Legendre, P., McGlinn, D., et al. (2019). Vegan: Community Ecology Package. R Package Version 2.5-5.
Olias, P., Mundhenk, L., Bothe, M., Ochs, A., Gruber, A. D., and Klopfleisch, R. (2012). Iron overload syndrome in the black rhinoceros (Diceros bicornis): microscopical lesions and comparison with other rhinoceros species. J. Comp. Path. 147, 542–549. doi: 10.1016/j.jcpa.2012.07.005
Paglia, D. E., and Tsu, I. H. (2012). Review of laboratory and necropsy evidence for iron storage disease acquired by browser rhinoceroses. J. Zoo Wildl. Med. 43, S92–S104.
Patil, D. P., Dhotre, D. P., Chavan, S. G., Sultan, A., Jain, D. S., Lanjekar, V. B., et al. (2012). Molecular analysis of gut microbiota in obesity among Indian individuals. J. Biosci. 37, 647–657. doi: 10.1007/s12038-012-9244-0
Polz, M. F., and Cavanaugh, C. M. (1998). Bias in template-to-product ratios in multitemplate PCR. Appl. Environ. Microbiol. 64, 3724–3730.
Proudman, C. J., Hunter, J. O., Darby, A. C., Escalona, E. E., Batty, C., and Turner, C. (2015). Characterisation of the faecal metabolome and microbiome of thoroughbred racehorses. Equine Vet. J. 47, 580–586. doi: 10.1111/evj.12324
R Core Team, (2019). R: A Language and Environment for Statistical Computing. Vienna: R Foundation for Statistical Computing.
Rahat-Rozenbloom, S., Fernandes, J., Gloor, G. B., and Wolever, T. M. (2014). Evidence for greater production of colonic short-chain fatty acids in overweight than lean humans. Int. J. Obes. 38, 1525–1531. doi: 10.1038/ijo.2014.46
Raman, M., Ahmed, I., Gillevet, P. M., Probert, C. S., Ratcliffe, N. M., Smith, S., et al. (2013). Fecal microbiome and volatile organic compound metabolome in obese humans with nonalcoholic fatty liver disease. Clin. Gastroenterol. Hepatol. 11, 868–875. doi: 10.1016/j.cgh.2013.02.015
Reddy, B. S., Pleasants, J. R., and Wostmann, B. S. (1972). Effect of intestinal microflora on iron and zinc metabolism and on activities of metalloenzymes in rats. J. Nutr. 102, 101–108.
Ríos-Covián, D., Ruas-Madiedo, P., Margolles, A., Gueimonde, M., de Los Reyes-Gavilán, C. G., and Salazar, N. (2016). Intestinal short chain fatty acids and their link with diet and human health. Front. Microbiol. 7:185. doi: 10.3389/fmicb.2016.00185
Roth, T., Metrione, L., Miller, M., Miller, E., Roca, A., Stoops, M., et al. (2019). Rhino Research Masterplan. Silver Spring, MD: Association of Zoos and Aquariums’ Rhino Advisory Group.
Roth, T., Miller, M., Dierenfeld, E., de Groot, P., Swaisgood, R., and Stoops, M. (2009). Rhino Research Masterplan. Association of Zoos and Aquariums’ Rhino Advisory Group. Cincinnati, OH: Cincinnati Zoo & Botanical Garden.
Roth, T. L., Reinhart, P. R., and Kroll, J. L. (2017). Serum ferritin concentration is not a reliable biomarker of iron overload disorder progression or hemochromatosis in the Sumatran rhinoceros (Dicerorhinus sumatrensis). J. Zoo Wildl. Med. 48, 645–658. doi: 10.1638/2017-0010.1
Schliep, K. P. (2011). Phangorn: phylogenetic analysis in R. Bioinformatics. 27, 592–593. doi: 10.1093/bioinformatics/btq706
Schook, M. W., Wildt, D. E., Raghanti, M. A., Wolfe, B. A., and Dennis, P. M. (2015). Increased inflammation and decreased insulin sensitivity indicate metabolic disturbances in zoo-managed compared to free-ranging black rhinoceros (Diceros bicornis). Gen. Comp. Endocrinol. 217, 10–19. doi: 10.1016/j.ygcen.2015.05.003
Schwiertz, A., Taras, D., Schafer, K., Beijer, S., Bos, N. A., Donus, C., et al. (2010). Microbiota and SCFA in lean and overweight healthy subjects. Obesity 18, 190–195. doi: 10.1038/oby.2009.167
Shrader, A. M., Owen-Smith, N., and Ogutu, J. O. (2006). How a mega-grazer copes with the dry season: food and nutrient intake rates by white rhinoceros in the wild. Funct. Ecol. 20, 376–384. doi: 10.1111/j.1365-2435.2006.01107.x
Survival Statistics Library, (2019). [Internet]. Chicago (IL): Lincoln Park Zoo/AZA Population Management Center; 2019 - [September 13, 2019]. Available at: https://www.aza.org/species-survival-statistics (September 13, 2019).
Sze, M. A., and Schloss, P. D. (2016). Looking for a signal in the noise: revisiting obesity and the microbiome. mBio 7:e01018-16. doi: 10.1128/mBio.01018-16
Thomas, F., Hehemann, J. H., Rebuffet, E., Czjzek, M., and Michel, G. (2011). Environmental and gut bacteroidetes: the food connection. Front. Microbiol. 2:93. doi: 10.3389/fmicb.2011.00093
Tompkins, G. R., O’Dell, N. L., Bryson, I. T., and Pennington, C. B. (2001). The effects of dietary ferric iron and iron deprivation on the bacterial composition of the mouse intestine. Curr. Microbiol. 43, 38–42. doi: 10.1007/s002840010257
Turnbaugh, P. J., Bäckhed, F., Fulton, L., and Gordon, J. I. (2008). Diet-induced obesity is linked to marked but reversible alterations in the mouse distal gut microbiome. Cell Host Microbe. 3, 213–223. doi: 10.1016/j.chom.2008.02.015
Turnbaugh, P. J., and Gordon, J. I. (2009). The core gut microbiome, energy balance and obesity. J. Physiol. 587, 4153–4158. doi: 10.1113/jphysiol.2009.174136
Van Strien, N. J. (1986). The Sumatran rhinoceros (Dicerorhinus sumatrensis (Fischer, 1814) - in the Gunung Leuser National Park, Sumatra, Indonesia: its distribution, ecology and conservation. Mammalia depicta 12. Hamburg: Parey.
Vernia, P., Caprilli, R., Latella, G., Barbetti, F., Magliocca, F. M., and Cittadini, M. (1988). Fecal lactate and ulcerative colitis. Gastroenterology 95, 1564–1568. doi: 10.1016/s0016-5085(88)80078-7
Vinolo, M. A., Rodrigues, H. G., Nachbar, R. T., and Curi, R. (2011). Regulation of inflammation by short chain fatty acids. Nutrients 3, 858–876. doi: 10.3390/nu3100858
Watanabe, M., Roth, T. L., Bauer, S. J., Lane, A., and Romick-Rosendale, L. E. (2016). Feasibility study of NMR based serum metabolomic profiling to animal health monitoring: a case study on iron storage disease in captive Sumatran rhinoceros (Dicerorhinus sumatrensis). PLoS One 11:e0156318. doi: 10.1371/journal.pone.0156318
Weinberg, E. D. (1997). The lactobacillus anomaly: total iron abstinence. Pers. Biol. Med. 40, 578–583. doi: 10.1353/pbm.1997.0072
Werner, T., Wagner, S. J., Martinez, I., Walter, J., and Chang, J. S. (2011). Depletion of luminal iron alters the gut microbiota and prevents Crohn’s disease-like ileitis. Gut 60, 325–334. doi: 10.1136/gut.2010.216929
Wilke, C. O. (2019). cowplot: Streamlined Plot Theme and Plot Annotations for ‘ggplot2’. R Package Version 1.0.0.
Willerslev, E., Thomas, M., Gilbert, P., Binladen, J., Ho, S. Y. W., Campos, P. F., et al. (2009). Analysis of complete mitochondrial genomes from extinct and extant rhinoceroses reveals lack of phylogenetic resolution. BMC Evol. Biol. 9:95. doi: 10.1186/1471-2148-9-95
Williams, C. L., Ybarra, A. R., Meredith, A. N., Durrant, B. S., and Tubbs, C. W. (2019). Gut microbiota and phytoestrogen-associated infertility in southern white rhinoceros. mBio 10:e00311-19. doi: 10.1128/mBio.00311-19
Wishart, D. S., Feunang, Y. D., Marcu, A., Guo, A. C., Liang, K., Vazquez-Fresno, R., et al. (2018). HMDB 4.0 — the human metabolome database for 2018. Nucleic Acids Res. 46, D608–D617. doi: 10.1093/nar/gkx1089
Wojtusik, J., and Roth, T. L. (2018). Investigation of factors potentially associated with serum ferritin concentrations in the black rhinoceros (Diceros bicornis) using a validated rhinoceros-specific assay. J. Zoo and Wildl. Med. 49, 297–306. doi: 10.1638/2017-0131.1
Wright, E. S. (2016). Using DECIPHER v2.0 to analyze big biological sequence data in R. R J. 8, 352–359.
Youngblut, N. D., Reischer, G. H., Walters, W., Schuster, N., Walzer, C., Stalder, G., et al. (2019). Host diet and evolutionary history explain different aspects of gut microbiome diversity among vertebrate clades. Nat. Comm. 10:2200. doi: 10.1038/s41467-019-10191-3
Keywords: black rhinoceros, Sumatran rhinoceros, rhinoceros, iron overload, microbiome, metabolome, microbial diversity, disease susceptibility
Citation: Roth TL, Switzer A, Watanabe-Chailland M, Bik EM, Relman DA, Romick-Rosendale LE and Ollberding NJ (2019) Reduced Gut Microbiome Diversity and Metabolome Differences in Rhinoceros Species at Risk for Iron Overload Disorder. Front. Microbiol. 10:2291. doi: 10.3389/fmicb.2019.02291
Received: 14 June 2019; Accepted: 19 September 2019;
Published: 04 October 2019.
Edited by:
David William Waite, The University of Auckland, New ZealandReviewed by:
Candace L. Williams, Zoological Society of San Diego, United StatesHai Li, University of Bern, Switzerland
Keylie M. Gibson, The George Washington University, United States
Copyright © 2019 Roth, Switzer, Watanabe-Chailland, Bik, Relman, Romick-Rosendale and Ollberding. This is an open-access article distributed under the terms of the Creative Commons Attribution License (CC BY). The use, distribution or reproduction in other forums is permitted, provided the original author(s) and the copyright owner(s) are credited and that the original publication in this journal is cited, in accordance with accepted academic practice. No use, distribution or reproduction is permitted which does not comply with these terms.
*Correspondence: Terri L. Roth, terri.roth@cincinnatizoo.org