- 1Genecology Research Centre and the School of Science and Engineering, University of the Sunshine Coast, Maroochydore, QLD, Australia
- 2Food Safety and Stability Group, Agriculture and Food, CSIRO, Werribee, VIC, Australia
- 3Department of Applied Sciences, Northumbria University, Newcastle upon Tyne, United Kingdom
Salmonella enterica is recognized as a major contributor of gastrointestinal illness worldwide. Concerns have been raised over the increasing prevalence of antibiotic resistant strains of Salmonella isolated from animals and food, and the role of antibiotics and other antimicrobial agents such as biocides and heavy metals in the selection and dissemination of antibiotic resistant bacteria to human hosts. In this study the antibiotic, heavy metal and disinfectant resistance genotypes and phenotypes of 19 S. enterica isolates from food-producing animals were established using whole genome sequence analysis, disc diffusion, as well as broth or agar dilution methods. This study also investigated the genomic environment of resistance genes on mobile genetic elements and chromosomal DNA. An ampicillin and streptomycin resistant S. Infantis isolate in this study harbored a β-lactamase (blaTEM–1), and two streptomycin resistance conferring genes (strA and strB) on a class 1 integron mobilized on a large conjugative plasmid. This plasmid also harbored two arsenic resistance gene cassettes. The arsenic resistance cassette, arsRCDAB, was also observed in two S. Singapore isolates with high tolerance to arsenate. A nalidixic acid resistant S. Typhimurium isolate was found to possess a mutation in gyrA resulting in amino acid change Asp87Gly and tetracycline resistant S. Typhimurium isolate was found to harbor efflux pump gene, tetA. No resistance (genotypic or phenotypic) was recorded to the disinfectants screened in this study. Taken together, results of this study showed a good correlation between predicted and measured resistances when comparing genotypic and phenotypic data, respectively. The findings of this study do not suggest resistance to clinically relevant antibiotics are widespread among Salmonella isolated from Australian food-producing animals.
Introduction
Salmonella enterica is a Gram-negative bacterial pathogen that is the cause of the foodborne illness, salmonellosis (Coburn et al., 2007). In 2011, non-typhoidal Salmonella spp. caused approximately 12,271 reported cases of gastroenteritis in Australia, the second highest causative agent of this disease after Campylobacter spp. (The OzFoodNet Working Group, 2011). Additionally, the incidences of salmonellosis in Australia has increased significantly in the past decade (Ford et al., 2016). Salmonella is most frequently transmitted through contaminated food products or by the handling of infected animals or feces in the farm environment (Hundy and Cameron, 2002; Pesciaroli et al., 2017). Outbreaks of salmonellosis are often attributed to the consumption of contaminated eggs or chicken but have also been reportedly attributed to contaminated pork, beef, and fresh produce (Delpech et al., 1998; Combs et al., 2006; Munnoch et al., 2009; Friesema et al., 2012; Moffatt et al., 2016). The food production and supply chain plays a critical role in the spread of Salmonella by connecting its environmental niches, animal and human hosts and consequently has been the focus of research and intervention strategies to reduce the bacterial load in food. Recently, the Australian and New Zealand Ministerial Forum on Food Regulation developed the “Australia’s Foodborne Illness Reduction Strategy 2018 – 2021+”1 with the vision of reducing the number of human cases of illness caused by Salmonella and Campylobacter (Australian Department of Health, 2018). A key feature of this strategy is the support of research and development on the epidemiology of salmonellosis and strategies for its prevention.
Antimicrobial resistance in Salmonella spp., including resistance to antibiotics, heavy metals and sanitizers, is a significant concern due to its relevance to human health (WHO, 2001). Antibiotics play an important role in the treatment of invasive salmonellosis and in gastrointestinal disease in immunocompromised patients. Despite increased antibiotic stewardship in Australia, antimicrobials are widely used as a treatment for animal infections, as growth promotors or for prophylactic uses (Mayrhofer et al., 2006; Lean et al., 2013). In modern food production disinfectants/sanitizers are used for controlling bacterial load in food processing facilities and on associated equipment. Antibiotic resistance (AMR) and multidrug resistance (MDR) in Salmonella spp. isolated in Australia has increased in recent decades, with MDR prevalence increasing from 3.4% in 1984 to 9.7% in 2013 (Williamson et al., 2018). Additionally, it has been suggested that exposure and adaptation to particular biocides, as well as heavy metals, could also lower the susceptibility of cells to some antibiotic compounds, a phenomenon called cross-resistance (Copitch et al., 2010; Condell et al., 2012). Disinfectant resistant strains of Salmonella have also been identified in food production facilities and retail foods presenting a significant public health concern (Long et al., 2016; Deng et al., 2017). Microflora and pathogens may also be exposed to non-essential heavy metals, such as cadmium, chromium, arsenic and mercury as a result of environmental pollution. Industrial processing and fertilizer use can lead to an accumulation of heavy compounds in the soil, air and water associated with food production facilities and the farming environment (Liu et al., 2015; Li et al., 2017). As cellular mechanisms employed by cells to expel heavy metals are similar to others responsible for antibiotic resistance (i.e., efflux pumps), resistance to heavy metals may also result in reduced susceptibility to clinically relevant antibiotics (Yu et al., 2017). Additionally, micronutrients such as copper and zinc are added to animal feed lots to increase feed efficiency and promote animal growth (Medardus et al., 2014). High concentrations of these elements can be cytotoxic to bacteria, and these compounds can upregulate the expression of stress response proteins (Lim et al., 2002). Despite this trend, only a small number of studies have analyzed underlying genetic mechanisms of disinfectant or heavy metal resistance among Salmonella strains isolated in Australia. Additionally, few studies have analyzed the genomic context of these mechanisms on mobile genetic elements such as plasmids, bacteriophage and transposons that can be disseminated to other bacterial cells via horizontal gene (Ghosh et al., 2000; Butaye et al., 2003; Bennett, 2008; Zhang et al., 2017).
Detection of resistance genotypes and mobile genetic elements (MGEs) has traditionally been conducted using PCR amplification with established primers or through DNA microarray analysis. However, these techniques have less resolution when compared to next generation sequencing technologies. Advances in whole genome sequencing (WGS) approaches and reduced costs have made WGS a viable alternative to conventional methods when used for “resistome” and/or “mobilome” identification and characterization (Alam et al., 2014; den Bakker et al., 2014; Tyson et al., 2015). WGS adds higher resolution to AMR investigation by allowing researchers to view the context in which AMR genes reside within the bacterial genome, for example on MGEs (Wilson et al., 2018). Given the importance of AMR and the role of MGEs in its dissemination, the aim of this study was to: (i) utilize phenotypic screening of AMR in Salmonella isolated in Australia to characterize resistance to antibiotics and biocides; (ii) utilize WGS and comparative genomics analyses to characterize the resistance genes present among a cohort of Salmonella isolated in Australia, and examine the location of these genes within the bacterial genome; (iii) characterize mobile genetic elements among the isolate cohort; (iv) examine the utility of WGS for predicting AMR phenotypes in Salmonella isolates. The presented study thus used traditional phenotypic susceptibility testing to determine any discrepancies between AMR genotype profile and phenotypic profiles. Findings of this study may assist in developing insights into the cellular mechanisms that are related to (or underlie) the pathogen’s survival in the presence of toxic compounds and may inform strategies to control Salmonella in food products and prevent human illness.
Materials and Methods
Salmonella Isolates Analyzed in This Study
This study evaluated 19 S. enterica strains isolated between 2001 and 2013 from Australian food production chains (Table 1). Sampling and confirmation of isolates has been previously characterized (Duffy et al., 2009, 2010). All isolates were sent to either the Microbiological Diagnostic Unit (University of Melbourne, Melbourne, VIC, Australia) or Queensland Health Scientific Services (Coopers Plains, Brisbane, QLD, Australia) for serotyping and phage−typing. Isolates represented serotypes of significance to public health, including S. Typhimurium, as well as S. Infantis and S. Singapore, which are highly prevalent within the poultry industry in Australia (Cox et al., 2002). In the case of S. Typhimurium, Phage Types 44, 135/135a, 108/9, and 170 were included as they are important to human illness in Australia (The OzFoodNet Working Group, 2010, 2011; Moffatt et al., 2017). Strains were grown on tryptic soya agar (TSA; Oxoid) for 24 h at 37°C before transfer in tryptic soy broth (Oxoid) containing 20% glycerol (v/v) for long-term storage at −80°C. Isolates were subcultured onto TSA with 16 ± 2 h incubation at 37°C prior to in vitro experimentation and DNA extraction.
Genome Sequencing, Assembly, and Phylogenic Analysis
Genomic DNA extractions were performed using the DNeasy Blood and Tissue Kit, as per the manufacturer’s instructions (Qiagen, United Kingdom). DNA quality (including measurements of protein, carbohydrate and phenol contamination) and concentration (ng/μl) was determined using a NanoDrop ND1000TM (Thermo Fisher Scientific, Australia) and agarose gel electrophoresis. DNA was standardized to 5 ng/μl before storage at −20°C. The Nextera XT Library Preparation Kit (Illumina, United States) was used, and sequencing was performed using the Illumina MiSeq platform (Illumina) by the Ramaciotti Centre for Genomics (UNSW Sydney, Australia).
Prior to assembly, FASTQ files were evaluated for quality using FastQC v0.70 (Andrews, 2010). Sequencing reads were trimmed using Trimmomatic v0.33 (Bolger et al., 2014) to remove Illumina adapters and low quality reads. The application was run on settings phred33, sliding window size of 4 bp and average quality requirement of lower than Q15. De novo assembly of trimmed paired-end libraries was performed using the open source platform Species Prediction and Diversity Estimation (SPAdes) v 3.11 (Bankevich et al., 2012) with specified k-mer sizes of 21, 33, 55, and 77 to create multiple contiguous sequences (FASTA files). Contiguous sequences of less than 200 bp or with a coverage value less of than 5× were then removed from the dataset. The FASTA files were processed through the online annotation platform Rapid Annotation using Subsystem Technology (RAST), to generate GENBANK files (Aziz et al., 2008; Overbeek et al., 2014; Brettin et al., 2015). GENBANK files were visualized and analyzed using Geneious v10.2.3 software (Biomatters Limited). Circular BLAST comparison of draft whole genome sequences was performed using an in-house instance of BLAST Ring Image Generator (BRIG) v0.95 (Alikhan et al., 2011).
Variant calling of SNPs and insertions/deletions in S. Typhimurium stains was performed using SNIPPY v2.52, by mapping the DNA sequence reads with the S. Typhimurium LT2 reference genome (Accession Number: AE006468). SNPs identified as phage, plasmid and repeat regions were removed from the dataset. Phylogenic analysis of S. enterica isolates was performed using SplitsTree4 (Huson, 1998) using the NeighborNet algorithm (Bryant and Moulton, 2004).
All genomes were submitted to NCBI under the following Accession Numbers: RQPK00000000, RQPL00000000, RQPM00000000, RQPN00000000, RQPO00000000, RQPP00000000, RQPQ00000000, RQPR00000000, RQPS00000000, RQPT00000000, RQPU00000000, RQPV00000000, RQPW00000000, RQPX00000000, RQPY00000000, RQPZ00000000, RQQA00000000, RQQB00000000, and RQQC00000000.
Identification and Classification of Mobile Genetic Elements
Plasmids were detected and typed using PlasmidFinder 2.0, a web tool designed to identify plasmids in Enterobacteriaceae sequence reads (Carattoli et al., 2014). Plasmid regions were manually extracted in Geneious as GenBank files and visualized and compared using EasyFig v2.2.3 (Sullivan et al., 2011). Prophage insertions were identified using the online platform PHASTER (Zhou et al., 2011; Arndt et al., 2016). Phage regions were extracted and analyzed in NCBI nucleotide BLASTn service to confirm their identity. Transposase and insertion sequence classification was performed using ISFinder (Siguier et al., 2006).
Identification of Antibiotic, Heavy Metal, and Disinfectant Resistance (AMR) Determinants
Identification of acquired antimicrobial resistance genes and chromosomal point mutations that confer antimicrobial resistance phenotypes was performed using Resistance Gene Identifier (RGI), a resistome prediction tool which uses BLAST algorithms using curated AMR genes and SNPs available in the Comprehensive Antibiotic Resistance Database (Jia et al., 2017). A secondary enquiry was performed using the webserver ResFinder 3.43. The databases include AMR determinants for β-lactams, fluoroquinolones, aminoglycosides, tetracyclines, phenicols, trimethoprim, and sulphonamides. Detection of mutations in the Quinolone Resistance Determining Regions of DNA gyrase genes gyrA and gyrB and DNA topoisomerase IV genes parC and parE was performed through alignment with reference genes downloaded from the National Centre for Biotechnology Information. The reference genes were pairwise aligned to the corresponding genes annotated from the isolate’s draft genome sequence using the ClustalW alignment tool (Thompson et al., 1994).
Identification of biocide and heavy metal resistance genes was performed using the BLASTn search engine available through BacMet4 a curated database of predicted and experimentally confirmed resistance genes (Pal et al., 2014). Text-based queries of sequence annotations was also used to search for antimicrobial resistance genes using Geneious v10.2.3 software package (5 Biomatters Limited).
Determination of Antibiotic Susceptibility Phenotype
The sensitivity of all 19 isolates in this study to a panel of 14 antibiotics was determined by disc diffusion performed in accordance with the Clinical Laboratory Standards Institute (CLSI) standards (Clinical and Laboratory Standards Institute, 2017). The antibiotic panel included for testing comprised of ampicillin (10 μg), streptomycin (10 μg), tetracycline (30 μg), cefoxitin (30 μg), sulphafurazole (100 μg), florfenicol (30 μg), norfloxacin (10 μg), kanamycin (30 μg), gentamicin (256 μg), ciprofloxacin (5 μg), chloramphenicol (30 μg), trimethoprim-sulfamethoxazole (23.75 μg), and nalidixic acid (30 μg). An Escherichia coli strain (ATCC® 25922TM) was used as quality control (QC) to achieve the minimum performance standards as dictated by the CLSI (Clinical and Laboratory Standards Institute, 2017). This assay was performed in duplicate on separate occasions.
Determination of Heavy Metal Susceptibility Phenotype
The isolates were subjected to heavy metal susceptibility testing by the agar dilution modified method to that previously described. Sensitivity was measured against six heavy metals: arsenic (NaAsO2; 0–20 mM), cadmium (CdCl2; 0–20 mM), cobalt (CoCl2; 0–20 mM), copper (CuSO4; 0–32 mM), lead [Pb(NO3)2; 0–15 mM], and zinc (ZnSO4; 0–20 mM). Cultures were grown for 16 ± 2 h on TSA plates at 37°C. Three to five colonies were then inoculated into 3 ml of Maximum Recovery Diluent (Oxoid) to make a solution with a turbidity equal to that of a 0.5 McFarland standard. A 20 μl aliquot of the bacterial suspension was transferred dropwise onto the surface of the Isosensitest agar (Oxoid) containing two-fold increases in heavy metal concentrations. The plates were allowed to dry before being incubated for 24 h at 37°C. Following incubation, the plates were observed for the presence of bacterial growth. The minimum inhibitory concentration (MIC) was interpreted as the minimum concentration of heavy metal that inhibited the growth of the bacterium. A sterile loop was used to scrape the surface of the agar plate where the bacteria culture was dropped to harvest any remaining viable cells. The cells were streaked across the surface of a TSA plate void of heavy metals and incubated for a further 24 h at 37°C. The minimum bactericidal concentration (MBC) was determined to be the lowest concentration of heavy metals where no growth occurred after re-culturing on TSA. This assay was performed in duplicate on separate occasions.
Determination of Disinfectant Susceptibility Phenotype
The isolate’s susceptibility profile was tested against three disinfectants commonly used in the food production facilities. Benzalkonium chloride (QAC; 0.5–256 μg/ml), chlorhexidine gluconate (cation; 0.06–64 μg/ml), and triclosan (phenol; 0.125–1024 μg/ml). The MIC and MBC for each disinfectant was determined by using a modified broth microdilution method to that previously described (Condell et al., 2012). Isolates were streaked on TSA and incubated at 37°C for 16 ± 2 h. A colony of each isolate was suspended in 10 ml of Mueller Hinton broth (MHB) and incubated at 37°C until late logarithmic phage. A 100 μl aliquot of the incubated culture was added to 9.9 ml of MHB to form a 1:10,000 dilution containing approximately 105 log10 CFU/ml. A 100 μl aliquot of the bacterial culture was then transferred into wells of 96-well microtiter test plate containing 100 μl of MHB containing 2-fold concentrations of the disinfectants/sanitizers. The microtiter plate was incubated for 24 h at 37°C. The minimum inhibitory concentration was assessed by turbidimetric analysis. Bacterial growth was determined using a spectrophotometer with a wavelength of 600 nm, recorded every 30 min over a 24 h period. To establish the background OD, readings taken at 0 m, 30 m, and 1 h were averaged. If the OD of the experimental wells exceeded the background OD, the well was considered positive for bacterial growth. The MIC was designated as the lowest biocide concentration at which the OD of the test well did not exceed the optical density of the background OD. Following the 24 h test period, a 100 μl aliquot of each well was transferred to 100 μl of Dey-Engley Neutralizing Broth (Sigma-Aldrich, St. Louis, MO, United States) and allowed to incubate for 2 minutes at room temperature. A 100 μl aliquot was then spread onto the surface of a MH agar plate and incubated at 37°C for 18–24 h (Skowron et al., 2018). The MBC was designated as the lowest biocide concentration which resulted in no viable growth of that isolate. This assay was performed in duplicate on separate occasions.
Ethics Statement
No ethical approval was obtained as this study did not involve animal or human subjects. The study used existing isolates from the CSIRO culture collection. New samples or isolates were not collected as part of the study and based on this no ethics approval was required for this study as defined by University of the Sunshine Coast’s research ethics arrangements and the National Statement on Ethical Conduct in Human Research. CSIRO own these samples and gave permission to use them.
Results
Overview of the Genomic Composition of S. enterica Isolates
A summary of the genomic characterization of 19 S. enterica isolates included in this study is shown in Table 2. The genomes ranged in size from 4.65 to 5.03 Mbp and GC contents were between 52.1 and 52.2%. The number of coding DNA sequences genes (CDSs) ranged from 4,623 in the smallest genome to 5,150 in the largest genome. The number of tRNA genes ranged from 78 up to 95 genes. The quantity of CDS and tRNA typically varied depending on the presence or absence of plasmids, prophage insertions or transposons.
Variant calling among the S. Typhimurium isolates was performed by mapping raw sequence reads with S. Typhimurium LT2 (NC_003197.2) using Snippy. The core SNP file identified 26,400 variable sites. Using the NeighborNet algorithm (Bryant and Moulton, 2004) in the SplitsTree4 package (Huson, 1998), a phylogenic network was constructed from the core SNP file. The phylogenic network of S. Typhimurium isolates in this study is shown in Figure 1. Six of eight S. Typhimurium PT135/PT135a isolates formed one clonal group, which also included the PT108 isolate. Other PT135 isolates were more divergent, including Sal-1657 which clustered near the S. Typhimurium LT2 reference genome. This strain is the only PT135/135a isolate recovered from a dairy factory. Two other clusters were evident, one of which included both PT170 strains, isolated from cattle feces and goat feces in 2002 and 2001, respectively. The other highly clonal group included both PT9 isolates.
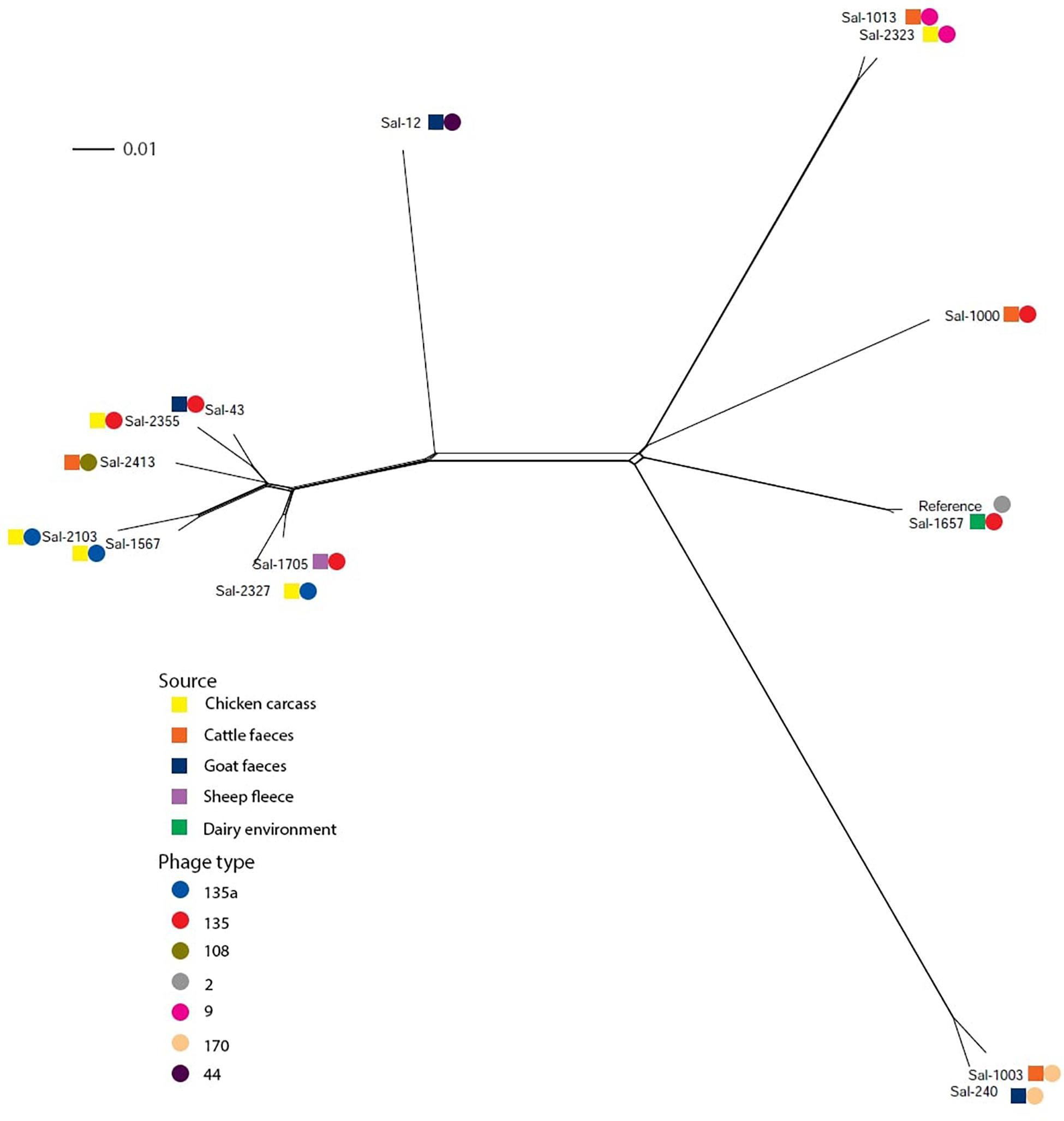
Figure 1. Phylogenic networks of S. Typhimurium genomes constructed using the Neighbor-Joining algorithm, Neighbor-Net, in SplitsTree4. Reference refers to S. Typhimurium LT2 reference genome (Accession Number: AE006468). Colored squares represent the isolation source and the colored circle represent the phage type. The scale bar distance represents 0.01 nucleotide substitutions per site.
Characterization of Mobile Genetic Elements of S. enterica Isolates
Characterization of Plasmids Carried by S. enterica in This Study
Analysis of WGS data using Plasmid Finder identified three types of plasmid profiles, as represented in Figure 2. Twelve of 14 S. Typhimurium isolates carried a ∼94 kbp IncF virulence plasmid. The plasmid contains three virulence operons, the spv virulence loci which including five genes spvRABCD, the ccdAB antitoxin/toxin loci and the vapBC antitoxin/toxin loci all of which were conserved in all genomes. It also carries a chromosome-independent fer fimbrial operon. When analyzed in the NBCI BLASTn platform, the plasmid sequence shared 99% sequence identity with plasmid pSLT plasmid carried by S. Typhimurium LT2 strain (ATCC® 14028TM, Accession Number: NC_003277). Multiple alignment of the 12 pSLT plasmid sequences extracted from genomes of isolates in this study identified 99.96% sequence homology with a total of 106 SNPs. Non-synonymous variances include a SNP leading to amino acid modification Gln216Leu in kappa-fimbrae chaperone gene pefD in isolate Sal-2323 and a SNP leading to AA change Trp141Cys and in the plasmid SOS inhibition protein of isolate Sal-2355. Additionally, two isolates of phage type PT135A, Sal-2103, and Sal-1576, possess an identical non-synonymous SNP in residue 26 of the ccdB toxin gene causing AA modification of aspartic acid (Asp) to valine (Val). The plasmid did not carry any identified antibiotic, heavy metal or biocide resistance genes.
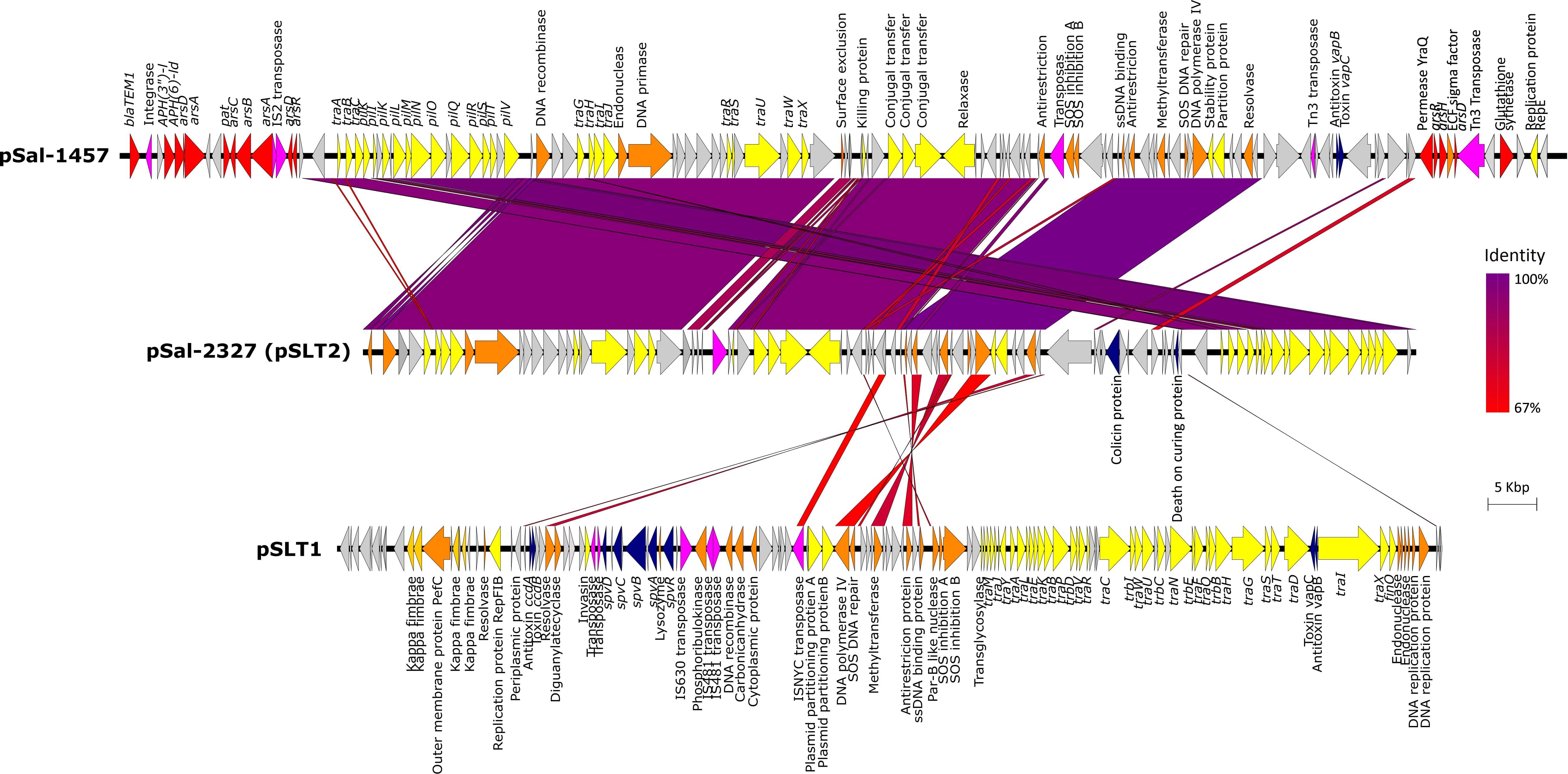
Figure 2. The alignment of three plasmids, pSal-1457, pSal-2327, and pSLT1 using Easyfig. Gene annotations are characterized by function as follows: resistance genes (red), conjugal transfer associated genes (yellow), virulence genes (blue), DNA replication (orange), transposons/integrases (pink), and hypothetical proteins (gray).
Only one isolate carried more than one plasmid. In addition to pSLT described above, Sal-2327 also possessed an additional IncI1 incompatibility group virulence plasmid 89.6 kbp in length. With the exception of the presence of a-DNA adenine methyltransferase and a small number of DNA replication proteins, this plasmid shares very little homology with pSLT1. However, BLASTn comparison showed 85.7% sequence identity to the S. Typhimurium pSLT2 plasmid (Accession Number: KF290378). While this plasmid did contain complete plasmid replication and transfer coding machinery, it did not contain any known virulence or antimicrobial resistance gene cassettes.
A third plasmid was identified in S. Infantis strain Sal-1457 which was isolated from a chicken carcass in 2005. This plasmid was 121.4 Kbp in length and contained a vapBC toxin/antitoxin loci and a number of antimicrobial resistance operons. The plasmid included a Class 1 integron containing an AMR gene cassette: integrase (intI1), aminoglycoside 3-phosphotransferase [aph(3″)-I], aminoglycoside 6-phosphotransferase [aph(6″)-ld], b-lactamase (blaTEM–1) and a trimethoprim resistance conferring dihydrogen reductase (dfrA5). Another feature of this plasmid is the presence of two separate transposons both of which harbored arsenic resistance operons. The first transposon included a Tn5393 transferase flanking an arsRHD operon, and the second containing two tandem IS2-family transposases followed by an arsABCD operon.
Plasmids were not identified in S. Typhimurium isolates of phage type PT170, both S. Singapore isolates, and two of three S. Infantis isolates.
Phage Insert Regions and Their Antimicrobial Resistance Determinants
Prophage detection using the online platform PHASTER identified 70 complete prophage insertions among the 19 S. enterica isolates in this study. Among the phage insertion sites, fourteen prophage types were identified (Table 3). Phage inserts accounted for the majority of genomic diversity between strains (Figure 3). All genomes contained between two to four prophage sequences with the exception of Sal-12 which harbored five different prophage insertions. The Lambda-like prophage insertions Gifsy-1, Gifsy-2, and ST64B were highly conserved among S. Typhimurium isolates. Prophage ST64B, a P22 family of prophage was complete in all S. Typhimurium isolates with the exception of two PT170 isolates, Sal-240 and Sal-1003, which were missing a phage antitermination protein, DNA invertase, phage holin, an endopeptidase rzpD and two hypothetical phage proteins of unknown function. Gifsy-2 prophage contains two virulence factors, superoxide dismutase (Cu and Zn) encoded by the sodC gene, and msgA, a virulence gene of unknown function. A Gifsy-2 phage was also identified in both S. Singapore isolates which also contains a spvRABC virulence operon.
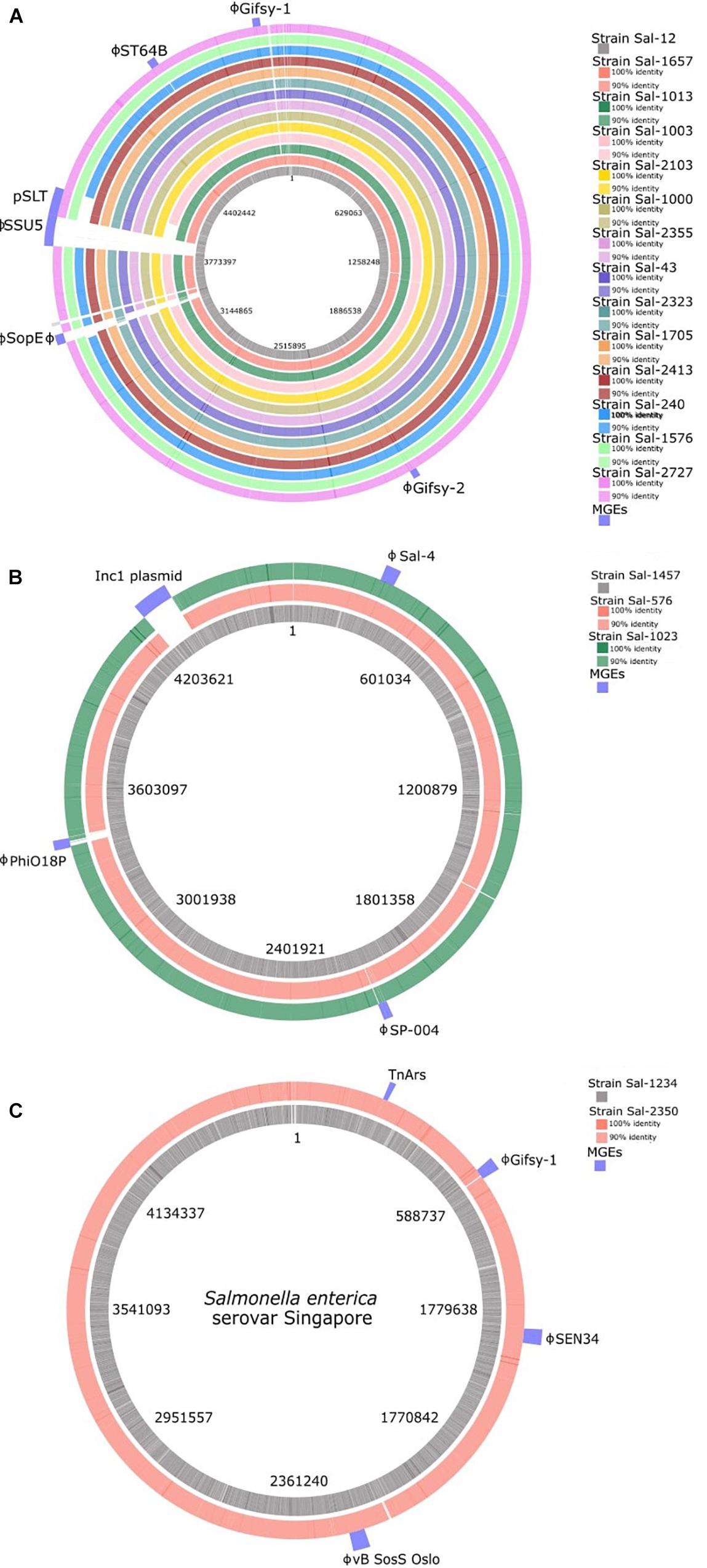
Figure 3. BLAST ring visualization of genomes of (A) S. Typhimurium, (B) S. Infantis, and (C) S. Singapore isolates analyzed in this study. The location of mobile genetic elements is indicated in the outer ring. Mobile genetic elements consist of the majority of intra-serotype variation between isolates. ϕ, complete prophage.
Sal-12 is the only isolate to carry SopEΦ, a P2-like phage closely related to Fels-2 found in LT2. This phage harbors virulence factor gene sopE. Phage SPN9CC (JF900176) a P22-like phage was identified in only three isolates: PT170 strains Sal-240, Sal-1003, and PT135 strain Sal-2413. Sal-1013 and Sal-2323, both phage type 9, carry a phage that shares 99.9% homology with Salmonella phage RE-2010.
Transposon-Conferred Resistance Genes
The nucleotide sequence of the two S. Singapore isolates examined in this study included a gene cluster containing an arsenic resistance operon. This included two integrase genes flanked by an arsenic resistance transcriptional repressor (arsR), arsenate reductase (arsC), arsenic resistance transacting repressor (arsD), arsenical pump-driving ATP-ase (arsA), and arsenical-resistance protein (arsB). The 5,166 bp transposon containing the arsRCDAB operon shared 100% homology with an arsenic resistance gene cassette identified in S. Thompson strain (ATCC® 8391TM, Accession Number: CP011396) and 99% query identity with S. Kentucky strain CVM29188 (Accession Number: ABAK02000001.1). The genome of S. Typhimurium isolates Sal-2103 contained an IS630-family transposon integrated into a Type IV secretion system. The transposon contains a tetracycline resistance gene cassette, inclusive of a tetracycline resistance regulatory gene tetR, tetracycline resistance efflux pump encoded tetA, and an EamA family multidrug/metabolite transporter flanked by two IS630-family transposases.
Characterization of Chromosomally Located Antimicrobial Resistance Determinants
Among all 19 isolates, there were no mutations identified in the quinolone resistance determining region of DNA topoisomerase genes gyrB, parC, and parE. Additionally, there were no isolates found to carry quinolone resistance genes qnrA, qnrB, or qnrS. An isolated SNP was identified in the gyrA gene of nalidixic acid-resistant isolate Sal-1657 in the 87th codon leading to the amino acid substitution aspartate (Asp) to glycine (Gly). Analysis of chromosomal DNA and mobile genetic elements found that there was little difference in the gene content that confers resistance to biocides that could account for any differentiation in the biocide resistance phenotype of isolates in this study. With the exception of the tetAR genotype identified in Sal-2103 there were no tetracycline genes (tetABCDGR) found in any isolate in this study.
Antimicrobial Susceptibility Profile of Isolates in This Study
The antibiotic resistance profiles of all 19 S. enterica isolates are shown in Table 4. Antibiotic susceptibility testing identified that 7 isolates were resistant to at least one antibiotic. All isolates (100%) were susceptible to chloramphenicol, ciprofloxacin, florfenicol, sulfamethoxazole-trimethoprim, gentamycin, and kanamycin. Isolate Sal-1457, which was found to harbor a plasmid-borne blaTEM–1 gene and strAB gene in a Class 1 integron, was found to be resistant to ampicillin, streptomycin and cefoxitin. All other isolates were susceptible to the β-lactam class of antibiotics with the exception of Sal-576 and Sal-1023 (both S. Infantis) that were resistant to cefoxitin. Isolate Sal-2103 was resistant to tetracycline, consistent with its transposon-carrying tetAR genotype. Isolate-1657, which possessed a mutation to DNA gyrase subunit gene gyrA, was resistant to nalidixic acid but not resistant to ciprofloxacin, also a fluoroquinolone antibiotic. Despite not having any antibiotic resistance genes known to confer sulphafurazole resistance (sul1, sul2, or sul3), isolates Sal-43 and Sal-1576 were found to be resistant to this antibiotic. Similarly, all three S. Infantis isolates are resistant to cefoxitin, however no resistance genes associated with resistance to this antibiotic were detected in the WGS data of these isolates when analyzed against the database libraries.
Heavy Metal and Disinfectant Susceptibility of Isolates in This Study
Heavy metal susceptibility testing of the 19 Salmonella isolates was performed against arsenic, lead, cadmium, copper, cobalt, and zinc (Table 5). Isolates Sal-1003, Sal-1023, Sal-1234, and Sal-2350, all of which possess arsenic resistance gene operons, were found to be viable at 30 mM NaAsO2, the highest concentration tested in this study. This concentration is more than 5× the MBC of the other 15 isolates at 6 mM NaAsO2. The MIC of all isolates to Pb(NO3)2 was recorded as 10 mM. However, viable cells were found at the highest concentration tested of 15 mM (MBC not determined). A concentration of 0.5 mM of CdCl2 was bactericidal to all but three isolates. Isolates Sal-1013, Sal-1657, Sal-2355 were viable at 0.5 mM but showed no visible growth at this concentration (inhibited). Isolate Sal-1705 showed visible growth and viability at 0.5 mM but was inhibited at 1.5 mM. A concentration of 1.5 mM of CdCl2 was bactericidal to all isolates. There were no differences in the MIC or MBC of all isolates utilized in this study when exposed to CoCl2, CuSO4, and ZnCl2.
Overall, all isolates had the same (16 μg/ml) MIC and MBC to benzalkonium chloride. While the MIC for triclosan ranged from 0.125 to 0.25 μg/ml, the MBC was more varied from 0.125 to 2 μg/ml with S. Infantis and S. Typhimurium ST135 isolates dominating the higher MBC concentration range. There was a marked difference between the MIC and MBC of chlorhexidine. MIC values ranged from 2 to 8 μg/ml and MBC values from 4 to 64 μg/ml. Sal-1657 recorded the highest MBC value, which was a 16-fold increase on the lowest MBC value recorded (Sal-576).
Discussion
This study utilized WGS and comparative genomics to characterize the resistance genes present in the genomes of 19 S. enterica strains isolated in Australia and determine the location of these genes within the chromosome and mobile genetic elements. Traditional phenotypic susceptibility testing was also performed to evaluate prediction of phenotypic traits from genotypic data, and determine any associated discrepancies. The use of WGS may reduce the rate of discrepancies caused by unstandardized approaches to phenotypic susceptibility testing and may also identify resistance markers not routinely screened for in phenotypic analysis (Zankari et al., 2013). WGS has the capacity to circumvent the limitations of traditional screening methods, allowing for the detection of AMR genes and their location within the associated bacterial genome, and identify mobile genetic elements which may lead to dissemination of AMR genes throughout the food production chain and clinical settings. Due to the high reliability, reproducibility and availability of WGS technology, this methodology is fast becoming the gold standard for molecular analysis (Zankari et al., 2013; Portmann et al., 2018). The potential application of WGS technologies for the detection of antibiotic susceptibility phenotypes has previously been demonstrated in E. coli and Klebsiella pneumoniae, where genome-based prediction yielded high specificity values of 96 and 97% (Stoesser et al., 2013). Similarly, quantitative correlations between Salmonella isolates studied in the United States with a resistant phenotype and a resistant genotype were identified in 99% of cases (McDermott et al., 2016). A recent study concerning S. enterica found that AMR phenotype and genotype profiles of 3,491 isolates were strongly correlated, with 97.82% in agreement (Neuert et al., 2018). This current study characterized the antibiotic, heavy metal and disinfectant resistance genotype and mobile genetic elements of 19 Salmonella enterica isolates using WGS data. Whilst this current study did not have a sufficient sample size to infer the statistical significance of a correlation, it could be elucidated that antibiotic resistance genotype profiles were correct, in the most part, in predicting the resistance phenotype. Bioinformatic analysis of WGS was able to detect tetracycline, penicillin, ampicillin and nalidixic acid resistant phenotypes in this study. However, sulphafurazole and cefoxitin resistance could not be predicted using the methodology employed. There is likely mechanisms currently unknown, or not included in the database libraries. Thus the mechanism(s) remain unknown in this case.
In addition to its utility in phenotypic prediction through genotypic interrogation, SNP subtyping shows promising application in epidemiological surveillance and outbreak investigations (Ford et al., 2018). In this study, the application of SNP subtyping could differentiate all isolates from one another, including those that shared a similar phage type. Interestingly, clustering did not group PT135 and PT135a in distinct subgroups. Separation of PT135 and PT135a through phage typing has been used in Australia to provide increased resolution in epidemiological investigations (McPherson et al., 2006).
A number of cellular pathways used by Salmonella to resist disinfectants have been previously identified. Resistance to quaternary ammonium compounds (QACs), as described in previous studies, can by conferred by the acquisition of transmembrane efflux pump genes including qacA, qacB, qacC, qacH, smr, and qacΔE1, the latter of which is conserved in the Class 1 integron (Deng et al., 2017). This study did not detect known QAC or triclosan resistance determinants in the genome sequences of the 19 isolates including in this study, which is supported by the phenotypic data. Although chlorhexidine MICs were within a small range (2–8 mg/L), MBC values showed higher variation (4–64 mg/L). The reasons for this disparity were not identified in this study and require further investigation. Arsenic resistance operons were detected in five isolates. Although four of these showed a higher MIC to arsenic, one isolate (Sal-1457) which possessed two plasmid-encoded arsenic resistance operons, did not demonstrate increased tolerance in vitro. Research in the area of biocide and heavy metal resistance is lacking, with much of the focus on validating WGS as an antibiotic resistance detection tool due to the clinical implications of AMR. Consequently, there are few bioinformatics tools available that have been validated that can detect biocide and heavy metal resistance in bacterial pathogens.
Antibiotic resistance presents a significant issue for public health and clinical outcomes for patients (Krueger et al., 2014). In response, the World Health Organization has identified antibiotic resistant Salmonella as a species of global public health significance and a priority for future research and development strategies (WHO, 2017). There have been a number of emerging reports of antibiotic resistant Salmonella isolated from Australian food production chains. Among 217 isolates from Australian beef cattle, a resistant phenotype was observed in the presence of ampicillin (7.5%), streptomycin (7.5%), tetracycline (6.6%), and trimethoprim-sulfamethoxazole (7.5%, Barlow et al., 2015). However, this prevalence is relatively low when compared to Salmonella AMR data from the United States, with a previous study reporting higher resistance rates to ampicillin (9.2%), streptomycin (15.5%), tetracycline (66.9%), and trimethoprim-sulfamethoxazole (14.9%) among cattle isolates in Food and Drug Administration [FDA] (2015). The results of the current study show similar trends to previous work (Barlow et al., 2015), and suggest that the overall prevalence of clinically relevant AMR in Australian Salmonella isolated from the environment is relatively low, with 7 out of 19 isolates demonstrating resistance to only one antibiotic and only one isolate with a MDR profile.
This study reported relatively high sensitivity to zinc (4 mM), copper (8 mM), and cobalt (4 mM) in all isolates. Previous studies have reported copper tolerance up to 24 mM and zinc tolerance at 8 mM in Salmonella isolated from swine feed and feces and the carriage of the copper, zinc cadmium efflux pump gene czcD was significant associated with increased MIC (Medardus et al., 2014). Tetracycline, nalidixic acid, and ampicillin are early generation antibiotics and have been used extensively in food producing animals (Silbergeld et al., 2008). This may have contributed to the representation of the resistance genotype/phenotype present in this study. Resistance to nalidixic acid was identified in one isolate in this study that possessed a SNP in the QRDR of DNA gyrase gene gyrA resulting in amino acid change Asp87Gly. Mutations to the Quinolone Resistance Determining Regions (QRDR) in DNA gyrase genes, gyrA and gyrB, and DNA Topoisomerase genes, parC and parE, have been detected in fluoroquinolone-resistant strains of Salmonella, including those resistant to ciprofloxacin, norfloxacin and nalidixic acid (Gal-Mor et al., 2010; Utrarachkij et al., 2017; Vital et al., 2017). Nalidixic acid is not currently used as a treatment option of salmonellosis in Australia, with amoxicillin, trimethoprim-sulfamethoxazole and azithromycin used in human cases and apromycin used in calves, pigs and broiler infections (Lean et al., 2013).
It is interesting to note that the majority of resistance determinants identified in this study were present on transposable elements, either on plasmids or chromosomally located MGEs. All but six strains harbored large conjugative plasmids, with an InclI plasmid carried by Sal-1457 conferring resistance to ampicillin and streptomycin, found on a class 1 operon. This plasmid also carried two transposons flanking arsenic resistance gene cassettes. Additionally, Sal-2103 carries a transposon that confers resistance to tetracycline while transposons carried by Sal-2350 and Sal-1234 conferred arsenic resistance. The presence of transposons and mobile genetic elements nested within larger mobile genetic elements, such as plasmids or phage, has been found to promote faster dissemination of AMR genes (Han et al., 2012; Sheppard et al., 2016). Plasmids analyzed in this study also harbored a number of virulence and toxin/antitoxin genes including the spv operon that is associated with survival in macrophages through actin depolarisation and host cell apoptosis (Guiney and Fierer, 2011). The superoxide dismutase encoding gene was also identified in two different prophage types, including Gifsy-2 found in all S. Typhimurium isolates. SodCI enzyme mutants have been found to possess reduced survivability to macrophage oxidative burst, through the inability to convert phagocytic superoxide to peroxide (Ammendola et al., 2008). A 38.5 kbp prophage (SopEφ) found in S. Typhimurium isolate Sal-12 contained the sopE gene, a TIII-secreted protein that enables the internalization of Salmonella into the host cell (Vonaesch et al., 2014). Whilst no AMR genes were present in these prophage, these findings suggest the role of these MGEs in the dissemination MDR resistance phenotypes in Salmonella.
Conclusion
Antibiotic resistance is an increasing important issue which has serious implications for human and veterinary science. The presented study suggests that there are low levels of clinically relevant antibiotic resistance among Salmonella isolated from Australian food-producing animals and that mobile genetic elements such as transposons and plasmids play a central role in the dissemination of AMR genes. Further surveillance in Australia of AMR prevalence among Salmonella from food animal-related sources, with an expanded cohort and larger serotype representation, is recommended and this study provides a basis for further investigation.
Author Contributions
AW completed the experiments, and collected and analyzed the data. AW, NF, EF, and DK conceived and designed the experiments, and wrote, revised, and approved the final version of the manuscript.
Funding
This work was supported by funding from the University of the Sunshine Coast and the Commonwealth Scientific and Industrial Research Organisation (CSIRO), Australia.
Conflict of Interest Statement
The authors declare that the research was conducted in the absence of any commercial or financial relationships that could be construed as a potential conflict of interest.
Acknowledgments
We thank the staff and students of the University of the Sunshine Coast, particularly the university’s technical officers Daniel Shelley, Dr. Daniel Powell, and Tara Nielson. We also thank Dr. Scott Chandry of the CSIRO for his technical advice.
Footnotes
- ^ http://health.gov.au/internet/fr/publishing.nsf/Content/australia-foodborne-illness-reduction-strategy-2018%E2%80%932021
- ^ https://github.com/tseemann/snippy
- ^ http://genomicepidemiology.org
- ^ http://bacmet.biomedicine.gu.se/
- ^ www.geneious.com
References
Alam, M. T., Petit, R. A., Crispell, E. K., Thornton, T. A., Conneely, K. N., Jiang, Y., et al. (2014). Dissecting vancomycin-intermediate resistance in Staphylococcus aureus using genome-wide association. Genome Biol. Evol. 6, 1174–1185. doi: 10.1093/gbe/evu092
Alikhan, N. F., Petty, N. K., Ben Zakour, N. L., and Beatson, S. A. (2011). Blast ring image generator (BRIG): simple prokaryote genome comparisons. BMC Genom. 12:402. doi: 10.1186/1471-2164-12-402
Ammendola, S., Pasquali, P., Pacello, F., Rotilio, G., Castor, M., Libby, S. J., et al. (2008). Regulatory and structural differences in the Cu,Zn-superoxide dismutases of Salmonella enterica and their significance for virulence. J. Biol. Chem. 283, 13688–13699. doi: 10.1074/jbc.M710499200
Andrews, S. (2010). FastQC: A Quality Control Tool for High Throughput Sequence Data. Available at: http://www.bioinformatics.babraham.ac.uk/projects/fastqc/ (accessed August 28, 2017).
Arndt, D., Grant, J. R., Marcu, A., Sajed, T., Pon, A., Liang, Y., et al. (2016). PHASTER: a better, faster version of the phast phage search tool. Nucleic Acids Res. 44, W16–W21. doi: 10.1093/nar/gkw387
Australian Department of Health (2018). Australia’s Foodborne Illness Reduction Strategy 2018 - 2021. Canberra: Australian Department of Health.
Aziz, R. K., Bartels, D., Best, A. A., DeJongh, M., Disz, T., Edwards, R. A., et al. (2008). The RAST server: rapid annotations using subsystems technology. BMC Genom. 9:75. doi: 10.1186/1471-2164-9-75
Bankevich, A., Nurk, S., Antipov, D., Gurevich, A. A., Dvorkin, M., Kulikov, A. S., et al. (2012). SPAdes: a new genome assembly algorithm and its applications to single-cell sequencing. J. Comput. Biol. 19, 455–477. doi: 10.1089/cmb.2012.0021
Barlow, R. S., McMillan, K. E., Duffy, L. L., Fegan, N., Jordan, D., and Mellor, G. E. (2015). Prevalence and antimicrobial resistance of Salmonella and Escherichia coli from Australian cattle populations at slaughter. J. Food Prot. 78, 912–920. doi: 10.4315/0362-028X.JFP-14-476
Bennett, P. M. (2008). Plasmid encoded antibiotic resistance: acquisition and transfer of antibiotic resistance genes in bacteria. Br. J. Pharmacol. 153, S347–S357. doi: 10.1038/sj.bjp.0707607
Bolger, A. M., Lohse, M., and Usadel, B. (2014). Trimmomatic: a flexible trimmer for illumina sequence data. Bioinformatics 30, 2114–2120. doi: 10.1093/bioinformatics/btu170
Brettin, T., Davis, J. J., Disz, T., Edwards, R. A., Gerdes, S., Olsen, G. J., et al. (2015). RASTtk: a modular and extensible implementation of the rast algorithm for building custom annotation pipelines and annotating batches of genomes. Sci. Rep. 5:8365. doi: 10.1038/srep08365
Bryant, D., and Moulton, V. (2004). Neighbor-net: an agglomerative method for the construction of phylogenetic networks. Mol. Biol. Evol. 21, 255–265. doi: 10.1093/molbev/msh018
Butaye, P., Cloeckaert, A., and Schwarz, S. (2003). Mobile genes coding for efflux-mediated antimicrobial resistance in gram-positive and gram-negative bacteria. Int. J. Antimicrob. Agents 22, 205–210. doi: 10.1016/S0924-8579(03)00202-4
Carattoli, A., Zankari, E., Garcia-Fernandez, A., Voldby Larsen, M., Lund, O., Villa, L., et al. (2014). In silico detection and typing of plasmids using plasmidfinder and plasmid multilocus sequence typing. Antimicrob. Agents Chemother. 58, 3895–3903. doi: 10.1128/aac.02412-14
Clinical and Laboratory Standards Institute (2017). Performance Standards for Antimicrobial Susceptibility Testing, 28th Edn. Wayne, PA: CLSI.
Coburn, B., Grassl, G. A., and Finlay, B. B. (2007). Salmonella, the Host and disease: a brief review. Immunol. Cell Biol. 85, 112–118. doi: 10.1038/sj.icb.7100007
Combs, B., Fitzsimmons, G., Fielding, J., Gregory, J., McPherson, M., Rice, B., et al. (2006). A multi-jurisdiction outbreak of Salmonella typhimurium phage type 135 associated with purchasing chicken meat from a supermarket chain. Commun. Dis. Intell. Q. Rep. 30, 449–455.
Condell, O., Iversen, C., Cooney, S., Power, K. A., Walsh, C., Burgess, C., et al. (2012). Efficacy of biocides used in the modern food industry to control Salmonella enterica, and links between biocide tolerance and resistance to clinically relevant antimicrobial compounds. Appl. Environ. Microbiol. 78, 3087–3097. doi: 10.1128/AEM.07534-11
Copitch, J. L., Whitehead, R. N., and Webber, M. A. (2010). Prevalence of decreased susceptibility to triclosan in Salmonella enterica isolates from animals and humans and association with multiple drug resistance. Int. J. Antimicrob. Agents 36, 247–251. doi: 10.1016/j.ijantimicag.2010.04.012
Cox, J. M., Woolcock, J. B., and Sartor, A. L. (2002). The Significance of Salmonella, Particularly S. Infantis, to the Australian Egg Industry: A report for the Rural Industries Research and Development Corporation. Canberra: Rural Industries Research and Development.
Delpech, V., McAnulty, J., and Morgan, K. (1998). A Salmonellosis outbreak linked to internally contaminated pork meat. Aust. N. Z. J. Public Health 22, 243–246. doi: 10.1111/j.1467-842X.1998.tb01181.x
den Bakker, H. C., Allard, M. W., Bopp, D., Brown, E. W., Fontana, J., Iqbal, Z., et al. (2014). Rapid whole-genome sequencing for surveillance of Salmonella enterica serovar enteritidis. Emerg. Infect. Dis. 20, 1306–1314. doi: 10.3201/eid2008.131399
Deng, W., Quan, Y., Yang, S., Guo, L., Zhang, X., Liu, S., et al. (2017). Antibiotic resistance in Salmonella from retail foods of animal origin and its association with disinfectant and heavy metal resistance. Microb. Drug Resist. 24, 782–791. doi: 10.1089/mdr.2017.0127
Duffy, L., Barlow, R., Fegan, N., and Vanderlinde, P. (2009). Prevalence and serotypes of Salmonella associated with goats at two Australian abattoirs. Lett. Appl. Microbiol. 48, 193–197. doi: 10.1111/j.1472-765X.2008.02501.x
Duffy, L. L., Small, A., and Fegan, N. (2010). Concentration and prevalence of Escherichia coli O157 and Salmonella serotypes in sheep during slaughter at two Australian Abattoirs. Aust. Vet. J. 88, 399–404. doi: 10.1111/j.1751-0813.2010.00623.x
Food and Drug Administration [FDA] (2015). NARMS Now Integrated Data. Rockville, MD: Department of Health and Human Services.
Ford, L., Carter, G. P., Wang, Q., Seemann, T., Sintchenko, V., Glass, K., et al. (2018). Incorporating whole-genome sequencing into public health surveillance: lessons from prospective sequencing of Salmonella typhimurium in Australia. Foodborne Pathog. Dis. 15, 161–167. doi: 10.1089/fpd.2017.2352
Ford, L., Glass, K., Veitch, M., Wardell, R., Polkinghorne, B., Dobbins, T., et al. (2016). Increasing incidence of Salmonella in Australia, 2000–2013. PLoS One 11:e0163989. doi: 10.1371/journal.pone.0163989
Friesema, I. H. M., Schimmer, B., Ros, J. A., Ober, H. J., Heck, M. E. O. C., Swaan, C. M., et al. (2012). A regional Salmonella enterica serovar Typhimurium outbreak associated with raw beef products, the Netherlands, 2010. Foodborne Pathog. Dis. 9, 12–107. doi: 10.1089/fpd.2011.0978
Gal-Mor, O., Valinsky, L., Weinberger, M., Guy, S., Jaffe, J., Schorr, Y. I., et al. (2010). Multidrug-resistant Salmonella enterica Serovar Infantis, Israel. Emerg. Infect. Dis. 16, 1754–1757. doi: 10.3201/eid1611.100100
Ghosh, A., Singh, A., Ramteke, P. W., and Singh, V. P. (2000). Characterization of large plasmids encoding resistance to toxic heavy metals in Salmonella abortus equi. Biochem. Biophys. Res. Commun. 272, 6–11. doi: 10.1006/bbrc.2000.2727
Guiney, D. G., and Fierer, J. (2011). The role of the spv genes in Salmonella pathogenesis. Front. Microbiol. 2:129. doi: 10.3389/fmicb.2011.00129
Han, J., Lynne, A. M., David, D. E., Tang, H., Xu, J., Nayak, R., et al. (2012). DNA sequence analysis of plasmids from multidrug resistant Salmonella enterica serotype heidelberg isolates. PLoS One 7:e51160. doi: 10.1371/journal.pone.0051160
Hundy, R. L., and Cameron, S. (2002). An outbreak of infections with a new Salmonella phage type linked to a symptomatic food handler. Commun. Dis. Intell. Q. Rep. 26, 562–567.
Huson, D. H. (1998). SplitsTree: analyzing and visualizing evolutionary data. Bioinformatics 14, 68–73. doi: 10.1093/bioinformatics/14.1.68
Jia, B., Raphenya, A. R., Alcock, B., Waglechner, N., Guo, P., Tsang, K. K., et al. (2017). CARD 2017: expansion and model-centric curation of the comprehensive antibiotic resistance database. Nucleic Acids Res. 45, D566–D573. doi: 10.1093/nar/gkw1004
Krueger, A. L., Greene, S. A., Barzilay, E. J., Henao, O., Vugia, D., Hanna, S., et al. (2014). Clinical outcomes of nalidixic acid, ceftriaxone, and multidrug-resistant nontyphoidal Salmonella infections compared with pansusceptible infections in foodnet sites, 2006-2008. Foodborne Pathog. Dis. 11, 335–341. doi: 10.1089/fpd.2013.1642
Lean, I., Page, S., Rabiee, A., and Willams, S. (2013). A Survey of Antibacterial Product Use in the Australian Cattle Industry. North Sydney, NSW: Meat & Livestock Australia.
Li, X., Meng, D., Li, J., Yin, H., Liu, H., Liu, X., et al. (2017). Response of soil microbial communities and microbial interactions to long-term heavy metal contamination. Environ. Pollut. 231, 908–917. doi: 10.1016/j.envpol.2017.08.057
Lim, S. Y., Joe, M. H., Song, S. S., Lee, M. H., Foster, J. W., Park, Y. K., et al. (2002). cuiD is a crucial gene for survival at high copper environment in Salmonella enterica Serovar typhimurium. Mol. Cells 14, 177–184.
Liu, M., Yang, Y., Yun, X., Zhang, M., and Wang, J. (2015). Concentrations, distribution, sources, and ecological risk assessment of heavy metals in agricultural topsoil of the three Gorges Dam Region, China. Environ. Monit. Assess. 187:147. doi: 10.1007/s10661-015-4360-6
Long, M., Lai, H., Deng, W., Zhou, K., Li, B., Liu, S., et al. (2016). Disinfectant susceptibility of different Salmonella serotypes isolated from chicken and egg production chains. J. Appl. Microbiol. 121, 672–681. doi: 10.1111/jam.13184
Mayrhofer, S., Paulsen, P., Smulders, F. J. M., and Hilbert, F. (2006). Short communication: antimicrobial resistance in commensal Escherichia coli isolated from muscle foods as related to the veterinary use of antimicrobial agents in food-producing animals in Austria. Microb. Drug Resist. 12, 278–283. doi: 10.1089/mdr.2006.12.278
McDermott, P. F., Tyson, G. H., Kabera, C., Chen, Y., Li, C., Folster, J. P., et al. (2016). Whole-genome sequencing for detecting antimicrobial resistance in nontyphoidal Salmonella. Antimicrob. Agents Chemother. 60, 5515–5520. doi: 10.1128/AAC.01030-16
McPherson, M. E., Fielding, J. E., Telfer, B., Stephens, N., Combs, B. G., Rice, B. A., et al. (2006). A multi-jurisdiction outbreak of Salmonella typhimurium phage type 135 associated with purchasing chicken meat from a supermarket chain. Commun. Dis. Intell. Q. Rep. 30, 449–455.
Medardus, J. J., Molla, B. Z., Nicol, M., Morrow, W. M., Rajala-Schultz, P. J., Kazwala, R., et al. (2014). In-feed use of heavy metal micronutrients in U.S. swine production systems and its role in persistence of multidrug-resistant Salmonellae. Appl. Environ. Microbiol. 80, 2317–2325. doi: 10.1128/aem.04283-13
Moffatt, C. R., Musto, J., Pingault, N., Miller, M., Stafford, R., Gregory, J., et al. (2016). Salmonella typhimurium and outbreaks of egg-associated disease in Australia, 2001 to 2011. Foodborne Pathog. Dis. 13, 379–385. doi: 10.1089/fpd.2015.2110
Moffatt, C. R. M., Musto, J., Pingault, N., Combs, B., Miller, M., Stafford, R., et al. (2017). Recovery of Salmonella enterica from Australian layer and processing environments following outbreaks linked to eggs. Foodborne Pathog. Dis. 14, 478–482. doi: 10.1089/fpd.2016.2268
Munnoch, S. A., Ward, K., Sheridan, S., Fitzsimmons, G. J., Shadbolt, C. T., Piispanen, J. P., et al. (2009). A multi-state outbreak of Salmonella Saintpaul in Australia associated with cantaloupe consumption. Epidemiol. Infect. 137, 367–374. doi: 10.1017/S0950268808000861
Neuert, S., Nair, S., Day, M. R., Doumith, M., Ashton, P. M., Mellor, K. C., et al. (2018). Prediction of phenotypic antimicrobial resistance profiles from whole genome sequences of non-typhoidal Salmonella enterica. Front. Microbiol. 9:592. doi: 10.3389/fmicb.2018.00592
Overbeek, R., Olson, R., Pusch, G. D., Olsen, G. J., Davis, J. J., Disz, T., et al. (2014). The SEED and the rapid annotation of microbial genomes using subsystems technology (RAST). Nucleic Acids Res. 42, D206–D214. doi: 10.1093/nar/gkt1226
Pal, C., Bengtsson-Palme, J., Rensing, C., Kristiansson, E., and Larsson, D. G. (2014). BacMet: antibacterial biocide and metal resistance genes database. Nucleic Acids Res. 42, D737–D743. doi: 10.1093/nar/gkt1252
Pesciaroli, M., Cucco, L., De Luca, S., Massacci, F. R., Maresca, C., Medici, L., et al. (2017). Association between pigs with high caecal Salmonella loads and carcass contamination. Int. J. Food Microbiol. 242, 82–86. doi: 10.1016/j.ijfoodmicro.2016.11.021
Portmann, A.-C., Fournier, C., Gimonet, J., Ngom-Bru, C., Barretto, C., and Baert, L. (2018). A validation approach of an end-to-end whole genome sequencing workflow for source tracking of Listeria monocytogenes and Salmonella enterica. Front. Microbiol. 9:446. doi: 10.3389/fmicb.2018.00446
Sheppard, A. E., Stoesser, N., Wilson, D. J., Sebra, R., Kasarskis, A., Anson, L. W., et al. (2016). Nested Russian doll-like genetic mobility drives rapid dissemination of the carbapenem resistance gene blaKPC. Antimicrob. Agents Chemother. 60, 3767–3778. doi: 10.1128/aac.00464-16
Siguier, P., Perochon, J., Lestrade, L., Mahillon, J., and Chandler, M. (2006). ISfinder: the reference centre for bacterial insertion sequences. Nucleic Acids Res. 34, 32–36. doi: 10.1093/nar/gkj014
Silbergeld, E. K., Graham, J., and Price, L. B. (2008). Industrial food animal production, antimicrobial resistance, and human health. Annu. Rev. Public Health 29, 151–169. doi: 10.1146/annurev.publhealth.29.020907.090904
Skowron, K., Hulisz, K., Gryń, G., Olszewska, H., Wiktorczyk, N., and Paluszak, Z. J. I. M. (2018). Comparison of selected disinfectants efficiency against Listeria monocytogenes biofilm formed on various surfaces. Int. Microbiol. 21, 23–33. doi: 10.1007/s10123-018-0002-5
Stoesser, N., Batty, E. M., Eyre, D. W., Morgan, M., Wyllie, D. H., Del Ojo Elias, C., et al. (2013). Predicting antimicrobial susceptibilities for Escherichia coli and Klebsiella pneumoniae isolates using whole genomic sequence data. J. Antimicrob. Chemother. 68, 2234–2244. doi: 10.1093/jac/dkt180
Sullivan, M. J., Petty, N. K., and Beatson, S. A. (2011). Easyfig: a genome comparison visualizer. Bioinformatics 27, 1009–1010. doi: 10.1093/bioinformatics/btr039
The OzFoodNet Working Group (2010). Monitoring the incidence and causes of diseases potentially transmitted by food in Australia: annual report of the OzFoodNet network, 2010. Commun. Dis. Intell. 36, E213–E241.
The OzFoodNet Working Group (2011). Monitoring the incidence and causes of diseases potentially transmitted by food in Australia: annual report of the OzFoodNet Network, 2011. Commun. Dis. Intell. 39, E236–E264.
Thompson, J. D., Higgins, D. G., and Gibson, T. J. (1994). CLUSTAL W: improving the sensitivity of progressive multiple sequence alignment through sequence weighting, position-specific gap penalties and weight matrix choice. Nucleic Acids Res. 22, 4673–4680. doi: 10.1093/nar/22.22.4673
Tyson, G. H., McDermott, P. F., Li, C., Chen, Y., Tadesse, D. A., Mukherjee, S., et al. (2015). WGS accurately predicts antimicrobial resistance in Escherichia coli. J. Antimicrob. Chemother. 70, 2763–2769. doi: 10.1093/jac/dkv186
Utrarachkij, F., Nakajima, C., Changkwanyeun, R., Siripanichgon, K., Kongsoi, S., Pornruangwong, S., et al. (2017). Quinolone resistance determinants of clinical Salmonella enteritidis in Thailand. Microb. Drug Resist. 23, 885–894. doi: 10.1089/mdr.2015.0234
Vital, P. G., Caballes, M. B. D., and Rivera, W. L. (2017). Antimicrobial resistance in Escherichia coli and Salmonella spp. isolates from fresh produce and the impact to food safety. J. Environ. Sci. Health 52, 683–689. doi: 10.1080/03601234.2017.1331676
Vonaesch, P., Sellin, M. E., Cardini, S., Singh, V., Barthel, M., and Hardt, W. D. (2014). The Salmonella typhimurium effector protein SopE transiently localizes to the early SCV and contributes to intracellular replication. Cell Microbiol. 16, 1723–1735. doi: 10.1111/cmi.12333
WHO (2001). WHO Global Strategy for the Containment of Antimicrobial Resistance. Geneva: World Health Organisation.
WHO (2017). Global Priority List of Antibiotic-Resistant Bacteria to Guide Research, Discovery, and Development of New Antibiotics. Geneva: World Health Organisation.
Williamson, D. A., Lane, C. R., Easton, M., Valcanis, M., Strachan, J., Veitch, M. G., et al. (2018). Increasing antimicrobial resistance in nontyphoidal Salmonella isolates in Australia from 1979 to 2015. Antimicrob. Agents Chemother. 62:e02012-17. doi: 10.1128/AAC.02012-17
Wilson, A., Gray, J., Chandry, P. S., and Fox, E. M. (2018). Phenotypic and genotypic analysis of antimicrobial resistance among Listeria monocytogenes isolated from Australian food production chains. Genes 9, 80–93. doi: 10.3390/genes9020080
Yu, Z., Gunn, L., Wall, P., and Fanning, S. (2017). Antimicrobial resistance and its association with tolerance to heavy metals in agriculture production. Food Microbiol. 64, 23–32. doi: 10.1016/j.fm.2016.12.009
Zankari, E., Hasman, H., Kaas, R. S., Seyfarth, A. M., Agersø, Y., Lund, O., et al. (2013). Genotyping using whole-genome sequencing is a realistic alternative to surveillance based on phenotypic antimicrobial susceptibility testing. J. Antimicrob. Chemother. 68, 771–777. doi: 10.1093/jac/dks496
Zhang, Y., Gu, A. Z., He, M., Li, D., and Chen, J. (2017). Subinhibitory concentrations of disinfectants promote the horizontal transfer of multidrug resistance genes within and across genera. Environ. Sci. Technol. 51, 570–580. doi: 10.1021/acs.est.6b03132
Keywords: Salmonella enterica, antibiotic resistance, heavy metals, Australia, next generation sequencing, blaTEM–1, tetA, biocides
Citation: Wilson A, Fox EM, Fegan N and Kurtböke DÍ (2019) Comparative Genomics and Phenotypic Investigations Into Antibiotic, Heavy Metal, and Disinfectant Susceptibilities of Salmonella enterica Strains Isolated in Australia. Front. Microbiol. 10:1620. doi: 10.3389/fmicb.2019.01620
Received: 29 January 2019; Accepted: 01 July 2019;
Published: 16 July 2019.
Edited by:
Giovanna Suzzi, University of Teramo, ItalyReviewed by:
Filip Boyen, Ghent University, BelgiumAlexander Van Parys, Ghent University, Belgium
Elin Verbrugghe, Ghent University, Belgium
Copyright © 2019 Wilson, Fox, Fegan and Kurtböke. This is an open-access article distributed under the terms of the Creative Commons Attribution License (CC BY). The use, distribution or reproduction in other forums is permitted, provided the original author(s) and the copyright owner(s) are credited and that the original publication in this journal is cited, in accordance with accepted academic practice. No use, distribution or reproduction is permitted which does not comply with these terms.
*Correspondence: Annaleise Wilson, Annaleise.wilson@csiro.au