- 1Institute of Molecular Biosciences, Goethe University Frankfurt, Frankfurt, Germany
- 2Institute of Ecology, Evolution and Diversity, Goethe University Frankfurt, Frankfurt, Germany
- 3Swiss Federal Research Institute for Forest, Snow and Landscape Research (WSL), Birmensdorf, Switzerland
- 4UMR 5175 CEFE – CNRS – Université de Montpellier – Université Paul Valéry Montpellier – EPHE, INSERM, Montpellier, France
- 5Institut National de la Recherche Agronomique, Unité Mixte de Recherche 1136 INRA – Université de Lorraine, Interactions Arbres/Microorganismes, Centre INRA-Grand Est-Nancy, Champenoux, France
- 6National Research Council (CNR), Institute of Biosciences and Bioresources, Division of Perugia, Perugia, Italy
Truffle fungi are well known for their enticing aromas partially emitted by microbes colonizing truffle fruiting bodies. The identity and diversity of these microbes remain poorly investigated, because few studies have determined truffle-associated bacterial communities while considering only a small number of fruiting bodies. Hence, the factors driving the assembly of truffle microbiomes are yet to be elucidated. Here we investigated the bacterial community structure of more than 50 fruiting bodies of the black truffle Tuber aestivum in one French and one Swiss orchard using 16S rRNA gene amplicon high-throughput sequencing. Bacterial communities from truffles collected in both orchards shared their main dominant taxa: while 60% of fruiting bodies were dominated by α-Proteobacteria, in some cases the β-Proteobacteria or the Sphingobacteriia classes were the most abundant, suggesting that specific factors (i.e., truffle maturation and soil properties) shape differently truffle-associated microbiomes. We further attempted to assess the influence in truffle microbiome variation of factors related to collection season, truffle mating type, degree of maturation, and location within the truffle orchards. These factors had differential effects between the two truffle orchards, with season being the strongest predictor of community variation in the French orchard, and spatial location in the Swiss one. Surprisingly, genotype and fruiting body maturation did not have a significant effect on microbial community composition. In summary, our results show, regardless of the geographical location considered, the existence of heterogeneous bacterial communities within T. aestivum fruiting bodies that are dominated by three bacterial classes. They also indicate that factors shaping microbial communities within truffle fruiting bodies differ across local conditions.
Introduction
Truffles are ascomycete ectomycorrhizal fungi that associate with the roots of a large number of trees and shrubs and that produce hypogeous fruiting bodies. Some truffle species such as Tuber melanosporum (Périgord black truffle) and T. aestivum (Burgundy truffle) are renowned worldwide for their delicate aroma and are considered as culinary delicacies. Although these truffles can be harvested in wild forests, over 80% of the truffles harvested in France are nowadays originating from artificially inoculated orchards (Murat, 2015). In this context, the controlled production of truffles is an economically important goal of research. Major progress has been made over the past 30 years to improve methods of truffle cultivation and to better understand the life cycle of these peculiar fungi (Paolocci et al., 2006; Rubini et al., 2007, 2011a, b; Murat, 2015; Molinier et al., 2016). The most comprehensive knowledge about truffle biology exist about T. melanosporum (Rubini et al., 2014; Le Tacon et al., 2016; Selosse et al., 2017) which was the first Tuber genome to be sequenced (Martin et al., 2010). However, mounting evidence based on genetics of T. aestivum (Molinier et al., 2013a, b, 2016) and the genomics of T. aestivum and T. magnatum suggests high similarities in terms of life cycle to T. melanosporum (Murat et al., 2018). In truffles, the life cycle starts with the germination of haploid spores. Hyphae produced from germinated spores colonize the fine roots of host plants and form ectomycorrhizae. This symbiotic mixed organ is the place of nutrients exchange between the two mutualistic partners (Smith and Read, 2008). Ectomycorrhizae also provide the maternal mycelium that will give birth to the fruiting body (or ascocarp) after mating with a paternal gamete of opposite mating type (Rubini et al., 2014; Selosse et al., 2017, Murat et al., 2018). In contrast to many other ectomycorrhizal fungi that produce fruiting bodies within a few days, the development of truffle fruiting bodies generally takes several months and occurs entirely belowground. In the case of T. melanosporum, it has been demonstrated that nutrients required for the development of the fruiting bodies are provided by the host plant all along fruiting body genesis (Le Tacon et al., 2013, 2015) and a similar process likely occurs for T. aestivum (Deveau et al., 2019). The production of fruiting bodies in all Tuber species varies greatly from year to year, ranging from none to several per tree. Additionally, considering trees with a sufficient degree of mycorrhization with T. aestivum or T. melanosporum, the yield of harvested truffles was shown to be unrelated to the host tree mycorrhization degree (Molinier et al., 2013a; De la Varga et al., 2017).
Beside the symbiotic association between the fungus and its host, it is now clear that complex microbial communities interact with truffle fungi both in the ectomycorrhizosphere and in the ascocarp. Based on a number of studies on truffle-associated bacterial communities, we know that the surface (peridium) and the inner tissues (gleba) of truffle fruiting bodies are colonized by complex bacterial communities composed of a few hundreds of species that can reach up to 107–108 cells per gram of truffle (Barbieri et al., 2007; Antony-Babu et al., 2014; Vahdatzadeh et al., 2015). The effects of these bacteria and of their interactions on the biology of truffles are still poorly understood. Yet, some bacteria have been shown to participate in the elaboration of some of the volatile organic compounds produced by the whitish truffle Tuber borchii (Splivallo et al., 2015), and it has been hypothesized that bacteria could be involved in the elaboration of the complex aroma of truffles (Vahdatzadeh et al., 2015). In addition, some bacteria of the Bradyrhizobiaceae family isolated from T. magnatum have shown the ability to fix nitrogen (Barbieri et al., 2010). It has been proposed that they could participate in the nutrition of the fungus during fruiting body development (Barbieri et al., 2010). Additional putative effects such as inhibition of pathogenic fungi, stimulation of the growth of Tuber mycelium, and ascocarp degradation have also been suggested based on potential functional activities of bacteria isolated from fruiting bodies (Citterio et al., 2001; Sbrana et al., 2002; Dominguez et al., 2012; Gryndler et al., 2013, 2015; Antony-Babu et al., 2014; Saidi et al., 2015; Deveau et al., 2016).
Despite differences between truffle species (Benucci and Bonito, 2016), the truffle microbiome is commonly dominated by bacteria belonging to the Rhizobiales order together with, to a lesser extent, members of the orders Actinomycetales, Burkholderiales, Enterobacteriales, Flavobacteriales, and Pseudomonadales (Barbieri et al., 2016). Yet, important variations in the composition of truffle microbiomes have been reported (Barbieri et al., 2016). Part of the discrepancies may be explained by the evolution of methodologies used to study microbial diversity, which cover from culture-dependent to various generations of culture-free methodologies (Sbrana et al., 2002; Barbieri et al., 2010; Deveau et al., 2016). Another part of this variability could be due to natural variation in microbiome composition among fruiting bodies of single Tuber species. Among the different factors that could influence truffle microbiome composition, the level of fruiting body maturation has been proposed as a potential driver of the microbiome composition in T. borchii, T. indicum, and T. melanosporum (Citterio et al., 2001; Antony-Babu et al., 2014; Splivallo et al., 2015; Ye et al., 2018). However, the extent to which other intrinsic (i.e., maturity, genotype, mating type) and extrinsic (i.e., season, location, spatial distance) factors drive the truffle microbiome is not known.
In this study, we filled this gap in knowledge by analyzing and comparing the microbiomes of more than 50 fruiting bodies of T. aestivum harvested over several years in two spatially distant orchards in Europe. T. aestivum is harvested and cultivated in numerous regions of the world (i.e., all over Europe, in Iran, Northern Africa) and its microbiome has not been extensively studied despite the fact that it represents one of the most relevant truffles in terms of traded volumes. We hypothesized that the microbial communities of T. aestivum would be dominated by bacteria of the Bradyrhizobiaceae family as in other truffle species but also that noticeable differences in microbial assemblages would be detectable between the two study sites due to variable environmental factors. To test and answer those hypotheses, (1) the “core” composition of the T. aestivum microbiome in both study sites was defined, (2) the variability in the truffle microbiome across orchards was assessed, and (3) the intrinsic factors (maturity, genotype, mating type) and extrinsic ones (season, location, spatial distance) determining the assembly of truffle-associated bacterial communities were evaluated.
Materials and Methods
Biological Material and Sampling
Fruiting bodies of Tuber aestivum (Vittad.) were collected from two artificially inoculated truffle orchards in France (FR) and Switzerland (SW). Exact GPS coordinates are not given here at the request of the orchard’s owners, but the closest city nearby is provided as an approximate location. These orchards have been described earlier (Splivallo et al., 2012; Molinier et al., 2013a, 2015). The French orchard, located near Daix/Dijon (FR), is a 30-year old truffle orchard that comprises two rows of inoculated hazels (Corylus avellana) at its center and two outer rows of fruit trees on the outer margins (see for details Molinier et al., 2013a). All hazel trees in the French orchard were inoculated with T. melanosporum in 1976 and produced T. melanosporum fruiting bodies for nine seasons from 1980 to 1989 (a few hundred grams to 12 kg per year; Molinier et al., 2013a). During 1990–1993 production was gradually and eventually fully replaced by native T. aestivum and in subsequent years, production ranged from a few hundred grams to a few kilograms of T. aestivum per year (Molinier et al., 2013a). The soil of the French truffle orchard has a calcareous nature and a pH of 7.9 (Molinier et al., 2013a). The Swiss orchard, located in Valais, near St-Triphon (CH), contains 42 trees – oaks (Quercus robur) and pines (Pinus nigra) that were commercially inoculated with T. aestivum and planted in 1999. In this orchard, pH of the soil is 7.6 and production of T. aestivum started in 2008/2009 and ranged since then to a few hundred grams to approximately 1 kg per year.
A total of 62 T. aestivum fruiting bodies were collected from the two artificially inoculated truffle orchards. Seventeen truffles were collected in the French orchard in 2010 and 2011, whereas 45 truffles were collected from the Swiss orchard during four consecutive years (2009–2012). The precise location of truffles was recorded at the time of the harvest (Figure 1). To avoid post-harvest drifts of microbial populations, all truffles were cooled to 4°C after collection and frozen to −20°C within 24 h for subsequent DNA extraction.
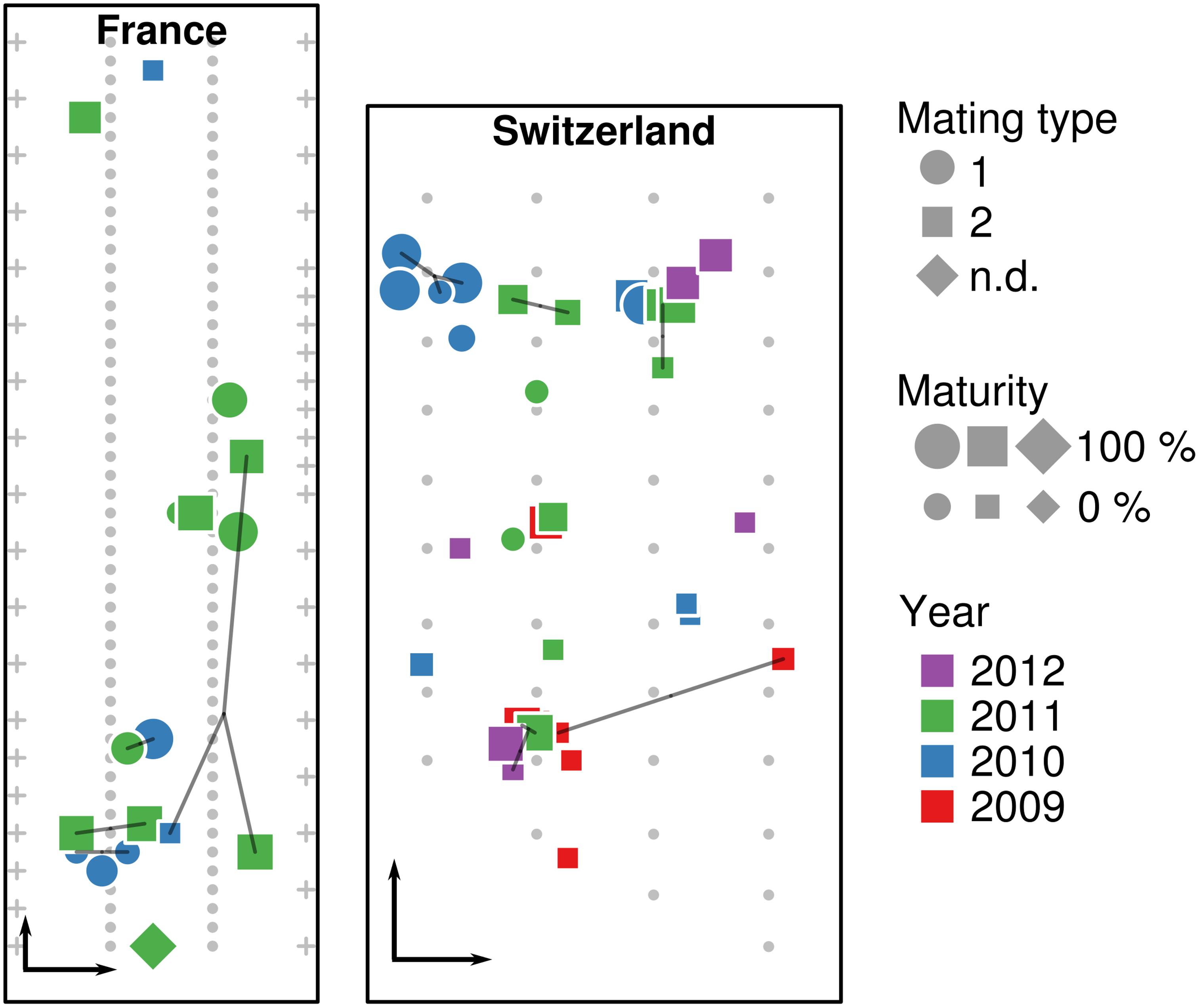
Figure 1. Location of truffle samples within the French and Swiss orchards. Location of truffles, their maturity, mating type, collection year, and identical multilocus genotypes (MLGs, connected by lines) are shown in the French and Swiss orchards, along the position of truffle-mycorrhized trees (small gray dots) and fruit trees surrounding the orchard (crosses). Black arrows in the lower left corner of each orchard represent a distance of 5 m.
Truffles were identified by spore morphology and via molecular methods (see the section “DNA Extraction and Truffle Genotyping”). The stage of fruiting body maturation was determined by estimating the percentage of ascii containing melanized spores, as previously described (Splivallo et al., 2012). An overview of the samples used in this study along with the analyses performed is shown in Table 1.
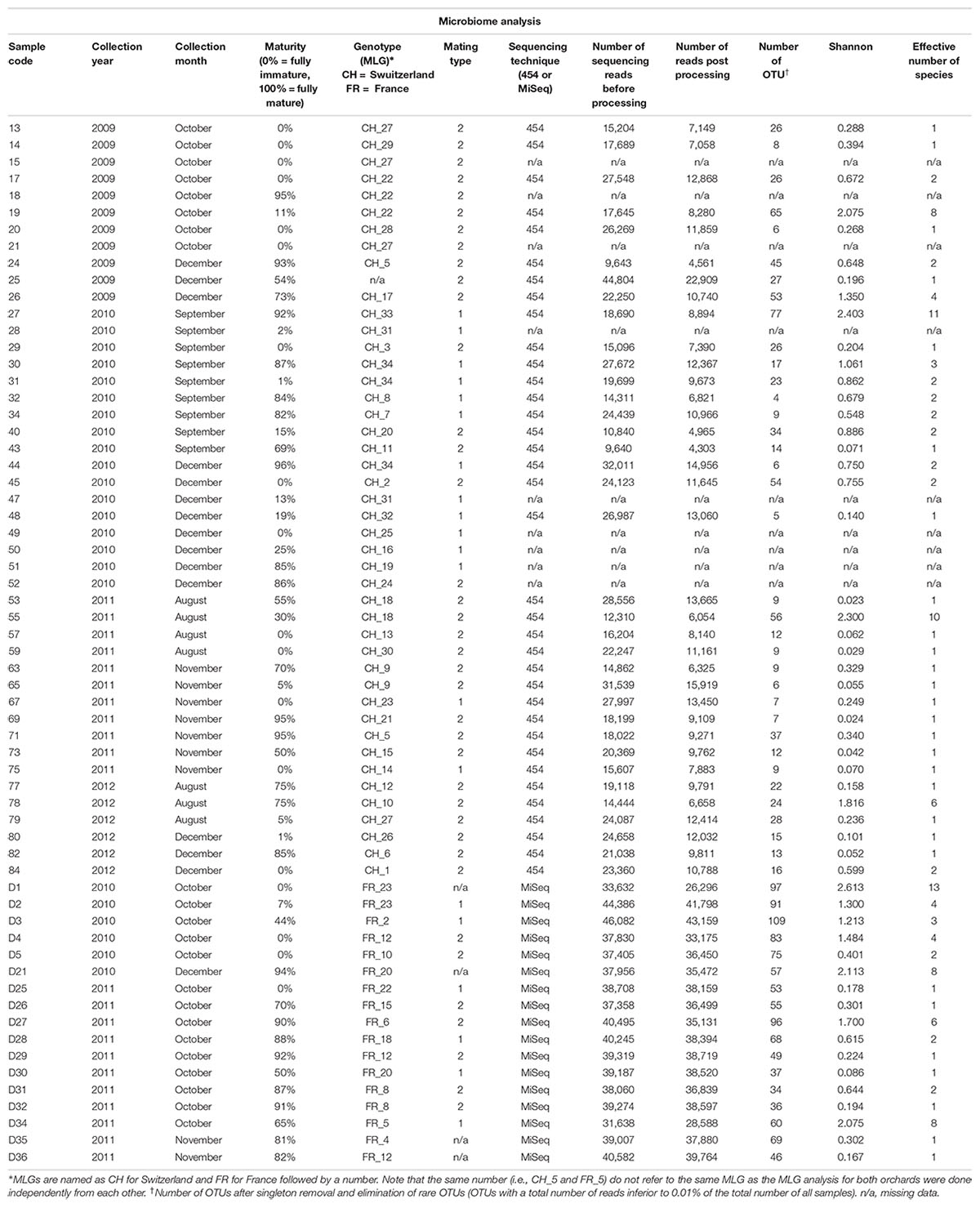
Table 1. Details of the Tuber aestivum samples originating from the Swiss and French orchard, and results of bacterial microbiome analysis.
DNA Extraction and Truffles Genotyping
Genomic DNA was extracted from the gleba (50–100 mg fresh weight excised from the central part of the gleba) of each fruiting body using the DNeasy® Plant Mini Kit (Qiagen, Hilden, Germany) following the manufacturer’s instructions. Even though this kit might have been used here for characterizing truffle’s microbiome for the first time, earlier works have demonstrated that various DNA extraction methods yielded comparable microbiome compositions for different truffle species (Antony-Babu et al., 2013; Benucci and Bonito, 2016). DNA qualities and concentrations were checked using a NanoDrop spectrometer and gel electrophoresis. Mating type identification was performed using the specific primers aest-MAT1-1f/aest-MAT1-1r and aest-MAT1-2f/aest-MAT1-2r as described elsewhere via multiplex polymerase chain reaction (PCR) (Molinier et al., 2016). In short, PCRs were carried out using 3 μl of template DNA (diluted 10 times) in a final volume of 20 μl containing 10 μl of JumpStart REDTaq ReadyMix (Sigma-Aldrich: P1107), 0.4 μl of each primer (0.2 μM each), and water to adjust to the final volume. Thermal cycles were conducted using the following program: an initial denaturation of 2 min at 94°C, 28 cycles at 94°C for 30 s, 57°C for 30 s, and 72°C for 1 min, followed by 72°C for 7 min. PCR products were checked on a 1.5% agarose gel and visualized after ethidium bromide staining by a UV transilluminator.
A total number of 14 SSR loci (aest01, aest06, aest07, aest10, aest15, aest18, aest24, aest25, aest26, aest28, aest29, aest31, aest35, and aest36) (Molinier et al., 2013b) were chosen for genotyping. The genotyping procedure followed that described by Molinier et al. (2016) but with a slightly modified PCR program: one cycle of 15 min at 95°C, 30 cycles of 30 s at 94°C, 90 s at 60°C, and 60 s at 72°C, and a final elongation cycle of 30 min at 60°C. To identify multilocus genotypes (MLGs) and true clones based on the 14 SSR markers, the software MLGSIM (Stenberg et al., 2003) was used as described elsewhere (Molinier et al., 2016).
Microbiome Analysis
Bacterial communities of Swiss fruiting bodies were analyzed by 454 pyrosequencing, while French samples were analyzed by MiSeq Illumina sequencing, because 454 pyrosequencing technique was no longer available at the time of the analysis. In both cases, the isolated DNA from the gleba of fruiting bodies was used to generate 16S rRNA gene amplicon libraries using the primers 787r (ATTAGATACCYTGTAGTCC) (Nadkarni et al., 2002) and 1073F (ACGAGCTGACGACARCCATG) (On et al., 1998), modified to include specific linkers and identification barcode sequences for the respective sequencing method. The same procedure as described by Antony-Babu et al. (2014) was used to generate 454 pyrosequencing amplicons. Briefly, the PCRs contained 10 μl of PCR Mastermix (5 PRIME), 1 μl of each forward and reverse primers (each 0.2 μM), and 2 μl of template DNA (or sterile water for negative control) in a final volume of 25 μl. For each truffle DNA sample, amplifications were performed in three parallel PCR tubes under the following conditions: an initial denaturation at 94°C for 10 min followed by 30 cycles of denaturation at 94°C for 30 s, annealing at 48°C for 45 s, extension at 72°C for 90 s, and a final extension at 72°C for 10 min. The three PCR products were pooled and quantified by gel electrophoresis and an equimolar mix of amplicons was used for pyrosequencing. Amplicon sequencing was performed by the GS-FLX 454 Titanium platform of Beckman Coulter Genomics (Danvers, MA, United States). Illumina MiSeq amplicons were produced using the same amplification protocol except that the identification barcode sequences were added through a second round of amplification as described by Barret et al. (2015). PCR cycling conditions were 94°C for 2 min, followed by 12 cycles of amplification at 94°C for 1 min, 55°C for 1 min, and 68°C for 1 min each, and a final extension step at 68°C for 10 min. All amplicons were purified with the Agincourt AMPure XP system and quantified with QuantIT PicoGreen. The purified amplicons were then pooled in equimolar concentrations, and the final concentration of the library was determined using a quantitative PCR (qPCR) next-generation sequencing (NGS) library quantification kit (Kapa Biosystems, Boston, MA, United States). Amplicon libraries were mixed with 10% PhiX control according to the 2 × 250 bp Illumina protocols. The second round of PCRs, the purification steps, and sequencing was performed by the GeT PLAGE sequencing platform according to standard procedures (INRA Toulouse). The standard procedure to generate libraries for Illumina Miseq is available here: https://support.illumina.com/documents/documentation/chemistry_documentation/16s/16s-metagenomic-library-prep-guide-15044223-b.pdf.
Both 454 pyrosequencing and MiSeq Illumina 16S rRNA sequences were analyzed using FROGS (Escudié et al., 2018). After quality control and demultiplexing, sequences were preprocessed by removing primers from sequences, sequences out of the amplicon size range (250–300 bp), sequences with only one primer, with at least one homopolymer longer than 7 bp and a Phred quality score <10, and replicates of identical sequences. For the MiSeq Illumina run, 16S rRNA paired-end sequences were first merged (289 bp). Sequences were clustered into operational taxonomic units (OTUs) at 97% sequence similarity based on the iterative Swarm algorithm, with subsequent removal of chimeras for further analysis. Taxonomy assignment to each cluster was carried out by BLAST comparisons against the SILVA database and using the RDP Classifier (Ribosomal Database Project; Cole et al., 2009). OTUs with poor affiliation or higher abundance in negative controls than samples were deleted for further analysis. Finally, OTUs with a total number of reads inferior to 0.01% of the total number of all samples were discarded. The raw data are deposited in the NCBI Sequence Read Archive website1 under the BioProject study accession number PRJNA506316 and the SRA accession numbers SRX5059925–SRX5059959 (454 sequences) and SRX5073276–SRX5073292 (Illumina sequences).
Statistical Analyses
All statistical analyses were performed in R (R Core Team, 2017) with the aid of relevant packages. The datasets from FR and SW were processed independently, but using the same procedure. The datasets were only combined to generate a joint heat tree using the R package Metacoder v0.1.3 (Foster et al., 2017), to summarize the overall taxonomic composition obtained and compare the relative proportion of taxa between both studies. Differences between sites in the relative abundances of the main bacterial taxa were assessed via one-way ANOVA followed by Tukey’s HSD post hoc test, after verifying normality of data with Shapiro–Wilk test. Overall and per-sample rarefaction curves were calculated in each dataset to assess sampling completeness, using function rarecurve() in package Vegan v3.5-1 (Oksanen et al., 2015). Based on these, subsequent analyses of diversity and community structure were performed on datasets where samples had been rarefied with the Phyloseq package (McMurdie and Holmes, 2013) to achieve equal read numbers of 26,295 for the FR dataset and 3,855 for the SW dataset. Rarefaction curves were used to verify that the subsampling was performed as close as possible to the asymptotes to allow comparison between samples in both French and Swiss sites (Supplementary Figure 1). Values of OTU richness and diversity based on Shannon’s index were calculated using functions available in Vegan. Effective numbers of species were calculated using Simpson index as proposed by Jost (2006).
Non-metric multidimensional scaling (NMDS) was used to visualize differences in community composition among samples. NMDS is an ordination method that represents pairwise (dis)similarities between samples in a low-dimensional space, so that samples placed closer in the graph are more similar than those further apart (Clarke, 1993; Legendre and Legendre, 2012). NMDSs were based on Bray–Curtis dissimilarities calculated among samples after a Hellinger transformation of data (Legendre and Gallagher, 2001).
We investigated the potential influence of factors on microbiome variation using variation partitioning based on distance-based redundancy analysis (db-RDA; Borcard et al., 2011; Legendre and Legendre, 2012), with the Hellinger-transformed dissimilarity matrix as response variable. db-RDA is a constrained ordination method in which a matrix of pairwise (dis)similarities between samples is modeled as a function of a set of explanatory variables (Legendre and Legendre, 2012). Variation partitioning can then be applied to measure the relative contribution of individual explanatory variables to overall community variation while accounting for the effects of other variables, by sequentially removing components from the db-RDA model and recording the resulting changes in the total variance explained.
The explanatory variables to be included in the variation partitioning analysis were selected, when possible, by means of permutational analysis of variance (PERMANOVA; Anderson, 2001), so that only those with a significant correlation with community variation (P < 0.05) in at least one dataset were retained. The potential influence on community structure of spatial distance among samples was first examined using Mantel correlograms (Legendre and Legendre, 2012), which enable to test whether samples that are spatially close are more similar than those farther apart. Then, to allow their inclusion in the variation partitioning analysis, the spatial relationships were summarized as principal coordinates of neighbor matrices (PCNM) vectors (Legendre and Legendre, 2012), calculated from the coordinates of each sample within the orchard using the function pcnm() of vegan. PCNMs describe non-random patterns in dissimilarity matrices at different scales, which can then be used to model potential sources or variation not accounted for by the measured explanatory variables, such as dispersal, species interactions, or historical causes (Peres-Neto and Legendre, 2010). PCNM vectors significantly associated with community variation in our datasets were forward-selected using package Packfor v0.0-8 (Dray et al., 2009). Because no PCNMs were significantly correlated with the FR dataset, in this case, we manually selected the first four to match the number of PCNMs retained for SW. As done with spatial distances among samples, the factor truffle genotype was assessed by summarizing Euclidean distances among SSR profiles with PCNM vectors and testing their association with microbiome variation by forward selection. After the selection of factors, the final db-RDA models included as explanatory variables truffle mating type, degree of maturity, year of collection, and spatial distance. Truffle maturity and SSR genotypes were excluded because they did not explain an important nor significant amount of microbiome variation in any location.
Results
High-Throughput Sequencing
A total of 661,164 and 757,177 quality-passed sequences were obtained for the French (Illumina sequencing) and Swiss (454 sequencing) orchards, respectively, with averages of 38,892 (±807 SE) and 21,033 (±1,209) reads per sample (Table 1). After quality filtering and removal of chimeric reads, a total of 623,440 and 362,697 sequences were retained, with an average of 36,673 (±1,023) and 10,075 (±610) reads per sample across the French and Swiss samples, respectively (Table 1). After taxonomy assignment, elimination of contaminants and of OTUs present in <0.01% of the total number of reads (128 and 1,177 OTUs, respectively), 183 and 147 OTUs were considered for further analyses in the French and Swiss samples, respectively.
Truffles From Two Distant Orchards Have Similar Microbiomes
We first compared the microbiome composition of truffles collected from the French and Swiss orchards. An average of 66 ± 6 (SE) OTUs were detected in the French samples, while 23 ± 3 were recorded in average in the Swiss ones. This important difference could be a bias due to the use of MiSeq Illumina sequencing (French orchard) and 454 pyrosequencing (Swiss orchard). Indeed, Illumina sequencing allows for larger numbers of reads per sample and may provide a better access to rare OTUs. This hypothesis was confirmed on another set of data obtained from T. melanosporum fruiting bodies analyzed both by 454 and MiSeq Illumina sequencing (Deveau et al., unpublished data). The two samples that were analyzed by both methods strongly differed in richness (21 vs. 98 OTUs for 454 and Illumina MiSeq, respectively) but the relative abundance of the dominant genera that were found in this study was similar no matter which methodology was used (Supplementary Figure 2). In accordance with this observation and despite the two different sequencing methods used, the general composition of the truffle microbiomes detected at each site was alike, as shown in Figure 2. In both locations, communities were dominated by OTUs affiliated to the α-Proteobacteria (FR: 67 ± 9% SE, CH: 63 ± 7%; P > 0.05) followed by closely related proportions of Bacteroidetes (FR: 9 ± 4%; CH: 14 ± 5%; P > 0.05), β-Proteobacteria (FR: 9 ± 6%, CH: 17 ± 5%; P > 0.05), and γ-Proteobacteria (FR: 10 ± 4%, CH: 4 ± 2%; P > 0.05). Overall, Actinobacteria (FR: 2.5 ± 0.7%, CH: 0.6 ± 0.2%; P < 0.01) and Firmicutes (FR: 2.7 ± 1.7%, CH: 0.1 ± 0.02%; P > 0.05) were less frequent, with Actinobacteria being the only phylum with a significantly different abundance between the two orchards. OTUs of d-Proteobacteria, Acidobacteria, and Verrucomicrobia were found at very low levels in some samples of the two sites. Strong similarities between the two orchard’s samples were also observed at the genus level, since the most represented OTUs belonged to the same genera: Bradyrhizobium (FR: 65.1 ± 8.8%, CH: 58.6 ± 6.9%), Pseudomonas (FR: 8.1 ± 3.4%, CH: 3.4 ± 1.4%), Pedobacter (FR: 4.3 ± 3.3%, CH: 13.8 ± 4.9%), Polaromonas (FR: 5.4 ± 5.0%, CH: 9.2 ± 4.4%), and Flavobacterium (FR: 2.5 ± 1.2%, CH: 0.8 ± 0.7%). Twenty-three additional genera were shared between the two datasets. This “core” microbiome contained genera belonging to five different Phyla (Supplementary Table 1). Differences nevertheless also existed between the two localities at the genera level as several dozens of genera were also specifically found on one of the two orchards. Yet it is here difficult to discriminate the part of sequencing methodology bias from true data.
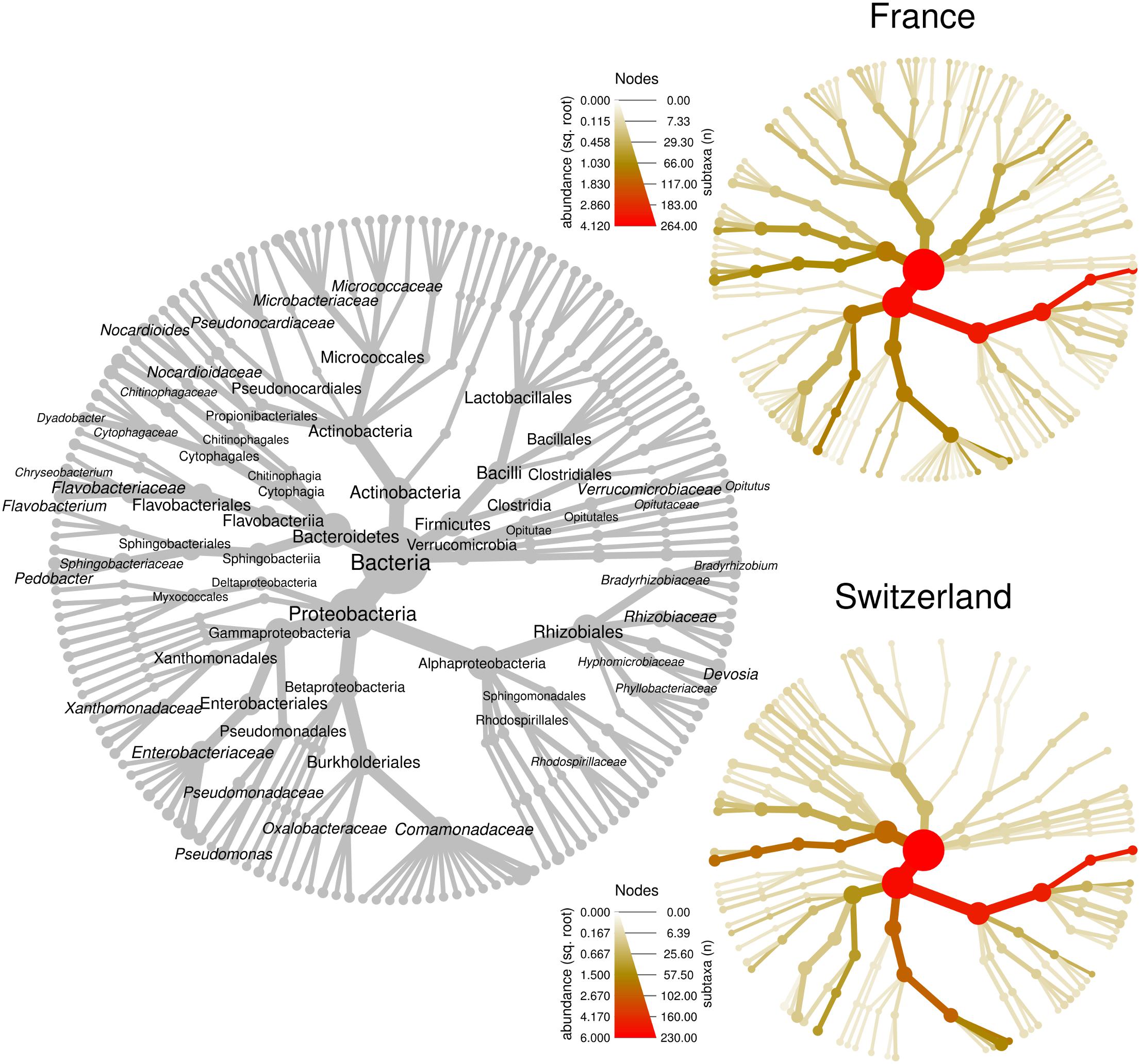
Figure 2. Bacterial community structure within T. aestivum fruiting bodies collected from two orchards. Each heat tree represents bacterial community structure as a taxonomic hierarchy up to genus level. The gray tree serves as a key for the smaller unlabeled trees, node labels highlight the most abundant taxa detected at both orchards. The smaller colored trees show community structure for each orchard, assessed with different amplicon sequencing technologies [MiSeq for France (FR), 454 for Switzerland (SW)]. Node and edge sizes are proportional to the number of OTUs within each taxon, whereas color represents taxon abundances (square root of read numbers).
α-, β-Proteobacteria, and Sphingobacteriia Dominate Single Fruiting Bodies
Having compared the overall microbiomes of the French and Swiss orchards, our next aim was to assess the variability in bacterial community structure and taxonomic composition among fruiting bodies. The bacterial community structure and composition was highly variable among single fruiting bodies collected within the same orchard. In both the French and Swiss orchards, the number of OTUs detected per truffle samples varied from a few OTUs to more than a hundred (Table 1). Such variation was independent from the sequencing depth obtained for each sample (Table 1). It is thus likely not due to a bias of sequencing depth but rather reflects different patterns of bacterial community structures, some truffles being colonized by a small number of species while others harbored a larger number of species. The evenness of the bacterial communities also deeply differed between samples in both orchards as illustrated by the strong variability of the Shannon and the effective species value (Table 1). While most truffle-associated bacterial communities were dominated by a few abundant OTUs and numerous rare OTUs, a few fruiting bodies of both sites showed more balanced patterns (data not shown). Such heterogeneity was also reflected when looking at the composition of the bacterial communities at different taxonomic levels (Figure 3). Overall, at the phylum level, 57% of the fruiting bodies showed communities dominated by α-Proteobacteria while 13% of the fruiting body communities were dominated by β-Proteobacteria, and 11% by Bacteroidetes. Eight percent of the fruiting bodies harbored balanced communities in which several phyla were co-dominants. A similar pattern was maintained at the genus level, with Bradyrhizobium (α-Proteobacteria), Polaromonas (β-Proteobacteria), and Pedobacter (Sphingobacteriia) being the most abundant genera depending on the fruiting body considered. To a lesser extent, OTUs from the Variovorax genus (β-Proteobacteria), Pseudomonas (g-Proteobacteria), Sphingomonas (α-Proteobacteria), and Flavobacterium (Flavobacteria) formed a significant part of the communities in some fruiting bodies. Thus, the large sampling effort realized over several years in the two truffle orchards revealed the existence of an unsuspected important variability in the composition of the microbiome of truffle ascocarps.
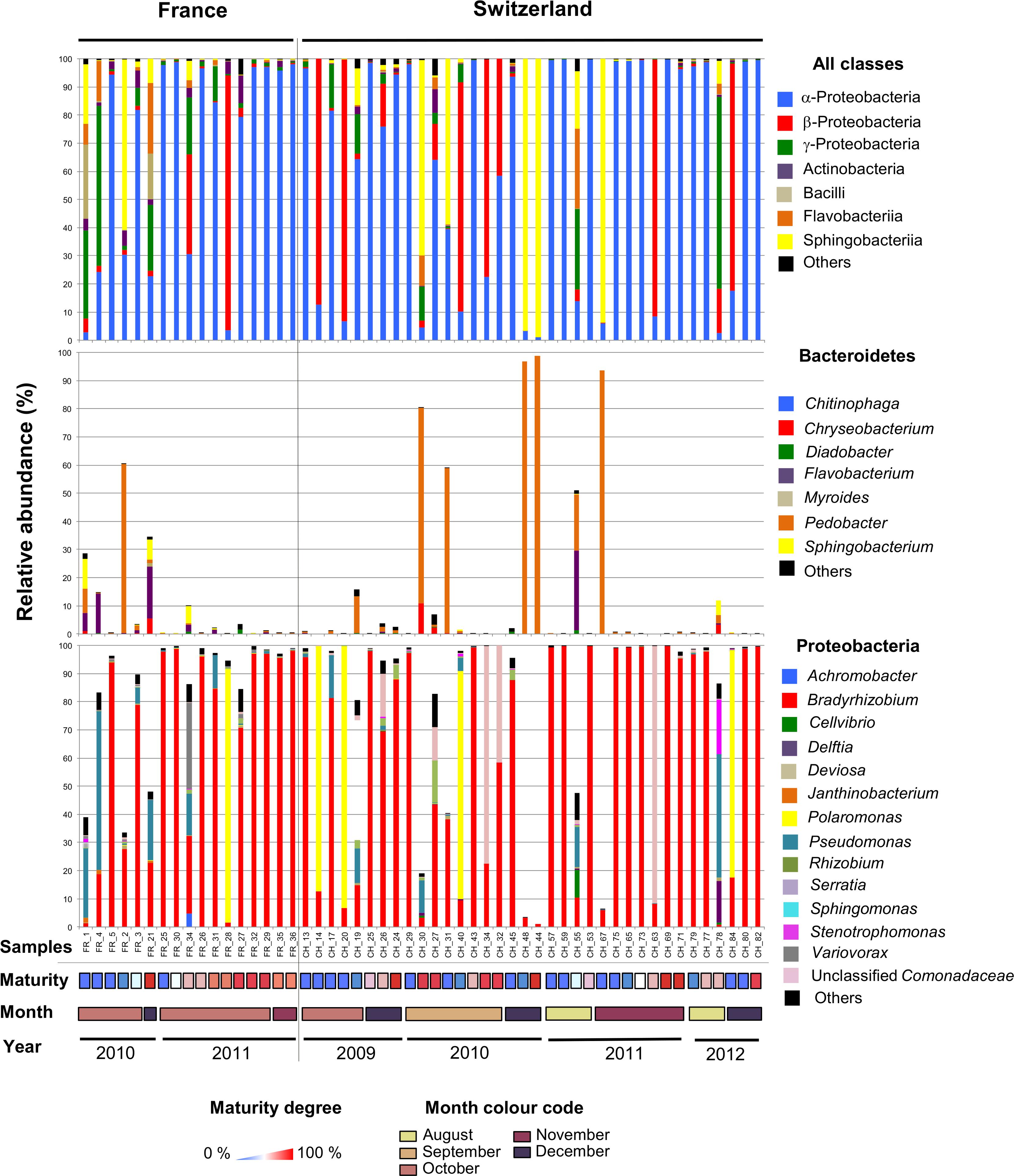
Figure 3. The microbiome of T. aestivum. Distribution of major bacterial classes (top panel) and genera (intermediate and bottom panels) in the French and Swiss truffle fruiting bodies analyzed here. Each column represents a single sample. For each sample, three pile-up plots are given: the relative distribution of reads among the major bacterial classes (top panel) and of the different genera forms the Bacteroidetes (intermediate panel) and Proteobacteria phyla (bottom panel). Samples were ordered according to the year and month of collection, and maturity degree (from low to high).
Mating Type and Multilocus Genotype Distribution of Truffle Fruiting Bodies Within the Orchards
Truffle fruiting bodies result from the fertilization of two individuals of opposite mating type (Martin et al., 2010; Rubini et al., 2011b). Whereas the truffle gleba (maternal tissue) is made up by one individual, the spores contain meiotic products of the two mating partners (Paolocci et al., 2006; Rubini et al., 2011b; Selosse et al., 2017). Here, we determined the genetic profile of the truffle gleba (maternal genotype) only, since the gleba harbors most of the truffle microbiome (Antony-Babu et al., 2014; Splivallo et al., 2015).
Genotyping of the truffles from the French orchard had been done in a previous study (Molinier et al., 2016). A large proportion of unique genotypes (i.e., genotypes that were recorded only once) was observed: eight truffles had unique genotypes and only two pairs with the same MLG (here FR12 and FR20) were detected over the 2010–2011 seasons (Table 1). Truffles of opposite mating types appeared to be evenly spread over the French orchard. In the Swiss orchard, out of the 44 fruiting bodies for which the MLG was identified, 26 had unique MLGs, whereas other MLGs were shared among the remaining 18 samples. In particular, four MLGs (namely CH_5, CH_9, CH_18, and CH_31) were shared between two individuals, two (CH_22 and CH_34) among three individuals, and only one (CH_27) among four individuals (Figure 1). In terms of collection season, five out of seven shared MLGs were found in truffles harvested in the same season, while only two MLGs were shared by truffles harvested 2–3 years apart. Last and by contrast to the French orchard, truffles of mating type 1 were strongly aggregated in one corner of the Swiss field, whereas the rest of the orchard was dominated by truffles of mating type 2 (Figure 1).
Different Factors Affect Truffle’s Microbial Communities in the French and Swiss Orchards
Having observed important differences in microbial community composition and structure within truffles of the same orchard, we explored whether this variability could be linked to a series of biotic and abiotic factors inherent to truffle ascocarps and to truffle orchards. Specifically, we considered seasonality, space (the location of truffles within an orchard), fruiting body genotype, mating type, and maturation as potential factors affecting the microbiome.
Non-metric multidimensional scaling was used to visualize pairwise dissimilarities between each truffle-associated microbiome and to explore their relationships with intrinsic (maturation, genotype, mating type) and extrinsic factors (collection season, year, or spatial distance) potentially explaining microbial community structure. NMDS ordinations based on Bray–Curtis dissimilarities among samples in each field showed no evident sample groupings related to truffle maturity, mating type, or MLGs (Figures 4A,B). However, in the Swiss orchard, mating type was significantly associated with microbiome variation based on PERMANOVA analysis (Figure 4B; F(1,35) = 4.6, Adj. R2 = 0.12, P < 0.002), whereas in the French field, a significant effect was found for the collecting year (F(1,16) = 3.2, Adj. R2 = 0.17, P = 0.016). Likewise, a different effect of spatial distance on bacterial communities was found in each site: whereas no association was found in the French orchard (Figure 4C), in SW, similarities among truffle microbial communities appeared to be significantly influenced by distance, with a strong aggregation pattern up to a distance of approximately 2 m (Figure 4D).
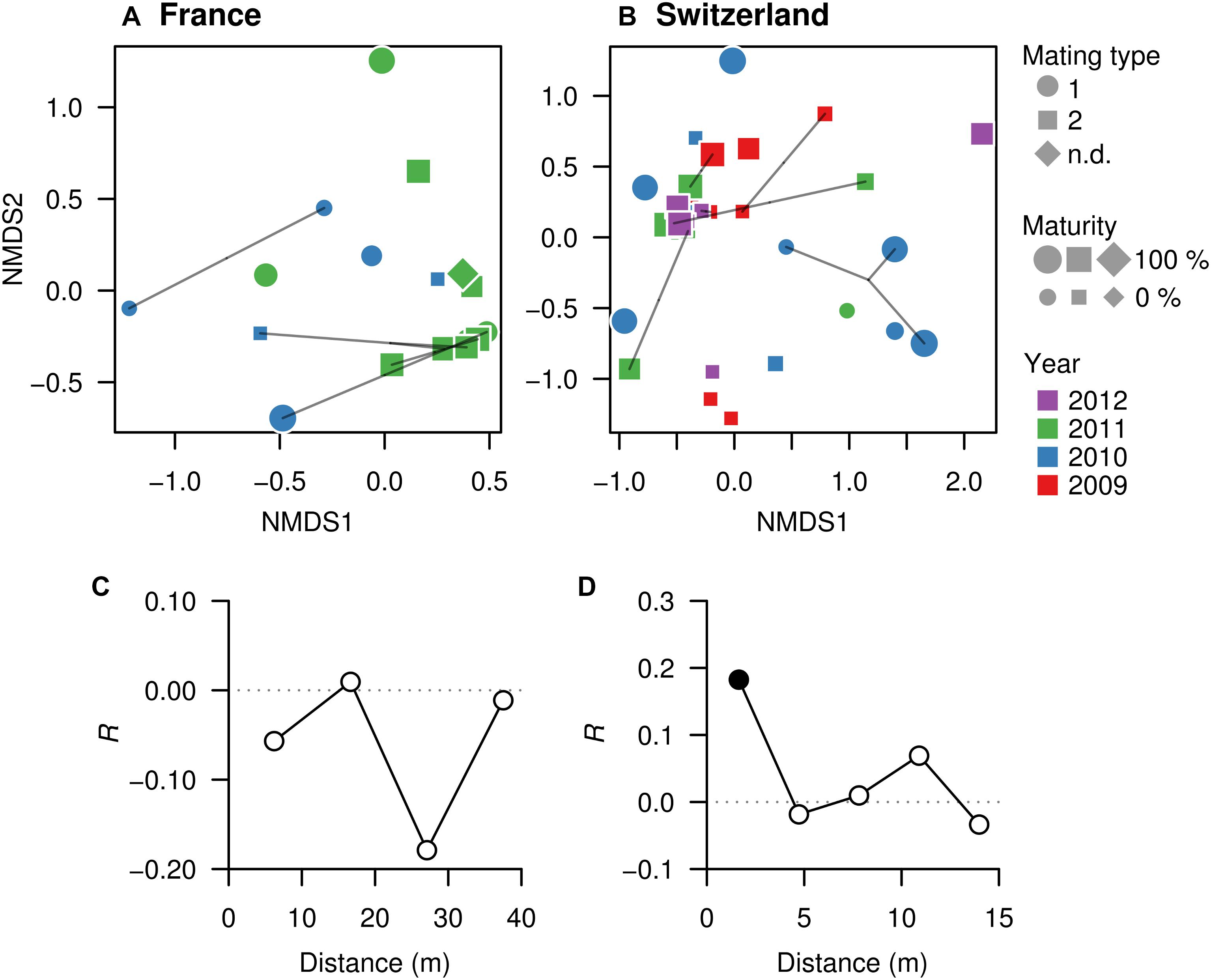
Figure 4. Microbial community similarities among truffles within orchards. (A, FR; B, SW) Non-metric multidimensional scaling (NMDS) ordinations based on Bray–Curtis dissimilarities of the OTU composition of microbiomes in truffle samples. The closer the samples, the more similar their microbiomes are. Different symbols denote mating type, symbol sizes represent maturity of fruiting bodies, and symbol colors represent collection year. Points linked with lines are fruiting bodies belonging to the same MLG. (C, FR; D, SW) Correlograms showing correlation of microbiome similarity among samples (y-axis, Mantel’s R) with spatial distance (x-axis). Solid and empty points denote significant (P < 0.05) and non-significant correlations for each distance class based on Bonferroni adjustment for multiple testing, indicating that space affects the truffles microbiomes in the Swiss but not the French site.
Distance-based redundancy analysis ordinations were applied to model the variation in truffle microbiomes in the French and Swiss orchards as a function of the explanatory variables that significantly influenced the microbiome: truffle mating type, year of collection, and spatial distribution of samples (PCNMs). Other factors (maturation and genotype) were excluded as they did not have a significant effect as demonstrated earlier (see also the section “Materials and Methods”). The db-RDAs models constrained by truffle mating type, year of collection, and spatial distribution explained 19% (F2 = 2.3, P = 0.002) and 18% (F2 = 1.5, P = 0.048) of overall community variation for the Swiss and French orchards, respectively. In the Swiss orchard, the associations of community structure with mating type and spatial factors previously reported were confirmed (Figure 4B), with spatial distance and mating type explaining an overall 18 and 9% of total variation, respectively, after accounting for the effects of other variables (Table 2). These values contrasted with a comparably low contribution (1.0%) of collection year (Table 2). In FR, the only variable with a significant correlation with microbiome structure was the collection year, with an overall 11% (P = 0.010) of the variance explained (Table 2).
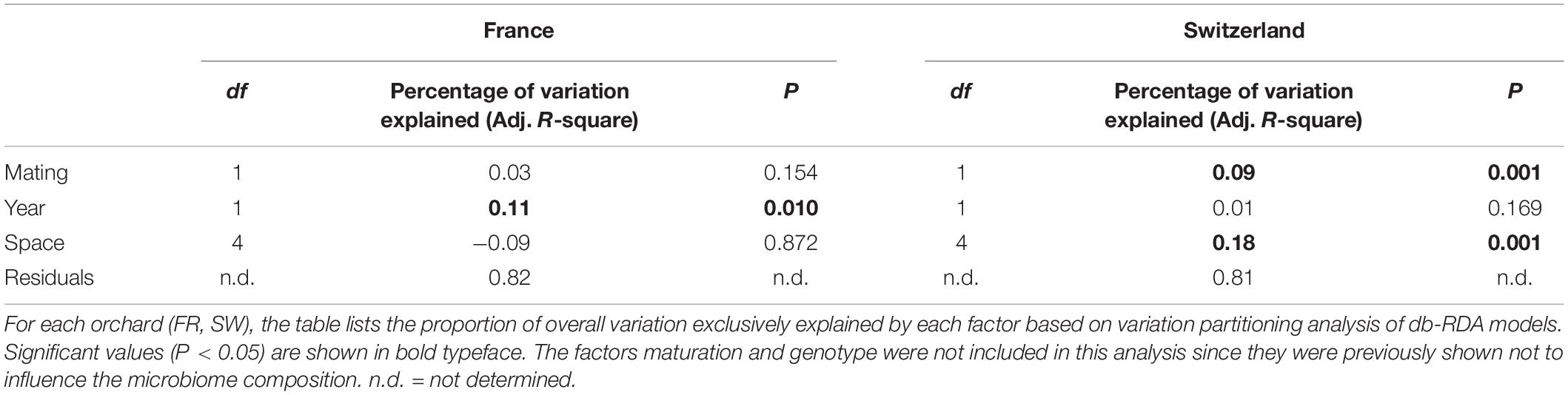
Table 2. Variation in microbial community composition explained by mating, year of collection, and space.
Discussion
Host-associated microbiomes are important for the nutrition and health of their hosts: plants, animals, and macrofungi are extensively colonized by microorganisms that play key roles in their life cycles (Berg et al., 2014; Bahrndorff et al., 2016; Webster and Thomas, 2016; Pent et al., 2018). Studies on animals and plants have revealed that host identity, genotype, and environmental variables all contribute to shaping the microbial communities colonizing eukaryotic tissues (Bulgarelli et al., 2012; Lundberg et al., 2012; Bouffaud et al., 2014; Hacquard et al., 2015; Glynou et al., 2016), but the relative importance of each factor varies depending on the host and on the type of environment. Fungi also host complex microbial communities that can associate to various fungal structures (i.e., mycorrhizas, mycelium, fruiting bodies) and colonize either the surface of hyphae or the intracellular compartments (Deveau et al., 2018). However, the factors that drive the assembly of these communities are poorly documented. A recent study on the microbiome structure of the epigeous fruiting bodies of the saprophytic fungus Marasmius oreades revealed that host genetic differences could be responsible for 25% of bacterial community structure variation (Pent et al., 2018). The authors thus proposed that, similarly to what’s known for animals and plants, host genetics could be an important driver of the structure and function of the microbiome of fungal fruiting bodies (Bulgarelli et al., 2012; Chaston et al., 2016). This was however not the case in this study for T. aestivum suggesting that microbiome drivers might thus differ in different fungal species.
Unexpected Truffle Microbiome Variations Revealed Through Extensive Sampling
The relevance of truffle microbiomes lies in their involvement in aroma production (Splivallo et al., 2015; Splivallo and Ebeler, 2015; Vahdatzadeh et al., 2015) and impact on truffle’s shelf-life/freshness (Rivera et al., 2010). We provide here the first extensive description of the microbiome of the summer black truffle T. aestivum. The overall structure of the bacterial communities observed in Swiss and French T. aestivum truffles corroborates earlier results obtained from other species of black and white truffles originating from Europe, China, and the United States (Antony-Babu et al., 2014; Barbieri et al., 2016; Benucci and Bonito, 2016; Ye et al., 2018). We confirmed that the T. aestivum fruiting body microbiome is characterized by an overall dominance of the α-Proteobacteria mainly affiliated to the Bradyrhizobium genus. However, unusual patterns were obtained for about 30% of the fruiting bodies from both Swiss and French truffle orchards. In these cases, microbiomes were dominated by OTUs affiliated to the genera Pedobacter (Bacteroidetes), Polaromonas (β-Proteobacteria), or Pseudomonas (γ-Proteobacteria), and not by α-Proteobacteria. The richness of the communities tended to be reduced to 10–20 OTUs when these genera dominated, suggesting that these particular genera massively invaded the gleba of the fruiting bodies and replaced or competed with Bradyrhizobium. By contrast, a few fruiting bodies were characterized by quite diverse and even bacterial communities containing up to 100 different OTUs (e.g., FR_34, CH_55, CH_78). These different microbiome patterns and the occasional preponderance of particular taxa have so far not been reported for any white and black truffle species (Antony-Babu et al., 2014; Vahdatzadeh et al., 2015; Benucci and Bonito, 2016; Ye et al., 2018) but it is in agreement with the discrepancies noticed between studies performed on identical species by different research groups (Barbieri et al., 2016). These differences might be explained by the low numbers of fruiting bodies of diverse truffle species analyzed so far (a few fruiting bodies vs. >50 in our study). Such variability in community composition between fruiting bodies is likely not a specificity of T. aestivum truffles, as preliminary results obtained on a large survey of T. melanosporum suggest the same trend (Deveau et al., unpublished data).
The ecological function of bacteria colonizing truffle fruiting bodies remains speculative but it has been hypothesized that they might contribute to truffle nutrition as well as aroma variability (Barbieri et al., 2010, 2015; Splivallo and Ebeler, 2015; Vahdatzadeh et al., 2015). It is tempting to speculate that differences in microbial communities might explain variability in aroma documented for T. aestivum truffles collected from the same orchard (Splivallo et al., 2012; Molinier et al., 2015). However, aroma variability in T. aestivum was linked earlier to truffle genotype, yet genotype did not contribute in the present study to microbial community structuring. This suggests that microorganisms might after all not play a major in the aroma variability of T. aestivum. Clearly, this hypothesis will need to be tested in the future, for example, by characterizing the volatile profiles of single major OTUs in the presence of truffle substrate (Splivallo et al., 2015).
The data presented here highlight the importance of three bacterial genera in truffles, namely Bradyrhizobium, Pedobacter, and Polaromonas. Even though the specific functions of these genera in truffles remain to be demonstrated, it has been suggested that Bradyrhizobium could be involved in the nutrition of the fruiting bodies since the role of this genus as nitrogen-fixing symbionts is well established in plant roots (Sulieman and Tran, 2014; Coba de la Peña et al., 2018). Nitrogen fixation by Bradyrhizobium strains isolated from the white truffle T. magnatum has been previously detected (Barbieri et al., 2010), even though several lines of evidence suggest that this might not occur in the black truffle T. melanosporum (Barbieri et al., 2016; Le Tacon et al., 2016) where Bradyrhizobium strains might be missing the nifH genes involved in nitrogen fixation (Antony-Babu et al., 2014; Deveau et al., unpublished data). This corroborates the recent proposition based on genome comparisons that symbiosis was not the dominant lifestyle of Bradyrhizobium but rather on form of specialization (VanInsberghe et al., 2015). Bradyrhizobium might also be involved in the production of specific sulfur volatile compounds responsible of truffle aroma perceived by humans (Splivallo et al., 2015). Bacteria of the Pedobacter genus have been reported to dominate microbial communities of decomposing fungal mycelium in forest soil and litter (Brabcová et al., 2016). These bacteria regroup generalists that possess a wide array of enzymes allowing degradation of diverse carbon sources. Additionally, some Pedobacter produce chitinases to degrade chitin of fungal cell wells. Although no obvious sign of degradation of the fruiting bodies was visible in our samples at the time of harvest, it is tempting to speculate that these bacteria could participate to the degradation of truffle fruiting bodies. Last, the role played by Polaromonas in truffles remains elusive. The genus comprises nine commonly occurring species that were originally reported from cold environments. Some members of the Polaromonas have the ability to fix nitrogen, hydrogen, and carbon dioxide (Sizova and Panikov, 2007; Hanson et al., 2012), suggesting that they could have similar functions in truffles. Demonstrating the exact function in truffles of these three bacterial genera will be the focus of future work.
Site-Specific Factors Drive Truffle’s Microbiome Assemblages
Multiple biotic and abiotic factors could drive the composition of the bacterial communities colonizing fruiting bodies of truffles. As the biochemical composition of fruiting bodies strongly changes during several months of maturation of T. melanosporum fruiting bodies (Harki et al., 2006), the level of maturity could be an important intrinsic driver of the bacterial communities. Indeed, a correlation was noticed between the bacterial community composition and the level of maturity of fruiting bodies of T. borchii, T. melanosporum, and T. indicum (Citterio et al., 2001; Antony-Babu et al., 2014; Ye et al., 2018). In contrast, the community composition of the white truffle T. magnatum remained stable along the maturation according to Barbieri et al. (2007). Such correlation between maturity degree and the composition of the microbiome was not evidenced in the present study, nor did we observe any link with the abundance of β-Proteobacteria or Bacteroidetes as previously reported by Antony-Babu et al. (2014) in T. melanosporum. Whether this is a specificity of T. aestivum remains to be determined. A possible reason might be the fact that T. aestivum, unlike T. melanosporum and other fungi, seems to pass through several lifecycles within a year with no clear seasonality, showing ripe and unripe fruiting bodies uncorrelated with size almost throughout a year (Büntgen et al., 2017). Such asynchrony of maturation might allow to more clearly disentangle maturation from spatial and temporal effects on bacterial communities in truffles. In agreement with this hypothesis, our data highlight a significant contribution of the spatial distance (Swiss orchard) and, to a lesser extent, of the collection year (French orchard) on the community composition of the bacterial communities in fruiting bodies. In addition, since truffle fruiting bodies are likely colonized by bacteria that thrive in the surrounding soil when the embryos are formed (Antony-Babu et al., 2014), such differences could be explained by variations in the bacterial communities of the soil surrounding truffles. Soils properties and climatic conditions likely differed between the two orchards. Similarly, root microbiomes are initially strongly influenced by the composition of the communities of the bulk soil and the environmental factors that influence this “starter” community (Zarraonaindia et al., 2015; Colin et al., 2017). Local pH, nutrients availability, or humidity levels have all been shown to significantly alter soil bacterial community composition (Uroz et al., 2016; Lladó et al., 2017). Although the general physico-chemical properties of soil are likely to be rather homogenous at the scale of a truffle orchard, it is well-known that small-scale heterogeneity exists in soil (Vos et al., 2013). We cannot exclude either that the differences between the factors influencing each orchard’s microbiomes are due to divergent sampling strategies in the two sites: firstly, samples were collected over 2 and 4 years, respectively, and secondly, the two orchards differed in surface area. Altogether this indicates that a better understanding of the interactions between soil microbial communities and truffle embryo at a microscopic scale is required to foresee the process of colonization of truffle fruiting bodies by bacteria.
Taken together, our results provide an unprecedented view of the microbiome associated to the black truffle T. aestivum. Microbiomes dominated by either the α-Proteobacteria class, and in some cases the β-Proteobacteria or the Sphingobacteriia classes could be evidenced regardless of geographical origin. The consistent occurrence of those microbes in fruiting bodies from orchards separated by hundreds of kilometers suggests that these bacteria might be highly relevant for truffles ecology and life cycle. Our results also highlight that factors shaping truffle’s microbiomes might differ based on local conditions, but unlike in other fungi, fruiting body maturation and genetic background did not seem to influence the microbiome. Overall, the findings presented here highlight the need to improve our understanding of truffle fruiting body development, of how the truffle microbiome is shaped, and what benefits it provides to truffles (or vice-versa). Complementary studies deploying large sampling efforts and functional studies of main bacterial players of the microbiome will be required to better understand these points.
Author Contributions
RS designed the experiments and collected the truffle samples. AD and VM characterized the microbiome with input from SU; genotyping by SSR was done by VM, MV, MP, and SE; mating type was determined by VM, MV, MP, SE, and FP. Data was analyzed by MV, RS, AD, and JGMV. AD, JGMV, MV, and RS wrote the manuscript with input from the other co-authors.
Funding
The research was funded by various grants and institutions. Specifically, the Deutsche Forschungsgemeinschaft (DFG) grants SP 1191/5-1 and MA7171/1-1 provided support to RS and JM-V, respectively. MV, JM-V, and RS were further supported through the program “Landes-Offensive zur Entwicklung Wissenschaftlich-Ökonomischer Exzellenz (LOEWE)” of the state of Hesse in Germany via the framework of the Cluster for Integrative Fungal Research (IPF). The Laboratory of Excellence Advanced Research on the Biology of Tree and Forest Ecosystems (ARBRE; ANR-11-LABX 0002 01) provided funding to AD for amplicon sequencing. VM was supported through the Swiss State Secretariat for Education, Research and Innovation (SERI) by the COST Action FP1203 and by the Montpellier University. We also acknowledge the DFG for providing funds for open access.
Conflict of Interest Statement
The authors declare that the research was conducted in the absence of any commercial or financial relationships that could be construed as a potential conflict of interest.
Acknowledgments
Henri Frochot and Jean-Pierre Schmidt are kindly acknowledged for supplying truffles and granting access to their orchards. We thank Nina Kirchhoff for the support she provided early on with microbial sequencing.
Supplementary Material
The Supplementary Material for this article can be found online at: https://www.frontiersin.org/articles/10.3389/fmicb.2019.01437/full#supplementary-material
Footnotes
References
Anderson, M. J. (2001). A new method for non-parametric multivariate analysis of variance. Aust. Ecol. 26, 32–46. doi: 10.1111/j.1442-9993.2001.01070
Antony-Babu, S., Deveau, A., Van Nostrand, J. D., Zhou, J., Le Tacon, F., Robin, C., et al. (2014). Black truffle-associated bacterial communities during the development and maturation of Tuber melanosporum ascocarps and putative functional roles. Environ. Microbiol. 16, 2831–2847. doi: 10.1111/1462-2920.12294
Antony-Babu, S., Murat, C., Deveau, A., Le Tacon, F., Frey-Klett, P., and Uroz, S. (2013). An improved method compatible with metagenomic analyses to extract genomic DNA from soils in Tuber melanosporum orchards. J. Appl. Microbiol. 115, 163–170. doi: 10.1111/jam.12205
Bahrndorff, S., Alemu, T., Alemneh, T., and Lund Nielsen, J. (2016). The microbiome of animals: implications for conservation biology. Int. J. Genomics 2016:5304028. doi: 10.1155/2016/5304028
Barbieri, E., Ceccaroli, P., Agostini, D., Zeppa, S. D., Gioacchini, A. M., and Stocchi, V. (2016). “Truffle-associated bacteria: extrapolation from diversity to function,” in True Truffle (Tuber spp.) in the World Soil Biology, eds A. Zambonelli, M. Iotti, and C. Murat (Cham: Springer), 301–317. doi: 10.1007/978-3-319-31436-5_18
Barbieri, E., Ceccaroli, P., Saltarelli, R., Guidi, C., Potenza, L., Basaglia, M., et al. (2010). New evidence for nitrogen fixation within the Italian white truffle Tuber magnatum. Fungal Biol. 114, 936–942. doi: 10.1016/j.funbio.2010.09.001
Barbieri, E., Guidi, C., Bertaux, J., Frey-Klett, P., Garbaye, J., Ceccaroli, P., et al. (2007). Occurrence and diversity of bacterial communities in Tuber magnatum during truffle maturation. Environ. Microbiol. 9, 2234–2246. doi: 10.1111/j.1462-2920.2007.01338.x
Barret, M., Briand, M., Bonneau, S., Préveaux, A., Valière, S., Bouchez, O., et al. (2015). Emergence shapes the structure of the seed microbiota. Appl. Environ. Microbiol. 81, 1257–1266. doi: 10.1128/AEM.03722-14
Benucci, G. M. N., and Bonito, G. M. (2016). The truffle microbiome: species and geography effects on bacteria associated with fruiting bodies of hypogeous Pezizales. Microb. Ecol. 72, 4–8. doi: 10.1007/s00248-016-0755-3
Berg, G., Grube, M., Schloter, M., and Smalla, K. (2014). Unraveling the plant microbiome: looking back and future perspectives. Front. Microbiol. 5:148. doi: 10.3389/fmicb.2014.00148
Borcard, D., Gillet, F., and Legendre, P. (2011). Numerical Ecology With R. New York, NY: Springer-Verlag.
Bouffaud, M.-L., Poirier, M.-A., Muller, D., and Moënne-Loccoz, Y. (2014). Root microbiome relates to plant host evolution in maize and other Poaceae. Environ. Microbiol. 16, 2804–2814. doi: 10.1111/1462-2920.12442
Brabcová, V., Nováková, M., Davidová, A., and Baldrian, P. (2016). Dead fungal mycelium in forest soil represents a decomposition hotspot and a habitat for a specific microbial community. New Phytol. 210, 1369–1381. doi: 10.1111/nph.13849
Bulgarelli, D., Rott, M., Schlaeppi, K., Ver Loren van Themaat, E., Ahmadinejad, N., Assenza, F., et al. (2012). Revealing structure and assembly cues for Arabidopsis root-inhabiting bacterial microbiota. Nature 488, 91–95. doi: 10.1038/nature11336
Büntgen, U., Bagi, I., Fekete, O., Molinier, V., Peter, M., Splivallo, R., et al. (2017). New insights into the complex relationship between weight and maturity of Burgundy truffles (Tuber aestivum). PLoS One 12:e0170375. doi: 10.1371/journal.pone.0170375
Chaston, J. M., Dobson, A. J., Newell, P. D., and Douglas, A. E. (2016). Host genetic control of the microbiota mediates the Drosophila nutritional phenotype. Appl. Environ. Microbiol. 82, 671–679. doi: 10.1128/AEM.03301-15
Citterio, B., Malatesta, M., Battistelli, S., Marcheggiani, F., Baffone, W., Saltarelli, R., et al. (2001). Possible involvement of Pseudomonas fluorescens and bacillaceae in structural modifications of Tuber borchii fruit bodies. Can. J. Microbiol. 47, 264–268. doi: 10.1139/w01-005
Clarke, K. R. (1993). Non-parametric multivariate analyses of changes in community structure. Aust. J. Ecol. 18, 117–143. doi: 10.1111/j.1442-9993.1993.tb00438.x
Coba de la Peña, T., Fedorova, E., Pueyo, J. J., and Lucas, M. M. (2018). The symbiosome: legume and rhizobia co-evolution toward a nitrogen-fixing organelle? Front. Plant Sci. 8:2229. doi: 10.3389/fpls.2017.02229
Cole, J. R., Wang, Q., Cardenas, E., Fish, J., Chai, B., Farris, R. J., et al. (2009). The ribosomal database project: improved alignments and new tools for rRNA analysis. Nucleic Acids Res. 37, D141–D145. doi: 10.1093/nar/gkn879
Colin, Y., Nicolitch, O., Nostrand, J. D. V., Zhou, J. Z., Turpault, M.-P., and Uroz, S. (2017). Taxonomic and functional shifts in the beech rhizosphere microbiome across a natural soil toposequence. Sci. Rep. 7:9604. doi: 10.1038/s41598-017-07639-1
De la Varga, H., Le Tacon, F., Lagoguet, M., Todesco, F., Varga, T., Miquel, I., et al. (2017). Five years investigation of female and male genotypes in périgord black truffle (Tuber melanosporum Vittad.) revealed contrasted reproduction strategies. Environ. Microbiol. 19, 2604–2615. doi: 10.1111/1462-2920.13735
Deveau, A., Antony-Babu, S., Le Tacon, F., Robin, C., Frey-Klett, P., and Uroz, S. (2016). Temporal changes of bacterial communities in the Tuber melanosporum ectomycorrhizosphere during ascocarp development. Mycorrhiza 26, 389–399. doi: 10.1007/s00572-015-0679-7
Deveau, A., Bonito, G., Uehling, J., Paoletti, M., Becker, M., Bindschedler, S., et al. (2018). Bacterial–fungal interactions: ecology, mechanisms and challenges. FEMS Microbiol. Rev. 42, 335–352. doi: 10.1093/femsre/fuy008
Deveau, A., Clowez, P., Petit, F., Maurice, J.-P., Todesco, F., Murat, C., et al. (2019). New insights into black truffle biology: discovery of the potential connecting structure between a Tuber aestivum ascocarp and its host root. Mycorrhiza 29, 219–226. doi: 10.1007/s00572-019-00892-4
Dominguez, J. A., Martin, A., Anriquez, A., and Albanesi, A. (2012). The combined effects of Pseudomonas fluorescens and Tuber melanosporum on the quality of Pinus halepensis seedlings. Mycorrhiza 22, 429–436. doi: 10.1007/s00572-011-0420-0
Dray, S., Legendre, P., and Blanchet, G. (2009). packfor: Forward Selection with Permutation (Canoco p.46) R package version 0.0-7/r58. Available at: https://rdrr.io/rforge/packfor/ (accessed March 19, 2018).
Escudié, F., Auer, L., Bernard, M., Mariadassou, M., Cauquil, L., Vidal, K., et al. (2018). FROGS: find, rapidly, OTUs with galaxy solution. Bioinformatics 34, 1287–1294. doi: 10.1093/bioinformatics/btx791
Foster, Z. S. L., Sharpton, T. J., and Grünwald, N. J. (2017). Metacoder: an R package for visualization and manipulation of community taxonomic diversity data. PLoS Comput. Biol. 13:e1005404. doi: 10.1371/journal.pcbi.1005404
Glynou, K., Ali, T., Buch, A.-K., Haghi Kia, S., Ploch, S., Xia, X., et al. (2016). The local environment determines the assembly of root endophytic fungi at a continental scale. Environ. Microbiol. 18, 2418–2434. doi: 10.1111/1462-2920.13112
Gryndler, M., Beskid, O., Hršelová, H., Bukovská, P., Hujslová, M., Gryndlerová, H., et al. (2015). Mutabilis in mutabili: spatiotemporal dynamics of a truffle colony in soil. Soil Biol. Biochem. 90, 62–70. doi: 10.1016/j.soilbio.2015.07.025
Gryndler, M., Soukupová, L., Hršelová, H., Gryndlerová, H., Borovička, J., Streiblová, E., et al. (2013). A quest for indigenous truffle helper prokaryotes. Environ. Microbiol. Rep. 5, 346–352. doi: 10.1111/1758-2229.12014
Hacquard, S., Garrido-Oter, R., González, A., Spaepen, S., Ackermann, G., Lebeis, S., et al. (2015). Microbiota and host nutrition across plant and animal kingdoms. Cell Host Microbe 17, 603–616. doi: 10.1016/j.chom.2015.04.009
Hanson, B. T., Yagi, J. M., Jeon, C. O., and Madsen, E. M. (2012). Role of nitrogen fixation in the autecology of Polaromonas naphthalenivorans in contaminated sediments. Environ. Microbiol. 14, 1544–1557. doi: 10.1111/j.1462-2920.2012.02743.x
Harki, E., Bouya, D., and Dargent, R. (2006). Maturation-associated alterations of the biochemical characteristics of the black truffle Tuber melanosporum Vitt. Food Chem. 99, 394–400. doi: 10.1016/j.foodchem.2005.08.030
Le Tacon, F., Rubini, A., Murat, C., Riccioni, C., Robin, C., Belfiori, B., et al. (2016). Certainties and uncertainties about the life cycle of the Périgord black truffle (Tuber melanosporum Vittad.). Ann. For. Sci. 73, 105–117. doi: 10.1007/s13595-015-0461-1
Le Tacon, F., Zeller, B., Plain, C., Hossann, C., Bréchet, C., Martin, F., et al. (2015). Study of nitrogen and carbon transfer from soil organic matter to Tuber melanosporum mycorrhizas and ascocarps using 15N and 13C soil labelling and whole-genome oligoarrays. Plant Soil 395, 351–373. doi: 10.1007/s11104-015-2557-7
Le Tacon, F., Zeller, B., Plain, C., Hossann, C., Bréchet, C., and Robin, C. (2013). Carbon transfer from the host to Tuber melanosporum mycorrhizas and ascocarps followed using a 13C pulse-labeling technique. PLoS One 8:e64626. doi: 10.1371/journal.pone.0064626
Legendre, P., and Gallagher, E. D. (2001). Ecologically meaningful transformations for ordination of species data. Oecologia 129, 271–280. doi: 10.1007/s004420100716
Legendre, P., and Legendre, L. F. J. (2012). Numerical Ecology, 3rd Edn, Vol. 24. Amsterdam: Elsevier.
Lladó, S., López-Mondéjar, R., and Baldrian, P. (2017). Forest soil bacteria: diversity, involvement in ecosystem processes, and response to global change. Microbiol. Mol. Biol. Rev. 81:e00063-16. doi: 10.1128/MMBR.00063-16
Lundberg, D. S., Lebeis, S. L., Paredes, S. H., Yourstone, S., Gehring, J., Malfatti, S., et al. (2012). Defining the core Arabidopsis thaliana root microbiome. Nature 488, 86–90. doi: 10.1038/nature11237
Martin, F., Kohler, A., Murat, C., Balestrini, R., Coutinho, P. M., Jaillon, O., et al. (2010). Périgord black truffle genome uncovers evolutionary origins and mechanisms of symbiosis. Nature 464, 1033–1038. doi: 10.1038/nature08867
McMurdie, P. J., and Holmes, S. (2013). phyloseq: an R package for reproducible interactive analysis and graphics of microbiome census data. PLoS One 8:e61217. doi: 10.1371/journal.pone.0061217
Molinier, V., Bouffaud, M.-L., Castel, T., Mounier, A., Colombet, A., Recorbet, G., et al. (2013a). Monitoring the fate of a 30-year-old truffle orchard in Burgundy: from Tuber melanosporum to Tuber aestivum. Agrofor. Syst. 87, 1439–1449. doi: 10.1007/s10457-013-9649-2
Molinier, V., Murat, C., Morin, E., Gollotte, A., Wipf, D., and Martin, F. (2013b). First identification of polymorphic microsatellite markers in the Burgundy truffle, Tuber aestivum (Tuberaceae). Appl. Plant Sci. 1:1200220. doi: 10.3732/apps.1200220
Molinier, V., Murat, C., Baltensweiler, A., Büntgen, U., Martin, F., Meier, B., et al. (2016). Fine-scale genetic structure of natural Tuber aestivum sites in southern Germany. Mycorrhiza 26, 895–907. doi: 10.1007/s00572-016-0719-y
Molinier, V., Murat, C., Frochot, H., Wipf, D., and Splivallo, R. (2015). Fine-scale spatial genetic structure analysis of the black truffle Tuber aestivum and its link to aroma variability. Environ. Microbiol. 17, 3039–3050. doi: 10.1111/1462-2920.12910
Murat, C. (2015). Forty years of inoculating seedlings with truffle fungi: past and future perspectives. Mycorrhiza 25, 77–81. doi: 10.1007/s00572-014-0593-4
Murat, C., Payen, T., Noel, B., Kuo, A., Morin, E., Chen, J., et al. (2018). Pezizomycetes genomes reveal the molecular basis of ectomycorrhizal truffle lifestyle. Nat. Ecol. Evol. 2, 1956–1965. doi: 10.1038/s41559-018-0710-4
Nadkarni, M. A., Martin, F. E., Jacques, N. A., and Hunter, N. (2002). Determination of bacterial load by real-time PCR using a broad-range (universal) probe and primers set. Microbiol. Read. Engl. 148, 257–266. doi: 10.1099/00221287-148-1-257
Oksanen, J., Blanchet, F. G., Kindt, R., Legendre, P., Minchin, P. R., O’Hara, R. B., et al. (2015). Vegan: Community Ecology Package. R package vegan, Version. 2.2–1.
On, S., Atabay, H., Corry, J., Harrington, C., and Vandamme, P. (1998). Emended description of Campylobacter sputorum and revision of its infrasubspecific (biovar) divisions, including C. sputorum (biovar) paraureolyticus, a urease-producing variant from cattle and humans. Int. J. Syst. Bacteriol. 48, 195–206. doi: 10.1099/00207713-48-1-195
Paolocci, F., Rubini, A., Riccioni, C., and Arcioni, S. (2006). Reevaluation of the life cycle of Tuber magnatum. Appl. Environ. Microbiol. 72, 2390–2393. doi: 10.1128/AEM.72.4.2390-2393.2006
Pent, M., Hiltunen, M., Põldmaa, K., Furneaux, B., Hildebrand, F., Johannesson, H., et al. (2018). Host genetic variation strongly influences the microbiome structure and function in fungal fruiting-bodies. Environ. Microbiol. 20, 1641–1650. doi: 10.1111/1462-2920.14069
Peres-Neto, P. R., and Legendre, P. (2010). Estimating and controlling for spatial structure in the study of ecological communities. Glob. Ecol. Biogeogr. 19, 174–184. doi: 10.1111/j.1466-8238.2009.00506.x
R Core Team (2017). R: A Language and Environment for Statistical Computing. Available at: http://www.R-project.org/ (accessed January 1, 2017).
Rivera, C. S., Blanco, D., Salvador, M. L., and Venturini, M. E. (2010). Shelf-life extension of fresh Tuber aestivum and Tuber melanosporum truffles by modified atmosphere packaging with microperforated films. J. Food Sci. 75, E225–E233. doi: 10.1111/j.1750-3841.2010.01602.x
Rubini, A., Belfiori, B., Riccioni, C., Arcioni, S., Martin, F., and Paolocci, F. (2011a). Tuber melanosporum: mating type distribution in a natural plantation and dynamics of strains of different mating types on the roots of nursery-inoculated host plants. New Phytol. 189, 723–735. doi: 10.1111/j.1469-8137.2010.03493.x
Rubini, A., Belfiori, B., Riccioni, C., Tisserant, E., Arcioni, S., Martin, F., et al. (2011b). Isolation and characterization of MAT genes in the symbiotic ascomycete Tuber melanosporum. New Phytol. 189, 710–722. doi: 10.1111/j.1469-8137.2010.03492.x
Rubini, A., Riccioni, C., Arcioni, S., and Paolocci, F. (2007). Troubles with truffles: unveiling more of their biology. New Phytol. 174, 256–259. doi: 10.1111/j.1469-8137.2007.01976.x
Rubini, A., Riccioni, C., Belfiori, B., and Paolocci, F. (2014). Impact of the competition between mating types on the cultivation of Tuber melanosporum: romeo and Juliet and the matter of space and time. Mycorrhiza 24, 19–27. doi: 10.1007/s00572-013-0551-6
Saidi, N., Deshaware, S., Romdhane, I. B., Nadim, M., and Ojamo, H. (2015). Endogenous bacteria of Tuber aestivum ascocarps are potential biocontrol agents of microbial post-harvest deterioration of truffles. Int. J. Eng. Appl. Sci. 7, 97–106.
Sbrana, C., Agnolucci, M., Bedini, S., Lepera, A., Toffanin, A., Giovannetti, M., et al. (2002). Diversity of culturable bacterial populations associated to Tuber borchii ectomycorrhizas and their activity on T. Borchii mycelial growth. FEMS Microbiol. Lett. 211, 195–201. doi: 10.1016/s0378-1097(02)00712-7
Selosse, M.-A., Schneider-Maunoury, L., Taschen, E., Rousset, F., and Richard, F. (2017). Black truffle, a hermaphrodite with forced unisexual behaviour. Trends Microbiol. 25, 784–787. doi: 10.1016/j.tim.2017.05.010
Sizova, M., and Panikov, N. (2007). Polaromonas hydrogenivorans sp. nov., a psychrotolerant hydrogen-oxidizing bacterium from Alaskan soil. Int. J. Syst. Evol. Microbiol. 57, 616–619. doi: 10.1099/ijs.0.64350-0
Splivallo, R., Deveau, A., Valdez, N., Kirchhoff, N., Frey-Klett, P., and Karlovsky, P. (2015). Bacteria associated with truffle-fruiting bodies contribute to truffle aroma. Environ. Microbiol. 17, 2647–2660. doi: 10.1111/1462-2920.12521
Splivallo, R., and Ebeler, S. E. (2015). Sulfur volatiles of microbial origin are key contributors to human-sensed truffle aroma. Appl. Microbiol. Biotechnol. 99, 2583–2592. doi: 10.1007/s00253-014-6360-9
Splivallo, R., Valdez, N., Kirchhoff, N., Ona, M. C., Schmidt, J.-P., Feussner, I., et al. (2012). Intraspecific genotypic variability determines concentrations of key truffle volatiles. New Phytol. 194, 823–835. doi: 10.1111/j.1469-8137.2012.04077.x
Stenberg, P., Lundmark, M., and Saura, A. (2003). mlgsim: a program for detecting clones using a simulation approach. Mol. Ecol. Notes 3, 329–331. doi: 10.1046/j.1471-8286.2003.00408.x
Sulieman, S., and Tran, L.-S. P. (2014). Symbiotic nitrogen fixation in legume nodules: metabolism and regulatory mechanisms. Int. J. Mol. Sci. 15, 19389–19393. doi: 10.3390/ijms151119389
Uroz, S., Buée, M., Deveau, A., Mieszkin, S., and Martin, F. (2016). Ecology of the forest microbiome: highlights of temperate and boreal ecosystems. Soil Biol. Biochem. 103, 471–488. doi: 10.1016/j.soilbio.2016.09.006
Vahdatzadeh, M., Deveau, A., and Splivallo, R. (2015). The role of the microbiome of truffles in aroma formation: a meta-analysis approach. Appl. Environ. Microbiol. 81, 6946–6952. doi: 10.1128/AEM.01098-15
VanInsberghe, D., Maas, K. R., Cardenas, E., Strachan, C. R., Hallam, S. J., and Mohn, W. W. (2015). Non-symbiotic bradyrhizobium ecotypes dominate North American forest soils. ISME J. 9, 2435–2441. doi: 10.1038/ismej.2015.54
Vos, M., Wolf, A. B., Jennings, S. J., and Kowalchuk, G. A. (2013). Micro-scale determinants of bacterial diversity in soil. FEMS Microbiol. Rev. 37, 936–954. doi: 10.1111/1574-6976.12023
Webster, N. S., and Thomas, T. (2016). The sponge hologenome. mBio 7:e00135-16. doi: 10.1128/mBio.00135-16
Ye, L., Li, Q., Fu, Y., Sourzat, P., Tan, H., Zou, J., et al. (2018). Host species effects on bacterial communities associated with the fruiting bodies of Tuber species from the Sichuan Province in Southwest China. Mycol. Prog. 17, 833–840. doi: 10.1007/s11557-018-1397-2
Keywords: Tuber aestivum, amplicon sequencing, bacterial communities, microbiome, multilocus genotype, mating type
Citation: Splivallo R, Vahdatzadeh M, Maciá-Vicente JG, Molinier V, Peter M, Egli S, Uroz S, Paolocci F and Deveau A (2019) Orchard Conditions and Fruiting Body Characteristics Drive the Microbiome of the Black Truffle Tuber aestivum. Front. Microbiol. 10:1437. doi: 10.3389/fmicb.2019.01437
Received: 28 November 2018; Accepted: 07 June 2019;
Published: 28 June 2019.
Edited by:
Andrey M. Yurkov, German Collection of Microorganisms and Cell Cultures GmbH (DSMZ), GermanyReviewed by:
Katharina Julia Huber, German Collection of Microorganisms and Cell Cultures GmbH (DSMZ), GermanyPetr Karlovsky, University of Göttingen, Germany
Copyright © 2019 Splivallo, Vahdatzadeh, Maciá-Vicente, Molinier, Peter, Egli, Uroz, Paolocci and Deveau. This is an open-access article distributed under the terms of the Creative Commons Attribution License (CC BY). The use, distribution or reproduction in other forums is permitted, provided the original author(s) and the copyright owner(s) are credited and that the original publication in this journal is cited, in accordance with accepted academic practice. No use, distribution or reproduction is permitted which does not comply with these terms.
*Correspondence: Richard Splivallo, richard.splivallo@a3.epfl.ch; Aurélie Deveau, aurelie.deveau@inra.fr