- 1Laboratory of Food Biotechnology, Institute of Food, Nutrition and Health, ETH Zurich, Zurich, Switzerland
- 2Department of Gastroenterology and Hepatology, University Hospital Zurich, Zurich, Switzerland
Murine models are valuable tools to study the role of gut microbiota in health or disease. However, murine and human microbiota differ in species composition, so further investigation of the murine gut microbiota is important to gain a better mechanistic understanding. Continuous in vitro fermentation models are powerful tools to investigate microbe-microbe interactions while circumventing animal testing and host confounding factors, but are lacking for murine gut microbiota. We therefore developed a novel continuous fermentation model based on the PolyFermS platform adapted to the murine caecum and inoculated with immobilized caecal microbiota. We followed a stepwise model development approach by adjusting parameters [pH, retention time (RT), growth medium] to reach fermentation metabolite profiles and marker bacterial levels similar to the inoculum. The final model had a stable and inoculum-alike fermentation profile during continuous operation. A lower pH during startup and continuous operation stimulated bacterial fermentation (115 mM short-chain fatty acids at pH 7 to 159 mM at pH 6.5). Adjustments to nutritive medium, a decreased pH and increased RT helped control the in vitro Enterobacteriaceae levels, which often bloom in fermentation models, to 6.6 log gene copies/mL in final model. In parallel, the Lactobacillus, Lachnospiraceae, and Ruminococcaceae levels were better maintained in vitro with concentrations of 8.5 log gene copies/mL, 8.8 log gene copies/mL and 7.5 log gene copies/mL, respectively, in the final model. An independent repetition with final model parameters showed reproducible results in maintaining the inoculum fermentation metabolite profile and its marker bacterial levels. Microbiota community analysis of the final model showed a decreased bacterial diversity and compositional differences compared to caecal inoculum microbiota. Most of the caecal bacterial families were represented in vitro, but taxa of the Muribaculaceae family were not maintained. Functional metagenomics prediction showed conserved metabolic and functional KEGG pathways between in vitro and caecal inoculum microbiota. To conclude, we showed that a rational and stepwise approach allowed us to model in vitro the murine caecal microbiota and functions. Our model is a first step to develop murine microbiota model systems and offers the potential to study microbiota functionality and structure ex vivo.
Introduction
The gastrointestinal tract of the mammalian host is inhabited by a dense, complex and diverse bacterial community, termed the gut microbiota (Robles Alonso and Guarner, 2013). The gut microbiota is involved in key processes beneficial for the host such as dietary compound metabolism, pathogen displacement, or immune system development (Round and Mazmanian, 2009; Robles Alonso and Guarner, 2013). A large proportion of the vast amount of evidence that the gut microbiota influences many physiological and pathological processes in the host comes from murine studies (Round and Palm, 2018). Mouse models are a valuable tool for human biology, disease and pharmaceutical research due to the high similarity in physiology, anatomy, and genetics (Nguyen et al., 2015). However, mice and humans differ in gastrointestinal tract anatomy, dietary habits, circadian rhythm, and environmental microbes and pathogens, which leads to differences in their gut microbiota composition and activity (Nguyen et al., 2015; Uhl and Warner, 2015; Hugenholtz and de Vos, 2017).
In humans, the large intestine is the main site of fermentation, and contains the highest microbial load and activity, whereas in mice, intestinal fermentation mainly takes place in the caecum, a “bag-like” link between the small intestine and the colon that is nearly absent in humans (Nguyen et al., 2015). Morphological differences in compartmentalization, retention times (RTs) and mixing conditions likely influences composition, richness and diversity of the gut microbiota in humans and mice (Nguyen et al., 2015). In both humans and mice, fermentation of indigestible food compounds produces the short-chain fatty acids (SCFAs) acetate, propionate and butyrate (Flint et al., 2012; Burokas et al., 2017; Herrmann et al., 2018). The bacterial composition influences the fermentation capacity and end microbial metabolite profiles (Flint et al., 2012). At phylum level, the murine and human intestinal microbiota are similar with the two dominating phyla Firmicutes and Bacteroidetes (Ley et al., 2006; Clavel et al., 2016; Hugenholtz and de Vos, 2017). However, when comparing the bacterial composition at a deeper phylogenetic level, mice and humans show clear differences in genera composition and abundance. First comparisons showed that 85% of detected bacterial genera in the mouse gut are not present in that of humans (Ley et al., 2005) and further studies identified mouse- and human-specific taxa (Nguyen et al., 2015; Xiao et al., 2015; Clavel et al., 2016). Genera such as Lactobacillus, Turicibacter and Coprobacillus (Erysipelotrichaceae), Anaerotruncus (Ruminococcaceae), Marvinbryantia (Lachnospiraceae), and Pseudoflavonifractor (unclassified Clostridiales) are present at higher levels in the murine intestinal microbiota, while Prevotella, Faecalibacterium, Ruminococcus, Oscillibacter, and Klebsiella are more abundant in human (Nguyen et al., 2015; Xiao et al., 2015). Deep metagenome sequencing revealed that only 4% of microbial genes in the mouse gut were shared with human gut samples, but almost 80% of their annotated functions were common (Xiao et al., 2015). This indicates a similar functionality of mouse and human gut microbiota, but performed by different species and strains.
Recently, efforts were made to isolate the murine gut bacteria (Lagkouvardos et al., 2016) to formulate minimal microbial consortia for studying microbiota-derived functions in gnotobiotic mouse models (Clavel et al., 2017). Simplified defined consortia may not recapitulate all functions of complex microbiota. Continuous in vitro fermentation models that contained the complex gut microbial communities mimicking the conditions of the modeled host were successful developed for ecological and mechanistic studies of human (Zihler et al., 2013; Dostal et al., 2015; Geirnaert et al., 2015; Lacroix et al., 2015; McDonald et al., 2015) and monogastric animal (Tanner et al., 2014) gut microbiota, which avoided ethical concerns and host confounding factors. However, to our knowledge no complex murine gut microbiota model has been developed, apart from simple, closed and short-term batch cultures (Salyer et al., 2013; Herrmann et al., 2017; Li et al., 2018) or continuous flow cultures without pH control and taxonomic validation (Freter et al., 1983). In contrast to batch cultures, continuous fermentation models offer the advantage of continuous substrate supply and removal of toxic fermentation products, which are required for growth and establishment of balanced and representative ecosystems. Physiological parameters like RT, temperature, pH, and redox potential are highly controlled in continuous fermentation, which facilitates the establishment of steady-state conditions (Payne et al., 2012). In addition, in vitro continuous gut fermentation models may allow simulation of the spatial, environmental and temporal features of a specific gut environment. Challenges of in vitro fermentation models include the large amount of gut microbiota sample (mainly feces) required for inoculation and the loss of less competitive bacteria by rapid washout. To address these challenges, fecal microbiota can be immobilized in porous gel beads and run in continuous culture, which allows models to operate at high cell density akin to the gut, prevents washout of slow growing bacteria, and reproduces both planktonic and sessile states of the gut microbiota (Zihler et al., 2013; Tanner et al., 2014; Fehlbaum et al., 2015; Poeker et al., 2018). In addition, only very small amounts of donor material (1–2 g) are required to establish a fermentation, therefore enabling modeling of small animal gut microbiota.
In this study, we developed a continuous fermentation model of the murine caecal microbiota based on the PolyFermS platform inoculated with immobilized caecal microbiota of C57BL/6 mice. In a first step, we determined the pH, the bacterial composition and the metabolic profile in the caeca of C57BL/6 mice. To support growth of the murine caecal bacterial populations and activity, a complex murine nutritive growth medium was developed to mimic substrate conditions encountered in mouse caecum chyme. Different factors of the fermentation model, including caecum sampling, fermentation starting mode, pH, RT and adjustment of growth medium were investigated and adjusted in a sequential order, using five different models inoculated with different pooled fresh immobilized caecum microbiota from 4-5 mice and operated up to 69 days. The microbiota composition in the reactor effluents was analyzed by quantitative real time PCR (qPCR) and 16S rRNA amplicon sequencing and compared with the caecal inoculum. Metabolic activity and functional stability of the microbiota was monitored by SCFA analysis.
Materials and Methods
Caecal Microbiota Collection
Healthy female WT C57BL/6J mice aged 9–13 weeks were obtained from Charles River (Lyon, France) and housed in groups at University Hospital of Zurich. Housing conditions were controlled at 22°C, room humidity and 12 h light/dark cycle. Mice were provided with mouse/rat maintenance chow (Kliba Nafag, Kaiseraugst, Switzerland) and ad libitum drinking water. Mice were housed in different cages and were sacrificed on the same day in the morning by cervical dislocation, after which the caecum was immediately removed and placed on a sterile petri plate. The pH of the fresh caecal content in the intact caecal pouch was immediately determined using a probe pre-calibrated pH meter (Metrohm 744 pH Meter, Metrohm Ltd., Herisau, Switzerland). The caecal content was collected into DNAase-free tubes, immediately snap frozen in liquid nitrogen, and then stored at -20°C until DNA and SCFA extraction. Mouse experiments were conducted according to Swiss animal welfare legislation, and the local veterinary office approved all procedures (Veterinäramt des Kantons Zürich; Nr. ZH220/2016).
In vitro Fermentation Model
Nutritive Medium
The nutritive medium was formulated from the validated bacterial growth medium described by Macfarlane et al. (1998) for cultivating the human gut microbiota in vitro. It was modified to approximate the carbohydrate and protein ratio in murine caecum chyme. Standard mouse chow is composed of approximately 18% (w/w) protein, 54% (w/w) soluble carbohydrate and 4.5% (w/w) crude fiber (Supplementary Table S1B). Considering an average daily chow intake of 3.5 g per C57BL/6J mouse (Champy et al., 2008), a daily carbohydrate intake of 1.9 g, 0.6 g protein and 0.14 g crude fibers was considered. To calculate the amount of carbohydrates (excluding crude fibers) and proteins reaching the caecum, upper gastrointestinal digestibility indices of 95% for carbohydrates (Dahlqvist and Thomson, 1963a,b; Lee et al., 2011) and 90% for proteins (Kerr et al., 2014) were applied. This resulted in 0.09 g of dietary carbohydrates and 0.06 g of dietary proteins reaching the caecum each day or a dietary carbohydrate:protein ratio of 60:40. Macfarlane medium was adapted accordingly. (Supplementary Table S1A). To simulate the mouse chow more closely, we excluded guar gum and inulin, and replaced soluble potato starch with soluble corn starch. Concentrations of the protein sources casein, peptone, tryptone and yeast extract were increased to meet the calculated murine caecum chyme protein concentration. The mucin concentration was kept at 4 g/L. The pectin concentration was kept at 2 g/L to avoid flow disturbance in tubes from increased viscosity. The carbohydrate:protein ratio was approximately 60:40 in medium 1 and 55:45 in medium 2 (Supplementary Table S1A), which did not include the contribution of yeast extract and mucin used for simulating the endogenous protein and carbohydrate sources in the gastrointestinal tract (Cornick et al., 2015). After sterilization (20 min, 120°C) and cooling to 4°C, 1 mL of a filter-sterilized (0.2 μm pore-size) vitamin solution (Michel et al., 1998) was added to the medium. For initial bead colonization, the nutritive growth medium was supplemented with 20% (v/v) effluent from a previous fermentation (acetate:propionate:butyrate 2.5:1.5:1) or 20% (v/v) rumen fluid (4.5:3:1) and 0.1% (m/v) cellobiose (Table 1A). All components were purchased from Sigma-Aldrich Chemie (Buchs, Switzerland), except for xylane (Angene, London, United Kingdom), peptone water (Oxoid AG, Pratteln, Switzerland), bile salts (Oxbile, Oxoid AG), tryptone (BD, Sparks, United States), yeast extract (Merck, Darmstadt, Germany), NaHCO3 (Fischer Scientific, Pittsburgh, PA, United States), NaCl and KH2PO4 (VWR International AG, Dietikon, Switzerland), MgSO4⋅anhydrous (Acros Organics, Geel, Belgium) and MnCl2⋅4H2O (Fluka, Buchs, Switzerland).
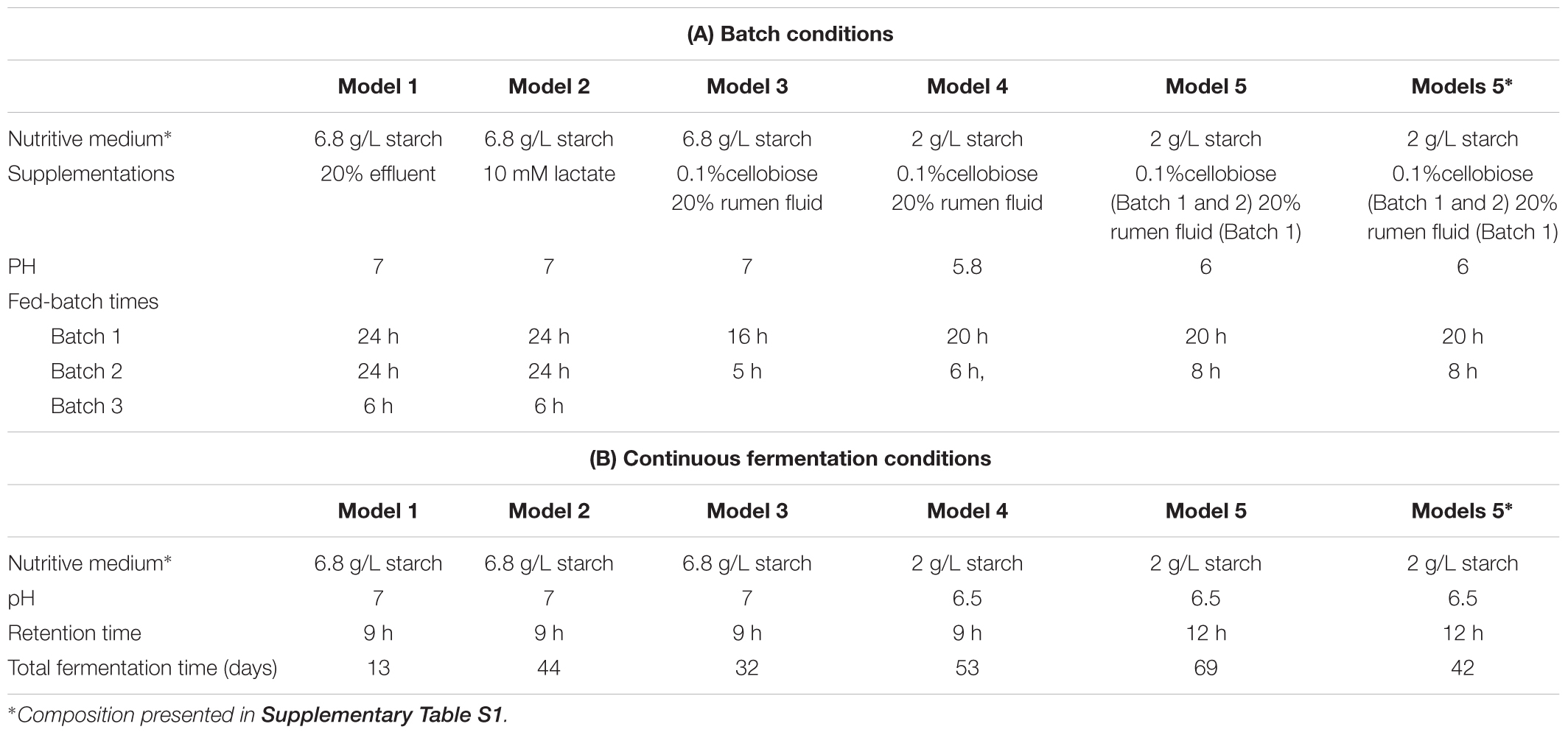
Table 1. Conditions of initial batch (bead colonization) (A) and continuous (B) fermentation for the different tested models of mouse caecal fermentation.
Caecal Microbiota Immobilization and Bead Colonization
For each immobilization procedure, four to five caeca from healthy 9- to 13- weeks old female C57BL/6 mice were collected to prepare the inoculum. All caecal contents were collected in the morning shortly (models 1 and 2) or a couple of hours (models 3–5) after the dark period. In model 1, caecal contents were dissected aerobically from the remaining gastrointestinal tract with surgical scissors, placed onto a sterile Petri dish and immediately transferred to an anaerobic box until further processing. In models 2–5, the caeca were tied off with surgical threads to avoid oxygen stress to the microbiota, placed onto a sterile Petri dish and immediately transferred to an anaerobic jar, and transferred into an anaerobic chamber (10% CO2, 5% H2, and 85% N2) (Coy Laboratories, Ann Arbor, MI, United States), for dissection and pooling contents. In models 3–5, caecal bacteria were washed with pre-reduced peptone water (0.1 %, pH 7, Thermo Fisher Diagnostics AG, Pratteln, Switzerland) before immobilization to remove potential interfering endogenous enzymes, salts and other cellular products that hinder proper gel bead formation. Caecal bacteria were immobilized in 1–2 mm gel beads consisting of gellan gum (2.5%, m/v), xanthan (0.25%, m/v), and sodium citrate (0.2%, m/v) in an anaerobic chamber as previously described (Poeker et al., 2018). Sixty milliliters of freshly produced caecal beads were transferred to a glass bioreactor containing 140 mL of sterile murine nutritive medium. For initial bead colonization, two or three consecutive fed-batch fermentations were carried out by replacing 100 mL of the spent medium by fresh nutritive medium every 6–24 h, depending on the model (Table 1A). The reactor headspace was continuously flushed with CO2, to ensure anaerobiosis in the system. The temperature was controlled at 37°C and the pH at selected values (depending on the model and fermentation step) by automatic addition of 2.5 M NaOH.
Continuous Caecum Microbiota Fermentation and Treatments
After batch fermentations, the reactor containing caecal beads was switched to continuous mode by continuously supplying fresh, sterile and anaerobic nutritive medium and removing an equivalent volume of fermented medium with peristaltic pumps (Reglo, Ismatec, Glattbrugg, Switzerland) (Table 1B). For all bioreactors, stirring speed was carried out at 180 rpm, working volume was 200 mL, temperature was set to 37°C and anaerobiosis was maintained by continuously flushing the headspace of bioreactors. In models 1–3, bioreactors were operated with constant conditions of pH 7 and a continuous flow rate of 22.2 mL/h (RT of 9 h) of medium 1 (6.8 g/L starch). Bioreactor in model 4 was maintained at pH 6.5 and supplied with fresh nutritive medium 2 (2 g/L starch) at a RT of 9 h. In model 5 and model 5∗, reactors were operated with constant conditions of pH 6.5 and a continuous flow rate of 16.7 mL/h (corresponds to a RT of 12 h) of medium 2. Effluent samples were taken daily and separated into bacterial pellets (10 min at 14,000 ×g at 4°C) and supernatant, and stored at -20°C until further analysis. Stability of in vitro microbiota was monitored by daily measurements of the main fermentation metabolite concentrations in sample supernatants.
Microbial Metabolite Analysis
Caecal samples were mixed with 300 μL 0.15 mM H2SO4, homogenized and centrifuged at 4°C at 9000 ×g for 20 min. Supernatant was filtered directly into HPLC vials through a 0.45 μm nylon membrane (Infochroma AG, Zug, Switzerland). Fermentation samples were centrifuged at 4°C at 14,000 ×g for 10 min. The pellet was used for DNA extraction and the supernatant was filtered into glass vials through a 0.45 μm nylon membrane. HPLC analysis (Thermo Fisher Scientific Inc. Accela, Wohlen, Switzerland) was performed to determine the concentrations of SCFAs (formate, propionate, acetate, butyrate, and valerate), branched-chain fatty acids (BCFAs) (isobutyrate and isovalerate) and intermediate metabolites (lactate and succinate). Analyses were performed with an Accela Chromatography System and RI-detector (Thermo Fisher Scientific Inc., Reinach, Switzerland), equipped with a Security Guard Carbo-H cartridge (4 × 3.0 mm) and a Rezex ROA-Organic Acid H+ column (300 × 7.8 mm). Volumes of 40 μL were injected into the HPLC with a flow rate of 0.4 mL/min and H2SO4 as an eluent (Poeker et al., 2018).
Microbial Community Analysis
Genomic DNA Extraction
Total genomic DNA was extracted from 100 to 200 mg of caecal contents and the pellet of 2 mL of fermentation effluent using the FastDNA® SPIN Kit for Soil (MP Biomedicals, Illkirch Cedex, France) according to the manufacturer’s instructions. Total DNA concentration was measured by spectrophotometry using a Nanodrop®ND-1000 Spectrophotometer (Wiltec AG, Littau, Switzerland) and samples were stored at -20°C until analysis.
Quantitative PCR Analysis
DNA extracts were used for qPCR to enumerate the gene copy numbers of total bacteria and the specific marker bacterial groups Firmicutes, Ruminococcaceae, Lachnospiraceae, Lactobacillus–Leuconostoc–Pediococcus, Bacteroidetes, Bacteroides, Enterobacteriaceae, and Akkermansia (Supplementary Table S2). qPCR assays were performed using 1 μL of 10- or 100- fold diluted genomic DNA, 2x SYBR Green qPCR Mastermix (Life Technologies, Labgene Scientific Instruments, Zug, Switzerland), 100 μM of each forward and reverse primer, resulting in a total volume of 25 μL in a 96-well plate. The analysis was performed in an ABI PRISM 7500-PCR -sequence detection system (Applied Biosystems, Zug, Switzerland). Each reaction was run in duplicate. For quantification, a dilution series of standards was obtained by amplification of the linearized plasmids containing the gene of a representative bacterial species belonging to the target group, and included in each run (Pham et al., 2017). Primer specificity and verification of the presence of the desired amplicon was determined by melting curve analysis. PCR efficiency (%) was calculated from the slope of the standard curve of each qPCR assay. Assays with an efficiency of 80–110% (slope of 3.2–3.9) were included in data analysis.
Microbiota Profiling With 16S rRNA Amplicon Sequencing
The bacterial community in caecal samples was analyzed using the primers 341F (5′-CCTAYGGGRBGCASCAG-3′) and 806R (5′-GGACTACNNGGGTATCTAAT-3′), which flank the V3-V4 region. MiSeq adaptors were added by PCR. Sequencing of caecum microbiota of different mice was performed with Illumina MiSeq (Genotoul, Castanet-Tolosan Cedex Mainz, France). For the microbiota analysis of caecal inocula and fermentation samples of the final models 5 and 5∗, the V4 region of the 16S rRNA gene was amplified with primers 515F (5′-GTGCCAGCMGCCGCGGTAA-3′) and 806R (5′-GGACTACHVGGGTWTCTAAT-3′). Sequencing was performed with Illumina MiSeq (Genomic Diversity Centre, ETH Zurich, Zurich, Switzerland) with V2 reagent kit for 2 × 250 bp paired end Next Tera chemistry supplemented with 20% of PhiX. The raw sequence data has been submitted to European Nucleotide Archive (ENA) database with accession number PRJEB30419. The open-source bioinformatics pipeline Quantitative Insight Into Microbial Ecology (QIIME) (Caporaso et al., 2010) was used to process the raw 16S rRNA gene sequencing data. The raw dataset containing pair-ended reads with corresponding quality scores were merged and trimmed using fastq_mergepairs and fastq_filter scripts implemented in the UPARSE pipeline (Edgar, 2013) as previously described (Krych et al., 2018). The minimum overlap length of trimmed reads was set to 50 bp (V4) or 20 bp (V3V4). The minimum length of merged reads was 180 bp (V4) or 200 bp (V3V4), the max expected error E = 2.0, and first truncating position with quality score N ≤ 4. Purging the dataset from chimeric reads and constructing de novo Operational Taxonomic Units (OTU) were conducted using the UPARSE pipeline (Edgar, 2013). The green genes 16S rRNA gene collection (version 13.8) was used as a reference database (Werner et al., 2012) and an OTU count table including taxonomy was generated. The OTUs assigned to the S24-7 family were reported in the manuscript as taxa of the Muribaculaceae family (Lagkouvardos et al., 2016). QIIME open source software package (1.8.0 and 1.9.0) was used for subsequent analysis, including alpha and beta diversity, and the PICRUSt (phylogenetic investigation of communities by reconstruction of unobserved states) (Langille et al., 2013) analysis to predict the KEGG metabolic pathways and COG functional groups from microbiota samples.
Statistical Analysis
To compare SCFA concentrations between fermentation models, statistical tests were carried out using SigmaPlot 13.0 (SigmaPlot Software, La Jolla, CA, United States). Significance level was set at 0.05. Normality of the data set was tested with the Kolmogorov–Smirnov test. In case of normality, mean values of two different groups were compared with an independent samples t-test. In case of non-normality, differences were tested with non-parametric Mann–Whitney U test. Data are expressed as means ± standard deviations (SD) of 3 days of the stabilization and treatment periods with standard observed variations of metabolites lower than 10% in the same reactor in each fermentation. To assess if there were significant differences in taxa abundance between caecal inoculum and PolyFermS microbiota, or between different PolyFermS microbiota, the DESeq2 method (Love et al., 2014) was used on the unnormalized count data as previously suggested by McMurdie and Holmes (2014).
Results
In the first step, caecal physiological parameters such as pH, bacterial fermentation metabolites and composition were investigated for the C57BL/6 mouse strain. This breed was selected to inoculate the fermenters because it is the most widely used strain in biomedical and gut microbiota research (Bryant, 2011). To establish the in vitro murine caecal microbiota model, we analyzed fermentation metabolites by HPLC and quantified specific bacterial populations, particularly the stress- and oxygen-sensitive families Lachnospiraceae and Ruminococcaceae, and the genus Lactobacillus by qPCR as main markers for representative bacterial groups of murine gut microbiota. Overgrowth of the Enterobacteriaceae family was also monitored to detect a potential lack of control of oxygen stress during sampling and start-up of fermentation. These microbial indicators were used to suggest subsequent changes during the sampling, bead colonization and continuous fermentation process to achieve a balance akin to the mouse caecum content used for immobilization and inoculation of the continuous fermentation model. The final conditions set for model 5 were repeated with two caecal inocula prepared from different mice (model 5∗).
Composition of Caecal WT C57BL/6 Mouse Microbiota and Metabolites
Caecal pH in WT C57BL/6 mice (n = 15) ranged from 6.2 to 6.9 with a mean of 6.5 ± 0.2 (Figure 1A). Caecal fermentation metabolites; the SCFAs acetate, propionate and butyrate; the intermediates succinate and lactate; and the BCFA isobutyrate, were detected in all murine caeca (Figure 1B). Important individual variations of total metabolite concentration (17.7–74.1 μmol/g; average of 56.7 ± 14.6 μmol/g), and of the main end metabolites acetate (28.5 ± 5.0 μmol/g), propionate (5.1 ± 1.4 μmol/g) and butyrate (23.5 ± 5.9 μmol/g) were measured, while succinate (between 1 and 11 μmol/g), lactate (1–2 μmol/g), and isobutyrate (1–2 μmol/g) were detected at lower concentrations. The average acetate:propionate:butyrate ratio was 50:9:41, which was in the range of previous reports in same mouse strain on similar chow (Krautkramer et al., 2017). Caecal microbiota were dominated by bacterial phyla Firmicutes (56.0 ± 8.0%) and Bacteroidetes (38.9 ± 7.3%), and also harbored Proteobacteria at lower levels (4.6 ± 1.3%) (Figure 1C). Within Bacteroidetes, Muribaculaceae (S24-7) and Rikenellaceae were the most abundant families, and within the Firmicutes an unclassified Clostridiales family, Lachnospiraceae and Ruminococcaceae (Figure 1D). The α-diversity Shannon index (H) ranged from 5.6 to 6.2 (average 5.9 ± 0.9) (data not shown). Principal Coordinate Analysis (PCoA) was used to analyze β-diversity to characterize the degree of individual variations among the caecal murine microbial communities. Mice of the same age co-housed within the same cage for 21 days or in different cages were analyzed. The caecal microbiota of mice housed in the same cage did not cluster and individual mouse microbiota scattered in both unweighted and weighted Unifrac distance PCoA (Supplementary Figure S1), indicating a large inter-individual variation in caecal microbiota composition.
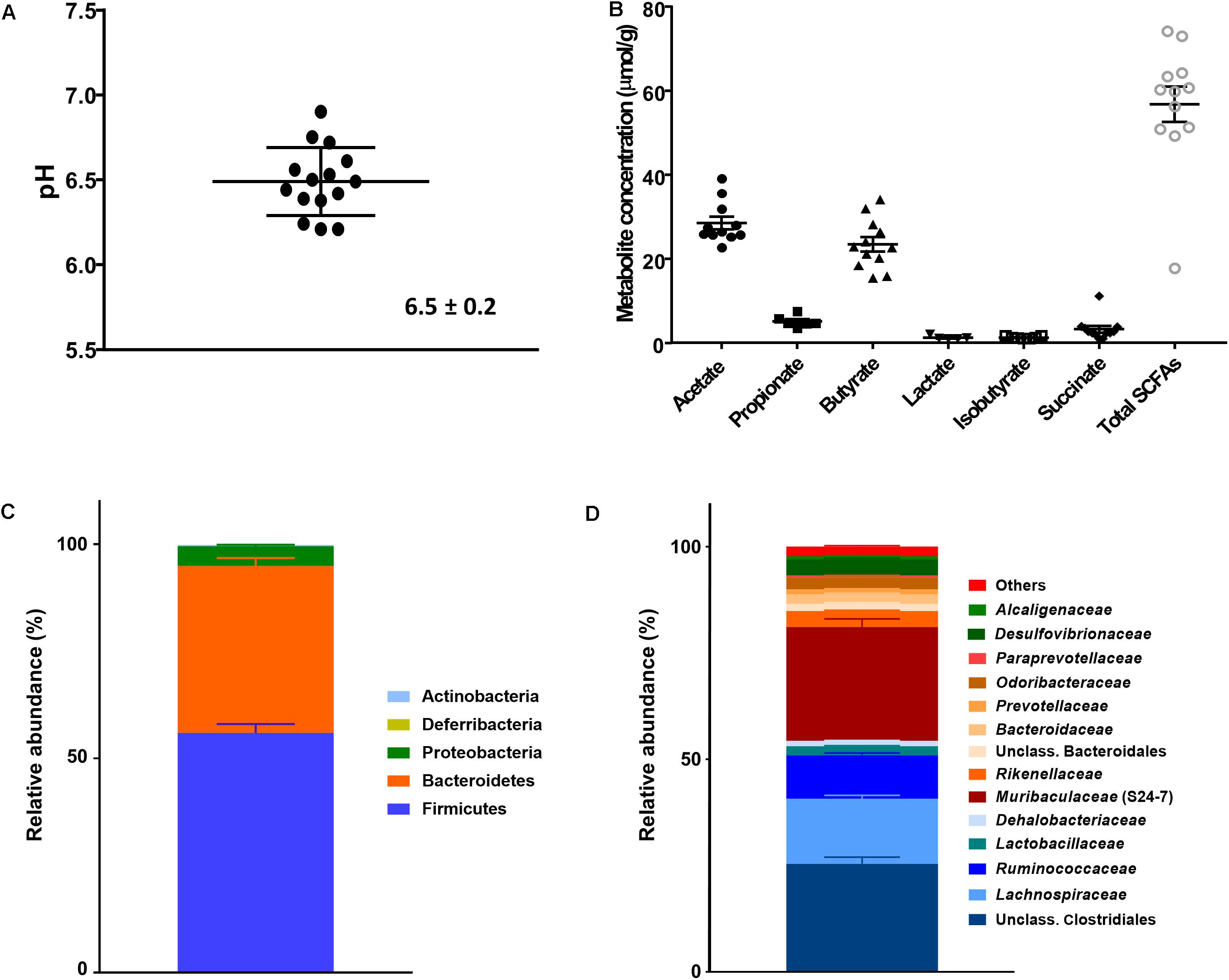
Figure 1. Analysis of caecal contents of WT C57BL/6 mice (means ± SEM; n = 15). (A) pH; (B) Metabolite concentrations (μmol/g); (C) Microbial composition obtained by 16S rRNA amplicon sequencing and expressed as relative abundance at phylum and (D) family level. Data are mean ± SEM. Values < 1% are summarized in the group «Others».
Development of in vitro Model for Murine Caecal Microbiota Fermentation
During bead colonization, the effects of pH in combination with different nutritive media as well as initial batch fermentations conditions for bead colonization were assessed. During continuous operation, the impact of pH in combination with different RTs were investigated to improve maintenance of activity and composition of the murine caecal microbiota in vitro and compare to in vivo caecum data.
In model 1, caecal content sampling and processing was performed rapidly but under aerobic conditions and bead colonization was done at pH 7 in nutritive medium 1 supplemented with 20% (v/v) fermentation effluent during three batch cultures (Table 1A). During continuous operation, constant conditions of pH 7 and RT 9 h were used. Metabolic stability was observed after 4 days of continuous fermentation with a mean total metabolite concentration of 115 ± 10 mM from day 4 to day 12 (Figure 2A and Supplementary Figure S2A). Acetate (65 ± 4 mM) was the main produced metabolite, followed by butyrate (14 ± 3 mM) and propionate (15 ± 4 mM) (Supplementary Table S3). We observed higher levels of lactate, an intermediate fermentation metabolite, in the caecal inoculum compared to the first sampled caecal contents (Figure 1B) and compared to in vitro levels (Figure 2A). This might be because caecal contents for model 1 were sampled in the morning shortly after dark period and it was previously observed that lactate levels were then highest (Hamaguchi et al., 2015; Tahara et al., 2018). At the bacterial compositional level, a decrease in butyrate-producing families Ruminococcaceae (-1.2 log gene copies) and Lachnospiraceae (-0.6 log gene copies) was observed compared to the corresponding caecal inoculum (Figure 2B and Supplementary Table S4). Lactobacillus spp. were also decreased in vitro compared to the caecal inoculum (-2.4 log gene copies vs. in vivo), while Enterobacteriaceae were increased (+1.5 log gene copies vs. in vivo).
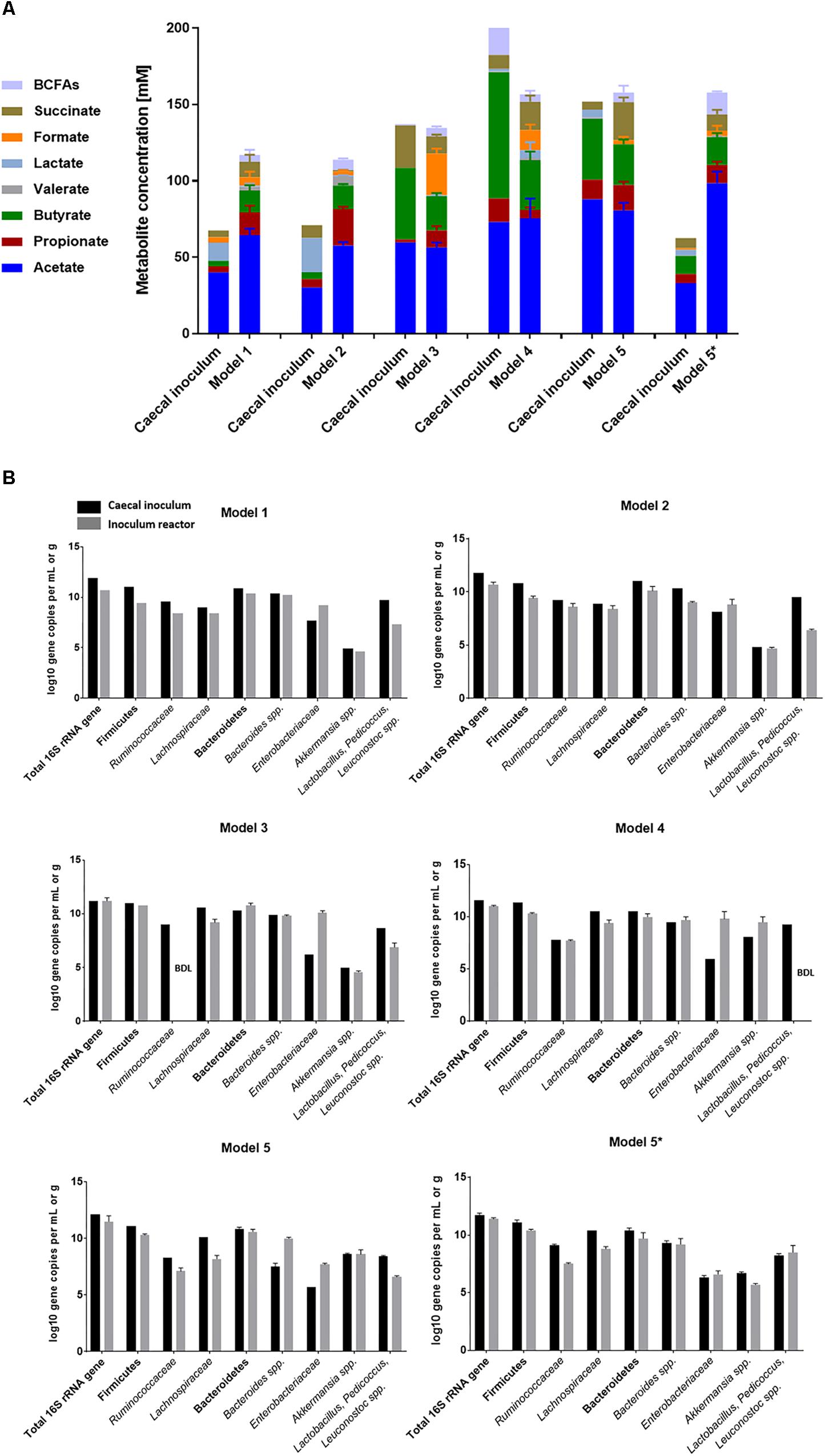
Figure 2. Bacterial activity and composition of caecal inocula and reactor effluents of different models. (A) Concentrations of metabolites (mM) in caecal inocula and reactor effluents of stabilization phases expressed as mean metabolite concentrations with standard error. (B) Quantification of key bacterial populations in caecal inocula and fermentation samples of different models by qPCR and expressed as means ± SD log gene copies/g or mL when n > 1. BDL, below detection limit of 4 log10 gene copies.
We assumed that the short exposure to oxygen during sample collection favored the growth of facultative anaerobes, including Enterobacteriaceae and was detrimental to strict anaerobes such as bacterial taxa within Clostridiales. Therefore, in model 2 the sampling procedure was adapted to better protect the microbiota from oxygen. In addition, the batch fermentation medium was supplemented with lactate to enhance the growth and activity of lactate-consuming butyrate-producers within the bacterial order Clostridiales (Bourriaud et al., 2005; Tao et al., 2016). Metabolic stability of model 2 was reached after 12 days of continuous operation (Figure 2A and Supplementary Figure S2B) with mean total metabolite production of 114 ± 3 mM. In model 2, an acetate:propionate:butyrate (AA:PA:BA) ratio of 61:24:15 was observed, with slightly increased propionate levels compared to the caecal inoculum (73:15:12) (Supplementary Table S3). Compared to the caecal microbiota inoculum, Enterobacteriaceae (+0.7 log gene copies) were increased in model 2 effluents, while Lactobacillus spp. (-3.1 log gene copies) and butyrate-producers (Lachnospiraceae: -0.5 log gene copies; Ruminococcaceae: -0.6 log gene copies) were decreased (Figure 2B and Supplementary Table S4).
The medium of model 3 was supplemented with both cellobiose and rumen fluid to improve bead colonization of butyrate-producing bacteria and to enhance the growth of Lactobacillus spp. Rumen fluid contains primary nutrients for cross-feeding and microbial growth factors (Bryant, 1959; Kamada et al., 2013; van Zanten et al., 2014). In addition, the number of bead colonization batch fermentations was reduced to two, and the duration for the first batch was extended to 16 h to decrease the growth advantage of fast-growing Enterobacteriaceae, promote growth and activity of butyrate-producing bacteria (Clostridia), and achieve a more complete carbohydrate fermentation with high re-utilization of intermediate metabolites (lactate, formate, acetate, succinate). Metabolic stability of model 3 was reached after 15 days, with a higher mean total metabolite concentration (135 ± 4 mM) compared to model 2 (p < 0.05) and model 1 (p < 0.05) and a AA:PA:BA molar ratio of 63:12:25 (Figure 2A, Supplementary Table S3, and Supplementary Figure S3A). However, concentrations of the butyrate-producing families Lachnospiraceae (-1.1 log gene copies vs. in vivo) and Ruminococcaceae (below detection limit) remained low compared to caecal inoculum. Further, lower levels of Lactobacillus spp. (-1.8 log gene copies) and higher levels of Enterobacteriaceae (+3.9 log gene copies) were detected in the effluent samples compared to the caecal inoculum (Figure 2B and Supplementary Table S4).
Other possible strategies to prevent the outgrowth of Enterobacteriaceae are reducing the concentration of simple carbohydrates in the nutritive medium (here: corn starch), and decreasing the pH (from pH 7 to 5.8 during batch fermentation and from pH 7 to 6.5 during continuous operation), since optimal growth pH of these bacteria is close to neutrality (Supplementary Table S1 and Table 1). After 19 days of continuous culture, steady metabolite production was reached with higher total metabolite production (154 ± 13 mM) compared to previous model 3 (p < 0.05) and a AA:PA:BA ratio of 66:4:29 (Figure 2A, Supplementary Table S3, and Supplementary Figure S3B). High butyrate levels were observed in caecal inoculum (83 mM) and reactor effluent (33 mM), which can be associated with high and comparable levels of butyrate-producing families Ruminococcaceae (7.7 ± 0.1 log gene copies/mL and 7.5 log gene copies/g, respectively) and Lachnospiraceae (9.4 ± 0.3 log gene copies/mL and 10.5 log gene copies/g, respectively; Figure 2B and Supplementary Table S4). However, the concentration of Enterobacteriaceae remained high (+3.8 log gene copies/mL compared to caecal inoculum) and Lactobacillus spp. remained below the detection limit.
A recent study found that some bacterial populations within Clostridiales order are positively associated with long RTs in humans, which may also promote growth conditions for the slow-growing bacterial populations in our model (Roager et al., 2016). Therefore, in model 5 we assessed whether an increase in RT from 9 h (model 4) to 12 h can prevent overgrowth of fast-growing Enterobacteriaceae. A high total metabolite production was obtained (158 ± 9 mM) with higher levels of propionate (p < 0.05) and lower levels of the intermediate metabolite formate (p < 0.05) compared to model 4 (Figure 2A, Supplementary Figure S4, and Supplementary Table S3). The AA:PA:BA ratio in vitro (65:14:22) was comparable with the ratio detected in the caecal inoculum (62:9:28). Concentrations of potential butyrate-producing bacterial markers Lachnospiraceae (8.2 ± 0.3 log gene copies/mL vs. 10.1 ± 0.0 log gene copies/g) and Ruminococcaceae (7.1 ± 0.3 log gene copies/mL vs. 8.3 ± 0.0 log gene 372 copies/g) were lower in effluent samples compared with caecal inoculum levels (Figure 2B and Supplementary Table S4). Despite this, increased retention resulted in less overgrowth of Enterobacteriaceae (5.7 ± 0.1 log gene copies/g caecal content vs. 7.7 ± 0.1 log gene copies/mL model 5 effluent) and no severe loss of Lactobacillus spp. (8.4 ± 0.1 log gene copies/g caecal content vs. 6.6 ± 0.1 log gene copies/mL model 5 effluent) compared to model 4.
As model 5 conditions reflected the metabolic and bacterial concentrations of the mouse caecum adequately, an independent repetition of model 5 was performed (starting from another caecal microbiota inoculum; referred to as model 5∗). The total fermentation metabolite production (161 ± 7 mM) was comparable to levels in model 5 (Figure 2A and Supplementary Table S3). Overall, the metabolite production in both model 5 and 5∗ was stable during continuous operation of 69 and 43 days, respectively (Supplementary Figures S4, S5). At the bacterial marker level, comparable concentrations of Bacteroides spp. and Akkermansia spp. were detected in model 5∗ effluent samples compared to its caecal inoculum (Supplementary Table S4). Interestingly, the in vitro Akkermansia spp. levels (5.7 ± 0.1 log gene copies/mL) reflected the lower concentrations present in caecal inoculum (6.7 ± 0.1 log gene copies/g in caecal inoculum 5∗ vs. 8.6 ± 0.1 log gene copies/g in caecal inoculum 5). Enterobacteriaceae spp. (6.6 ± 0.3 log gene copies/mL) and Lactobacillus spp. (8.5 ± 0.6 log gene copies/mL) established at comparable levels to those detected in the caecal inoculum (6.3 ± 0.1 log gene copies/g and 8.2 ± 0.2 log gene copies/g, respectively).
Microbiota Analysis of Final in vitro Continuous Fermentation Murine Caecum Model
To assess the overall microbiota composition and diversity in comparison to the caecal inocula, reactor effluent microbiota of model 5 and 5∗ were further analyzed by 16S rRNA gene sequencing. The composition of reactor microbiota differed from the caecal inoculum microbiota as indicated in a PCoA-biplot on weighted and unweighted UniFrac distance (Figures 3A,B). Furthermore, model 5 microbiota differed from model 5∗ microbiota as shown by a spatial separation and clustering in both unweighted and weighted UniFrac PCoA biplots. The two caecal inocula microbiota showed scattering in the unweighted UniFrac PCoA, indicating qualitative differences in their composition.
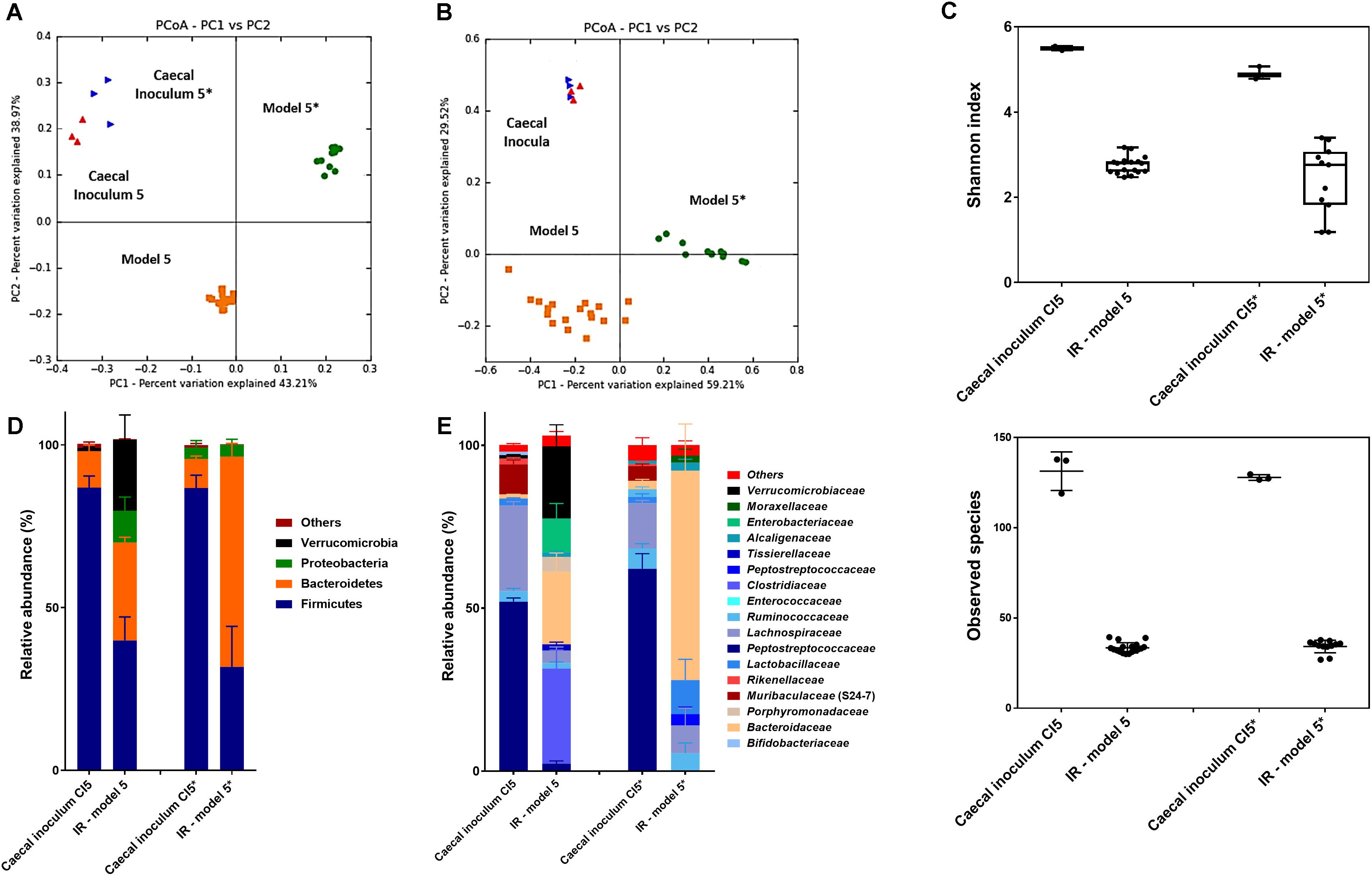
Figure 3. Microbial composition and diversity analysis in caecal inocula and reactor effluents of model 5 and 5∗. (A,B) Principle Coordinate Analysis (PCoA) of caecal inocula and reactor microbiota based on unweighted (A) and weighted (B) UniFrac analysis matrix on OTU level. Each point represents a microbiota sample from murine caecal content used as inoculum for model 5 (red ) and model 5∗ (blue
) or from stabilized reactor effluents of model 5 (orange
) and model 5∗ (green
). (C) Alpha diversity measured by Shannon diversity index and observed species. The box depicts distribution of diversity index for caecal inocula and fermentation samples. Boxes represent the interquartile range (IQR) between the 5th and 95th percentiles, respectively. (D,E) Microbial composition in caecal samples of WT C57BL/6 mice obtained by 16S rRNA amplicon sequencing. Relative abundance at phylum and family level of caecal inocula and fermentation samples of model 5 and 5∗. Data are mean ± SD. Values < 1% are summarized in the group «Others».
The bacterial diversity within the reactor microbiota was lower compared to the caecal inocula microbiota (Figure 3C). Both the Shannon diversity-index that takes into account the number of observed OTUs and their relative evenness and the observed species diversity decreased from 5.2 for caecal inocula to 2.7 ± 0.6 for reactor effluent samples and from 130 to 35, respectively.
Overall abundance shifts from in vivo to in vitro caecal microbial communities occurred (Figures 3D,E). Compared to the caecal inocula, the Bacteroidetes phylum abundance increased while Firmicutes phylum abundance decreased in both in vitro microbiota; this shift was most pronounced in model 5∗ (Figure 3D and Supplementary Table S5). Certain genera and species from the caecal inoculum flourished in vitro, while others became established at a lower abundance or below the detection limit. This resulted in significant and large log2 fold changes in abundance when comparing caecal inoculum to corresponding fermentation samples using DeSeq2 analysis (Supplementary Figures S6, S7). Taxa from Bacteroides, Anaerococcus and unclassified Clostridiaceae genera were enriched in vitro in both models with a log2 fold change exceeding 4. Some abundant (>1%) taxa in the caecal inocula established at a lower abundance (unclassified Clostridiales and Lactobacillus genera) or were not detected (taxa from Muribaculaceae (S24-7) family).
Compositional differences between the two in vitro microbiota were observed, which were in line with the quantitative differences detected by qPCR (Supplementary Figure S8). Model 5 in vitro microbiota was characterized by taxa belonging to Akkermansia, Enterobacteriaceae, Parabacteroides, and Clostridiaceae, while in model 5∗, in vitro microbiota taxa belonging to Lactobacillus, Peptostreptococcaceae, Blautia, and Anaerofilum established better compared to model 5.
We next predicted the gene content from the 16S rRNA sequence data by PICRUSt (phylogenetic investigation of communities by reconstruction of unobserved states) for revealing potential functional differences between both in vitro- and caecal inocula microbiota. Conserved metabolic and functional KEGG pathways were observed in both microbiota types (Supplementary Figure S9), indicating a similar microbial functional potential between in vitro- and caecal inocula microbiota.
Discussion
In this study, we aimed to develop a continuous in vitro fermentation model that reflects the metabolic activity and phylogeny of healthy WT mouse caecal microbiota. An important prerequisite for in vitro studies is the rational selection of models and conditions, while keeping in mind that these models can never completely represent reality (Lacroix et al., 2015). Therefore, we followed a stepwise approach for model development by adjusting parameters to reach fermentation metabolite profiles and marker bacterial levels similar to the in vivo situation.
During continuous operation a stable in vitro fermentation with main fermentation metabolites acetate, propionate and butyrate was reached, which is in line with in vivo measurements in this and other studies reporting ratios of murine caecal microbial fermentation metabolites (Burokas et al., 2017; Krautkramer et al., 2017; Herrmann et al., 2018). In vivo, the SCFA are continuous and efficiently absorbed by the intestinal epithelium (Morrison and Preston, 2016), resulting in an underestimation of the actual caecal fermentation capacity based on caecal SCFA measurements. Accordingly, the total SCFA concentrations in vitro were higher than in vivo because absorption is not simulated. Hence, model values reflect the total fermentation capacity of the modeled caecal microbiota. First models showed a limited fermentation capacity, but thanks to the optimization steps the total metabolite production increased from 115 mM in model 1 to 159 mM and 161 mM in model 5 and 5∗, respectively. Along with the improved fermentation capacity, a simultaneous longer stabilization time of the models was observed; this might be explained by the growth and balance of a more complex microbiota reliant on cross-feeding mechanisms. A lower pH during startup and continuous operation (models 3–4) stimulated bacterial fermentation as previously observed in human microbiota fermentation models (Walker et al., 2005; Zihler et al., 2013). Increased RT (model 4–5) resulted in decreased accumulation of the intermediate metabolites formate and lactate, and higher levels of branched SCFA, which are specific markers for protein fermentation and associated with long RTs (Davila et al., 2013; Tottey et al., 2017). Succinate was after acetate, propionate and butyrate the microbial metabolite detected at the highest concentrations in all caecal murine fermentation models and their respective caecal inocula. In humans, succinate is considered an intermediate metabolite in the global intestinal microbiota fermentation process (Macfarlane and Macfarlane, 2003), since several gut bacteria can convert succinate to propionate or butyrate (Louis and Flint, 2017). In mice, high levels of caecal succinate were reported in response to dietary fiber treatment (Everard et al., 2014) and high succinate levels have been demonstrated to improve glucose metabolism via intestinal gluconeogenesis (De Vadder et al., 2016). When sufficient carbohydrates are present, Bacteroides taxa show reduced need to decarboxylate succinate; thus succinate accumulates instead of propionate (Macy et al., 1978). Furthermore, in Bifidobacteria succinate production is associated with growth (Van Der Meulen et al., 2006), which may explain the high levels detected in our continuous fermentation model due to continuous supply of carbohydrates and therefore growth of these bacteria. The qualitative assessment of the predicted microbial functions by PICRUSt indicated that the gene contents of most pathways were maintained in our model, despite changes in abundances of bacterial populations. These results also suggest that the reactor microbiota as a whole did not change its functional fermentation potential, such as metabolic cross-feeding pathways, from in vivo (caecal inoculum) to in vitro (reactor).
The in vitro murine caecal microbiota was mainly composed of the bacterial phyla Firmicutes and Bacteroidetes, both in the range of in vivo caecal microbiota compositions, previously reported (Ley et al., 2006; Clavel et al., 2016; Hugenholtz and de Vos, 2017). However, there was a shift toward higher Bacteroidetes levels compared to caecal inocula; similar shifts were reported for in vitro human intestinal microbiota models (Rajilic-Stojanovic et al., 2010; Van den Abbeele et al., 2010; McDonald et al., 2013; Fehlbaum et al., 2015). The high levels of complex carbohydrates in nutritive media used for intestinal fermentation models may favor the growth of Bacteroidetes taxa, since they have a higher glycan-degrading capacity compared to Firmicutes species (Mahowald et al., 2009). The taxa within murine bacterial families that were maintained in the in vitro fermentations belong to important functional groups for intestinal fermentation such as primary fibrolytic (Bacteroidaceae, Ruminococcaceae, Bifidobacteriaceae), glycolytic (Lactobacillaceae, Enterococcaceae, Enterobacteriaceae) and mucolytic (Verrucomicrobiaceae, Bacteroidaceae) bacteria; and secondary butyrate- and propionate-producing bacteria (Lachnospiraceae, Ruminococcaceae, Erysipelotrichaceae, Rikenellaceae, Verrucomicrobiaceae) (Chassard and Lacroix, 2013). The prevalent murine intestinal bacterial family Muribaculaceae (S24-7) was not maintained in our in vitro model. Only recently, Lagkouvardos et al. (2016) succeeded to isolate and cultivate the first strain of this family in a medium containing meat and blood. Interestingly, a recent study reported that this Muribaculaceae (S24-7) strain is extremely sensitive to high osmolality (Tropini et al., 2018) and the higher osmolality in our reactors compared to caecum may also explain its low establishment in vitro. Further characterization of the physiology and nutritional requirements of strains from the Muribaculaceae family and other taxa that were not maintained in vitro will be important to further optimize our murine nutritive fermentation medium. Adjustments to nutritive medium, pH and RT helped to control the in vitro levels of Enterobacteriaceae, which often bloom in fermentation models (Fehlbaum et al., 2015; McDonald et al., 2015) due to their competitive advantage during initial colonization and balancing of the fermentation model.
There was an important overall decrease in bacterial diversity in vitro compared to the high caecal inoculum diversity, which were in the range of published Shannon diversity indices ranging from 4.5 to 6 (Holm et al., 2015; Hu et al., 2017). Such effects were also observed in other in vitro fermentation models inoculated with high diverse intestinal microbial communities from humans (infants, adult and elderly) (Rajilic-Stojanovic et al., 2010; Van den Abbeele et al., 2010; McDonald et al., 2013; Feria-Gervasio et al., 2014; Poeker et al., 2018) and swine (Tanner et al., 2014). In vitro models cannot simulate all the conditions occurring in the host, which are not well-known or cannot be mimicked such as immune response, variation in feed rates and composition, hormonal and digestive secretions (e.g., bile), feedback mechanisms, absorption and peristaltic movements, all of which influence microbial diversity and can only be an approximation of realistic conditions (Lacroix et al., 2015). The well-controlled conditions in vitro may result in a loss of redundant species or species thriving on specific host secretions. Murine intestinal bacterial isolation efforts (Lagkouvardos et al., 2016) offer the opportunity to identify species-specific growth requirements and will allow adaptation of fermentation conditions for their improved establishment in vitro. Finally, the presence of transient bacteria, i.e., from upper gastrointestinal tract, diet and ingested microbes due to coprophagy, in the caecal inocula may also overestimate the bacterial diversity of the resident caecal community. By mimicking the murine caecal conditions, we obtained a microbial community composed of caecal murine-derived bacteria, with reduced complexity but resulting in a stable and functional caecal fermentation.
Mouse experiments have gained attention as tool to study the gut microbiota in health and disease. However, their poor reproducibility within and between facilities was associated with high variability among the mouse gut microbiota, which makes it hard to draw robust conclusions (Laukens et al., 2015). Factors contributing to the heterogeneity of the mouse gut microbiota within a facility include differences in food intake (Zarrinpar et al., 2014), maternal effects (Hufeldt et al., 2010), hormones (Org et al., 2016), cage (Hildebrand et al., 2013), presence of surrounding animals (Rausch et al., 2016), and other stressors (Bangsgaard Bendtsen et al., 2012) and environmental factors. To control for these factors, our strategy is to uncouple the microbiota from the host and study it under very well-controlled conditions (e.g., fermenter). In contrast to in vivo models, in vitro models allow study of the dynamics of the complex microbiota following manipulations, particularly to follow production of in situ fermentation metabolites, which are partly absorbed in vivo. Identified microbes or metabolites that drive microbiota functionality can then be validated further in vivo. Moreover, the continuous cultivated in vitro murine caecal microbiota can be used as transplantation material to study host–microbiota interactions in murine models of health and disease. This approach is currently performed with pooled murine fecal material, which is less controlled and available, or with bacterial consortia of human- or mouse-derived strains (Clavel et al., 2016). Recently the Oligo-Mouse-Microbiota (Brugiroux et al., 2016), a mixture of up to 15 intestinal murine-derived strains from the mouse intestinal bacterial collection (Lagkouvardos et al., 2016), was established following a bottom-up approach of rational strain selection. Alternatively, with our novel murine caecal fermentation model we can follow a top–down approach of creating functional different murine-derived bacterial communities with higher diversity compared to current consortia.
Conclusion
We showed that it is feasible to maintain a stable and simplified, but yet representative in vitro murine microbiota in a continuous murine caecal fermentation model inoculated with immobilized caecal microbiota. Our simplified, yet representative in vitro murine bacterial community showed a similar functionality to inoculum microbiota. We demonstrated that it is feasible to continuously cultivate caecal murine microbiota while maintaining its overall functionality over a long time period. Our model is a first step in the development of a mouse microbiota model system. With the expected increased knowledge of mouse gut isolates, further improvements of our murine in vitro model can be carried out by fine-tuning operational or nutritional requirements, and hence to increase preservation of microbial diversity in our model. In addition, our model can be expanded further with second-stage reactors, continuously inoculated with reactor effluent to allow parallel testing of different manipulations on the same microbiota. Hence, our novel in vitro model is a promising tool for studying the murine microbe–microbe interactions in response to biotic or abiotic factors that are linked to gut microbial functionality and structure.
Ethics Statement
Mouse experiments were conducted according to Swiss animal welfare legislation, and the local veterinary office approved all procedures (Veterinäramt des Kantons Zürich; Nr. ZH220/2016).
Author Contributions
SP, AG, TW, and CL conceived the experiments. SP, AG, and TW conducted the experiments. SP and AG analyzed 16S rRNA sequence, HPLC and qPCR data. SP prepared the figures. SP, AG, MRS, MS, and CL interpreted the results. SP, AG, and CL wrote the manuscript. MRS and MS bred and sacrificed the mice. All authors reviewed the manuscript.
Funding
This study was funded by the Swiss National Science Foundation (Sinergia Project No. CRSII3_154488).
Conflict of Interest Statement
The authors declare that the research was conducted in the absence of any commercial or financial relationships that could be construed as a potential conflict of interest.
Acknowledgments
The authors would like to thank Annica Jucker, Helen Hobi, and Jasmin Hirt for technical assistance, Alfonso Die for the technical assistance during SCFA analysis and Anna Greppi and people from Genetic Diversity Center at ETH Zurich for support during 16S amplicon sequencing preparation.
Supplementary Material
The Supplementary Material for this article can be found online at: https://www.frontiersin.org/articles/10.3389/fmicb.2019.01166/full#supplementary-material
References
Bangsgaard Bendtsen, K. M., Krych, L., Sørensen, D. B., Pang, W., Nielsen, D. S., Josefsen, K., et al. (2012). Gut microbiota composition is correlated to grid floor induced stress and behavior in the BALB/c mouse. PLoS One 7:e46231. doi: 10.1371/journal.pone.0046231
Bourriaud, C., Robins, R. J., Martin, L., Kozlowski, F., Tenailleau, E., Cherbut, C., et al. (2005). Lactate is mainly fermented to butyrate by human intestinal microfloras but inter-individual variation is evident. J. Appl. Microbiol. 99, 201–212. doi: 10.1111/j.1365-2672.2005.02605.x
Brugiroux, S., Beutler, M., Pfann, C., Garzetti, D., Ruscheweyh, H.-J., Ring, D., et al. (2016). Genome-guided design of a defined mouse microbiota that confers colonization resistance against Salmonella enterica serovar Typhimurium. Nat. Microbiol. 2:16215. doi: 10.1038/nmicrobiol.2016.215
Bryant, C. D. (2011). The blessings and curses of C57BL/6 substrains in mouse genetic studies. Ann. N. Y. Acad. Sci. 1245, 31–33. doi: 10.1111/j.1749-6632.2011.06325.x
Burokas, A., Arboleya, S., Moloney, R. D., Peterson, V. L., Murphy, K., Clarke, G., et al. (2017). Targeting the microbiota-gut-brain axis: prebiotics have anxiolytic and antidepressant-like effects and reverse the impact of chronic stress in mice. Biol. Psychiatry 82, 472–487. doi: 10.1016/j.biopsych.2016.12.031
Caporaso, J. G., Kuczynski, J., Stombaugh, J., Bittinger, K., Bushman, F. D., Costello, E. K., et al. (2010). QIIME allows analysis of high-throughput community sequencing data. Nat. Methods 7, 335–336. doi: 10.1038/nmeth.f.303
Champy, M. F., Selloum, M., Zeitler, V., Caradec, C., Jung, B., Rousseau, S., et al. (2008). Genetic background determines metabolic phenotypes in the mouse. Mamm. Genome 19, 318–331. doi: 10.1007/s00335-008-9107-z
Chassard, C., and Lacroix, C. (2013). Carbohydrates and the human gut microbiota. Curr. Opin. Clin. Nutr. Metab. Care 16, 453–460. doi: 10.1097/MCO.0b013e3283619e63
Clavel, T., Lagkouvardos, I., Blaut, M., and Stecher, B. (2016). The mouse gut microbiome revisited: from complex diversity to model ecosystems. Int. J. Med. Microbiol. 306, 316–327. doi: 10.1016/j.ijmm.2016.03.002
Clavel, T., Lagkouvardos, I., and Stecher, B. (2017). From complex gut communities to minimal microbiomes via cultivation. Curr. Opin. Microbiol. 38, 148–155. doi: 10.1016/j.mib.2017.05.013
Cornick, S., Tawiah, A., and Chadee, K. (2015). Roles and regulation of the mucus barrier in the gut. Tissue Barriers 3:e982426. doi: 10.4161/21688370.2014.982426
Dahlqvist, A., and Thomson, D. L. (1963a). The digestion and absorption of maltose and trehalose by the intact rat. Acta Physiol. Scand. 59, 111–125. doi: 10.1111/j.1748-1716.1963.tb02728.x
Dahlqvist, A., and Thomson, D. L. (1963b). The digestion and absorption of sucrose by the intact rat. J. Physiol. 167, 193–209. doi: 10.1113/jphysiol.1963.sp007141
Davila, A. M., Blachier, F., Gotteland, M., Andriamihaja, M., Benetti, P. H., Sanz, Y., et al. (2013). Re-print of “intestinal luminal nitrogen metabolism: role of the gut microbiota and consequences for the host.”. Pharmacol. Res. 69, 114–126. doi: 10.1016/j.phrs.2013.01.003
De Vadder, F., Kovatcheva-Datchary, P., Zitoun, C., Duchampt, A., Bäckhed, F., and Mithieux, G. (2016). Microbiota-produced succinate improves glucose homeostasis via intestinal gluconeogenesis. Cell Metab. 24, 151–157. doi: 10.1016/j.cmet.2016.06.013
Dostal, A., Lacroix, C., Bircher, L., Pham, V. T., Follador, R., Zimmermann, M. B., et al. (2015). Iron modulates butyrate production by a child gut microbiota In Vitro. mBio 6:e01453-15. doi: 10.1128/mBio.01453-15
Edgar, R. C. (2013). UPARSE: highly accurate OTU sequences from microbial amplicon reads. Nat. Methods 10, 996–998. doi: 10.1038/nmeth.2604
Everard, A., Lazarevic, V., Gaïa, N., Johansson, M., Ståhlman, M., Backhed, F., et al. (2014). Microbiome of prebiotic-treated mice reveals novel targets involved in host response during obesity. ISME J. 8, 2116–2130. doi: 10.1038/ismej.2014.45
Fehlbaum, S., Chassard, C., Haug, M. C., Fourmestraux, C., Derrien, M., and Lacroix, C. (2015). Design and investigation of PolyFermS In Vitro continuous fermentation models inoculated with immobilized fecal microbiota mimicking the elderly colon. PLoS One 10:e0142793. doi: 10.1371/journal.pone.0142793
Feria-Gervasio, D., Tottey, W., Gaci, N., Alric, M., Cardot, J.-M., Peyret, P., et al. (2014). Three-stage continuous culture system with a self-generated anaerobia to study the regionalized metabolism of the human gut microbiota. J. Microbiol. Methods 96, 111–118. doi: 10.1016/j.mimet.2013.11.015
Flint, H. J., Scott, K. P., Louis, P., and Duncan, S. H. (2012). The role of the gut microbiota in nutrition and health. Nat. Rev. Gastroenterol. Hepatol. 9, 577–589. doi: 10.1038/nrgastro.2012.156
Freter, R., Stauffer, E., Cleven, D., Holdeman, L. V., and Moore, W. E. (1983). Continuous-flow cultures as in vitro models of the ecology of large intestinal flora. Infect. Immun. 39, 666–675.
Geirnaert, A., Wang, J., Tinck, M., Steyaert, A., Van den Abbeele, P., Eeckhaut, V., et al. (2015). Interindividual differences in response to treatment with butyrate-producing Butyricicoccus pullicaecorum 25-3T studied in an in vitro gut model. FEMS Microbiol. Ecol. 91:fiv054. doi: 10.1093/femsec/fiv054
Hamaguchi, Y., Tahara, Y., Kuroda, H., Haraguchi, A., and Shibata, S. (2015). Entrainment of mouse peripheral circadian clocks to < 24 h feeding/fasting cycles under 24 h light/dark conditions. Sci. Rep. 5:14207. doi: 10.1038/srep14207
Herrmann, E., Young, W., Reichert-Grimm, V., Weis, S., Riedel, C., Rosendale, D., et al. (2018). In Vivo assessment of resistant starch degradation by the caecal microbiota of mice using RNA-based stable isotope probing—a proof-of-principle study. Nutrients 10:179. doi: 10.3390/nu10020179
Herrmann, E., Young, W., Rosendale, D., Conrad, R., Riedel, C. U., and Egert, M. (2017). Determination of resistant starch assimilating bacteria in fecal samples of mice by In vitro RNA-based stable isotope probing. Front. Microbiol. 8:1331. doi: 10.3389/fmicb.2017.01331
Hildebrand, F., Nguyen, T. L. A., Brinkman, B., Yunta, R. G., Cauwe, B., Vandenabeele, P., et al. (2013). Inflammation-associated enterotypes, host genotype, cage and inter-individual effects drive gut microbiota variation in common laboratory mice. Genome Biol. 14:R4. doi: 10.1186/gb-2013-14-1-r4
Holm, J. B., Sorobetea, D., Kiilerich, P., Ramayo-Caldas, Y., Estellé, J., Ma, T., et al. (2015). Chronic trichuris muris infection decreases diversity of the intestinal microbiota and concomitantly increases the abundance of lactobacilli. PLoS One 10:e0125495. doi: 10.1371/journal.pone.0125495
Hu, J., Luo, H., Jiang, Y., and Chen, P. (2017). Dietary capsaicin and antibiotics act synergistically to reduce non-alcoholic fatty liver disease induced by high fat diet in mice. Oncotarget 8, 38161–38175. doi: 10.18632/oncotarget.16975
Hufeldt, M. R., Nielsen, D. S., Vogensen, F. K., Midtvedt, T., and Hansen, A. K. (2010). Family relationship of female breeders reduce the systematic inter-individual variation in the gut microbiota of inbred laboratory mice. Lab. Anim. 44, 283–289. doi: 10.1258/la.2010.010058
Hugenholtz, F., and de Vos, W. M. (2017). Mouse models for human intestinal microbiota research: a critical evaluation. Cell. Mol. Life Sci. 75, 149–160. doi: 10.1007/s00018-017-2693-8
Kamada, N., Chen, G. Y., Inohara, N., and Nunez, G. (2013). Control of pathogens and pathobionts by the gut microbiota. Nat. Immunol. 14, 685–690. doi: 10.1038/ni.2608
Kerr, K. R., Kappen, K. L., Garner, L. M., and Swanson, K. S. (2014). Commercially available avian and mammalian whole prey diet items targeted for consumption by managed exotic and domestic pet felines: macronutrient, mineral, and long-chain fatty acid composition. Zoo Biol. 33, 327–335. doi: 10.1002/zoo.21147
Krautkramer, K. A., Kreznar, J. H., Romano, K. A., Vivas, E. I., Barrett-wilt, G. A., Rabaglia, M. E., et al. (2017). Diet-microbiota interactions mediate global epigenetic programming in multiple host tissues. Mol. Cell 64, 982–992. doi: 10.1016/j.molcel.2016.10.025
Krych,Ł., Kot, W., Bendtsen, K. M. B., Hansen, A. K., Vogensen, F. K., and Nielsen, D. S. (2018). Have you tried spermine? a rapid and cost-effective method to eliminate dextran sodium sulfate inhibition of PCR and RT-PCR. J. Microbiol. Methods 144, 1–7. doi: 10.1016/j.mimet.2017.10.015
Lacroix, C., de Wouters, T., and Chassard, C. (2015). Integrated multi-scale strategies to investigate nutritional compounds and their effect on the gut microbiota. Curr. Opin. Biotechnol. 32, 149–155. doi: 10.1016/j.copbio.2014.12.009
Lagkouvardos, I., Pukall, R., Abt, B., Foesel, B. U., Meier-Kolthoff, J. P., Kumar, N., et al. (2016). The mouse intestinal bacterial collection (miBC) provides host-specific insight into cultured diversity and functional potential of the gut microbiota. Nat. Microbiol. 1:16131. doi: 10.1038/nmicrobiol.2016.131
Langille, M. G. I., Zaneveld, J., Caporaso, J. G., McDonald, D., Knights, D., Reyes, J. A., et al. (2013). Predictive functional profiling of microbial communities using 16S rRNA marker gene sequences. Nat. Biotechnol. 31, 814–821. doi: 10.1038/nbt.2676
Laukens, D., Brinkman, B. M., Raes, J., De Vos, M., and Vandenabeele, P. (2015). Heterogeneity of the gut microbiome in mice: guidelines for optimizing experimental design. FEMS Microbiol. Rev. 40, 117–132. doi: 10.1093/femsre/fuv036
Lee, T. T., Huang, Y. F., Chiang, C. C., Chung, T. K., Chiou, P. W., and Yu, B. (2011). Starch characteristics and their influences on in vitro and pig prececal starch digestion. J. Agric. Food Chem. 59, 7353–7359. doi: 10.1021/jf200402u
Ley, R. E., Backhed, F., Turnbaugh, P., Lozupone, C. A., Knight, R. D., and Gordon, J. I. (2005). Obesity alters gut microbial ecology. Proc. Natl. Acad. Sci. U.S.A. 102, 11070–11075. doi: 10.1073/pnas.0504978102
Ley, R. E., Peterson, D. A., and Gordon, J. I. (2006). Ecological and evolutionary forces shaping microbial diversity in the human intestine. Cell 124, 837–848. doi: 10.1016/j.cell.2006.02.017
Li, L., Zhang, X., Ning, Z., Mayne, J., Moore, J. I., Butcher, J., et al. (2018). Evaluating In Vitro culture medium of gut microbiome with orthogonal experimental design and a metaproteomics approach. J. Proteome Res. 17, 154–163. doi: 10.1021/acs.jproteome.7b00461
Louis, P., and Flint, H. J. (2017). Formation of propionate and butyrate by the human colonic microbiota. Environ. Microbiol. 19, 29–41. doi: 10.1111/1462-2920.13589
Love, M. I., Huber, W., and Anders, S. (2014). Moderated estimation of fold change and dispersion for RNA-seq data with DESeq2. Genome Biol. 15, 1–21. doi: 10.1186/s13059-014-0550-8
Macfarlane, G. T., Macfarlane, S., and Gibson, G. R. (1998). Validation of a three-stage compound continuous culture system for investigating the effect of retention time on the ecology and metabolism of bacteria in the human colon. Microb. Ecol. 35, 180–187. doi: 10.1007/s002489900072
Macfarlane, S., and Macfarlane, G. T. (2003). Regulation of short-chain fatty acid production. Proc. Nutr. Soc. 62, 67–72. doi: 10.1079/PNS2002207
Macy, J. M., Ljungdahl, L. G., and Gottschalk, G. (1978). Pathway of succinate and propionate formation in Bacteroides fragilis. J. Bacteriol. 134, 84–91.
Mahowald, M. A., Rey, F. E., Seedorf, H., Turnbaugh, P. J., Fulton, R. S., Wollam, A., et al. (2009). Characterizing a model human gut microbiota composed of members of its two dominant bacterial phyla. Proc. Natl. Acad. Sci. U.S.A. 106, 5859–5864. doi: 10.1073/pnas.0901529106
McDonald, J. A. K., Fuentes, S., Schroeter, K., Heikamp-deJong, I., Khursigara, C. M., de Vos, W. M., et al. (2015). Simulating distal gut mucosal and luminal communities using packed-column biofilm reactors and an in vitro chemostat model. J. Microbiol. Methods 108, 36–44. doi: 10.1016/j.mimet.2014.11.007
McDonald, J. A. K., Schroeter, K., Fuentes, S., Heikamp-deJong, I., Khursigara, C. M., de Vos, W. M., et al. (2013). Evaluation of microbial community reproducibility, stability and composition in a human distal gut chemostat model. J. Microbiol. Methods 95, 167–174. doi: 10.1016/j.mimet.2013.08.008
McMurdie, P. J., and Holmes, S. (2014). Waste not, want not: why rarefying microbiome data is inadmissible. PLoS Comput. Biol. 10:e1003531. doi: 10.1371/journal.pcbi.1003531
Michel, C., Kravtchenko, T. P., David, A., Gueneau, S., Kozlowski, F., and Cherbut, C. (1998). In vitro prebiotic effects of Acacia gums onto the human intestinal microbiota depends on both botanical origin and environmental pH. Anaerobe 4, 257–266. doi: 10.1006/anae.1998.0178
Morrison, D. J., and Preston, T. (2016). Formation of short chain fatty acids by the gut microbiota and their impact on human metabolism. Gut Microbes 7, 189–200. doi: 10.1080/19490976.2015.1134082
Nguyen, T. L. A., Vieira-Silva, S., Liston, A., and Raes, J. (2015). How informative is the mouse for human gut microbiota research? Dis. Model. Mech. 8, 1–16. doi: 10.1242/dmm.017400
Org, E., Mehrabian, M., Parks, B. W., Shipkova, P., Liu, X., Drake, T. A., et al. (2016). Sex differences and hormonal effects on gut microbiota composition in mice. Gut Microbes 7, 313–322. doi: 10.1080/19490976.2016.1203502
Payne, A. N., Zihler, A., Chassard, C., and Lacroix, C. (2012). Advances and perspectives in in vitro human gut fermentation modeling. Trends Biotechnol. 30, 17–25. doi: 10.1016/j.tibtech.2011.06.011
Pham, V. T., Lacroix, C., Braegger, C. P., and Chassard, C. (2017). Lactate-utilizing community is associated with gut microbiota dysbiosis in colicky infants. Sci. Rep. 7:11176. doi: 10.1038/s41598-017-11509-1
Poeker, S. A., Geirnaert, A., Berchtold, L., Greppi, A., Krych, L., Steinert, R. E., et al. (2018). Understanding the prebiotic potential of different dietary fibers using an in vitro continuous adult fermentation model (PolyFermS). Sci. Rep. 8, 1–12. doi: 10.1038/s41598-018-22438-y
Rajilic-Stojanovic, M., Maathuis, A., Heilig, H. G., Venema, K., de Vos, W. M., and Smidt, H. (2010). Evaluating the microbial diversity of an in vitro model of the human large intestine by phylogenetic microarray analysis. Microbiology 156, 3270–3281. doi: 10.1099/mic.0.042044-0
Rausch, P., Basic, M., Batra, A., Bischoff, S. C., Blaut, M., Clavel, T., et al. (2016). Analysis of factors contributing to variation in the C57BL/6J fecal microbiota across German animal facilities. Int. J. Med. Microbiol. 306, 343–355. doi: 10.1016/j.ijmm.2016.03.004
Roager, H. M., Hansen, L. B., Bahl, M. I., Frandsen, H. L., Carvalho, V., Gobel, R. J., et al. (2016). Colonic transit time is related to bacterial metabolism and mucosal turnover in the gut. Nat. Microbiol. 1:16093. doi: 10.1038/nmicrobiol.2016.93
Robles Alonso, V., and Guarner, F. (2013). Linking the gut microbiota to human health. Br. J. Nutr. 109(Suppl.), S21–S26. doi: 10.1017/S0007114512005235
Round, J. L., and Mazmanian, S. K. (2009). The gut microbiome shapes intestinal immune responses during health and disease. Nat. Rev. Immunol. 9, 313–323. doi: 10.1038/nri2515
Round, J. L., and Palm, N. W. (2018). Causal effects of the microbiota on immune-mediated diseases. Sci. Immunol. 3:eaao1603. doi: 10.1126/sciimmunol.aao1603
Salyer, J., Park, S. H., Ricke, S. C., and Lee, S. K. (2013). Analysis of microbial populations and metabolism of anthocyanins by mice gut microflora fed with blackberry powder. J. Nutr. Food Sci. 3:178. doi: 10.4172/2155-9600.1000178
Tahara, Y., Yamazaki, M., Sukigara, H., Motohashi, H., Sasaki, H., Miyakawa, H., et al. (2018). Gut microbiota-derived short chain fatty acids induce circadian clock entrainment in mouse peripheral tissue. Sci. Rep. 8, 1–12. doi: 10.1038/s41598-018-19836-7
Tanner, S. A., Zihler Berner, A., Rigozzi, E., Grattepanche, F., Chassard, C., Lacroix, C., et al. (2014). In Vitro continuous fermentation model (PolyFermS) of the swine proximal colon for simultaneous testing on the same gut microbiota. PLoS One 9:e94123. doi: 10.1371/journal.pone.0094123
Tao, Y., Hu, X., Zhu, X., Jin, H., Xu, Z., Tang, Q., et al. (2016). Production of butyrate from lactate by a newly isolated clostridium sp. BPY5. Appl. Biochem. Biotechnol. 179, 361–374. doi: 10.1007/s12010-016-1999-6
Tottey, W., Feria-Gervasio, D., Gaci, N., Laillet, B., Pujos, E., Martin, J.-F., et al. (2017). Colonic transit time is a driven force of the gut microbiota composition and metabolism: in vitro evidence. J. Neurogastroenterol. Motil. 23, 124–134. doi: 10.5056/jnm16042
Tropini, C., Moss, E. L., Merrill, B. D., Ng, K. M., Higginbottom, S. K., Casavant, E. P., et al. (2018). Transient osmotic perturbation causes long-term alteration to the gut microbiota. Cell 173, 1742–1754.e17. doi: 10.1016/j.cell.2018.05.008
Uhl, E. W., and Warner, N. J. (2015). Mouse models as predictors of human responses: evolutionary medicine. Curr. Pathobiol. Rep. 3, 219–223. doi: 10.1007/s40139-015-0086-y
Van den Abbeele, P., Grootaert, C., Marzorati, M., Possemiers, S., Verstraete, W., Gérard, P., et al. (2010). Microbial community development in a dynamic gut model is reproducible, colon region specific, and selective for Bacteroidetes and Clostridium cluster IX. Appl. Environ. Microbiol. 76, 5237–5246. doi: 10.1128/AEM.00759-10
Van Der Meulen, R., Adriany, T., Verbrugghe, K., and De Vuyst, L. (2006). Kinetic analysis of bifidobacterial metabolism reveals a minor role for succinic acid in the regeneration of NAD+ through its growth-associated production. Appl. Environ. Microbiol. 72, 5204–5210. doi: 10.1128/AEM.00146-06
van Zanten, G. C., Krych, L., Roytio, H., Forssten, S., Lahtinen, S. J., Abu Al-Soud, W., et al. (2014). Synbiotic Lactobacillus acidophilus NCFM and cellobiose does not affect human gut bacterial diversity but increases abundance of lactobacilli, bifidobacteria and branched-chain fatty acids: a randomized, double-blinded cross-over trial. FEMS Microbiol. Ecol. 90, 225–236. doi: 10.1111/1574-6941.12397
Walker, A. W., Duncan, S. H., McWilliam Leitch, E. C., Child, M. W., and Flint, H. J. (2005). pH and peptide supply can radically alter bacterial populations and short-chain fatty acid ratios within microbial communities from the human colon. Appl. Envinron. Microbiol. 71, 3692–3700. doi: 10.1128/AEM.71.7.3692-3700.2005
Werner, J. J., Koren, O., Hugenholtz, P., DeSantis, T. Z., Walters, W. A., Caporaso, J. G., et al. (2012). Impact of training sets on classification of high-throughput bacterial 16s rRNA gene surveys. ISME J. 6, 94–103. doi: 10.1038/ismej.2011.82
Xiao, L., Feng, Q., Liang, S., Sonne, S. B., Xia, Z., Qiu, X., et al. (2015). A catalog of the mouse gut metagenome. Nat. Biotechnol. 33, 1103–1108. doi: 10.1038/nbt.3353
Zarrinpar, A., Chaix, A., Yooseph, S., and Panda, S. (2014). Diet and feeding pattern affect the diurnal dynamics of the gut microbiome. Cell Metab. 20, 1006–1017. doi: 10.1016/j.cmet.2014.11.008
Keywords: microbiome, C57BL/6, mouse caecum, cultivation, in vitro model
Citation: Poeker SA, Lacroix C, de Wouters T, Spalinger MR, Scharl M and Geirnaert A (2019) Stepwise Development of an in vitro Continuous Fermentation Model for the Murine Caecal Microbiota. Front. Microbiol. 10:1166. doi: 10.3389/fmicb.2019.01166
Received: 15 January 2019; Accepted: 07 May 2019;
Published: 29 May 2019.
Edited by:
David William Waite, The University of Auckland, New ZealandReviewed by:
Fátima C. Pereira, University of Vienna, AustriaSabrina Duranti, University of Parma, Italy
Copyright © 2019 Poeker, Lacroix, de Wouters, Spalinger, Scharl and Geirnaert. This is an open-access article distributed under the terms of the Creative Commons Attribution License (CC BY). The use, distribution or reproduction in other forums is permitted, provided the original author(s) and the copyright owner(s) are credited and that the original publication in this journal is cited, in accordance with accepted academic practice. No use, distribution or reproduction is permitted which does not comply with these terms.
*Correspondence: Annelies Geirnaert, annelies.geirnaert@hest.ethz.ch