- 1Key Laboratory of Environmental Biotechnology, Research Center for Eco-Environmental Sciences, Chinese Academy of Sciences, Beijing, China
- 2College of Resources and Environment, University of Chinese Academy of Sciences, Beijing, China
- 3School of Safety and Environmental Engineering, Capital University of Economics and Business, Beijing, China
Water system degradation has a severe impact on daily life, especially in developing countries. However, microbial changes associated with this degradation, especially changes in microbes related to sulfur (S) cycling, are poorly understood. In this study, the abundance, structure, and diversity of sulfate-reducing microorganisms (SRM) and sulfur-oxidizing microorganisms (SOM) in the sediments from the Ziya River Basin, which is polluted by various human interventions (urban and agricultural activities), were investigated. Quantitative real-time PCR showed that the S cycling-related (SCR) genes (dsrB and soxB) were significantly elevated, reaching 2.60 × 107 and 1.81 × 108 copies per gram of dry sediment, respectively, in the region polluted by human urban activities (RU), and the ratio of dsrB to soxB abundance was significantly elevated in the region polluted by human agricultural activities (RA) compared with those in the protected wildlife reserve (RP), indicating that the mechanisms underlying water system degradation differ between RU and RA. Based on a 16S rRNA gene analysis, human interventions had substantial effects on microbial communities, particularly for microbes involved in S cycling. Some SCR genera (i.e., Desulfatiglans and Geothermobacter) were enriched in the sediments from both RA and RU, while others (i.e., Desulfofustis and Desulfonatronobacter) were only enriched in the sediments from RA. A redundancy analysis indicated that NH4+-N and total organic carbon significantly influenced the abundance of SRM and SOM, and sulfate significantly influenced only the abundance of SRM. A network analysis showed high correlation between SCR microorganisms and other microbial groups for both RU and RA, including those involved in carbon and metal cycling. These findings indicated the different effects of different human interventions on the microbial community composition and water quality degradation.
Introduction
Odorous black water is a crisis in lakes, rivers, or seashores, particularly during the summer (Feng et al., 2014). Hydrogen sulfide together with other reduced sulfur (S) compounds are the main sources of malodor in severely degraded waters (Holmer and Storkholm, 2001; Zhang et al., 2010; Watson and Juttner, 2017). In these conditions, water is highly hypoxic, leading to the death of fish and other aquatic organisms and threatening drinking water safety. During the mid-1990s and early 2000s, the phenomenon was reported in many locations, including Lake Kasumigaura in Japan, Lower Mystic Lake in the United States, Taihu Lake in China, and Subalpine Lake Garda in Italy (Zhang et al., 2010; Feng et al., 2014; Watson and Juttner, 2017). Water system degradation has a severe impact on the daily life of residents along the aquatic basin and provides an appropriate environment for pathogen growth (Feng et al., 2014; Li et al., 2014; Watson and Juttner, 2017). Thus, odorous black water induced by human urban or agricultural activities is a serious crisis, especially in developing countries where aquatic systems are more seriously and easily polluted (Kivaisi, 2001; Li et al., 2014). The phenomenon is closely related to high levels of organic matter, ammonium, and other oxygen-consuming pollutants (Feng et al., 2014; Watson and Juttner, 2017; Hu et al., 2018). However, few studies have examined microbial changes leading to the phenomenon, especially changes in microbes involved in S cycling.
Sulfur exists in a variety of reduced and oxidized compounds in inland aquatic systems (Jorgensen, 1982; Edwardson and Hollibaugh, 2017; Bao et al., 2018). S cycling, a key microbial metabolic process, is dominated by strictly anaerobic sulfate-reducing microorganisms (SRM) and sulfur-oxidizing microorganisms (SOM) (Varon-Lopez et al., 2014; Guo et al., 2016; Meier et al., 2017). SRM is an essential group for the reduction reaction of sulfate, while SOM are involved in the oxidation of sulfide in the sediments (Varon-Lopez et al., 2014; Bao et al., 2018). S cycling is connected to the cycling of other elements, such as carbon (e.g., oxidation of organic compounds and formation of organo-sulfur compounds), nitrogen (thiodenitrification), and metal (formation of metal sulfides) cycling (Edwardson and Hollibaugh, 2017; Tian et al., 2017; Bao et al., 2018). Globally, recent research has suggested that the remineralization of up to 29% of the organic matter deposited on the seafloor is facilitated by SRM (Bowles et al., 2014). In turn, hydrogen sulfide and other reduced sulfur compounds serve as electron donors for SOM or are abiotically oxidized (Bowles et al., 2014; Edwardson and Hollibaugh, 2017). Historically, S and arsenic (As) have been studied together owing to their co-occurrence in the minerals arsenopyrite, orpiment, and realgar (Edwardson and Hollibaugh, 2017; Uhrynowski et al., 2017). For example, some soluble arsenic-sulfur oxyanions (thioarsenic compounds) play important roles in As geochemistry, especially in alkaline waters (Fisher et al., 2008; Edwardson and Hollibaugh, 2017). Moreover, sulfide oxidation by SOM in aquatic sediments can be coupled to the reduction of Fe(III) to Fe(II) (Feng et al., 2014; Hausmann et al., 2016; Watson and Juttner, 2017). Thus, studying the abundance and diversity of SRM and SOM is important for revealing the roles of these microorganisms in the biogeochemical cycling of sulfur and other elements.
Both SRM and SOM differ in abundance and diversity among environments and are substantially influenced by environmental factors, such as temperature, organic matter, sulfides, geochemical gradients, and sampling depth (Varon-Lopez et al., 2014; Robador et al., 2016; Edwardson and Hollibaugh, 2017; Meier et al., 2017; Zhang M.J. et al., 2017; Zhang Y. et al., 2017). Generally, SRM are dominated by Deltaproteobacteria and Firmicutes (Haller et al., 2011; Mueller et al., 2015; Robador et al., 2016; Zhang Y. et al., 2017), while Gammaproteobacteria and Epsilonproteobacteria are considered dominant SOM on aquatic sediment surfaces (Lenk et al., 2012; Meier et al., 2017). However, some genera (e.g., Desulfitobacterium and Desulfitibacter in Firmicutes and Pyrobaculum in Archaea) of SRM have functional genes for sulfate reduction but cannot use sulfate as a terminal electron acceptor (Mueller et al., 2015; Edwardson and Hollibaugh, 2017). Unlike most SRM, SOM do not share a common sulfur metabolism pathway, but exploit various, partially redundant enzyme systems for the oxidation of a range of reduced sulfur compounds with intermediate oxidation states (Friedrich et al., 2005; Mueller et al., 2015). To explore the abundance and diversity of SRM and SOM in the environment, the dsrB and soxB genes, encoding the DsrB and SoxB subunit of Dsr and Sox enzyme systems in SRM and SOM, respectively, are widely used (Lenk et al., 2012; Varon-Lopez et al., 2014; Guo et al., 2016; Edwardson and Hollibaugh, 2017; Tian et al., 2017).
Various molecular methods have been used to characterize the community diversity and structure of SRM and SOM based on functional gene markers (Varon-Lopez et al., 2014; Angermeyer et al., 2016; Tian et al., 2017; Zhang Y. et al., 2017). However, traditional molecular methods are limited for characterizing diverse SRM and SOM communities for the following reasons: (i) incomplete databases of functional genes; (ii) low-throughput analysis and lack of complete cover the complex microbial community; and (iii) low amplification efficiency (Varon-Lopez et al., 2014; Angermeyer et al., 2016; Zhang Y. et al., 2017). High-throughput sequencing constitutes a powerful approach for achieving complete coverage of microbial communities (Zhou et al., 2011; Zhang Y. et al., 2017). The community structure and diversity of SRM and SOM have been effectively evaluated by the high-throughput sequencing of 16S rRNA (Haller et al., 2011; Hausmann et al., 2016; Robador et al., 2016; Edwardson and Hollibaugh, 2017; Meier et al., 2017; Tian et al., 2017). However, the community structure and diversity of SRM and SOM in odorous river sediments are unclear.
The Hai River Basin (HRB) in northern China is an important center of politics, economy, and culture (Li et al., 2014). There are eight sub-river basins in the HRB, of which the Ziya River Basin (ZRB) is the most odorous black one (Ding et al., 2016). Combined with a water shortage due to local anthropogenic activities, the ZRB has become one of the most polluted basins in China, with high levels of organic matter, NH4+-N, and sulfate, which will affect the groundwater, farmlands, lakes, and coastal areas (Li et al., 2014; Ding et al., 2016; Hu et al., 2018). Odorous rivers contain high levels of nutrients and frequently experience anoxic conditions in which sulfur transformation is a highly active cycling process (Weber et al., 2006; He et al., 2017; Sela-Adler et al., 2017; Hu et al., 2018). We hypothesized that shifts in S cycling-related microorganisms (SCM) communities are related to different levels of pollutants (Lenk et al., 2012; Feng et al., 2014; Varon-Lopez et al., 2014; Hausmann et al., 2016). Based on this hypothesis, river regions with distinct interventions might show distinct microbial community compositions. In this study, we analyzed the abundance, structure, and diversity of SRM and SOM in the sediments from ZRB polluted by different types of human intervention (urban and agricultural activities) using a high-throughput sequencing approach. Additionally, using a single copy of each SRM or SOM cell (Mueller et al., 2015), key marker genes (dsrB and soxB) were applied to quantify the abundance of SRM and SOM in different polluted regions. The results of this study provide a basis for how different human interventions influence the microbial community compositions.
Materials and Methods
Study Site and Sampling
Sediment samples were collected in April 2016 from an odorous river basin (ZRB) in Hebei province, China. The river could be divided into three regions, an upstream region belonging to a protected wildlife reserve with little nutrients pollution (designated RP), a midstream region localized near the city center and polluted by urban waste and sludge with high concentrations of organic matter and nitrogen pollutants (RU), and a downstream region polluted by runoff from farmlands with large amounts of sulfate fertilizer application (RA) (Supplementary Figure S1 and Supplementary Table S1) (Li et al., 2014; Ding et al., 2015). A total of 27 samples were collected from three regions (RP, RU and RA) and each region had three sampling areas to ensure the representativeness. Three samples were collected in each sampling areas as replicates. A sample (or replicate) was constituted with three fully mixed sediment cores and each sediment core was obtained by a cylindrical sampler of 20 cm in depth and 10 cm in diameter. Each sample was entirely homogenized and frozen at -80°C until parameter testing and DNA extraction.
Sediment Parameters and DNA Extraction
Sediment samples were air-dried and pH was determined using a soil-to-distilled water ratio of 1:2. Sulfate was extracted with deionized water at a ratio of 1:5 and measured using an Ion Chromatography System with an AS14 column and an ECD50 conductivity detector (ICS-1000; Dionex, Sunnyvale, CA, United States) (Liu et al., 2014). Total S (TS) was determined using an Elemental Analyzer (Vario EL III; Elementar, Langenselbold, Germany). Ammonium and nitrate in the sediment samples were extracted with 2 M KCl and determined using a Continuous Flow Analyzer (AA3; SEAL Analytical, Norderstedt, Germany) (Liu et al., 2014). Total organic carbon was determined using a Total Organic Carbon Analyzer (Vario TOC; Elementar, Germany) (de Mora et al., 2004). Sediment parameters are shown in Supplementary Table S1. Total DNA from each sample was extracted using the MoBio Powersoil DNA Extraction Kits (MoBio, Carlsbad, CA, United States) according to the manufacturer’s protocol (Wright et al., 2008) and quantified using a Nanodrop 2000 (Thermo Scientific, Wilmington, DE, United States). The purified DNA was stored at -80°C until use.
Quantification of 16S rRNA and Functional Genes
Quantitative real-time PCR (q-PCR) is a highly sensitive method for quantitative analyses of microbial abundance (Suzuki et al., 2000; Quillet et al., 2012; Tourna et al., 2014; Varon-Lopez et al., 2014; Tian et al., 2017). In this study, abundance was determined by q-PCR using a CFX Connect Real-Time System (Bio-Rad, Hercules, CA, United States) based on 16S rRNA, dsrB, and soxB (Supplementary Table S2). The primer set BACT1369F/PROK1492R was used for 16S rRNA quantification (Suzuki et al., 2000). Primer sets DSRp2060F/DSR4R (Wagner et al., 1998; Geets et al., 2006; Varon-Lopez et al., 2014) and soxB-710F/soxB-1184R (Beller et al., 2006; Tian et al., 2017) were used for dsrB and soxB amplification, respectively. Gene abundance was quantified in a 25-μl reaction mixture containing SYBR® Premix Ex TaqTM from Takara Biotechnology (Kusatsu, Japan).
High-Throughput Sequencing
The V4–V5 regions of 16S rRNA were amplified and prepared for sequencing using the Illumina MiSeq platform with the primer set F515/R907 (Muyzer et al., 1995; Wagner et al., 1998). Purified amplicons were pooled in equimolar ratios and paired-end sequenced (2 × 300 bp) was performed using the Illumina MiSeq platform (Illumina, San Diego, CA, United States). The raw reads were deposited in the NCBI Sequence Read Archive (SRA) database (Accession Number: SRR6905915-SRR6905941). Raw fastq files were quality-filtered using Trimmomatic (Bolger et al., 2014) and merged using FLASH (Magoc and Salzberg, 2011) with the following criteria. (i) Reads were truncated at any site receiving an average quality score < 20 over a 50-bp sliding window. (ii) Sequences whose overlap being longer than 10 bp were merged according to their overlap with mismatch no more than 2 bp. (iii) Sequences for each sample were separated according to barcodes (exactly matching) and primers (allowing 2 nucleotide mismatching), and reads containing ambiguous bases were removed. Thereafter, operational taxonomic units (OTUs) were clustered with 97% similarity cutoff using UPARSE (version 7.1)1 with a novel “greedy” algorithm that performs chimera filtering and OTU clustering simultaneously. Taxon assignments for each 16S rRNA gene sequence were determined using the RDP Classifier algorithm2 against the Silva (SSU123) 16S rRNA database with a confidence threshold of 70% (Amato et al., 2013).
Statistical Analysis
Bray–Curtis distance metrics were used to analyze OTUs for each sample, and a principal coordinate analysis (PCoA) was conducted according to the distance matrix. Cladograms were drawn using the Huttenhower Galaxy web application (Huttenhower Lab, Boston, MA, United States) with the LEfSe algorithm3. Linear discriminant analysis (LDA) effect size was used to elucidate differences in microbial taxa (Segata et al., 2011). An LDA score of ≥ 2 indicated an important contribution to the model. A redundancy analysis was applied to evaluate linkages between proteobacterial SCM abundance and environmental variables (Andreote et al., 2009; Varon-Lopez et al., 2014) using Canoco (Canoco 4.5; Biometris, Wageningen, Netherlands). All three correlation network analysis were constructed using the Maslov-Sneppen procedure (Maslov and Sneppen, 2002; Wang et al., 2017) and visualized using Gephi 0.9.2.4 Origin (version 9.1) was used to create the figures. One-way analysis of variance was performed using IBM SPSS Statistics (version 22) to evaluate significant differences (P < 0.05) among groups.
Results
Quantification of 16S rRNA and Functional Genes
The SRM and SOM abundances in the three regions, as determined by 16S rRNA, dsrB, and soxB, are summarized in Figure 1. Amplification efficiencies were 0.98, 0.959, and 0.95 for 16s rRNA, dsrB, and soxB, respectively (R2 = 0.999 for all genes). The 16S rRNA gene was significantly more abundant in all RU sampling areas than in all RP areas and two RA areas (P < 0.05). There were no significant differences between RP and RA areas (Figure 1A). In all three regions, soxB was more abundant than dsrB, ranging from 1.97 × 107 to 1.81 × 108 for soxB and from 2.21 × 106 to 2.60 × 107 for dsrB (copies per gram of dry sediment). The absolute values for both genes in RU1 and RU3 were significantly greater than those for the RP and RA areas (P < 0.05). Moreover, the absolute values for dsrB in RA1 and RA3 were significantly greater than those for the RP areas, but there were no significant differences in the absolute values for soxB between RP and RA (Figure 1B).
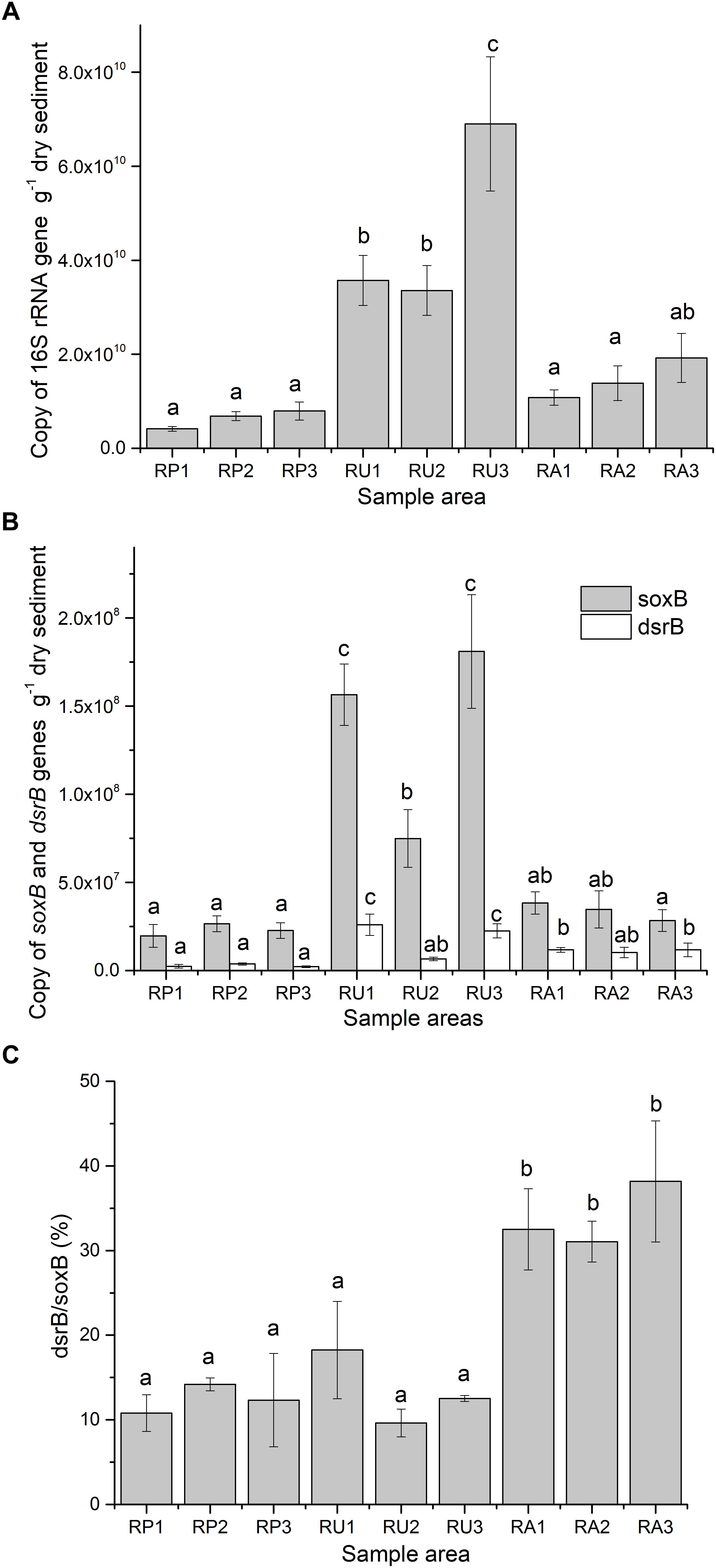
Figure 1. Abundance of 16S rRNA (A), aprA, and dsrB gene copies (B) and the ratio of dsrB to soxB (C) in odorous river sediments with different types of human intervention. Values are represented by average values from three replications with standard errors. Different letters indicate a significant difference (P < 0.05). RP, protected wildlife reserve region; RU, region polluted by human urban activity; RA, region polluted by human agricultural activity.
Clearer differences between the effects of human activities on SCR genes were observed when the abundance ratio of dsrB to soxB was calculated. While similar ratios were observed in the RP (12.44%) and RU areas (13.47%), significantly higher ratios (P < 0.05) were detected in the RA areas, with values of 32.52% for RA1, 31.07% for RA2, and 38.19% for RA3 (Figure 1C).
Differences in Microbial Diversity With Respect to Human Interventions
Total microbial diversity was determined by 16S rRNA gene sequencing targeting the V4–V5 regions using the Illumina MiSeq platform. In total, 1020911 high-quality sequences were obtained for three regions, with an average of 37812 sequences per sample (three replicates per area, three areas per region). Random re-sampling was performed with 18,000 sequences per sample, and this resampled OTU summary table was used for further statistical analysis. An analysis of OTUs at the 97% similarity level (6954 total OTUs) showed that microbial communities were highly diverse and were dominated by Proteobacteria (20.30–39.34% of sequences) and Firmicutes (8.07–49.25% of sequences) in all areas. α-Diversity indices (Table 1) showed significant differences (P < 0.01) between RP and RU and between RP and RA, including indices of OTU richness (Chao index) and community diversity (Shannon index). However, there were no significant differences between RU and RA samples (P > 0.05). PCoA of sequence data showed significant clustering according to regions, with main principal component (PC) scores of PC1 = 22.12%, PC2 = 15.79%, and PC3 = 12.29% (Supplementary Figure S2).
Using a community bar-plot analysis, we evaluated the relative abundance of microbial communities in the three regions at the phylum level (Supplementary Figure S3). The dominant phyla of the three regions were Proteobacteria, Firmicutes, Chloroflexi, Bacteroidetes, and Actinobacteria. The proportion of Firmicutes was significantly lower in RA and RU than in RP, and the proportion of Chloroflexi was significantly higher in these regions. Both RU and RA showed significant differences (P < 0.05) in microbial community structure from that of RP; ten phyla were significantly different between RU and RP and between RA and RP. Significant differences between RU and RA were only obtained for four phyla, indicating a similar microbial community structure at the phylum level. Our further analyses mainly focused on the Proteobacteria in the three regions because most well-characterized SRM and SOM belonged to this phylum (Varon-Lopez et al., 2014; Angermeyer et al., 2016; Robador et al., 2016). Moreover, the Proteobacteria were more highly represented in RU and RA than in RP and we hypothesized that the difference could be related to an increase in SRM and SOM.
Differences in Proteobacterial SCR Microbial Community Structures Among Regions
We further investigated whether different human interventions affect community structure of SRM and SOM at various taxonomic levels (Figure 2A). Sediment microbial taxa in Proteobacteria with the greatest differences among regions are displayed in Figure 2B based on LDA scores (top 50). At the Proteobacteria level, the three regions were not distinguishable. However, RU and RA could be clearly distinguished from RP at the class level; RU was characterized by an increase in Betaproteobacteria and Deltaproteobacteria, while RA was characterized by an increase in Epsilonproteobacteria. Furthermore, clearer differences among regions were detected at the order and family levels. RU was characterized by an increase in Hydrogenophilales, Rhodocyclales, Syntrophobacterales, and Desulfurellales at the order level, while RA was characterized by an increase in Desulfobacterales, Desulfuromonadales, Campylobacterales, and Desulfarculales. At the family level, Hydrogenophilaceae, Rhodocyclaceae, Desulfurellaceae, and Acidiferrobacteraceae were enriched in RU, while Desulfobacteraceae, Desulfobulbaceae, Geobacteraceae, and Helicobacteraceae were enriched in RA. The number of characterized proteobacterial microbial phylotypes in each region was calculated (Supplementary Table S3). At the order level, 23.81% of microbes belonged to SRM in RU, and the proportion increased to 36.84% in RA. At the family level, 21.88% of the microbes belonged to SRM in RU, while the proportion increased dramatically to 83.33% in RA. Moreover, the ratio of characterized SRM to SOM was 2:5 in RU at the family level and increased dramatically to 8:2 in RA at the family level.
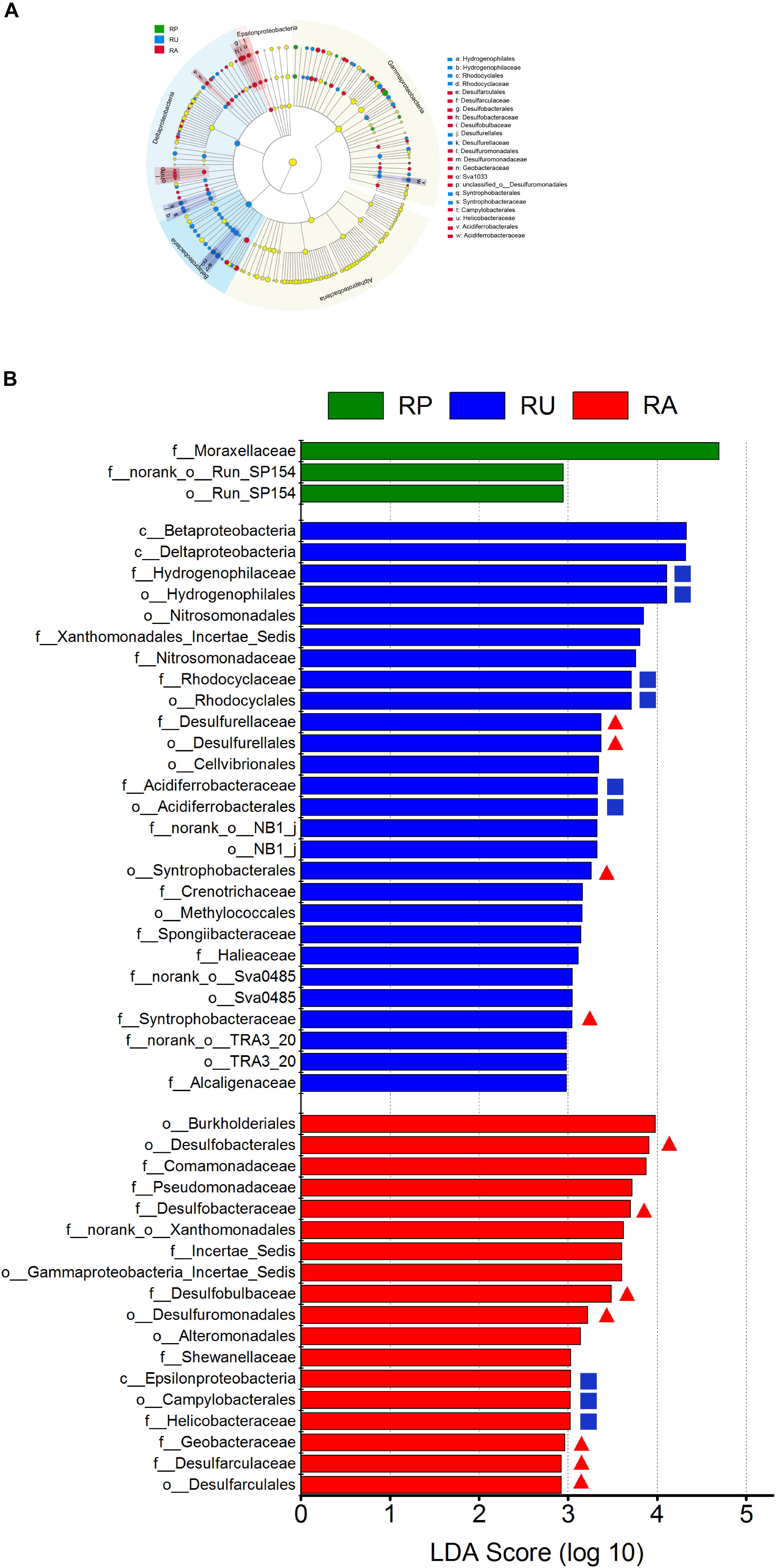
Figure 2. Taxonomic differences among polluted and non-polluted regions by a linear discriminant analysis (LDA) coupled with effect size (LEfSe). (A) Taxonomic representation of statistically and biologically consistent differences among different regions. Differences are represented by the color of the most abundant class (green: RP; blue: RU; red: RA; and yellow: insignificant). The diameter of each circle is proportional to the taxon abundance. (B) LDA scores were calculated by the LDA effect size, using the linear discriminant analysis to assess the effect size for each differential taxon (green: RP; blue: RU; red: RA; red triangle: sulfate-reducing microorganisms; blue square: sulfur-oxidizing microorganisms). The microbial taxa with high LDA scores (rank 50) are visualized. n = 9 for each region. c__: class; o__: order; f__: family.
Community Composition Changes and Enrichment of SCM
We further investigated differences in the relative abundances of various SCR genera among regions (Supplementary Figure S4). Diversity was greater in the SRM community structure than in the SOM community in all regions. RA showed a greater SRM community diversity than that of the other regions. In the SRM community, Desulfobulbus had a high relative abundance, but did not differ significantly (P < 0.05) among regions. H16 and Sva0081_sediment_group each had a significantly greater (P < 0.05) relative abundance in RU, reaching 25.21 and 15.66%, respectively, than those in the other two regions. Furthermore, as a dominant SRM genus in RP, the relative abundance of Desulfoprunum was 12.54%, and this was significantly greater than those in RU and RA, i.e., 3.73 and 1.80%, respectively. When considering the SOM community structure, Thiobacillus had a significant greater relative abundance than that of any other SOM genus in all regions, reaching 83.39% in RP, 82.69% in RU, and 76.34% in RA. Each region had characteristic SOM genera, such as Sulfuricella for RP, Sulfurifustis for RU, and Sulfurovum for RA. At the family level, SRM were dominated by Desulfobacteraceae and Desulfobulbaceae in all three regions. The proportion of Desulfurellaceae in the sediments from RU was significantly greater (P < 0.05) than those in the sediments from the other two regions. Additionally, the proportion of Desulfobacteraceae in RA was 41.03%, which was significantly greater than those in RP and RU (25.09 and 28.87%). Interestingly, Hydrogenophilaceae, a dominant SOM phylotype, maintained its overwhelming superiority over other families, i.e., greater than 75% in the sediments from all the regions. Acidiferrobacteraceae and Helicobacteraceae were the second largest phylotypes in the sediments from RU and RA regions, respectively.
To further validate differences in functional populations involved in S cycling associated with human intervention, a heat map of major genera of SRM and SOM was generated (Figure 3). Clearly, most of the SRM and SOM genera were enriched in RU and RA when compared with RP. Some SCR phylotypes, such as Desulfatiglans, Geothermobacter, and unclassified_f_Desulfobacteraceae, were enriched in the sediments from both RA and RU, while others, such as Desulfofustis, Desulfonatronobacter, and Thiocapsa, were only enriched in the sediments from RA. As a dominant SOM genus, Thiobacillus had the highest abundance in all the regions compared with other SCR genera. The environmental parameters NH4+-N, TOC, and sulfate were significantly highly correlated with the phylotype abundance of proteobacterial SRM, while only NH4+-N and TOC were significantly correlated with the phylotype abundance of proteobacterial SOM based on a redundancy analysis (Table 2).
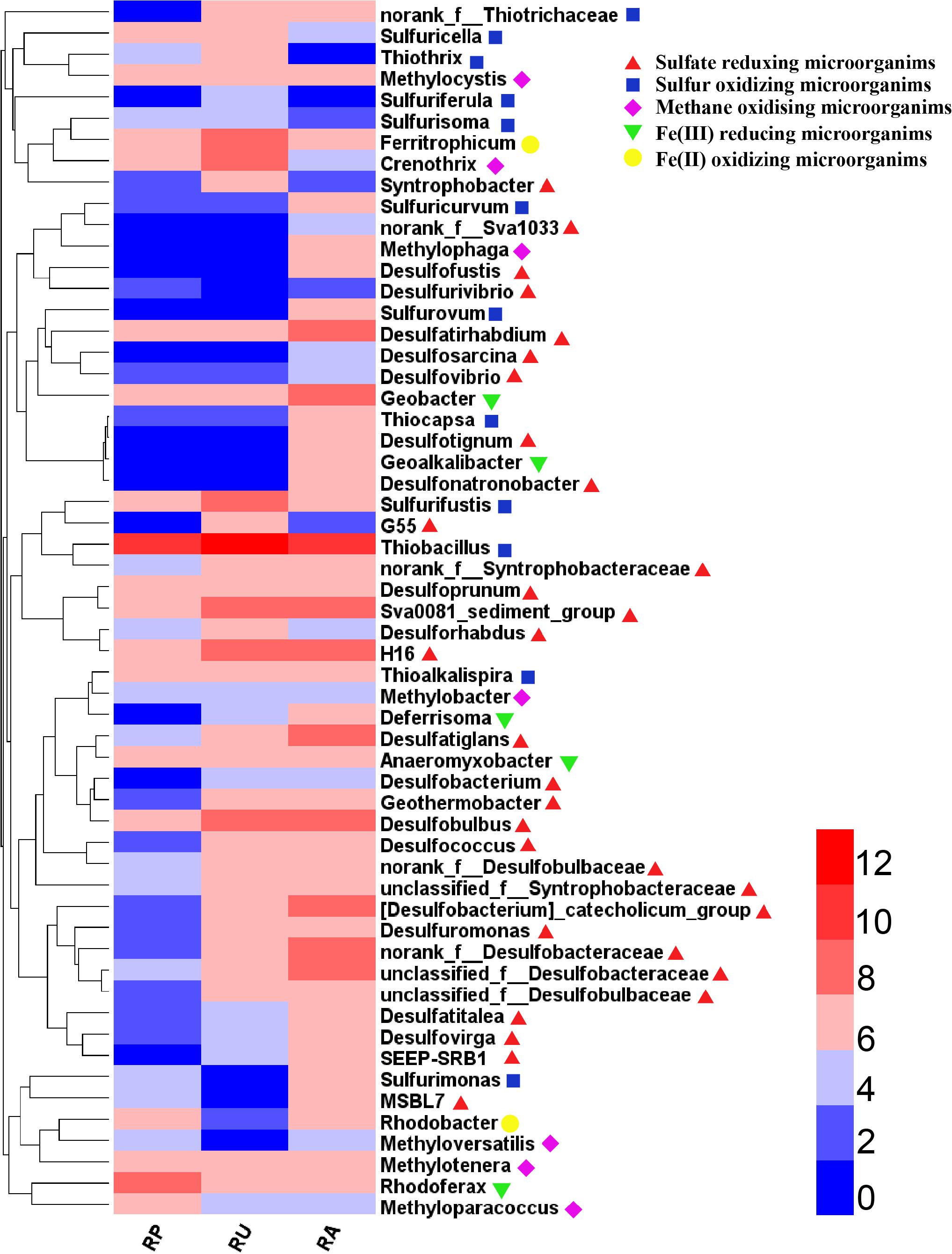
Figure 3. Heatmap of genera in odorous river sediments with different types of human intervention. Colored bars indicate the abundance normalized on a log2 scale, based on the color key at the top right.
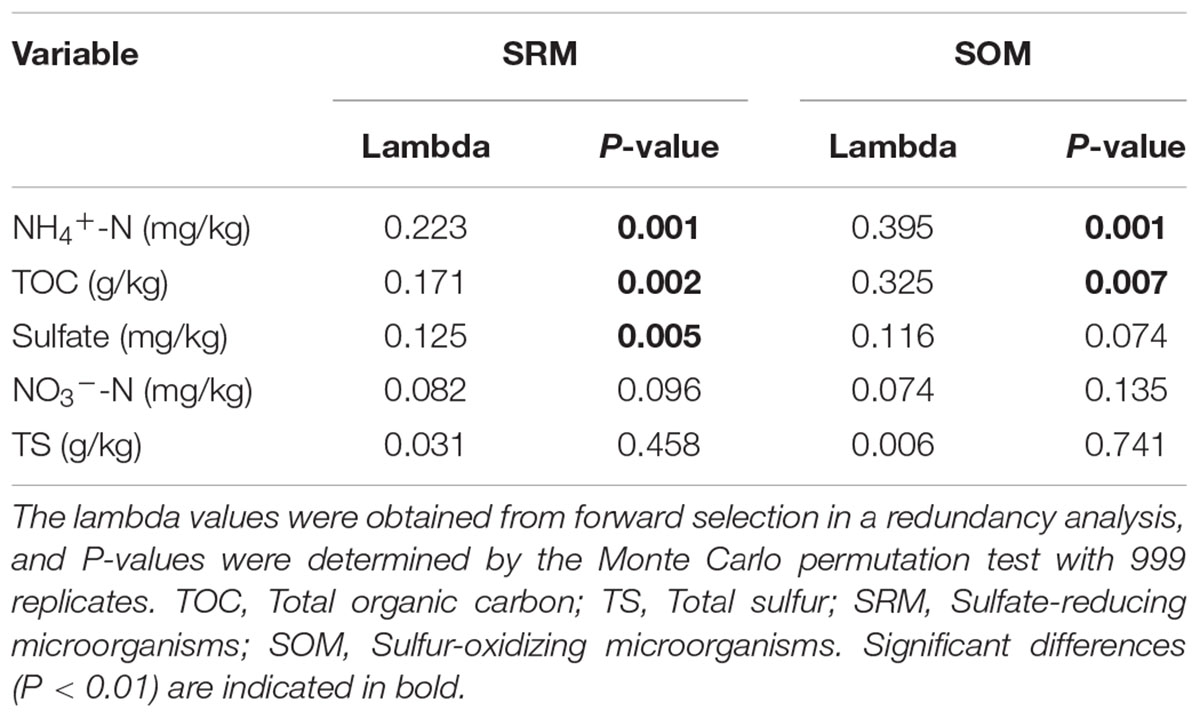
Table 2. Significance of environmental variables with respect to the abundance of proteobacterial sulfate-reducing and sulfur-oxidizing microorganisms based on 16S rRNA sequences.
Highly Complex Microbial Interactions in Polluted Regions
Three correlation networks based on the relative abundance matrix of genus-level OTUs with 97% similarity were computed for the RP, RU, and RA samples. Different microbial groups involved in S, C, and Fe cycling and their interaction networks were selected for further analysis (Figure 4). Overall, the 16S rRNA gene-based networks consisted of 32 (with 31 nodes), 140 (with 65 nodes), and 139 (with 64 nodes) pairs of significant (P < 0.01) and robust (q > 0.9 or q < -0.9) correlations in RP, RU, and RA sediments, respectively. Modularity indices were 0.725 for RP, 0.544 for RU, and 0.496 for RA correlation networks, suggesting that all of the networks had modular structures (Liu and Conrad, 2017; Sun et al., 2018). The network structures for the three regions differed substantially in terms of composition, interaction patterns (positive, zero, or negative), and node overlap, indicating that different human interventions greatly altered the interactions among microbial phylotypes. A small and simple connected module of nodes corresponded to S cycling members in the sediments from RP, primarily including Desulfitobacterium, Desulfobulbus, and Thiobacillus, and these species show minimal interactions. However, in the sediments from RU and RA, large nodes directly or indirectly corresponded to members involved in S cycling, primarily including Fe(III)-reducing microorganisms (i.e., Geobacter, Deferrisoma, and Geoalkalibacter) and methane-oxidizing microorganisms (i.e., Methyloparacoccus, Methylocystis, and Methylotenera), indicating that S cycling is connected to C and metal cycling (Mueller et al., 2015; Hausmann et al., 2016; Edwardson and Hollibaugh, 2017; Liu and Conrad, 2017; Sun et al., 2018).
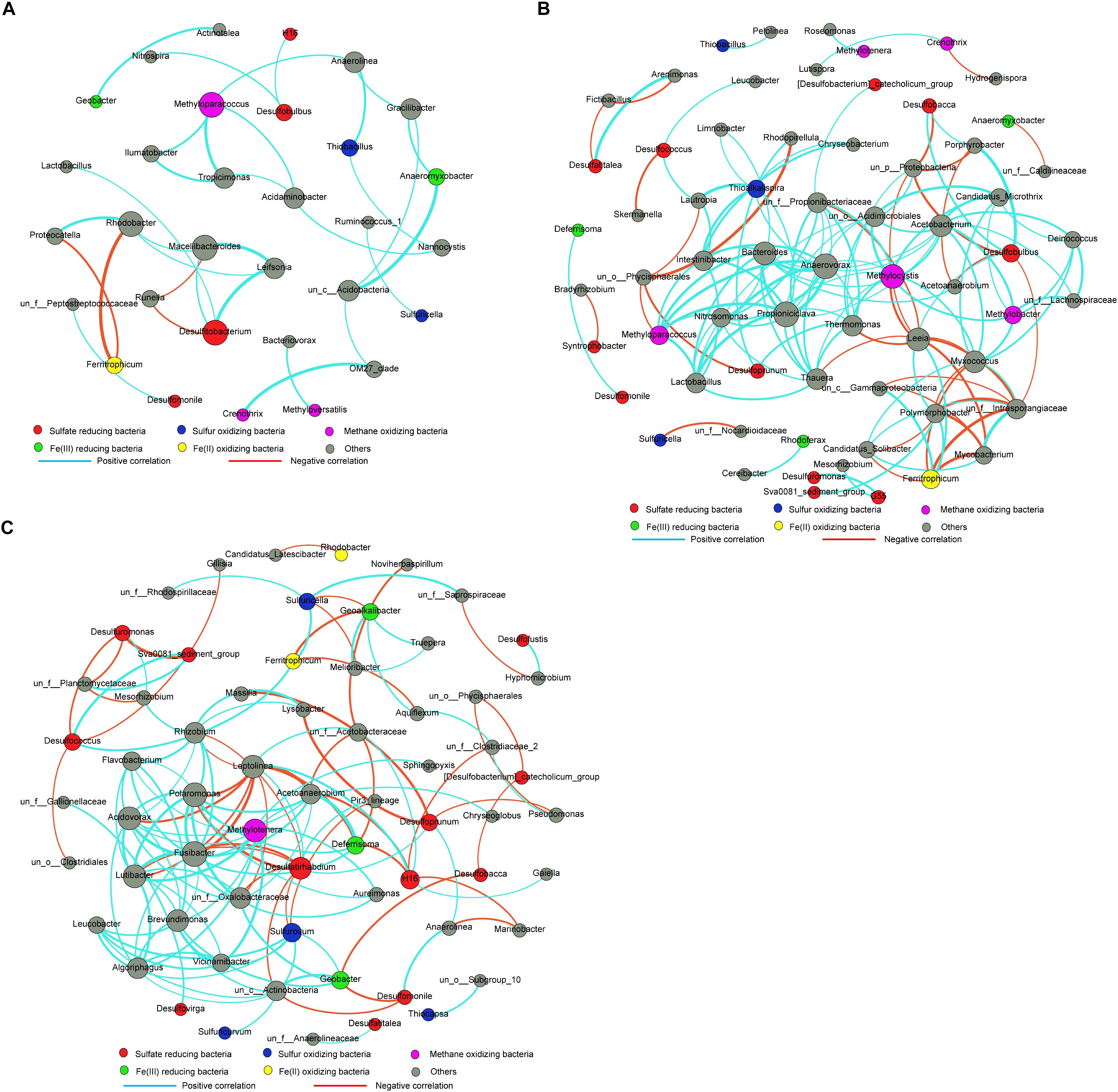
Figure 4. Bacterial 16S rRNA genera-based correlation network for the sediments from RP (A), RU (B), and RA (C). A node represents a genus. A connection stands for a strong (Spearman’s q > 0.9 or q < –0.9) and significant (P < 0.01) correlation. Edge widths were scaled according to their weights and edge colors indicated a positive or negative correlation for the nodes they connect. The size of each node is proportional to the number of connections (the degree). n = 9 per group. un, unclassified.
Discussion
Previous studies have demonstrated relationships between SCM and environmental factors (Ramos-Padron et al., 2011; Varon-Lopez et al., 2014; Xia et al., 2014; Angermeyer et al., 2016; Pelikan et al., 2016). However, the roles of SRM and SOM in odorous river sediments resulting from various human activities are unclear. In this study, we compared the patterns of the 16S rRNA, dsrB, and soxB genes in river sediments to reveal the effects of distinct human activities on the abundance and diversity of SCM.
In the sediments of the odorous river, we found that microorganisms were significantly more abundant in all of the RU areas than in the RP and RA areas, except for RA3. The abundance of 16S rRNA was significantly (P < 0.001) correlated with TOC, NH4+-N, and NO3--N (0.752, 0.827, and 0.820) in the sediment samples. Previous studies have found a similar relationship between microbial abundance and high nutriment concentrations (Varon-Lopez et al., 2014; Xia et al., 2014). The abundance of the soxB gene was always greater than the abundance of the dsrB gene in all sediment samples. Quillet observed 6.6 × 109 and 4.6 × 108 dsrB per gram of dry sediments from a salt marsh in the Medway Estuary (UK) and Tourna found 9.4 × 108 soxB per gram of dry sediments from livestock-grazed pastures in New Zealand (Quillet et al., 2012; Tourna et al., 2014). However, in this study, the average values for dsrB and soxB were only 1.06 × 107 and 6.47 × 107 copies, respectively, per gram of dry sediment. This difference could be caused by variation in the depth of sediment sampled together with the availability of organic matter and the sulfate concentration (Quillet et al., 2012; Varon-Lopez et al., 2014). Various functional genes increased significantly in the areas influenced by human urban activities. Similar results were obtained in previous studies, where SRM and SOM increased significantly when nutrients were added to the sediments (Taketani et al., 2010; Xia et al., 2014; Hausmann et al., 2016). This response could be related to a decrease in oxygen and an increase in pollutants, possibly limiting the growth of aerobes (Varon-Lopez et al., 2014; Pelikan et al., 2016). However, with respect to the abundance ratio of dsrB to soxB, a significant difference was only detected in the areas influenced by human agricultural activities, while similar ratios were obtained for areas influenced by human urban activities and protected areas. A Spearman’s correlation analysis (Supplementary Table S4) indicated that the ratio was significantly correlated (P < 0.001) with sulfate (Spearman correlation coefficient, 0.628), indicating that sulfate was one of the main factors determining the balance between SRM and SOM communities (Varon-Lopez et al., 2014; Xia et al., 2014; Hausmann et al., 2016). These results indicated that the mechanisms underlying water system degradation differ between RU and RA.
High-throughput sequencing data showed that different human activities significantly influenced the microbial composition in odorous river sediments, with a high relative abundance of Proteobacteria and Firmicutes (Lenk et al., 2012; Varon-Lopez et al., 2014; Guo et al., 2016). As the dominant phylum, Proteobacteria always exhibits a high relative abundance across all sampling areas, accounting for 20.3–39.34% of taxa. The relative abundance of Proteobacteria was elevated in both of the polluted regions, and this could be explained by the increases in SRM and SOM, as they typically belong to Proteobacteria (Mueller et al., 2015; Meier et al., 2017; Zhang Y. et al., 2017). The increase in relative abundance in RU and RA was further quantified by a LEfSe analysis (Figure 2A). Deltaproteobacteria and Betaproteobacteria, the dominant SRM and SOM classes (Friedrich et al., 2005; Lenk et al., 2012; Mueller et al., 2015; Zhang Y. et al., 2017), were also detected in RU areas. However, not only was Alphaproteobacteria undetected in these regions, but no SCR orders or families in Alphaproteobacteria were detected in any of the regions, different from the results of previous studies (Lenk et al., 2012; Mueller et al., 2015; Hausmann et al., 2016; Robador et al., 2016; Tian et al., 2017; Zhang Y. et al., 2017). As a dominant SOM class in previous studies (Angermeyer et al., 2016; Robador et al., 2016; Zhang Y. et al., 2017), Epsilonproteobacteria was frequent in RA. Moreover, most of the SCR microbial taxa were detected in polluted regions (RU or RA), but not in RP (Figure 2B). Sulfate could stimulate the rate of sulfate reduction and the growth of SRM, especially for SRM with a relative low abundance (Hausmann et al., 2016). At the family level, we also observed eight SRM phylotypes in RA, but only two phylotypes in RU (Supplementary Table S3), which may be due to the high levels of sulfate (Strickman et al., 2016). Interestingly, microorganisms belonging to Deferribacteres were also detected in RU (data not shown). These microorganisms can reduce Fe(III) to Fe(II), which could be converted to FeS, thereby resulting in black water (Feng et al., 2014; Edwardson and Hollibaugh, 2017; Watson and Juttner, 2017). These results indicated an interaction between SCM and Fe(III)-reducing microorganisms.
SRM in all the sediment samples were dominated by Desulfobacteraceae and Desulfobulbaceae in Deltaproteobacteria (Mueller et al., 2015; Robador et al., 2016; Zhang Y. et al., 2017) (Supplementary Figure S4C). SOM mainly included members of Hydrogenophilaceae, which belongs to Epsilonproteobacteria (Lenk et al., 2012; Meier et al., 2017; Zhang Y. et al., 2017) (Supplementary Figure S4D). Meanwhile, some studies also indicated that Epsilonproteobacteria is a non-negligible phylotype of SOM in aquatic environment (Yakimov et al., 2007; Wang et al., 2012; Zhang Y. et al., 2017), which is consistent with our results. At the genus level, two groups were clearly observed; group I contained the SCR genera enriched only in the sediments from RA, and group II contained the SCR genera enriched in the sediments from both RU and RA. A high sulfate level in RA seemed to stimulate growth in many genera, especially various low abundance genera of SRM (e.g., Desulfonatronobacter, Desulfofustis, and Desulfatirhabdium) and SOM (e.g., Sulfurovum and Thiocapsa) as determined by a Spearman’s correlation analysis (P < 0.01) (Hausmann et al., 2016; Strickman et al., 2016). In fact, 21 genera of SRM were significantly correlated (P < 0.05) with the sulfate level, accounting for 63.64% of all SRM genera. In the SRM reducing process, sulfate is reduced to hydrogen sulfide, the dissolved metal ions are precipitated as metal sulfides, and the concentration of metal ions in solution decreases. At the same time, hydrogen ions are consumed and the pH increases (Lee et al., 2014;Zhang M.J. et al., 2017). The increased pH might lead to the excess combination of Fe2+ and S2- to FeS that is one of main substances leading to black water (Weber et al., 2006; Feng et al., 2014).
We further explored differences in microbial interactions associated with different types of human intervention. More complex correlation networks were observed for RU and RA than for RP. Additionally, much more complex correlations between SCM (i.e., Desulfuromonas, Desulfatirhabdium, and H16 for SRM; Sulfuricella and Sulfurovum for SOM) and other microbial groups could also be observed for RU and RA, but not RP. SRM utilize propionate and straight-chain fatty acids for sulfate reduction, although many phylotypes are incomplete oxidizers (Ghattas et al., 2017; Liu and Conrad, 2017; Sun et al., 2018). Desulfobulbus is important for sulfate reduction in freshwater and marine sediments (Laanbroek and Pfennig, 1981; Taylor and Parkes, 1983; Laanbroek et al., 1984). In the RU region, Desulfobulbus had more complex correlations with other microbial groups (i.e., Desulfobacca, Porphyrobacter, and Acetoanaerobium) than those for RP and RA. A significant enrichment of Desulfobulbus was also observed in the RU areas (Figure 3). Methane-oxidizing microorganisms (i.e., Methylocystis, Methyloparacoccus, and Methylotenera) also had complex correlations with other microbial groups for RU and RA. The correlation networks were highly complex for RU and RA for microbial phylotypes involved in S and C cycling (Asakura et al., 2014; Liu and Conrad, 2017; Sun et al., 2018). It is notable that Geobacter, which was significantly enriched in RA, was the most important Fe(III)-reducing genus identified at the current study site (Haller et al., 2011; Sun et al., 2018). Previous studies have indicated that Geobacter is highly competitive in rice paddies, using ferrihydrite as an electron acceptor, and Anaeromyxobacter spp. use goethite as an electron acceptor (Hori et al., 2010). In the RA region, more highly complex correlations between Geobacter and SCM (i.e., Desulfomonile, Desulfobacca, and Sulfurovum) were observed, particularly positive correlations with SOM and negative correlations with SRM. Fe(III) reduction in aquatic sediments may produce a large quantity of FeS, resulting in black water (Weber et al., 2006). Collectively, these data indicate that dynamic Fe and S cycling occurs in river sediments and may be an indicator of black water.
Conclusion
In this study, the effects of various human interventions (urban and agricultural activity) on the abundance and diversity of SRM and SOM communities were characterized in an odorous black river system. The abundance of SCR microorganisms and the abundance ratio of dsrB to soxB were particularly high in RU and RA, respectively, indicating that RU and RA affect the odorous river system via different mechanisms. High-throughput sequencing of 16S rRNA indicated that different polluted regions were characterized by different types of SCR microorganisms. In a network analysis, the correlations between SCR microorganisms and many other microbial groups were high for RU and RA, including microbial groups involved in C and Fe cycling. Our results showed the difference of microbial community composition influenced by different human interventions. It also indicated that sulfur cycling might play a crucial role in water quality degradation and exhibited different mechanisms for water quality degradation in different polluted areas.
Author Contributions
RW, SX, and XZ designed the research and wrote the paper. RW, CJ, YZ, and NB performed the research. RW, CJ, YZ, GZ, and ZB analyzed the data.
Funding
This work was supported by the National Natural Science Foundation of China (Nos. 31670507 and 31700429), the Interdisciplinary Innovation Team Program of the Chinese Academy of Sciences (No. 2015, Team of Directional Biotransformation of Environmental Pollutants), and the Major Science and Technology Program for Water Pollution Control and Treatment of China (Nos. 2015ZX07206-006 and 2014ZX07204-005).
Conflict of Interest Statement
The authors declare that the research was conducted in the absence of any commercial or financial relationships that could be construed as a potential conflict of interest.
Supplementary Material
The Supplementary Material for this article can be found online at: https://www.frontiersin.org/articles/10.3389/fmicb.2019.00231/full#supplementary-material
Footnotes
- ^ http://drive5.com/uparse/
- ^ http://rdp.cme.msu.edu/
- ^ http://huttenhower.sph.harvard.edu/galaxy
- ^ https://gephi.org
References
Amato, K. R., Yeoman, C. J., Kent, A., Righini, N., Carbonero, F., Estrada, A., et al. (2013). Habitat degradation impacts black howler monkey Alouatta pigr gastrointestinal microbiomes. ISME J. 7, 1344–1353. doi: 10.1038/ismej.2013.16
Andreote, F. D., Azevedo, J. L., and Araujo, W. L. (2009). Assessing the diversity of bacterial communities assosiated with plants. Braz. J. Microbiol. 40, 417–432. doi: 10.1590/s1517-83822009000300001
Angermeyer, A., Crosby, S. C., and Huber, J. A. (2016). Decoupled distance-decay patterns between dsrA and 16S rRNA genes among salt marsh sulfate-reducing bacteria. Environ. Microbiol. 18, 75–86. doi: 10.1111/1462-2920.12821
Asakura, T., Date, Y., and Kikuchi, J. (2014). Comparative analysis of chemical and microbial profiles in estuarine sediments sampled from Kanto and Tohoku regions in Japan. Anal. Chem. 86, 5425–5432. doi: 10.1021/ac5005037
Bao, P., Li, G. X., Sun, G. X., Xu, Y. Y., Meharg, A. A., and Zhu, Y. G. (2018). The role of sulfate-reducing prokaryotes in the coupling of element biogeochemical cycling. Sci. Total Environ. 613, 398–408. doi: 10.1016/j.scitotenv.2017.09.062
Beller, H. R., Chain, P. S. G., Letain, T. E., Chakicherla, A., Larimer, F. W., Richardson, P. M., et al. (2006). The genome sequence of the obligately chemolithoautotrophic, facultatively anaerobic bacterium Thiobacillus denitfificans. J. Bacteriol. 188, 1473–1488. doi: 10.1128/jb.188.4.1473-1488.2006
Bolger, A. M., Lohse, M., and Usadel, B. (2014). Trimmomatic: a flexible trimmer for illumina sequence data. Bioinformatics 30, 2114–2120. doi: 10.1093/bioinformatics/btu170
Bowles, M. W., Mogollon, J. M., Kasten, S., Zabel, M., and Hinrichs, K.-U. (2014). Global rates of marine sulfate reduction and implications for sub-sea-floor metabolic activities. Science 344, 889–891. doi: 10.1126/science.1249213
de Mora, S., Sheikholeslami, M. R., Wyse, E., Azemard, S., and Cassi, R. (2004). An assessment of metal contamination in coastal sediments of the caspian Sea. Mar. Pollut. Bull. 48, 61–77. doi: 10.1016/s0025-326x(03)00285-6
Ding, Y., Rong, N., and Shan, B. (2016). Impact of extreme oxygen consumption by pollutants on macroinvertebrate assemblages in plain rivers of the ziya river basin, north China. Environ. Sci. Pollut. Res. 23, 14147–14156. doi: 10.1007/s11356-016-6404-z
Ding, Y., Shan, B., and Zhao, Y. (2015). Assessment of river habitat quality in the hai river basin, northern China. Int. J. Environ. Res. Public Health 12, 11699–11717. doi: 10.3390/ijerph120911699
Edwardson, C. F., and Hollibaugh, J. T. (2017). Metatranscriptomic analysis of prokaryotic communities active in sulfur and arsenic cycling in mono lake, california, USA. ISME J. 11, 2195–2208. doi: 10.1038/ismej.2017.80
Feng, Z., Fan, C., Huang, W., and Ding, S. (2014). Microorganisms and typical organic matter responsible for lacustrine “black bloom”. Sci. Total Environ. 470, 1–8. doi: 10.1016/j.scitotenv.2013.09.022
Fisher, J. C., Wallschlager, D., Planer-Friedrich, B., and Hollibaugh, J. T. (2008). A new role for sulfur in arsenic cycling. Environ. Sci. Technol. 42, 81–85. doi: 10.1021/es0713936
Friedrich, C. G., Bardischewsky, F., Rother, D., Quentmeier, A., and Fischer, J. (2005). Prokaryotic sulfur oxidation. Curr. Opin. Microbiol. 8, 253–259. doi: 10.1016/j.mib.2005.04.005
Geets, J., Borrernans, B., Diels, L., Springael, D., Vangronsveld, J., van der Lelie, D., et al. (2006). DsrB gene-based DGGE for community and diversity surveys of sulfate-reducing bacteria. J. Microbiol. Methods 66, 194–205. doi: 10.1016/j.mimet.2005.11.002
Ghattas, A. K., Fischer, F., Wick, A., and Ternes, T. A. (2017). Anaerobic biodegradation of (emerging) organic contaminants in the aquatic environment. Water Res. 116, 268–295. doi: 10.1016/j.watres.2017.02.001
Guo, F., Li, B., Yang, Y., Deng, Y., Qiu, J. W., Li, X. D., et al. (2016). Impacts of human activities on distribution of sulfate-reducing prokaryotes and antibiotic resistance genes in marine coastal sediments of Hong Kong. FEMS Microbiol. Ecol. 92:fiw128. doi: 10.1093/femsec/fiw128
Haller, L., Tonolla, M., Zopfi, J., Peduzzi, R., Wildi, W., and Pote, J. (2011). Composition of bacterial and archaeal communities in freshwater sediments with different contamination levels (lake geneva, Switzerland). Water Res. 45, 1213–1228. doi: 10.1016/j.watres.2010.11.018
Hausmann, B., Knorr, K.-H., Schreck, K., Tringe, S. G., del Rio, T. G., Loy, A., et al. (2016). Consortia of low-abundance bacteria drive sulfate reduction-dependent degradation of fermentation products in peat soil microcosms. ISME J. 10, 2365–2375. doi: 10.1038/ismej.2016.42
He, Z. H., Long, X. X., Li, L. Y., Yu, G. W., Chong, Y. X., Xing, W., et al. (2017). Temperature response of sulfide/ferrous oxidation and microbial community in anoxic sediments treated with calcium nitrate addition. J. Environ. Manag. 191, 209–218. doi: 10.1016/jjenvman.2017.01.008
Holmer, M., and Storkholm, P. (2001). Sulphate reduction and sulphur cycling in lake sediments: a review. Freshw. Biol. 46, 431–451. doi: 10.1046/j.1365-2427.2001.00687.x
Hori, T., Muller, A., Igarashi, Y., Conrad, R., and Friedrich, M. W. (2010). Identification of iron-reducing microorganisms in anoxic rice paddy soil by 13C-acetate probing. ISME J. 4, 267–278. doi: 10.1038/ismej.2009.100
Hu, J., Dong, H. Y., Xu, Q., Ling, W. C., Qu, J. H., and Qiang, Z. M. (2018). Impacts of water quality on the corrosion of cast iron pipes for water distribution and proposed source water switch strategy. Water Res. 129, 428–435. doi: 10.1016/j.watres.2017.10.065
Jorgensen, B. B. (1982). Mineralization of organic matter in the sea bed – the role of sulfate reduction. Nature 296, 643–645. doi: 10.1038/296643a0
Kivaisi, A. K. (2001). The potential for constructed wetlands for wastewater treatment and reuse in developing countries: a review. Ecol. Eng. 16, 545–560. doi: 10.1016/s0925-8574(00)00113-0
Laanbroek, H. J., Geerligs, H. J., Sijtsma, L., and Veldkamp, H. (1984). Competition for sulfate and ethanol among Desulfobacter, Desulfobulbus, and Desulfovibrio species isolated from intertidal sediments. Appl. Environ. Microbiol. 47, 329–334.
Laanbroek, H. J., and Pfennig, N. (1981). Oxidation of short-chain fatty acids by sulfate-reducing bacteria in freshwater and in marine sediments. Arch. Microbiol. 128, 330–335. doi: 10.1007/bf00422540
Lee, D.-J., Liu, X., and Weng, H.-L. (2014). Sulfate and organic carbon removal by microbial fuel cell with sulfate-reducing bacteria and sulfide-oxidising bacteria anodic biofilm. Bioresour. Technol. 156, 14–19. doi: 10.1016/j.biortech.2013.12.129
Lenk, S., Moraru, C., Hahnke, S., Arnds, J., Richter, M., Kube, M., et al. (2012). Roseobacter clade bacteria are abundant in coastal sediments and encode a novel combination of sulfur oxidation genes. ISME J. 6, 2178–2187. doi: 10.1038/ismej.2012.66
Li, W., Li, X., Su, J., and Zhao, H. (2014). Sources and mass fluxes of the main contaminants in a heavily polluted and modified river of the North China Plain. Environ. Sci. Pollut. Res. Int. 21, 5678–5688. doi: 10.1007/s11356-013-2461-8
Liu, P. F., and Conrad, R. (2017). Syntrophobacteraceae-affiliated species are major propionate-degrading sulfate reducers in paddy soil. Environ. Microbiol. 19, 1669–1686. doi: 10.1111/1462-2920.13698
Liu, Y. R., Zheng, Y. M., Zhang, L. M., and He, J. Z. (2014). Linkage between community diversity of sulfate-reducing microorganisms and methylmercury concentration in paddy soil. Environ. Sci. Pollut. Res. 21, 1339–1348. doi: 10.1007/s11356-013-1973-6
Magoc, T., and Salzberg, S. L. (2011). FLASH: fast length adjustment of short reads to improve genome assemblies. Bioinformatics 27, 2957–2963. doi: 10.1093/bioinformatics/btr507
Maslov, S., and Sneppen, K. (2002). Specificity and stability in topology of protein networks. Science 296, 910–913. doi: 10.1126/science.1065103
Meier, D. V., Pjevac, P., Bach, W., Hourdez, S., Girguis, P. R., Vidoudez, C., et al. (2017). Niche partitioning of diverse sulfur-oxidizing bacteria at hydrothermal vents. ISME J. 11, 1545–1558. doi: 10.1038/ismej.2017.37
Mueller, A. L., Kjeldsen, K. U., Rattei, T., Pester, M., and Loy, A. (2015). Phylogenetic and environmental diversity of DsrAB-type dissimilatory (bi) sulfite reductases. ISME J. 9, 1152–1165. doi: 10.1038/ismej.2014.208
Muyzer, G., Teske, A., Wirsen, C. O., and Jannasch, H. W. (1995). Phylogenetic relationships of thiomicrospira species and their identification in deep-sea hydrothermal vent samples by denaturing gradient gel-electrophoresis of 16S rDNA fragments. Arch. Microbiol. 164, 165–172. doi: 10.1007/bf02529967
Pelikan, C., Herbold, C. W., Hausmann, B., Muller, A. L., Pester, M., and Loy, A. (2016). Diversity analysis of sulfite- and sulfate-reducing microorganisms by multiplex dsrA and dsrB amplicon sequencing using new primers and mock community-optimized bioinformatics. Environ. Microbiol. 18, 2994–3009. doi: 10.1111/1462-2920.13139
Quillet, L., Besaury, L., Popova, M., Paisse, S., Deloffre, J., and Ouddane, B. (2012). Abundance, diversity and activity of sulfate-reducing prokaryotes in heavy metal-contaminated sediment from a salt marsh in the medway estuary (UK). Mar. Biotechnol. 14, 363–381. doi: 10.1007/s10126-011-9420-5
Ramos-Padron, E., Bordenave, S., Lin, S. P., Bhaskar, I. M., Dong, X. L., Sensen, C. W., et al. (2011). Carbon and sulfur cycling by microbial communities in a gypsum-treated oil sands tailings pond. Environ. Sci. Technol. 45, 439–446. doi: 10.1021/es1028487
Robador, A., Mueller, A. L., Sawicka, J. E., Berry, D., Hubert, C. R. J., and Loy, A. (2016). Activity and community structures of sulfate-reducing microorganisms in polar, temperate and tropical marine sediments. ISME J. 10, 796–809. doi: 10.1038/ismej.2015.157
Segata, N., Izard, J., Waldron, L., Gevers, D., Miropolsky, L., and Garrett, W. S. (2011). Metagenomic biomarker discovery and explanation. Genome Biol. 12:R60. doi: 10.1186/gb-2011-12-6-r60
Sela-Adler, M., Ronen, Z., Herut, B., Antler, G., Vigderovich, H., and Eckert, W. (2017). Co-existence of methanogenesis and sulfate reduction with common substrates in sulfate-rich estuarine sediments. Front. Microbiol. 8:11. doi: 10.3389/fmicb.2017.00766
Strickman, R. J. S., Fulthorpe, R. R., Wasik, J. K. C., Engstrom, D. R., and Mitchell, C. P. J. (2016). Experimental sulfate amendment alters peatland bacterial community structure. Sci. Total Environ. 566, 1289–1296. doi: 10.1016/j.scitotenv.2016.05.189
Sun, W. M., Xiao, E. Z., Pu, Z. L., Krumins, V., Dong, Y. R., Li, B. Q., et al. (2018). Paddy soil microbial communities driven by environment- and microbe-microbe interactions: a case study of elevation-resolved microbial communities in a rice terrace. Sci. Total Environ. 612, 884–893. doi: 10.1016/j.scitotenv.2017.08.275
Suzuki, M. T., Taylor, L. T., and DeLong, E. F. (2000). Quantitative analysis of small-subunit rRNA genes in mixed microbial populations via 5′-nuclease assays. Appl. Environ. Microbiol. 66, 4605–4614. doi: 10.1128/aem.66.11.4605-4614.2000
Taketani, R. G., Yoshiura, C. A., Franco Dias, A. C., Andreote, F. D., and Tsai, S. M. (2010). Diversity and identification of methanogenic archaea and sulphate-reducing bacteria in sediments from a pristine tropical mangrove. Antonie Van Leeuwenhoek 97, 401–411. doi: 10.1007/s10482-010-9422-8
Taylor, J., and Parkes, R. J. (1983). The cellular fatty acids of the sulphate-reducing bacteria, Desulfobacter sp., Desulfobulbus sp. and Desulfovibrio desulfuricans. Microbiology 129, 3303–3309.
Tian, H. M., Gao, P. K., Chen, Z. H., Li, Y. S., Li, Y., Wang, Y. S., et al. (2017). Compositions and abundances of sulfate-reducing and sulfur oxidizing microorganisms in water-flooded petroleum reservoirs with different temperatures in China. Front. Microbiol. 8:14. doi: 10.3389/fmicb.2017.00143
Tourna, M., Maclean, P., Condron, L., O’Callaghan, M., and Wakelin, S. A. (2014). Links between sulphur oxidation and sulphur-oxidising bacteria abundance and diversity in soil microcosms based on soxB functional gene analysis. FEMS Microbiol. Ecol. 88, 538–549. doi: 10.1111/1574-6941.12323
Uhrynowski, W., Debiec, K., Sklodowska, A., and Drewniak, L. (2017). The role of dissimilatory arsenate reducing bacteria in the biogeochemical cycle of arsenic based on the physiological and functional analysis of Aeromonas sp O23A. Sci. Total Environ. 598, 680–689. doi: 10.1016/j.scitotenv.2017.04.137
Varon-Lopez, M., Dias, A. C. F., Fasanella, C. C., Durrer, A., Melo, I. S., Kuramae, E. E., et al. (2014). Sulphur-oxidizing and sulphate-reducing communities in Brazilian mangrove sediments. Environ. Microbiol. 16, 845–855. doi: 10.1111/1462-2920.12237
Wagner, M., Roger, A. J., Flax, J. L., Brusseau, G. A., and Stahl, D. A. (1998). Phylogeny of dissimilatory sulfite reductases supports an early origin of sulfate respiration. J. Bacteriol. 180, 2975–2982.
Wang, X. H., Zheng, Q., Yuan, Y., Hai, R. T., and Zou, D. X. (2017). Bacterial community and molecular ecological network in response to Cr2O3 nanoparticles in activated sludge system. Chemosphere 188, 10–17. doi: 10.1016/j.chemosphere.2017.08.072
Wang, Y., Sheng, H. F., He, Y., Wu, J. Y., Jiang, Y. X., Tam, N. F. Y., et al. (2012). Comparison of the levels of bacterial diversity in freshwater, intertidal wetland, and marine sediments by using millions of illumina tags. Appl. Environ. Microbiol. 78, 8264–8271. doi: 10.1128/aem.01821-12
Watson, S. B., and Juttner, F. (2017). Malodorous volatile organic sulfur compounds: sources, sinks and significance in inland waters. Crit. Rev. Microbiol. 43, 210–237. doi: 10.1080/1040841x.2016.1198306
Weber, K. A., Achenbach, L. A., and Coates, J. D. (2006). Microorganisms pumping iron: anaerobic microbial iron oxidation and reduction. Nat. Rev. Microbiol. 4, 752–764. doi: 10.1038/nrmicro1490
Wright, M. S., Baker-Austin, C., Lindell, A. H., Stepanauskas, R., Stokes, H. W., and McArthur, J. V. (2008). Influence of industrial contamination on mobile genetic elements: class 1 integron abundance and gene cassette structure in aquatic bacterial communities. ISME J. 2, 417–428. doi: 10.1038/ismej.2008.8
Xia, F. F., Su, Y., Wei, X. M., He, Y. H., Wu, Z. C., Ghulam, A., et al. (2014). Diversity and activity of sulphur-oxidizing bacteria and sulphate-reducing bacteria in landfill cover soils. Lett. Appl. Microbiol. 59, 26–34. doi: 10.1111/lam.12240
Yakimov, M. M., La Cono, V., Denaro, R., D’Auria, G., Decembrini, F., Timmis, K. N., et al. (2007). Primary producing prokaryotic communities of brine, interface and seawater above the halocline of deep anoxic lake L’Atalante, eastern mediterranean sea. ISME J. 1, 743–755. doi: 10.1038/ismej.2007.83
Zhang, M. J., Liu, X. Y., Li, Y. B., Wang, G. Y., Wang, Z. N., and Wen, J. K. (2017). Microbial community and metabolic pathway succession driven by changed nutrient inputs in tailings: effects of different nutrients on tailing remediation. Sci. Rep. 7:474. doi: 10.1038/s41598-017-00580-3
Zhang, Y., Wang, X. G., Zhen, Y., Mi, T. Z., He, H., and Yu, Z. G. (2017). Microbial diversity and community structure of sulfate-reducing and sulfur-oxidizing bacteria in sediment cores from the east china sea. Front. Microbiol. 8:17. doi: 10.3389/fmicb.2017.02133
Zhang, X. J., Chen, C., Ding, J. Q., Hou, A. X., Li, Y., Niu, Z. B., et al. (2010). The 2007 water crisis in wuxi, china: analysis of the origin. J. Hazard. Mater. 182, 130–135. doi: 10.1016/j.jhazmat.2010.06.006
Keywords: human activities, odorous black water, sulfate-reducing microorganisms, sulfur-oxidizing microorganisms, community structure, microbial interactions
Citation: Wang R, Xu S, Jiang C, Zhang Y, Bai N, Zhuang G, Bai Z and Zhuang X (2019) Impacts of Human Activities on the Composition and Abundance of Sulfate-Reducing and Sulfur-Oxidizing Microorganisms in Polluted River Sediments. Front. Microbiol. 10:231. doi: 10.3389/fmicb.2019.00231
Received: 15 June 2018; Accepted: 28 January 2019;
Published: 12 February 2019.
Edited by:
Jonathan P. Zehr, University of California, Santa Cruz, United StatesReviewed by:
Beverly E. Flood, University of Minnesota Twin Cities, United StatesSonja Kristine Fagervold, UMS2348 Observatoire Océanologique de Banyuls-sur-Mer (OOB), France
Copyright © 2019 Wang, Xu, Jiang, Zhang, Bai, Zhuang, Bai and Zhuang. This is an open-access article distributed under the terms of the Creative Commons Attribution License (CC BY). The use, distribution or reproduction in other forums is permitted, provided the original author(s) and the copyright owner(s) are credited and that the original publication in this journal is cited, in accordance with accepted academic practice. No use, distribution or reproduction is permitted which does not comply with these terms.
*Correspondence: Shengjun Xu, sjxu@rcees.ac.cn Xuliang Zhuang, xlzhuang@rcees.ac.cn