- 1National Centre for Microbial Resource, National Centre for Cell Science, Pune, India
- 2Department of Gastroenterology and Human Nutrition, All India Institute of Medical Sciences, New Delhi, India
- 3Department of Transplant Immunology and Immunogenetics, All India Institute of Medical Sciences, New Delhi, India
- 4AgriGenome Labs Pvt. Ltd., Kerala, India
Recent studies on celiac disease (CeD) have reported alterations in the gut microbiome. Whether this alteration in the microbial community is the cause or effect of the disease is not well understood, especially in adult onset of disease. The first-degree relatives (FDRs) of CeD patients may provide an opportunity to study gut microbiome in pre-disease state as FDRs are genetically susceptible to CeD. By using 16S rRNA gene sequencing, we observed that ecosystem level diversity measures were not significantly different between the disease condition (CeD), pre-disease (FDR) and control subjects. However, differences were observed at the level of amplicon sequence variant (ASV), suggesting alterations in specific ASVs between pre-disease and diseased condition. Duodenal biopsies showed higher differences in ASVs compared to fecal samples indicating larger disruption of the microbiota at the disease site. The duodenal microbiota of FDR was characterized by significant abundance of ASVs belonging to Parvimonas, Granulicatella, Gemella, Bifidobacterium, Anaerostipes, and Actinomyces genera. The duodenal microbiota of CeD was characterized by higher abundance of ASVs from genera Megasphaera and Helicobacter compared to the FDR microbiota. The CeD and FDR fecal microbiota had reduced abundance of ASVs classified as Akkermansia and Dorea when compared to control group microbiota. In addition, predicted functional metagenome showed reduced ability of gluten degradation by CeD fecal microbiota in comparison to FDRs and controls. The findings of the present study demonstrate differences in ASVs and predicts reduced ability of CeD fecal microbiota to degrade gluten compared to the FDR fecal microbiota. Further research is required to investigate the strain level and active functional profiles of FDR and CeD microbiota to better understand the role of gut microbiome in pathophysiology of CeD.
Introduction
Celiac disease is a common, chronic immune mediated enteropathy of the small intestine which affects approximately 0.7% of the global population (Singh et al., 2018). Once thought to be uncommon in Asia, CeD is now prevalent in many Asian countries including India (Makharia et al., 2011). Recently, the prevalence of CeD has been on the rise, especially in developing countries (Lohi et al., 2007). This rapid rise in disease prevalence cannot be attributed only to the underlying genetic makeup of the population but rather to the environmental factors including infant feeding practices, reduction in infectious diseases, reovirus infection, and use of antibiotics (Jabri and Sollid, 2006; Lohi et al., 2007; Myléus et al., 2009; Volta and De Giorgio, 2012; Mårild et al., 2013; Bouziat et al., 2017).
CeD is caused by the consumption of gluten proteins present in cereals such as wheat, barley and rye in genetically susceptible individuals (Caminero et al., 2015). While many genes are involved in the development of CeD, thus far only the presence of HLA-DQ2 or DQ8 haplotype is considered to be essential (Sanz et al., 2011). Additional factors that contribute to pathogenesis include other co-genetic factors (genome wide association studies have identified several markers), wheat-related factors (age of ingestion, type and quantity of wheat) and the way gluten is metabolized in the intestine (Van De Wal et al., 1998; Kagnoff, 2007; Verdu et al., 2015). About 30–40% of the gluten protein consists of glutamine and proline. Since humans are unable to enzymatically break the molecular bonds between these two amino-acids, many immunogenic peptides are produced (Jabri and Sollid, 2006). There remains a possibility that enzymes secreted by the small intestinal microbiota convert some of these immunogenic peptides to non-immunogenic peptides.
While 20–30% of individuals in many countries including India are genetic susceptibility to develop CeD and the majority of them are exposed to wheat, only 1% of them develop CeD. This brings forth the role of other factors such as the gut microbiota in the pathogenesis of CeD (Sanz et al., 2011; Sánchez et al., 2012). Recently, numerous studies have highlighted the potential role of gut microbiota in inflammatory gastrointestinal diseases (Png et al., 2010; Fernandez-Feo et al., 2013; de Sousa Moraes et al., 2014; Schneeberger et al., 2015; Rivière et al., 2016; Zeng et al., 2017).
However, whether the changes in the microbial community structure and function in patients with CeD are cause or effect of the disease state remains unclear to date. In order to answer this question, one has to examine the status of the gut microbiota in the pre-disease state. Recently two studies investigated the microbiota of at-risk children who developed CeD few years after birth. One study observed an increase in Bifidobacterium breve and Enterococcus spp. in infants that developed active CeD (Olivares et al., 2018). Another study, did not observe any association between microbiota composition and development of CeD during the age of 9 and 12 months (Rintala et al., 2018). Nevertheless, potential microbiota related triggers for development of CeD in later adult life still remain unclear. While 70–80% percent of first-degree relatives (FDRs) of patients with CeD have HLADQ2/DQ8 haplotype (compared to 30% in the general population); only approximately 8.5% of FDRs develop CeD (Singh et al., 2015). Thus, the question arises; why do only few FDRs develop CeD and what is the role of the gut microbiome in disease protection? Indirect evidence of altered microbiota in relatives of patients with CeD is suggested by significantly lower levels of acetic acid and total short chain fatty acids (SCFA), and higher fecal tryptic activity (Tjellström et al., 2007). There is a lack of information regarding the gut microbial composition and function in adult FDRs of patients with CeD. Additionally, it is important to explore the status of the microbiota in both the small intestine, the site of the disease, and feces, as representative of whole gut microbiome. To test the hypothesis that gut microbiome of FDR is different from CeD and could potentially play an important role in the pathogenesis of CeD, we explored the composition of both small intestinal and the whole gut microbiome using Illumina MiSeq in a subset of patients with CeD, FDR and controls. We further investigated the potential microbial functions that are characteristic of FDR and CeD microbiota.
Materials and Methods
Human Subjects, Duodenal Biopsies and Fecal Sample Collection
A total of 62 subjects participated in this study including 23 treatment naïve patients with CeD [all HLA-DQ2/DQ8+, having high titre of anti-tissue transglutaminase antibodies (tTG Ab) and having villous abnormalities of modified Marsh grade 2 or more], 15 healthy FDRs of patients with CeD [having normal titre of anti-tTG Ab and having no villous abnormalities of modified Marsh grade 0 or 1], and 24 controls (patients with Hepatitis B Virus carriers or those having functional dyspepsia; having normal titre of anti-tTG Ab and having no villous abnormalities; Table 1). Duodenal biopsies and fecal samples were collected from each of the above mentioned subjects at All India Institute of Medical Sciences, New Delhi, and sent to National Centre for Cell Sciences, Pune for microbiome analysis. The ethics committees of All India Institute of Medical Sciences, New Delhi, and National Centre for Cell Sciences, Pune, India approved the study. Informed and written consent was obtained from all the participants. There was a significant different in the age between the three groups (p < 0.05, Kruskal-Wallis test). Further details of patients and controls have been provided in the (Supplementary Table S3).
DNA Extraction and 16S rRNA Gene Sequencing
Total DNA was extracted from duodenal biopsies using QIAGEN DNeasy Blood and Tissue kit (QIAGEN, Germany) and fecal samples using the QIAamp fast DNA stool Mini Kit (QIAGEN, Germany) according to the manufacturer’s instructions. We used Illumina MiSeq sequencing to determine the microbial composition of the duodenal biopsies and fecal samples. PCR was set up in 50 μl reaction using AmpliTaq Gold PCR Master Mix (Life Technologies, United States) and with 16S rRNA V4 variable region-specific bacterial primers 515F (5′-GTGCCAGCMGCCGCGGTAA-3′) and 806R (5′- GGACTACHVGGGTWTCTAAT-3′) (Walters et al., 2016).
Sequence Processing and Bacterial Community Analysis
Illumina Miseq platform rendered a total of 76058052 raw 16S rRNA sequence reads for the 102 fecal and biopsy samples of the diagnosis groups, with an average of 745667 ± 194667 reads per sample. Adapter sequences were trimmed by using Cutadapt (1.18) tool (Martin, 2011) and trimmed reads were pooled as Fasta.gz file format for further analysis in DADA2 (v 1.6.0) pipeline (Callahan et al., 2016). In the first step reads were inspected for read quality profile, the read quality score was decreased (< 30) after 240 bases for forward read and 160 bases for reverse reads. We truncated the forward reads at position 240 (trimming the last 10 nucleotides) and reverse reads at position 160 (trimming the last 90 nucleotide). After quality filtering and removal of bases with a total of 70502947 (92.69%) high-quality reads of the 16S rRNA amplicons were obtained, with an average 691205 ± 181263 reads per sample, ranging from 325350 to 1207169 among samples (Supplementary Table S4). Finally, taxonomic assignment was done by the naive Bayesian classifier method with default setting as implemented in DADA2, against Human Intestinal 16S rRNA gene reference taxonomy database (HITdb v 1.00). Briefly, HITdb is a 16S rRNA gene database based on high-quality sequences specific for human intestinal microbiota, this database provides improved taxonomic up to the species level (Ritari et al., 2015). Unassigned chimeric and sequences of chloroplast and mitochondria were excluded from downstream analysis. Taxonomic assignment successfully mapped 6567144 ASVs, with an average of 64383 ± 29929 ASVs per sample. Finally, from these ASVs, ASV table was constructed and the ASVs generated by the contaminants were removed by using decontam software (Davis et al., 2018) and the output ASV table was used for downstream analyses.
Microbial diversity and composition analysis were done using the R-package phyloseq (v1.22.3) (McMurdie and Holmes, 2013) and microbiome R package (v1.0.2).1 To test for similarities in microbial communities between sample types and diagnosis groups Analysis of similarities (ANOSIM) on Bray-Curtis distances was used. ANOSIM is a function in vegan package (v 2.4-4) to calculate significance of PCoA clustering based on the Bray-Curtis distances (Oksanen et al., 2011).
To identify differentially abundant ASVs in pairwise comparisons between diagnosis groups we used DESeq2 (v1.18.0) (Love et al., 2014). All ASVs that were significantly (alpha = 0.01) different in abundance between the diagnosis groups were reported and were adjusted for multiple comparisons using the Benjamini-Hochberg, false discovery rate procedure. Data was visualized using ggplot2 (v 2.2.1) in R (Wickham, 2011, 2017).
Analysis of 16S rRNA Gene Copies of H. pylori
Available full-length 16S rRNA gene sequences of H. pylori were downloaded from LPSN-list of prokaryotic names with standing in nomenclature (Parte, 2013). To identify and extract the V4 regions from these 16S rRNA gene sequences, V4 variable region-specific primers 515F (5′-GTGCCAGCMGCCGCGGTAA-3′) and 806R (5′-GGACTACHVGGGTWTCTAAT-3′) were used. Next, to highlight possible similarities and differences between the V4 regions we performed multiple sequence alignment (MSA) using CLUSTAL W 2.0.11 (Larkin et al., 2007). The full-length 16S rRNA gene sequence of E. coli strain U 5/41 was used as a reference sequence (Supplementary Information).
Metagenomic Imputation
Piphillin tool was used to infer metagenome from 16S rRNA ASV counts table and representative sequence of each ASV. Briefly, this tool predicts metagenomes with high accuracy by leveraging the most-current genome reference databases (Iwai et al., 2016). It uses direct nearest-neighbor matching between 16S rRNA amplicons and genomes to predict the represented genomes. Latest version (May, 2017) of KEGG database and 97% of the identity cutoff was selected for the prediction. The output from Piphillin was further analyzed by STAMP statistical tool, ANOVA with post hoc Tukey-kramer test was used to identify statistically different KEGG orthologies between diagnosis groups (Parks et al., 2014).
Results
Comparison of Fecal and Duodenal Microbial Community in the Study Cohort
The characteristics of the study subjects have been summarized in the Table 1. All the participants were on staple gluten containing diet during sampling for this particular study. After diagnosis of CeD the patients underwent therapy with dietary recommendation to avoid gluten in daily diet. However, in the present study, we do not include samples after dietary changes. The duodenal biopsies and fecal samples were included to investigate differences in both site-specific and whole gut microbial diversity and community structure in patients with CeD, FDRs and DC (non-celiac Disease-Control group). Irrespective of the diagnosis group i.e., CeD, FDR, or DC, the microbial community in the fecal samples was different when compared to the microbial community in duodenal biopsies (ANOSIM statistic R: 0.4998, Significance: 0.001), (Supplementary Figure S1A).
However, there was no significant difference in alpha diversity between the sampling sites (Supplementary Figure S1B).
Site Specific Comparison of Duodenal and Fecal Microbiota of FDRs, CeD and Control Group
To investigate if patients with CeD, FDRs or DC had site specific dissimilarities in microbiota composition, further analysis was divided based on the sampling site.
Pairwise comparison of alpha diversity of the duodenal microbiota of FDRs, CeD and controls showed no significant difference (Figure 1A). Similarly, for fecal microbiota, no significant difference was observed in the alpha diversity between the diagnosis groups (Figure 1B).
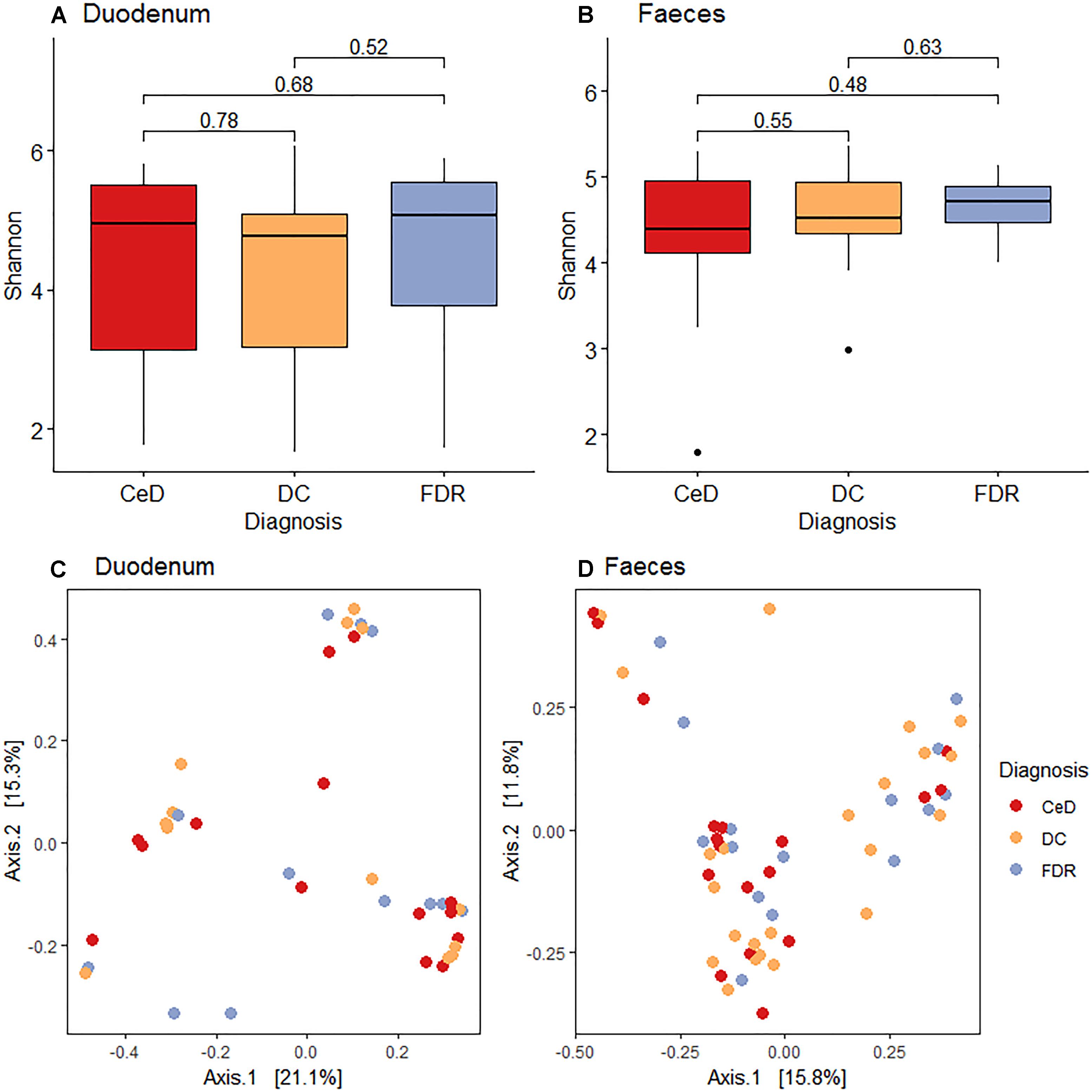
Figure 1. (A) Pairwise comparison of alpha diversity of the duodenal microbiota of diagnosis groups. (B) Pairwise comparison of alpha diversity of fecal microbiota of diagnosis groups. (C) Principle coordinates analysis of microbial community based on Bray-Curtis distance between diagnosis groups in duodenal microbiota. (D) Principle coordinates analysis of microbial community based on Bray-Curtis distance between diagnosis groups in fecal microbiota.
Unconstrained ordination using the Bray-Curtis dissimilarity showed no significant differences in the duodenal microbiota of CeD, FDRs and DC (Analysis of similarities; ANOSIM test; R-statistic = 0.0014, p = 0.427) (Figure 1C). Similarly, there was no significant difference in the fecal microbiota of the three diagnosis groups (Analysis of similarities; ANOSIM test; R-statistic = 0.051, p = 0.058) (Figure 1D).
Comparison of Duodenal Microbiota Composition Between the Diagnosis Groups
At phylum level, Actinobacteria, Bacteroides, Euryarchaeota, Firmicutes and Proteobacteria were the dominant members in the duodenal microbiota (Figure 2).
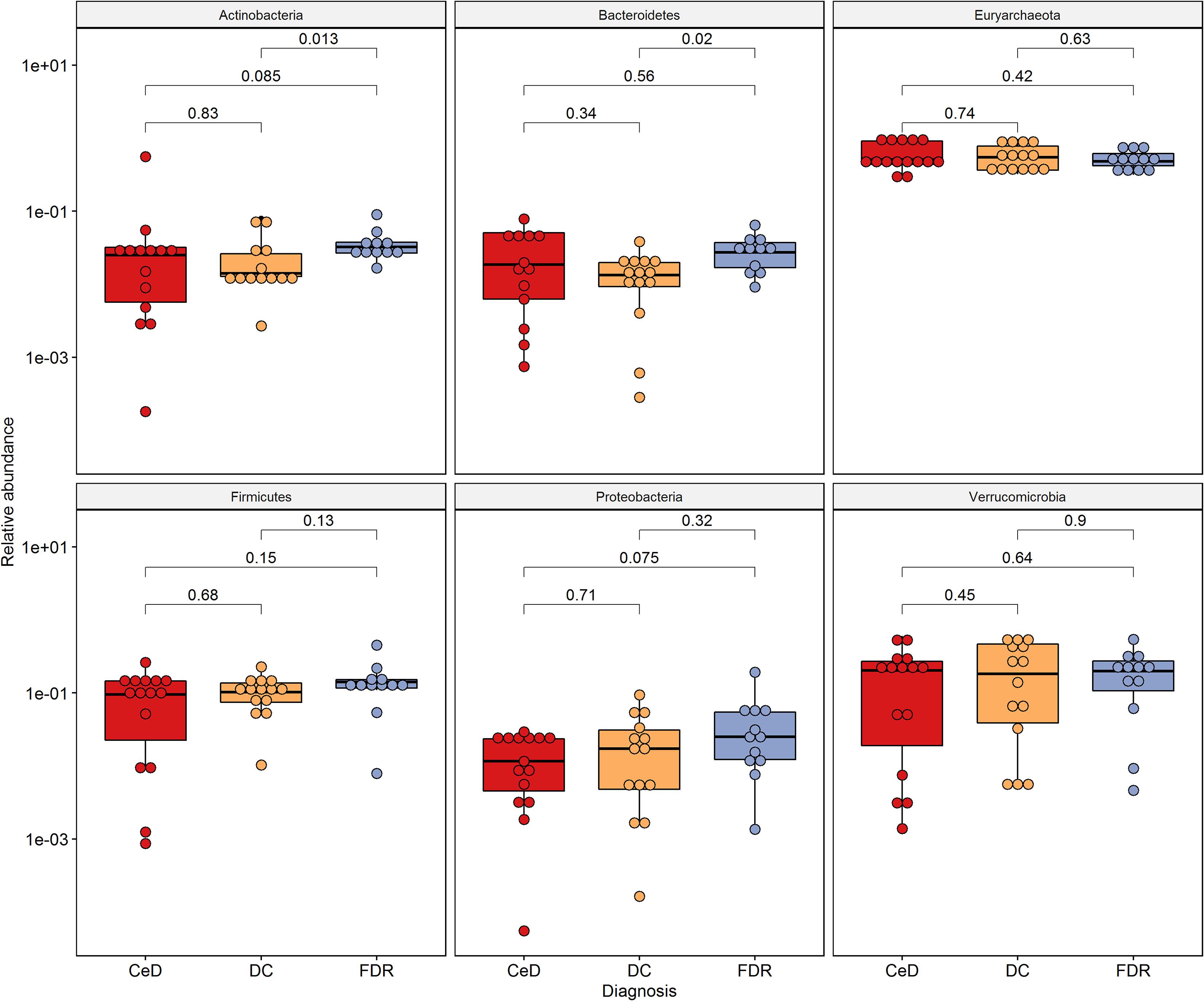
Figure 2. Phylum level distribution of ASVs in duodenal microbiota. Pairwise comparisons were done using Wilcoxon tests.
0 The phyla, Actinobacteria (p = 0.013) and Bacteroides (p = 0.02) were significantly higher in pre-disease state (FDR) in comparison to the DC microbiota. Although at the phylum level there was no significant difference in the abundance of Firmicutes, the order Clostridiales within this phylum showed significantly higher abundance in FDR microbiota compared to DC microbiota (p < 0.05) (Supplementary Figure S2). There was no significant difference in abundance of the other major phyla between the FDR and CeD as well as the CeD and DC microbiota (Figure 2).
Differences in ASV Abundances in Duodenal Microbiota of the Diagnosis Groups
Differential abundance analysis of ASVs using DESeq2 revealed more than 20-fold higher abundance of ASVs belonging to genera Parvimonas, Granulicatella, Gemella, Bifidobacterium, Anaerostipes, and Actinomyces in the FDR group compared to both CeD and DC groups (p-value < 0.01, Figures 3A,C). In the CeD group, ASVs belonging to genera Helicobacter and Megasphaera were highly abundant compared to both FDR and DC group (Figures 3B,C).
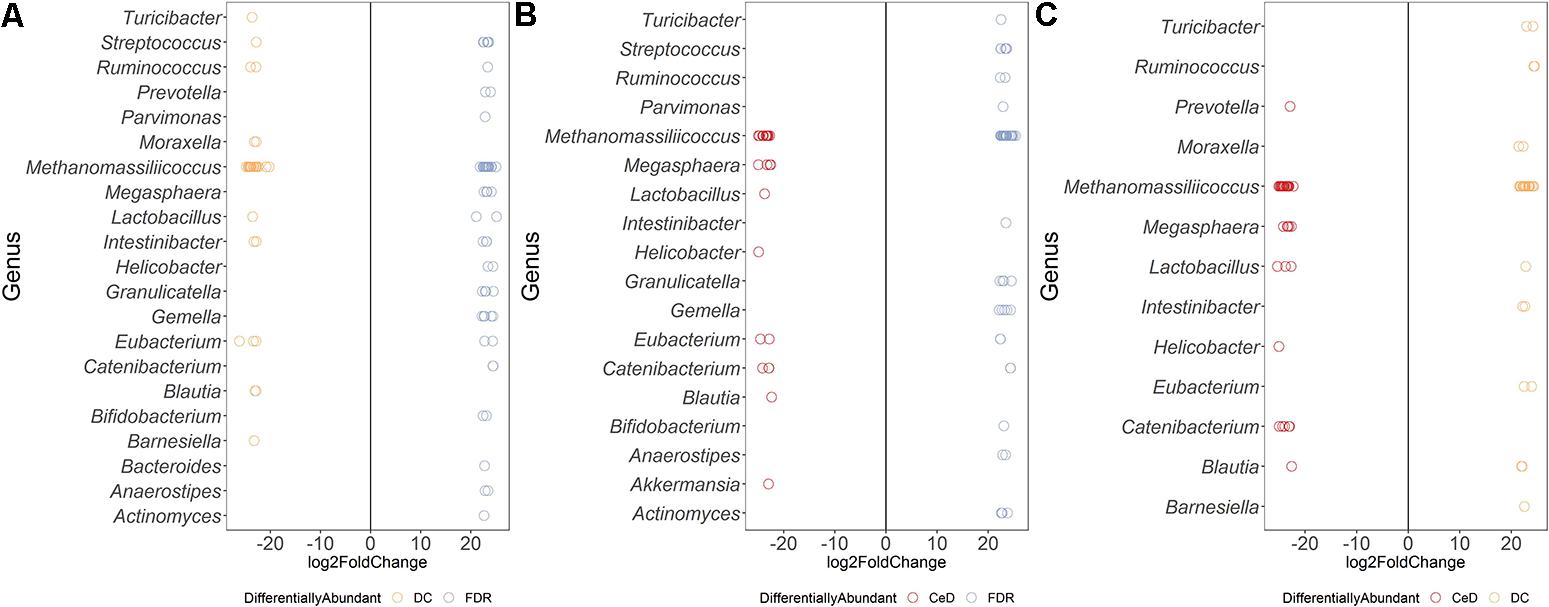
Figure 3. Comparison of differential abundance of microbial ASVs between the diagnosis groups in duodenal microbiota. (A) Differential abundance DC vs FDR. (B) Differential abundance CeD vs DC (C) Differential abundance CeD vs FDR. Only ASVs with significant differences (P 0.01) in log2 fold change are depicted.
Investigation of the differentially abundant ASVs between the FDR and CeD group revealed differences in the abundances of specific ASVs within the same genus (Figure 3C). Some ASVs within the archaeal genus Methanomassiliicoccus were highly abundant in the CeD group while others were highly abundant in FDR group (Figure 3C). Notably, there is only one species of this archaeal genus, Methanomassiliicoccus luminyensis, validly described in literature with a single copy of 16S rRNA gene in its genome (Dridi et al., 2012; Gorlas et al., 2012). This suggests a possibility of either species- or strain- level differences in Methanomassiliicoccus between the CeD and FDR which requires further investigation. Additionally, ASVs belonging to genera Eubacterium and Catenibacterium showed ASV level variation in abundance between the CeD and FDR duodenal microbiota (Figure 3C).
Comparison of both the FDR and CeD duodenal microbiota with that of the DC duodenal microbiota revealed similarities in the abundances of specific ASVs. Both the CeD and FDR duodenal microbiota were characterized by the abundance of ASVs belonging to genus Prevotella, Megasphaera, Helicobacter and Catenibacterium when compared to the DC duodenal microbiota (Figures 3A,B).
We further checked for similarities and differences between these Helicobacter ASVs because, the ASVs of Helicobacter were abundant in FDR and CeD duodenal microbiota when compared to DC duodenal microbiota and one specific ASV was abundant in CeD compared to FDR duodenal microbiota. We observed that the ASV identified as differentially abundant in the CeD duodenal microbiota when compared to both FDR and DC microbiota was ASV1811. Contrary to this, ASV2016 and ASV4095 belonging to H. pylori were high in abundance in the FDR duodenal microbiota compared to DC duodenal microbiota (Supplementary Information). In view of intra-genomic differences in 16S rRNA gene, we compared the 16S rRNA gene copies of H. pylori in publically available genomes. We observed that on average the H. pylori genome has two copies of 16S rRNA gene and we did not observe differences between the two copies within a single genome in the V4 region investigated here (Supplementary Information text page 3–7). The analysis used for microbial profiling in the present study employs a well-established algorithm to identify finer sequence level variation and differentiate single nucleotide level difference in the 16S rRNA gene amplicon (Callahan et al., 2016). We suggest that future investigation of CeD and FDR duodenal microbiota need to focus on strain level variations and functional aspects of H. pylori using metagenomics and functional omics.
Both CeD and FDR duodenal microbiota were characterized by low abundance (20-fold) of Barnesiella when compared to that in DC (Figures 3A,B). Interestingly, the genus Lactobacillus showed variation in abundances at ASV level between the three diagnosis groups (Figures 3A–C). Strains of Lactobacillus are reported to have probiotic effects, ability to degrade gluten and are often associated with health benefits (Lorenzo Pisarello et al., 2014; Orlando et al., 2014). The differences at ASV level observed in our study indicate the need to investigate the potential impact of species- and/or strain-level differences within the genus Lactobacillus in CeD and FDR subjects.
Comparison of Fecal Microbiota Composition Between the Diagnosis Groups
There was no significant difference in abundance of the phyla Proteobacteria, Actinobacteria, Euryarchaeota and Firmicutes (Figure 4) between the diagnosis groups. The phylum Bacteroidetes was found to be marginally lower in abundance in the FDR group when compared to the DC group (p = 0.054). Similar trend was observed for order Bacteroidales (p = 0.054, Supplementary Figure S3). The order Clostridiales was significantly abundant in FDRs in comparison to DC group (p = 0.017) (Supplementary Figure S3). The order Clostridiales was also observed to abundant in duodenal microbiota of FDRs (Supplementary Figure S2).
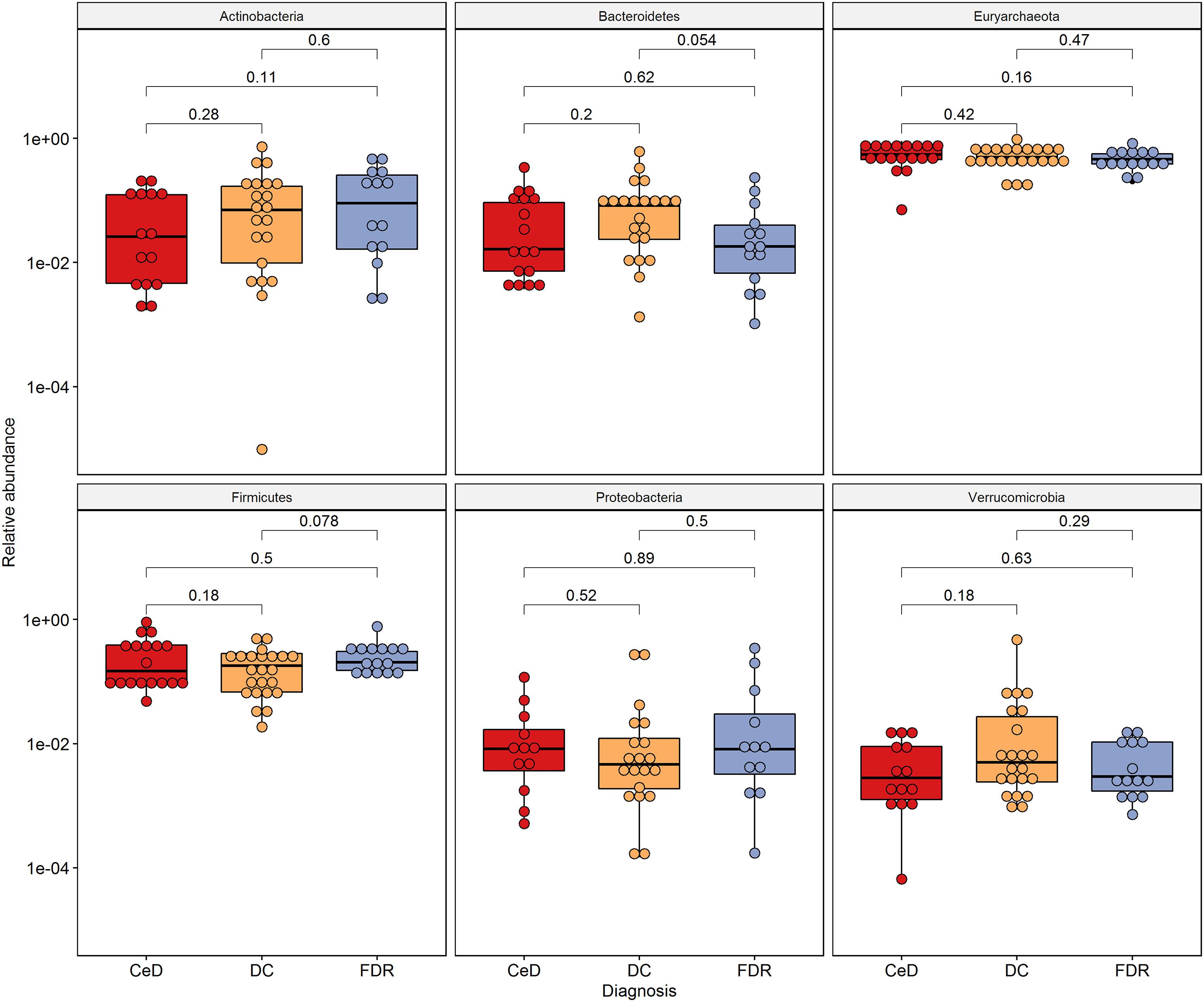
Figure 4. Phylum level distribution of ASVs in fecal microbiota. Pairwise comparisons were done using Wilcoxon tests.
Differences in ASV Abundances in Fecal Microbiota of the Diagnosis Groups
When compared to the duodenal microbiota, fewer ASVs were differentially abundant between the diagnosis groups which suggests a lower variation in fecal microbiota at ASV-level.
Both FDR and CeD fecal microbiota was characterized by a 20-fold decrease in abundance of ASVs belonging to genera Dorea and Akkermansia (Figures 5A,B). In addition, when compared to DC, FDR fecal microbiota showed lower abundance of ASVs belonging to Lactobacillus and Haemophilus while, fecal microbiota of CeD had lower abundance of Prevotella (Figures 5A,B). Interestingly, CeD fecal microbiota had ASV level differences in abundance of Lactobacillus when compared to DC fecal microbiota (p < 0.01, Figure 5B). Similarly, Methanomassiliicoccus showed ASV level variation in abundances between the three diagnosis groups (p < 0.01, Figures 5A–C).
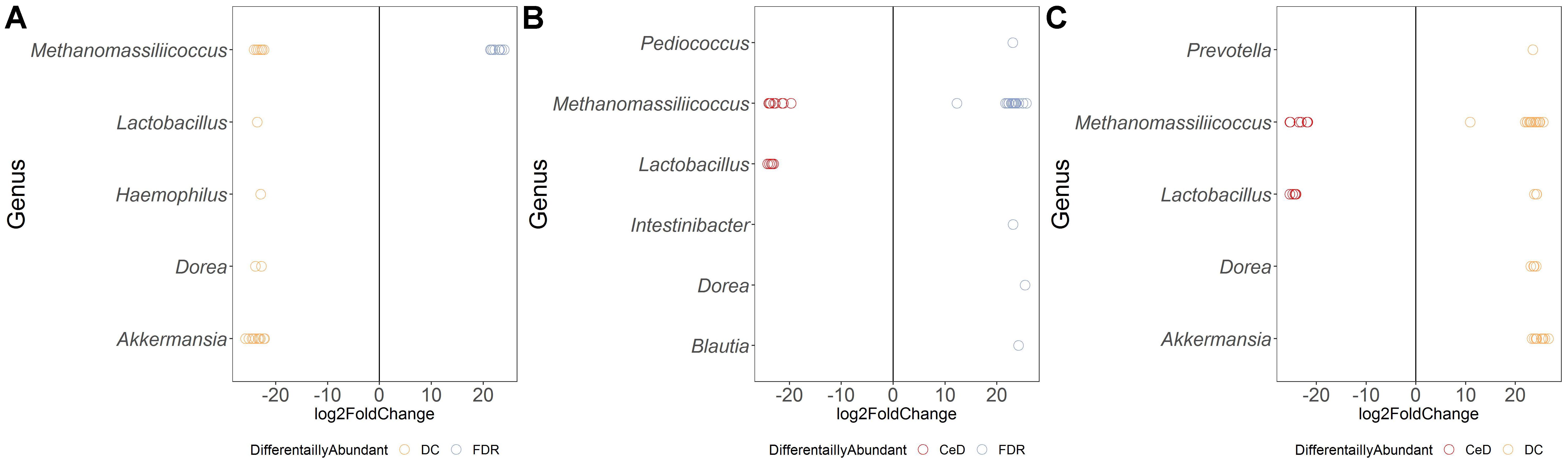
Figure 5. Comparison of differential abundance of microbial ASVs between the diagnosis groups in fecal microbiota. (A) Differential abundance DC vs FDR. (B) Differential abundance CeD vs FDR. (C) Differential abundance CeD vs DC. Only ASVs with significant differences (P < 0.01) in average log2 fold change are depicted.
Comparison of ASV abundances between the FDR and CeD fecal microbiota revealed higher abundance of ASVs from genera Pediococcus, Intestinibacter, Blautia and Dorea in the FDR microbiota (p < 0.01) (Figure 5C). However, in comparison with DC fecal microbiota, FDR microbiota had 20-fold lower abundance of ASVs related to Akkermansia and Dorea (p < 0.01).
Imputed Metagenome of FDR and CeD Fecal Microbiome Shows Reduced Proportion of Genes Involved in Gluten Metabolism
In addition to differentially abundant microbial ASVs, different study groups might have altered metabolic potential. Of specific interest were the enzymes related to glutenases as they play a role in breakdown of gliadin residues. We followed Piphillin workflow to predict functional profile of fecal and duodenal microbiota (Iwai et al., 2016). A total of 159 KEGG orthologies (KO) were significantly different between diagnosis groups in the fecal microbiota (Supplementary Table S1). Among these the KO abundance for Xaa-pro dipeptidase (K01271, Prolidase) enzyme which is known to have role in gluten degradation was found to be significantly reduced in CeD as compared to FDR and DC fecal microbiota (Figure 6). Notably, we did not observe any significant difference in the predicted abundance of prolidase in the duodenal microbiota (Supplementary Table S2).
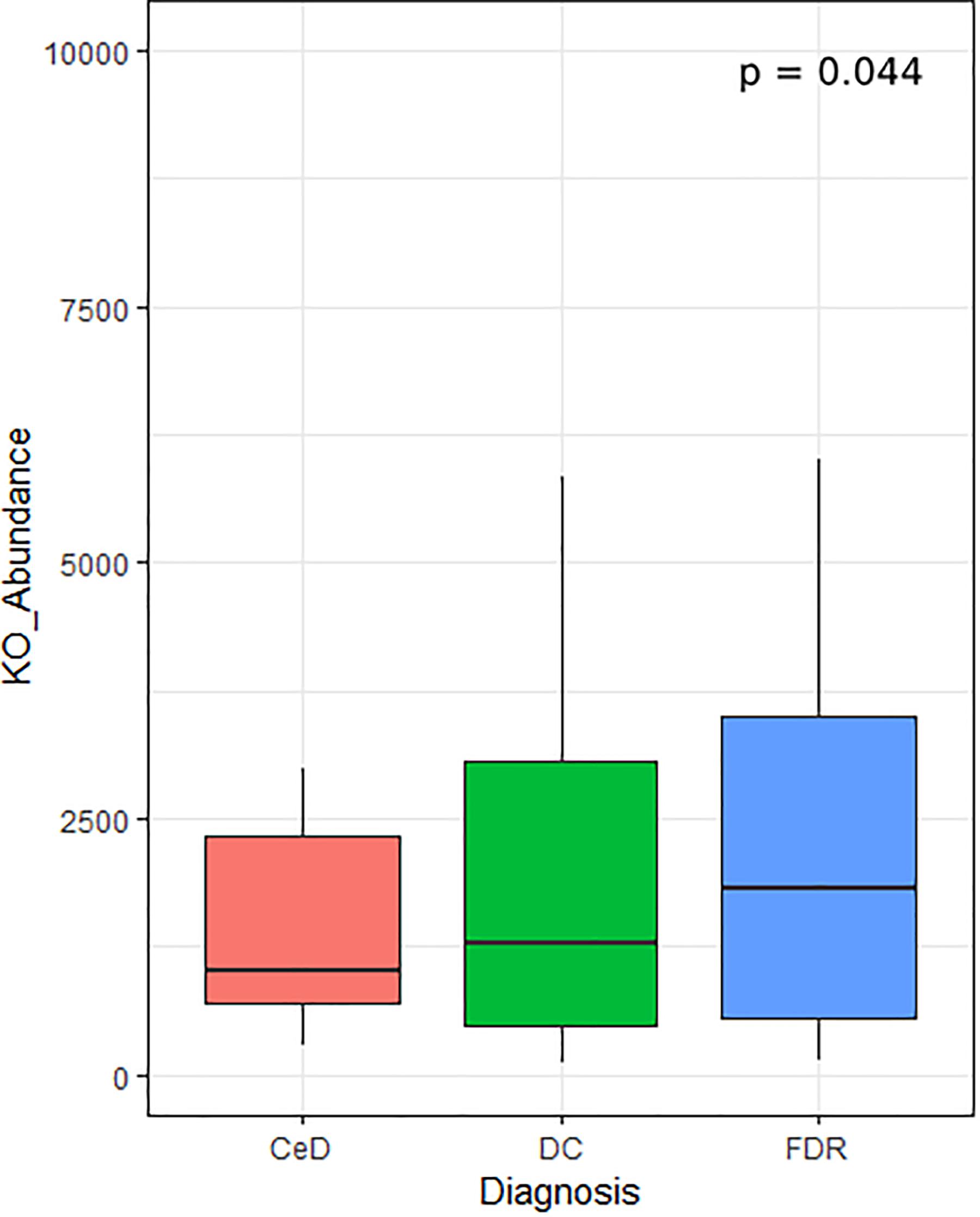
Figure 6. KO abundance for Xaa-pro dipeptidase (K01271) enzyme in feces inferred from predicted metagenome for fecal samples. Comparison was done using ANOVA.
Discussion
The aim of the present study was to investigate differences in the duodenal and fecal microbiota of pre-diseased state i.e., FDRs subjects compared to diseased state i.e., CeD and DC. The FDR group was included for two main reasons: (1) They represent a population which is genetically-susceptible to develop CeD; (2) They provide a unique opportunity to identify features of the host as well as of the associated microbiota that may be involved in the protection against developing CeD. We collected both duodenal biopsies and fecal samples to investigate both local and overall changes in the microbiota in FDR, patients with CeD and DC. To the best of our knowledge, reports on site specific microbiota patterns in adult patients with CeD remain scarce, and no results on both site specific and whole gut microbiome on FDRs have been reported to date. Present study provides an overall view on differences of both site-specific changes as well as changes in the fecal microbiota of FDRs, CeD and DC.
The duodenal microbiota of CeD was characterized by high abundance of Helicobacter and Megasphaera compared to both FDR and DC group. In addition, comparison of Helicobacter ASVs suggested ASV-level variation between FDR and CeD duodenal microbiota. Previously, CeD patients with H. pylori gastritis were reported to have an increased number of intraepithelial lymphocytes in the duodenal mucosa (Villanacci et al., 2006). On the contrary, there are also reports which have failed to reveal a relationship between H. pylori and CeD and found that H. pylori presence was inversely associated with CeD (Lebwohl et al., 2013). In the present study, the ASV which is abundant in CeD is different from those ASVs which are enriched in FDR, emphasizing the need for investigating strain level variations in H. pylori and its potential impact on pathophysiology of CeD. There were other genera which showed ASV level difference between the microbiota of the CeD, FDR and DC such as, Streptococcus, Ruminococcus, Methanomassiliicoccus, Catenibacterium, Intestinibacter, and Blautia. Specific interest would be for identifying those ASVs that are differentially abundant between the FDR and CeD microbiota. In this comparison between the pre-disease and disease state, we observed a reduced abundance of ASVs related to Ruminococcus, Parvimonas, Intestinibacter, Granulicatella, Gemella, Bifidobacterium, Anaerostipes, and Actinomyces in the diseased state. The species from genera Ruminococcus and Anaerostipes are known to produce SCFA such as acetate and butyrate that have beneficial effect on the host (Ze et al., 2012; Morrison and Preston, 2016; Shetty et al., 2017). The genus Granulicatella is commonly associated with diseases such as cancer, Crohn’s disease and bacteremia (Woo et al., 2003; Nakatsu et al., 2015; Qiu et al., 2017). The bacterial species within the genera Actinomyces, Streptococcus, Bifidobacterium, and Anaerostipes are known to possess gluten degrading enzymes, probiotic properties and ability to produce SCFA, respectively (Barrangou et al., 2009; Fernandez-Feo et al., 2013, Couvigny et al., 2015; Morrison and Preston, 2016, Rivière et al., 2016). Moreover, a strain belonging to Bifidobacterium was recently reported to prevent gluten-related immunopathology in mice (McCarville et al., 2017). Higher abundances of ASVs belonging to the above-mentioned genera in small intestine of FDRs compared to CeD may indicate their potential protective role in pre-disease state.
In comparison to duodenal biopsies, a smaller number of ASVs were differentially abundant between the diagnosis groups in fecal samples. This indicates more disrupted microbiome at disease site than faecal gut microbiome and highlights the importance of inclusion of biopsy samples in present study. We observed ASV level variation in the both fecal and duodenal microbiota between the diagnosis groups. Previously, a higher abundance of Lactobacillus was observed in the oral microbiome of patients with CeD (Tian et al., 2017). Moreover, there are reports stating that the certain Lactobacillus species degrade gliadin and increases the availability of antigenic peptides (Engström et al., 2015). In the present study, higher abundance of different ASVs of Lactobacillus in CeD and in FDR microbiota may suggest strain level differences in ability to breakdown gluten into either pro-inflammatory or anti-inflammatory peptides in the small intestine. However, this will require more in-depth characterization of the strain-level variation in functional capabilities of Lactobacillus species. Another important observation from differential abundance analysis was the significantly lower abundance of Barnesiella in CeD and FDR compared to DC duodenal microbiota and significantly lower abundance of Akkermansia in fecal microbiota of both CeD and FDR compared to DC. While Akkermansia was highly abundant in CeD microbiota when compared to FDR microbiota. Both of these genera are known to degrade mucus and produce SCFAs which in turn strengthens the health of enterocytes and inhibits intestinal inflammation (Desai et al., 2016; Ravcheev and Thiele, 2017). Therefore, there is a need for more detailed investagion of these bacteria commonly residing in the mucus layer.
Through metagenome prediction method, we found that the gene abundance for Xaa-pro Dipeptidase enzymes was less in CeD as compared to FDR and DC microbiota. This enzyme shows a high specificity for proline residues present in gluten and hydrolyze the peptide bond (Park et al., 2004). These observations suggest that the FDR and CeD fecal microbiota differs in the bacterial composition and that there is a difference in specific bacteria that are capable of gluten degradation. As a consequence, this may impact gluten processing and the presentation of immunogenic gluten epitopes to the immune system. However, the observations of the predicted metagenome have to be validated with in vitro enzyme assay.
Overall, we observe differences at ASV level between the FDR and CeD microbiota. We do not observe major differences in community diversity and structure from both alpha diversity and community dissimilarity analysis. The potential species and/or strain level variations and functional aspects identified in this study emphasize the need for well-designed mechanistic follow-up studies using bacteria identified as different between the disease and pre-disease states.
However, metagenomic studies of biopsy samples remain a challenge because of high proportion of host DNA. Thus, predictive metagenomics using 16S rRNA gene as a practical solution was employed for biopsies. In this initial exploratory study, we investigated the gut microbiome with respect to the disease status only and future studies considering other confounding factors such as diet, body mass index age, sex, frequency and quantity of gluten intake among others will be required for a better understanding the gut microbiome in CeD and FDRs. Additionally, the control group in our study was not healthy subjects but patients with functional dyspepsia. These subjects were used as proxy since invasive sampling procedures such as endoscopy from clinically healthy subjects is not permitted under the institutional regulations. Since one of the aims of the present study was to identify similarities and differences between the FDR and CeD microbiota, a control group with a different disease was used as comparison group. This procedure of using a control group with a disease other than the focus of the main study is common practice in epidemiological studies (Coggon et al., 2009). However, this could have also resulted in underestimating the number of ASVs that could be variable between FDR vs DC and CeD vs DC, especially in the CeD group because the proposed disease model for functional dyspepsia includes low-grade duodenal inflammation (Talley et al., 2017). Despite the fact that, H. pylori is a recognized causative agent of functional dyspepsia, we observe high abundance of ASVs related to H. pylori in CeD and FDR microbiota (Sugano et al., 2015). Therefore, the possible role of H. pylori in pathophysiology of CeD requires further investigation. Based on the findings of the present study, it can be hypothesized that the microbiota of FDR represents a potentially balanced, non-inflammatory state as compared to that of microbiota of patients with CeD in genetically pre-disposed subjects. High abundance of known pro-inflammatory bacteria such as those related to Helicobacter could have a critical role in the pathogenesis of CeD. In addition, expansion of specific species or strains of gluten degrading bacteria which breakdown gluten into pro-inflammatory peptides may have a pivotal role in the pathogenesis of CeD. Exposure to drugs (e.g., proton pump inhibitors) and antibiotics have been hypothesized to select particular strains of bacteria in humans (Domínguez-Bello et al., 2008). A long-term follow-up of FDRs of patients with CeD will be crucial to identify triggers such as dietary changes, lifestyle changes, medications, specifically antibiotics that could affect the microbiota homeostasis in them and factors that lead to transition toward a pro-inflammatory microbiota from a non-inflammatory microbiota.
Conclusion
Significant differences at ASV level suggest that specific bacteria like Helicobacter may be important for pathogenesis of CeD. Higher abundance of potentially beneficial bacterial ASVs especially those belonging to SCFA producing genera in FDRs suggest that there may be a protective role of these in CeD development. Moreover, the predicted differences in gluten metabolism potential by FDR and CeD microbiota point toward the need for investigating functional capabilities of specific bacteria in healthy FDR and CeD patients.
Data Availability
Sequence data generated in this study is available from the NCBI Sequence Read Archive within the Bioproject ID accession PRJNA385740 (https://www.ncbi.nlm.nih.gov/bioproject/?term~=~PRJNA385740) and to reproduce the analysis done in R, the R Markdown file and required data are available at https://github.com/rahulnccs/Comparison-of-Small-Gut-and-Whole-Gut-Microbiota-of-First-Degree-Relatives-with-Adult-Celiac-Disease.
Author Contributions
GM, YS, and VA conceived, designed, and supervised the study. GM recruited the patients, and performed the diagnosis and endoscopic examination. GK performed the HLA test. AV, KB, and AM were responsible for storage and maintenance of the collected biological samples (duodenal biopsy/stool). RB and PP extracted the genomic DNA. DB, BS, and RP were involved in amplicon sequencing. SS, DD, and RB performed the bioinformatics analysis for amplicon data. SS, RB, DD and GM acquired and interpreted the data, and drafted the manuscript. YS, DD, and VA critically reviewed the manuscript. All authors have read and approved the final manuscript.
Funding
We acknowledge the support of the Food and Nutrition Division of Department of Biotechnology, Government of India who provided the research grant for this study (Sanction No. BT/PR-14956/FNS/20/497/2010).
Conflict of Interest Statement
DB, BS, and RP were employed by company AgriGenome Labs Pvt Ltd. Kerala, India.
The remaining authors declare that the research was conducted in the absence of any commercial or financial relationships that could be construed as a potential conflict of interest.
Acknowledgments
We acknowledge the help of Aishwairya Sharma, a dietician, who counseled the patients for gluten free diet. We are grateful to Dr. Nachiket Marathe for fruitful discussion related to the project. We thank Johanna Gutleben for proofreading the manuscript. We would like to acknowledge the effort made by the reviewers during the peer review process and for assisting us to improve the overall quality of data presented in this study. We sincerely acknowledge the support of each participant of this study who accepted to participate in this study.
Supplementary Material
The Supplementary Material for this article can be found online at: https://www.frontiersin.org/articles/10.3389/fmicb.2019.00164/full#supplementary-material
FIGURE S1 | (A) Principal coordinates analysis (PCoA) of the fecal and duodenal microbiota based on Bray–Curtis distance. (B) Comparison of alpha diversity measures between sampling sites.
FIGURE S2 | Order level distribution of ASVs in duodenal microbiota. Pairwise comparisons were done using Wilcoxon tests.
FIGURE S3 | Order level distribution of ASVs in fecal microbiota. Pairwise comparisons were done using Wilcoxon tests.
TABLE S1 | Significantly different KEGG orthologies (KO) between diagnosis groups in fecal microbiota. Analyzed using the STAMP statistical tool, ANOVA with post hoc Tukey–kramer test was used to identify statistically different KEGG orthologies between diagnosis groups.
TABLE S2 | Significantly different KEGG orthologies (KO) between diagnosis groups in duodenal microbiota. Analyzed using the STAMP statistical tool, ANOVA with post hoc Tukey–kramer test was used to identify statistically different KEGG orthologies between diagnosis groups.
TABLE S3 | Details of the study subjects including disease status, Marsh values, age, gender, and tTg titre.
TABLE S4 | Number of sequencing reads per sample at each stage of data analysis is given below.
INFORMATION | Differential Abundance of Amplicon Sequence Variants of Helicobacter. Multiple sequence alignment was performed by CLUSTAL 2.0.11.
Abbreviations
ANOSIM, analysis of similarities; ASV, amplicon sequence variant; CeD, celiac disease; DADA2, divisive amplicon denoising algorithm 2; DC, diseased controls (dyspeptic); FDR, first degree relatives; HLA, human leukocyte antigen; KEGG, kyoto encyclopedia of genes and genomes; PCoA, principal coordinates analysis; rRNA, ribosomal ribonucleic acid; SCFA, short chain fatty acids; tTG, tissue transglutaminase.
Footnotes
References
Barrangou, R., Briczinski, E. P., Traeger, L. L., Loquasto, J. R., Richards, M., Horvath, P., et al. (2009). Comparison of the complete genome sequences of Bifidobacterium animalis subsp. lactis DSM 10140 and Bl-04. J. Bacteriol. 191, 4144–4151. doi: 10.1128/JB.00155-09
Bouziat, R., Hinterleitner, R., Brown, J. J., Stencel-Baerenwald, J. E., Ikizler, M., Mayassi, T., et al. (2017). Reovirus infection triggers inflammatory responses to dietary antigens and development of celiac disease. Science 356, 44–50. doi: 10.1126/science.aah5298
Callahan, B. J., McMurdie, P. J., Rosen, M. J., Han, A. W., Johnson, A. J. A., and Holmes, S. P. (2016). DADA2: high-resolution sample inference from Illumina amplicon data. Nat. Methods 13:581. doi: 10.1038/nmeth.3869
Caminero, A., Nistal, E., Herrán, A. R., Pérez-Andrés, J., Ferrero, M. A., Ayala, L. V., et al. (2015). Differences in gluten metabolism among healthy volunteers, coeliac disease patients and first-degree relatives. Br. J. Nutr. 114, 1157–1167. doi: 10.1017/S0007114515002767
Coggon, D., Barker, D., and Rose, G. (2009). Epidemiology for the Uninitiated. Hoboken, NJ: John Wiley & Sons.
Couvigny, B., De Wouters, T., Kaci, G., Jacouton, E., Delorme, C., Doré, J., et al. (2015). Commensal Streptococcus salivarius modulates PPARγ transcriptional activity in human intestinal epithelial cells. PLoS One 10:e0125371. doi: 10.1371/journal.pone.0125371
Davis, N. M., Proctor, D. M., Holmes, S. P., Relman, D. A., and Callahan, B. J. (2018). Simple statistical identification and removal of contaminant sequences in marker-gene and metagenomics data. Microbiome 6:226. doi: 10.1186/s40168-018-0605-2
de Sousa Moraes, L. F., Grzeskowiak, L. M., de Sales Teixeira, T. F., and Peluzio, M. D. C. G. (2014). Intestinal microbiota and probiotics in celiac disease. Clin. Microbiol. Rev. 27, 482–489. doi: 10.1128/CMR.00106-13
Desai, M. S., Seekatz, A. M., Koropatkin, N. M., Kamada, N., Hickey, C. A., Wolter, M., et al. (2016). A dietary fiber-deprived gut microbiota degrades the colonic mucus barrier and enhances pathogen susceptibility. Cell 167, 1339.e21–1353.e21. doi: 10.1016/j.cell.2016.10.043
Domínguez-Bello, M. G., Pérez, M. E., Bortolini, M. C., Salzano, F. M., Pericchi, L. R., Zambrano-Guzman, O., et al. (2008). Amerindian Helicobacter pylori strains go extinct, as European strains expand their host range. PLoS One 3:e3307. doi: 10.1371/journal.pone.0003307
Dridi, B., Fardeau, M.-L., Ollivier, B., Raoult, D., and Drancourt, M. (2012). Methanomassiliicoccus luminyensis gen. nov., sp. nov., a methanogenic archaeon isolated from human faeces. Int. J. Syst. Evol. Microbiol. 62, 1902–1907. doi: 10.1099/ijs.0.033712-0
Engström, N., Sandberg, A.-S., and Scheers, N. (2015). Sourdough fermentation of wheat flour does not prevent the interaction of transglutaminase 2 with α2-gliadin or gluten. Nutrients 7, 2134–2144. doi: 10.3390/nu7042134
Fernandez-Feo, M., Wei, G., Blumenkranz, G., Dewhirst, F. E., Schuppan, D., Oppenheim, F. G., et al. (2013). The cultivable human oral gluten-degrading microbiome and its potential implications in coeliac disease and gluten sensitivity. Clin. Microbiol. Infect 19, E386–E394. doi: 10.1111/1469-0691.12249
Gorlas, A., Robert, C., Gimenez, G., Drancourt, M., and Raoult, D. (2012). Complete genome sequence of Methanomassiliicoccus luminyensis, the largest genome of a human-associated Archaea species. J. Bacteriol. 194, 4745–4745. doi: 10.1128/JB.00956-12
Iwai, S., Weinmaier, T., Schmidt, B. L., Albertson, D. G., Poloso, N. J., Dabbagh, K., et al. (2016). Piphillin: improved prediction of metagenomic content by direct inference from human microbiomes. PLoS One 11:e0166104. doi: 10.1371/journal.pone.0166104
Jabri, B., and Sollid, L. M. (2006). Mechanisms of disease: immunopathogenesis of celiac disease. Nat. Rev. Gastroenterol. Hepatol. 3:516. doi: 10.1038/ncpgasthep0582
Kagnoff, M. F. (2007). Celiac disease: pathogenesis of a model immunogenetic disease. J. Clin. Invest. 117, 41–49. doi: 10.1172/JCI30253
Larkin, M. A., Blackshields, G., Brown, N., Chenna, R., McGettigan, P. A., McWilliam, H., et al. (2007). Clustal W and Clustal X version 2.0. Bioinformatics 23, 2947–2948. doi: 10.1093/bioinformatics/btm404
Lebwohl, B., Blaser, M. J., Ludvigsson, J. F., Green, P. H., Rundle, A., Sonnenberg, A., et al. (2013). Decreased risk of celiac disease in patients with Helicobacter pylori colonization. Am. J. Epidemiol. 178, 1721–1730. doi: 10.1093/aje/kwt234
Lohi, S., Mustalahti, K., Kaukinen, K., Laurila, K., Collin, P., Rissanen, H., et al. (2007). Increasing prevalence of coeliac disease over time. Aliment. Pharmacol. Ther. 26, 1217–1225. doi: 10.1111/j.1365-2036.2007.03502.x
Lorenzo Pisarello, M. J., Vintiñi, E. O., González, S. N., Pagani, F., and Medina, M. S. (2014). Decrease in lactobacilli in the intestinal microbiota of celiac children with a gluten-free diet, and selection of potentially probiotic strains. Can. J. Microbiol. 61, 32–37. doi: 10.1139/cjm-2014-0472
Love, M. I., Huber, W., and Anders, S. (2014). Moderated estimation of fold change and dispersion for RNA-seq data with DESeq2. Genome Biol. 15:550. doi: 10.1186/s13059-014-0550-8
Makharia, G. K., Verma, A. K., Amarchand, R., Bhatnagar, S., Das, P., Goswami, A., et al. (2011). Prevalence of celiac disease in the northern part of India: a community based study. J. Gastroenterol. Hepatol. 26, 894–900. doi: 10.1111/j.1440-1746.2010.06606.x
Mårild, K., Ye, W., Lebwohl, B., Green, P. H., Blaser, M. J., Card, T., et al. (2013). Antibiotic exposure and the development of coeliac disease: a nationwide case–control study. BMC Gastroenterol. 13:109. doi: 10.1186/1471-230X-13-109
Martin, M. (2011). Cutadapt removes adapter sequences from high-throughput sequencing reads. EMBnet J. 17, 10–12. doi: 10.14806/ej.17.1.200
McCarville, J., Dong, J., Caminero, A., Bermudez-Brito, M., Jury, J., Murray, J., et al. (2017). A commensal Bifidobacterium longum strain improves gluten-related immunopathology in mice through expression of a serine protease inhibitor. Appl. Environ. Microbiol. doi: 10.1128/AEM.01323-17 [Epub ahead of print].
McMurdie, P. J., and Holmes, S. (2013). phyloseq: an R package for reproducible interactive analysis and graphics of microbiome census data. PLoS One 8:e61217. doi: 10.1371/journal.pone.0061217
Morrison, D. J., and Preston, T. (2016). Formation of short chain fatty acids by the gut microbiota and their impact on human metabolism. Gut Microbes 7, 189–200. doi: 10.1080/19490976.2015.1134082
Myléus, A., Ivarsson, A., Webb, C., Danielsson, L., Hernell, O., Högberg, L., et al. (2009). Celiac disease revealed in 3% of Swedish 12-year-olds born during an epidemic. J. Pediatr. Gastroenterol. Nutr. 49, 170–176. doi: 10.1097/MPG.0b013e31818c52cc
Nakatsu, G., Li, X., Zhou, H., Sheng, J., Wong, S. H., Wu, W. K. K., et al. (2015). Gut mucosal microbiome across stages of colorectal carcinogenesis. Nat. Commun. 6:8727. doi: 10.1038/ncomms9727
Oksanen, J., Blanchet, F. G., Kindt, R., Legendre, P., Minchin, P. R., O’hara, R. B., et al. (2011). vegan: Community Ecology Package. R Package Version 117–118.
Olivares, M., Walker, A. W., Capilla, A., Benítez-Páez, A., Palau, F., Parkhill, J., et al. (2018). Gut microbiota trajectory in early life may predict development of celiac disease. Microbiome 6:36. doi: 10.1186/s40168-018-0415-6
Orlando, A., Linsalata, M., Notarnicola, M., Tutino, V., and Russo, F. (2014). Lactobacillus GG restoration of the gliadin induced epithelial barrier disruption: the role of cellular polyamines. BMC Microbiol. 14:19. doi: 10.1186/1471-2180-14-19
Park, M.-S., Hill, C. M., Li, Y., Hardy, R. K., Khanna, H., Khang, Y.-H., et al. (2004). Catalytic properties of the PepQ prolidase from Escherichia coli. Arch. Biochem. Biophys. 429, 224–230. doi: 10.1016/j.abb.2004.06.022
Parks, D. H., Tyson, G. W., Hugenholtz, P., and Beiko, R. G. (2014). STAMP: statistical analysis of taxonomic and functional profiles. Bioinformatics 30, 3123–3124. doi: 10.1093/bioinformatics/btu494
Parte, A. C. (2013). LPSN—list of prokaryotic names with standing in nomenclature. Nucleic Acids Res. 42, D613–D616. doi: 10.1093/nar/gkt1111
Png, C. W., Lindén, S. K., Gilshenan, K. S., Zoetendal, E. G., McSweeney, C. S., Sly, L. I., et al. (2010). Mucolytic bacteria with increased prevalence in IBD mucosa augment in vitro utilization of mucin by other bacteria. Am. J. Gastroenterol. 105, 2420–2428. doi: 10.1038/ajg.2010.281
Qiu, Z., Yang, H., Rong, L., Ding, W., Chen, J., and Zhong, L. (2017). Targeted metagenome based analyses show gut microbial diversity of inflammatory bowel disease patients. Indian J. Microbiol. 57, 307–315. doi: 10.1007/s12088-017-0652-6
Ravcheev, D. A., and Thiele, I. (2017). Comparative genomic analysis of the human gut microbiome reveals a broad distribution of metabolic pathways for the degradation of host-synthetized mucin glycans and utilization of mucin-derived monosaccharides. Front. Genet. 8:111. doi: 10.3389/fgene.2017.00111
Rintala, A., Riikonen, I., Toivonen, A., Pietilä, S., Munukka, E., Pursiheimo, J.-P., et al. (2018). Early fecal microbiota composition in children who later develop celiac disease and associated autoimmunity. Scand. J. Gastroenterol. 53, 403–409. doi: 10.1080/00365521.2018.1444788
Ritari, J., Salojärvi, J., Lahti, L., and de Vos, W. M. (2015). Improved taxonomic assignment of human intestinal 16S rRNA sequences by a dedicated reference database. BMC Genomics 16:1056. doi: 10.1186/s12864-015-2265-y
Rivière, A., Selak, M., Lantin, D., Leroy, F., and De Vuyst, L. (2016). Bifidobacteria and butyrate-producing colon bacteria: importance and strategies for their stimulation in the human gut. Front. Microbiol. 7:979. doi: 10.3389/fmicb.2016.00979
Sánchez, E., Laparra, J., and Sanz, Y. (2012). Discerning the role of Bacteroides fragilis in celiac disease pathogenesis. Appl. Environ. Microbiol. 78, 6507–6515. doi: 10.1128/AEM.00563-12
Sanz, Y., Palma, G. D., and Laparra, M. (2011). Unraveling the ties between celiac disease and intestinal microbiota. Int. Rev. Immunol. 30, 207–218. doi: 10.3109/08830185.2011.599084
Schneeberger, M., Everard, A., Gómez-Valadés, A. G., Matamoros, S., Ramírez, S., Delzenne, N. M., et al. (2015). Akkermansia muciniphila inversely correlates with the onset of inflammation, altered adipose tissue metabolism and metabolic disorders during obesity in mice. Sci. Rep. 5:16643. doi: 10.1038/srep16643
Shetty, S. A., Hugenholtz, F., Lahti, L., Smidt, H., and de Vos, W. M. (2017). Intestinal microbiome landscaping: insight in community assemblage and implications for microbial modulation strategies. FEMS Microbiol. Rev. 41, 182–199. doi: 10.1093/femsre/fuw045
Singh, P., Arora, A., Strand, T. A., Leffler, D. A., Catassi, C., Green, P. H., et al. (2018). Global prevalence of celiac disease: systematic review and meta-analysis. Clin. Gastroenterol. Hepatol. 16, 823.e2–836.e2. doi: 10.1016/j.cgh.2017.06.037
Singh, P., Arora, S., Lal, S., Strand, T. A., and Makharia, G. K. (2015). Risk of celiac disease in the first-and second-degree relatives of patients with celiac disease: a systematic review and meta-analysis. Am. J. Gastroenterol. 110:1539. doi: 10.1038/ajg.2015.296
Sugano, K., Tack, J., Kuipers, E. J., Graham, D. Y., El-Omar, E. M., Miura, S., et al. (2015). Kyoto global consensus report on Helicobacter pylori gastritis. Gut 64, 1353–1367. doi: 10.1136/gutjnl-2015-309252
Talley, N. J., Goodsall, T., and Potter, M. (2017). Functional dyspepsia. Austr. Prescr. 40:209. doi: 10.18773/austprescr.2017.066
Tian, N., Faller, L., Leffler, D. A., Kelly, C. P., Hansen, J., Bosch, J. A., et al. (2017). Salivary gluten degradation and oral microbial profiles in health and celiac disease. Appl. Environ. Microbiol. 83:3330-16. doi: 10.1128/AEM.03330-16
Tjellström, B., Stenhammar, L., Högberg, L., Fälth-Magnusson, K., Magnusson, K.-E., Midtvedt, T., et al. (2007). Gut microflora associated characteristics in first-degree relatives of children with celiac disease. Scand. J. Gastroenterol. 42, 1204–1208. doi: 10.1080/00365520701320687
Van De Wal, Y., Kooy, Y. M., Van Veelen, P. A., Peña, S. A., Mearin, L. M., Molberg, Ø., et al. (1998). Small intestinal T cells of celiac disease patients recognize a natural pepsin fragment of gliadin. Proc. Natl. Acad. Sci. 95, 10050–10054. doi: 10.1073/pnas.95.17.10050
Verdu, E. F., Galipeau, H. J., and Jabri, B. (2015). Novel players in coeliac disease pathogenesis: role of the gut microbiota. Nat. Rev. Gastroenterol. Hepatol. 12:497. doi: 10.1038/nrgastro.2015.90
Villanacci, V., Bassotti, G., Liserre, B., Lanzini, A., Lanzarotto, F., and Genta, R. M. (2006). Helicobacter pylori infection in patients with celiac disease. Am. J. Gastroenterol. 101:1880.
Volta, U., and De Giorgio, R. (2012). New understanding of gluten sensitivity. Nat. Rev. Gastroenterol. Hepatol. 9:295. doi: 10.1038/nrgastro.2012.15
Walters, W., Hyde, E. R., Berg-Lyons, D., Ackermann, G., Humphrey, G., Parada, A., et al. (2016). Improved bacterial 16S rRNA gene (V4 and V4-5) and fungal internal transcribed spacer marker gene primers for microbial community surveys. mSystems 1:e00009-15. doi: 10.1128/mSystems.00009-15
Wickham, H. (2011). “ggplot2,” in Wiley Interdisciplinary Reviews: Computational Statistics, Vol. 3, eds J. E. Gentle and D. W. Scott (Hoboken, NJ: Wiley), 180–185. doi: 10.1002/wics.147
Woo, P. C.-Y., Fung, A. M.-Y., Lau, S. K.-P., Chan, B. Y.-L., Chiu, S.-K., Teng, J. L.-L., et al. (2003). Granulicatella adiacens and Abiotrophia defectiva bacteraemia characterized by 16S rRNA gene sequencing. J. Med. Microbiol. 52, 137–140. doi: 10.1099/jmm.0.04950-0
Ze, X., Duncan, S. H., Louis, P., and Flint, H. J. (2012). Ruminococcus bromii is a keystone species for the degradation of resistant starch in the human colon. ISME J. 6:1535. doi: 10.1038/ismej.2012.4
Keywords: celiac, gut microbiota, gluten, H. pylori, butyrate, duodenal microbiota
Citation: Bodkhe R, Shetty SA, Dhotre DP, Verma AK, Bhatia K, Mishra A, Kaur G, Pande P, Bangarusamy DK, Santosh BP, Perumal RC, Ahuja V, Shouche YS and Makharia GK (2019) Comparison of Small Gut and Whole Gut Microbiota of First-Degree Relatives With Adult Celiac Disease Patients and Controls. Front. Microbiol. 10:164. doi: 10.3389/fmicb.2019.00164
Received: 17 August 2018; Accepted: 22 January 2019;
Published: 08 February 2019.
Edited by:
Learn-Han Lee, Monash University Malaysia, MalaysiaReviewed by:
Steven Thomas Leach, University of New South Wales, AustraliaDaniel Martinez Garcia, EveryWhere Schools, Spain
Copyright © 2019 Bodkhe, Shetty, Dhotre, Verma, Bhatia, Mishra, Kaur, Pande, Bangarusamy, Santosh, Perumal, Ahuja, Shouche and Makharia. This is an open-access article distributed under the terms of the Creative Commons Attribution License (CC BY). The use, distribution or reproduction in other forums is permitted, provided the original author(s) and the copyright owner(s) are credited and that the original publication in this journal is cited, in accordance with accepted academic practice. No use, distribution or reproduction is permitted which does not comply with these terms.
*Correspondence: Yogesh S. Shouche, eW9nZXNoQG5jY3MucmVzLmlu Govind K. Makharia, Z292aW5kbWFraGFyaWFAZ21haWwuY29t
†These authors have contributed equally to this work
‡Present address: Sudarshan A. Shetty, Laboratory of Microbiology, Wageningen University and Research, Wageningen, Netherlands Anil K. Verma, Celiac Disease Research Laboratory, Department of Pediatrics, Università Politecnica delle Marche, Ancona, Italy