- 1Nutrition and Bromatology Group, Department of Analytical and Food Chemistry, Faculty of Food Science, University of Vigo, Ourense, Spain
- 2Department of Microbiology and Biochemistry of Dairy Products, Instituto de Productos Lácteos de Asturias, Consejo Superior de Investigaciones Científicas, Villaviciosa, Spain
- 3Department of Immunology, Hospital Universitario Central de Asturias, Oviedo, Spain
There are strong evidences that probiotics influence the immune status of the host, in a strain-specific manner, acting in the gastrointestinal tract. On the hypothesis that certain extracellular proteins and peptides from gut bacteria may mediate part of this immunomodulation and assuming they are able to diffuse through the mucus layer and interact with immune cells we have developed this work. Our study attempts to understand the immunomodulatory mechanisms of (i) Pext, the extracellular protein fraction of Lactobacillus acidophilus DSM20079T, (ii) HM14, a peptide encrypted in an extracellular glycoside hydrolase from Bifidobacterium longum NCIMB 8809 and (iii) Escherichia coli O111:B4 lipopolysaccharide (LPS), a well-known pro-inflammatory molecule, over human peripheral blood mononuclear cells (PBMCs). An untargeted LC-ESI-QTOF-MS metabolomics approach was applied to reveal intracellular changes in treated-PBMCs isolated from healthy donors. Differences in NADH arrest, NAD+ concentration reduction, as well as increases in palmitic acid and methanephrin were observed in HM14 and Pext treated-cells compared to those stimulated with LPS. This would support an anti-inflammatory molecular mechanism of action of such proteinaceous molecules. Moreover, this methodology has confirms the importance of metabolomics approaches to better understanding immune cell responses to gut bacterial-derived molecules.
Introduction
According to the Food and Agricultural Organization of the United Nations, probiotics are defined as “live microorganisms, which when administered in adequate amounts confer health benefits on the host” (FAO/WHO, 2002). Among the different traits that can be held by a probiotic strain, immunomodulation is widely reported (Holmes et al., 2012; Hemarajata and Versalovic, 2013; Quévrain et al., 2015) but at the same time is one of the most rare and strain-specific beneficial effects over host health (Hill et al., 2014). This immunomodulation mechanism, performed in the gastrointestinal tract, could be achieved through changes in cytokine production and modulation of signaling pathways in intestinal epithelial and immune cells (Hemarajata and Versalovic, 2013).
During the last years, we have worked on the hypothesis that certain extracellular proteins, and peptides derived from by the action of intestinal proteases, may mediate some of the immunomodulatory mechanisms of probiotic bacteria, as these molecules are able to diffuse through the mucus layer and interact with immune cells, notably in the last sections of the small intestine (Sanchez et al., 2010). The existence of an influence of probiotic intake over the immune status of the host is well-known, and as an example soluble factors from Lactobacillus reuteri inhibit production of pro-inflammatory cytokines and signaling immune cells Thomas et al. (2012). S-layer protein A (SlpA) produced by the probiotic Lactobacillus acidophilus NCFM strain is known to bind the surface lectin DC-SIGN, which is expressed on the surface of intestinal dendritic cells leading to an increase in the production of the anti-inflammatory cytokine IL-10 and an inability to induce further T-cell proliferation (Konstantinov et al., 2008). In this work, we have used the extracellular protein fraction of L. acidophilus DSM20079T (Pext). This fraction is characterized by containing a major protein, SlpA, which accounts for more than the 95% of the total protein production (Sánchez et al., 2011). SlpA abundance makes Pext a very suitable fraction for the study of the molecular mechanism of action of extracellular proteins. In addition, the proteolytic activity of this bacterium avoids interference of other proteins present in the bacteria growth media, some of them possessing also immunomodulatory properties such as porcine serpin (Urdaci and Sánchez, 2009).
Our own work has identified encrypted peptides which, when released by the action of intestinal proteases, interact with dendritic cells rendering an anti-inflammatory profile and modulating functional properties such as the capacity to imprint different home markers on the surface of T-cells (Bernardo et al., 2012). With this hypothesis in mind, we have generated a bioinformatics resource denominated MAHMI (Molecular mechanism of Action of the Human Microbiome) database (http://www.mahmi.org), which contains all the peptide generated by the in-silico action of intestinal proteases over the non-redundant protein catalog originated from the METAHIT project (Blanco-Míguez et al., 2017). This resource was queried against a knowledge database of bioactive proteins, allowing us to identify potential immunomodulatory peptides encrypted in larger proteins. This was the case of FR-16 and LR-17, 16-mer and 17-mer peptides derived from Bifidobacterium longum DJ010A and Bacteroides fragilis YCH46 respectively, which polarized human peripheral blood mononuclear cell (PBMCs) responses toward increases in the Th17/Th1 balance (Hidalgo-Cantabrana et al., 2017). The same resource allowed us to identify peptide HM14, encrypted in an extracellular glycoside hydrolase from B. longum and which was predicted as a potential anti-inflammatory peptide (unpublished results).
Metabolomics is an omics technique, which aim is the comprehensive analysis of the biological system's metabolome, identifying the wide variety of low molecular weight metabolites that are chemically transformed during metabolism (Patti et al., 2012). Moreover, metabolomics cell phenotyping, the identification of metabolic events, and the interpretation of cell responses to different signals is the correct approach of endo-metabolome (León et al., 2013). Untargeted metabolomic strategy is intended to detect as many known and unknown metabolites as possible in a biological sample through magnetic resonance (NMR) or mass spectrometry (MS) platforms (Shah et al., 2012). This approach provides the opportunity to observe changes when biological samples or clinical states are compared.
In this context, the present study attempts to evaluate changes in the intracellular metabolite composition of PBMCs exposed to different bacterial components by using a LC-EI-QTOF-MS methodology and an untargeted profiling approach. PBMCs exposed to lipopolysaccharide (LPS) from Escherichia coli O111:B4, a well-known pro-inflammatory molecule, and two probiotic-derived molecules: a peptide derived from an extracellular glycoside hydrolase from B. longum NCIMB 8809 (HM14) and the extracellular protein fraction from L. acidophilus DSM20079T (Pext), were submitted to this untargeted metabolomics approach to infer changes in the metabolic pathways. Main results are presented next.
Experimental
Chemicals, Solutions and Materials
Standards of DL-Norvaline, Succinic acid-2,2,3,3-d4 and trans-Cinnamic-d7 acid, used as surrogates, were purchased from Sigma Aldrich (Missouri, United States). Each standard was prepared at 100 mM in water or methanol depending on the solubility of the chemical. Surrogate solution and a mix of standards were prepared in methanol at 1.0 mM. These solutions were stored in amber flasks at −20°C. RPMI 1640 medium containing 2.0 × 10−3 M l-glutamine and HEPES (Lonza, Basilea, Switzerland) was supplemented with 10% fetal bovine serum (FBS) (ICN Flow; Costa Mesa, CA, United States) and antibiotics. Phosphate buffered saline (PBS) was purchased from Oxoid Limited (Hampshire, UK) and Ficoll-Paque Plus (Lymphoprep) from Nycomed (Oslo, Norway). Flow cytometry reagents: anti-CD4 (PerCP), anti-CD8 (PE), anti-CD19 (APC), anti-CD56 anti-CD16 (FITC) antibodies and 7-aminoactinomycin D (7-AAD) were purchased from eBioscience (San Diego, CA, USA). The bacterial peptide HM14 from B. longum NCIMB 8809 was synthesized at GeneCust facilities to >95% purity (Ellange, Luxemburg). The extracellular protein fraction from L. acidophilus DSM20079T, denominated Pext, was isolated from spent growth supernatant as described previously (Sánchez et al., 2009). Lipopolysaccharides (LPS) from Escherichia coli O111:B4 purified by phenol extraction was purchased from Sigma-Aldrich (Madrid, Spain).
Analytical grade C-45 nitrogen and helium was supplied by Carburos Metálicos (Vigo, Spain). Additional equipment included a TurboVap (LifeScience, Hopkinton, MA, United States), an ultrasonic bath (P-Selecta, Barcelona, Spain), an analytical precision scale (Sartorius, Madrid, Spain) and a vortex shaker (Heidolph, Barcelona, Spain). Disposables used were micropipettes (200–1000 μL) and injection vials (2.0 mL) furnished with screw caps and PTFE-lined butyl rubber septa and inserts (0.35 mL). The used solvents were high quality suitable to the chromatographic analysis: Acetone (CHROMASOLV for HPLC ≥99.8%), Methanol (CHROMASOLV for HPLC ≥99.9%), Chloroform (CHROMASOLV for HPLC ≥99.9%), Water (CHROMASOLV for HPLC ≥99.9%), Acetonitrile (CHROMASOLV for HPLC ≥99.9%).
Ethics approval for this study (reference code AGL2013-44039-R) was obtained from the Regional Ethics Committee for Clinical Research (Comité de Ética de la Investigación del Principado de Asturias) in compliance with the Declaration of Helsinki. Samples used in this study were obtained from anonymous donors from our blood donation system.
Peripheral Blood Mononuclear Cells Isolation
To analyse the metabolomics pattern induced by bacterial components in the human immune system, PBMCs were isolated from the buffy coat of 5 healthy donors obtained from the Community Center for Blood and Tissues of Asturias (Oviedo, Spain). In short, 5.0 mL of buffy were diluted with equal volume of PBS added on top of 5.0 mL Ficoll-Hypaque Plus for gradient separation. Cells were separated by centrifugation (1800 rpm, 30 min) with two further steps of washing with PBS; the first one at 1200 rpm, 10 min (to discard platelets) and second one at 1500 rpm, 5.0 min. Then, PBMCs were counted in Neubauer chamber and adjusted to 2.5 × 107 mL−1 in RPMI 1640 supplemented with 10 % FBS and antibiotics.
Co-cultivation of Peptides and PBMCs
The PBMCs were cultivated in flat bottom 12-wells microplates using 2.0 mL cell suspension at high density (2.5 × 107 cells mL−1 per well). Positive LPS (1.0 μg mL−1) and negative controls (no stimulus) were included for each donor. The peptide HM14 was added at a final concentration of 50 μg mL−1 (Hidalgo-Cantabrana et al., 2017). The Pext fraction from L. acidophilus DSM20079T was added at a final concentration of 10 μg mL−1, as the concentration giving the highest responses in our immunomodulatory tests (unpublished results). In addition, blank samples (with no cells) were included in the study (N = 5). The microplates were incubated for 2 days at 37°C with 5.0 % CO2.
Sample Preparation
Quenching Extraction
After 48 h culture of PBMCs cells were submitted to a quenching protocol to preserve metabolite levels of cells to avoid degradation. The importance of quenching metabolic processes during cell harvesting is widely reported in the literature (Dettmer et al., 2011; McNamara et al., 2012; León et al., 2013). Shortly, cells were transferred to ice and harvested (1500 rpm, 5.0 min, 4.0 °C). Supernatants were collected and stored at −80°C for further analysis. Cells were then resuspended for 5.0 min in 20 μL of cold surrogate standards solution. Then, 800 μL of a pre-cooled extraction solvent chloroform/methanol/water (1:3:1) was added, and cell suspension was incubated for 1.0 h, at 4.0°C in an oscillating stirrer. Subsequently, cells were transferred to cold micro centrifuge tubes and supernatants (containing intracellular metabolites) were harvested (13,000 g, 3.0 min, 4.0°C). Prior to injection, 400 μL of supernatants were reduced to dryness under gentle nitrogen stream, redissolved in 200 μL ACN:H2O (1:9, v/v) and, finally, transferred to vials for LC-QTOF-MS analysis. Figure 1 summarizes a diagram flow of the selected experimental protocol.
Quality Control Sample
Two different types of Quality Control (QC) samples have been employed (QC and QC surrogate). QC sample was made by pooling 45 μL from each of analyzed samples and surrogate QC sample (QC surrogate), by spiking with surrogate standards to the QC sample.
Flow Cytometry Analysis
Lymphocyte subsets from PBMCs were analyzed in a BD Accuri C6 flow cytometer (BD Biosciences, Allschwil, Switzerland) at starting point (0 h) and after 48 h incubation. Cells were labeled with human anti-CD4 (PerCP) antibody to target CD4+ T-lymphocytes, anti-CD8 (PE) for CD8+ T-lymphocytes, anti-CD19 (APC) for B-lymphocytes, anti-CD56 and anti-CD16 (FITC) for Natural killer (NK) cells. Also, after 48 h incubation, cells were stained 7-AAD to checked cell viability, as 7-AAD labeled DNA only if the cells are not alive. Briefly, 1.0 × 106 cells were collected into a flow cytometry tube and washed with PBS (1500 rpm, 5.0 min). Cell pellet was stained with human antibodies and 7-AAD for 20 min at 4.0°C. Then, cells were washed with 1.0 mL of PBS and resuspended in 200 μL of PBS for flow cytometry quantification.
Chromatography and Detection Technique
For the untargeted screening, an Agilent (Wilmington, DE) 1200 Series LC was used. The LC system was interfaced to a quadrupole-time-of-flight (QTOF) HRMS model Agilent 6520 equipped with a Dual electrospray (ESI) ion source, operating in positive ion mode. Nitrogen was used as nebulizing (42 psig) and drying gas (350°C, 5.0 L min−1) in the dual ESI source. The QTOF instrument was operated in extended dynamic range 2.0 GHz mode. This mode provides a full width at half-maximum (fwhm) resolution of ca. 10600 at m/z 118.0862 and ca. 16900 at m/z 922.0097. A reference calibration solution, supplied by Agilent, was continuously sprayed in the source during the chromatographic run, providing the required accuracy of mass assignations. Chromatographic separations were performed with a Kinetex™ 2.6 μm C18 (100 Å, LC Column 100 × 2.1 mm) and with a Kinetex™ 2.6 μm Polar C18 (100 Å, LC Column 100 × 2.1 mm; Phenomenex, Torrance, CA, United States). The optimal working conditions were obtained with the Polar C18, in which the solvents consisted of A: 0.10% HCOOH in H2O and B: 0.10% HCOOH in acetonitrile. The gradient was: 100% A during 2.0 min, change to 100% B in 18 min and hold during 5.0 min, change to initial conditions in 0.10 min and hold for 10 min giving an analysis time of 35 min at 35°C. The injection volume was set to 5.0 μL by LC flow rate of 0.20 mL min−1. Adjustment and operation of the instrument were performed by means of MassHunter Workstation Software B.07.00.
Data Processing and Statistical Analysis
The resulting raw data files (.d files) were processed using MassHunter Profinder Software B.08.00 (Agilent Technologies) with Batch Recursive Feature Extraction (BRFE) algorithm. This option includes two steps: firstly, performs chromatographic deconvolution of samples to find features (compounds) through an untargeted Molecular Feature Extraction (MFE) algorithm. It finds co-eluting ions that are related such as isotopes, common adducts ([M+H]+, [M+Na]+, [M+K]+) and dimmers, besides filtering noise and create a compound chromatogram for the group of ions, unifying all these ion signals into one value, so that one feature is equivalent to one compound. Followed by mass and retention time alignment of molecular features across all of the selected samples. Last, Find by Ion feature extraction (FbI) algorithm uses the median values derived from the MFE step to perform a targeted extraction to improve the reliability in finding features. In this way, it improves the accuracy of the feature extraction by reducing the number of false negatives and false positives in the dataset. The MFE parameters included for feature extraction a minimum peak height of 150 counts, a tolerance window for compound binning and alignment of 0.50 min for retention time and a mass accuracy of 20 ppm for m/z values. FbI parameters for peak filtering were set a minimum absolute height at 1,000 counts.
Extracted compounds, comprised of a neutral mass, retention time, and abundance, were exported as compound exchange files (.CEF) for further feature alignment, data processing and multivariate statistical analysis using Mass Profiler Professional B.14.08 (Agilent Technologies). Data were filtered with a retention time tolerance of 0.15 min and mass tolerance of 2.0 mDa. One way ANOVA and Tukey's honest significance difference (HSD) post hoc test were applied to identify which entities were responsible for significant differences between conditions (p-value < 0.001). Compounds differing by a fold change >2 between groups were considered for putative identification.
Multivariate statistical analysis was also performed for comparative metabolite profiling. Unsupervised methods as Principal component analysis (PCA) and hierarchical cluster analysis (HCA) were used for data exploration and visualization, providing information about the presence of outliers, sample dispersion and data clustering. Samples were classified into discrete classes also by supervised Partial Least Square Discriminant Analysis (PLS-DA).
Metabolite Identification and Pathway Analysis Interpretation
Statistically significant metabolites selected by MPP were putatively identified through MassHunter METLIN Metabolite PCDL (Agilent Technologies). In case of unknown metabolites, molecular formulas were generated by the software. Mass accuracy tolerance of 5.0 ppm was used as mass window for database search. Also, other freely available online databases were employed as the Human Metabolome database (HMDB; http://hmdb.ca/), METLIN database (http://metlin.scripps.edu/) and KEGG database (http://www.genome.jp/kegg).
Biological interpretation was performed through the Pathway analysis tool of MPP software that allows searching the annotated metabolites in the curated database of human metabolic pathways BioCyc (http://www.biocyc.org/).
Results and Discussion
Lymphocyte Quantification and Cell Viability
Total lymphocytes and lymphocytes subpopulations were quantified at the initial point of the experiments and after 48 h incubation, as depicted for one representative donor in Figure 2A. Results showed the percentage of positive cells for each subset population. As expected, total lymphocytes (Figure 2A, first plot) were similar between the five healthy donors at initial point (0.0 h) (75.78% ± 4.31) and remained stable after 48 h incubation (72.53% ± 10.85). The different lymphocytes subsets, quantified with human antibodies labeling, remained stable after 48 h incubation in each case of study. The average (mean ± SD) of each lymphocytes subset referred to the lymphocytes gate was 13% ± 3,85 NK cells, 5.5% ± 1.43 B-lymphocytes, 45% ± 8.88 CD4 + T cells, 30.10% ± 7.07 CD8 + T cells, as depicted for one representative in Figure 2A.
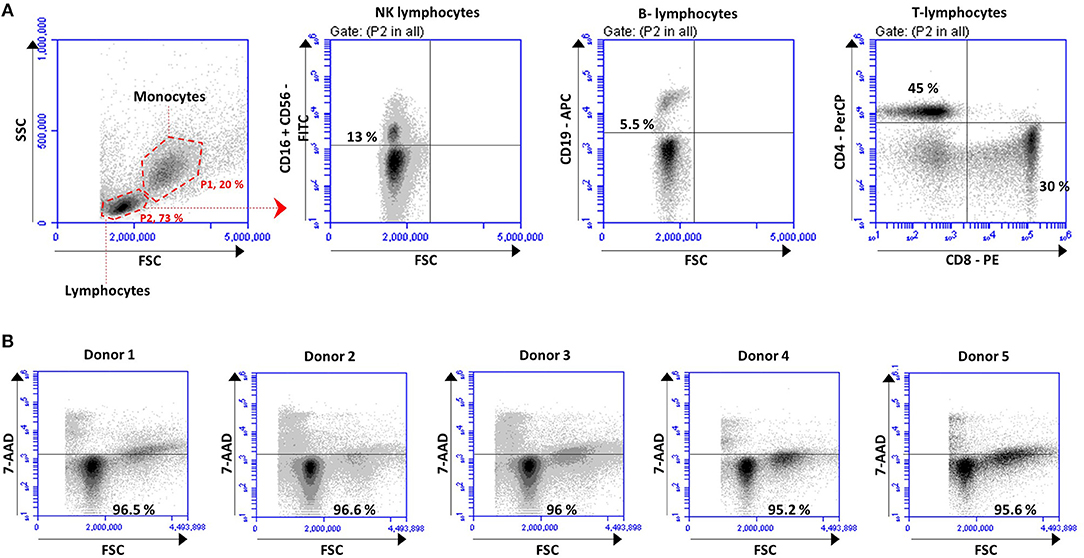
Figure 2. PBMCs isolated from the buffy of 5 healthy donors were analyzed before and after co-culture for 48 h to (A) quantified lymphocyte subsets and (B) cell viability. (A) Gating strategy used to identified monocytes (P1, 20%), lymphocytes (P2, 73%) and to quantified the different lymphocytes subsets: NK lymphocytes (CD16+ CD56+), B-lymphocytes (CD19+), CD4 T-lymphocytes (CD4+) and CD8 T-lymphocytes (CD8+). Density plots correspond to one representative donor. The percentage of positive cells in each subset is expressed based on the gate of lymphocytes (P2). (B) Cell viability analyses performed after 48 h based on the percentage of cells of each donor not labeled with 7-AAD.
Cell viability was measured by flow cytometry based on 7-AAD labeling (Figure 2B). The cell viability, quantified as the percentage of cells not labeled with 7-AAD, remained very high (96.08% ± 0.64) after 48 h incubation with no differences between the five donors (Figure 2B).
All in common established that lymphocytes subsets distribution and cell viability were not affected by high the number of cells assayed in each well, neither by the potential effect of the HM14 bacterial peptide from B. longum, Pext extracellular protein from L. acidophilus or LPS, at least during the incubation time tested. So on, it is expected that PBMCs metabolism remain closed to natural conditions, as closed as in vitro test support, and differences in the metabolism pattern will be directly related with the immune modulation capability of the studied stimuli.
Non-targeted Analytical Method
The seven parameters established by the international guidelines for analytical method validation such as accuracy, precision, specificity, limit of detection and quantification, linearity and range (US FDA, 2000, 2015; Thompson et al., 2002; ICH Q2A, 2005) are not adjusted to the aim of the non-targeted metabolomic approach. For this reason, researchers have proposed various strategies to validate the analytical methodology for untargeted metabolomics (Naz et al., 2014) and they are as follows: to validate the method in terms of accuracy and precision for selected compounds or spiked standards, the use of pooled QCs and analytical blanks during method performance. It is of great importance the employment of QC samples in metabolic profiling analysis, as well-described in the literature (Sangster et al., 2006). The idea of using QC is to have an appropriate representative sample for the matrix to be analyzed in a regular intervals throughout the analytical run in order to monitor the quality of the resulting data (Gika et al., 2016). Aware of this need, the latest guideline for Bioanalytical method validation includes QCs as bioanalytical parameters for method validation (US FDA, 2018).
Therefore, taking into account all the indications described above, was performed the methodological validation of the present study.
Data Quality Assessment
Recoveries of surrogate standards were measured in all samples ranged from 68 to 120% with relative standard deviations < 20%.
QC samples were analyzed intermittently during the analytical run every 5 samples and the acquired data was used to determine the experiment precision. Accordingly, RSDs for spiked-standards QC samples (QC surrogate) were calculated and resulted in all cases < 10%. It was reported that data should exhibit RSD lower than 20% in LC-MS analysis. Besides check that no sensitivity loss across the analytical batch was observed, it is necessary to also check for retention time precision, which was evaluated through visual inspection of overlapped Total Ion Chromatograms (TICs) of both spiked QC and QC pooled replicates.
Multivariate analysis on QC samples also provides information about the quality of the assay. PCA score plot shows that QC and QC surrogate replicates form a tighter cluster than the rest of samples, reflecting the instrumental repeatability. Note that although QC and QC surrogate samples appears to be not as close as we would expect on the PCA score plot, hierarchical clustering algorithm shows a clear relationship between both groups.
Moreover, two types of blanks were used for method quality assessment. Blanks for quenching protocol assessment (BQuench samples) and analytical blanks, which contain the injection solvent. Analytical blanks allow controlling the correct method development and identifying background features. And, on the other hand, BQuench samples allow to identify the background related to the entire methodological process from quenching to injection.
LC-QTOF -MS Method Development
Different chromatographic columns (Kinetex™ 2.6 μm C18 (100 Å, LC Column 100 × 2.1 mm) and Kinetex™ 2.6 μm Polar C18 (100 Å, LC Column 100 × 2.1 mm) (both packed with core-shell materials) and mobile phases were tested in order to study the retention of both polar and nonpolar substances. Since, reverse phase liquid chromatography (RPLC) is used for the detection of nonpolar compounds, a Kinetex Polar C18 was used in order to retain mixtures of multiple polar and nonpolar compounds. Moreover, they were also able to isolate closely related compounds, such as impurities or metabolites. A generic gradient was selected in order to identify most symmetric and intense peaks with a high resolution. Mobile phases A consisted in 5.0 mM NH4Ac with 0.10 % HCOOH or 0.10 % HCOOH were tested, as well as, methanol or acetonitrile as mobile phases B. Since no differences were detected using methanol as modifier with NH4Ac to prevent secondary retention mechanism, acetonitrile and HCOOH were selected as mobile phases in order to achieve more retention with polar compounds. In this work, QC surrogates were used for such purposes. Kinetex™ 2.6 μm Polar C18 was selected as working column because it gave the best chromatographic separation with a good peak shape. Flow rate of 0.20 mL/min, column temperature of 35°C and injection volume of 5.0 μL were also optimized.
Data Pre-processing, Statistical Analysis and Metabolite Identification
After data processing by MPP, 1155 of 2976 entities among groups were significantly differentiated after applying filtration using frequency (entities that appear in the 100 % of samples in at least one condition), p-value < 0.001 and fold change > 2.0.
Tukey's honest significance difference (HSD) post hoc test was applied to identify which entities were responsible for significant differences in the five groups (Pext, HM14, LPS, Blank, and Basal). In order to present data in easy-to-understand visualization, a Venn diagram is given to show all possible logical relations between the four groups (Pext, HM14, LPS, and Blank) regarding to Basal condition (Figure 3). It was found that the number of entities responsible for the differences among treatment groups and control were 302 for Pext, 228 for HM14 and 271 for LPS.
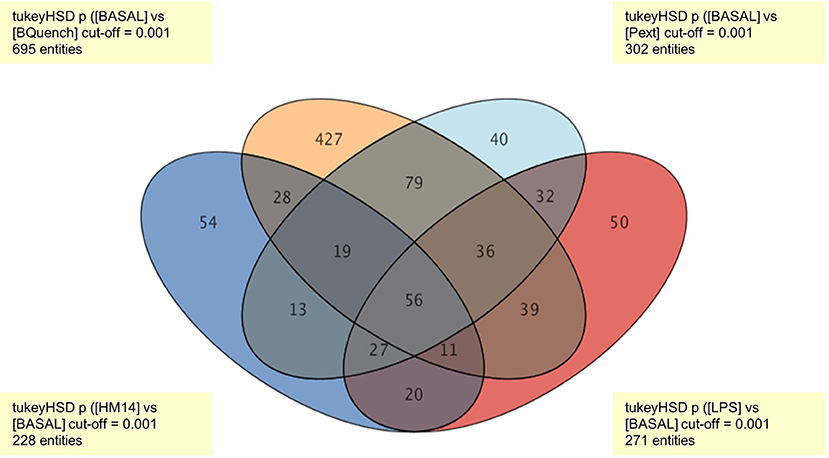
Figure 3. Venn diagram representing all relationships between the four groups (Pext, HM14, LPS, and Blank) in comparison to Basal conditions. The number of entities responsible for the differences between treatment groups and control were 302 for Pext, 228 for HM14 and 271 for LPS.
For multivariate analysis, about 56% of the total variance in the data was represented by the three first principal components in the PCA (Figure 4A). The 3D-PCA score plot represent each sample as a single point. No outlier samples were observed and the data showed a grouped distribution, revealing a remarkable difference between conditions. Furthermore, some lightly similarity among Basal condition and HM14 samples can be observed and both can be distinguished from LPS and Pext conditions, which seem to be also related one to each other. The cluster of QC samples provides a reliable stability and repeatability of the analysis sequence. In order to maximize the separation achieved with the PCA, a supervised analysis PLS-DA (Figure 4B) was subsequently performed. In the PLS-DA score plot, samples exhibited lower dispersion within the group, emphasizing the separation between the different clusters with an overall accuracy of the model of 94.828% (Figure 4C), R2 = 0.657 and Q2 = 0.478. Hierarchical clustering analysis was also performed by applying Pearson's Center-Absolute similarity measure and complete linkage, to produce a dendogram for clustering of sample groups using normalized intensities values of the processed data (Figure 5). Similar results to those explained in the PCA and PLS-DA are observed in the dendogram, indicated close clustering between HM14-Basal, and LPS-Pext. The dendogram represents a measure of dissimilarity with the length of the vertical lines and, therefore, provides an insight into the existing relationship among groups. Clustering the seven groups or conditions in six classes. Class I shows that BQuench group is the most dissimilar from the other groups with a dissimilarity level of 0.985. In class II is represented the dissimilarity between QC groups (SurroQC and QC) and the rest of treatment groups and control (class IV), with a dissimilarity level of 0.881, showing a reasonable difference with respect to class IV. Being the dissimilarity level among SurroQC and QC of 0.608, clustered together in class III. Most relevant conclusions were obtained from clustering observed in class IV, which explains dissimilarity between class V (clustering P14 and LPS groups with a dissimilarity level of 0.470) and class VI (clustering HM14 and Basal group with a dissimilarity level of 0.457) with 0.565.
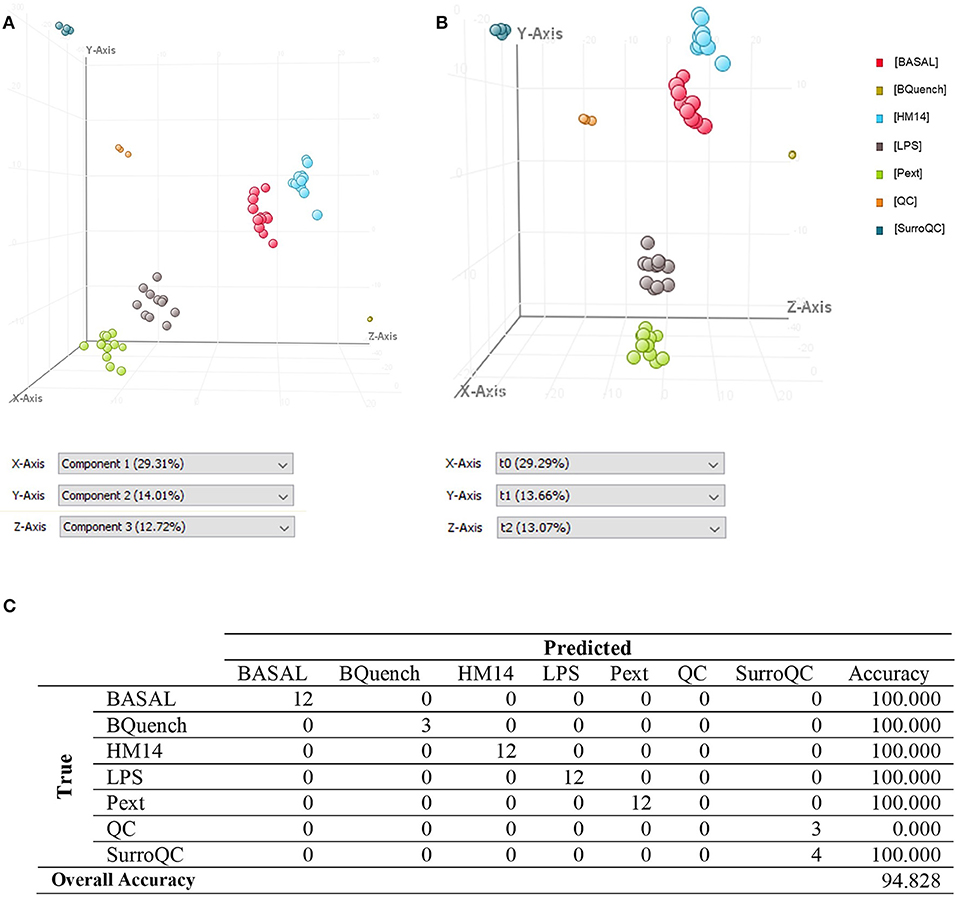
Figure 4. (A) Three-dimensional PCA score plot, (B) PLS-DA score plot and (C) Confusion matrix of the PLSDA model.
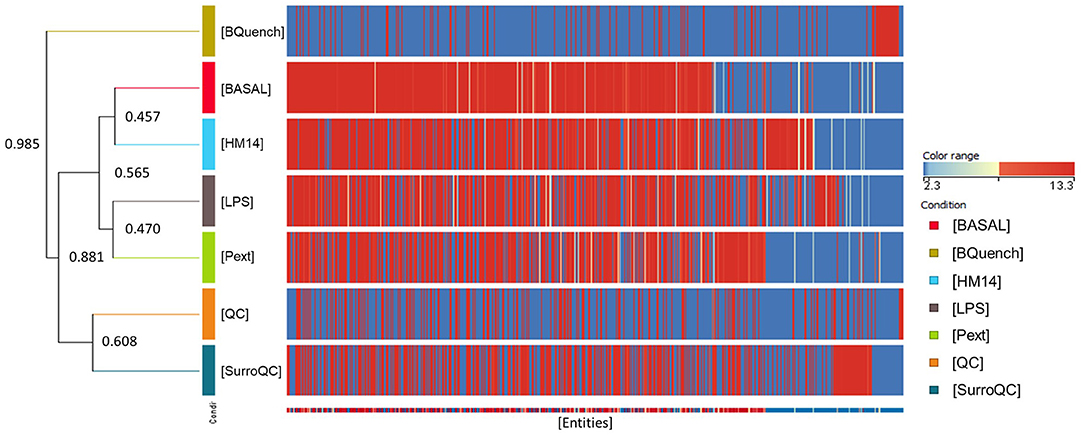
Figure 5. Comparison of seven groups of samples using normalized intensities of annotated metabolites. The dendogram was produced by applying a hierarchical clustering algorithm.
Pathway Analysis
Pathway analysis was performed in MPP software from significantly differentiated metabolites. Those that have not been identified were excluded from this analysis by the software. Finally, a total of 50 annotated metabolites, named as compounds and classified in a total of 13 entities, were found related with different metabolic pathways included in the BioCyc database (Table 1 and Supplementary Table 1).
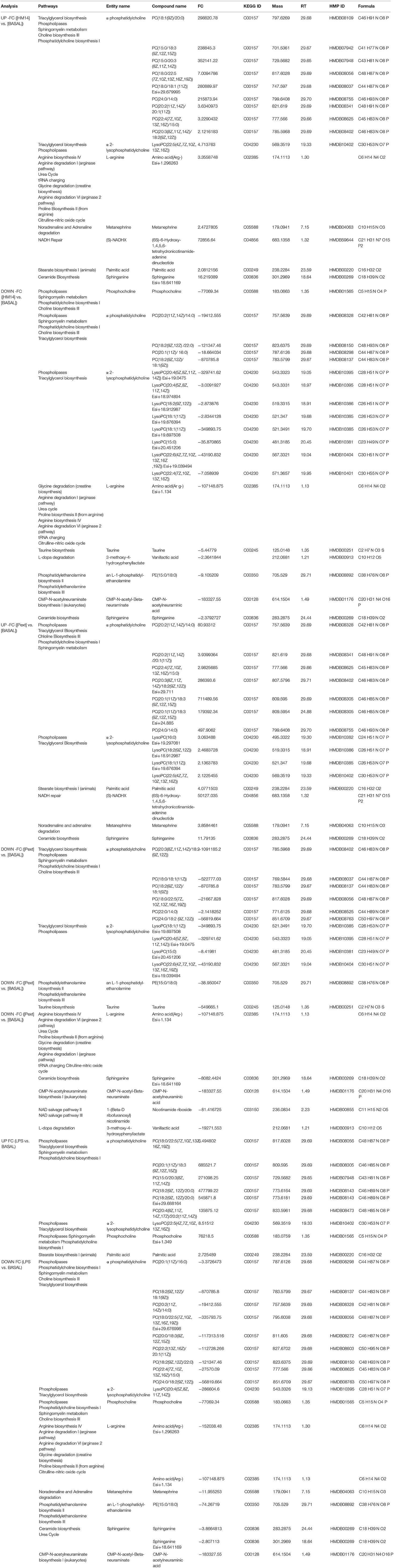
Table 1. List of 50 putatively identified metabolites after pathway analysis, which were classified in 13 entities, showing related metabolic pathways.
Entities or compound classes that have been found were: α-phosphatidylcholine (PC), α-2-lysophosphatidylcholine (LysoPC), L-arginine (Arg(-)), metanephrine, (S)-NADHX, palmitic acid, sphinganine, phosphocholine, taurine, 3-methoxy-4-hydroxyphenyllactate, an-L-1-phosphatidyl-ethanolamine (PE), CMP-N-acetyl-β-neuraminate (CMP-Neu5Ac) and 1-(β-D ribofuranosyl)nicotinamide. Among metabolic pathways, in which these entities are involved, were found representatives of lipid metabolism such as phospholipases metabolism (PC, LysoPC and phosphocholine) and biosynthetic pathways of triacylglycerol (PC and LysoPC), sphingomyelin, choline, phosphatidylcholine (PC and phosphocholine), phosphatidylethanolamine (PE), ceramide (sphinganine), and stearate (palmitic acid). Amino acid metabolism pathways such as arginine metabolism, urea and citrulline-nitric oxide cycle, proline biosynthesis, glycine degradation, tRNA charging (L-Arg(-)) and taurine biosynthesis (taurine). Representatives of catecholamine metabolism such as L-dopamine (3-methoxy-4-hydroxyphenyllactate), noradrenaline and adrenaline (metanephrine) degradation. In addition, entities involved in NAD salvage (1-(β-D ribofuranosyl) nicotinamide), NADH repair ((S)-NADHX) and CMP-N-acetylneuraminate biosynthesis (CMP-Neu5Ac) pathways were also found related with this study.
Common Metabolomics Response to the Different Microbiota-Derived Molecules
Results interpretation was quite complex as some of the entities were found both over and underproduced after incubation with either LPS, peptide HM14 or Pext, such as α-phosphatidylcholine, 2-lysophosphatidylcholine, L-arginine or sphinganine. For instance, in the case of sphinganine entity, two compounds were putatively identified, sphinganine Esi+18.641169 (302.2969 m/z; 18.64 min) and sphinganine (284.2875 m/z; 24.44 min). As it has been reported by (Mi et al., 2016), fragment at 284.3 m/z can result from the fragmentation of both protonated adducts of sphinganine, as a result of the dehydration of their protonated molecular ion, and sphinganine-1-phosphate, through loss of phosphoric acid from the molecular structure, in positive ion mode. Since sphinganine-1-phosphate elutes later than sphinganine, this suggest that sphinganine (284.2875 m/z; 24.440 min) could come from the phosphorylated form and therefore, lead to the identification of two different compounds with the same value of m/z and different retention time that appears both over and under-expressed within the same condition.
Another example is phosphocholine, head group of one class of phospholipids, with a predominant fragment at 184 m/z, which could be produced by the fragmentation, in positive ion mode, of protonated adducts of phosphatidylcholines and sphingomyelins (Jackson et al., 2005). This could explain the reason why two molecular ions of phosphocholine, phosphocholine (183.0663 m/z; 1.35 min) and phosphocholine Esi+1.349 (183.0759 m/z; 1.35 min), were found both in over and underproduction in LPS condition, being the response to HM14 an overproduction of the compound phosphocholine in contrast to the response observed against LPS.
L-arginine was also found both up- and down-regulated by HM14, through the ionic forms of Amino acid(Arg-) Esi+1.134 (174.1113 m/z; 1.13 min) and Amino acid(Arg-) Esi+1.296263 (174.1113 m/z; 1.30 min). After a visual inspection of the chromatogram, due to the peak form, it could be possible that both ionic forms belonged to the same molecular structure. Moreover, although the software identified the entity name as L-arginine, D-arginine could also be present and this fact could explain the peak form as well (Han et al., 2018). Thus, further quantitative analysis by MS/MS is needed to identify this compound and to know the magnitude of the change and therefore, pathways involved (such as arginine biosynthesis/degradation) will not be discussed at this time. However, it is noteworthy that Pext only down-regulated the concentration of this amino acid, suggesting that Pext may be interacting through different mechanisms with the immune cell populations. Therefore, as the implication of different ionic forms corresponding to the same molecules requires a more exhaustive understanding of the metabolic response of the different immune cells to the molecules involved in this work, the metabolic pathways corresponding to these compounds will not be evaluated at this time. Further experimentation with cellular subsets may identify the immune populations, in which a given pathway is up or down-regulated. As well as performing a targeted MS/MS analysis, it will be possible to elucidate fragmentation patterns that allow the identification of each molecular structure.
Among the entities that showed down-regulation patterns as affected by HM14, Pext and LPS we identified CMP-Neu5Ac and L-1-phosphatidyl-ethanolamine. CMP-Neu5Ac is the activated form of 5-N-Acetylneuraminic acid (Neu5Ac), the major sialic acid found in animal cell surfaces (Li and Chen, 2012). Alterations on cell surface glycoconjugates by removal of terminal sialic acids residues by sialidases influence cellular activity. (Nan et al., 2007) showed an association of desialylation of specific glycoconjugates on the cell surface of activated human T lymphocytes, and suggested a correlation between sialidase inhibition and decreases in the production of IFN-γ (Stamatos et al., 2004). Regarding PE, it is the second most abundant lipid on cellular membranes and plays an important role in membrane fusion, cell cycle, autophagy and apoptosis (Pavlovic and Bakovic, 2013). It can be de novo synthesized from ethanolamine, which plays a significant role in modulating intestinal inflammation and in the proliferation and differentiation of intestinal cells (Zhou et al., 2017). (Martins et al., 2016) also reported decreased PE concentrations in THP-1 cells exposed to methyl salicylate, a respiratory irritant chemical. Decreases in these two metabolites may represent common response pathways to conserved microbial-associated molecules.
A deeper analysis into what metabolites increased/decreased their intracellular concentrations following challenges with LPS, HM14 peptide or Pext fraction, revealed interesting patterns. Figure 6 represents Venn diagrams of over- and under-produced metabolites, always referred to basal conditions, in the different experimental settings as well as their intersections. It is noteworthy the amount of metabolites downregulated by the three bacterial molecules: L-1-phosphatidyl-ethanolamine, phosphatidylcholine, 2-lysophosphatidylcholine, CMP-N-acetyl-β-neuraminate, L-arginine and sphinganine. This suggests some kind of central response to commensal bacteria components, regardless their biochemical nature (proteic or lipoglucidic) or if they are originated from a potential pathogen (E. coli) or a potential probiotic (L. acidophilus). The boundary between both bacterial features (probiotic vs. pathogen) is somehow diffuse and strain-dependent, and we can find either E. coli probiotic strains such as strain Nissle 1917 (Henker et al., 2008), or Lactobacillus sp. strains (Martinez et al., 2014) contributing to infections.
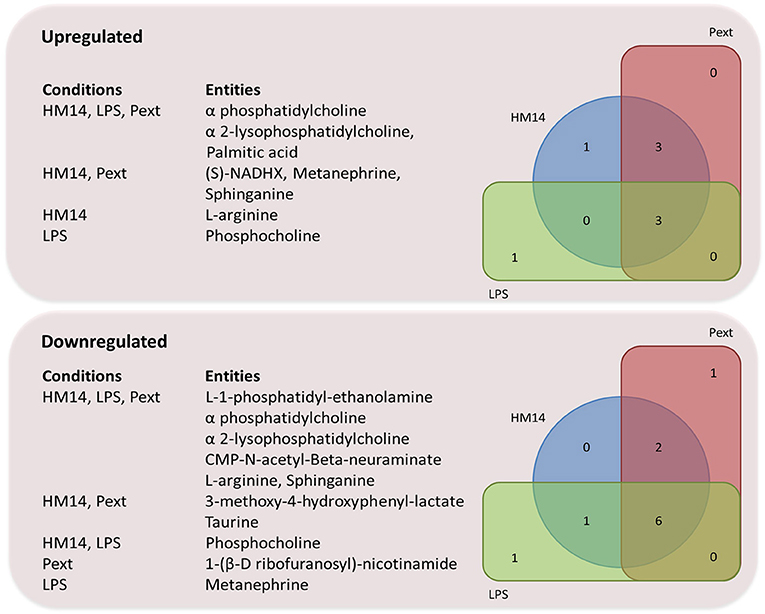
Figure 6. Venn diagram representing over-and under-produced entities, referred to basal conditions, after MPP pathway analysis.
Specific HM14 and Pext Immunological Effects
It is noteworthy that both intestinal bacteria derived molecules, HM14 and Pext, induced metabolic responses that somehow diverged from E. coli LPS. A schematic representation of the possible metabolic pathways in which these molecules may be involved is shown in Figure 7. Among the compounds upregulated specifically by the presence of peptide HM14 we found palmitic acid, which participates in the stearate biosynthesis I pathway. Palmitic acid is by far the most abundant saturated fatty acid in living beings, but it is well-known in our society by being the major component of palm oil, being present in a wide array of food products. This long chain fatty acid interacts with toll-like receptor 4 (TLR4) expressing cells, such as dendritic cells (Nicholas et al., 2017). Palmitic acid also binds to the TLR4 accessory protein MD2 and triggers pro-inflammatory responses, both in vivo (mouse model) and in vitro, being the mechanisms by which circulating palmitic acid induces myocardial injury (Wang et al., 2017). Indeed md2 -/- mice are protected against the pro-inflammatory effects under a high-fat diet. A recent report has indicated that either a palmitic acid-rich diet or injection supressed arthritis in a mouse model by modulating directly the function of invariant natural killer T (iNKT) cells, being able to decrease antibody-induced joint inflammation. This was elicited through an inhibition of the pro-inflammatory mediators IL-4 and IFN-γ and a reduction in the level of the transcription of the gata-3 and t-bet genes, after stimulation in the T-cell receptor (Ko et al., 2017).
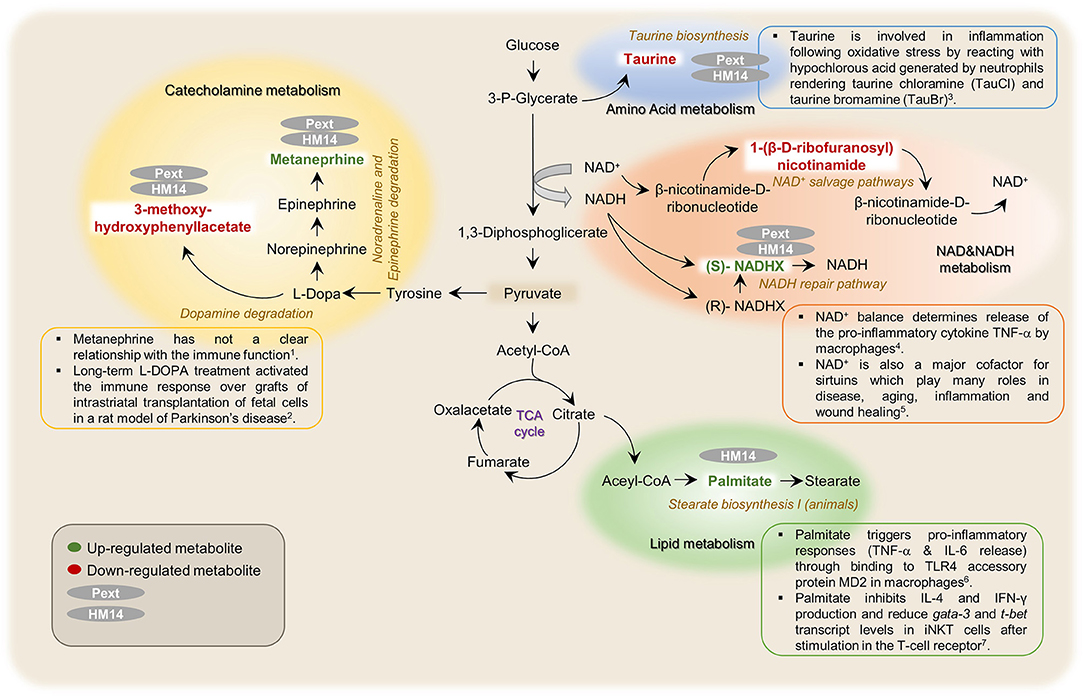
Figure 7. Schematic representation of metabolic pathways that were found related with the possible immunomodulatory effects induced by the studied proteinaceous molecules (HM14 and Pext) over PBMCs. 1(Breger et al., 2017), 2(Marcinkiewicz and Kontny, 2014), 3((Al-Shabany et al., 2016), 4(Qiang et al., 2017), 5(Wang et al., 2017), and 6(Ko et al., 2017).
HM14 and Pext increased the intracellular concentrations of (S)-NADHX, an enantiomer of the NADH hydrate formed after glyceraldehyde 3-phosphate dehydrogenase action, which is bioconverted back to NADH by the action of an ATP-dependent NAD(P)H dehydratase. As a whole, these reactions form part of the so-called NADH repair pathway. NADH is the reduced form of the coenzyme nicotinamide adenine dinucleotide, a well-known electron carrier in redox reactions. NAD+ balance determines release of the pro-inflammatory cytokine TNF-α by macrophages in response to LPS, which signals through TLR4 (Al-Shabany et al., 2016). Interestingly, LPS did not affect the NADHX levels in our experimental setting. NAD+ is also a major cofactor for sirtuins, which are a family of 7 proteins with deacetylase and ADP ribosyltransferase activities. These proteins play many roles in disease, aging, inflammation and wound healing (Qiang et al., 2017). Finally, NAD+ has also been identified as an extracellular signaling molecule, being for instance released by neurons in the large intestine with inhibitory activity over the smooth muscle surrounding the intestinal mucosa (Mutafova-Yambolieva et al., 2007; Hwang et al., 2011).
Both HM14 and Pext increased also the concentration of metanephrine, a metabolite from the noradrenaline/adrenaline degradation. This molecule has not a clear relationship with the immune function, but higher plasma levels have been correlated to a high risk of prediabetes, and it has been also proposed as a biomarker for pheochromocytoma, a type of neuroendocrine tumor affecting the adrenal glands (Lenders et al., 2002; Wang et al., 2015). Interestingly, the effect of LPS was inverse as this pro-inflammatory compound decreased the levels of metanephrine. Therefore, unless requiring more experimental evidence, this might be interpreted as an anti-inflammatory effect as being opposite to what is observed for LPS.
Regarding the metabolites downregulated by the incubation of HM14 and Pext over PBMCs, our analysis identified 3-methoxy-4-hydroxyphenyl-lactate involved in L-DOPA degradation and the amino acid taurine. L-DOPA is the metabolic precursor of dopamine and it is among the most effective treatments for Parkinson's disease. In a recent paper, it has been observed that long-term L-DOPA treatment activated the immune response over both allogeneic and xenogeneic grafts of intrastriatal transplantation of fetal cells, even in the presence of the immunesupressor cefalosporine (Breger et al., 2017). In the same way, it has been suggested a role for taurine in inflammation following oxidative stress by reacting with hypochlorous acid generated by neutrophils rendering taurine chloramine (TauCl) and taurine bromamine (TauBr) (Marcinkiewicz and Kontny, 2014). Therefore, an anti-inflammatory role can be proposed to both molecules that will deserve further experimentation.
Finally, Pext decreased the concentration of 1-(β-D ribofuranosyl)-nicotinamide in PBMCs, a metabolite involved in the NAD salvage pathway II and III. As pointed out before, NAD+ is a central coenzyme for energy metabolism, cell growth and many others housekeeping pathways. Inhibition of this pathway and therefore the NAD+ levels combined with many cancer treatments returned often higher cytotoxicity over cancer cells (Kennedy et al., 2016).
Conclusions
In conclusion, an untargeted metabolic fingerprinting was performed in order to evaluate intracellular changes in PBMCs, in the presence of proteinaceous molecules derived from gut bacteria and a pro-inflammatory endotoxin, by using LC-QTOF-MS. This, reinforces the importance of metabolomics approaches to better understanding immune cell response. Results suggest that HM14 and Pext, a peptide derived from an extracellular protein of B. longum and the extracellular protein fraction of L. acidophilus, induced metabolic responses in PBMCs that diverged from those observed for the pro-inflammatory E. coli LPS. NADH arrest, NAD+ reduction, as well as increases in palmitic acid and methanephrin, would support an anti-inflammatory molecular mechanism of action of these two molecules, although this will require further studies using human translational models for inflammation: skin patch.
Author Contributions
BS and EM-C conceived the experiments. RA-A and MM-G designed the immunological study. NC-P, CH-C, and MM-G performed biological experiments. NC-P, EM-C, and JS-G performed metabolomics analysis. NC-P, EM-C, BS, and JS-G wrote the manuscript. The manuscript was reviewed by all the authors.
Funding
This work was funded by the Autonomic Investigadores Emerxentes do Sistema Universitario de Galicia (Grant EM2014/046) and the Spanish Programa Estatal de Investigación, Desarrollo e Innovación Orientada a los Retos de la Sociedad (Grant AGL2013-44039R). This work has received also financial support from the Xunta de Galicia (Plan de mellora do Centro de Investigacións Agroalimentarias CIA3 do Campus de Ourense).
Conflict of Interest Statement
The authors declare that the research was conducted in the absence of any commercial or financial relationships that could be construed as a potential conflict of interest.
Acknowledgments
The authors wish to thank Jaume Morales and Rubén García from Agilent Technologies for technical support and to the PhD Associate Professor José Benito Quintana from the Institute of Food Analysis and Research Department of Analytical Chemistry at the University of Santiago de Compostela for instrument support.
Supplementary Material
The Supplementary Material for this article can be found online at: https://www.frontiersin.org/articles/10.3389/fmicb.2018.02701/full#supplementary-material
References
Al-Shabany, A. J., Moody, A. J., Foey, A. D., and Billington, R. A. (2016). Intracellular NAD+ levels are associated with LPS-induced TNF- release in pro-inflammatory macrophages. Biosci. Rep. 36:e00301. doi: 10.1042/BSR20150247
Bernardo, D., Sánchez, B., Al-Hassi, H. O., Mann, E. R., Urdaci, M. C., Knight, S. C., et al. (2012). Microbiota/host crosstalk biomarkers: regulatory response of human intestinal dendritic cells exposed to Lactobacillus extracellular encrypted peptide. PLoS ONE 7:e36262. doi: 10.1371/journal.pone.0036262
Blanco-Míguez, A., Gutiérrez-Jácome, A., Fdez-Riverola, F., Lourenço, A., and Sánchez, B. (2017). MAHMI database: a comprehensive MetaHit-based resource for the study of the mechanism of action of the human microbiota. Database 2017:baw157. doi: 10.1093/database/baw157
Breger, L. S., Kienle, K., Smith, G. A., Dunnett, S. B., and Lane, E. L. (2017). Influence of chronic L-DOPA treatment on immune response following allogeneic and xenogeneic graft in a rat model of Parkinson's disease. Brain. Behav. Immun. 61, 155–164. doi: 10.1016/j.bbi.2016.11.014
Dettmer, K., Nürnberger, N., Kaspar, H., Gruber, M. a., Almstetter, M. F., and Oefner, P. J. (2011). Metabolite extraction from adherently growing mammalian cells for metabolomics studies: optimization of harvesting and extraction protocols. Anal. Bioanal. Chem. 399, 1127–1139. doi: 10.1007/s00216-010-4425-x
Gika, H. G., Zisi, C., Theodoridis, G., and Wilson, I. D. (2016). Protocol for quality control in metabolic profiling of biological fluids by U(H)PLC-MS. J. Chromatogr. B 1008, 15–25. doi: 10.1016/j.jchromb.2015.10.045
Han, M., Xie, M., Han, J., Yuan, D., Yang, T., and Xie, Y. (2018). Development and validation of a rapid, selective, and sensitive LC–MS/MS method for simultaneous determination of d- and l-amino acids in human serum: application to the study of hepatocellular carcinoma. Anal. Bioanal. Chem. 410, 2517–2531. doi: 10.1007/s00216-018-0883-3
Hemarajata, P., and Versalovic, J. (2013). Effects of probiotics on gut microbiota: mechanisms of intestinal immunomodulation and neuromodulation. Therap. Adv. Gastroenterol. 6, 39–51. doi: 10.1177/1756283X12459294
Henker, J., Laass, M. W., Blokhin, B. M., Maydannik, V. G., Bolbot, Y. K., Elze, M., et al. (2008). Probiotic escherichia coli nissle 1917 versus placebo for treating diarrhea of greater than 4 days duration in infants and toddlers. Pediatr. Infect. Dis. J. 27, 494–499. doi: 10.1097/INF.0b013e318169034c
Hidalgo-Cantabrana, C., Moro-García, M. A., Blanco-Míguez, A., Fdez-Riverola, F., Lourenço, A., Alonso-Arias, R., et al. (2017). In silico screening of the human gut metaproteome identifies Th17-promoting peptides encrypted in proteins of commensal bacteria. Front. Microbiol. 8:1726. doi: 10.3389/fmicb.2017.01726
Hill, C., Guarner, F., Reid, G., Gibson, G. R., Merenstein, D. J., Pot, B., et al. (2014). The international scientific association for probiotics and prebiotics consensus statement on the scope and appropriate use of the term probiotic. Nat. Rev. Gastroenterol. Hepatol. 11, 506–514. doi: 10.1038/nrgastro.2014.66
Holmes, E., Li, J. V., Marchesi, J. R., and Nicholson, J. K. (2012). Gut microbiota composition and activity in relation to host metabolic phenotype and disease risk. Cell Metab. 16, 559–564. doi: 10.1016/j.cmet.2012.10.007
Hwang, S. J., Durnin, L., Dwyer, L., Rhee, P. L., Ward, S. M., Koh, S. D., et al. (2011). β-Nicotinamide adenine dinucleotide is an enteric inhibitory neurotransmitter in human and nonhuman primate colons. Gastroenterology 140, 608–617. doi: 10.1053/j.gastro.2010.09.039
Jackson, S. N., Wang, H.-Y. J., Woods, A. S., Ugarov, M., Egan, T., and Schultz, J. A. (2005). Direct tissue analysis of phospholipids in rat brain using MALDI-TOFMS and MALDI-ion mobility-TOFMS. J. Am. Soc. Mass Spectrom. 16, 133–138. doi: 10.1016/j.jasms.2004.10.002
Kennedy, B. E., Sharif, T., Martell, E., Dai, C., Kim, Y., Lee, P. W. K., et al. (2016). NAD+salvage pathway in cancer metabolism and therapy. Pharmacol. Res. 114, 274–283. doi: 10.1016/j.phrs.2016.10.027
Ko, J. S., Koh, J. M., So, J.-S., Jeon, Y. K., Kim, H. Y., and Chung, D. H. (2017). Palmitate inhibits arthritis by inducing t-bet and gata-3 mRNA degradation in iNKT cells via IRE1α-dependent decay. Sci. Rep. 7:14940. doi: 10.1038/s41598-017-14780-4
Konstantinov, S. R., Smidt, H., de Vos, W. M., Bruijns, S. C. M., Singh, S. K., Valence, F., et al. (2008). S layer protein A of Lactobacillus acidophilus NCFM regulates immature dendritic cell and T cell functions. Proc. Natl. Acad. Sci. U. S. A. 105, 19474–19479. doi: 10.1073/pnas.0810305105
Lenders, J. W. M., Pacak, K., Walther, M. M., Linehan, W. M., Mannelli, M., Friberg, P., et al. (2002). Biochemical diagnosis of pheochromocytoma. JAMA 287, 1427–1434. doi: 10.1001/jama.287.11.1427
León, Z., García-Cañaveras, J. C., Donato, M. T., and Lahoz, A. (2013). Mammalian cell metabolomics: experimental design and sample preparation. Electrophoresis 34, 2762–2775. doi: 10.1002/elps.201200605
Li, Y., and Chen, X. (2012). Sialic acid metabolism and sialyltransferases: natural functions and applications. Appl. Microbiol. Biotechnol. 94, 887–905. doi: 10.1007/s00253-012-4040-1
Marcinkiewicz, J., and Kontny, E. (2014). Taurine and inflammatory diseases. Amino Acids 46, 7–20. doi: 10.1007/s00726-012-1361-4
Martinez, R. M., Hulten, K. G., Bui, U., and Clarridge, J. E. (2014). Molecular analysis and clinical significance of Lactobacillus spp. Recovered from clinical specimens presumptively associated with disease. J. Clin. Microbiol. 52, 30–36. doi: 10.1128/JCM.02072-13
Martins, J. D., Maciel, E. A., Silva, A., Ferreira, I., Ricardo, F., Domingues, P., et al. (2016). Phospholipidomic profile variation on THP-1 cells exposed to skin or respiratory sensitizers and respiratory irritant. J. Cell. Physiol. 231, 2639–2651. doi: 10.1002/jcp.25365
McNamara, L. E., Sjostrom, T., Meek, R. M. D., Oreffo, R. O. C., Su, B., Dalby, M. J., et al. (2012). Metabolomics: a valuable tool for stem cell monitoring in regenerative medicine. J. R. Soc. Interface 9, 1713–1724. doi: 10.1098/rsif.2012.0169
Mi, S., Zhao, Y.-Y., Dielschneider, R. F., Gibson, S. B., and Curtis, J. M. (2016). An LC/MS/MS method for the simultaneous determination of individual sphingolipid species in B cells. J. Chromatogr. B 1031, 50–60. doi: 10.1016/j.jchromb.2016.07.028
Mutafova-Yambolieva, V. N., Hwang, S. J., Hao, X., Chen, H., Zhu, M. X., Wood, J. D., et al. (2007). beta-Nicotinamide adenine dinucleotide is an inhibitory neurotransmitter in visceral smooth muscle. Proc. Natl. Acad. Sci. U.S.A. 104, 16359–16364. doi: 10.1073/pnas.0705510104
Nan, X., Carubelli, I., and Stamatos, N. M. (2007). Sialidase expression in activated human T lymphocytes influences production of IFN-gamma. J. Leukoc. Biol. 81, 284–296. doi: 10.1189/jlb.1105692
Naz, S., Vallejo, M., García, A., and Barbas, C. (2014). Method validation strategies involved in non-targeted metabolomics. J. Chromatogr. A 1353, 99–105. doi: 10.1016/j.chroma.2014.04.071
Nicholas, D. A., Zhang, K., Hung, C., Glasgow, S., Aruni, A. W., Unternaehrer, J., et al. (2017). Palmitic acid is a toll-like receptor 4 ligand that induces human dendritic cell secretion of IL-1β. PLoS ONE 12:e0176793. doi: 10.1371/journal.pone.0176793
Patti, G. J., Yanes, O., and Siuzdak, G. (2012). Innovation: metabolomics: the apogee of the omics trilogy. Nat. Rev. Mol. Cell Biol. 13, 263–269. doi: 10.1038/nrm3314
Pavlovic, Z., and Bakovic, M. (2013). Regulation of phosphatidylethanolamine homeostasis—The Critical Role of CTP:Phosphoethanolamine Cytidylyltransferase (Pcyt2). Int. J. Mol. Sci. 14, 2529–2550. doi: 10.3390/ijms14022529
Qiang, L., Sample, A., Liu, H., Wu, X., and He, Y.-Y. (2017). Epidermal SIRT1 regulates inflammation, cell migration, and wound healing. Sci. Rep. 7:14110. doi: 10.1038/s41598-017-14371-3
Quévrain, E., Maubert, M. A., Michon, C., Chain, F., Marquant, R., Tailhades, J., et al. (2015). Identification of an anti-inflammatory protein from Faecalibacterium prausnitzii, a commensal bacterium deficient in Crohn's disease. Gut 65, 415–425. doi: 10.1136/gutjnl-2014-307649
Sánchez, B., Chaignepain, S., Schmitter, J.-M., and Urdaci, M. C. (2009). A method for the identification of proteins secreted by lactic acid bacteria grown in complex media. FEMS Microbiol. Lett. 295, 226–229. doi: 10.1111/j.1574-6968.2009.01599.x
Sánchez, B., Ruiz, L., Suárez, A., De los Reyes-Gavilán, C. G., and Margolles, A. (2011). Human cecum content modulates production of extracellular proteins by food and probiotic bacteria. FEMS Microbiol. Lett. 324, 189–194. doi: 10.1111/j.1574-6968.2011.02408.x
Sanchez, B., Urdaci, M. C., and Margolles, A. (2010). Extracellular proteins secreted by probiotic bacteria as mediators of effects that promote mucosa-bacteria interactions. Microbiology 156, 3232–3242. doi: 10.1099/mic.0.044057-0
Sangster, T., Major, H., Plumb, R., Wilson, A. J., and Wilson, I. D. (2006). A pragmatic and readily implemented quality control strategy for HPLC-MS and GC-MS-based metabonomic analysis. Analyst 131:1075. doi: 10.1039/b604498k
Shah, S. H., Kraus, W. E., and Newgard, C. B. (2012). Metabolomic profiling for the identification of novel biomarkers and mechanisms related to common cardiovascular diseases: form and function. Circulation 126, 1110–1120. doi: 10.1161/CIRCULATIONAHA.111.060368
Stamatos, N. M., Curreli, S., Zella, D., and Cross, A. S. (2004). Desialylation of glycoconjugates on the surface of monocytes activates the extracellular signal-related kinases ERK 1/2 and results in enhanced production of specific cytokines. J. Leukoc. Biol. 75, 307–313. doi: 10.1189/jlb.0503241
Thomas, C. M., Hong, T., van Pijkeren, J. P., Hemarajata, P., Trinh, D. V., Hu, W., et al. (2012). Histamine derived from probiotic lactobacillus reuteri suppresses TNF via modulation of PKA and ERK signaling. PLoS One 7:e31951. doi: 10.1371/journal.pone.0031951
Thompson, M., Ellison, S. L. R., and Wood, R. (2002). Harmonized guidelines for single-laboratory validation of methods of analysis (IUPAC Technical Report). Pure Appl. Chem. 74, 835–855. doi: 10.1351/pac200274050835
Urdaci, M. C., and Sánchez, B. (2009). Some immunomodulatory effects of probiotic bacteria might be due to porcine neutrophil elastase inhibitor, a serpin present in MRS broth. Immunol. Lett. 122, 99–100. doi: 10.1016/j.imlet.2008.11.004
US FDA (2000). Guidance for Industry (Draft): Analytical Procedures and Methods Validation: Chemistry, Manufacturing, and Controls and Documentation. US FDA.
US FDA (2015). Guidance for Industry: Analytical Procedures and Methods Validation for Drugs and Biologics. US FDA.
Wang, W., Mu, L., Su, T., Ye, L., Jiang, Y., Jiang, L., et al. (2015). Plasma metanephrines are associated with glucose metabolism in patients with essential hypertension. Medicine (Baltimore). 94:e1496. doi: 10.1097/MD.0000000000001496
Wang, Y., Qian, Y., Fang, Q., Zhong, P., Li, W., Wang, L., et al. (2017). Saturated palmitic acid induces myocardial inflammatory injuries through direct binding to TLR4 accessory protein MD2. Nat. Commun. 8:13997. doi: 10.1038/ncomms13997
Keywords: bacterial peptides, human peripheral blood mononuclear cells (PBMCs), untargeted metabolomics, LC-ESI-QTOF-MS, host immunomodulation
Citation: Cambeiro-Pérez N, Hidalgo-Cantabrana C, Moro-García MA, Alonso-Arias R, Simal-Gándara J, Sánchez B and Martínez-Carballo E (2018) A Metabolomics Approach Reveals Immunomodulatory Effects of Proteinaceous Molecules Derived From Gut Bacteria Over Human Peripheral Blood Mononuclear Cells. Front. Microbiol. 9:2701. doi: 10.3389/fmicb.2018.02701
Received: 14 August 2018; Accepted: 23 October 2018;
Published: 13 November 2018.
Edited by:
Juarez Antonio Simões Quaresma, Instituto Evandro Chagas, BrazilReviewed by:
Robin Mesnage, King's College London, United KingdomDimiter Dimitrov, Diavita Ltd., Bulgaria
Copyright © 2018 Cambeiro-Pérez, Hidalgo-Cantabrana, Moro-García, Alonso-Arias, Simal-Gándara, Sánchez and Martínez-Carballo. This is an open-access article distributed under the terms of the Creative Commons Attribution License (CC BY). The use, distribution or reproduction in other forums is permitted, provided the original author(s) and the copyright owner(s) are credited and that the original publication in this journal is cited, in accordance with accepted academic practice. No use, distribution or reproduction is permitted which does not comply with these terms.
*Correspondence: Borja Sánchez, Ym9yamEuc2FuY2hlekBjc2ljLmVz
Elena Martínez-Carballo, ZWxlbmEubWFydGluZXpAdXZpZ28uZXM=