- Key Laboratory of Dairy Biotechnology and Engineering, Ministry of Education, Key Laboratory of Dairy Products Processing, Ministry of Agriculture, Inner Mongolia Agricultural University, Huhhot, China
Traditional sour cream and butter are widely popular fermented dairy products in Russia for their flavor and nutrition, and contain rich microbial biodiversity, particularly in terms of lactic acid bacteria (LAB). However, few studies have described the microbial communities and metabolic character of traditional sour cream and butter. The objective of this study was to determine the bacterial microbiota and metabolic character of eight samples collected from herdsmen in Buryatia, Russia. Using single-molecule real-time (SMRT) sequencing techniques, we identified a total of 294 species and/or subspecies in 169 bacterial genera, belonging to 14 phyla. The dominant phylum was Firmicutes (81.47%) and the dominant genus was Lactococcus (59.28%). There were differences between the bacterial compositions of the sour cream and butter samples. The relative abundances of Lactococcus lactis, Lactococcus raffinolactis, and Acetobacter cibinongensis were significantly higher in sour cream than in butter, and the abundance of Streptococcus thermophilus was significantly lower in sour cream than in butter. Using a pure culture method, 48 strains were isolated and identified to represent seven genera and 15 species and/or subspecies. Among these isolates, Lactococccus lactis subsp. lactis (22.50%) was the dominant LAB species. Ultra-performance liquid chromatography–quadrupole–time of flight mass spectrometry at elevated energy was used in combination with statistical methods to detect metabolite differences between traditional sour cream and butter. A total of 27,822 metabolites were detected in all samples, and Lys-Lys, isohexanal, palmitic acid, Leu-Val, and 2′-deoxycytidine were the most dominant metabolites found in all samples. In addition, 27 significantly different metabolites were detected between the sour cream and butter samples, including short peptides, organic acids, and amino acids. Based on correlation analyses between the most prevalent bacterial species and the main metabolites in sour cream, we conclude that there may be a connection between the dominant LAB species and these metabolites. This study combined omics techniques to analyze the bacterial diversity and metabolic character of traditional sour cream and butter, and we hope that our findings will enrich species resource libraries and provide valuable resources for further research on dairy product flavor.
Introduction
Russian sour cream, a widely popular fermented cream product, plays an important role in the diet of Russian people. Sour cream has a milky white color, fragrant flavor, and delicious taste. It can be eaten directly with bread, and is widely used as a condiment, in cooking, and baking various types of pastry. Russia consists of 193 different ethnic groups and indigenous peoples, and each region has its own unique culture and customs, as well as its own types and methods of preparing dairy products. The processing technique used to make sour cream (susegei) in Buryatia, Russia is simple: raw milk is stored in casks in a dark and dry room for 3–4 days. Natural fermentation leads to layering of the fermented milk, with the top layer being sour cream. The addition of hot water and stirring are performed to make butter (tohon), which is then stored in a sheep stomach. Butter has been consumed for 1000s of years in Buryatia. Obviously, many natural microorganisms originating from the raw milk and environment are involved in these fermentation processes. Therefore, a comprehensive and systematic analysis of the complex bacterial communities within sour cream and butter in Russia would be useful in the commercialization of these products.
In previous studies, traditional culture methods have been used to investigate the microbial communities in traditionally fermented dairy products (Mathara et al., 2004; Elionora Hantsis-Zacharov, 2007; Giannino et al., 2009). However, microbial communities described solely on the basis of culturing are often incomplete, as many species are unculturable or poorly represented by the culturing process (Duniere et al., 2017). With the development of polymerase chain reaction (PCR) technology, alternative culture-independent techniques have facilitated investigations of the complex microbial microbiota in traditional dairy products. Among these, metagenomic sequencing of microbial DNA is an important culture-independent method that could provide a comprehensive microbiota profile independent of phenotypic traits and the cultivability of individual microbes. This modern molecular approach has been used to identify the microbiota of different types of traditional fermented dairy products, such as Irish artisanal cheese (Quigley et al., 2012), Italian Plaisentif cheese (Dalmasso et al., 2016), Belgian Herve cheese (Delcenserie et al., 2014), Mongolian naturally fermented cow’s milk (Liu et al., 2015), and Xinjiang yogurts (Xu et al., 2015). Recently, a new high-throughput sequencing tool, the third-generation Pacific Biosciences (Menlo Park, CA, United States) single-molecule real-time (SMRT) sequencing technology, was developed to generate long reads and allow high taxonomic resolution at the species level when coupled with full-length 16S ribosomal RNA (rRNA) gene sequencing (Mosher et al., 2014). This technique has been successfully applied to evaluate infant formula safety (Zheng et al., 2016) and to investigate bacterial profiles in traditional fermented koumiss (Gesudu et al., 2016) and artisanal Kazakhstan cheese (Li et al., 2017). To our knowledge, microbiota studies have focused on cheeses and fermented milks; no simultaneous studies of the microbial communities of traditional sour cream and butter have been conducted.
Given the wide popularity of Russian traditional sour cream and butter, and that the Buryatia region maintains traditional dairy processing methods, we collected samples of traditional sour cream and butter from local households in Buryatia, Russia. We first used SMRT sequencing technology to assess the bacterial population present in sour cream and butter samples, and tested for differences in bacterial composition between sour cream and butter. We then isolated lactic acid bacteria (LAB) using culture methods with the aim of preserving these valuable LAB resources for subsequent research and industrial applications. Third, to further understand the nutritional characteristics of sour cream and butter, we conducted ultra-performance liquid chromatography–quadrupole–time of flight (UPLC–Q–TOF) mass spectrometry at elevated energy (MSE) to detect the metabolites of sour cream and butter.
Materials and Methods
Sample Collection
A total of five traditional sour cream (BL9, BL11, BL17, BL21, and BL26) and three butter (BL14, BL18, and BL20) samples were collected from six different regions in Buryatia, Russia. The pH values of these samples ranged from 4.51 to 5.03. Samples were collected in sterilized containers and transported to the laboratory in a 4°C vehicle-mounted refrigerator.
Isolation and Identification of LAB
Lactic acid bacteria strains were isolated from the eight samples using a previously described culture method (Yu et al., 2015). A total of 48 Gram-positive and catalase-negative isolates were obtained from the samples. Isolates were preserved in milk containing 0.1% sodium glutamate and stored at −40°C.
We identified the 48 isolates using 16S rRNA gene sequencing technology. The 16S rRNA gene primers used were 16S-FA (GCAGAGTTCTCGGAGTCACGAAG AGTTTGATCCTGGCTCAG) and 16S-RA (AGCGGATCACTTCACACAGGACTA CGGCTACCTTGTTACGA). Genomic DNA extraction and PCR amplification were performed as previously described (Yu et al., 2011). DNA sequencing was performed by Majorbio Bio-Pharm Technology Corporation Limited (Shanghai, China). A phylogenetic tree was constructed based on the generated sequences using MEGA v. 7.0 software1.
PacBio SMRT Sequencing and Bioinformatics Analysis
We centrifuged 3 g sour cream and butter samples at 10,000 × g for 20 min in an Eppendorf 5810R centrifuge. The supernatant was then removed and the cream attached to the tube was removed using sterile swabs. The remaining pellet was re-suspended in 500 mL of sterile water; 500 mg of lysozyme was added prior to incubation for 12 h at 37°C to maximize bacterial DNA extraction. Genomic DNA was extracted using the DNeasy Mericon Food Kit (69514, Qiagen, Hilden, Germany) according to the manufacturer’s instructions. DNA concentration and purity were evaluated using 0.8% agarose gel electrophoresis and optical density using a NanoDrop ND-1000 spectrophotometer (Thermo Fisher Scientific, Wilmington, DE, United States).
Genomic DNA was used as a template for PCR amplification of the full-length 16S rRNA gene for SMRT sequencing. The primers and reaction conditions were performed as described previously (Hou et al., 2015). The quality of the PCR products was verified using the Agilent DNA 1000 Kit and an Agilent 2100 Bioanalyser (Agilent Technologies, Santa Clara, CA, United States). Purified PCR products were used to construct DNA libraries with the Pacific Biosciences Template Prep Kit 2.0. The P6–C4 chemistry of the PacBio RS II instrument (Pacific Biosciences) was used to sequence the purified amplification products.
Raw sequence data were generated using the RS_ReadsOfinsert.1 protocol available on the SMRT Portal (v. 2.7) (Hou et al., 2015). The Quantitative Insights into Microbial Ecology (QIIME) package (v. 1.7) was used to extract high-quality sequences, and these sequences were then aligned under 100% sequence identity clustering to obtain representative sequences using the PyNAST and UCLUST systems (Caporaso et al., 2010; Edgar, 2010). Sequences were subsequently classified into operational taxonomic units (OTUs) below a threshold of 97% using UCLUST software (Lozupone et al., 2006). The Ribosomal Database Project II database was used to assign the taxonomy of each OTU-representative sequence at an 80% confidence threshold (Cole et al., 2007). A de novo taxonomic tree was constructed using a representative OTU set in the FastTree software for downstream analysis (Price et al., 2009). The α-diversity was evaluated using Shannon–Wiener, Simpson’s diversity, Chao1, and rarefaction estimators. R programming language v. 3.1.22 and Origin v. 8.5 software were used to test correlations and produce graphs.
Determination of Metabolites by UPLC–Q–TOF MSE
The dairy products were centrifuged at 4000 × g for 10 min, and 1 mL of suspension was collected after the fat layer was discarded. We added 7 mL of acetonitrile solution to the suspension, which was then vortexed for 2 min before high-speed centrifugation at 12,000 × g. The supernatant was concentrated in a vacuum concentrator, reconstituted in 500 μL of 40% (v/v) acetonitrile solution, filtered through a 0.22-μm water-insoluble microporous membrane, and stored at −20°C. An ACQUITY UPLC/Xevo G2 Q TOF–MSE chromatography system (Waters Corp., Milford, MA, United States) was used to extract MS spectral peaks of dairy products; it was equipped with an electrospray ionization (ESI) source that operated in both positive and negative ion modes (ESI+ and ESI−). Pre-treated milk samples were chromatographed using a C18-UPLC system. The column was HSS T3 (2.1 mm × 100 mm × 1.8 μm, Waters Corp.), the flow rate was 0.45 mL/min, and the column temperature was 40°C. The ion scan mode, liquid phase, mass spectrometry conditions, and sample volume settings were selected according to a previous study (Pan et al., 2018). Raw data obtained by UPLC-Q-TOF MSE were collected in continuum mode and processed using the MassLynx and Progenesis QI software (Waters Corp.). Data preprocessing procedures including peak alignment, peak identification, and deconvolution were completed, and the resulting data set was imported into EZinfo software. PCA and orthogonal projections to latent structures discriminant analysis (OPLS-DA) were then performed on the data set. Significant differences in metabolites between dairy products were determined when VIP ≥ 1, fold change ≥ 2, and P < 0.05. The selected differential metabolites were identified using the Human Metabolome Database (HMDB3), Kyoto Encyclopedia of Genes and Genomes (KEGG)4, ChemSpider5, and METLIN6 databases.
Nucleotide Sequence Accession Number
All PacBio SMRT sequencing data reported in this study were deposited at Metagenomic Rapid Annotations using the Subsystems Technology database (accession no. mgp83644)7. All 16S rDNA sequences from LAB isolates were submitted to the National Center for Biotechnology Information database (accession nos. MF784091–MF784111 and MF784124–MF784150).
Results
Enumeration and Identification of LAB
Viable counts of LAB present in the eight dairy samples of butter and sour cream ranged from 5.19 ± 0.01 to 8.37 ± 0.01 l g CFU/g. We obtained 48 isolates by pure culture, which were presumptively identified as LAB based on Gram-positive and catalase-negative phenotypes. Partial 16S rRNA gene sequencing was further used to identify these isolates belonging to seven genera and 15 species, including Enterococcus casseliflavus (2 strains), E. italicus (2 strains), E. faecium (2 strains), Lactobacillus fermentum (2 strains), Lactobacillus paracasei (1 strain), Lactobacillus plantarum (7 strains), Lactobacillus curvatus (1 strain), Lactococcus lactis (16 strains), Lactococcus garvieae (2 strains), Leuconostoc mesenteroides (3 strains), Leuconostoc garlicum (2 strains), Leuconostoc lactis (1 strain), Pediococcus acidilactici (2 strains), Streptococcus thermophilus (4 strains), and Weissella hellenica (1 strain) (Figure 1).
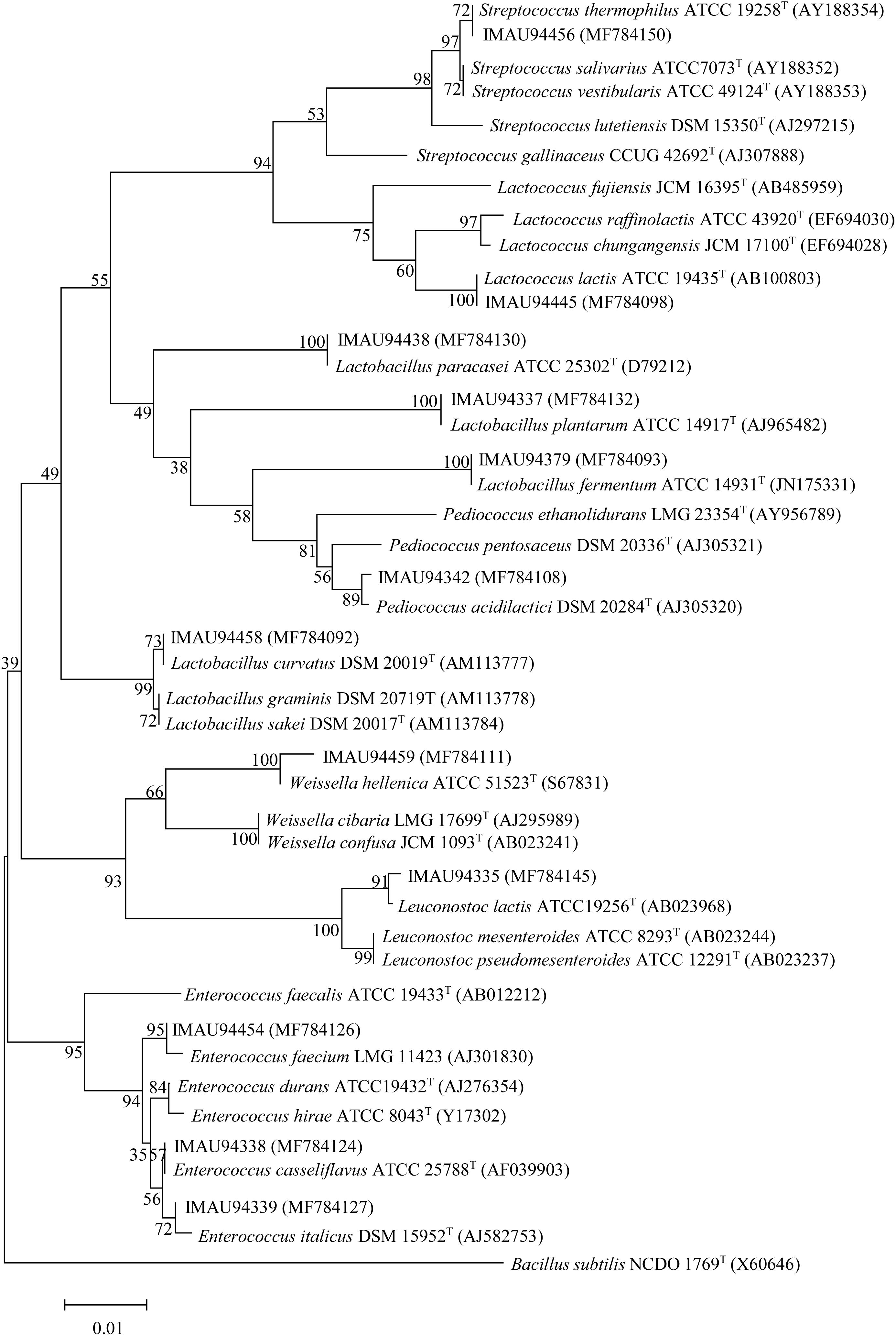
FIGURE 1. Neighbor-joining tree showing the phylogenetic relationships between isolates and the type strains of related genera based on 16S rRNA gene sequences. Bacillus subtilis was used as an outgroup.
Bacterial Community Diversity and Richness in Butter and Sour Cream
A total of 81,730 high-quality SMRT sequencing reads (average: 10,216 reads/sample, range: 7,034–15,783, standard deviation [SD]: 2,875) were obtained from the eight samples. Following alignment and sequence identity clustering, 7,301 OTUs were generated with an average of 912.63 representative OTUs (SD: 551.18) for each individual sample. The number of reads, observed species (155.34–1689.80), Chao1 index (484.77–13724.40), Simpson’s index (0.267–0.909), and Shannon–Weiner index (1.275–6.314) of the eight samples are shown in Table 1. As shown in Figure 2, the Shannon diversity and rarefaction curves suggest that the overall bacterial phenotypes were well captured at the current sequence depth.
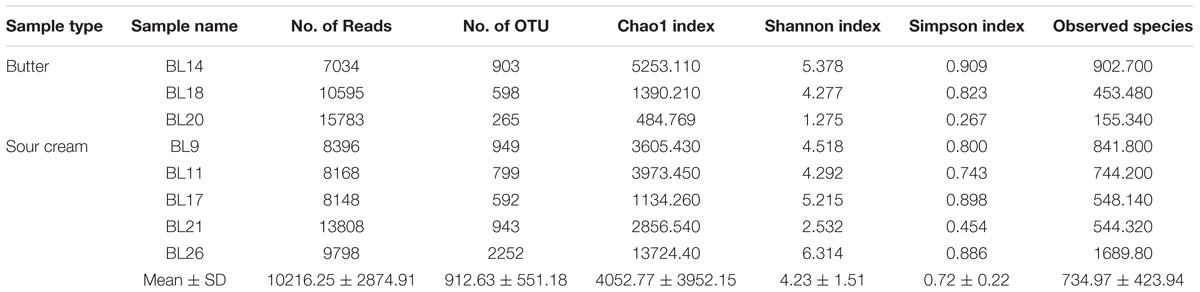
TABLE 1. Operational taxonomic units (OTUs) number of sequence and OTUs, microbial diversity and richness indexes of sour cream and butter samples.
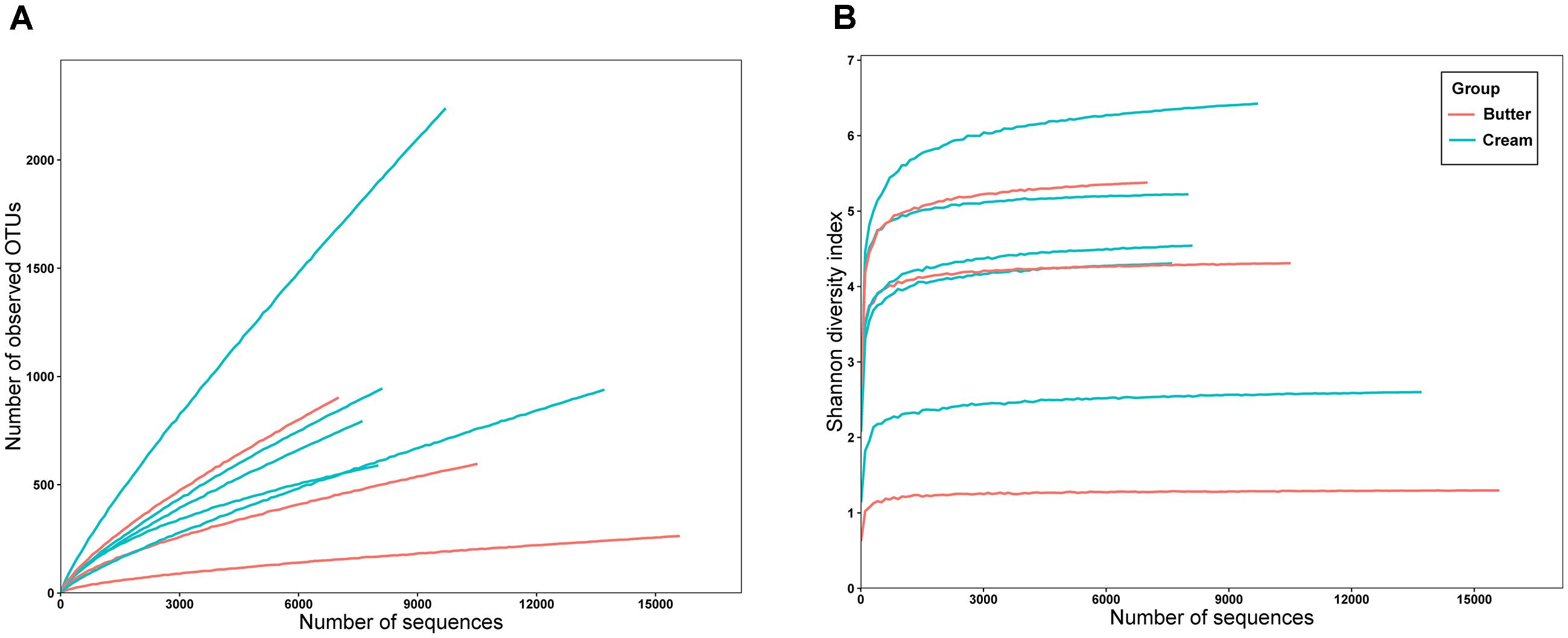
FIGURE 2. Rarefaction analysis (A) and Shannon diversity (B) estimates of the SMRT sequencing reads of bacteria in sour cream and butter samples.
Bacterial Composition of Butter and Sour Cream
We identified nine bacterial phyla in butter samples and 14 in sour cream samples. The major bacterial phyla (with average relative abundance > 1%) in all samples were Firmicutes (81.47%), Proteobacteria (16.11%), and Bacteroidetes (2.05%); there were no significant differences in bacterial relative abundance between butter and sour cream samples. At the genus level, 75 and 155 bacterial genera were detected in butter and sour cream, respectively. Lactococcus (77.73%), Acetobacter (7.80%), Leuconostoc (4.10%), Lactobacillus (3.09%), and Curvibacter (1.92%) were the dominant genera in butter samples, whereas Lactococcus (48.21%), Streptococcus (23.63%), Curvibacter (8.15%), Acinetobacter (5.43%), Lactobacillus (2.21%), Chryseobacterium (1.90%), Pseudomonas (1.71%), and Carnobacterium (1.13%) were the dominant genera in sour cream samples. The relative abundances of Lactococcus and Acetobacter were significantly higher in sour cream than in butter, and those of Streptococcus, Curvibacter, and Acinetobacteras were significantly lower in sour cream than in butter (Figure 3A). At the species level, a total of 110 and 267 different bacterial species were identified in butter and sour cream, respectively (Supplementary Table S1). In butter samples, the most abundant species (with average relative abundance > 1%) were Lactococcus lactis (53.48%), Lactococcus raffinolactis (20.00%), Acetobacter cibinongensis (7.72%), Lactococcus chungangensis (3.30%), Lactobacillus helveticus (2.72%), Leuconostoc lactis (2.48%), and Leuconostoc mesenteroides (1.43%). The most abundant species (with average relative abundance > 1%) in sour cream samples were Lactococcus lactis (39.39%), S. thermophilus (10.60%), Lactococcus raffinolactis (6.11%), Acinetobacter lwoffii (3.07%), Lactococcus chungangensis (1.76%), Acetobacter cibinongensis (7.72%), and Acinetobacter johnsonii (1.38%). The relative abundances of Lactococcus lactis, Lactococcus raffinolactis, and Acetobacter cibinongensis were significantly higher in sour cream than in butter, and those of S. thermophilus were significantly lower in sour cream than in butter (Figure 3B).
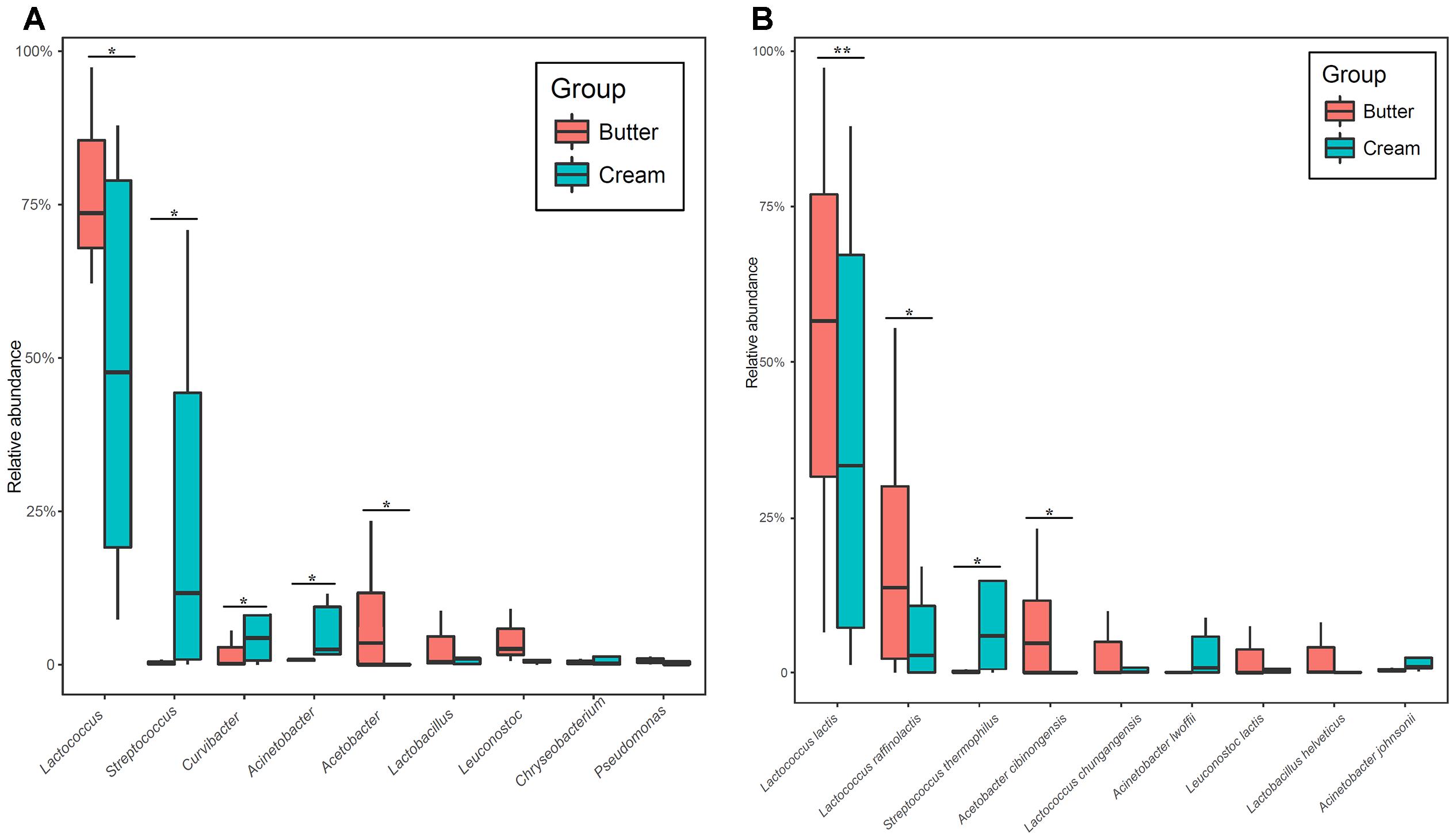
FIGURE 3. Boxplots of the relative abundance of high abundance (>1%) bacteria detected in sour cream and butter samples at the genera (A) and species level (B) with significant differences.
Comparison of the Bacterial Community Structures of Butter and Sour Cream
To compare the bacterial communities of butter and sour cream, we performed weighted and unweighted UniFrac principal co-ordinate analysis (PCoA) based on the OTU abundance table. As shown in Figure 4, both unweighted (principal components PC1 and PC3 accounted for 20.08 and 15.99% of the total variance, respectively) and weighted (PC1 and PC3 accounted for 66.41 and 7.49% of the total variance, respectively) UniFrac distances revealed apparent structural differences between the bacterial communities in the butter and sour cream samples.
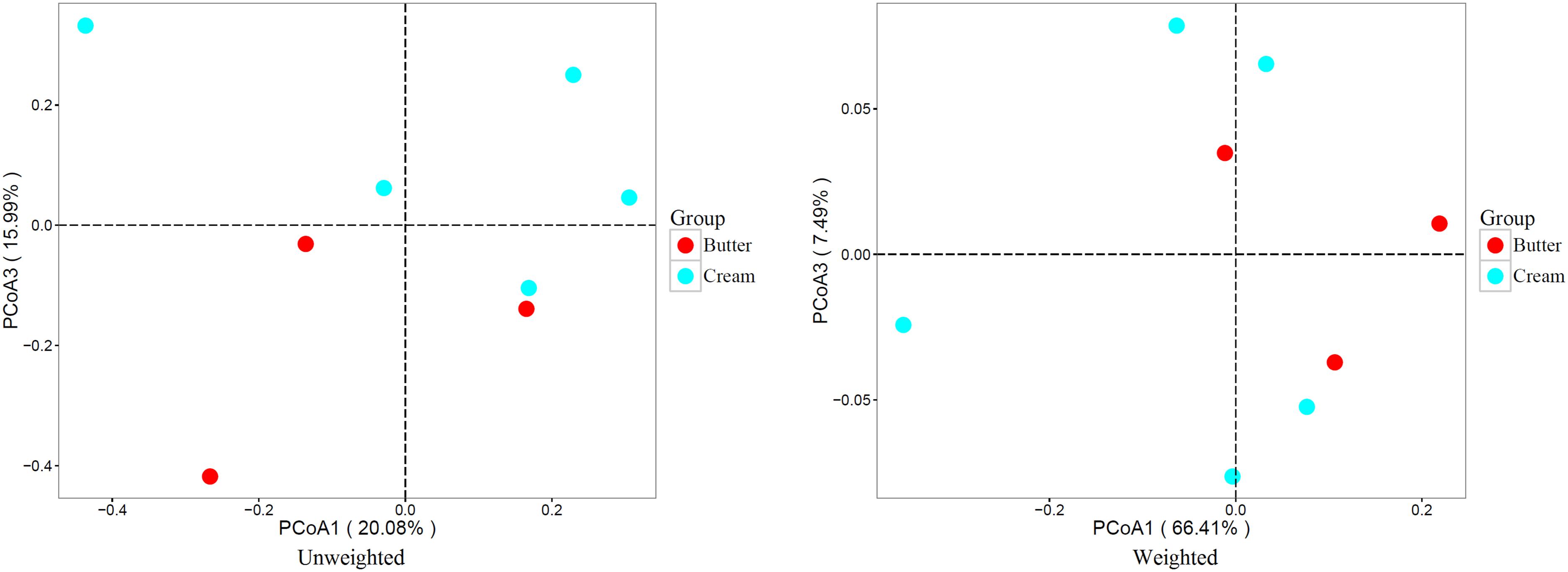
FIGURE 4. Weighted UniFrac principal coordinate analysis (PCoA) of the bacterial communities in butter and sour cream samples. Each symbol represents the butter and sour cream microbiota of one sample; butter and sour cream sample is represented by the respective color.
Metabolite Profiling of Butter and Sour Cream Samples
A total of 27,822 metabolites (1,182 and 26,640 identified by positive and negative ion mode, respectively) were detected in all eight samples. Among these, Lys-Lys, isohexanal, palmitic acid, Leu-Val, and 2′-deoxycytidine were the most dominant metabolites in all samples. To visualize the metabolic differences between sour cream and butter, an unsupervised PCA was performed on the metabolome (Figure 5A). Principal components 1 and 2 represented 67.7 and 23.6% of the total variance, respectively. In the PCA score plots, symbols representing triplicate samples of sour cream and butter were not clearly separated, indicating the presence of many similar metabolites in the cream and butter samples. These data were further processed with a supervised OPLS-DA to identify differential metabolites. The score plot and S-plots are shown in Figure 5B, which demonstrates a clear clustered pattern between the two groups of samples. All R2Y and Q2 values were greater than 0.75, indicating high fitness and prediction ability. In the S-plot, each point represents a variable; a greater distance between a point and the origin indicates a greater contribution by that variable to the group difference, as well as greater confidence. According to the screening principle, the condition-matched metabolic differential variables were further analyzed and identified; finally, 27 significantly different metabolites were detected between the sour cream and butter samples (Table 2). Among these, the abundances of piperidine, Gly-Ser, Asn-Tyr, threonine, N-acetyl-L-leucine, Gln-Thr, Ser-Asn, tartaric acid, threonine, His-Ala, Pro-Asn, purine, butyric acid, and hexanal were higher in butter than in sour cream, whereas those of Met-Tyr, His-Asn, L-xylulose, decanoic acid, cystine, L-cysteine, L-lysine, Lys-Val, Tyr-Cys, L-alanine, uridine, aspartic acid, serine, and Gly-Ser-Pro-Met-Phe-Ala-Val were greater in sour cream than in butter.
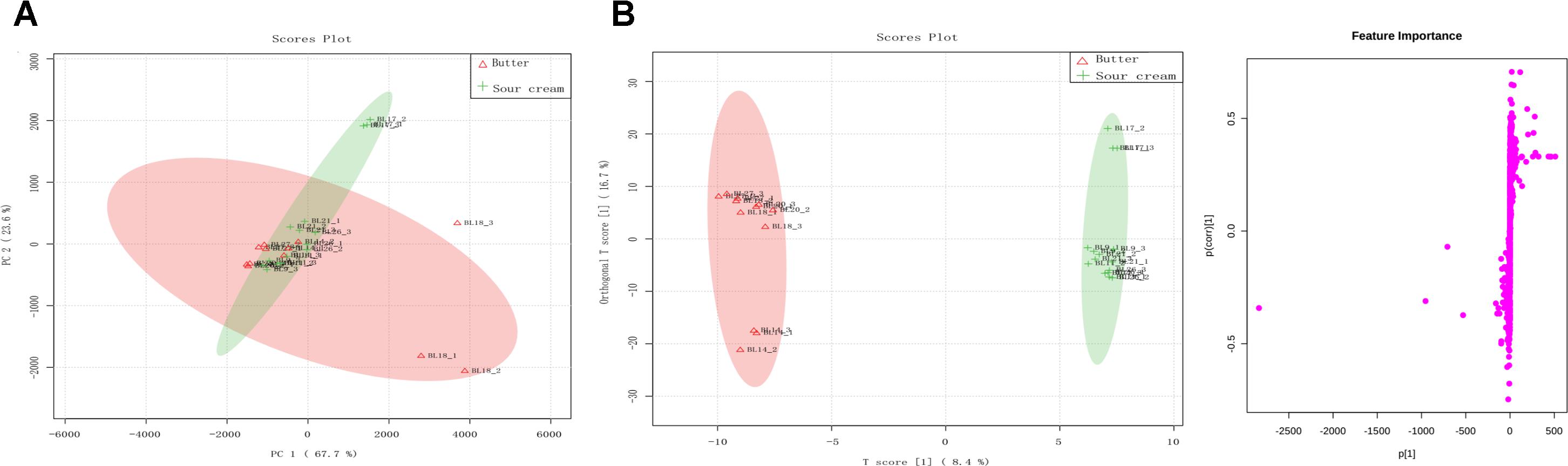
FIGURE 5. (A) Principal component analysis (PCA) score plots of the sour cream and butter metabolome. Color version available online. (B) Orthogonal partial least squares discriminant analysis (OPLS-DA): S-plots of the data for sour cream and butter samples.
Correlation Between the Relative Abundance of Dominant Bacteria and Metabolites in Sour Cream
To investigate the relationship between the dominant bacteria and metabolites in the sour cream samples, we performed a correlation analysis between the most prevalent bacterial species (average relative abundance > 0.5%) and 19 main metabolites in sour cream. Because there were fewer butter samples, it was difficult to calculate the correlation between the bacterial species and metabolites in these samples. As shown in Figure 6, the predominant species L. lactis was positively correlated with the 12 metabolites that were of higher relative abundance, including decanoic acid, palmitic acid, L-xylulose, isohexanal, and some amino acids, and significantly negatively correlated with L-lysine. The sub-dominant species S. thermophilus was positively correlated with cystine, aspartic acid, uridine L-lysine, Lys-Lys, Tyr-Cys, and His-Asn, and significantly negatively correlated with L-xylulose. L. chungangensis was significantly positively correlated with cysteine, and A. johnsonii was significantly negatively correlated with uridine. L. raffinolactis, L. delbrueckii, and Carnobacterium divergens were significantly positively correlated with the peptide Gly-Ser-Pro-Met-Phe-Ala-Val, and significantly negatively correlated with decanoic acid. A. lwoffii was significantly negatively correlated with decanoic acid, Lys-Val, and Met-Tyr. Macrococcus caseolyticus was significantly positively correlated with Leu-Val, and negatively correlated with His-Asn. The results indicate that some of the metabolites in sour cream were the products of fermentation by the species with which they were correlated.
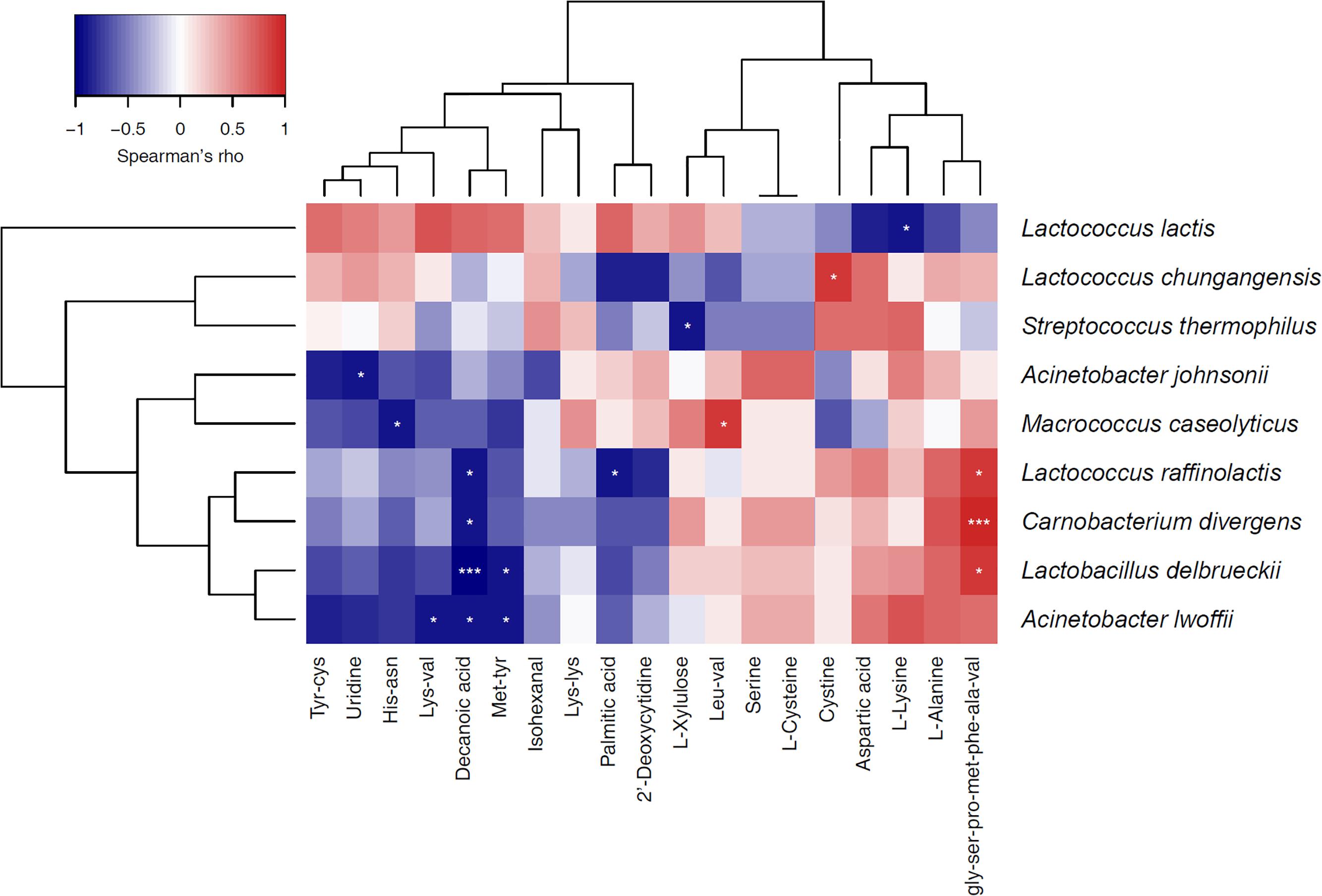
FIGURE 6. Heatmap showing the correlation relationships between the most prevalent bacterial genera (with average relative abundance of > 0.5%) and main metabolites in sour cream samples.
Discussion
Traditionally fermented dairy products offer unique flavor and nutrition, which are closely related to the bacterial composition and milk resource. Therefore, the objective of this study was to combine omics techniques to analyze the bacterial diversity and metabolic character of traditional sour cream and butter collected in Buryatia, Russia. A total of 48 LAB strains were isolated and identified as seven genera and 15 species. A greater number of strains were identified as Lactococcus lactis, Lactobacillus plantarum, and S. thermophilus than as other species. SMRT sequencing results also indicated that Lactococcus lactis and S. thermophilus were the most abundant species in the sour cream and butter samples (Figure 3 and Supplementary Table S1). This result is similar to that of a previous study on traditional sour cream, which reported Leuconostoc mesenteroides, Lactococcus lactis, Lactobacillus plantarum, and Lactobacillus helveticus strains as the dominant isolates in sour cream from Kalmykiya and Tuva, respectively (Yu et al., 2015). In addition, all 15 isolated species were detected by SMRT sequencing, but these accounted for only 5.1% (15/294 species) of all species detected by SMRT sequencing. It is known that approximately 99% of microorganisms in the natural environment are not readily cultivable by traditional culture methods (Amann et al., 1995). Although the PacBio SMRT platform is indispensable for the systematic investigation of bacterial diversity and community structure in environmental samples, it can be coupled with full-length 16S rRNA sequencing to determine bacterial profiles at the species level. Using the SMRT sequencing data, we could improve medium components and culture conditions to isolate the desirable strains in a future study.
The 16S rRNA profiling of the microbiota found slight variations in the microbial compositions of the sour cream and butter samples. The relative abundances of Lactococcus lactis and S. thermophilus were significantly different (P < 0.05) between the butter and sour cream samples, and there were no significant differences among those of the other species between sample types. These samples were all prepared from cow’s milk, and the sampling region was more isolated; we speculate that these conditions led to the similarities in indigenous microflora between sample types. Liu et al. (2015) investigated microbial populations in naturally fermented cow milk samples from the Russian republics of Kalmykia and Chita using ribosomal-gene-targeted 454 pyrosequencing. Lactobacillus and Lactococcus were the predominant genera in samples from both Kalmykia and Chita in the present study. However, Lactococcus (51.46%) and Streptococcus (17.81%) have been reported to be the most dominant bacterial species in traditional Buryatian cottage cheese (Jin et al., 2018). The results of that study indicated that raw milk, external environment, processing method, and geographic location attributed to differences in microbial composition among traditional dairy products. As in our study, previous reports have confirmed that the exposure of traditional dairy products to different external environments during the manufacturing process impacted the microbiota composition of the final products (Feurer et al., 2004; Quigley et al., 2012). Furthermore, the abundances of lactococci, such as species of Lactococcus, Leuconostoc, and Streptococcus, were higher than lactobacilli in sour cream and butter in the present study. We conclude that lactococci may be able to better adapt to high-fat environments than lactobacilli. Although LAB have a low ability to hydrolyze fat, they could survive in sour cream and butter. Therefore, these strains should be further screened as starting cultures for the industrial production of sour cream.
Streptococcus thermophilus and Lactococcus lactis are recognized as the most important microorganisms in the dairy industry, and they commonly isolated from traditional fermented dairy products. It has been reported that Lactococcus raffinolactis coupled with Lactococcus lactis could be used as a new starter culture in fermented milk due to their strong complementarity during the fermentation process (Kimotonira et al., 2012). However, it is worth noting that low relative abundances were detected for certain taxa in the present study, including the genera Acetobacter, Pseudomonas, and Chryseobacterium, and species Acinetobacter lwoffii, Acinetobacter johnsonii, Carnobacterium divergens, and Macrococcus caseolyticus. Acetobacter can oxidize alcohol or sugars incompletely to form acetic acid, which is widely found in traditional fermented foods (Ishida et al., 2005; Kiryu et al., 2012). Members of the genus Pseudomonas demonstrate broad metabolic diversity and consequently are able to colonize a wide range of niches. Pseudomonas is a dominant active bacterial contributor to spoilage under aerobic conditions, even at refrigeration temperatures (Ercolini et al., 2011), and some species are opportunistic human and plant pathogens (Palleroni, 2010). Some cold-tolerant Chryseobacterium species, including C. oranimense, C. haifense, and C. bovis, have been detected in raw milk in Israel (Hantsis-Zacharov and Halpern, 2007). Acinetobacter lwoffii and Acinetobacter johnsonii commonly occur in marine fish and water, and are regarded as opportunistic fish pathogens. Carnobacterium divergens, a psychrotrophic and microaerophilic but oxygen-tolerating bacterium (Leisner et al., 2007), is predominant in industrial foods and is frequently associated with the spoilage of refrigerated meat and fish products (Barakat et al., 2000). These potential pathogens may cross-contaminate via several pathways, including animal bodies, feces, and processing environments. We therefore suggest that herdsmen be vigilant with respect to sanitary conditions during dairy production.
It is well known that cow milk contains, on average, 87.3% water, 3.4% protein, 3.6% fat, and 4.6% lactose per 100 g (Guy and Fenaille, 2006). However, Boudonck et al. (2009) found that cow milk contains a complex mixture of metabolites, and they identified 223 metabolites by liquid and gas chromatography/mass spectrometry methods, including amino acids, lipids, carbohydrates, nucleotides, energy metabolites, vitamins, cofactors, and short peptides. Fermentation is a metabolic process leading to the release of different chemical substances as metabolites, and these metabolites result from the activity of the microorganisms present within the food, the concentration of which changes over time from the manufacture of a fermented food to its consumption. Therefore, more studies are now focusing on the detection of the metabolic fingerprints and bacterial communities of fermented foods to evaluate their quality (Lee et al., 2015; Young et al., 2016). To date, reports regarding the identification of metabolites in traditionally fermented sour cream and butter are limited. The current study detected 27,822 metabolites, and significant amounts of Lys-Lys, isohexanal, palmitic acid, Leu-Val, and 2′-deoxycytidine were detected in the Russian sour cream and butter samples. Palmitic acid, the most common saturated fatty acid, is naturally present in butter, cheese, milk, and meat, as well as cocoa butter, soybean oil, and sunflower oil. The World Health Organization indicated that consumption of palmitic acid increases the risk of developing cardiovascular disease and may increase LDL levels in the blood. Furthermore, it has been reported that palmitic acid has the strongest effect in boosting the metastatic potential of CD36+ metastasis-initiating cells (Pascual et al., 2016). In addition, 27 significantly different metabolites were detected between the sour cream and butter samples (Table 2). Because sour cream and butter possess rich bacterial resources and bacterial community diversity, the co-fermentation of all the strains present in the food products may lead to the metabolic differences between the sour cream and butter samples.
To further understand the correlation relationships between the most prevalent bacterial species and the main metabolites, correlation analysis was performed between the most prevalent bacterial species (average relative abundance of >0.5%) and 19 main metabolites in sour cream. The predominant species, L. lactis, was positively correlated with the 12 metabolites with higher relative abundances, including decanoic acid, palmitic acid, L-xylulose, isohexanal, and some short chain polypeptides, and significantly negatively correlated with some amino acids. Decanoic acid and palmitic acid have been detected in metabolic profiling of L. lactis subsp. cremoris MG1363 under different culture conditions (Azizan et al., 2012). Previous study on metabolic fingerprinting of bacterial metabolism in a model cheese also demonstrated some amino acids or volatile metabolites can be easily linked to L. lactis metabolism (Le Boucher et al., 2013). L. lactis has many nutritional requirements, including amino acid, which was the reason of some free amino acids negative with L. lactis. The results revealed that prevalent bacterial species were correlated with the main metabolites. However, detailed explanation of the correlation relationship between bacterial species and metabolites in sour cream should perform metagenomic sequencing and metabolic pathway analysis in future study.
We analyzed the bacterial communities and metabolic character of Russian sour cream and butter using a combination of SMRT sequencing techniques and UPLC–Q–TOF mass spectrometry. This study clearly showed that the bacterial community of sour cream and butter are diverse and abundant, and the bacterial compositions have differences between sour cream and butter samples. Meanwhile, a total of 27,822 metabolites were detected in all samples, and 27 significantly different metabolites were detected between the sour cream and butter samples, including short peptides, organic acids, and amino acids. Based on correlation analyses between the most prevalent bacterial species and the main metabolites in sour cream, we conclude that there may be a connection between the dominant LAB species and these metabolites. In addition, 48 strains were isolated by pure culture method and they were identified into seven genera and 15 species and/or subspecies. Those LAB isolates could be used to screen probiotic strains and design starter culture. In conclusion, this study combined omics techniques to analyze the bacterial diversity and metabolic character of traditional sour cream and butter, and we hope that our findings provide valuable information for industrial production of traditional fermented cream.
Author Contributions
WL and HZ designed the experiments. JY, XA, LM, and CY performed the experiments. LP and DR analyzed the data. JY and TT drafted the manuscript. All authors read and approved the final manuscript.
Funding
This research was supported by the Excellent Young Scientist Foundation of Inner Mongolia Agricultural University of China (Grant No. 2017XYQ-4), the China Agriculture Research System (Grant CARS-36) and the National Natural Science Foundation of China (Beijing, China; Grant No. 31671871). The funders had no role in study design, data collection and interpretation, or the decision to submit the work for publication.
Conflict of Interest Statement
The authors declare that the research was conducted in the absence of any commercial or financial relationships that could be construed as a potential conflict of interest.
Acknowledgments
The authors are indebted to the members of the Key Laboratory of Dairy Biotechnology and Engineering, Inner Mongolia Agri cultural University, Hohhot, for their technical supports.
Supplementary Material
The Supplementary Material for this article can be found online at: https://www.frontiersin.org/articles/10.3389/fmicb.2018.02496/full#supplementary-material
FIGURE S1 | Relative abundances and diversity of bacteria in the sour cream and butter samples at the genus (A) and species (B) level.
TABLE S1 | All species content of butter and sour cream samples.
Footnotes
- ^ http://www.megasoftware.net
- ^ https://www.r-project.org/
- ^ http://www.hmdb.ca/
- ^ www.genome.jp/kegg
- ^ www.chemspider.com
- ^ http://www.metlin.scripps.edu/
- ^ http://metagenomics.anl.gov/
References
Amann, R. I., Ludwig, W., and Schleifer, K. H. (1995). Phylogenetic identification of individual microbial cells without cultivation. Microbiol. Rev. 59, 143–169.
Azizan, K. A., Baharum, S. N., and Mohd, N. N. (2012). Metabolic profiling of Lactococcus lactis under different culture conditions. Molecules 17, 8022–8036. doi: 10.3390/molecules17078022
Barakat, R. K., Griffiths, M. W., and Harris, L. J. (2000). Isolation and characterization of Carnobacterium, Lactococcus, and Enterococcus spp. from cooked, modified atmosphere packaged, refrigerated, poultry meat. Int. J. Food Microbiol. 62, 83–94. doi: 10.1016/S0168-1605(00)00381-0
Boudonck, K. J., Mitchell, M. W., Wulff, J., and Ryals, J. A. (2009). Characterization of the biochemical variability of bovine milk using metabolomics. Metabolomics 5, 375–386. doi: 10.1007/s11306-009-0160-8
Caporaso, J. G., Bittinger, K., Bushman, F. D., Desantis, T. Z., Andersen, G. L., and Knight, R. (2010). PyNAST: a flexible tool for aligning sequences to a template alignment. Bioinformatics. 26, 266–267. doi: 10.1093/bioinformatics/btp636
Cole, J. R., Chai, B., Farris, R. J., Wang, Q., Kulamsyedmohideen, A. S., Mcgarrell, D. M., et al. (2007). The ribosomal database project (RDP-II): introducing myRDP space and quality controlled public data. Nucleic Acids Res. 35, D169–D172. doi: 10.1093/nar/gkl889
Dalmasso, A., Soto del Rio, M. D. L. D., Civera, T., Pattono, D., Cardazzo, B., and Bottero, M. T. (2016). Characterization of microbiota in Plaisentif cheese by high-throughput sequencing. LWT Food Sci. Technol. 69, 490–496. doi: 10.1016/j.lwt.2016.02.004
Delcenserie, V., Taminiau, B., Delhalle, L., Nezer, C., Doyen, P., Crevecoeur, S., et al. (2014). Microbiota characterization of a Belgian protected designation of origin cheese, Herve cheese, using metagenomic analysis. J. Dairy Sci. 97, 6046–6056. doi: 10.3168/jds.2014-8225
Duniere, L., Xu, S., Long, J., Elekwachi, C., Wang, Y., Turkington, K., et al. (2017). Bacterial and fungal core microbiomes associated with small grain silages during ensiling and aerobic spoilage. BMC Microbiol. 17:50. doi: 10.1186/s12866-017-0947-0
Edgar, R. C. (2010). Search and clustering orders of magnitude faster than BLAST. Bioinformatics. 26, 2460–2461. doi: 10.1093/bioinformatics/btq461
Elionora Hantsis-Zacharov, M. H. (2007). Culturable psychrotrophic bacterial communities in raw milk and their proteolytic and lipolytic traits. Appl. Environ. Microb. 73, 7162–7168. doi: 10.1128/AEM.00866-07
Ercolini, D., Ferrocino, I., Nasi, A., Ndagijimana, M., Vernocchi, P., La Storia, A., et al. (2011). Monitoring of microbial metabolites and bacterial diversity in beef stored under different packaging conditions. Appl. Environ. Microb. 77, 7372–7381. doi: 10.1128/AEM.05521-11
Feurer, C., Vallaeys, T., Corrieu, G., and Irlinger, F. (2004). Does smearing inoculum reflect the bacterial composition of the smear at the end of the ripening of a french soft, red-smear cheese? J. Dairy Sci. 87, 3189–3197. doi: 10.3168/jds.S0022-0302(04)73454-2
Gesudu, Q., Zheng, Y., Xi, X. X., Hou, Q. C., Xu, H. Y., Huang, W. Q., et al. (2016). Investigating bacterial population structure and dynamics in traditional koumiss from inner Mongolia using single molecule real-time sequencing. J. Dairy Sci. 99, 7852–7863. doi: 10.3168/jds.2016-11167
Giannino, M. L., Marzotto, M., Dellaglio, F., and Feligini, M. (2009). Study of microbial diversity in raw milk and fresh curd used for Fontina cheese production by culture-independent methods. Int. J. Food Microbiol. 130, 188–195. doi: 10.1016/j.ijfoodmicro.2009.01.022
Guy, P. A., and Fenaille, F. (2006). Contribution of mass spectrometry to assess quality of milk-based products. Mass Spectrome. Rev. 25, 290–326. doi: 10.1002/mas.20074
Hantsis-Zacharov, E., and Halpern, M. (2007). Chryseobacterium haifense sp. nov., a psychrotolerant bacterium isolated from raw milk. Int. J. Syst. Evol. Microbiol. 57, 2344–2348. doi: 10.1099/ijs.0.65115-0
Hou, Q., Xu, H., Zheng, Y., Xi, X., Kwok, L. Y., Sun, Z., et al. (2015). Evaluation of bacterial contamination in raw milk, ultra-high temperature milk and infant formula using single molecule, real-time sequencing technology. J. Dairy Sci. 98, 8464–8472. doi: 10.3168/jds.2015-9886
Ishida, T., Yokota, A., Umezawa, Y., Toda, T., and Yamada, K. (2005). Identification and characterization of lactococcal and acetobacter strains isolated from traditional Caucasusian fermented milk. J. Nutr. Sci. Vitaminol. 51, 187–193. doi: 10.3177/jnsv.51.187
Jin, H., Mo, L., Pan, L., Hou, Q., Li, C., Darima, I., et al. (2018). Using PacBio sequencing to investigate the bacterial microbiota of traditional Buryatian cottage cheese and comparison with Italian and Kazakhstan artisanal cheeses. J. Dairy Sci. 101, 6885–6896. doi: 10.3168/jds.2018-14403
Kimotonira, H., Aoki, R., Mizumachi, K., Sasaki, K., Naito, H., Sawada, T., et al. (2012). Interaction between Lactococcus lactis and Lactococcus raffinolactis during growth in milk: development of a new starter culture. J. Dairy Sci. 95, 2176–2185. doi: 10.3168/jds.2011-4824
Kiryu, T., Yamauchi, K., Masuyama, A., Ooe, K., Kimura, T., Kiso, T., et al. (2012). Optimization of lactobionic acid production by Acetobacter orientalis isolated from Caucasian fermented milk, “Caspian Sea yogurt”. Biosci. Biotechnol. Biochem. 76, 361–363. doi: 10.1271/bbb.110608
Le Boucher, C., Courant, F., Jeanson, S., Chereau, S., Maillard, M. B., Royer, A. L., et al. (2013). First mass spectrometry metabolic fingerprinting of bacterial metabolism in a model cheese. Food Chem. 141, 1032–1040. doi: 10.1016/j.foodchem.2013.03.094
Lee, S. H., Ji, Y. J., and Che, O. J. (2015). Bacterial community dynamics and metabolite changes in myeolchi-aekjeot, a korean traditional fermented fish sauce, during fermentation. Int. J. Food Microbiol. 203, 15–22. doi: 10.1016/j.ijfoodmicro.2015.02.031
Leisner, J. J., Laursen, B. G., Prévost, H., Drider, D., and Dalgaard, P. (2007). Carnobacterium: positive and negative effects in the environment and in foods. FEMS Microbiol. Rev. 31, 592–613. doi: 10.1111/j.1574-6976.2007.00080.x
Li, J., Zheng, Y., Xu, H., Xi, X., Hou, Q., Feng, S., et al. (2017). Bacterial microbiota of Kazakhstan cheese revealed by single molecule real time (SMRT) sequencing and its comparison with Belgian, Kalmykian and Italian artisanal cheeses. BMC Microbiol. 17:13. doi: 10.1186/s12866-016-0911-4
Liu, W., Zheng, Y., Kwok, L. Y., Sun, Z., Zhang, J., Guo, Z., et al. (2015). High-throughput sequencing for the detection of the bacterial and fungal diversity in Mongolian naturally fermented cow’s milk in Russia. BMC Microbiol. 15:45. doi: 10.1186/s12866-015-0385-9
Lozupone, C., Hamady, M., and Knight, R. (2006). UniFrac – An online tool for comparing microbial community diversity in a phylogenetic context. BMC Bioinformatics 7:371. doi: 10.1186/1471-2105-7-371
Mathara, J. M., Schillinger, U., Kutima, P. M., Mbugua, S. K., and Holzapfel, W. H. (2004). Isolation, identification and characterisation of the dominant microorganisms of kule naoto: the Maasai traditional fermented milk in Kenya. Int. J. Food Microbiol. 94, 269–278. doi: 10.1016/j.ijfoodmicro.2004.01.008
Mosher, J. J., Bowman, B., Bernberg, E. L., Shevchenko, O., Kan, J., Korlach, J., et al. (2014). Improved performance of the PacBio SMRT technology for 16S rDNA sequencing. J. Microbiol. Meth. 104, 59–60. doi: 10.1016/j.mimet.2014.06.012
Palleroni, N. J. (2010). The Pseudomonas story. Environ. Microbiol. 12, 1377–1383. doi: 10.1111/j.1462-2920.2009.02041.x
Pan, L., Yu, J., Mi, Z., Mo, L., Jin, H., Yao, C., et al. (2018). A metabolomics approach uncovers differences between traditional and commercial dairy products in Buryatia (Russian Federation). Molecules 23:E735. doi: 10.3390/molecules23040735
Pascual, G., Avgustinova, A., Mejetta, S., Martín, M., Castellanos, A., Attolini, C. S., et al. (2016). Targeting metastasis-initiating cells through the fatty acid receptor CD36. Nature 541, 41–45. doi: 10.1038/nature20791
Price, M. N., Dehal, P. S., and Arkin, A. P. (2009). FastTree: computing large minimum evolution trees with profiles instead of a distance matrix. Mol. Biol. Evol. 26, 1641–1650. doi: 10.1093/molbev/msp077
Quigley, L., O’Sullivan, O., Beresford, T. P., Ross, R. P., Fitzgerald, G. F., and Cotter, P. D. (2012). High-throughput sequencing for detection of subpopulations of bacteria not previously associated with artisanal cheeses. Appl. Environ. Microb. 78, 5717–5723. doi: 10.1128/AEM.00918-12
Xu, H., Liu, W., Gesudu, Q., Sun, Z., Zhang, J., Guo, Z., et al. (2015). Assessment of the bacterial and fungal diversity in home-made yoghurts of Xinjiang, China by pyrosequencing. J. Sci. Food Agric. 95, 2007–2015. doi: 10.1002/jsfa.6912
Young, J. J., Jung, L. H., Hee, C. B., and Ok, J. C. (2016). Effects of temperature on bacterial communities and metabolites during fermentation of myeolchi-aekjeot, a traditional korean fermented anchovy sauce. PLoS One 11:e0151351. doi: 10.1371/journal.pone.0151351
Yu, J., Wang, H. M., Menghe, B. L. G., Jiri, M. T., Wang, H. M., Liu, W. J., et al. (2011). Diversity of lactic acid bacteria associated with traditional fermented dairy products in Mongolia. J. Dairy Sci. 94, 3229–3241. doi: 10.3168/jds.2010-3727
Yu, J., Wang, H. M., Zha, M. S., Qing, Y. T., Bai, N., Ren, Y., et al. (2015). Molecular identification and quantification of lactic acid bacteria in traditional fermented dairy foods of Russia. J. Dairy Sci. 98, 5143–5154. doi: 10.3168/jds.2015-9460
Keywords: Buryatia, sour cream, butter, bacterial microbiota, metabolic character
Citation: Yu J, Mo L, Pan L, Yao C, Ren D, An X, Tsogtgerel T, Zhang H and Liu W (2018) Bacterial Microbiota and Metabolic Character of Traditional Sour Cream and Butter in Buryatia, Russia. Front. Microbiol. 9:2496. doi: 10.3389/fmicb.2018.02496
Received: 22 June 2018; Accepted: 28 September 2018;
Published: 22 October 2018.
Edited by:
Chunbao Li, Nanjing Agricultural University, ChinaReviewed by:
Lianzhong Ai, University of Shanghai for Science and Technology, ChinaYujun Jiang, Northeast Agricultural University, China
Copyright © 2018 Yu, Mo, Pan, Yao, Ren, An, Tsogtgerel, Zhang and Liu. This is an open-access article distributed under the terms of the Creative Commons Attribution License (CC BY). The use, distribution or reproduction in other forums is permitted, provided the original author(s) and the copyright owner(s) are credited and that the original publication in this journal is cited, in accordance with accepted academic practice. No use, distribution or reproduction is permitted which does not comply with these terms.
*Correspondence: Wenjun Liu, d2psaXUxNjhAMTYzLmNvbQ==