- 1BioTechnology Institute, University of Minnesota, St. Paul, MN, United States
- 2Bioinformatics and Computational Biology, University of Minnesota, Minneapolis, MN, United States
- 3Division of Environmental Engineering, Graduate School of Engineering, Hokkaido University, Sapporo, Japan
- 4Center for Life Science Research, University of Yamanashi, Yamanashi, Japan
- 5National Institute of Polar Research, Tokyo, Japan
- 6Department of Computer Science and Engineering, University of Minnesota, Minneapolis, MN, United States
- 7Department of Soil, Water, and Climate, University of Minnesota, St. Paul, MN, United States
Current approach to identify sources of human pathogens is largely dependent on the cultivation and isolation of target bacteria. For rapid pathogen source identification, culture-independent strain typing method is necessary. In this study, we designed new primer set that broadly covers flaA short variable region (SVR) of various Campylobacter species, and applied the flaA SVR sequencing method to examine the diversity of Campylobacter spp. in geese fecal samples (n = 16) with and without bacteria cultivation. Twenty-three Campylobacter strains isolated from the 16 geese fecal samples were grouped similarly by conventional flaA restriction fragment length polymorphism (RFLP) method and by the flaA SVR sequencing method, but higher discriminant power was observed in the flaA SVR sequencing approach. For culture-independent flaA SVR sequencing analysis, we developed and optimized the sequence data analysis pipeline to identify as many genotypes as possible, while minimizing the detection of genotypes generated by sequencing errors. By using this pipeline, 51,629 high-quality flaA sequence reads were clustered into 16 operational taxonomic units (=genotypes) by using 98% sequence similarity and >50 sequence duplicates. Almost all flaA genotypes obtained by culture-dependent method were also identified by culture-independent flaA SVR MiSeq sequencing method. In addition, more flaA genotypes were identified probably due to high throughput nature of the MiSeq sequencing. These results suggest that the flaA SVR sequencing could be used to analyze the diversity of Campylobacter spp. without bacteria isolation. This method is promising to rapidly identify potential sources of Campylobacter pathogens.
Introduction
Campylobacter species are one of the most common bacterial foodborne pathogens, causing gastrointestinal disorders such as bloody diarrhea, dysentery syndrome, and cramps (Silva et al., 2011). Campylobacter is estimated to cause more than two million illnesses, 13,000 hospitalizations, and >100 deaths each year in the United States (Silva et al., 2011), and the incidence of Campylobacter illness has been increased by 13% since 2006–2008 (Crim et al., 2015). The main source of the Campylobacter infection is the consumption of contaminated water and foods, especially poultry products (Silva et al., 2011).
Although the most common hosts for Campylobacter spp. are avian species, including commercial poultry species (e.g., chickens and turkeys) and waterfowl (e.g., geese; Pacha et al., 1988), they are also found in other animals such as cattle, pigs, and dairy cows (Westrell et al., 2009; Silva et al., 2011). Campylobacter species cannot grow but can survive well in various environments such as fresh and coastal waters and sediment after they are released from their primary hosts (e.g., birds and animals). Thus, the environments can act as self-perpetuating reservoir of Campylobacter infection cycling between humans, wildlife, and domestic animals (Jones, 2001). In fact, Campylobacter spp. is frequently and abundantly detected in various environmental water samples (Ishii et al., 2014a; Oster et al., 2014; Zhang et al., 2016).
To protect human health, it is important to monitor levels of Campylobacter in food and water samples, and identify the sources of pathogens. While pathogen monitoring can be done by culture-independent quantitative PCR (qPCR) method (Ishii et al., 2013; Banting et al., 2016; Zhang et al., 2016), current pathogen source identification methods largely depend cultivation and isolation of pathogens. Various genotyping methods have been used to identify sources of Campylobacter strains, including pulsed-field gel electrophoresis (PFGE), restriction fragment length polymorphism (RFLP), multilocus sequence typing (MLST), antimicrobial resistance profiling, the CRISPR1 typing, comparative genomic fingerprinting, and flaA short variable region (SVR) sequencing (Taboada et al., 2013; Duarte et al., 2016; Calleros et al., 2017). Although these methods are useful in discriminating different Campylobacter strains, some of them require time and labor, while others do not have enough discriminating power. In addition, all methods are designed to characterize isolated Campylobacter strains, which is time and labor intensive (On, 2013). Sometimes Campylobacter cells enter viable-but-non-culturable (VBNC) state and become unavailable for isolation (Tholozan et al., 1999). Therefore, for rapid and comprehensive identification of Campylobacter sources, culture-independent strain typing method is desired.
Here, we propose to use culture-independent flaA SVR sequencing to rapidly identify Campylobacter genotypes in environmental samples. The objectives of this study were to (1) design new primer set that broadly covers flaA SVR of various Campylobacter species, (2) compare strain discrimination obtained by the flaA SVR sequencing done with the new primers with those obtained by a commonly used flaA RFLP method, and (3) apply the flaA SVR sequencing to examine the diversity of Campylobacter spp. in geese fecal samples without bacteria cultivation.
Materials and Methods
Geese Fecal Samples
Fecal samples from greater white-fronted goose (Anser albifrons) were collected from agricultural fields near Lake Miyajimanuma, Hokkaido, Japan, in September and October 2013. Details of the sampling location are described elsewhere (Ishii et al., 2014a). The collected geese fecal samples (n = 77) were stored in Whirl-Pak bags and kept on the ice during the transportation (<2 h). DNA was directly extracted from 0.2 g of geese fecal samples by using a QIAamp DNA Stool Mini kit (Qiagen). DNA samples were stored at −20°C until use.
Campylobacter Strains
Campylobacter sp. strains were isolated from geese fecal samples as described below. One gram of fecal sample was added to 9 ml phosphate-buffered saline (PBS) gelatin buffer in a 15-ml centrifuge tube (Hamilton et al., 2010) and vigorously mixed for 30–45 s; 1 ml of the fecal suspension was transferred to 9 ml of Bolton broth (Oxoid) supplemented with laked horse blood (Remel) and antibiotics (20 μg/ml cefoperazone, 20 μg/ml trimethoprim, 20 μg/ml vancomycin, and 50 μg/ml cyclohexamide). The tubes were placed in microaerobic conditions created using AnaeroPack MicroAero (Mitsubishi Gas Chemical), and incubated at 37°C for 4 h followed by 42°C for 44 h. After incubation, a loopful culture (ca. 10 μl) was streaked onto modified Campylobacter blood-free agar (m-CCDA) medium (Oxoid) supplemented with 32 μg/ml cefoperazone and 10 μg/ml amphotericin B, and incubated under microaerobic conditions at 42°C for 48 h. Colonies formed on the m-CCDA medium were re-streaked on a new m-CCDA medium, and incubated under microaerobic conditions at 42°C for 48 h.
Colonies were confirmed as being Campylobacter species by sequencing the 16S rRNA gene sequences. In brief, DNA were extracted from well-isolated single colonies formed on the m-CCDA medium, by heating cells in 100 μl 0.05 M NaOH at 95°C for 15 min. After centrifugation, the supernatant was diluted 10-fold in MilliQ water, and used for PCR done with C412F and C1228R primers (Linton et al., 1996) as described previously (Ishii et al., 2006). PCR products were purified and sequenced as described previously (Ishii et al., 2016). Campylobacter jejuni JCM 2013, Campylobacter coli JCM 2529T, and Campylobacter lari JCM 14870T were used for quality control of growth media and PCR.
flaA RFLP
The flaA RFLP was performed to genotype Campylobacter strains. The flaA fragments (ca. 1,800 bp) were PCR-amplified using flaA-F (5′-ATGGGATTTCGTATTAACAC-3′) and flaA-R (5′-CTGTAGTAATCTTAAAACATTTTG-3′) primers (Wassenaar and Newell, 2000). The PCR reaction mixture (20 μl) contained 1 × Ex Taq buffer (Takara Bio), 0.2 μM of each primer, 0.2 mM of each dNTP, 0.4 U of Ex Taq DNA polymerase (Takara Bio), and 1 μl of DNA template. PCR was performed using a Veriti 96-well thermal cycler (ThermoFisher Scientific) with the following conditions: initial annealing at 95°C for 5 min, followed by 35 cycles of 95°C for 30 s, 55°C for 30 s, and 72°C for 2 min. After final extension at 72°C for 7 min, the PCR mixtures were stored at 4°C.
The PCR products (7 μl) were individually digested with Dde I and Hinf I (Takara Bio) according to the manufacturer’s instruction. Digested fragments were separated by 1.5% agarose gel electrophoresis. Gels were stained with GelRed (Biotium, Hayward, United States) and visualized under UV light. Sizes of the DNA fragments were calculated based on the external size standards (i.e., 100-bp DNA ladder) by using BioNumerics software ver. 6.6. Dendrogram was constructed using Jaccard similarity and the unweighted pair group method with arithmetic means (UPGMA) clustering method.
flaA SVR Sequencing for Pure Culture Strains
A new primer set was designed using DegePrime (Hugerth et al., 2014) to broadly cover flaA SVR of various Campylobacter species. About 400-bp flaA fragment was amplified using Campy_flaA_235F (5′-GATAARGCWATGGATGAGCA-3′) and Campy_flaA_635R (5′-CHGTYCCWACWGAAGTWGAA-3′; Supplementary Figure S1). The PCR reaction mixture (20 μl) contained 1 × Ex Taq buffer (Takara Bio), 0.5 μM of each primer, 0.2 mM of each dNTP, 0.4 U of Ex Taq DNA polymerase (Takara Bio), and 1 μl of DNA template. PCR was performed using a Veriti 96-well thermal cycler with the following conditions: initial annealing at 95°C for 5 min, followed by 35 cycles of 95°C for 30 s, 52°C for 30 s, and 72°C for 30 s. After final extension at 72°C for 7 min, the PCR mixtures were stored at 4°C. The PCR products were purified using the FastGene Gel/PCR Purification Kit (Nippon Genetics) and sequenced bidirectionally using the BigDye Terminator v3.1 Cycle Sequencing Kit (ThermoFisher Scientific) and ABI 3730xl capillary sequencer (ThermoFisher Scientific). The resulting nucleotide sequences were assembled using Phred-Phrap program (Ewing et al., 1998).
flaA SVR Sequencing for Environmental Samples
To sequence flaA SVR from environmental samples, MiSeq sequence library was prepared. In brief, flaA SVR was amplified using the flaA-specific primers tagged with Illumina overhang adaptor sequences: Campy_flaA_235F_adaptor, 5′-tcgtcggcagcgtcagatgtgtataagagacagGATAARGCWATGGATGAGCA-3′ and Campy_flaA_635R_adaptor, 5′-gtctcgtgggctcggagatgtgtataagagacagCHGTYCCWACWGAAGTWGAA-3′ where Illumina overhang adaptor sequences are shown in lower case. Optimal number of PCR cycles was determined by qPCR. The qPCR reaction mixture (10 μl) contained 1 × SYBR Premix Ex Taq (Tli RNase H plus) ROX plus (Takara Bio), 0.4 μM of each primer, and 1 μl of DNA template. PCR was performed using a StepOnePlus Real-Time PCR System (ThermoFisher Scientific) with the following conditions: initial annealing at 95°C for 30 s, followed by 30–40 cycles of 95°C for 5 s, 58°C for 30 s, and 72°C for 30 s. The cycle numbers were set to reach the log-linear phase of the PCR kinetics based on the qPCR results to minimize PCR-dependent biases during amplification (Ishii et al., 2014b). The PCR products were purified using the LaboPass PCR Kit (Cosmo Genetech).
The purified PCR products were tagged with sample-unique index and Illumina adapter sequences at their 5′ end (Nextera XT index Kit v2; Illumina) by PCR. The PCR reaction mixture (25 μl) contained 1 × KAPA HiFi HS ReadyMix (Kapa Biosystems), 2.5 μl each forward and reverse primers, and 2.5 μl of the purified first PCR products. The PCR was performed under the following conditions: 95°C for 3 min, 8 cycles of 95°C for 30 s, 55°C for 30 s, and 72°C for 30 s, and 72°C for 5 min. After agarose gel electrophoresis, PCR products (ca. 500 bp) were excised from the gel and purified using the FastGene Gel/PCR Purification Kit. Tagged amplicons were pooled and 8 pmol of the pooled amplicons were sequenced by Illumina MiSeq platform in 300 bp paired-end sequencing reaction with v3 reagent kit (Illumina) according to the manufacturer’s instruction. PhiX Control v3 (Illumina) was added at 20% of the input DNA amount as a quality control for the MiSeq run.
Analysis of flaA SVR Sequencing
The raw MiSeq sequencing reads were processed with the SHI7 program (Al-Ghalith et al., 2018). The scripts are available at GitHub1. In brief, Illumina adaptor and primer sequences were removed, and paired-end sequences were assembled. The resulting sequences were further filtered by size of the expected flaA amplicon length (355–370 bp) and by removing singletons. Sequence denoising was done using two parameters: number of duplicated reads (D) and the sequence alignment similarity (S) of operational taxonomic units (OTUs). Specifically, we systematically varied D (1, 2, 3, 5, 10, 25, 50, and 100 reads) and S values (97, 97.25, 97.5, 97.75, 98, 98.25, 98.5, 98.75, and 99%) to identify the largest number of duplicates and the highest sequence alignment similarity to cluster sequences to OTUs that represent >95% of all length-trimmed amplicon data. In this study, optimizing this criterion led to the selection of 98% alignment similarity and 50 duplicates. These optimized parameters were used to align all of the trimmed amplicon data to the representative set with the exhaustive BURST aligner (Al-Ghalith and Knights, 2017).
The flaA nucleotide sequences were aligned using ClustalW, and used to generate a phylogenetic tree. Phylogenetic trees were constructed based on the maximum likelihood, maximum parsimony, and neighbor-joining methods by using MEGA ver. 6.06 (Tamura et al., 2013).
Nucleotide Sequence Accession Numbers
The flaA sequences of Campylobacter strains identified in this study were deposited to DDBJ/Emble/Genbank databases under accession numbers MG694324-MG694346. MiSeq data were deposited to DDBJ/Emble/Genbank Short Read Archive (SRA) under accession number SRP127268.
Results
Comparison Between flaA RFLP and flaA SVR Sequencing
A total of 23 Campylobacter strains were isolated from 16 geese fecal samples, and were characterized by flaA RFLP and flaA SVR sequencing. The Campylobacter strains were grouped similarly by the two methods (Figure 1). Based on the flaA RFLP and flaA SVR sequencing, 23 Campylobacter strains were clustered to 14 and 13 groups, respectively. When excluding singletons, five and four groups were formed by the flaA RFLP and flaA SVR sequencing, respectively. Same strains were grouped together by both methods, except Strains 13.166 and 13.174. While Strain 13.166 was a member of Group (i) by flaA SVR sequencing, this strain was clustered differently by the flaA RFLP. Similarly, Strain 13.174 was grouped differently between the flaA SVR sequencing and the flaA RFLP. This is probably due to the insufficient fragment digestion by restriction endonucleases. When we summed the size of the digested fragments, it exceeds the size of the original PCR product (ca. 1.8 kbp) in both Strains 13.166 and 13.174, suggesting the occurrence of insufficient fragment digestion. The same results were obtained when we repeated the restriction digestion with extended incubation. To verify the enzyme recognition sites, we sequenced the entire 1.8 kbp fragments of flaA of Strains 13.166 and 13.174, and digested in silico by using NEBcutter V2.0 software2. Based on the in silico flaA RFLP done with the sequenced flaA data (Supplementary Figure S2), Strains 13.166 and 13.172 were confirmed to belong to Group (i) and (ii), respectively, confirming that the different results obtained by the two methods were due to insufficient fragment digestion during the RFLP experiment.
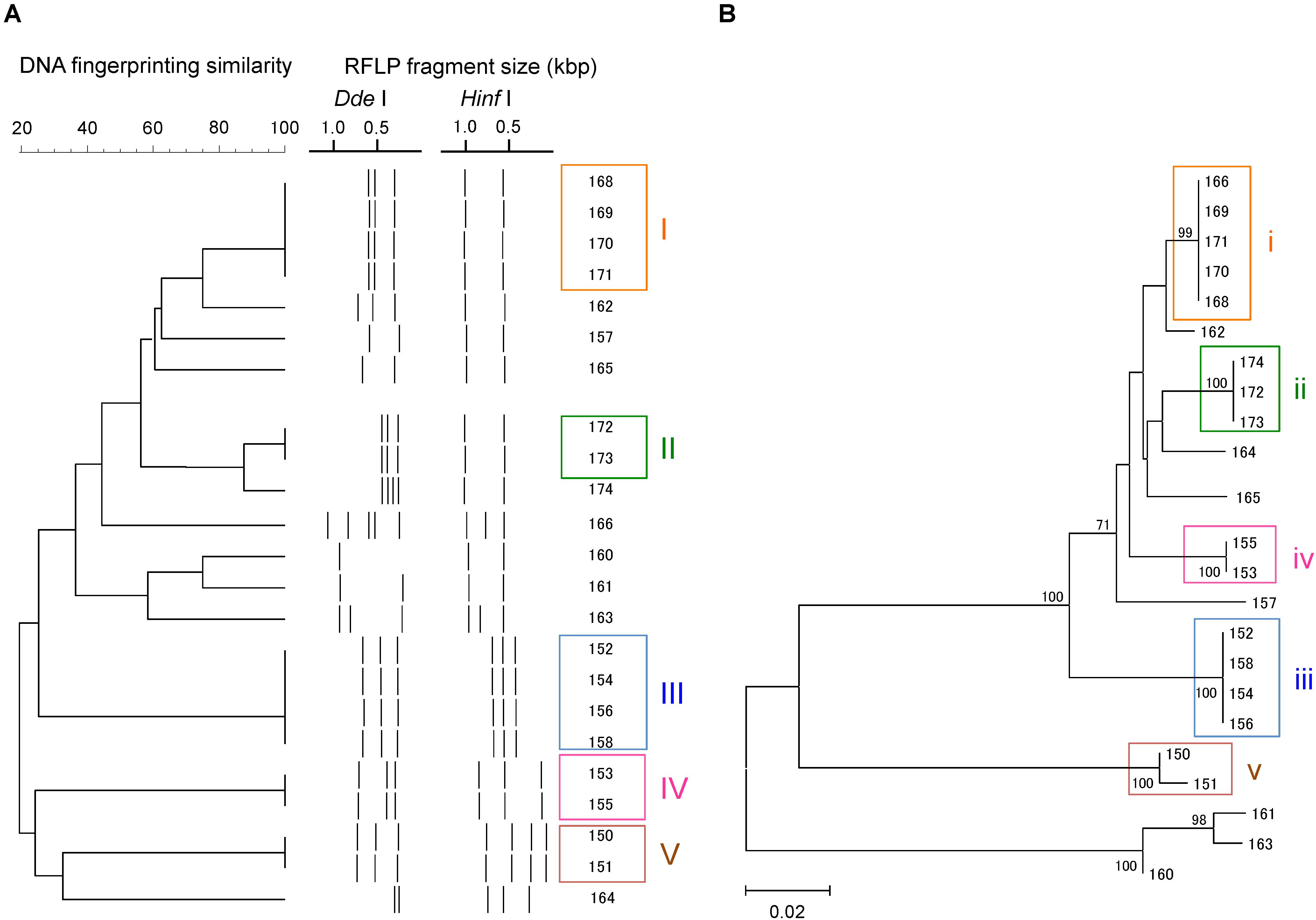
FIGURE 1. Clustering patterns of the Campylobacter strains isolated in this study, based on (A) flaA RFLP and (B) flaA SVR sequencing. Clustering was done using UPGMA method for flaA RFLP, while maximum likelihood method was used for flaA SVR sequencing.
We also observed that some strains that were indistinguishable by flaA RFLP could be separated by flaA SVR sequencing. For example, Strains 13.150 and 13.151 were 100% identical based on the flaA RFLP, although these strains were distinguished by flaA SVR. This is probably due to the presence of base mismatches in the flaA SVR region that could not be recognized by the restriction endonucleases, suggesting the higher discriminant power of flaA SVR than flaA RFLP.
Quantity of flaA in Geese Samples
By using SYBR Green assay, we could quantitatively detect flaA in environmental samples. The PCR efficiency was 66.7% with a linear dynamic range from 20 to 2 × 106 copies/μl. We detected flaA in 18.2% of geese fecal samples (14 out of 77 samples), with average density of 4.06 log10 copies/g feces. Sixteen out of 77 samples were positive for Campylobacter isolates; however, only five out of these 16 samples (31.3%) were positive for flaA qPCR (Supplementary Table S1). Nine samples were negative for Campylobacter isolates, but were positive for flaA qPCR. Densities of Campylobacter in isolate-positive and -negative fecal samples were 4.07 and 4.05 log10 copies/g feces and were not significantly different. Quantification limit of flaA in fecal samples was 3 log10 copies/g feces.
Campylobacter Diversity Assessed by High-Throughput flaA SVR Sequencing
Our flaA SVR sequencing done with MiSeq identified great diversity of Campylobacter spp. in geese fecal samples. More than 16.6 M reads/run were obtained, but only sequences from the 16 geese fecal samples were used in this study. A total of 51,629 high-quality sequences with average Phred quality score of 37 and sequence length 355–370 bp were obtained from 16 geese fecal samples. By using our newly developed sequence data analysis pipeline, 49,135 out of 51,629 sequences (95.2%) were clustered into 16 OTUs. Most of these OTUs (81%; 13 out of 16 OTUs) were commonly detected in multiple fecal samples (Figure 2). Some of them (e.g., OTUs denovo_7, denovo_12, denovo_13, and denovo_14) appeared in multiple fecal samples collected at different sampling dates (September 24th and October 7th, 2013), suggesting that these flaA genotypes were common and indigenous member of geese gut flora.
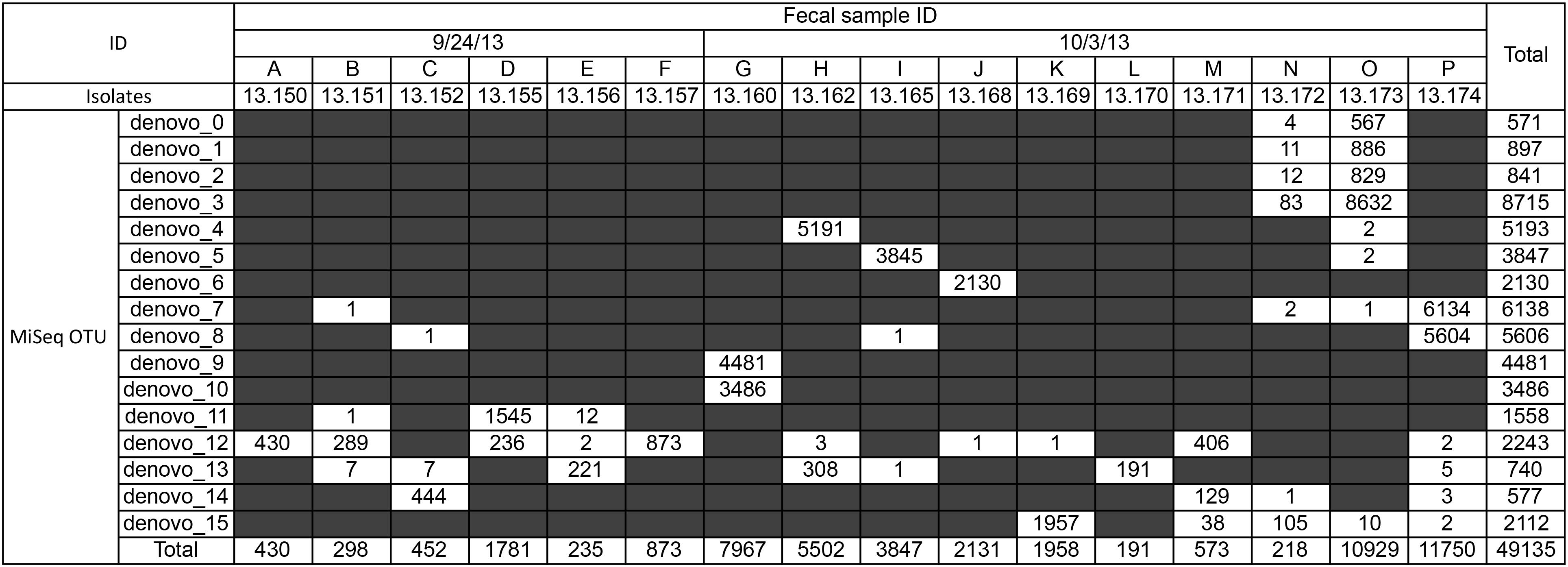
FIGURE 2. Number of sequence reads for each flaA operational taxonomic units (OTUs) in each fecal sample. Sequences were obtained by MiSeq analysis, and clusterd into OTU by using the SHI7 program (Al-Ghalith et al., 2018). Strain ID isolated from each fecal sample is also shown.
To compare the relatedness of the flaA of the isolated Campylobacter strains and those obtained by MiSeq, we constructed a phylogenetic tree (Figure 3). Almost all flaA genotypes obtained by culture-dependent method were also detected by culture-independent flaA SVR MiSeq sequencing, suggesting that the flaA SVR sequencing could be used to analyze the diversity of Campylobacter spp. without bacteria isolation. More flaA genotypes were identified by MiSeq probably because of the larger number of sequence reads in MiSeq. It is important to note that some flaA sequences obtained by MiSeq (five OTUs) were closely related to those of Helicobacter spp., which were not recovered by our isolation procedure.
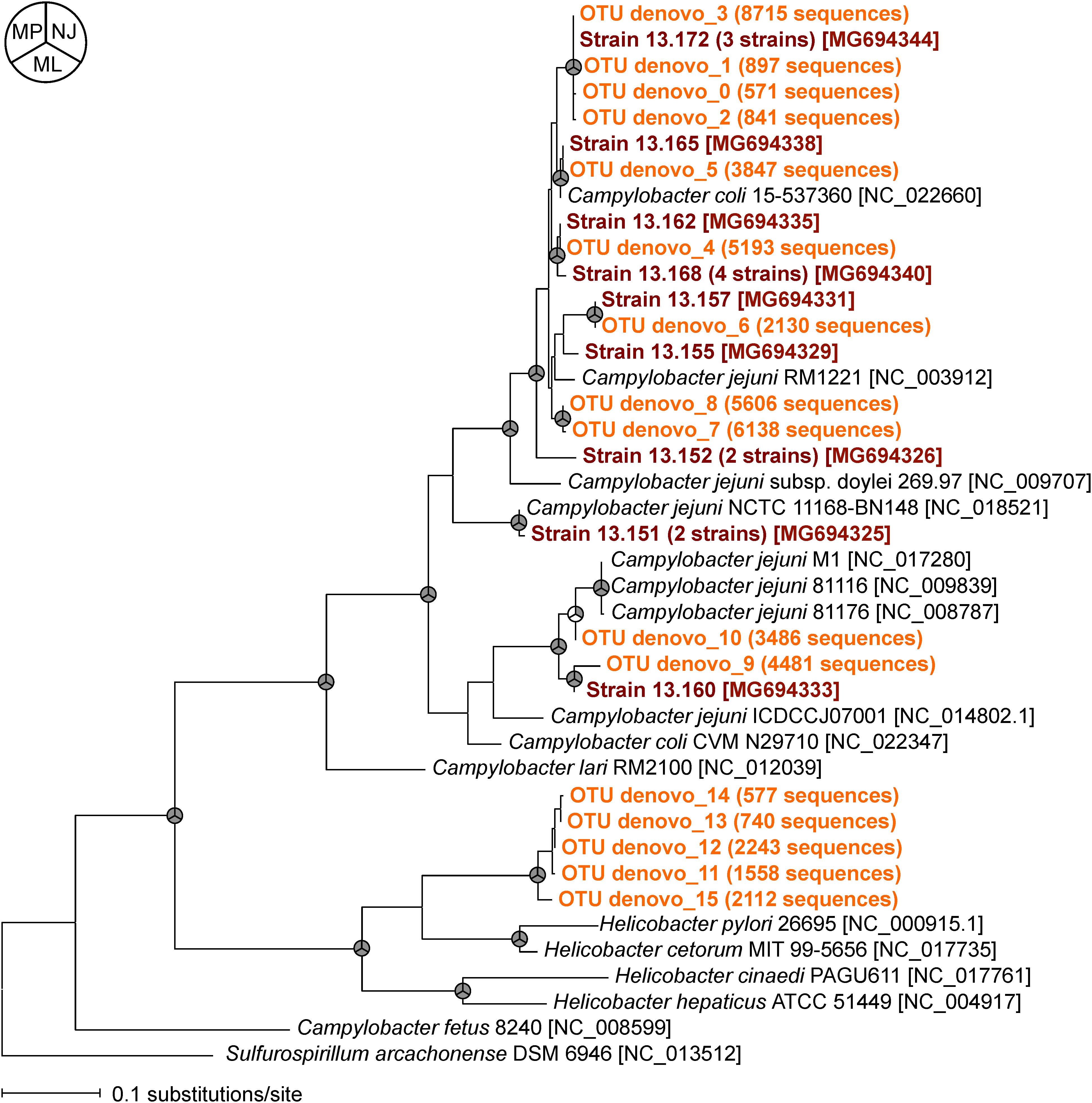
FIGURE 3. Phylogenetic relationships between flaA SVR sequences obtained by culture-dependent method (shown in brown) and those obtained by culture-independent MiSeq analysis (shown in orange). Phylogenetic tree constructed by maximum likelihood (ML) method is shown here, but similar trees were obtained by using neighbor-joining (NJ) and maximum parsimony (MP) methods. The nodes supported by bootstrap values (>70%) from 1000 replicates in the phylogenetic trees constructed by NJ, ML, and MP methods are indicated as gray in the pie charts next to the branches. The accession numbers of the reference strains in the DDBJ/EMBL/GenBank databases are indicated in brackets.
Discussion
Culture-independent flaA SVR sequencing could provide high-throughput information of Campylobacter diversity. To show this, we first designed a new primer set targeting flaA SVR to identify and differentiate Campylobacter strains, and compared the results with those obtained by a conventional DNA fingerprinting method. Then we applied the flaA SVR sequencing approach to analyze diversity of Campylobacter in geese fecal samples without bacteria isolation.
Similar degree of discrimination was identified between the flaA SVR sequencing and flaA RFLP methods. However, flaA RFLP could mistakenly assign some identical Campylobacter strains to a different group most likely due to insufficient fragment digestion. We also observed that some strains that were indistinguishable by flaA RFLP could be separated by flaA SVR sequencing. Our results were in agreement with previous comparative studies between DNA fingerprinting and sequencing genotyping methods. DNA sequencing methods such as those targeting rpoB, slpA, and set genes had higher reproducibility and higher discriminant power than DNA fingerprinting (Li et al., 2009). Therefore, the flaA SVR sequencing done with the new primers could be used as an alternative method to flaA RFLP to genotype Campylobacter strains.
The flaA SVR sequencing could be also used to analyze the diversity of Campylobacter spp. without bacteria isolation. For this purpose, we developed and optimized the sequence data analysis pipeline. Since our application purpose of flaA SVR sequencing was to identify genotypes of Campylobacter strains, not to compare Campylobacter populations among different samples, commonly used microbiome data analysis pipeline (e.g., Qiime; Caporaso et al., 2010) does not work well. We tried to recover as many genotypes (=OTUs) as possible, while removing genotypes generated by sequencing errors as much as possible. For this purpose, we used two parameters (D and S) to remove sequencing errors and identify OTUs. The optimized values for these parameters can vary by samples and target gene sequences. In our dataset, we identified that OTUs that contained >50 sequence reads (i.e., D = 50) at >98% alignment similarity (i.e., S = 98) represented >95% of all length-trimmed amplicon data (Supplementary Table S2). In general, increasing similarity value (S) provides increased number of OTUs; however, it also lowers the number of required duplicates (D) to represent >95% of all length-trimmed amplicon data. OTUs that contain small number of sequence reads (i.e., small D value) have higher chance of being generated by sequencing errors. Therefore, optimal S and D values should be determined based on the balance between resolution power and confidence required in a particular study.
Detection of same flaA genotype by both culture-dependent and -independent MiSeq approaches supports the usefulness of the flaA SVR sequencing for a rapid Campylobacter genotyping in environmental samples. Some flaA sequences obtained by MiSeq were closely related to those of Helicobacter spp. We designed a new primer set that can detect flaA SVR from Campylobacter spp. more broadly than the previous primers (Meinersmann et al., 1997), but it unexpectedly detected some flaA from Helicobacter spp. Our primers have several base mismatches to the flaA of Helicobacter spp. in the database; however, the database strains did not include Helicobacter strains isolated from geese. Helicobacter spp. were abundantly present in geese fecal samples (Green et al., 2012), and probably these Helicobacter strains may have flaA dissimilar to those of the database strains. However, it is unclear whether these geese-borne Helicobacter cause human diseases or not (Fox et al., 2006). Impact of these Helicobacter to public health should be investigated in the future.
In addition to the diversity information, we could also obtain quantitative data by using flaA primers for qPCR. Although PCR amplification efficiency was low with this flaA assay most likely due to relatively long amplicon size (ca. 400 bp), this could be overcome by using highly efficient DNA polymerases that are engineered or designed for long target amplification (e.g., KOD, Sso7d). Alternatively, quantification by digital PCR is independent of PCR amplification efficiency (Hindson et al., 2011), and therefore, can provide more accurate results of flaA copy numbers than conventional qPCR.
The density of Campylobacter in geese fecal samples as measured by flaA qPCR (4.06 log10 copies/g feces) was comparable to those reported previously (3.97 log10 copies/g feces) done with qPCR targeting ciaB of C. jejuni (Ishii et al., 2014a). There was disagreement between culture-dependent and culture-independent qPCR results. Samples positive for Campylobacter isolates were not always positives for flaA qPCR, and some samples negative for Campylobacter isolates showed positive results for flaA qPCR. This was probably due to (i) different detection sensitivity, (ii) detection of dead or VBNC cells by qPCR, and/or (ii) heterogeneous distribution of Campylobacter in the fecal samples. Culture-dependent method could detect 1 cell/g feces; while the quantification limit of our flaA assay was much higher (3 log10 copies/g feces). PCR-based methods could detect dead or dying cells. Although not tested in this study, use of propidium monoazide (PMA) could eliminate or significantly decrease PCR signals from dead Campylobacter cells (Castro et al., 2018), and therefore, could overcome this issue. We used 1 and 0.2 g of fecal samples for strain isolation and qPCR, respectively, and there is a possibility that Campylobacter was present only one of these subsamples.
While our culture-independent flaA SVR sequencing could provide high-throughput information of Campylobacter diversity in many environmental samples, there are several limitations. First, as mentioned above, DNA-based methods including flaA SVR sequencing could detect dead or dying cells. Use of PMA could overcome this issue and therefore should be tested in the future. Second, since flaA SVR sequencing method uses PCR, amplification biases could occur during PCR (Acinas et al., 2005). To minimize these effects, we carefully selected the PCR cycle numbers based on the qPCR results (Ishii et al., 2014b) and used a newly developed sequence analysis pipeline (Al-Ghalith et al., 2018). Lastly, PCR-based methods cannot provide information that isolated strains can do, such as pathogenic potential and antimicrobial resistance. One approach to assess potential health risks associated with flaA genotypes would be to use a comprehensive flaA database that includes clinical strains. Whole genomes of many clinical strains have been identified (Llarena et al., 2017), and therefore, it is possible to create such database. When flaA genotype highly similar to clinical strains is detected in environmental samples, it could pose greater health risks than other flaA genotypes. This approach should be examined in the future.
Conclusion
This study shows that the culture-independent flaA SVR sequencing could provide high-throughput information of Campylobacter diversity in many environmental samples. By comparing flaA genotypes of environmental samples (e.g., water and food) and those of known animal sources, we could identify potential sources of Campylobacter in the environmental samples (Ishii, 2017). This kind of source tracking has been done using fecal indicator bacteria such as E. coli and Bacteroides (Ram et al., 2004; Unno et al., 2010), but has not been done targeting pathogens. This study was done to provide a tool for rapid Campylobacter source identification.
The same approach could be applied to study other pathogens. In addition, amplicon sequencing library preparation could be done in high throughput by using microfluidic qPCR format (Oshiki et al., 2018); therefore, source tracking of multiple pathogens could be done in a timely manner.
Author Contributions
SI designed the research. MK, MM, and SI collected the samples. QZ, MK, TS, and MM performed the experiments. QZ, GA-G, MK, TS, DK, and SI analyzed the data. QZ and SI wrote the draft of the manuscript. All authors contributed to manuscript revision and read and approved the submitted version.
Funding
This work was supported in part by Mitsui & Co., Ltd., Environment Fund, MnDRIVE Initiative of the University of Minnesota, and Healthy Foods Healthy Lives Institute of the University of Minnesota.
Conflict of Interest Statement
The authors declare that the research was conducted in the absence of any commercial or financial relationships that could be construed as a potential conflict of interest.
Acknowledgments
We thank Ayumi Akiyoshi and Reiko Hirano for technical assistance.
Supplementary Material
The Supplementary Material for this article can be found online at: https://www.frontiersin.org/articles/10.3389/fmicb.2018.02201/full#supplementary-material
Footnotes
References
Acinas, S. G., Sarma-Rupavtarm, R., Klepac-Ceraj, V., and Polz, M. F. (2005). PCR-induced sequence artifacts and bias: insights from comparison of two 16s rRNA clone libraries constructed from the same sample. Appl. Environ. Microbiol. 71, 8966–8969. doi: 10.1128/AEM.71.12.8966-8969.2005
Al-Ghalith, G., and Knights, D. (2017). BURST Enables Optimal Exhaustive DNA Alignment for Big Data. Available at: https://github.com/knights-lab/BURST
Al-Ghalith, G. A., Hillmann, B., Ang, K., Shields-Cutler, R., and Knights, D. (2018). SHI7 is a self-learning pipeline for multipurpose short-read dna quality control. mSystems 3:e00202-17. doi: 10.1128/mSystems.00202-17
Banting, G. S., Braithwaite, S., Scott, C., Kim, J., Jeon, B., Ashbolt, N., et al. (2016). Evaluation of various Campylobacter-specific quantitative PCR (qPCR) assays for detection and enumeration of Campylobacteraceae in irrigation water and wastewater via a miniaturized most-probable-number–qPCR assay. Appl. Environ. Microbiol. 82, 4743–4756. doi: 10.1128/AEM.0007716
Calleros, L., Betancor, L., Iraola, G., Méndez, A., Morsella, C., Paolicchi, F., et al. (2017). Assessing the intra-species genetic variability in the clonal pathogen Campylobacter fetus: CRISPRs are highly polymorphic DNA markers. J. Microbiol. Methods 132, 86–94. doi: 10.1016/j.mimet.2016.11.012
Caporaso, J. G., Kuczynski, J., Stombaugh, J., Bittinger, K., Bushman, F. D., Costello, E. K., et al. (2010). QIIME allows analysis of high-throughput community sequencing data. Nat. Methods 7, 335–336. doi: 10.1038/nmeth.f.303
Castro, A. G. S. A., Dorneles, E. M. S., Santos, E. L. S., Alves, T. M., Silva, G. R., Figueiredo, T. C., et al. (2018). Viability of Campylobacter spp. in frozen and chilled broiler carcasses according to real-time PCR with propidium monoazide pretreatment. Poult. Sci. 97, 1706–1711. doi: 10.3382/ps/pey020
Crim, S. M., Griffin, P. M., Tauxe, R., Marder, E. P., Gilliss, D., Cronquist, A. B., et al. (2015). Preliminary incidence and trends of infection with pathogens transmitted commonly through food—Foodborne diseases active surveillance network, 10 US sites, 2006–2014. MMWR Morb. Mortal. Wkly. Rep. 64, 495–499.
Duarte, A., Seliwiorstow, T., Miller, W. G., De Zutter, L., Uyttendaele, M., Dierick, K., et al. (2016). Discriminative power of Campylobacter phenotypic and genotypic typing methods. J. Microbiol. Methods 125, 33–39. doi: 10.1016/j.mimet.2016.03.004
Ewing, B., Hillier, L., Wendl, M. C., and Green, P. (1998). Base-calling of automated sequencer traces using Phred. I. accuracy assessment. Genome Res. 8, 175–185. doi: 10.1101/gr.8.3.175
Fox, J. G., Taylor, N. S., Howe, S., Tidd, M., Xu, S., Paster, B. J., et al. (2006). Helicobacter anseris sp. nov. and Helicobacter brantae sp. nov., isolated from feces of resident Canada geese in the greater Boston area. Appl. Environ. Microbiol. 72, 4633–4637. doi: 10.1128/AEM.02876-05
Green, H. C., Dick, L. K., Gilpin, B., Samadpour, M., and Field, K. G. (2012). Genetic markers for rapid PCR-based identification of gull, Canada goose, duck, and chicken fecal contamination in water. Appl. Environ. Microbiol. 78, 503–510. doi: 10.1128/AEM.05734-11
Hamilton, M. J., Hadi, A. Z., Griffith, J. F., Ishii, S., and Sadowsky, M. J. (2010). Large scale analysis of virulence genes in Escherichia coli strains isolated from Avalon Bay, CA. Water Res. 44, 5463–5473. doi: 10.1016/j.watres.2010.06.058
Hindson, B. J., Ness, K. D., Masquelier, D. A., Belgrader, P., Heredia, N. J., Makarewicz, A. J., et al. (2011). High-throughput droplet digital PCR system for absolute quantitation of DNA copy number. Anal. Chem. 83, 8604–8610. doi: 10.1021/ac202028g
Hugerth, L. W., Wefer, H. A., Lundin, S., Jakobsson, H. E., Lindberg, M., Rodin, S., et al. (2014). DegePrime, a program for degenerate primer design for broad-taxonomic-range PCR in microbial ecology studies. Appl. Environ. Microbiol. 80, 5116–5123. doi: 10.1128/AEM.01403-14
Ishii, S. (2017). “Campylobacter and Arcobacter source tracking in a freshwater lake environment,” in Proceedings of the 19th International Symposium on Health-Related Water Microbiology, Chapel Hill, NC.
Ishii, S., Joikai, K., Otsuka, S., Senoo, K., and Okabe, S. (2016). Denitrification and nitrate-dependent Fe (II) oxidation in various Pseudogulbenkiania strains. Microbes Environ. 31, 293–298. doi: 10.1264/jsme2.ME16001
Ishii, S., Nakamura, T., Ozawa, S., Kobayashi, A., Sano, D., and Okabe, S. (2014a). Water quality monitoring and risk assessment by simultaneous multipathogen quantification. Environ. Sci. Technol. 48, 4744–4749. doi: 10.1021/es500578s
Ishii, S., Song, Y. J., Rathnayake, L., Tumendelger, A., Satoh, H., Toyoda, S., et al. (2014b). Identification of key nitrous oxide production pathways in aerobic partial nitrifying granules. Environ. Microbiol. 16, 3168–3180. doi: 10.1111/1462-2920.12458
Ishii, S., Segawa, T., and Okabe, S. (2013). Simultaneous quantification of multiple food- and waterborne pathogens by use of microfluidic quantitative PCR. Appl. Environ. Microbiol. 79, 2891–2898. doi: 10.1128/AEM.00205-13
Ishii, S., Yan, T., Shively, D. A., Byappanahalli, M. N., Whitman, R. L., and Sadowsky, M. J. (2006). Cladophora (Chlorophyta) spp. harbor human bacterial pathogens in nearshore water of Lake Michigan. Appl. Environ. Microbiol. 72, 4545–4553. doi: 10.1128/AEM.00131-06
Jones, K. (2001). Campylobacters in water, sewage and the environment. J. Appl. Microbiol. 90, 68S–79S. doi: 10.1046/j.1365-2672.2001.01355.x
Li, W., Raoult, D., and Fournier, P. E. (2009). Bacterial strain typing in the genomic era. FEMS Microbiol. Rev. 33, 892–916. doi: 10.1111/j.1574-6976.2009.00182.x
Linton, D., Owen, R., and Stanley, J. (1996). Rapid identification by PCR of the genus Campylobacter and of five Campylobacter species enteropathogenic for man and animals. Res. Microbiol. 147, 707–718. doi: 10.1016/S0923-2508(97)85118-2
Llarena, A. K., Taboada, E., and Rossi, M. (2017). Whole-genome sequencing in epidemiology of Campylobacter jejuni infections. J. Clin. Microbiol. 55, 1269–1275. doi: 10.1128/JCM.00017-17
Meinersmann, R. J., Helsel, L. O., Fields, P. I., and Hiett, K. L. (1997). Discrimination of Campylobacter jejuni isolates by fla gene sequencing. J. Clin. Microbiol. 35, 2810–2814.
On, S. L. (2013). Isolation, identification and subtyping of Campylobacter: where to from here? J. Microbiol. Methods 95, 3–7. doi: 10.1016/j.mimet.2013.06.011
Oshiki, M., Segawa, T., and Ishii, S. (2018). Nitrogen cycle evaluation (NiCE) chip for the simultaneous analysis of multiple N-cycle associated genes. Appl. Environ. Microbiol. doi: 10.1128/AEM.02615-17 [Epub ahead ofprint].
Oster, R. J., Wijesinghe, R. U., Haack, S. K., Fogarty, L. R., Tucker, T. R., and Riley, S. C. (2014). Bacterial pathogen gene abundance and relation to recreational water quality at seven great Lakes beaches. Environ. Sci. Technol. 48, 14148–14157. doi: 10.1021/es5038657
Pacha, R., Clark, G., Williams, E., and Carter, A. (1988). Migratory birds of central Washington as reservoirs of Campylobacter jejuni. Can. J. Microbiol. 34, 80–82. doi: 10.1139/m88-015
Ram, J. L., Ritchie, R. P., Fang, J., Gonzales, F. S., and Selegean, J. P. (2004). Sequence-based source tracking of based on genetic diversity of β-glucuronidase. J. Environ. Qual. 33, 1024–1032. doi: 10.2134/jeq2004.1024
Silva, J., Leite, D., Fernandes, M., Mena, C., Gibbs, P. A., and Teixeira, P. (2011). Campylobacter spp. as a foodborne pathogen: a review. Front. Microbiol. 2:200. doi: 10.3389/fmicb.2011.00200
Taboada, E. N., Clark, C. G., Sproston, E. L., and Carrillo, C. D. (2013). Current methods for molecular typing of Campylobacter species. J. Microbiol. Methods 95, 24–31. doi: 10.1016/j.mimet.2013.07.007
Tamura, K., Stecher, G., Peterson, D., Filipski, A., and Kumar, S. (2013). MEGA6: molecular evolutionary genetics analysis version 6.0. Mol. Biol. Evol. 30, 2725–2729. doi: 10.1093/molbev/mst197
Tholozan, J. L., Cappelier, J. M., Tissier, J. P., Delattre, G., and Federighi, M. (1999). Physiological characterization of viable-but-nonculturable Campylobacter jejuni cells. Appl. Environ. Microbiol. 65, 1110–1116.
Unno, T., Jang, J., Han, D., Kim, J. H., Sadowsky, M. J., Kim, O.-S., et al. (2010). Use of barcoded pyrosequencing and shared OTUs to determine sources of fecal bacteria in watersheds. Environ. Sci. Technol. 44, 7777–7782. doi: 10.1021/es101500z
Wassenaar, T. M., and Newell, D. G. (2000). Genotyping of Campylobacter spp. Appl. Environ. Microbiol. 66, 1–9. doi: 10.1128/AEM.66.1.1-9.2000
Westrell, T., Ciampa, N., Boelaert, F., Helwigh, B., Korsgaard, H., Chríel, M., et al. (2009). Zoonotic infections in Europe in 2007: a summary of the EFSA-ECDC annual report. Euro surveill. 14:19100.
Keywords: Campylobacter, diversity, pathogen, source tracking, genotyping, flaA, next generation sequencing
Citation: Zhang Q, Al-Ghalith GA, Kobayashi M, Segawa T, Maeda M, Okabe S, Knights D and Ishii S (2018) High-Throughput flaA Short Variable Region Sequencing to Assess Campylobacter Diversity in Fecal Samples From Birds. Front. Microbiol. 9:2201. doi: 10.3389/fmicb.2018.02201
Received: 14 February 2018; Accepted: 28 August 2018;
Published: 25 September 2018.
Edited by:
Abd El-Latif Hesham, Assiut University, EgyptReviewed by:
Shannon D. Manning, Michigan State University, United StatesBeatrix Stessl, Veterinärmedizinische Universität Wien, Austria
Copyright © 2018 Zhang, Al-Ghalith, Kobayashi, Segawa, Maeda, Okabe, Knights and Ishii. This is an open-access article distributed under the terms of the Creative Commons Attribution License (CC BY). The use, distribution or reproduction in other forums is permitted, provided the original author(s) and the copyright owner(s) are credited and that the original publication in this journal is cited, in accordance with accepted academic practice. No use, distribution or reproduction is permitted which does not comply with these terms.
*Correspondence: Satoshi Ishii, ishi0040@umn.edu