- 1Department of Pathology, University of Texas Medical Branch, Galveston, TX, United States
- 2Department of Pharmacology and Toxicology, Sealy Center for Structural Biology and Molecular Biophysics, University of Texas Medical Branch, Galveston, TX, United States
- 3Department of Computer Science, University of Houston, Houston, TX, United States
- 4Department of Pharmacology and Toxicology, Sealy Center for Structural Biology, University of Texas Medical Branch, Galveston, TX, United States
- 5Department of Microbiology and Immunology, University of Texas Medical Branch, Galveston, TX, United States
- 6Department of Zoology, University of Cambridge, Cambridge, United Kingdom
- 7Harris County Public Health, Mosquito & Vector Control Division, Houston, TX, United States
- 8Department of Pathology, Institute for Human Infections and Immunity, Center for Tropical Diseases, Center for Biodefense and Emerging Infectious Disease, University of Texas Medical Branch, Galveston, TX, United States
Microbial interactions are an underappreciated force in shaping insect microbiome communities. Although pairwise patterns of symbiont interactions have been identified, we have a poor understanding regarding the scale and the nature of co-occurrence and co-exclusion interactions within the microbiome. To characterize these patterns in mosquitoes, we sequenced the bacterial microbiome of Aedes aegypti, Ae. albopictus, and Culex quinquefasciatus caught in the field or reared in the laboratory and used these data to generate interaction networks. For collections, we used traps that attracted host-seeking or ovipositing female mosquitoes to determine how physiological state affects the microbiome under field conditions. Interestingly, we saw few differences in species richness or microbiome community structure in mosquitoes caught in either trap. Co-occurrence and co-exclusion analysis identified 116 pairwise interactions substantially increasing the list of bacterial interactions observed in mosquitoes. Networks generated from the microbiome of Ae. aegypti often included highly interconnected hub bacteria. There were several instances where co-occurring bacteria co-excluded a third taxa, suggesting the existence of tripartite relationships. Several associations were observed in multiple species or in field and laboratory-reared mosquitoes indicating these associations are robust and not influenced by environmental or host factors. To demonstrate that microbial interactions can influence colonization of the host, we administered symbionts to Ae. aegypti larvae that either possessed or lacked their resident microbiota. We found that the presence of resident microbiota can inhibit colonization of particular bacterial taxa. Our results highlight that microbial interactions in mosquitoes are complex and influence microbiome composition.
Introduction
The microbiome of mosquitoes can be highly variable, both within and between species, and is often dominated by relatively few genera (Wang et al., 2011; Boissière et al., 2012; Osei-Poku et al., 2012; Buck et al., 2016; Muturi et al., 2017). Understanding the factors that influence this variation is important as microbes drastically alter host biology. For mosquitoes, bacteria can affect a diverse number of traits including immunity, reproduction, survival, and vector competence (Jupatanakul et al., 2014; Hegde et al., 2015). These phenotypes have ramifications for the vectorial capacity of pathogens, and as such, microbial-based vector control strategies are under investigation to reduce the burden of arthropod-borne diseases (Bourtzis et al., 2014; Dennison et al., 2014; Saldana et al., 2017). While our understanding of the contributing factors that affect the composition and abundance of the microbiome is expanding, there are still many unanswered questions regarding microbiome assembly and maintenance within mosquito hosts.
Exposure to environmental microbes is undoubtedly a major influence on the mosquito microbiome. These effects are particularly pronounced at the aquatic stage as larvae and pupae are immersed in water and can acquire bacteria by filter feeding. Indeed, several studies have shown the larval stages possess a similar microbiome as their larval water environment (Vázquez-martínez et al., 2009; Duguma et al., 2013; Gimonneau et al., 2014), and exposure to bacteria at these immature stages has implications for adult traits (Dickson et al., 2017). Furthermore, the larval habitat can influence the composition of the adult microbiome. Bacteria can be transstadially transmitted to the adult (Jadin et al., 1966; Coon et al., 2014; Gonçalves et al., 2014; Chen et al., 2015), and newly emerged adults are known to imbibe their larval water, which likely seeds the gut with microbiota (Lindh et al., 2008).
Host and bacterial genetics also contribute to microbiome composition and microbial abundance. Mosquitoes can maintain microbiome homeostasis by a variety of different mechanisms. Host pathways and processes known to influence microbiota in mosquitoes include immunity, amino acid metabolism, reactive oxygen species, and calcium transport (Kumar et al., 2010; Stathopoulos et al., 2014; Pang et al., 2016; Short et al., 2017; Xiao et al., 2017; Zhao et al., 2017). Additionally, serial passaging of gut symbionts in mosquitoes selected for isolates that persist in the gut for longer periods of time (Riehle et al., 2007; Dennison et al., 2016), indicating that bacterial genetics is also important in shaping the microbiome.
Adult mosquito feeding behavior also has important implications for microbiome community structure. It is likely that bacteria can be acquired from the nectar of plants (Gusmão et al., 2007), and taking a blood meal alters the microbiome considerably. At 24 h post-blood meal, the bacterial load in the gut drastically increases while species diversity decreases (Kumar et al., 2010; Oliveira et al., 2011; Wang et al., 2011; Terenius et al., 2012). Culture based assays show that bacterial loads revert to pre-blood fed levels 2–3 days after the blood meal (Demaio et al., 1996; Pumpuni et al., 1996; Oliveira et al., 2011), although other studies have seen high bacterial loads persist for some time and species richness not reverting to the original composition seen prior to the blood meal (Gusmão et al., 2010; Wang et al., 2011). Most of these studies either used laboratory-reared mosquitoes to examine culturable bacterial load, or relocated field mosquitoes to the laboratory for experimentation, and as such, there are few studies examining the effect of blood feeding on the microbiome community structure in field populations.
Recently, it has become evident that a further force affects microbiome composition in mosquitoes – interactions between the microbes themselves. These interactions were first highlighted with the discovery that Wolbachia and Asaia are antagonistic to one another, thereby affecting the vertical transmission of Wolbachia in Anopheles mosquitoes (Hughes et al., 2014; Rossi et al., 2015). Further comparisons exploiting 16S rRNA amplicon high throughput sequencing have identified interactions between Wolbachia and other microbes in both Drosophila and mosquitoes (Zink et al., 2015; Audsley et al., 2017b; Simhadri et al., 2017). In addition to the specific interactions between Wolbachia and other bacterial taxa, pairwise negative and positive microbial interactions within bacteria or fungi, as well as cross-kingdom interactions (bacterial-fungal) have been reported in the La Crosse virus vectors, Aedes triseriatus, and Ae. japonicus (Muturi et al., 2016a). Taken together, these studies suggest that microbial interactions are important in dictating the composition and abundance of host-associated microbiota. Yet it is unclear how ubiquitous and complex these interactions are within mosquitoes.
16S rRNA amplicon sequencing datasets have been analyzed to create microbial co-occurrence networks for several species and environments (Chaffron et al., 2010; Barberán et al., 2012; Faust and Raes, 2012; Faust et al., 2012; Goodrich et al., 2014), but these networks are lacking for mosquitoes and insects in general. These methods use presence/absence metrics, relative abundance, or both, to examine pairwise interactions to develop a network. Usually, interacting pairs of taxa are designated as having co-occurring or co-exclusionary relationships. Each method used for the identification of co-occurrence/co-exclusion networks has strengths and weaknesses in identifying particular patterns. CoNet (Faust and Raes, 2016), uses an ensemble approach that combines results from a collection of algorithms (Pearson, 1895; Kullback and Leibler, 1951; Bray and Curtis, 1957; Cover and Thomas, 2012; Sedgwick, 2014) using presence/absence and relative abundance data to identify statistically significant interactions. Interaction networks provide another methodology to examine the community structure of the microbiome of mosquitoes. Comparing microbiome networks generated from mosquitoes exposed to different conditions may provide insights into factors influencing microbiome structure in mosquitoes and identify pairwise interactions not affected by environmental conditions.
To expand our understanding of the forces that shape the bacterial microbiome of mosquitoes, we examined the microbial composition and community structure from three major mosquito arboviral vectors, Ae. aegypti, Ae. albopictus, and Cx. quinquefasciatus, collected from the field or reared under uniform insectary conditions. For the field collections, we utilized two trapping methods that primarily attract mosquitoes in different physiological states: host- or oviposition-seeking (Reiter et al., 1986; Maciel-de-Freitas et al., 2006; Dennett et al., 2007; Figuerola et al., 2012). Our sampling regime allowed us to examine how factors such as host species, environment, and physiological state in the field influenced the composition of the mosquito microbiome. We also compared the microbiome of mosquitoes that were naturally infected (Ae. albopictus and Cx. quinquefasciatus) and uninfected (Ae. aegypti) with Wolbachia. Furthermore, we developed microbial interaction networks to explore the complexity and nature of microbial interactions in mosquitoes. To demonstrate that microbial interactions influence host colonization, we infected Ae. aegypti larvae either possessing or lacking their native microbiota with a range of bacterial symbionts. Our results highlight the complexities of microbial networks in field collected Ae. aegypti mosquitoes and indicate the native microbiome induces colonization resistance to certain gut microbes.
Materials and Methods
Mosquito Collections and DNA Extractions
Female Ae. albopictus and Cx. quinquefasciatus were collected from an abandoned tire repository in south-eastern Harris County, Houston, TX, United States, while female Ae. aegypti were collected from a separate site in Houston (Supplementary Figure S1). Further details describing the tire repository location were previously reported (Dennett et al., 2004). All mosquitoes were trapped over a 24 h period with either the Biogents Sentinel (BG) or Harris County gravid (G) traps, which selectively collect host-seeking or gravid female mosquitoes, respectively (Maciel-de-Freitas et al., 2006; Dennett et al., 2007; Figuerola et al., 2012). Mosquito species were identified using morphological characteristics, surface sterilized (5 min in 70% ethanol followed by three washes in 1× PBS each for 5 min), and stored in ethanol at -20°C while awaiting DNA extraction. 5–7 days old adult sugar fed laboratory-colonized mosquitoes (Ae. aegypti; Galveston strain, Ae. albopictus; Galveston strain, and Cx. quinquefasciatus; Houston strain) were reared under conventional conditions and then processed in the same manner as field samples. All laboratory-reared mosquitoes were reared in the insectary at the University of Texas Medical Branch.
High Throughput Sequencing and Bioinformatics Analysis
High-throughput sequencing of the bacterial 16S ribosomal RNA gene was performed using gDNA isolated from each sample. Sequencing libraries for each isolate were generated using universal 16S rRNA V3-V4 region primers (Klindworth et al., 2012) in accordance with Illumina 16S rRNA metagenomic sequencing library protocols. The samples were barcoded for multiplexing using Nextera XT Index Kit v2. Sequencing was performed on an Illumina MiSeq instrument using a MiSeq Reagent Kit v2 (500-cycles). The NCBI Bioproject accession number for the raw sequencing data reported here is PRJNA422599.
To identify the presence of known bacteria, sequences were analyzed using the CLC Genomics Workbench 8.0.1 Microbial Genomics Module1. Reads containing nucleotides below the quality threshold of 0.05 (using the modified Richard Mott algorithm) and those with two or more unknown nucleotides or sequencing adapters were trimmed out. All reads were trimmed to 264 bases for subsequent operational taxonomic unit (OTU) classification. Reference based OTU picking was performed using the SILVA SSU v119 97% database (Quast et al., 2013). Sequences present in more than one copy but not clustered to the database were placed into de novo OTUs (97% similarity) and aligned against the reference database with 80% similarity threshold to assign the “closest” taxonomical name where possible. Chimeras were removed from the dataset if the absolute crossover cost was three using a k-mer size of six. Alpha diversity was measured using Shannon entropy (OTU level), rarefaction sampling without replacement, and with 100,000 replicates at each point. Beta diversity was calculated using the Bray-Curtis diversity measure (OTU level). PERmutational Multivariate ANalysis Of VAriance (PERMANOVA) analysis was used to measure effect size and significance on beta diversity for grouping variables (Anderson, 2014). The significance is obtained by a permutation test. For each assessment, a permutation of 99,999 was chosen. Differentially abundant bacteria (genus level, >0.1%) were identified using analysis of composition of microbiomes (ANCOM) (Mandal et al., 2015) with a significance level of P < 0.05, while values quantifying fold change were obtained using the log2 fold change formula (Quackenbush, 2002).
Detection of Complex Interaction Patterns
Network analysis was conducted independently on each group within a species. OTUs with read counts below 0.1% of total number of reads in all samples were excluded from analysis. The remaining OTUs were combined based on lowest common taxonomy assignments down to genus level, and relative abundance tables were generated by normalizing read counts against total number of reads in the original data. The resulting number of unique entries identified in samples was 33. Interactions (such as co-occurrence and co-exclusion) among these were identified using CoNet app (Faust and Raes, 2016) in Cytoscape (Shannon et al., 2003) using the following ensemble of methods: Pearson correlation (Pearson, 1895), Spearman correlation (Sedgwick, 2014), mutual information (Cover and Thomas, 2012), Bray-Curtis dissimilarity (Bray and Curtis, 1957), and Kullback-Leibler divergence (Kullback and Leibler, 1951). Statistical significance of each pair was tested using the row-shuffle randomization option and interactions that scored at the top and bottom 5% of 1000 bootstraps were reported. Resulting statistically significant interactions were categorized by the software into three groups: co-presence, co-exclusion, and unknown. Unknown interactions represent statistically significant patterns that cannot be clearly categorized as co-exclusion or co-occurrence. Since we could not ascribe an interaction pattern, the unknown interactions were excluded from the network. Resulting interaction networks were visualized using Cytoscape software (Shannon et al., 2003).
Estimation of Microbial Load and Screening for Wolbachia by PCR
Total bacterial load within each mosquito species or group was assessed by qPCR using gDNA as a template. qPCR was conducted using universal bacterial primers (16rRNA-RTF: TCCTACGGGAGGCAGCAGT and 16rRNA-RTR: GGACTACCAGGGTATCTAATCCTGTT) (Kumar et al., 2010) that amplified the bacterial 16S rRNA gene or primers that specifically amplified Wolbachia (W-Spec-16S-F: CATACCTATTCGAAGGGATA and W-Spec-16s-R: AGCTTCGAGTGAAACCAATTC) (Werren and Windsor, 2000). Primers specific to S7 gene (aeg-S7-F: ACCGCCG TCTACGATGCCA, aeg-S7-R: ATGGTGGTCTGCTGGTTCTT, Cq-S7-F: CTGGAGATGAACTGGACCT, and Cq-S7-R: CTT GTACACCGACGTGAAGG) or actin gene (alb-act-F: CCCACACAGTCCCCATCTAC, alb-act-F: CGAGTAGCCACGTTCAGTCA) (Xia and Zwiebel, 2006; Isoe et al., 2011; Calvitti et al., 2015) were used to quantify host genomic copies. The PCR was carried out in a 10 μl reaction containing 1 μM of each primer, 1× SYBR Green (Applied Biosystems) and 2 μl of genomic DNA template. Cycling conditions involved an initial denaturation at 95°C for 10 min, 40 cycles of 15 s at 95°C, 1 min at 60°C. Fluorescence readings were taken at 60°C after each cycle before deriving a melting curve (60–95°C) to confirm the identity of the PCR product. The PCR was carried out on the ABI StepOnePlus Real-Time PCR System.
Relative abundance was calculated by comparing the load of all bacteria or Wolbachia to a single copy mosquito gene. PCRs amplifying the 16S rRNA and wsp genes of Wolbachia were used to screen for Wolbachia in field caught Ae. aegypti (Zhou et al., 1998; Werren and Windsor, 2000; Baldo et al., 2006), and nematode specific primers were used to screen for nematode infections (Casiraghi et al., 2004).
Re-Infection of Bacteria Into Mosquito Larvae
Aedes aegypti gnotobiotic larvae were generated as previously described (Coon et al., 2014). All manipulations of mosquitoes were undertaken in a sterile environment. To synchronize hatching, sterile eggs were transferred to a conical flask and placed under a vacuum for 45 min. To verify sterility, larval water was plated on non-selective LB agar plates. L1 larvae grown without bacteria have slow growth rates and do not reach pupation (Coon et al., 2014). Forty-five L1 larvae were transferred to a T75 tissue culture flask and inoculated with 1 × 107 CFU/ml of transgenic symbionts possessing the plasmid the pRAM18dRGA-mCherry that was derived from pRAM18dRGA (Burkhardt et al., 2011). Bacterial cultures were quantified with a spectrophotometer (DeNovix DS-11, DeNovix) and validated by plating to determine CFU. For conventional rearing of mosquitoes, eggs (non-sterilized) were vacuum hatched and grown under non-aseptic conditions in a T75 tissue culture flask supplemented with transgenic symbionts at the concentration of 1 × 107 CFU/ml. To feed mosquitoes, ground fish food pellets were sterilized by autoclaving, and mixed with sterile water. The equivalent of 6 mg of fish food was fed to both gnotobiotic and conventionally reared mosquitoes every second day. To quantify the bacterial load of symbionts, surface sterilized L4 larvae were homogenized and plated on a selective media (50 μg/ml Kanamycin) on which only transgenic symbionts grew (pRAM18dRGA-mCherry induces resistance to kanamycin). After incubation at 30 or 37°C (depending on symbiont) for 2–3 days, colonies (expressing mCherry fluorescent protein) were counted. All colonies observed on the kanamycin plate were confirmed to have mCherry fluorescence.
Results
Microbiome Diversity
We sequenced amplicons of the V3/V4 region of the 16S rRNA gene from whole individual adult female mosquitoes either collected from the field or reared in the laboratory. In total, we sequenced 130 adult mosquitoes obtaining 10,668,291 reads (sample size per group and species is reported in Supplementary Table S1). After quality filtering, 7,051,256 reads were assigned to OTUs at 97% identity threshold and on average, there were 54,240 reads per mosquito sample. Rarefaction curve analysis indicated that our sequencing depth was sufficient to observe all OTUs in mosquito samples (Supplementary Figure S2). We identified a total of 4,419 bacterial OTUs in the three mosquito species, but only 58 were present at an infection frequency of over 0.1% within the dataset (Supplementary Table S2). When abundant microbes were classified at higher taxonomic levels, our analysis found 22 families, with Enterobacteriaceae being the most common when disregarding Wolbachia. Bacteria found in mosquitoes were classified into five phyla with bacteria in the phylum Proteobacteria most prevalent in the microbiome, which is consistent with previous studies (Osei-Poku et al., 2012; David et al., 2016; Muturi et al., 2016b; Audsley et al., 2017a), although other reports indicated Bacteroidetes or Acinetobacter phyla can be a major component of the microbiome (Coon et al., 2014, 2016b; Minard et al., 2014, 2015).
When examining the sequencing data at the genus level, the microbiomes of Cx. quinquefasciatus and Ae. albopictus were dominated by the endosymbiont Wolbachia with 87 and 81% of total reads, respectively (Supplementary Figure S3 and Table S2). While Wolbachia accounted for many of the reads, rarefaction analysis indicated our sampling depth was sufficient to identify rare OTUs. Other highly abundant genera in field-collected mosquitoes included Halomonas, Shewanella, and Asaia in Cx. quinquefasciatus, Halomonas, Pseudomonas, and Zymobacter in Ae. albopictus, and Pseudomonas, Zymobacter, Tatumella, and Enterobacter in Ae. aegypti (Supplementary Figure S3 and Table S2). Similar to a recent finding (Coon et al., 2016b), we found a small number of Wolbachia reads in a few Ae. aegypti individuals collected from the field in G traps. It is thought that Ae. aegypti are naturally uninfected by Wolbachia (Iturbe-Ormaetxe et al., 2011; Gloria-Soria et al., 2018), although Coon et al. (2016b) suggested that some populations may be infected. However, samples that contained Wolbachia reads from our sequencing data could not be independently validated by PCR using several Wolbachia genes (wsp and MLST genes) commonly used to screen for the bacterium, nor were they PCR positive for filarial nematode infection (that carry Wolbachia) when amplifying with primers that detect nematode DNA.
Given the above finding and since microbiome sequencing can be susceptible to contamination (Tourlousse et al., 2017; Pollock et al., 2018), we examined our data for other possible contamination signatures. While we could not find conclusive evidence of contamination, in this process we observed a possible batch effect for a specific bacterium in field-collected Ae. aegypti. These samples were extracted in two batches (see Supplementary Table S1) and the field-collected samples from the latter extraction were found to have higher loads of Burkholderia compared to those extracted in the first batch. However, all laboratory-reared mosquitoes extracted during this second extraction did not contain high levels of Burkholderia, indicating the prevalence of this microbe in the field-collected samples was not likely due to laboratory contamination. Despite this occurrence, the dataset is suitable for constructing networks as this analysis examines pairwise interactions, and as such, other interacting pairs will not be influenced by Burkholderia. Future studies should consider the use of reagent-only controls and spike controls to help determine if cross-contamination of samples occurs in the sequencing process (Tourlousse et al., 2017; Pollock et al., 2018), although it is questionable if these controls would have been of assistance in this case given that laboratory-reared mosquitoes did not have elevated Burkholderia reads.
The Shannon diversity index was used to estimate the species richness in mosquitoes (Shannon, 2001). There were significant differences in diversity between the microbial communities of Aedes mosquitoes compared to Cx. quinquefasciatus when compared at the OTU level (Figure 1; Kruskal-Wallis P < 0.0001). When comparing groups within Ae. aegypti, field collected mosquitoes from either the BG or G trap had a significantly greater Shannon diversity index compared to the laboratory mosquitoes. No differences were seen between groups in Ae. albopictus, while Cx. quinquefasciatus caught in the G trap had a significantly lower Shannon diversity index compared to laboratory-reared mosquitoes. Across all species, we found no significant differences in species richness between mosquitoes caught in the BG and G traps.
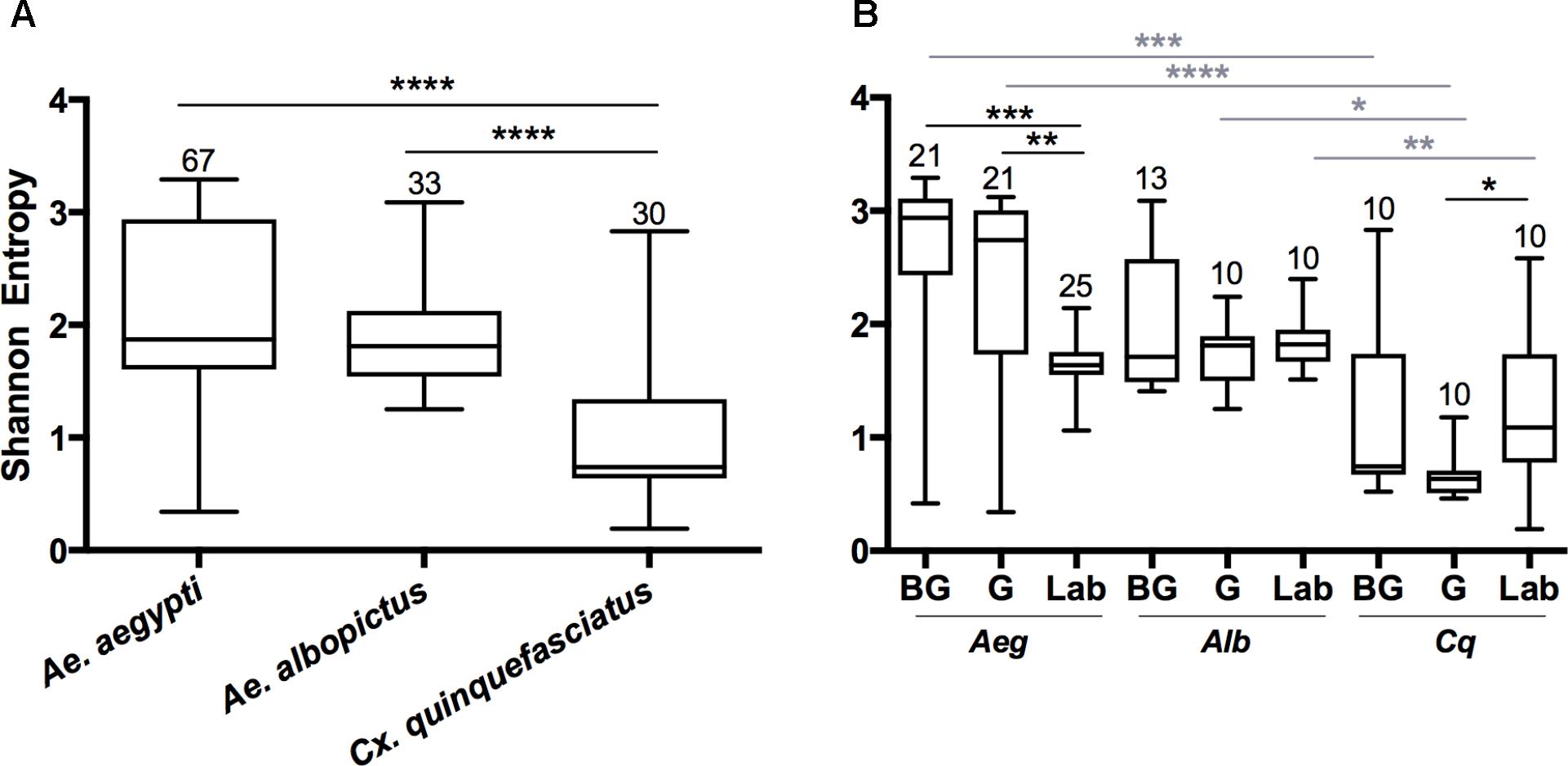
FIGURE 1. Shannon diversity indices at the OTU level for all mosquito species (A) or for each group within a species (B). A Kruskal-Wallis test with a Dunn’s multiple comparison test was used to determine significance (∗P < 0.05, ∗∗P < 0.01, ∗∗∗P < 0.001, ∗∗∗∗P < 0.0001) within a species (black) or group (gray). Abbreviations: Aeg, Ae. Aegypti; Alb, Ae. Albopictus; Cq, Cx. quinquefasciatus. Bars on the box plots show maximum to minimum range. Sample size for species and group is indicated by the numbers above the box-plots and in Supplementary Table S1.
Since both Ae. albopictus and Cx. quinquefasciatus were heavily infected with Wolbachia, we examined alpha diversity (OTU level) in these mosquitoes when this endosymbiont was computationally excluded from the dataset (Supplementary Figure S4). In all cases, we observed an increase in Shannon diversity when Wolbachia was excluded. This was significant when analyzed by species (Ae. albopictus P < 0.0001; Cx. quinquefasciatus P < 0.0001), and for all groups with the exception of Ae. albopictus caught in the G traps (Ae. albopictus: BG P < 0.005, Lab P < 0.02; Cx. quinquefasciatus: BG P < 0.04, G P < 0.001, Laboratory P < 0.01). No significant differences were seen when comparing the BG to G groups after removal of Wolbachia (Ae. albopictus: P = 0.23; Cx. quinquefasciatus: P = 0.57).
Factors That Influence Microbiome Community Structure
We examined how the environmental, physiological state in the field, and host species affected the bacterial community structure using beta diversity analysis by comparing the microbiomes of the three mosquito species or groups. Within each group, distinct microbiome clustering patterns were observed between the three mosquito species and all pairwise comparisons were significantly different (Figure 2; PERMANOVA with Bray-Curtis distance comparison P < 0.05). In general, the microbiome of Ae. aegypti was more divergent compared to the microbiomes of Cx. quinquefasciatus and Ae. albopictus regardless of origin (Figure 2A). When Wolbachia was computationally excluded from the analysis (Figure 2A), the microbiomes of Cx. quinquefasciatus and Ae. albopictus became more divergent in the laboratory, but were not significantly different when considering mosquitoes caught in the BG trap (P = 0.24). Interestingly, there was tight clustering of samples from Cx. quinquefasciatus and Ae. albopictus caught in the G trap (Figures 2A), although these two groups were significantly different (P = 0.00001). When Wolbachia was removed, this clustering became more divergent, yet still was significantly different (P = 0.0052) (Figure 2A).
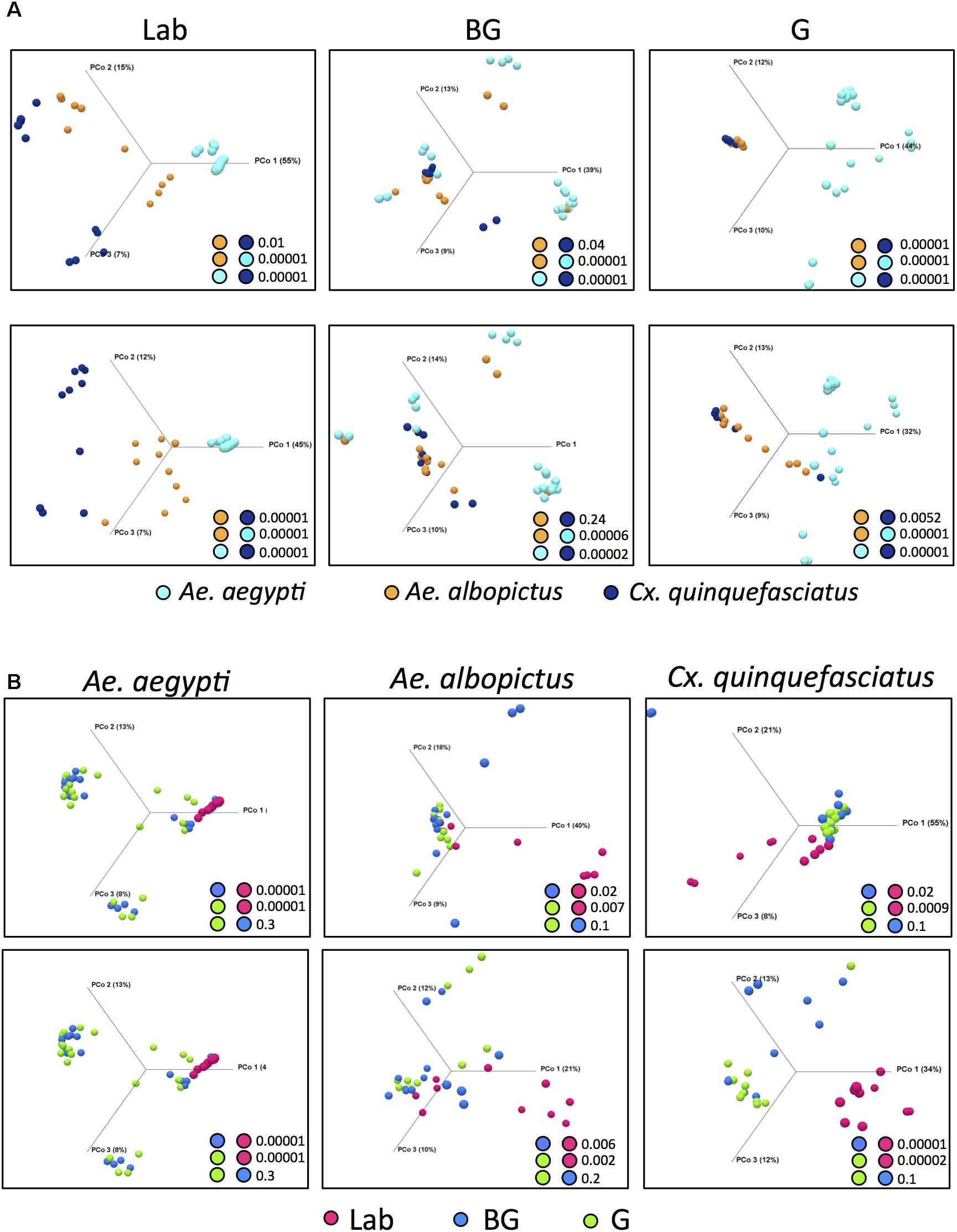
FIGURE 2. Principal Coordinates Analysis (OTU level) using Bray-Curtis dissimilarity, comparing identified OTUs within a group (A) or species (B). PERMANOVA significance values for pairwise comparison are reported in the lower right corner for each analysis. For (A,B), upper plots include Wolbachia while Wolbachia has been computationally excluded in the lower plots.
To determine how the environment and physiological state influences microbial composition we compared the microbiome of laboratory-reared and field-caught (BG or G) individuals within each mosquito species. For all species, laboratory-reared mosquitoes had a significantly distinct microbiota compared to their field counterparts (Figure 2B). This was most pronounced in Ae. aegypti but less distinct for Ae. albopictus and Cx. quinquefasciatus. No significant differences were observed in the microbiome community structure of mosquitoes caught in the BG or G traps for any of the three mosquito species. No differences were observed when these microbiomes were analyzed with Wolbachia computationally excluded (Figure 2B).
Common and Differentially Abundant Bacterial Between and Within Mosquito Species
We examined our data for bacterial genera that were unique to or shared between mosquito species. The majority of bacteria were common between species with the notable exception that Ae. aegypti caught in the G trap possessed 12 genera not present in the other two mosquito species (Figure 3A and Supplementary Table S3). Similarly, when comparing within a species between groups, most bacteria were common to all groups (Laboratory, BG, G). To detect bacteria that may contribute to the observed differences in microbiome community structure of a particular mosquito species, we completed pairwise comparisons to identify bacteria that were differentially abundant (Figure 3B). These comparisons were completed within each group (Laboratory, BG or G). The largest differences in the microbiome were seen when comparing Ae. aegypti to the other two species, which agreed with our beta diversity analysis findings. Several bacterial taxa were differentially abundant between species regardless of group (Laboratory, BG and G traps), suggesting environmental factors are not greatly influential on these specific host-microbe associations. For example, Aeromonas, Serratia, Shewanella, and Wolbachia were less abundant in Ae. aegpyti compared to both Ae. albopictus and Cx. quinquefasciatus regardless of environmental conditions. When comparing differential abundance within a group, we were able to find infection gradients of specific microbes across species. The two examples of these infection clines were for Serratia and Aeromonas in laboratory-reared mosquitoes. These bacteria heavily infected Cx. quinquefasciatus, had moderate infection densities in Ae. albopictus and poorly infected or were absent from Ae. aegypti, despite the fact these three mosquito species were reared under common environmental laboratory conditions (Figure 3B and Supplementary Figure S5).
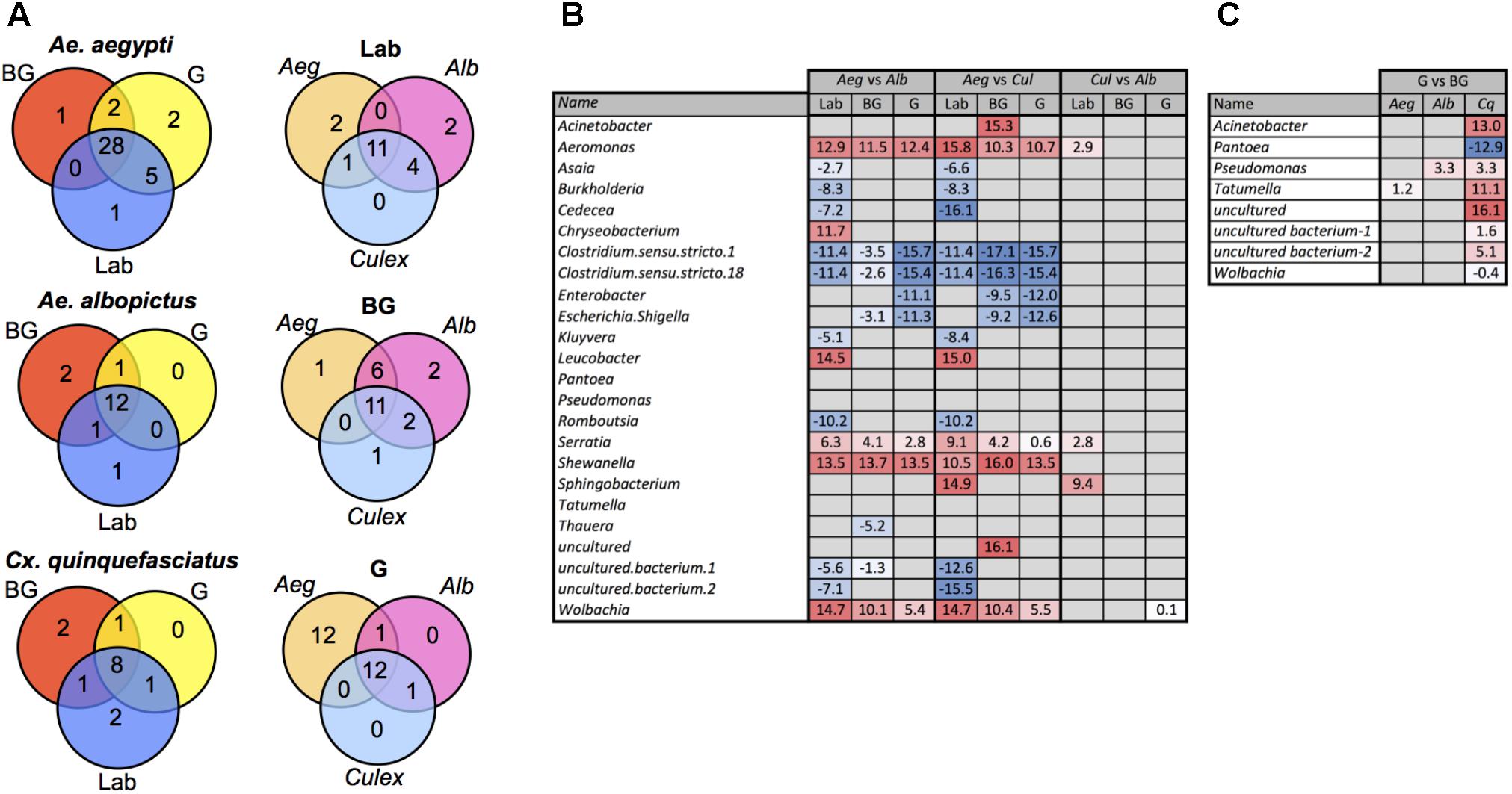
FIGURE 3. Common and differentially abundant bacteria (genus level) within mosquitoes. Venn diagram showing number of common bacterial genera between mosquito groups and species (A). Pairwise comparison of bacterial density between each mosquito species within each group (B). Pairwise comparison of bacterial density for mosquito caught in the G trap compared the BG trap (Ae. Aegypti, Aeg; Ae. Albopictus, Alb; Cx. Quinquefasciatus, Cq.) (C). Log2 values indicated fold change in bacterial density. List of common taxa for each species and group are presented in Supplementary Table S3.
Several studies have reported that blood feeding alters the species richness in mosquito guts (Kumar et al., 2010; Oliveira et al., 2011; Wang et al., 2011; Terenius et al., 2012). While at the community level, the microbiomes of G and BG were not significantly different (Figure 2), we did find specific bacteria that were differentially abundant between these groups (Figure 3C). These changes were mainly seen in Cx. quinquefasciatus. Of the known bacteria, the greatest changes were seen in Acinetobacter, Tatumella, and Pantoea, with the former two being more abundant in the mosquitoes caught in BG traps, while the latter was more abundant in mosquitoes caught in the G trap.
Total Bacterial Load in Mosquitoes
While high throughput sequencing allows characterization of the composition of the microbiota, it only provides a relative measure of bacterial density (Gloor et al., 2017). Therefore, to obtain an estimate of the total bacterial load in each vector species, we completed qPCR on mosquitoes with universal eubacterial primers that broadly amplify bacterial species (Kumar et al., 2010). Culex mosquitoes were seen to have a higher total bacterial load when compared to either of the two Aedes species (Figure 4; Kruskal Wallis P < 0.0001). When comparing within a species between groups, we found that laboratory-reared Ae. aegypti had significantly greater load than those caught in the field (Kruskal Wallis P < 0.0001). This was also the case for Cx. quinquefasciatus (Kruskal Wallis P < 0.0001) while no significant differences were seen between Ae. albopictus groups. As both Ae. albopictus and Cx. quinquefasciatus are infected by Wolbachia, we also quantified Wolbachia by qPCR to determine its relative density in proportion to the total bacterial load of mosquitoes. While it is inappropriate to statistically compare amplicons that have different amplification efficiencies, it is evident that Wolbachia comprises a major component of the microbiome in Ae. albopictus and Cx. quinquefasciatus, which corroborates the high throughput sequencing data.
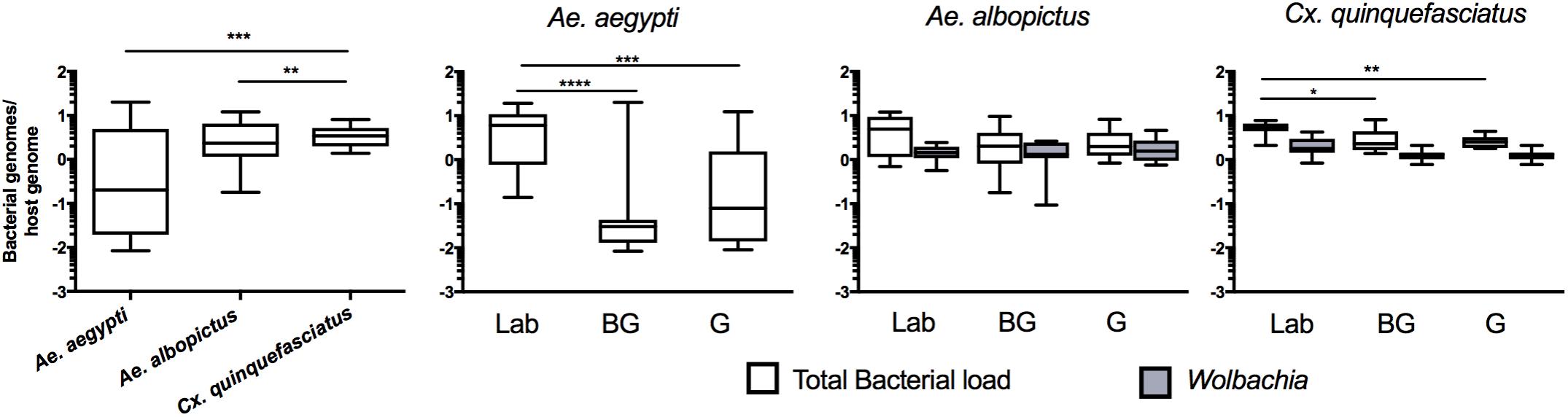
FIGURE 4. Total bacterial load of mosquitoes. Comparison of bacterial load for each species (left) or within each group for each mosquito species (right). Bacterial load is represented as a ratio between 16S rRNA gene copies to S7 copies (Ae. aegypti and Cx. quinquefasciatus) or actin (Ae. albopictus) genes. The density of Wolbachia was estimated for Ae. albopictus (Wolbachia 16s:Actin) and Cx. quinquefasciatus (ftsZ:S7). A Kruskal-Wallis test with a Dunn’s multiple comparison test was used to determine significance (∗P < 0.05, ∗∗P < 0.01, ∗∗∗P < 0.001, ∗∗∗∗P < 0.0001) of total bacterial loads within a species. Bars on the box plots show maximum to minimum range. Sample size for all species and groups is described in Supplementary Table S1.
Microbial Interaction Networks Within Mosquitoes
The 16S rRNA sequencing data can be analyzed to create microbial interaction networks, providing information on potential interaction patterns of microbes such as co-occurrence and co-exclusion. We created network maps of bacterial interactions using a variety of models that use presence/absence and relative abundance data to identify pairwise relationships (Faust and Raes, 2016). In general, we saw that interaction networks from Ae. aegypti were complex, in that they had more nodes and connections compared to networks from Ae. albopictus and Cx. quinquefasciatus (Figure 5 and Supplementary Table S4). For all mosquito species, both co-occurrence and co-exclusion interaction patterns were observed in all networks (examples of these patterns are shown in Supplementary Figure S6). We were able to identify taxa, or groups of bacteria, within these interactions that appear to be important to the overall structure of the network. For example, in the Ae. aegypti networks from field collected mosquitoes, Enterobacter and Pseudomonas are highly interconnected species having between 6 and 15 interactions in these networks. In the laboratory-reared Ae. aegypti, an Enterobacteriaceae had several interactions with other bacteria. Three-way interactions were also seen in many of the networks, and often these involved Pseudomonas and Enterobacter. Examples of tripartite co-occurrences interactions in Ae. aegypti networks include Pseudomonas, Asaia, and a Clostridium isolate in laboratory-reared mosquitoes, Pseudomonas, Serratia, and Enterobacter and Pseudomonas, Acidovorax, and Enterobacter in the G and BG groups, respectively. Common co-exclusionary interactions were found in the networks generated from field-collected mosquitoes such as Pseudomonas co-excluding Pantoea and Tatumella. Burkholderia co-excluded Enterobacter, Acidovorax, and Escherichia-Shigella, however, these Burkholderia interactions could possibly be an artifact due to extraction batch variation.
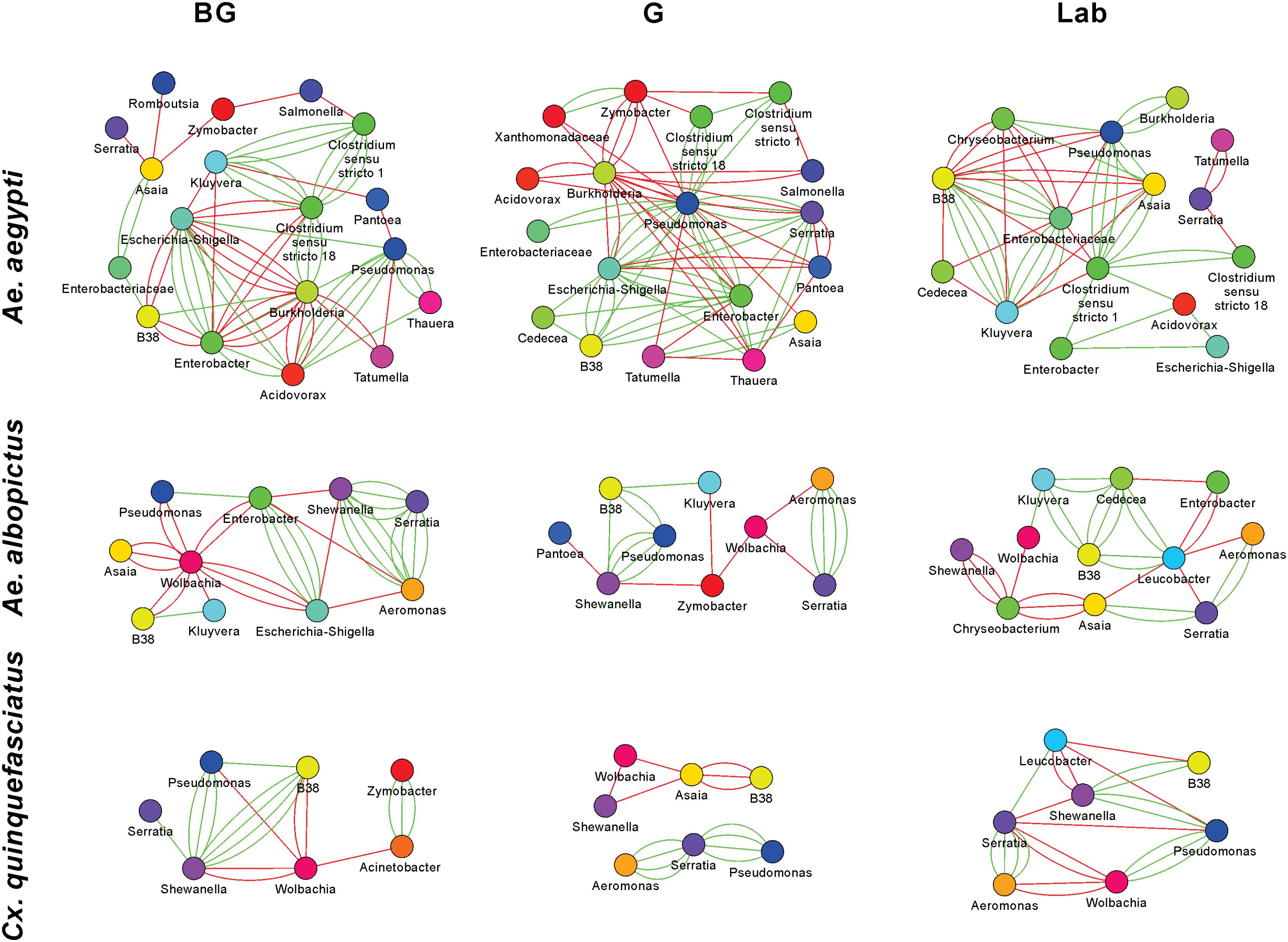
FIGURE 5. Microbial interaction networks for mosquitoes. Interaction networks were built using CoNet. Node colors represent unique taxonomy identifiers. Red edges represent co-exclusion/negative correlation, green edges represent co-occurrence/positive correlation interactions between relative abundance profiles. Multiple edges connecting the same nodes indicate significance from more than one metric (Bray-Curtis dissimilarity, Kullback-Leibler divergence, mutual information, Spearman correlation, and Pearson correlation). Undetermined interactions have been removed from the network. Wolbachia was removed from the Ae. aegypti G network given that infection could not be independently verified by PCR.
Wolbachia was a highly interconnected taxon in the interaction networks generated from Ae. albopictus and Cx. quinquefasciatus mosquitoes, and often had co-exclusionary relationship with other bacteria. In BG-collected Ae. albopictus, Wolbachia co-excluded six other bacteria including Asaia and Pseudomonas. In other groups, Wolbachia was seen to repeatedly exclude Aeromonas, Serratia, and Shewanella. The three-way interaction of Wolbachia co-excluding the co-occurring Aeromonas and Serratia was observed in both Ae. albopictus and Cx. quinquefasciatus. The Aeromonas and Serratia co-occurrence pattern appears highly robust and independent of environmental factors as this interaction was observed in five of the six Ae. albopictus and Cx. quinquefasciatus groups.
Artificial Infection of Symbionts in Germ-Free or Septic Mosquitoes
The microbial interaction networks highlight the multifarious interactions that occur within mosquito systems. We undertook preliminary validation experiments using Ae. aegypti larvae to further demonstrate that microbial interactions can influence microbiome composition and abundance. To this end, we exploited the recently developed gnotobiotic rearing approach where mosquito larvae can be infected with a single bacterial taxon (Coon et al., 2014). We compared the density and prevalence of artificially infected symbionts in gnotobiotic lines (infected with the single symbiont) compared to conventionally reared septic mosquitoes (that possessed their resident microbiota) (Figure 6). We completed this with six bacteria isolated from Aedes mosquitoes. Three of the bacteria were isolated from Ae. aegypti (Pantoea, Cedecea [Cedecea-aeg], Asaia) while another three were isolated from Ae. albopictus (Serratia, Cedecea [Cedecea-alb], and Enterobacter). Three bacteria (Serratia; Mann Whitney P < 0.0001, Cedecea-alb; Mann Whitney P < 0.0001, and Cedecea-aeg; Mann Whitney P < 0.0001) were observed to significantly infect Ae. aegypti mosquitoes at higher densities when inoculated into axenic rather than non-axenic mosquitoes. The prevalence (number of individuals infected) of Serratia (Fisher’s exact test, P < 0.0001) and Cedecea-alb (Fisher’s exact test, P < 0.0002) was also significantly higher in gnotobiotic compared to conventionally reared mosquitoes. No change in either the prevalence or density of infection was seen for Enterobacter, Pantoea, or Asaia. Taken together, these data indicate that microbial interactions influence colonization and infection dynamics of specific bacterial species within mosquitoes.
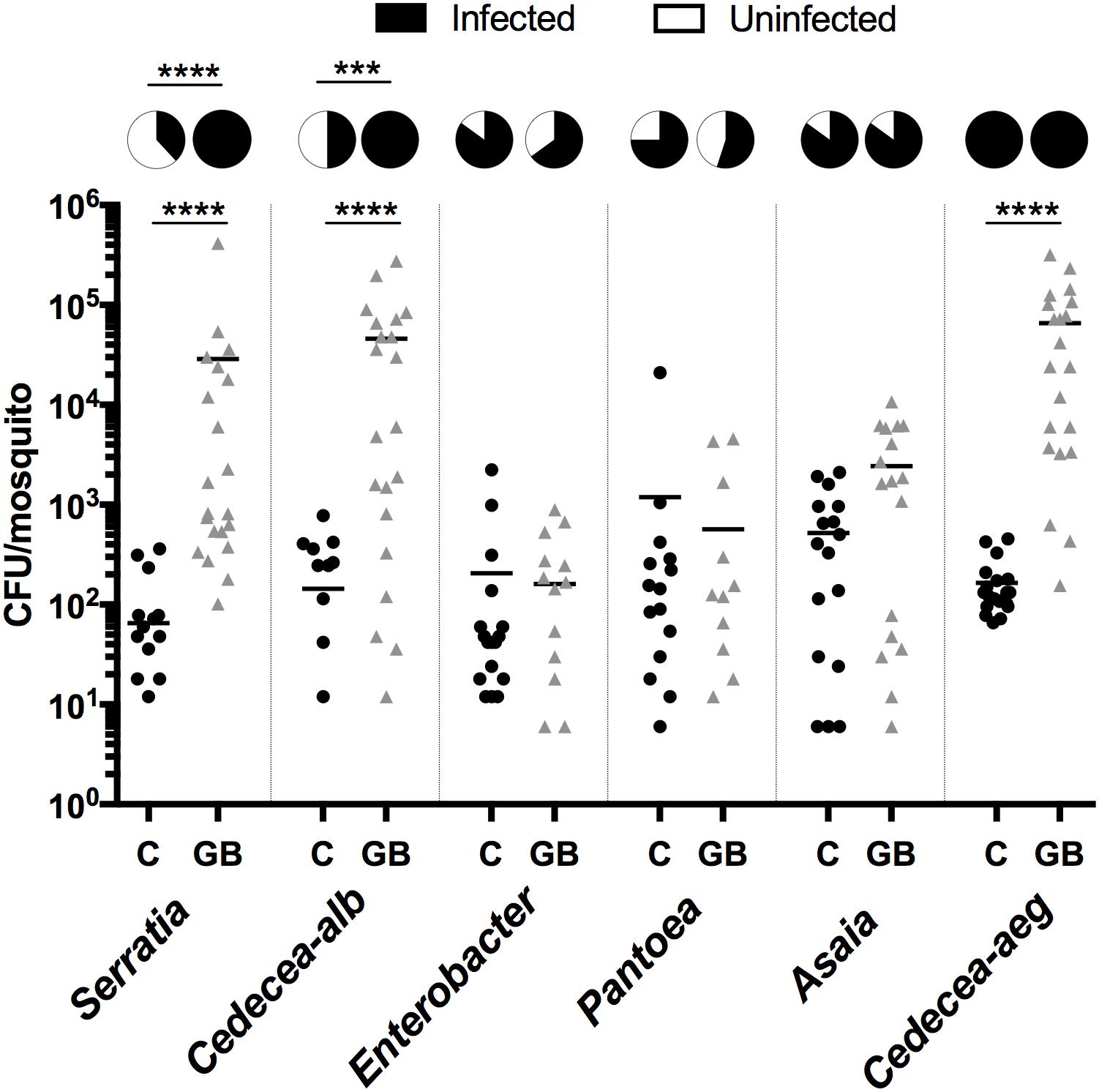
FIGURE 6. Infection density and prevalence of bacteria inoculated into conventionally reared or axenic mosquitoes. As such, gnotobiotic reared lines (GB) only possessed the inoculated bacteria while conventionally reared lines (C) possess their native microbiota in addition to the inoculated strain. Inoculated bacteria possessed a plasmid expressing antibiotic resistance and mCherry fluorescent protein. The bacterial load was quantified by counting mCherry colonies on selective plates. Serratia, Cedecea-alb, and Enterobacter were isolated from Ae. albopictus (Galveston) while Pantoae, Asaia and Cedecea-aeg were isolated from Ae. aegypti (Galveston). A Mann-Whitney test with a Dunn’s multiple comparison test was used to determine significance (∗∗∗∗P < 0.0001). Pie charts indicate prevalence of infection (Fisher’s exact test, ∗∗∗∗P < 0.0001, ∗∗∗P < 0.0002).
Discussion
It is clear that complex factors combine to shape the microbiome of an organism. To further increase our understanding of factors that affect the microbiome of mosquitoes, we sequenced the microbiome of laboratory-reared and field-caught adult mosquitoes exploiting traps that attract host- or oviposition-seeking individuals. Anautogenous mosquitoes require a blood meal to provide the necessary nutrition for egg development and this dramatic influx of blood has been shown to substantially alter the microbiome of laboratory-reared mosquitoes or those caught in the field and blood-fed in the laboratory (Kumar et al., 2010; Oliveira et al., 2011; Wang et al., 2011; Terenius et al., 2012). Intriguingly, we saw few differences in the microbiome between host- and oviposition-seeking mosquitoes. Across all species, there were no significant differences in alpha or beta diversity for mosquitoes caught in either trap. Similarly, the total bacterial load was similar between mosquitoes caught in the BG and G traps, and this was consistent across all three species. However, when comparing beta diversity between traps, we did see less variation in the groupings of individuals from both Ae. albopictus and Cx. quinquefasciatus in the G trap compared to the BG trap. Overall, our results are in contrast to studies using lab-reared mosquitoes or mosquitoes caught in the field and blood-fed in the laboratory that indicate increased bacterial load, but decreased diversity over a 24–48 h window following a blood meal (Kumar et al., 2010; Oliveira et al., 2011; Wang et al., 2011; Terenius et al., 2012).
Several factors could explain the differences between our results examining field-collected samples and those of previous studies (Kumar et al., 2010; Oliveira et al., 2011; Wang et al., 2011; Terenius et al., 2012). First, mosquitoes often take multiple blood meals, particularly Ae. aegypti (Scott et al., 1993). In our collections, the post-blood feeding history of mosquitoes is unknown, and it is possible that mosquitoes caught in either trap may have had a blood meal (or several) prior to being caught. The body of work examining the influence of blood feeding on the microbiome has only examined the effect of a single blood meal, not several, and while it appears the microbiome reverts to a pre-blood fed microbiome several days post-blood meal, it is unknown how quickly this occurs in the field. In field collected Anopheles mosquitoes reared in the laboratory, reversion to a Bacteroidetes-dominated microbiome, which was the dominant phylum seen in sugar fed mosquitoes, was seen 4 days post-blood meal (Wang et al., 2011). In our samples collected from G traps, females may have gone several days without a blood meal before finding a suitable oviposition site (i.e., the trap), possibly even relying on nectar-based food sources for sustenance. It is also possible that mosquitoes in search of an oviposition site may have never taken a blood meal. While most mosquitoes usually require a blood meal to develop eggs, autogeny has been reported in these species (Chambers and Klowden, 1994; Olejnícek and Gelbic, 2000; Ariani et al., 2015). Autogeny rates, which are variable and depend on temperature and nutrition, have been reported to range from 3 to 34% for Ae. aegypti, around 5% for Ae. albopictus, and up to 87% in Culex mosquitoes (Trpis, 1977; Chambers and Klowden, 1994; Olejnícek and Gelbic, 2000; Ariani et al., 2015). Little is known regarding the influence of microbiota and autogeny, although in the autogenous mosquito Ae. atropalpus, specific gut taxa have been shown to influence egg production (Coon et al., 2016a). Finally, while reports indicate that BG and G traps preferential catch mosquitoes in different physiological states (Reiter et al., 1986; Maciel-de-Freitas et al., 2006; Dennett et al., 2007; Figuerola et al., 2012), we did not explicitly examine if females were gravid or not. While we contemplated dissecting mosquitoes to examine their parity, we chose not to, as it would increase the potential for contamination of the samples. Although in our work we did not see dramatic differences in the mosquitoes caught in BG or G traps, the earlier points highlight the challenges in undertaking studies on field-derived samples and could explain the disparity between our results and those from studies undertaken in laboratory settings.
Since both Ae. albopictus and Cx. quinquefasciatus are heavily infected with Wolbachia, we analyzed our data with and without this endosymbiont to garner a better understanding of the other bacterial microbiota in these mosquitoes. Our high throughput sequencing and qPCR results demonstrated that Wolbachia was the most abundant bacterium in Ae. albopictus and Cx. quinquefasciatus. In spite of this, we obtained sufficient sequencing depth to identify other bacterial taxa – a common challenge when characterizing Wolbachia-infected species using amplicon sequencing (Minard et al., 2014). Removing Wolbachia from our analysis increased the Shannon diversity index, indicating the remaining microbiota within these mosquitoes is relatively even. For beta diversity, we found that our results were mixed and dependent on group. When comparing within a group, removing Wolbachia made the microbiomes of laboratory-reared Ae. albopictus and Cx. quinquefasciatus more divergent while the microbiomes of field mosquitoes tended to be less different. These findings are consistent with a study by Novakova et al. (2017) that found the removal of Wolbachia from their analysis led to less distinct differences for field-collected mosquitoes. When comparing groups within a species, exclusion of Wolbachia made the comparison of field and laboratory mosquitoes more distinct, likely due to differences in the gut-associated microbiota. Similar to a recently published study (Coon et al., 2016b), we found a small number of Wolbachia reads in Ae. aegypti mosquitoes collected in G traps. However, we could not confirm the presence of the bacteria with conventional PCR-based approaches, suggesting these results could be a sequencing or laboratory artifact. A recent PCR-based screening of Ae. aegypti mosquitoes found no evidence for Wolbachia infection (Gloria-Soria et al., 2018), although cryptic low titer infections may be beyond the detection threshold of conventional PCR. Should populations of Ae. aegypti be naturally infected with Wolbachia, this could have important ramifications for biological control strategies being implemented into the field (Bourtzis et al., 2014; Hughes and Rasgon, 2014; Flores and O’Neill, 2018), and as such, further research in this area is warranted.
Similar to findings in other mosquito species, many bacteria were shared between different mosquito species, and laboratory-reared mosquitoes were seen to have a divergent microbiome from their field counterparts (Boissière et al., 2012; Coon et al., 2016b). These common taxa, particularly those that infect at high abundance, could be candidate bacteria for consideration in novel pan-mosquito microbial control strategies as they would likely be compatible for all three vector species (Saldana et al., 2017). When focusing specifically on bacterial titers, few bacteria were seen to be significantly different between mosquitoes caught in either traps. In Culex mosquitoes, Pantoea was more abundant in individuals caught in the G trap. Similar to this finding, Pantoea has been found to increase in abundance after a blood meal in Anopheles mosquitoes (Wang et al., 2012). When considering pairwise comparisons between species, there were more differentially abundant genera when comparing Ae. aegypti to the other two species, which explains the greater divergence of the Ae. aegypti microbiome to the microbiome of the other two species. The Ae. aegypti samples were collected from a different location than the other two species, thus, it is possible that environmental factors could explain these differences. However, we also saw common changes that were consistent regardless of groups (BG, G and Laboratory), such as Aeromonas, Clostridium, Serratia, Shewanella, and Wolbachia, indicating these bacteria were not greatly influenced by the environment and that other factors affected their presence in the particular mosquito species. Notably, we found that in laboratory-reared mosquitoes, Culex harbored significantly higher titers of Serratia and Aeromonas, compared to Ae. albopictus, while these bacteria were at low abundance or absent from Ae. aegypti. These results suggest there are host and/or bacterial related factors that make this particular strain of Ae. aegypti (Galveston) inhospitable for Serratia and Aeromonas as all three mosquito species were subjected to similar uniform environmental conditions when reared in the insectary.
Current evidence of microbial interactions within mosquitoes is mainly limited to interactions between Wolbachia and other microbiota (Hughes et al., 2014; Rossi et al., 2015; Zink et al., 2015; Audsley et al., 2017a), or between vertically transmitted symbionts in other arthropod systems (Macaluso et al., 2002; Kondo et al., 2005; Goto et al., 2006; Rock et al., 2017). As such, our understanding is generally restricted to inherited symbionts and we have a poor understanding of the scale of interactions between microbes that are associated with insect guts. To address this, we created microbial interaction networks to identify pairwise co-occurrence and co-exclusion patterns. To avoid spurious interactions, which could be due to the presence or absence of a microbe in one environmental condition but not another, we limited our network analysis to within a group for each particular species. Our analysis identified 116 co-occurrence or co-exclusion interactions, substantially increasing the number of bacterial interactions observed in mosquitoes. Bacterial interaction networks generated from Ae. aegypti mosquitoes were more complex than Ae. albopictus or Cx. quinquefasciatus in that they had more nodes and connections. Species richness may explain the differences observed in network structure, as in general, the more complex networks had a greater number of OTUs. Other factors that may have influenced the identification of interacting bacteria are the presence of Wolbachia in Ae. albopictus and Cx. quinquefasciatus, as well as the differences in sample size between mosquito species. Further studies are warranted to determine why some networks are highly interconnected while others are not. Approaches that artificially manipulate the microbiome composition of a mosquito may be particularly useful in this regard.
Interestingly, in the more complex networks, we saw evidence of hub microbial taxa that were highly interconnected. Pseudomonas and bacteria within the Enterobacteriacaea appear to be important hub taxa. Some of the interactions observed here have been previously reported in Ae. triseriatus and Ae. japonicus (Muturi et al., 2016a), including negative interactions between bacteria within the Burkholderiaceae and Pseudomonas and Acinetobacter as well as Asaia-Enterobacter and Asaia-Ralstonia interactions. The majority of interactions reported in Ae. triseriatus and Ae. japonicus were negative (Muturi et al., 2016a), whereas here we see a mix of both co-occurrence and co-exclusion patterns. Specific hub microbes that are strongly interconnected have been found in plant microbiomes and these taxa have a profound effect on overall microbiome structure (Agler et al., 2016). In our data, we also saw three-way interactions. Often these relationships were co-occurring, or were formed by two co-occurring bacteria both co-exclude another bacterium, suggesting the existence of multi-taxa interactions. Further work is required to determine the functionality of these multi-interacting partners and if these interactions represent keystone guilds (Banerjee et al., 2018). The identification of common interaction pairings across several groups indicates that these interactions, and the methods we employed to identify them, are robust and presumably not influenced by physiological state or other environmental conditions.
It is important to highlight that these network maps represent patterns, and not direct interactions. Many of the observed interactions may be due to microbes sharing a similar ecological niche, and a substantial challenge, particularly for highly interconnected taxa, will be to investigate these interactions further. To undertake initial validation steps and to demonstrate that microbial interactions are an important factor influencing the colonization of gut-associated microbiota, we infected six culturable bacterial taxa into Ae. aegypti larvae that either possessed or lacked their resident microbiota. We used larval infections, rather than adults, as we could exploit the use of the gnotobiotic infection procedure in this life stage (Coon et al., 2014). Serratia and Cedecea, which were isolated from Ae. albopictus, poorly infected Ae. aegypti when it possessed its native microbiome. However, when mosquitoes lacked their native microbiota, these bacteria infected at a higher titer. Even more striking was the effect on prevalence (number of individuals infected), which changed from 38 and 50% for Serratia and Cedecea-alb, respectively, in conventionally reared mosquitoes that possessed their resident microbiota, to 100% infection when infected into axenic larvae. This indicated that microbial incompatibility, rather than host or bacterial genetics, was a major factor causing the poor infection rates of mosquitoes. The ability of Serratia and Cedecea-alb to infect some conventionally reared mosquitoes that possessed their native microbiota could be due to variation in the microbiome between individuals, which is likely caused by stochastic processes. In our networks, Serratia and Cedecea have several co-exclusionary relationships with dominant bacterial taxa such as Asaia, Pseudomonas, and Enterobacter, which may explain these results, however specific examination of these interactions in adult mosquitoes is required. Importantly, not all of the bacterial taxa artificially infected into larvae increased in prevalence and density suggesting these enhanced colonization effects are not simply due to mosquitoes lacking their microbiota, but rather are specific for each taxa, likely due to specific microbial interactions. Similar to our findings, it has been reported that antibiotic treatment prior to bacterial supplementation in a sugar meal can increase the prevalence of infection of gut microbes in female Anopheles and Aedes mosquitoes (Ramirez et al., 2014), indicating that resident gut bacteria that are susceptible to antibiotics are antagonistic to the supplemented bacterium. It is important to note that our reinfection study, which exploited the gnotobiotic rearing system, examined interactions in larvae, not adults, and that differences in the gut morphology and function between these two life stages may alter microbial interactions (Engel and Moran, 2013). However, our findings combined with the work of Ramirez et al. (2014), suggest that microbial interactions between gut-associated bacteria occur within mosquitoes and influence symbiont colonization in aquatic and adult life stages, which likely affects microbiome species richness and evenness. These colonization traits and co-exclusionary associations could offer a possible explanation for the variability seen in the mosquito microbiome between individuals, as bacteria that initially infect the gut may impede colonization by other microbes.
In this study, we compared microbial interaction networks from field and laboratory mosquitoes to examine the influence of the general environment but it is possible other factors may influence network structure. In particular, it would be interesting to determine if microbial networks differ between tissues within mosquitoes, such as the salivary glands, germline, and the gut. For example, microbial network analysis from the human microbiome project found strong niche specialization in their networks, whereby different body sites had contrasting microbial networks (Faust et al., 2012). In Anopheles mosquitoes, salivary glands have a more diverse microbiome compared to the gut (Sharma et al., 2014), and it is conceivable that elevated species richness would allow for greater network interactions. Furthermore, the germlines of male and female Anopheles mosquitoes share some common taxa but there are also quantitative differences (Segata et al., 2016). These differing microbial niches could be exploited to determine the influence of species evenness on microbial networks. Performing network analysis on gut samples of Ae. albopictus and Cx. quinquefasciatus may also overcome any issue with Wolbachia sequestering the majority of the reads, as Wolbachia primarily resides within the germline in these mosquito species. Alternatively, approaches that reduce amplification of Wolbachia could be implemented (Simhadri et al., 2017). Here, we assessed whole mosquitoes to give an initial overall picture of microbial interactions, but analysis of distinct tissues may identify interactions of bacteria that are proximal to one another, and these interactions are more likely to reflect true microbe-microbe interactions, rather than patterns associated with environmental exposure. Within a species we collected mosquitoes from a single site, but future studies examining interaction networks should incorporate diverse sites. Common pairwise interactions identified across sites would indicate robust relationships not influenced by environmental factors.
In summary, we examined the microbiome of three important mosquito vectors, Ae. aegypti, Ae. albopictus, and Cx. quinquefasciatus. While the overall microbiome structure between host-seeking or ovipositing females was similar, we identified specific bacteria that changed in abundance between mosquito species. Our analysis identified a suite of pairwise interactions used for generating microbial interaction networks, and together with re-infection studies we have demonstrated that microbial interactions affect microbiome composition and abundance of specific bacterial taxa. These findings add to our understanding of microbiome community structure of mosquitoes and factors that influence microbiome acquisition and maintenance in these important disease vectors.
Data Accessibility
The NCBI accession number for the raw sequencing data reported here is PRJNA422599.
Author Contributions
SH, KK, LA, JD, EH, YF, and GH designed the experiments. GM, CF, JD, and MD oversaw the fieldwork. SH and MP completed the experiments. KK, MR, LA, SH, EH, and GH undertook analysis. SH, KK, JD, MD, and GH wrote and edited the manuscript. All authors agreed to the final version. YF and GH acquired funding and supervised the work.
Funding
GH is supported by NIH grants (R21AI138074, R21AI124452, and R21AI129507), a University of Texas Rising Star award, the John S. Dunn Foundation Collaborative Research Award, the Robert J. Kleberg, Jr. and Helen C. Kleberg Foundation, and the Centers for Disease Control and Prevention (CDC) (Cooperative Agreement Number U01CK000512). The paper’s contents are solely the responsibility of the authors and do not necessarily represent the official views of the CDC or the Department of Health and Human Services. This work was also supported by a James W. McLaughlin postdoctoral fellowship at the University of Texas Medical Branch to SH, a NIH T32 fellowship (2T32AI007526) to MS and a Marie Curie Fellowship (within the 7th European Community Framework Programme; project no. 330136) to EH.
Conflict of Interest Statement
The authors declare that the research was conducted in the absence of any commercial or financial relationships that could be construed as a potential conflict of interest.
Acknowledgments
We thank the UTMB insectary core for providing the laboratory mosquitoes.
Supplementary Material
The Supplementary Material for this article can be found online at: https://www.frontiersin.org/articles/10.3389/fmicb.2018.02160/full#supplementary-material
FIGURE S1 | Map of Houston, Texas, indicating the field collection sites.
FIGURE S2 | Shannon entropy rarefied at intervals between 0 and 100,000 reads in each sample from different groups (G, BG, Laboratory) in all three mosquito species.
FIGURE S3 | Heat maps indicating bacterial relative abundance for the three mosquito species. OTUs were grouped to genus level or higher ranks (when genus was ambiguous) and the relative abundance indicated by color for each individual (column) is shown. The upper heat map is with Wolbachia present while the lower has Wolbachia excluded. The dendrogram/clustering of the bacteria is generated based on their relative abundance correlation across samples.
FIGURE S4 | Shannon diversity of Ae. albopictus and Cx. quinquefasciatus with and without Wolbachia. For analysis of samples without the endosymbiont, Wolbachia reads were computationally excluded from the analysis and then Shannon diversity was recalculated (∗P < 0.05, ∗∗P < 0.01, ∗∗∗∗P < 0.0001).
FIGURE S5 | Relative abundance of Serratia and Aeromonas from high-throughput sequencing in Cx. quinquefasciatus, Ae. albopictus and Ae. aegypti mosquitoes reared in the lab. Data were analyzed using a one-way ANOVA using Tukeys method for pairwise comparisons (∗∗∗∗P < 0.0001).
FIGURE S6 | Examples of co-occurrence and co-exclusion microbial pairs identified in the interaction networks. Scatterplots of relative abundance profiles displaying statistically significant co-occurrence and co-exclusion patterns in mosquito groups. Points represent the relative abundance values of the pair in each sample.
TABLE S1 | Number of samples used in the study and their division into groups by metadata.
TABLE S2 | Complete and filtered to 0.1% OTU table with read counts from each individual mosquito (Ae. aegypti, Ae. albopictus, and Cx. quinquefasciatus). Each library was constructed from a single female mosquito.
TABLE S3 | List of the bacterial taxa present or absent within each species and group which was used to create Venn diagrams (Figure 3A).
TABLE S4 | OTU tables (relative abundance profiles) used in CoNet analysis. Relative abundance of each OTU in a sample was calculated by dividing number of reads by total number of reads of the sample and OTUs with read counts below 0.1% across all mosquito samples were excluded from analysis. OTU relative abundance profiles were summed based on lowest common taxonomy, down to genus level. When building networks for each mosquito group (Ae. aegypti BG, G or Lab, Ae. albopictus BG, G or Lab, or Cx. quinquefasciatus BG, G or Lab), abundance profiles present (non-zero abundance) in less than five samples were excluded from analysis.
Footnotes
References
Agler, M. T., Ruhe, J., Kroll, S., Morhenn, C., Kim, S.-T., Weigel, D., et al. (2016). Microbial hub taxa link host and abiotic factors to plant microbiome variation. PLoS Biol. 14:e1002352. doi: 10.1371/journal.pbio.100 2352
Anderson, M. J. (2014). Permutational Multivariate Analysis of Variance (PERMANOVA), 3rd Edn. Chichester: John Wiley & Sons, Ltd, doi: 10.1002/9781118445112.stat07841
Ariani, C. V., Smith, S. C. L., Osei-Poku, J., Short, K., Juneja, P., and Jiggins, F. M. (2015). Environmental and genetic factors determine whether the mosquito Aedes aegypti lays eggs without a blood meal. Am. J. Trop. Med. Hyg. 92, 715–721. doi: 10.4269/ajtmh.14-0471
Audsley, M. D., Seleznev, A., Joubert, D. A., Woolfit, M., O’Neill, S. L., and McGraw, E. A. (2017a). Wolbachia infection alters the relative abundance of resident bacteria in adult Aedes aegypti mosquitoes, but not larvae. Mol. Ecol. 27, 297–309. doi: 10.1111/mec.14436
Audsley, M. D., Ye, Y. H., and McGraw, E. A. (2017b). The microbiome composition of Aedes aegypti is not critical for Wolbachia-mediated inhibition of dengue virus. PLoS Negl. Trop. Dis. 11:e0005426. doi: 10.1371/journal.pntd.0005426
Baldo, L., Hotopp, J. C. D., Jolley, K. A., Bordenstein, S. R., Biber, S. A., Choudhury, R. R., et al. (2006). Multilocus sequence typing system for the endosymbiont Wolbachia pipientis. Appl. Environ. Microbiol. 72, 7098–7110. doi: 10.1128/AEM.00731-06
Banerjee, S., Schlaeppi, K., and van der Heijden, M. G. A. (2018). Keystone taxa as drivers of microbiome structure and functioning. Nat. Rev. Microbiol. 15, 579. doi: 10.1038/s41579-018-0024-1
Barberán, A., Bates, S. T., Casamayor, E. O., and Fierer, N. (2012). Using network analysis to explore co-occurrence patterns in soil microbial communities. ISME J. 6, 343–351. doi: 10.1038/ismej.2011.119
Boissière, A., Tchioffo, M. T., Bachar, D., Abate, L., Marie, A., Nsango, S. E., et al. (2012). Midgut microbiota of the malaria mosquito vector Anopheles gambiae and Interactions with Plasmodium falciparum infection. PLoS Pathog. 8:e1002742. doi: 10.1371/journal.ppat.1002742
Bourtzis, K., Dobson, S. L., Xi, Z., Rasgon, J. L., Calvitti, M., Moreira, L. A., et al. (2014). Harnessing mosquito-Wolbachia symbiosis for vector and disease control. Acta Trop. 132(Suppl.), S150–S163. doi: 10.1016/j.actatropica.2013.11.004
Bray, J. R., and Curtis, J. T. (1957). An ordination of the upland forest communities of Southern Wisconsin. Ecol. Monogr. 27, 325–349. doi: 10.2307/1942268
Buck, M., Nilsson, L. K. J., Brunius, C., Dabiré, R. K., Hopkins, R., and Terenius, O. (2016). Bacterial associations reveal spatial population dynamics in Anopheles gambiae mosquitoes. Sci. Rep. 6:22806. doi: 10.1038/srep22806
Burkhardt, N. Y., Baldridge, G. D., Williamson, P. C., Billingsley, P. M., Heu, C. C., Felsheim, R. F., et al. (2011). Development of shuttle vectors for transformation of diverse Rickettsia species. PLoS One 6:e29511. doi: 10.1371/journal.pone.0029511
Calvitti, M., Marini, F., Desiderio, A., Puggioli, A., and Moretti, R. (2015). Wolbachia density and cytoplasmic incompatibility in Aedes albopictus: concerns with using artificial Wolbachia infection as a vector suppression tool. PLoS One 10:e0121813. doi: 10.1371/journal.pone.0121813
Casiraghi, M., Bain, O., Guerrero, R., Martin, C., Pocacqua, V., Gardner, S. L., et al. (2004). Mapping the presence of Wolbachia pipientis on the phylogeny of filarial nematodes: evidence for symbiont loss during evolution. Int. J. Parasitol. 34, 191–203. doi: 10.1016/j.ijpara.2003.10.004
Chaffron, S., Rehrauer, H., Pernthaler, J., and von Mering, C. (2010). A global network of coexisting microbes from environmental and whole-genome sequence data. Genome Res. 20, 947–959. doi: 10.1101/gr.104521.109
Chambers, G. M., and Klowden, M. J. (1994). Nutritional reserves of autogenous and anautogenous selected strains of Aedes albopictus (Diptera: Culicidae). J. Med. Entomol. 31, 554–560. doi: 10.1093/jmedent/31.4.554
Chen, S., Bagdasarian, M., and Walker, E. D. (2015). Elizabethkingia anophelis: molecular manipulation and interactions with mosquito hosts. Appl. Environ. Microbiol. 81, 2233–2243. doi: 10.1128/AEM.03733-14
Coon, K. L., Brown, M. R., and Strand, M. R. (2016a). Gut bacteria differentially affect egg production in the anautogenous mosquito Aedes aegypti and facultatively autogenous mosquito Aedes atropalpus (Diptera: Culicidae). Parasit. Vectors 9:375. doi: 10.1186/s13071-016-1660-9
Coon, K. L., Brown, M. R., and Strand, M. R. (2016b). Mosquitoes host communities of bacteria that are essential for development but vary greatly between local habitats. Mol. Ecol. 25, 5806–5826. doi: 10.1111/mec.13877
Coon, K. L., Vogel, K. J., Brown, M. R., and Strand, M. R. (2014). Mosquitoes rely on their gut microbiota for development. Mol. Ecol. 23, 2727–2739. doi: 10.1111/mec.12771
David, M. R., Santos, L. M. B. D., Vicente, A. C. P., and Maciel-de-Freitas, R. (2016). Effects of environment, dietary regime and ageing on the dengue vector microbiota: evidence of a core microbiota throughout Aedes aegypti lifespan. Mem. Inst. Oswaldo Cruz 111, 577–587. doi: 10.1590/0074-02760160238
Demaio, J., Pumpuni, C. B., Kent, M., and Beier, J. C. (1996). The midgut bacterial flora of wild Aedes triseriatus, Culex pipiens, and Psorophora columbiae mosquitoes. Am. J. Trop. Med. Hyg. 54, 219–223. doi: 10.4269/ajtmh.1996.54.219
Dennett, J. A., Vessey, N. Y., and Parsons, R. E. (2004). A comparison of seven traps used for collection of Aedes albopictus and Aedes aegypti originating from a large tire repository in Harris County (Houston), Texas. J. Am. J. Am. Mosq. Control Assoc. 20, 342–349.
Dennett, J. A., Wuithiranyagool, T., Reyna-Nava, M., Bala, A., Tesh, R. B., Parsons, I. R. E., et al. (2007). Description and use of the Harris County gravid trap for West Nile virus surveillance 2003-06. J. Am. Mosq. Control Assoc. 23, 359–362. doi: 10.2987/8756-971X(2007)23[359:DAUOTH]2.0.CO;2
Dennison, N. J., Jupatanakul, N., and Dimopoulos, G. (2014). The mosquito microbiota influences vector competence for human pathogens. Curr. Opin. Insect. Sci. 3, 6–13. doi: 10.1016/j.cois.2014.07.004
Dennison, N. J., Saraiva, R. G., Cirimotich, C. M., Mlambo, G., Mongodin, E. F., and Dimopoulos, G. (2016). Functional genomic analyses of Enterobacter, Anopheles and Plasmodium reciprocal interactions that impact vector competence. Malar. J. 15:425. doi: 10.1186/s12936-016-1468-2
Dickson, L. B., Jiolle, D., Minard, G., Moltini-Conclois, I., Volant, S., Ghozlane, A., et al. (2017). Carryover effects of larval exposure to different environmental bacteria drive adult trait variation in a mosquito vector. Sci. Adv. 3:e1700585. doi: 10.1126/sciadv.1700585
Duguma, D., Rugman-Jones, P., Kaufman, M. G., Hall, M. W., Neufeld, J. D., Stouthamer, R., et al. (2013). Bacterial communities associated with Culex mosquito larvae and two emergent aquatic plants of bioremediation importance. PLoS One 8:e72522. doi: 10.1371/journal.pone.0072522
Engel, P., and Moran, N. A. (2013). The gut microbiota of insects - diversity in structure and function. FEMS Microbiol. Rev. 37, 699–735. doi: 10.1111/1574-6976.12025
Faust, K., and Raes, J. (2012). Microbial interactions: from networks to models. Nat. Rev. Microbiol. 10, 538–550. doi: 10.1038/nrmicro2832
Faust, K., and Raes, J. (2016). CoNet app: inference of biological association networks using Cytoscape. F1000Res 5:1519. doi: 10.12688/f1000research.9050.2
Faust, K., Sathirapongsasuti, J. F., Izard, J., Segata, N., Gevers, D., Raes, J., et al. (2012). Microbial co-occurrence relationships in the human microbiome. PLoS Comput. Biol. 8:e1002606. doi: 10.1371/journal.pcbi.1002606
Figuerola, J., Muñoz, J., Soriguer, R., Roussel, M., Roiz, D., and Ruiz, S. (2012). Efficacy of mosquito traps for collecting potential West Nile mosquito vectors in a natural Mediterranean wetland. Am. J. Epidemiol. 86, 642–648. doi: 10.4269/ajtmh.2012.11-0326
Flores, H. A., and O’Neill, S. L. (2018). Controlling vector-borne diseases by releasing modified mosquitoes. Nat. Rev. Microbiol. 33:330. doi: 10.1038/s41579-018-0025-0
Gimonneau, G., Tchioffo, M. T., Abate, L., Boissière, A., Awono-Ambene, P. H., Nsango, S. E., et al. (2014). Composition of Anopheles coluzzii and Anopheles gambiae microbiota from larval to adult stages. Infect. Genet. Evol. 28, 715–724. doi: 10.1016/j.meegid.2014.09.029
Gloor, G. B., Macklaim, J. M., Pawlowsky-Glahn, V., and Egozcue, J. J. (2017). Microbiome datasets are compositional: and this is not optional. Front. Microbiol. 8:2224. doi: 10.3389/fmicb.2017.02224
Gloria-Soria, A., Chiodo, T. G., and Powell, J. R. (2018). Lack of evidence for natural Wolbachia infections in Aedes aegypti (Diptera: Culicidae). J. Med. Entomol. 7:e1002415. doi: 10.1093/jme/tjy084
Gonçalves, C. M., Melo, F. F., Bezerra, J. M. T., Chaves, B. A., Silva, B. M., Silva, L. D., et al. (2014). Distinct variation in vector competence among nine field populations of Aedes aegypti from a Brazilian dengue-endemic risk city. Parasit. Vectors 7:320. doi: 10.1186/1756-3305-7-320
Goodrich, J. K., Waters, J. L., Poole, A. C., Sutter, J. L., Koren, O., Blekhman, R., et al. (2014). Human genetics shape the gut microbiome. Cell 159, 789–799. doi: 10.1016/j.cell.2014.09.053
Goto, S., Anbutsu, H., and Fukatsu, T. (2006). Asymmetrical interactions between Wolbachia and Spiroplasma endosymbionts coexisting in the same insect host. Appl. Environ. Microbiol. 72, 4805–4810. doi: 10.1128/AEM.00416-06
Gusmão, D. S., Santos, A. V., Marini, D. C., Bacci, M., Berbert-Molina, M. A., and Lemos, F. J. A. (2010). Culture-dependent and culture-independent characterization of microorganisms associated with Aedes aegypti (Diptera: Culicidae) (L.) and dynamics of bacterial colonization in the midgut. Acta Trop 115, 275–281. doi: 10.1016/j.actatropica.2010.04.011
Gusmão, D. S., Santos, A. V., Marini, D. C., Russo Ede, S., Peixoto, A. M. D., Bacci Júnior, M., et al. (2007). First isolation of microorganisms from the gut diverticulum of Aedes aegypti (Diptera: Culicidae): new perspectives for an insect-bacteria association. Mem. Inst. Oswaldo Cruz 102, 919–924. doi: 10.1590/S0074-02762007000800005
Hegde, S., Rasgon, J. L., and Hughes, G. L. (2015). The microbiome modulates arbovirus transmissionin mosquitoes. Curr. Opin. Virol. 15, 97–102. doi: 10.1016/j.coviro.2015.08.011
Hughes, G. L., Dodson, B. L., Johnson, R. M., Murdock, C. C., Tsujimoto, H., Suzuki, Y., et al. (2014). Native microbiome impedes vertical transmission of Wolbachia in Anopheles mosquitoes. PNAS 111, 12498–12503. doi: 10.1073/pnas.1408888111
Hughes, G. L., and Rasgon, J. L. (2014). Transinfection: a method to investigate Wolbachia-host interactions and control arthropod-borne disease. Insect. Mol. Biol. 23, 141–151. doi: 10.1111/imb.12066
Isoe, J., Collins, J., Badgandi, H., Day, W. A., and Miesfeld, R. L. (2011). Defects in coatomer protein I (COPI) transport cause blood feeding-induced mortality in Yellow Fever mosquitoes. PNAS 108, E211–E217. doi: 10.1073/pnas.1102637108
Iturbe-Ormaetxe, I., Walker, T., and Neill, S. L. O. (2011). Wolbachia and the biological control of mosquito-borne disease. EMBO Rep. 12, 508–518. doi: 10.1038/embor.2011.84
Jadin, J., Vincke, I. H., Dunjic, A., Delville, J. P., Wery, M., Bafort, J., et al. (1966). Role of Pseudomonas in the sporogenesis of the hematozoon of malaria in the mosquito. Bull. Soc. Pathol. Exot. Filiales 59, 514–525.
Jupatanakul, N., Sim, S., and Dimopoulos, G. (2014). The insect microbiome modulates vector competence for arboviruses. Viruses 6, 4294–4313. doi: 10.3390/v6114294
Klindworth, A., Pruesse, E., Schweer, T., Peplies, J., Quast, C., Horn, M., et al. (2012). Evaluation of general 16S ribosomal RNA gene PCR primers for classical and next-generation sequencing-based diversity studies. Nucleic Acids Res. 41, e1. doi: 10.1093/nar/gks808
Kondo, N., Shimada, M., and Fukatsu, T. (2005). Infection density of Wolbachia endosymbiont affected by co-infection and host genotype. Biol. Lett. 1, 488–491. doi: 10.1098/rsbl.2005.0340
Kullback, S., and Leibler, R. A. (1951). On information and sufficiency. Ann. Math. Stat. 22, 79–86. doi: 10.1016/j.transproceed.2017.12.043
Kumar, S., Molina-Cruz, A., Gupta, L., Rodrigues, J., and Barillas-Mury, C. (2010). A peroxidase/dual oxidase system modulates midgut epithelial immunity in Anopheles gambiae. Science 327, 1644–1648. doi: 10.1126/science.1184008
Lindh, J. M., Borg-Karlson, A.-K., and Faye, I. (2008). Transstadial and horizontal transfer of bacteria within a colony of Anopheles gambiae (Diptera: Culicidae) and oviposition response to bacteria-containing water. Acta Trop. 107, 242–250. doi: 10.1016/j.actatropica.2008.06.008
Macaluso, K. R., Sonenshine, D. E., Ceraul, S. M., and Azad, A. F. (2002). Rickettsial infection in Dermacentor variabilis (Acari: Ixodidae) inhibits transovarial transmission of a second Rickettsia. J. Med. Entomol. 39, 809–813. doi: 10.1603/0022-2585-39.6.809
Maciel-de-Freitas, R., Eiras, A. E., and Lourenco-De-Oliveira, R. (2006). Field evaluation of effectiveness of the BG-Sentinel, a new trap for capturing adult Aedes aegypti (Diptera: Culicidae). Mem. Inst. Oswaldo Cruz 101, 321–325. doi: 10.1590/S0074-02762006000300017
Mandal, S., Van Treuren, W., White, R. A., Eggesbø, M., Knight, R., and Peddada, S. D. (2015). Analysis of composition of microbiomes: a novel method for studying microbial composition. Microbial Ecol. Health Dis. 26:7663. doi: 10.3402/mehd.v26.27663
Minard, G., Tran, F. H., Dubost, A., Tran-Van, V., Mavingui, P., and Moro, C. V. (2014). Pyrosequencing 16S rRNA genes of bacteria associated with wild tiger mosquito Aedes albopictus: a pilot study. Front. Cell Infect. Microbiol. 4:59. doi: 10.3389/fcimb.2014.00059
Minard, G., Tran, F.-H., Van Tran Van, Goubert, C., Bellet, C., Lambert, G., et al. (2015). French invasive Asian tiger mosquito populations harbor reduced bacterial microbiota and genetic diversity compared to Vietnamese autochthonous relatives. Front. Microbiol. 6:970. doi: 10.3389/fmicb.2015.00970
Muturi, E. J., Bara, J. J., Rooney, A. P., and Hansen, A. K. (2016a). Midgut fungal and bacterial microbiota of Aedes triseriatus and Aedes japonicus shift in response to La Crosse virus infection. Mol. Ecol. 25, 4075–4090. doi: 10.1111/mec.13741
Muturi, E. J., Kim, C.-H., Bara, J., Bach, E. M., and Siddappaji, M. H. (2016b). Culex pipiens and Culex restuans mosquitoes harbor distinct microbiota dominated by few bacterial taxa. Parasit Vectors 9:18. doi: 10.1186/s13071-016-1299-6
Muturi, E. J., Ramirez, J. L., Rooney, A. P., and Kim, C.-H. (2017). Comparative analysis of gut microbiota of mosquito communities in central Illinois. PLoS Negl. Trop. Dis. 11:e0005377. doi: 10.1371/journal.pntd.0005377
Novakova, E., Woodhams, D. C., Rodríquez-Ruano, S. M., Brucker, R. M., Leff, J. W., Maharaj, A., et al. (2017). Mosquito microbiome dynamics, a background for prevalence and seasonality of West Nile virus. Front. Microbiol. 8:526. doi: 10.3389/fmicb.2017.00526
Olejnícek, J., and Gelbic, I. (2000). Autogeny in Culex pipiens quinquefasciatus say. J. Vector Ecol. 25, 118–122.
Oliveira, J. H. M., Gonçalves, R. L. S., Lara, F. A., Dias, F. A., Gandara, A. C. P., Menna-Barreto, R. F. S., et al. (2011). Blood meal-derived heme decreases ROS levels in the midgut of Aedes aegypti and allows proliferation of intestinal microbiota. PLoS Pathog. 7:e1001320. doi: 10.1371/journal.ppat.1001320
Osei-Poku, J., Mbogo, C. M., Palmer, W. J., and Jiggins, F. M. (2012). Deep sequencing reveals extensive variation in the gut microbiota of wild mosquitoes from Kenya. Mol. Ecol. 21, 5138–5150. doi: 10.1111/j.1365-294X.2012.05759.x
Pang, X., Xiao, X., Liu, Y., Zhang, R., Liu, J., Liu, Q., et al. (2016). Mosquito C-type lectins maintain gut microbiome homeostasis. Nat. Microbiol. 1:16023. doi: 10.1038/nmicrobiol.2016.23
Pearson, K. (1895). Note on regression and inheritance in the case of two parents. Proc. Biol. Sci. 58, 240–242. doi: 10.2307/115794
Pollock, J., Glendinning, L., Wisedchanwet, T., and Watson, M. (2018). The madness of microbiome: attempting to find consensus “best practice” for 16S microbiome studies. Appl. Environ. Microbiol. 84:e02627-17. doi: 10.1128/AEM.02627-17
Pumpuni, C. B., Demaio, J., Kent, M., Davis, J. R., and Beier, J. C. (1996). Bacterial population dynamics in three anopheline species: the impact on Plasmodium sporogonic development. Am. J. Epidemiol. 54, 214–218. doi: 10.4269/ajtmh.1996.54.214
Quackenbush, J. (2002). Microarray data normalization and transformation. Nat. Publish. Group 32(Suppl.), 496–501. doi: 10.1038/ng1032
Quast, C., Pruesse, E., Yilmaz, P., Gerken, J., Schweer, T., Yarza, P., et al. (2013). The SILVA ribosomal RNA gene database project: improved data processing and web-based tools. Nucleic Acids Res. 41, D590–D596. doi: 10.1093/nar/gks1219
Ramirez, J. L., Short, S. M., Bahia, A. C., Saraiva, R. G., Dong, Y., Kang, S., et al. (2014). Chromobacterium Csp_P reduces malaria and dengue infection in vector mosquitoes and has entomopathogenic and in vitro anti-pathogen activities. PLoS Pathog. 10:e1004398. doi: 10.1371/journal.ppat.1004398
Reiter, P., Jakob, W. L., Francy, D. B., and Mullenix, J. B. (1986). Evaluation of the CDC gravid trap for the surveillance of St. Louis encephalitis vectors in Memphis, Tennessee. J Am Mosq Control Assoc 2, 209–211.
Riehle, M. A., Moreira, C. K., Lampe, D., Lauzon, C., and Jacobs-Lorena, M. (2007). Using bacteria to express and display anti-Plasmodium molecules in the mosquito midgut. Int. J. Parasitol. 37, 595–603. doi: 10.1016/j.ijpara.2006.12.002
Rock, D. I., Smith, A. H., Joffe, J., Albertus, A., Wong, N., O’Connor, M., et al. (2017). Context-dependent vertical transmission shapes strong endosymbiont community structure in the pea aphid, Acyrthosiphon pisum. Mol. Ecol. 27, 2039–2056. doi: 10.1111/mec.14449
Rossi, P., Ricci, I., Cappelli, A., Damiani, C., Ulissi, U., Mancini, M. V., et al. (2015). Mutual exclusion of Asaia and Wolbachia in the reproductive organs of mosquito vectors. Parasit Vectors 8, 278. doi: 10.1186/s13071-015-0888-0
Saldana, M. A., Hegde, S., and Hughes, G. L. (2017). Microbial control of arthropod-borne disease. Mem. Inst. Oswaldo Cruz 112, 81–93. doi: 10.1590/0074-02760160373
Scott, T. W., Clark, G. G., Lorenz, L. H., Amerasinghe, P. H., Reiter, P., and Edman, J. D. (1993). Detection of multiple blood feeding in Aedes aegypti (Diptera: Culicidae) during a single gonotrophic cycle using a histologic technique. J. Med. Entomol. 30, 94–99. doi: 10.1093/jmedent/30.1.94
Sedgwick, P. (2014). Spearman’s rank correlation coefficient. BMJ 349, g7327. doi: 10.1136/bmj.g7327
Segata, N., Baldini, F., Pompon, J., Garrett, W. S., Truong, D. T., Dabiré, R. K., et al. (2016). The reproductive tracts of two malaria vectors are populated by a core microbiome and by gender- and swarm-enriched microbial biomarkers. Sci. Rep. 6:24207. doi: 10.1038/srep24207
Shannon, C. E. (2001). A Mathematical Theory of Communication, Vol. 5. New York, NY: ACM SIGMOBILE Mobile Computing and Communications Review, 3–55.
Shannon, P., Markiel, A., Ozier, O., Baliga, N. S., Wang, J. T., Ramage, D., et al. (2003). Cytoscape: a software environment for integrated models of biomolecular interaction networks. Genome Res. 13, 2498–2504. doi: 10.1101/gr.1239303
Sharma, P., Sharma, S., Maurya, R. K., De Das, T., Thomas, T., Lata, S., et al. (2014). Salivary glands harbor more diverse microbial communities than gut in Anopheles culicifacies. Parasit Vectors 7:235. doi: 10.1186/1756-3305-7-235
Short, S. M., Mongodin, E. F., MacLeod, H. J., Talyuli, O. A. C., and Dimopoulos, G. (2017). Amino acid metabolic signaling influences Aedes aegypti midgut microbiome variability. PLoS Negl. Trop. Dis. 11:e5677–e5629. doi: 10.1371/journal.pntd.0005677
Simhadri, R. K., Fast, E. M., Guo, R., Schultz, M. J., Vaisman, N., Ortiz, L., et al. (2017). The gut commensal microbiome of Drosophila melanogaster is modified by the endosymbiont Wolbachia. mSphere 2:e00287-17. doi: 10.1128/mSphere.00287-17
Stathopoulos, S., Neafsey, D. E., Lawniczak, M. K. N., Muskavitch, M. A. T., and Christophides, G. K. (2014). Genetic dissection of Anopheles gambiae gut epithelial responses to Serratia marcescens. PLoS Pathog. 10:e1003897. doi: 10.1371/journal.ppat.1003897.s015
Terenius, O., Lindh, J. M., Eriksson-Gonzales, K., Bussière, L., Laugen, A. T., Bergquist, H., et al. (2012). Midgut bacterial dynamics in Aedes aegypti. FEMS Microbiol. Ecol. 80, 556–565. doi: 10.1111/j.1574-6941.2012.01317.x
Tourlousse, D. M., Yoshiike, S., Ohashi, A., Matsukura, S., Noda, N., and Sekiguchi, Y. (2017). Synthetic spike-in standards for high-throughput 16S rRNA gene amplicon sequencing. Nucleic Acids Res. 45:e23. doi: 10.1093/nar/gkw984
Trpis, M. (1977). Autogeny in diverse populations of Aedes aegypti from East Africa. Tropenmed. Parasitol. 28, 77–82.
Vázquez-martínez, M. G., Rodriguez, M. H., Arredondo-Jiménez, J. I., Méndez-Sánchez, J. D., Bond-Compeán, J. G., and Gold-Morgan, M. (2009). Cyanobacteria Associated with Anopheles albimanus (Diptera: Culicidae) Larval Habitats in Southern Mexico. J. Med. Entomol. 39, 825–832. doi: 10.1603/0022-2585-39.6.825
Wang, S., Ghosh, A. K., Bongio, N., Stebbings, K. A., Lampe, D. J., and Jacobs-Lorena, M. (2012). Fighting malaria with engineered symbiotic bacteria from vector mosquitoes. PNAS 109, 12734–12739. doi: 10.1073/pnas.1204158109
Wang, Y., Gilbreath, T. M. III, Kukutla, P., Yan, G., and Xu, J. (2011). Dynamic gut microbiome across life history of the malaria mosquito Anopheles gambiae in Kenya. PLoS One 6:e24767. doi: 10.1371/journal.pone.0024767
Werren, J. H., and Windsor, D. M. (2000). Wolbachia infection frequencies in insects: evidence of a global equilibrium? Proc. Biol. Sci. 267, 1277–1285. doi: 10.1098/rspb.2000.1139
Xia, Y., and Zwiebel, L. J. (2006). Identification and characterization of an odorant receptor from the West Nile Virus mosquito, Culex quinquefasciatus. Insect biochem. Mol. Biol. 36, 169–176. doi: 10.1016/j.ibmb.2005.12.003
Xiao, X., Yang, L., Pang, X., Zhang, R., Zhu, Y., Wang, P., et al. (2017). A Mesh-Duox pathway regulates homeostasis in the insect gut. Nat. Microbiol. 2:17020. doi: 10.1038/nmicrobiol.2017.20
Zhao, B., Lucas, K. J., Saha, T. T., Ha, J., Ling, L., Kokoza, V. A., et al. (2017). MicroRNA-275 targets sarco/endoplasmic reticulum Ca2+ adenosine triphosphatase (SERCA) to control key functions in the mosquito gut. PLoS Genet. 13:e1006943. doi: 10.1371/journal.pgen.1006943
Zhou, W., Rousset, F., and O’Neill, S. L. (1998). Phylogeny and PCR-based classification of Wolbachia strains using wsp gene sequences. Proc. Biol. Sci. 265, 509–515. doi: 10.1098/rspb.1998.0324
Keywords: interaction networks, microbe-microbe interactions, gnotobiotic, gut symbiont, gut microbiome, Wolbachia
Citation: Hegde S, Khanipov K, Albayrak L, Golovko G, Pimenova M, Saldaña MA, Rojas MM, Hornett EA, Motl GC, Fredregill CL, Dennett JA, Debboun M, Fofanov Y and Hughes GL (2018) Microbiome Interaction Networks and Community Structure From Laboratory-Reared and Field-Collected Aedes aegypti, Aedes albopictus, and Culex quinquefasciatus Mosquito Vectors. Front. Microbiol. 9:2160. doi: 10.3389/fmicb.2018.02160
Received: 05 June 2018; Accepted: 23 August 2018;
Published: 10 September 2018.
Edited by:
David William Waite, University of Auckland, New ZealandReviewed by:
Guillaume Henri Tetreau, UMR5075 Institut de Biologie Structurale (IBS), FranceSibao Wang, Shanghai Institutes for Biological Sciences (CAS), China
Eva Novakova, University of South Bohemia České Budějovice, Czechia
Copyright © 2018 Hegde, Khanipov, Albayrak, Golovko, Pimenova, Saldaña, Rojas, Hornett, Motl, Fredregill, Dennett, Debboun, Fofanov and Hughes. This is an open-access article distributed under the terms of the Creative Commons Attribution License (CC BY). The use, distribution or reproduction in other forums is permitted, provided the original author(s) and the copyright owner(s) are credited and that the original publication in this journal is cited, in accordance with accepted academic practice. No use, distribution or reproduction is permitted which does not comply with these terms.
*Correspondence: Grant L. Hughes, Z3JhbnQuaHVnaGVzQGxzdG1lZC5hYy51aw==; Z2xodWdoZXNAdXRtYi5lZHU=
†These authors have contributed equally to this work
‡Present address: Grant L. Hughes, Departments of Vector Biology and Parasitology, Liverpool School of Tropical Medicine, Liverpool, United Kingdom