- 1Li Ka Shing Faculty of Medicine, School of Public Health, The University of Hong Kong, Hong Kong, China
- 2School of Public Health, Shanghai Jiao Tong University School of Medicine, Shanghai, China
- 3Jockey Club School of Public Health and Primary Care, Chinese University of Hong Kong, Hong Kong, China
- 4Shenzhen Research Institute, Chinese University of Hong Kong, Shenzhen, China
- 5School of Nursing, The Hong Kong Polytechnic University, Hong Kong, China
- 6School of Public Health, Nanjing Medical University, Nanjing, China
- 7Department of Applied Mathematics, Hong Kong Polytechnic University, Hong Kong, China
In this study, we conducted an ecological study to examine their effects in the early phase of the pandemic (from December 2019 to February 2020) in China. We found that the associations between the average concentrations of NO2, SO2, and CO and the COVID-19 transmissibility are not statistically clear.
Introduction
The ongoing Coronavirus 2019–20 pandemic has caused a huge impact on global public health. Many studies showed that a high level of air pollutant concentrations increases the risk of pneumonia infection and deaths. The pollutant concentrations were significant for severe acute respiratory infection (1). Nitrogen dioxide (NO2) exposure increases the occurrence, severity, hospitalization, and 30-day mortality, especially in cold months (2). Long-term exposure to higher levels of NO2 increases the increased hospitalization for community-acquired pneumonia (CAP) in older adults (3). Short-term exposure to higher levels of NO2 and carbon monoxide (CO) increases pneumonia-related hospitalization, emergency department visits, and outpatient visits in adults (4, 5). The concentrations of NO2, sulfur dioxide (SO2), and CO were positively associated with the upper respiratory tract infection and CAP (6). Becker and Soukop reported a non-linear dose-response effect between respiratory syncytial virus (RSV) internalization by airway epithelial cells and the level of NO2 (positive association at a low-level 0.5 ppm of NO2 and a negative association at a high-level 1.5 ppm) (7). Both the release of infectious virus 48-h post-exposure and virus-induced cytokine production were reduced at a high level of NO2.
It is of significance to study the impact of gaseous air pollutants, especially NO2, SO2, and CO on the transmission of coronavirus diseases 2019 (COVID-19) in China. Many studies have been done on the impact of meteorological factors and air pollutants on the spread and COVID-19 and the patient outcome, for example, focusing in China (8–11), as well as other studies focusing in Italy, Spain, and the United States. However, some of these studies adopted a chronic disease approach, using either the linear regression model or time series model. However, COVID-19 is a highly transmissible infectious disease. We cannot merely adopt a statistical model for chronic disease to gain insights on the effects on the transmissibility (12). For instance, the serial correlation in daily new cases should be removed in a time series model (9). Riccò et al. (13) pointed out several other issues. For example, the lockdown of cities not only reduced the transmission of COVID-19 but also reduced the level of air pollution. Thus, the correlation between the level of certain pollutants and the transmission rate over time does not mean causation. The correlation between the transmission and meteorological conditions and air pollution could be non-linear (11).
Methods
We collected daily confirmed COVID-19 cases from 303 cities in China from the Chinese provincial health agencies and China National Health Commission. We calculated the basic reproductive number, R0, which is a unit-free measure of infectivity of a virus and is commonly used in infectious disease epidemiology. For the estimation of R0, we first estimated the exponential (or intrinsic) growth rate, denoted by r, of the epidemic curve over the a 16-day period starting from the confirmation of the first case in each city (14–16). The number of cases at the t-th day, Ct, is modeled as Ct = C0exp(rt), where C0 denoted the number of seed cases at the start of the outbreak. Using the formula R0 = 1/M(–r) that is derived from the Lotka-Euler equation (17), we substitute r into the moment generation function, M(·), of the probability distribution of the COVID-19 serial interval. The distribution of serial interval is approximated by a Gamma distribution with mean at 5.5 days and standard deviation at 3.3 days (17–20). This analytical approach is also used in previous COVID-19 studies as well as in other infectious diseases (20–23).
We obtained air pollutant data in 1,642 observation stations from the China National Environmental Center and obtained meteorological data from the National Meteorological Data Center. We computed a raster for each pollutant by the kriging interpolation based on the averaged values across the period December 10, 2019, to February 29, 2020. Then we interpolated the level of each pollutant for each city. We adopted generalized (i) univariable, and (ii) multivariable linear regression, using R0 as the response and air pollutant as the factor, adjusted by temperature and relative humidity. In addition, we employ the spline regression for sensitivity analysis.
Results
The descriptive statistics of the basic productive numbers, three pollutants, temperature, and relative humidity for 154 cities are given in Table 1. The maximum R0 = 2.5 was detected in Wuhan City. The average concentrations of NO2, SO2, and CO were 31.0, 11.9 μg/m3, and 1.0 mg/m3, respectively. The spatial distributions of NO2, SO2, and CO are shown in Figure 1. Pearson and Spearman's ranked correlation coefficients between the level of pollutants and the basic reproductive number are given in Table 2. We observed no significant correlation.
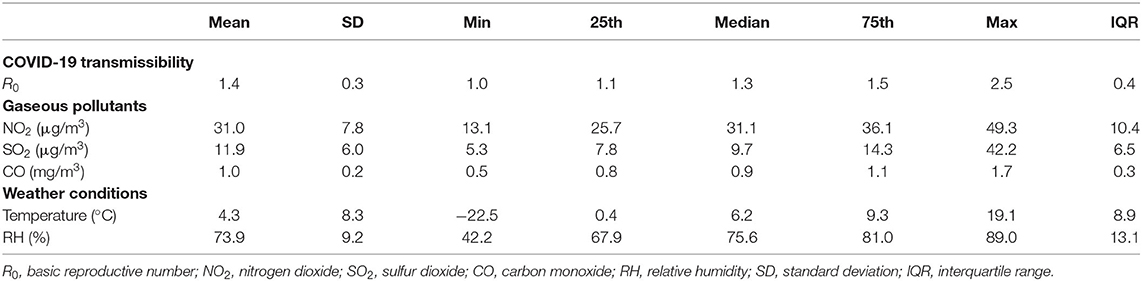
Table 1. Descriptive statistics of the basic reproductive numbers, three gaseous pollutants, temperature, and relative humidity across 154 Chinese cities.
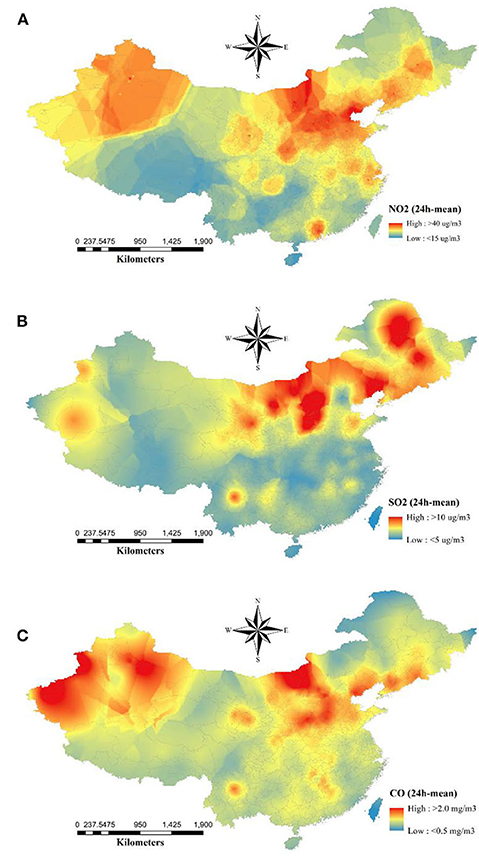
Figure 1. Spatial distribution of average concentrations of ambient (A) NO2, (B) SO2, and (C) CO from December 10, 2019 to February 29, 2020. The raster maps were converted by the kriging interpolation after averaging their concentrations.
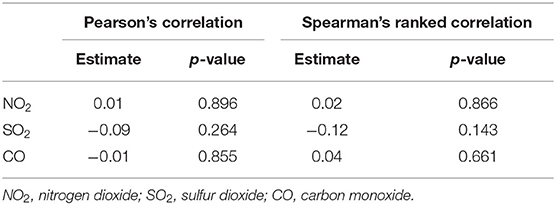
Table 2. Correlation coefficients of COVID-19 R0s with the three gaseous pollutants across 154 Chinese cities.
In Figure 2, using either univariable or multivariable linear regression, we found no clear effects of NO2, SO2, and CO on the initial transmissibility of COVID-19 across Chinese cities. We performed sensitive tests by using spline regression (three degrees of freedom) and restricting the cities outside Hubei province only and found no significant association.
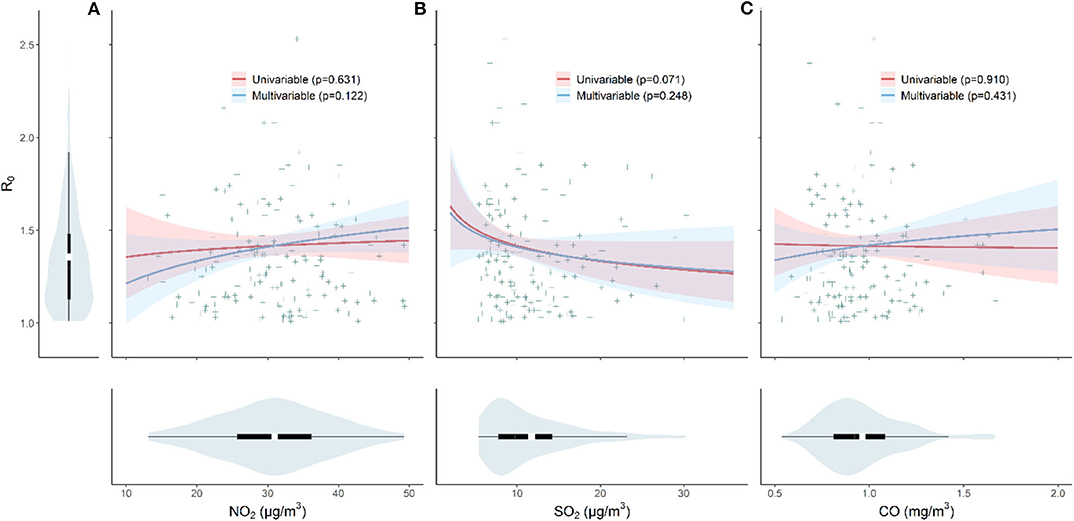
Figure 2. The effects of pollutant (A) NO2, (B) SO2, and (C) CO concentrations and the initial transmissibility of COVID-19. Violin plots shows the distributions of R0, NO2, SO2, and CO, respectively. The red line indicates the result from the univariable model, and the blue line represents result from the multivariable model (adjusted for temperature and relative humidity). The p-values are given in the legend.
Discussion
The merit of this work is that we focus on the transmissibility in the initial phase of the pandemics in each city, which may reflect the intrinsic feature of the local outbreak to some extent. However, several limitations should be noted. Population density is a potential confounder since it may be positively related to gaseous pollutant concentrations and may catalyze the spread of COVID-19 (24, 25). Besides observed associations are not of statistical significance between gaseous air pollutants and the COVID-19 transmission, we may still overestimate the true effects because the unadjusted confounder, population density, would positively bias the associations (26). Population flow from Wuhan City may be another potential confounding factor since it may result in disentangled imported or local cases for other Chinese cities. An emerging study found that correlations between gaseous pollutants and R0 varied in provinces with different population flow (27). However, the effect is likely uniform across cities, and the lockdown of Wuhan mitigated the COVID-19 exportation to other cities effectively. Previously works found the importation was reasonable uniform except for very few cities. This approach has been used previously by other teams and our team (28–30). We avoid using a time series model over a more extended period since it may suffer more issues discussed and demonstrated in a recent study (13). We argue that if there were indeed an “evident” statistical association, our approach should pick it up. At least, we avoid picking up a spurious association, for example, the entangled effects between pollution reduction (if any) and city lockdown. Although the results are not of statistical significance, our method is straightforward on one hand, and we nevertheless consider the possibility of the non-linearity of association on the other hand.
In this large-scale ecological study, we find no significant association between the three gaseous air pollutants (NO2, SO2, and CO) and the initial transmissibility of COVID-19 in Chinese cities. Since we focus on the initial 16 days after the confirmation of the first case, the lockdown of cities should not have reduced the pollutant level in each city, as normally it will take time for pollutants to drop. Our averaged concentration should be a fair indicator of the level of air quality in each city.
Other factors for the insignificant results include that the outbreak period covered the spring festival when factories were closed. And the closure of factories was extended by the government. Many cities adopted different levels of lockdown, and emission of vehicles were reduced. Thus, the concentration of air pollutants may be low in general. Strict and effective mitigation measures stopped the transmission timely.
Data Availability Statement
All datasets presented in this study are included in the article/Supplementary Material.
Author Contributions
JR and SZ conceived the study and carried out the analysis. LH collected the data. JR, SZ, and DH drafted the letter and discussed the results. All authors critically read and revised the letter and gave final approval for publication.
Funding
DH was supported by the General Research Fund (Grant Number 15205119) of the Research Grants Council (RGC) of Hong Kong, China, and an Alibaba (China)-Hong Kong Polytechnic University Collaborative Research project. The funding agencies had no role in the design and conduct of the study; collection, management, analysis, and interpretation of the data; preparation, review, or approval of the manuscript; or decision to submit the manuscript for publication.
Conflict of Interest
The authors declare that the research was conducted in the absence of any commercial or financial relationships that could be construed as a potential conflict of interest.
Supplementary Material
The Supplementary Material for this article can be found online at: https://www.frontiersin.org/articles/10.3389/fmed.2020.575839/full#supplementary-material
References
1. Silva DR, Viana VP, Müller AM, Livi FP, Dalcin PDTR. Respiratory viral infections and effects of meteorological parameters and air pollution in adults with respiratory symptoms admitted to the emergency room. Influenza Other Respir Viruses. (2014) 8:42–52. doi: 10.1111/irv.12158
2. Pirozzi CS, Jones BE, Van Derslice JA, Zhang Y, Paine R, Dean NC. Short-term air pollution and incident pneumonia a case-crossover study. Ann Am Thorac Soc. (2018) 15:449–59. doi: 10.1513/AnnalsATS.201706-495OC
3. Neupane B, Jerrett M, Burnett RT, Marrie T, Arain A, Loeb M. Long-term exposure to ambient air pollution and risk of hospitalization with community-acquired pneumonia in older adults. Am J Respir Crit Care Med. (2010) 181:47–53. doi: 10.1164/rccm.200901-0160OC
4. Fusco D, Forastiere F, Michelozzi P, Spadea T, Ostro B, Arcà M, et al. Air pollution and hospital admissions for respiratory conditions in Rome, Italy. Eur Respir J. (2001) 17:1143–50. doi: 10.1183/09031936.01.00005501
5. Cheng MF, Tsai SS, Wu TN, Chen PS, Yang CY. Air pollution and hospital admissions for pneumonia in a tropical city: Kaohsiung, Taiwan. J Toxicol Environ Health A. (2007) 70:2021–6. doi: 10.1080/15287390701601020
6. Li R, Jiang N, liu Q, Huang J, Guo X, Liu F, et al. Impact of air pollutants on outpatient visits for acute respiratory outcomes. Int J Environ Res Public Health. (2017) 14:47. doi: 10.3390/ijerph14010047
7. Becker S, Soukup JM. Effect of nitrogen dioxide on respiratory viral infection in airway epithelial cells. Environ Res. (1999) 81:159–66. doi: 10.1006/enrs.1999.3963
8. Yao Y, Pan J, Wang W, Liu Z, Kan H, Qiu Y, et al. Association ofparticulate matter pollution and case fatality rate of COVID-19 in 49 Chinese cities. Sci Total Environ. (2020) 741:140396. doi: 10.1016/j.scitotenv.2020.140396
9. Zhu Y, Xie J, Huang F, Cao L. Association between short-term exposure to air pollution and COVID-19 infection: evidence from China. Sci Total Environ. (2020) 727:138704. doi: 10.1016/j.scitotenv.2020.138704
10. Xu H, Yan C, Fu Q, Xiao K, Yu Y, Han D, et al. Possible environmental effects on the spread of COVID-19 in China. Sci Total Environ. (2020) 731:139211. doi: 10.1016/j.scitotenv.2020.139211
11. Zhang Z, Xue T, Jin X. Effects of meteorological conditions and air pollution on COVID-19 transmission: evidence from 219 Chinese cities. Sci Total Environ. (2020) 741:140244. doi: 10.1016/j.scitotenv.2020.140244
12. Zhao S. To avoid the noncausal association between environmental factor and COVID-19 when using aggregated data: simulation-based counterexamples for demonstration. Sci Total Environ. (2020) 748:141590. doi: 10.1016/j.scitotenv.2020.141590
13. Riccò M, Ranzieri S, Balzarini F, Bragazzi NL, Corradi M. SARS-CoV-2 infection and air pollutants: correlation or causation? Sci Total Environ. (2020) 734:139489. doi: 10.1016/j.scitotenv.2020.139489
14. de Silva UC, Warachit J, Waicharoen S, Chittaganpitch M. A preliminary analysis of the epidemiology of influenza A (H1N1) v virus infection in Thailand from early outbreak data, June-July 2009. Eurosurveillance. (2009) 14:19292. doi: 10.2807/ese.14.31.19292-en
15. Zhao S, Lin Q, Ran J, Musa SS, Yang G, Wang W, et al. Preliminary estimation of the basic reproduction number of novel coronavirus (2019-nCoV) in China, from 2019 to 2020: a data-driven analysis in the early phase of the outbreak. Int J Infect Dis. (2020) 92:214–7. doi: 10.1016/j.ijid.2020.01.050
16. Chowell G, Viboud C, Simonsen L, Moghadas SM. Characterizing the reproduction number of epidemics with early subexponential growth dynamics. J R Soc Interface. (2016) 13:20160659. doi: 10.1098/rsif.2016.0659
17. Wallinga J, Lipsitch M. How generation intervals shape the relationship between growth rates and reproductive numbers. Proc R Soc B Biol Sci. (2007) 274:599–604. doi: 10.1098/rspb.2006.3754
18. He X, Lau EHY, Wu P, Deng X, Wang J, Hao X, et al. Temporal dynamics in viral shedding and transmissibility of COVID-19. Nat Med. (2020) 26:672–5. doi: 10.1038/s41591-020-0869-5
19. Ferretti L, Wymant C, Kendall M, Zhao L, Nurtay A, Abeler-Dörner L, et al. Quantifying SARS-CoV-2 transmission suggests epidemic control with digital contact tracing. Science. (2020) 368:eabb6936. doi: 10.1101/2020.03.08.20032946
20. Li Q, Guan X, Wu P, Wang X, Zhou L, Tong Y, et al. Early transmission dynamics in Wuhan, China, of novel coronavirus-infected pneumonia. N Engl J Med. (2020) 382:1199–207. doi: 10.1056/NEJMoa2001316
21. Zhao S, Musa SS, Lin Q, Ran J, Yang G, Wang W, et al. Estimating the unreported number of novel coronavirus (2019-nCoV) cases in China in the first half of January 2020: a data-driven modelling analysis of the early outbreak. J Clin Med. (2020) 9:388. doi: 10.3390/jcm9020388
22. Cowling BJ, Fang VJ, Riley S, Malik Peiris JS, Leung GM. Estimation of the serial interval of influenza. Epidemiology. (2009) 20:344–7. doi: 10.1097/EDE.0b013e31819d1092
23. Fraser C, Donnelly CA, Cauchemez S, Hanage WP, Van Kerkhove MD, Hollingsworth TD, et al. Pandemic potential of a strain of influenza A (H1N1): early findings. Science. (2009) 324:1557–61. doi: 10.1126/science.1176062
24. Rocklöv J, Sjödin H. High population densities catalyse the spread of COVID-19. J Travel Med. (2020) 27:1–2. doi: 10.1093/jtm/taaa038
25. Lamsal LN, Martin RV, Parrish DD, Krotkov NA. Scaling relationship for NO2 pollution and urban population size: a satellite perspective. Environ Sci Technol. (2013) 47:7855–61. doi: 10.1021/es400744g
26. Mehio-Sibai A, Feinleib M, Sibai TA, Armenian HK. A positive or a negative confounding variable? A simple teaching aid for clinicians and students. Ann Epidemiol. (2005) 15:421–3. doi: 10.1016/j.annepidem.2004.10.004
27. Lin S, Wei D, Sun Y, Chen K, Yang L, Liu B, et al. Region-specific air pollutants and meteorological parameters influence COVID-19: a study from mainland China. Ecotoxicol Environ Saf . (2020) 204:111035. doi: 10.1016/j.ecoenv.2020.111035
28. Ran J, Zhao S, Han L, Liao G, Wang K, Wang MH, et al. A re-analysis in exploring the association between temperature and COVID-19 transmissibility: an ecological study with 154 Chinese cities. Eur Respir J. (2020) 56:2001253. doi: 10.1183/13993003.01253-2020
29. Ran J, Zhao S, Han L, Chen D, Yang Z, Yang L, et al. The ambient ozone and COVID-19 transmissibility in China: a data-driven ecological study of 154 cities. J Infect. (2020) 81:11–3. doi: 10.1016/j.jinf.2020.07.011
Keywords: gaseous air pollutant, COVID-19, transmissibility, ecological study, China
Citation: Ran J, Zhao S, Han L, Peng Z, Wang MH, Qiu Y and He D (2020) Initial COVID-19 Transmissibility and Three Gaseous Air Pollutants (NO2, SO2, and CO): A Nationwide Ecological Study in China. Front. Med. 7:575839. doi: 10.3389/fmed.2020.575839
Received: 24 June 2020; Accepted: 13 August 2020;
Published: 24 September 2020.
Edited by:
Matteo Convertino, Hokkaido University, JapanCopyright © 2020 Ran, Zhao, Han, Peng, Wang, Qiu and He. This is an open-access article distributed under the terms of the Creative Commons Attribution License (CC BY). The use, distribution or reproduction in other forums is permitted, provided the original author(s) and the copyright owner(s) are credited and that the original publication in this journal is cited, in accordance with accepted academic practice. No use, distribution or reproduction is permitted which does not comply with these terms.
*Correspondence: Daihai He, ZGFpaGFpLmhlQHBvbHl1LmVkdS5oaw==
†These authors have contributed equally to this work and share first authorship