- 1Department of Laboratory Medicine, Tongji Hospital, Tongji Medical College, Huazhong University of Science and Technology, Wuhan, China
- 2Department of Clinical Immunology, Tongji Hospital, Tongji Medical College, Huazhong University of Sciences and Technology, Wuhan, China
Background: The predictive value of prealbumin for the prognosis of coronavirus disease 2019 (COVID-19) has not been extensively investigated.
Methods: A total of 1,115 patients with laboratory-confirmed COVID-19 were enrolled at Tongji hospital from February to April 2020 and classified into fatal (n = 129) and recovered (n = 986) groups according to the patient's outcome. Prealbumin and other routine laboratory indicators were measured simultaneously.
Results: The level of prealbumin on admission was significantly lower in fatal patients than in recovered patients. For predicting the prognosis of COVID-19, the performance of prealbumin was better than most routine laboratory indicators, such as albumin, lymphocyte count, neutrophil count, hypersensitive C-reactive protein, d-dimer, lactate dehydrogenase, creatinine, and hypersensitive cardiac troponin I. When a threshold of 126 mg/L was used to discriminate between fatal and recovered patients, the sensitivity and specificity of prealbumin were, respectively, 78.29 and 90.06%. Furthermore, a model based on the combination of nine indexes showed an improved performance in predicting the death of patients with COVID-19. Using a cut-off value of 0.19, the prediction model was able to distinguish between fatal and recovered individuals with a sensitivity of 86.82% and a specificity of 90.37%.
Conclusions: A lower level of prealbumin on admission may indicate a worse outcome of COVID-19. Immune and nutritional status may be vital factors for predicting disease progression in the early stage of COVID-19.
Introduction
Coronavirus disease 2019 (COVID-19) as a rampant disease caused by the emerging infection of severe acute respiratory syndrome coronavirus 2 (SARS-CoV-2), the outbreak of which has initiated an extreme health concern (1, 2). Many patients might progress to acute respiratory disease or other complications in a short period of time (3, 4). Since no effective vaccine or anti-viral treatment is currently available, this epidemic is difficult to manage and control. As of 7 May 2020, the number of patients infected with SARS-CoV-2 has exceeded 3.5 million globally, and there has been more than 250,000 reported cases of COVID-19-related deaths worldwide (5). Therefore, it is urgent and desirable to establish a model which can be used to predict the progression of disease and help clinicians to better choose a therapeutic strategy.
Up to now, many studies have reported that the risk factors for death in patients with COVID-19 are attributed to advanced age and co-morbidities including hypertension, myocardial injury, liver damage, and kidney failure (6–10). Some indicators such as creatinine (CR), lactate dehydrogenase (LDH), aspartate aminotransferase (AST), and hypersensitive cardiac troponin I (hs-cTnI) have been found to be helpful to assess the severity of the disease and predict the prognosis of COVID-19 (9, 11, 12). In addition, some studies discovered and elaborated the potential value of coagulation indicators represented by prothrombin time (PT) and d-dimer (DD) in predicting the prognosis and outcome of patients with COVID-19 (13–15). Moreover, some recent studies focused on the body's immune status, including inflammatory responses and the number and phenotype of lymphocytes, and found that some inflammatory indicators or cytokines including hypersensitive C-reactive protein (hsCRP), procalcitonin (PCT), interleukin-6, interleukin-2 receptor, interferon-gamma, and the number of lymphocytes also contributed to the outcome of the disease (16–19).
Additionally, some indicators, such as albumin (ALB) and prealbumin (PAB), can partially reflect the nutritional and immune status of the host (20–23). However, the potential value of them for the prognosis of COVID-19 has not been fully explored. Theoretically, in view of the decrease in the number of lymphocytes and their subsets in the early stage of the disease (24, 25) and the poor immune function potentially caused by various complications (26, 27), ALB and PAB are potential and feasible predictors for the prognosis of COVID-19. In this study, we did a comprehensive evaluation of various laboratory indicators in fatal and recovered patients with confirmed COVID-19 on admission. We also compared the predictive value of PAB and other routine laboratory makers for the prognosis of COVID-19. It is hoped that the information obtained in this study will offer a better understanding on the disease progression that occurs after SARS-CoV-2 infection, and establish a basis to optimize the current treatment.
Methods
Study Design and Participants
The current study was conducted at Tongji hospital (the largest hospital in the central region of China) in Wuhan, China. Consecutive hospitalized patients with confirmed COVID-19 were enrolled between February and April 2020. COVID-19 was diagnosed if patients met the following criteria: (1) having typical clinical symptoms, (2) having typical imaging findings, and (3) positive for SARS-CoV-2 real-time reverse transcription-polymerase chain reaction. The patients who died during hospitalization were defined as the fatal group, and those who recovered and were finally discharged after hospitalization were defined as the recovered group. All recovered patients with COVID-19 met the following criteria: having completely resolved symptoms and signs, having significant improvement in pulmonary and extrapulmonary organ dysfunction, no longer need supportive care, and with confirmed viral clearance by repeated tests for SARS-CoV-2 before hospital discharge. This study was reviewed and approved by the ethical committee of Tongji Hospital, Tongji Medical College, Huazhong University of Science and Technology, Wuhan, China (TJ-C20200128).
Real Time Reverse Transcription-Polymerase Chain Reaction
The clinical samples, including throat and nasal swab obtained from patients at admission or during the hospital stay, were maintained in a viral-transport medium. SARS-CoV-2 was confirmed by using TaqMan One-Step reverse transcription-polymerase chain reaction (RT-PCR) Kits from Shanghai Huirui Biotechnology Co., Ltd and Shanghai BioGerm Medical Biotechnology Co., Ltd. Briefly, RNA was extracted from clinical samples. 5 μL of RNA was used for real-time RT-PCR, which targeted the ORF1ab and N gene. Real-time RT-PCR was performed using the following conditions: 50°C for 15 min and 95°C for 5 min, 45 cycles of amplification at 95°C for 10 s and 55°C for 45 s. The positive SARS-CoV-2 real time RT-PCR result was defined if both ORF1ab and N cycle thresholds were <35.
Laboratory Tests
The measurements of white blood cell count (WBC#), neutrophil count (NEU#), lymphocyte count (LYM#), and platelet count (PLT#) were performed using XN-9000 Sysmex (Sysmex Co., Kobe, Japan). The measurements of total protein (TP), PAB, ALB, globulin (GLB), CR, AST, LDH, hsCRP, PCT, and hs-cTnI were performed using ROCHE COBAS 8000 (Mannheim, Germany). PT and DD were detected using STA-R coagulation analyzers (Diagnostic Stago, France) according to the manufacturer's instructions.
Statistical Analysis
Continuous variables were expressed as mean ± standard deviation (SD) and categorical variables were reported as numbers and percentages (%). The comparison between continuous variables was performed using the Wilcoxon test or Mann-Whitney U test. The chi-square test was used for comparison of categorical data. The area under the curves (AUCs) were compared using the z statistic with the procedure of (28). A two-sided P-value below 0.05 was considered to be statistically significant. A prediction model for predicting the outcome of death was established by using a multivariate logistic regression method. All variables with statistical significance were taken as candidates for multivariable logistic regression analyses, and the regression equation (prediction model) was obtained. The regression coefficients of the prediction model were regarded as the weights for the respective variables and a score for each patient was calculated. Receiver operating characteristic (ROC) analysis was performed on these scores to assess the ability for distinguishing between fatal and recovered COVID-19 patients. AUC, sensitivity, specificity, positive predictive value (PPV), negative predictive value (NPV), positive likelihood ratio (PLR), and negative likelihood ratio (NLR), together with their 95% confidence intervals (CI), were calculated. Data were analyzed by using SPSS version 25.0 (SPSS, Chicago, IL, USA), GraphPad Prism version 6 (GraphPad Software, San Diego, CA, USA), and MedCalc version 11.6 (Medcalc, Mariakerke, Belgium).
Results
Demographic and Clinical Characteristics of Study Participants
Our study enrolled 129 patients who died during hospitalization and 986 recovered patients (Table 1). There were 552 women (49.51%) and 563 men (50.49%) in this cohort, with ages ranging from 16 to 95 years old. The mean age of fatal patients (69.98 ± 12.05 years) was significantly older than recovered patients (58.64 ± 15.17 years) (P < 0.001). Male sex was more predominant in fatal patients (67.44%) than in recovered patients (48.28%) (P < 0.001). Cough and fever were the most prevalent symptoms at disease onset in both fatal (51.16 and 62.02%) and recovered patients (58.42 and 65.72%). Other prevalent symptoms at the onset of illness in fatal patients included shortness of breath and chest tightness; less common symptoms included diarrhea, headache, nausea, vomiting, muscle ache, and pharyngalgia. Shortness of breath was significantly higher in fatal patients (34.88%) than in recovered patients (12.78%) (P < 0.001). Underlying diseases including diabetes mellitus, hypertension, chronic obstructive pulmonary disease, cardiovascular disease, and hematological malignancy were more frequent in fatal patients (19.38, 43.41, 3.88, 17.83, and 1.15%) than in recovered patients (7.3, 25.56, 1.42, 9.63, and 0.2%). The mean time from admission to death was 16.72 ± 11.9 days in fatal patients. The mean time from admission to discharge was 21.66 ± 12.01 days in recovered patients (Table 1).
Using PAB for Predicting the Prognosis of COVID-19
We observed substantial differences in the levels of proteins including PAB, ALB, GLB, and TP between patients who died of COVID-19 and those who recovered from the disease. It was found that the concentrations of PAB, ALB, and TP on admission were markedly lower in fatal patients than in recovered patients (P < 0.001). On the contrary, the level of GLB on admission was found to be significantly higher in the fatal group than in the recovered group (P < 0.001) (Figure 1A). If using these indexes for distinguishing these two conditions, the best AUC was for PAB [0.915, (95% CI, 0.894–0.937)] (Table 2, Figure 1B). Notably, the level of PAB ≤ 126 mg/L produced a sensitivity of 78.29 % and a specificity of 90.06% (Table 2). In addition, ROC analysis showed that the AUC of ALB was 0.825 (95% CI, 0.792–0.859), with a sensitivity of 45.74% and a specificity of 90.37% when a cutoff value of 29.7 g/L was used to differentiate between fatal and recovered individuals (Table 2, Figure 1B).
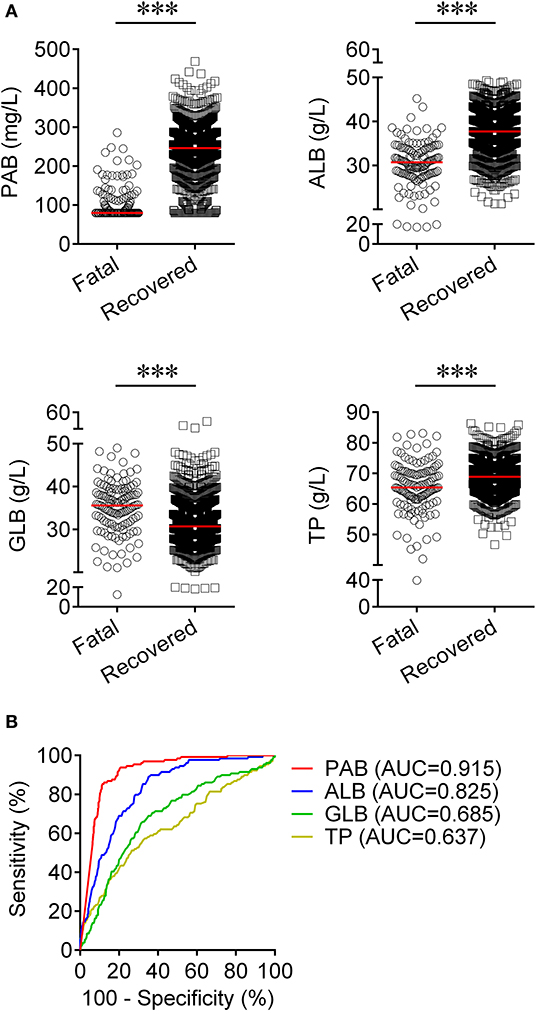
Figure 1. Using PAB on admission for discriminating fatal patients from recovered patients. (A) Scatter plots showing the concentrations of PAB, ALB, GLB, and TP in fatal (n = 129) and recovered (n = 986) patients. Horizontal lines indicate the median. ***P < 0.001 (Mann-Whitney U test). (B) ROC analysis showing the performance of PAB, ALB, GLB, and TP in distinguishing fatal patients from recovered patients. PAB, prealbumin; ALB, albumin; GLB, globulin; TP, total protein; ROC, receiver operating characteristic curve; AUC, area under the curve.
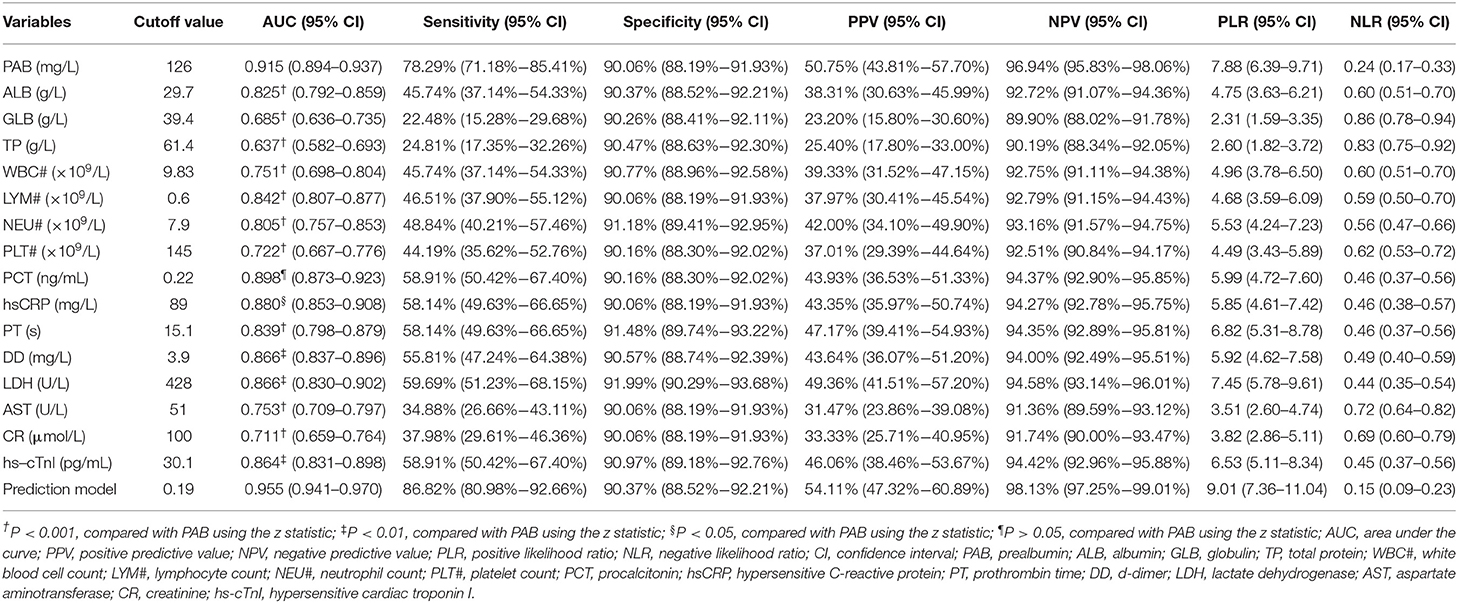
Table 2. The performance of various methods for distinguishing between fatal and recovered patients.
Change of the Level of PAB in the Same Patients
We compared the level of PAB in fatal patients between admission and death. It was found that the level of PAB was significantly decreased at the time of death compared to admission (P < 0.001) (Figure 2A). Furthermore, the concentration of PAB was further compared between admission and discharge in recovered individuals. Conversely, recovered patients showed a significantly higher level of PAB on discharge compared with on admission (P < 0.001) (Figure 2B).
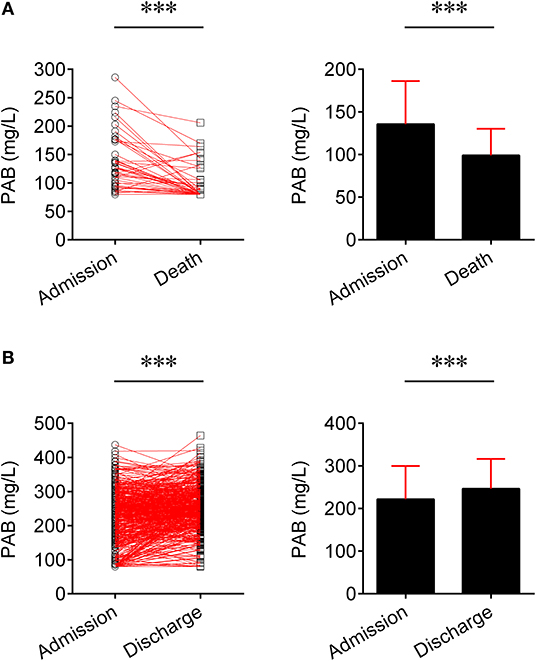
Figure 2. Change of the level of PAB in the same patients. (A) Line graphs showing the level of PAB for each fatal patient on admission and death (n = 45). One line represents one patient. ***P < 0.001 (Wilcoxon test). Bar graphs showing the level of PAB on admission and death in fatal patients (n = 45). Data are shown as means ± SD. ***P < 0.001 (Wilcoxon test). (B) Line graphs showing the level of PAB for each recovered patient on admission and discharge (n = 501). One line represents one patient. ***P < 0.001 (Wilcoxon test). Bar graphs showing the level of PAB on admission and discharge in recovered patients (n = 501). Data are shown as means ± SD. ***P < 0.001 (Wilcoxon test). PAB, prealbumin.
The Comparison of Predictive Value Between PAB and Other Routine Laboratory Indicators for the Prognosis of COVID-19
Routine laboratory markers including WBC#, LYM#, NEU#, PLT#, PCT, hsCRP, PT, DD, LDH, AST, CR, and hs-cTnI were also measured in both fatal and recovered patients on admission. LYM# and PLT# were significantly lower in the fatal group than in the recovered group (P < 0.001) (Figure 3A). Conversely, it was found that WBC#, NEU#, PCT, hsCRP, PT, DD, LDH, AST, CR, and hs-cTnI in the fatal group was significantly higher than in the recovered group (P < 0.001) (Figure 3A). ROC analysis showed that the AUCs of LYM#, NEU#, PCT, hsCRP, PT, DD, LDH, and hs-cTnI were over 0.8 for distinguishing between fatal patients and recovered subjects (Figure 3B). Using a cut-off value of 0.22 ng/ml, the sensitivity and specificity of PCT for discriminating fatal cases from recovered individuals were 58.91 and 90.16%, respectively (Table 2). With a threshold of 89 mg/L, hsCRP was able to distinguish fatal patients from recovered patients with a sensitivity of 58.14% and a specificity of 90.06% (Table 2). Moreover, with a threshold of 15.1 s, PT had an AUC of 0.839 (95% CI, 0.798–0.879) with a sensitivity of 58.14% and a specificity of 91.48% (Table 2). The predictive utility of PAB was better than WBC#, LYM#, NEU#, PLT#, hsCRP, PT, DD, LDH, AST, CR, and hs-cTnI, and was comparable to PCT for the prognosis of patients with COVID-19 (Table 2).
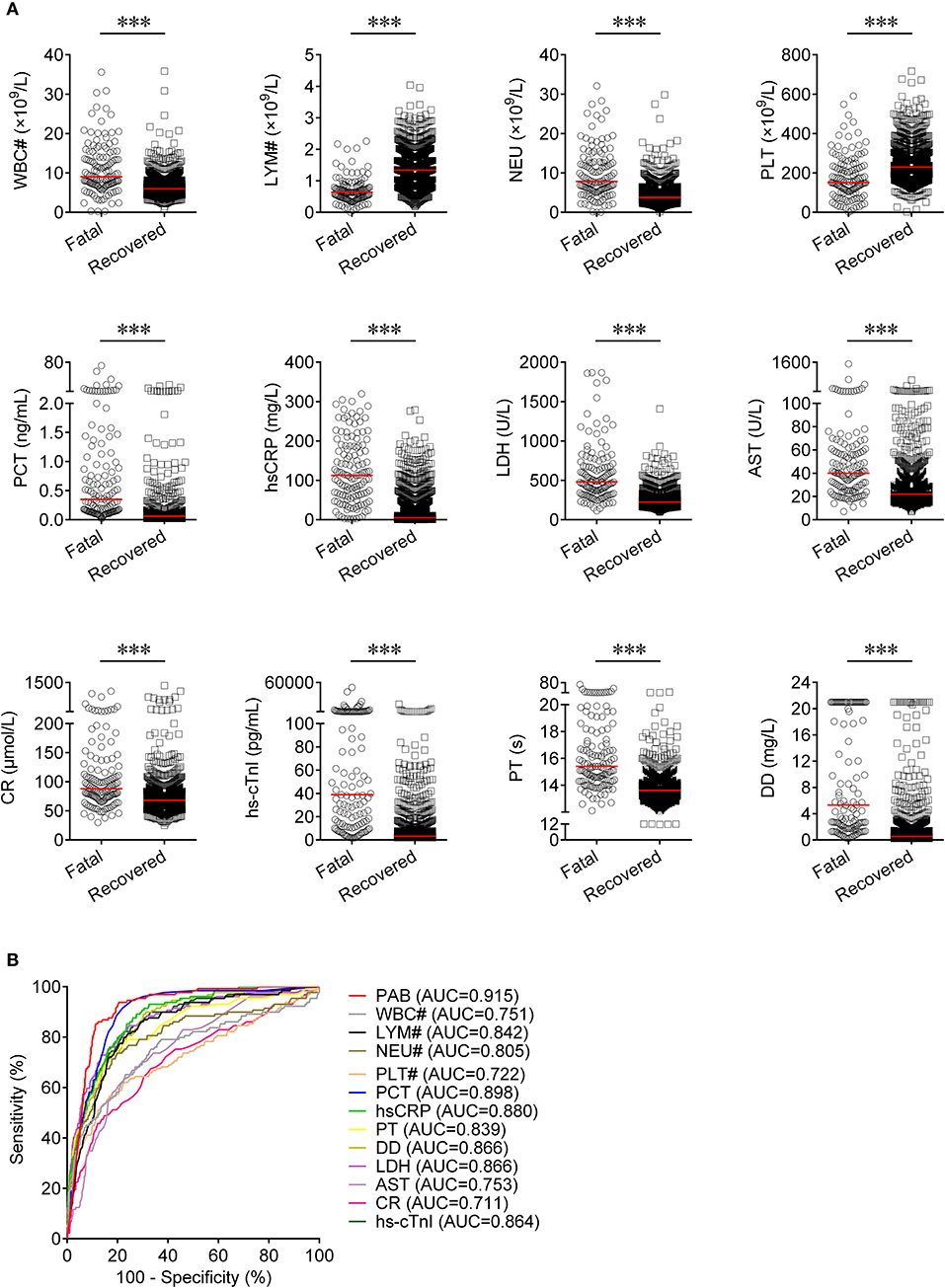
Figure 3. The comparison of predictive values between PAB and other route laboratory markers for the prognosis of COVID-19. (A) Scatter plots showing the levels of WBC#, LYM#, NEU#, PLT#, PCT, hsCRP, PT, DD, LDH, AST, CR, and hs-cTnI in fatal (n = 129) and recovered (n = 986) patients. Horizontal lines indicate the median. ***P < 0.001 (Mann-Whitney U test). (B) ROC analysis showing the performance of PAB, WBC#, LYM#, NEU#, PLT#, PCT, hsCRP, PT, DD, LDH, AST, CR, and hs-cTnI in distinguishing fatal patients from recovered patients. COVID-19, coronavirus disease 2019; PAB, prealbumin; WBC#, white blood cell count; LYM#, lymphocyte count; NEU#, neutrophil count; PLT#, platelet count; PCT, procalcitonin; hsCRP, hypersensitive C-reactive protein; PT, prothrombin time; DD, d-dimer; LDH, lactate dehydrogenase; AST, aspartate aminotransferase; CR, creatinine; hs-cTnI, hypersensitive cardiac troponin I; ROC, receiver operating characteristic curve; AUC, area under the curve.
Establishing a Model for Predicting the Death of Patients With COVID-19
To establish a prediction model based on the combination of PAB and other routine laboratory markers on admission for distinguishing fatal patients from recovered individuals, all variables with statistical significance were used for multivariable logistic regression analysis. A prediction model was built as the following: P = 1/[1 + e−(−0.016*PAB−0.908*LYM#+0.067*NEU#+0.06*PCT+0.005*hsCRP+0.154*PT +0.003*LDH+0.002*CR+0.001*hs−cTnI−3.036)] P, predictive value; e, natural logarithm (Supplementary Table 1). ROC analysis showed that the AUC of the prediction model was 0.955 (95% CI, 0.941-0.970) (Figure 4). When the cutoff value was set at 0.19, the following diagnostic parameters of the model were obtained: sensitivity, 86.82% (95% CI, 80.98–92.66%); specificity, 90.37% (95% CI, 88.52–92.21%) (Table 2). These data suggested that our established model, based on the combination of a nine-indicator biosignature, had good performance for predicting the death of patients with COVID-19.
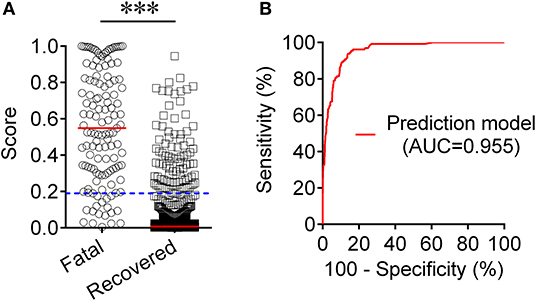
Figure 4. Establishment of prediction model for prognosis of COVID-19 based on the combination of PAB and other routine laboratory indexes. (A) Scatter plots showing the score of prediction model in fatal patients (n = 129) and survived cases (n = 986). Horizontal lines indicate the median. ***P < 0.001 (Mann-Whitney U test). Blue dotted lines indicate the cutoff value in distinguishing these two groups. (B) ROC analysis showing the performance of the prediction model in distinguishing fatal patients from recovered cases. COVID-19, coronavirus disease 2019; ROC, receiver operating characteristic curve; AUC, area under the curve.
Discussion
The rapidly increasing number of laboratory-confirmed COVID-19 cases worldwide has put a heavy burden on the medical resources in countries with large outbreaks (29–31). The World Health Organization has declared COVID-19 to be a public health emergency of international concern. The determination of the outcome of the disease is of crucial importance in regulating limited medical resources and providing better care for patients (32, 33). Meanwhile, progression at the early stage is very important to the outcome or the prognosis of the disease. Therefore, after patient admission, identifying predictors that can predict the likelihood of disease progression would help physicians to decide which group of patients can be managed safely at district hospitals and who needs early transfer to tertiary centers.
Although previous studies have found that a series of parameters on admission are correlated with mortality risk (34), there is limited information in the existing literature regarding the relationship between nutrition indexes and disease progression in SARS-CoV-2-infected patients. Several studies have reported the value of nutritional indicators represented by PAB on determining the severity of viral infections and predicting the prognosis of patients (35, 36). In addition, there is some literature describing the roles of inflammatory indicators (hsCRP and PCT), coagulation indicators (DD), and the number of lymphocytes in monitoring the disease progression during viral infection and prediction of disease outcome (36–38). Our study comprehensively described the differences in PAB and other routine laboratory parameters between the patients who died of COVID-19 and those who recovered from the disease. It was found that a low level of PAB on admission may indicate a poor prognosis and that the predictive value of PAB is superior to most routine laboratory indicators that reflect functional impairment or disorder of organs for prognosis of COVID-19. To our knowledge, this is the first study to report the relationship between PAB level and the outcome of COVID-19.
Host factors trigger an immune response against the pathogens during viral infection (39–41). Immune insufficiency may contribute to viral replication and cause tissue damage, resulting in a bad outcome (42, 43). The systematic overwhelming inflammation and multi-organ dysfunction are more common in deceased COVID-19 patients than in recovered patients (44). This may be caused by the poor basic immune status of patients. In accordance with recent reports, advanced age and comorbidities such as diabetes and hypertension are believed to be risk factors of death from COVID-19 (45). This suggests that immune and nutritional status may be critical in predicting disease progression at the early stage of COVID-19. In other words, poor immunity may play a role in COVID-19-related death. Thus, early vigilant monitoring along with high quality supportive care are needed for patients at high risk of death. Although the number of lymphocytes can partially reflect the host's immune function, our data indicates that the nutritional indicators represented by PAB may show this effect better. A single use of PAB could achieve a modest prediction performance for the prognosis of COVID-19. When combined with other conventional laboratory indicators, PAB could produce a better performance.
In addition, opposite trends were found in PAB level between fatal and recovered groups. The level of PAB gradually decreased with growing hospital stay in fatal patients but had an increased trend in recovered patients (Supplementary Figure 1). These data suggest that the dynamic monitoring of PAB provides a potential value for mastering the process of the disease. These findings would also alert clinicians to pay special attention not only to inflammatory indexes but also to nutritional and immune status.
Some limitations in this study should be addressed. First, since the number of participants recruited in this one-center study is limited, a further design with a large multicenter cohort will provide more conclusive and valuable data. Second, some indicators, such as PAB and DD, were over the detection limit, which would lead to bias. Finally, medical history such as malnutrition and the use of steroid drugs were not included in the regression analysis and it would affect our results.
Collectively, our study provides the evidence that PAB level on admission could be used to predict in-hospital mortality in patients with COVID-19. The information provided in our study shows potential value in enriching knowledge about this critical disease, helping clinicians to identify patients with poor prognosis at an early stage before they die from COVID-19, guiding appropriate and effective management for future patients, and consequently helping to improve patients' outcomes and decrease the fatality rate.
Data Availability Statement
All datasets presented in this study are included in the article/Supplementary Material.
Ethics Statement
This study was reviewed and approved by the ethical committee of Tongji Hospital, Tongji Medical College, Huazhong University of Science and Technology, Wuhan, China (TJ-C20200128). The patients/participants provided their written informed consent to participate in this study.
Author Contributions
YL, FW, and ZS conceived the research, designed the study, interpreted data, and wrote the manuscript. YL, YX, LM, and XY contributed to the acquisition of clinical data. YL, QL, GT, and HS recruited the participants, performed experiments, and analyzed data. All authors approved the final draft of the manuscript.
Funding
This work was supported by grants from the National Mega Project on Major Infectious Disease Prevention of China (2017ZX10103005-007) and the National Natural Science Foundation (81401639).
Conflict of Interest
The authors declare that the research was conducted in the absence of any commercial or financial relationships that could be construed as a potential conflict of interest.
Acknowledgments
We thank all the patients and their families and the clinical staff who treated the patients.
Supplementary Material
The Supplementary Material for this article can be found online at: https://www.frontiersin.org/articles/10.3389/fmed.2020.00374/full#supplementary-material
Supplementary Figure 1. Dynamic monitoring of PAB in the representative of fatal and recovered patients. (A) Line diagrams showing the level of PAB in four representatives of the fatal group. (B) Line diagrams showing the level of PAB in four representatives of the recovered group. *days from admission onset to detection. PAB, prealbumin.
Supplementary Table 1. Logistic regression analysis.
References
1. Holshue ML, DeBolt C, Lindquist S, Lofy KH, Wiesman J, Bruce H, et al. First case of 2019 novel coronavirus in the United States. N Engl J Med. (2020) 382:929–36. doi: 10.1056/NEJMoa2001191
2. Zhu N, Zhang D, Wang W, Li X, Yang B, Song J, et al. A novel coronavirus from patients with pneumonia in China, 2019. N Engl J Med. (2020) 382:727–33. doi: 10.1056/NEJMoa2001017
3. Chen T, Wu D, Chen H, Yan W, Yang D, Chen G, et al. Clinical characteristics of 113 deceased patients with coronavirus disease 2019: retrospective study. BMJ. (2020) 368:m1091. doi: 10.1136/bmj.m1091
4. Richardson S, Hirsch JS, Narasimhan M, Crawford JM, McGinn T, Davidson KW, et al. Presenting characteristics, comorbidities, and outcomes among 5700 patients hospitalized with COVID-19 in the New York City Area. JAMA. (2020) 323:2052–9. doi: 10.1001/jama.2020.6775
5. World Health Organization. Novel Coronavirus (2019-ncov) Situation Report 108. Available online at: https://www.who.int/emergencies/diseases/novel-coronavirus-2019/situation-reports/ (accessed May 8, 2020).
6. Chen R, Liang W, Jiang M, Guan W, Zhan C, Wang T, et al. Risk factors of fatal outcome in hospitalized subjects with coronavirus disease 2019 from a nationwide analysis in China. Chest. (2020). doi: 10.1016/j.chest.2020.04.010. [Epub ahead of print].
7. Zhang J, Wang X, Jia X, Li J, Hu K, Chen G, et al. Risk factors for disease severity, unimprovement, and mortality in COVID-19 patients in Wuhan, China. Clin Microbiol Infect. (2020) 26:767–772. doi: 10.1016/j.cmi.2020.04.012
8. Li X, Xu S, Yu M, Wang K, Tao Y, Zhou Y, et al. Risk factors for severity and mortality in adult COVID-19 inpatients in Wuhan. J Allergy Clin Immunol. (2020). doi: 10.1016/j.jaci.2020.04.006. [Epub ahead of print].
9. Zheng Z, Peng F, Xu B, Zhao J, Liu H, Peng J, et al. Risk factors of critical & mortal COVID-19 cases: A systematic literature review and meta-analysis. J Infect. (2020). doi: 10.1016/j.jinf.2020.04.021. [Epub ahead of print].
10. Gao L, Jiang D, Wen XS, Cheng XC, Sun M, He B, et al. Prognostic value of NT-proBNP in patients with severe COVID-19. Respir Res. (2020) 21:83. doi: 10.1186/s12931-020-01352-w
11. Henry BM, de Oliveira MHS, Benoit S, Plebani M, Lippi G. Hematologic, biochemical and immune biomarker abnormalities associated with severe illness and mortality in coronavirus disease 2019 (COVID-19): a meta-analysis. Clin Chem Lab Med. (2020) 58:1021–8. doi: 10.1515/cclm-2020-0369
12. Du R-H, Liang L-R, Yang C-Q, Wang W, Cao T-Z, Li M, et al. Predictors of mortality for patients with COVID-19 pneumonia caused by sars-CoV-2: a prospective cohort study. Eur Respir J. (2020) 55:2000524. doi: 10.1183/13993003.00524-2020
13. Tang N, Li D, Wang X, Sun Z. Abnormal coagulation parameters are associated with poor prognosis in patients with novel coronavirus pneumonia. J Thromb Haemost. (2020) 18:844–7. doi: 10.1111/jth.14768
14. Yang X, Yang Q, Wang Y, Wu Y, Xu J, Yu Y, et al. Thrombocytopenia and its association with mortality in patients with COVID-19. J Thromb Haemost. (2020) 18:1469–72. doi: 10.1111/jth.14848
15. Zhang L, Yan X, Fan Q, Liu H, Liu X, Liu Z, et al. D-dimer levels on admission to predict in-hospital mortality in patients with Covid-19. J Thromb Haemost. (2020) 18:1324–29. doi: 10.1111/jth.14859
16. Hou H, Zhang B, Huang H, Luo Y, Wu S, Tang G, et al. Using IL-2R/lymphocyte for predicting the clinical progression of patients with COVID-19. Clin Exp Immunol. (2020) 201:76–84. doi: 10.1111/cei.13450
17. Wang F, Hou H, Luo Y, Tang G, Wu S, Huang M, et al. The laboratory tests and host immunity of COVID-19 patients with different severity of illness. JCI Insight. (2020) 5:e137799. doi: 10.1172/jci.insight.137799
18. Liu F, Li L, Xu M, Wu J, Luo D, Zhu Y, et al. Prognostic value of interleukin-6, C-reactive protein, and procalcitonin in patients with COVID-19. J Clin Virol. (2020) 127:104370. doi: 10.1016/j.jcv.2020.104370
19. Lagunas-Rangel FA, Chávez-Valencia V. High IL-6/IFN-γ ratio could be associated with severe disease in COVID-19 patients. J Med Virol. (2020). doi: 10.1002/jmv.25900. [Epub ahead of print].
20. Braga M, Vignali A, Gianotti L, Cestari A, Profili M, Carlo VD. Immune and nutritional effects of early enteral nutrition after major abdominal operations. Eur J Surg. (1996) 162:105–12.
21. Moulias R, Devillechabrolle A, Lesourd B, Proust J, Marescot MR, Doumerc S, et al. Respective roles of immune and nutritional factors in the priming of the immune response in the elderly. Mech Ageing Dev. (1985) 31:123–37. doi: 10.1016/S0047-6374(85)80023-3
22. Loftus TJ, Brown MP, Slish JH, Rosenthal MD. Serum Levels of Prealbumin and Albumin for Preoperative Risk Stratification. Nutr Clin Pract. (2019) 34:340–8. doi: 10.1002/ncp.10271
23. Gunerhan Y, Koksal N, Sahin UY, Uzun MA, Eksioglu-Demiralp E. Effect of preoperative immunonutrition and other nutrition models on cellular immune parameters. World J Gastroenterol. (2009) 15:467–72. doi: 10.3748/wjg.15.467
24. Xu B, Fan C-Y, Wang A-L, Zou Y-L, Yu Y-H, He C, et al. Suppressed T cell-mediated immunity in patients with COVID-19: a clinical retrospective study in Wuhan, China. J Infect. (2020) 81:e51–60. doi: 10.1016/j.jinf.2020.04.012
25. Liu Z, Long W, Tu M, Chen S, Huang Y, Wang S, et al. Lymphocyte subset (CD4+, CD8+) counts reflect the severity of infection and predict the clinical outcomes in patients with COVID-19. J Infect. (2020). doi: 10.1016/j.jinf.2020.03.054. [Epub ahead of print].
26. Fu L, Wang B, Yuan T, Chen X, Ao Y, Fitzpatrick T, et al. Clinical characteristics of coronavirus disease 2019. (COVID-19) in China: a systematic review and meta-analysis. J Infect. (2020) 80:656–65. doi: 10.1016/j.jinf.2020.03.041
27. Guo W, Li M, Dong Y, Zhou H, Zhang Z, Tian C, et al. Diabetes is a risk factor for the progression and prognosis of COVID-19. Diabetes Metab Res Rev. (2020) 2020:e3319. doi: 10.1002/dmrr.3319
28. DeLong ER, DeLong DM, Clarke-Pearson DL. Comparing the areas under two or more correlated receiver operating characteristic curves: a nonparametric approach. Biometrics. (1988) 44:837–45. doi: 10.2307/2531595
29. Spiteri G, Fielding J, Diercke M, Campese C, Enouf V, Gaymard A, et al. First cases of coronavirus disease 2019 (COVID-19) in the WHO European Region, 24 January to 21 February 2020. Euro Surveill. (2020) 25:2000178. doi: 10.2807/1560-7917.ES.2020.25.9.2000178
30. Yuan J, Li M, Lv G, Lu ZK. Monitoring transmissibility and mortality of COVID-19 in Europe. Int J Infect Dis. (2020) 95:311–15. doi: 10.1016/j.ijid.2020.03.050
31. Escalera-Antezana JP, Lizon-Ferrufino NF, Maldonado-Alanoca A, Alarcon-De-la-Vega G, Alvarado-Arnez LE, Balderrama-Saavedra MA, et al. Clinical features of the first cases and a cluster of Coronavirus Disease 2019 (COVID-19) in Bolivia imported from Italy and Spain. Travel Med Infect Dis. (2020) 2020:101653. doi: 10.1016/j.tmaid.2020.101653
32. National Institute for H, Care Excellence in collaboration with NHSE, Improvement NHS. Managing COVID-19 symptoms (including at the end of life) in the community: summary of NICE guidelines. BMJ. (2020) 369:m1461. doi: 10.1136/bmj.m1461
33. Phua J, Weng L, Ling L, Egi M, Lim C-M, Divatia JV, et al. Intensive care management of coronavirus disease 2019 (COVID-19): challenges and recommendations. Lancet Resp Med. (2020). 8:506–17. doi: 10.1016/S2213-2600(20)30161-2
34. Wu C, Chen X, Cai Y, Xia J, Zhou X, Xu S, et al. Risk factors associated with acute respiratory distress syndrome and death in patients with coronavirus disease 2019 pneumonia in Wuhan, China. JAMA Intern Med. (2020) e200994. doi: 10.1001/jamainternmed.2020.0994. [Epub ahead of print].
35. Mkhize BT, Mabaso MHL, Madurai S, Mkhize-Kwitshana ZL. The investigation of the use of prealbumin as a tool for nutritional assessment in adults coinfected with HIV and intestinal helminth parasites in kwazulu-natal, South Africa. Biomed Res Int. (2018) 2018:7805857. doi: 10.1155/2018/7805857
36. Sharma A, Giraddi G, Krishnan G, Shahi AK. Efficacy of serum prealbumin and crp levels as monitoring tools for patients with fascial space infections of odontogenic origin: a clinicobiochemical study. J Maxillofac Oral Surg. (2014) 13:1–9. doi: 10.1007/s12663-012-0376-4
37. Bateman RM, Sharpe MD, Jagger JE, Ellis CG, Solé-Violán J, López-Rodríguez M, et al. 36th international symposium on intensive care and emergency medicine: Brussels, Belgium. Crit Care. (2016) 20(Suppl 2):94. doi: 10.1186/s13054-016-1208-6
38. Hatzistilianou M. Diagnostic and prognostic role of procalcitonin in infections. ScientificWorldJournal. (2010) 10:1941–6. doi: 10.1100/tsw.2010.181
39. Aoshi T, Koyama S, Kobiyama K, Akira S, Ishii KJ. Innate and adaptive immune responses to viral infection and vaccination. Curr Opin Virol. (2011) 1:226–32. doi: 10.1016/j.coviro.2011.07.002
40. Newton AH, Cardani A, Braciale TJ. The host immune response in respiratory virus infection: balancing virus clearance and immunopathology. Semin Immunopathol. (2016) 38:471–82. doi: 10.1007/s00281-016-0558-0
41. Luo Y, Xie Y, Zhang W, Lin Q, Tang G, Wu S, et al. Combination of lymphocyte number and function in evaluating host immunity. Aging (Albany NY). (2019) 11:12685–707. doi: 10.18632/aging.102595
42. Holly MK, Diaz K, Smith JG. Defensins in Viral Infection and Pathogenesis. Annu Rev Virol. (2017) 4:369–91. doi: 10.1146/annurev-virology-101416-041734
43. Hanada S, Pirzadeh M, Carver KY, Deng JC. Respiratory viral infection-induced microbiome alterations and secondary bacterial pneumonia. Front Immunol. (2018) 9:2640. doi: 10.3389/fimmu.2018.02640
44. Guan WJ, Ni ZY, Hu Y, Liang WH, Ou CQ, He JX, et al. Clinical Characteristics of Coronavirus Disease 2019 in China. N Engl J Med. (2020) 382:1708–20. doi: 10.1056/NEJMoa2002032
Keywords: coronavirus disease 2019, severe acute respiratory syndrome coronavirus 2, prealbumin, routine laboratory tests, prognosis, immune status, fatal patients, recovered patients
Citation: Luo Y, Xue Y, Mao L, Yuan X, Lin Q, Tang G, Song H, Wang F and Sun Z (2020) Prealbumin as a Predictor of Prognosis in Patients With Coronavirus Disease 2019. Front. Med. 7:374. doi: 10.3389/fmed.2020.00374
Received: 14 May 2020; Accepted: 18 June 2020;
Published: 26 June 2020.
Edited by:
Abdallah Samy, University of Kansas, United StatesReviewed by:
Vijaya Anand, Bharathiar University, IndiaJianxiong Zeng, University of Southern California, United States
Mehmet Demirci, Beykent University, Turkey
Rusliza Basir, Universiti Putra Malaysia, Malaysia
Copyright © 2020 Luo, Xue, Mao, Yuan, Lin, Tang, Song, Wang and Sun. This is an open-access article distributed under the terms of the Creative Commons Attribution License (CC BY). The use, distribution or reproduction in other forums is permitted, provided the original author(s) and the copyright owner(s) are credited and that the original publication in this journal is cited, in accordance with accepted academic practice. No use, distribution or reproduction is permitted which does not comply with these terms.
*Correspondence: Feng Wang, fengwang@tjh.tjmu.edu.cn; Ziyong Sun, zysun@tjh.tjmu.edu.cn