- 1School of Earth and Atmospheric Sciences, Georgia Institute of Technology, Atlanta, GA, United States
- 2Rosenstiel School of Marine and Atmospheric Science, University of Miami, Miami, FL, United States
- 3Marine Science Institute, The University of Texas Austin, Port Aransas, TX, United States
- 4Marine Physical Laboratory, Scripps Institution of Oceanography, La Jolla, CA, United States
- 5Department of Marine Sciences, University of Georgia, Athens, GA, United States
- 6Department of Physical Oceanography, Woods Hole Oceanographic Institution, Woods Hole, MA, United States
The 2010 Macondo oil well blowout consisted in a localized, intense infusion of petroleum hydrocarbons to the deep waters of the Gulf of Mexico. A substantial amount of these hydrocarbons did not reach the ocean surface but remained confined at depth within subsurface plumes, the largest and deepest of which was found at ∼ 1000–1200 m of depth, along the continental slope (the deep plume). This review outlines the challenges the science community overcame since 2010, the discoveries and the remaining open questions in interpreting and predicting the distribution, fate and impact of the Macondo oil entrained in the deep plume. In the past 10 years, the scientific community supported by the Gulf of Mexico Research Initiative (GoMRI) and others, has achieved key milestones in observing, conceptualizing and understanding the physical oceanography of the Gulf of Mexico along its northern continental shelf and slope. Major progress has been made in modeling the transport, evolution and degradation of hydrocarbons. Here we review this new knowledge and modeling tools, how our understanding of the deep plume formation and evolution has evolved, and how research in the past decade may help preparing the scientific community in the event of a future spill in the Gulf or elsewhere. We also summarize briefly current knowledge of the plume fate – in terms of microbial degradation and geochemistry – and impacts on fish, deep corals and mammals. Finally, we discuss observational, theoretical, and modeling limitations that constrain our ability to predict the three-dimensional movement of waters in this basin and the fate and impacts of the hydrocarbons they may carry, and we discuss research priorities to overcome them.
Introduction and Background
On April 20th, 2010, a deep-sea blowout caused the ultra-deep drilling platform Deepwater Horizon (DWH) to explode, killing 11 workers. Two days later the platform sank at the Macondo Prospect in the Mississippi Canyon Leasing Block 252 (MC 252), located about 66 km offshore the southeast coast of Louisiana. The sinking further ruptured the buckled riser pipe and lead to an uncontrolled release of live (containing dissolved gas) oil and free gas from a depth of approximately 1522 m into the waters of the Gulf of Mexico (GoM). The Macondo well was capped 87 days later, on July 15th, and permanently sealed September 19th. By then, the largest oil spill in US history had discharged over 4 million barrels of oil and about 250,000 metric tons of hydrocarbon gases into the northern GoM (Joye, 2015; Gros et al., 2017). More than 7000 tons of chemical dispersants, namely Corexit 9500A and Corexit EC9527A, were applied at the ocean surface (∼ 5000 tons) and injected at depth at the broken wellhead (∼ 2000–3000 tons) in the attempt to break the oil into small droplets and ease its dispersal and degradation (Lubchenco et al., 2012). Such subsea dispersant injection (SSDI) was unprecedented and was tested by deploying increasing volumes of dispersant beginning on day 10 of the spill until the Macondo well was capped (Gros et al., 2017; Paris et al., 2018).
The explosive outgassing at the blowout preventer (BOP) resulted in a turbulent jet that atomized the live oil into a spectrum of small drops (Aliseda et al., 2010; Bandara and Yapa, 2011; Johansen et al., 2013; Zhao et al., 2014a,b, 2015, 2016, 2017; Nissanka and Yapa, 2016; Socolofsky et al., 2016; Li et al., 2017; Wang et al., 2018; Murawski et al., 2020). Together, they formed a prominent plume of petroleum hydrocarbons at the trap height, about 300 meter above the wellhead (Socolofsky et al., 2011; Bp Gulf Science Data1, 2016), the so-called deep plume. Constraining the amount and fate of oil and gas released from the Macondo Blowout appeared immediately difficult as there was no metering of the flow underwater until the installation of an oil collecting cap (Aliseda et al., 2010; Griffiths, 2012). The initial estimates challenging the rate of the flow were based on quantitative analysis of video of the oil as it exited the broken pipes (Crone and Tolstoy, 2010). The deep plume, together with other smaller, secondary plumes found higher up in the water column (Diercks et al., 2010; Joye et al., 2011; Reddy et al., 2012) entrained nearly 50% of the discharged oil and most of the low molecular weight gases (McNutt et al., 2012; Joye, 2015), and largely contributed to the uncertainty.
The deep plume, for which more observations and numerical simulations are available than for the secondary ones, was distinct from the natural hydrocarbon seep plumes (Rogener et al., 2018). Specifically, it was characterized by extremely high methane concentrations (>380 μM) according to observations taken in May 2010 (Joye et al., 2011). These elevated gas concentrations were likely due to fact that the oil and gas fluid mixture that exited from the reservoir at enormous pressure, above of 900 bars, and at high temperature (Satter and Iqbal, 2016) came into sudden contact with the low temperature (4–6°C) and lower hydrostatic pressure (100–150 bar) of the deep water (Reddy et al., 2012). This abrupt change in the environmental conditions likely caused a super saturation of gas, its expansion and the formation of hydrates and gas bubble nucleation of the liquid oil phase (Joye et al., 2011; Pesch et al., 2018). Oil concentrations were elevated both in the deep plume and in the secondary plumes – BTEX (benzene, toluene, ethylbenzene and xylene) concentrations were as high as 50–150 μg L–1 (Camilli et al., 2010) – as oil droplets segregated along the isopycnals in which they were neutrally buoyant (Paris et al., 2012).
Non-hydrostatic buoyant forces advected the deep plume until its density matched the density of the surrounding water. From this point onward, the deep plume, composed of dissolved hydrocarbon compounds, small oil droplets and gas bubbles entrained with seawater, was transported by the ocean currents downstream and slowly developed laterally (Socolofsky et al., 2011; Gros et al., 2017; Dissanayake et al., 2018). This lateral multiphase plume gradually moved away from its source and some of the hydrocarbons contained in it rose out into the water column. At this stage, physical processes, varying on spatial scales from centimeters to tens of kilometers, and complex biogeochemical processes influenced the final distribution and fate of the liquid and gas phases of petroleum.
Increasing computational capabilities over the two decades preceding the 2010 spill allowed the development of complex hydrodynamic ocean models that implement complex data assimilation methods and could predict reasonably well the time evolution of circulations at the ocean mesoscales (∼20–200 km) over 5–7 days (e.g., Liu et al., 2011). At the same time, advances in oil science and in parameterizations of essential processes, such as oil droplet formation and fate (e.g., Yapa and Zheng, 1997; Yapa et al., 1999) largely improved numerical models for forecasting oil spill dispersal (reviews by Spaulding, 1988; ASCE, 1996; Reed et al., 1999). Over the same period, industry, government and academia undertook efforts to develop numerical models to simulate the complexities of deep-water spills following a sustained increase over time in the interest for deepwater and ultra-deepwater exploitation (Dutta, 1997; Ji et al., 2004). Further technical advances in super-computer and parallel computing allowed high-throughput and thus more realistic 3D modeling and visualization of the deep plume generated during the DWH blowout, as discussed in detail later in this review (e.g., Paris et al., 2012; Yapa et al., 2012).
Background: Physical Processes
In terms of the physical processes affecting the evolution of the deep plume, boundary mixing (e.g., mixing of fluid near a vertical solid boundary provided by the continental slope and/or ocean bottom) is the primary process at play, and it provides intense velocity gradients in regions of stratified fluid. Three broad categories of forcing mechanisms control boundary mixing, as briefly summarized here in relation to the GoM configuration and conditions.
One class emphasizes either that the large-scale inclination of the continental slope of the GoM approximately matches that of ray trajectories associated with the energy-containing frequencies of the internal wavefield (Figure 1), or that topographic roughness scatters an impinging internal wavefield to smaller scales (e.g., Müller and Liu, 2000). It should be noted that in the first case reflection and/or generation of internal waves under these approximate matching, critical conditions often result in significant velocity gradients and mixing (e.g., Eriksen, 1985; Garrett and Gilbert, 1988). Imprinted upon this class are the spatial and temporal homogeneity of the forcing parameters (i.e., tides, the background wavefield, topography).
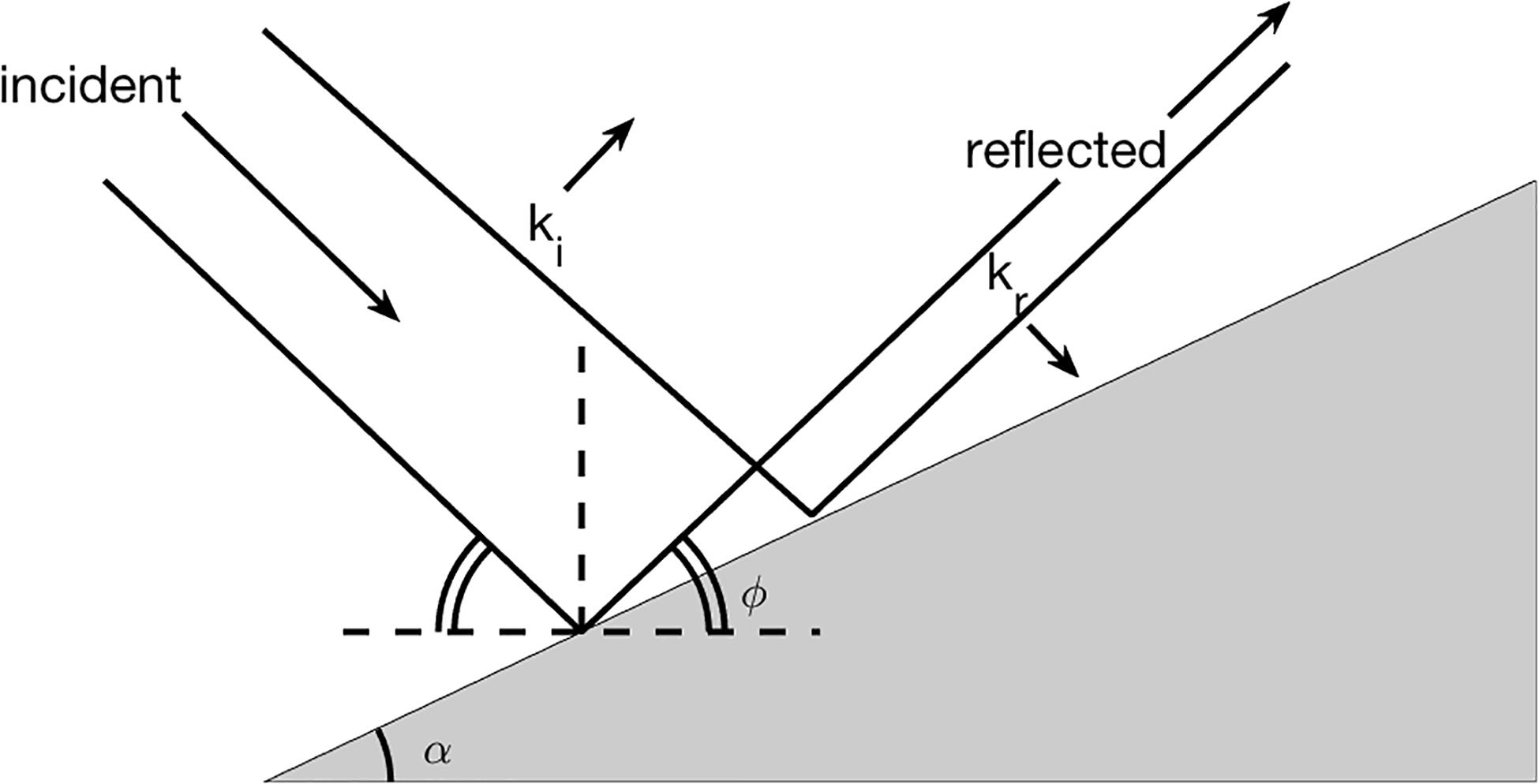
Figure 1. Class #1: Ambient wave reflection. The key to understanding how the kinematic bottom boundary condition of no-normal flow changes the internal wave field over variable topography is contained in the internal wave dispersion relation. The internal wave frequency is determined not by wavelength, but rather by the angle of the wave vector with respect to the vertical, φ, and this determines the trajectories of water parcels. A simple visualization can be achieved by drawing the crests and troughs of a wave incident at the boundary and requiring that the reflected wave matches the propagation of these crests and troughs along the boundary (black solid lines). Depicted are incident and reflected wavenumbers ki and kr with phase propagating with the wavevectors. Wave frequency, and hence φ, are preserved under reflection, independent of the orientation of the slope, α. Transformation of incident energy to very high wavenumber kr occurs at a frequency for which φ≅α, leading to enhanced wave-breaking and mixing (e.g., Chalamalla et al., 2013). Figure after Garrett (2001).
The second class of turbulence forcing mechanisms emphasizes eddy flow in combination with topographic variability superimposed upon the large-scale continental slope. When the amplitude of the topographic variability is small and has length scales permitting the radiation of internal waves, then internal lee waves (Bretherton, 1969; Thorpe, 1992) are generated (Figure 2A). When the topographic variability is large, however, near-boundary wave breaking, hydraulic like effects, flow separation and vortex shedding arise, and dominate the radiating response (e.g., Baines, 1995; Figure 2B). Boundary mixing associated with these finite amplitude phenomena can be quite intense and typically occurs in stratified waters apart from the boundary.
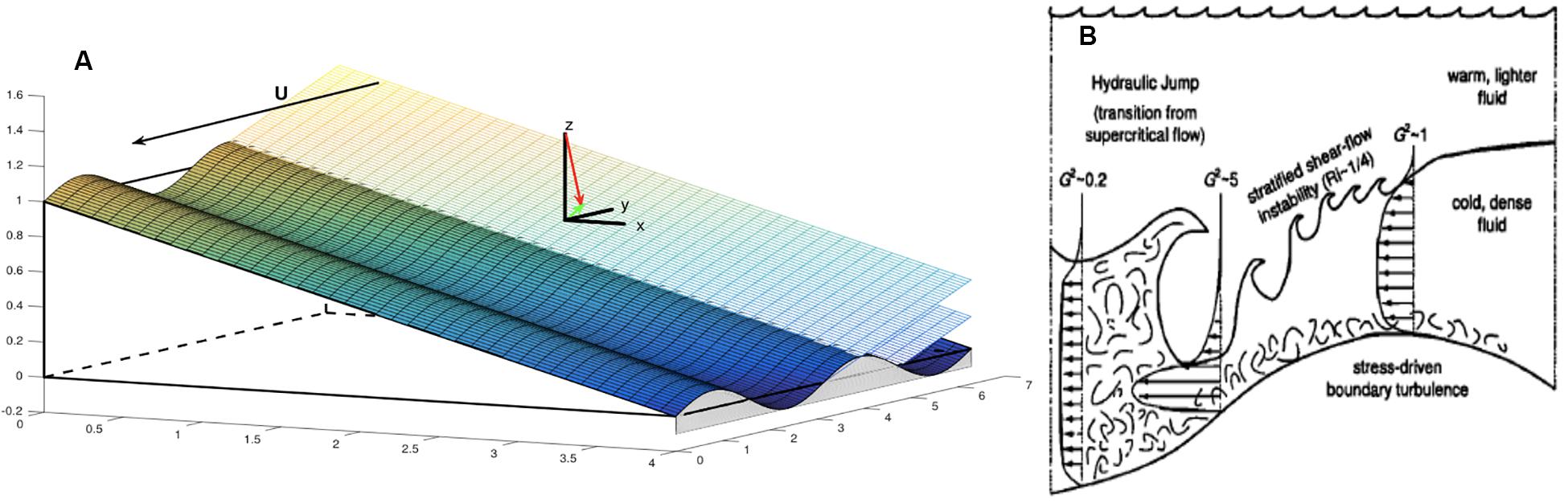
Figure 2. Class #2: Mean flows – lee waves and hydraulics. Mean flow over irregular topography can induce radiating internal waves if the topographic irregularities superimposed on the slope have a “small” amplitude (A, after Thorpe, 1992). In this case one assumes a quasi-stationary internal wave response that varies on the time scale of the background flow. Mean flow direction is indicated by the arrow in association with U. A right-hand x – y – z coordinate system defines the orientation of the wave vector (red) normal to the wave phase planes and its projection upon the horizontal (green), in the upslope direction. Intense mixing can occur if the topographic irregularities are ‘finite amplitude’ and a parameter regime of internal hydraulics is entered (B, following Nash and Moum, 2001). Turbulence and mixing occur in conjunction with bottom stress in an accelerating downslope jet, high shear on an interface with overlying fluid and within the hydraulic transition (jump). Without the inclined plane, the dynamical transition between waves and hydraulics occurs when the topographic perturbations have an amplitude similar to that of the lee wave, flow blocking initiates and vertical shear in the wave response is approximately equal to the buoyancy frequency N. The dynamical impact of the inclined plane is largely unexplored.
Intense mixing can occur if the topographic irregularities are “finite amplitude” and a parameter regime of internal hydraulics is entered (Panel B, following Nash and Moum, 2001). Turbulence and mixing occur in conjunction with bottom stress in an accelerating downslope jet, high shear on an interface with overlying fluid and within the hydraulic transition (jump). Without the inclined plane, the dynamical transition between waves and hydraulics occurs when the topographic perturbations have an amplitude similar to that of the lee wave, flow blocking initiates and vertical shear in the wave response is approximately equal to the buoyancy frequency N. The dynamical impact of the inclined plane is largely unexplored.
A third class is provided by turbulent stresses associated with the viscous condition of no-flow at the bottom boundary and an insulating condition on temperature (buoyancy). Over sloping topography, such as along the continental slope of the GoM, the bottom boundary conditions trigger cross-slope mass and buoyancy transport in the Ekman layer (Figure 3A), leading to a reduction in the near-bottom flow and a decrease in drag as the near-boundary buoyancy anomalies come into geostrophic balance. The steady-state limit, with zero bottom velocity, is known as the arrested Ekman layer (Garrett et al., 1993). In addition to the potential reduction in turbulence associated with drag decrease, the reduction of stratification within the Ekman layer opens the possibility for a less efficient conversion of turbulent stress to mixing (i.e., the “mixing of mixed fluid” in Garrett, 1979). Thus, in this third class it is important to avoid the arrested state (i.e., the state at which the flow is totally stopped) by getting mixed water efficiently into and out of the boundary layer. One of the more intriguing prospects raised in recent years is that submesoscale instabilities related to a reversal in the sign of Ertel potential vorticity may contribute to this end.
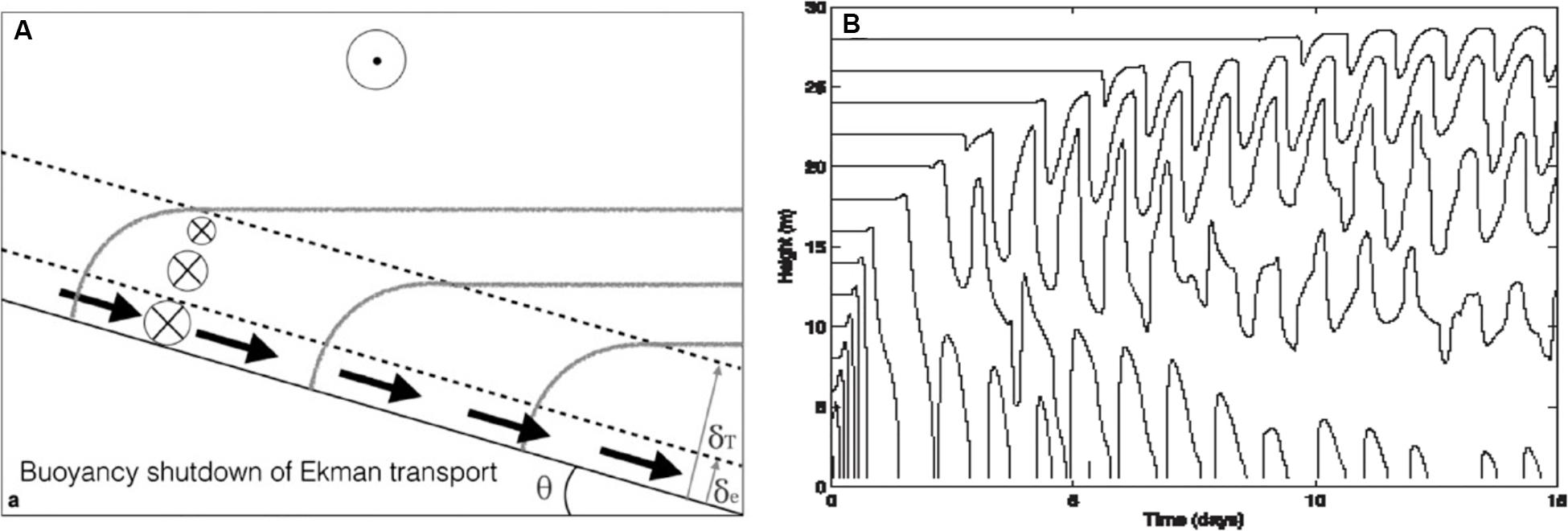
Figure 3. Class #3: mean flows – Ekman layers. The intuition concerning the impact of the viscous bottom boundary condition of no-flow at the boundary comes through rotating into a one-dimensional coordinate system, characterizing the bottom boundary condition via a quadratic drag and solving for a stationary state. Pictured in (A) (from Ruan and Thompson, 2016) is the Ekman layer in its downwelling configuration. This boundary layer system is driven by downslope transports (bold arrows) of light water which results in statically unstable conditions on a height scale δe over which the quadratic drag projects. These unstable conditions are coupled to internal wave band motions (B, from Brink and Lentz, 2010a) that parallel the slope and are driven to sufficient amplitude as to mix fluid far above the height scale over which the quadratic drag projects. In (A), the height scale over which the bottom stress projects is depicted by the lowest dashed line and the height scale over which mixing impacts the near-boundary density field is depicted by the upper dashed line (δT). The direction of the overlying flow is indicated at the top center, solid arrows depict the associated downslope Ekman transport, isopycnals are in gray shading. In (B), isopycnals are plotted as a function of depth versus time with frequency corresponding to the topographic angle θ in (A). The steady flow regimes depicted are clearly naive.
The second and third classes are temporally intermittent, depending upon the presence or absence of near boundary eddy variability. In the second class, mixing can be localized to specific small-scale features in the topography. Such intermittency leads to large uncertainties when trying to predict plume dispersion.
Background: The Deep Plume
The behavior of accidental deep-sea oil and gas release was theoretically described (Socolofsky and Adams, 2002, 2005) and observed in laboratory and experimental applications (Leitch and Baines, 1989; Zheng and Yapa, 2000; Johansen et al., 2003), also following the observation of a propagating oil plume in the south-west Atlantic at 150–200 m of depth in 1978 (Harvey et al., 1979). Yet the DWH oil spill was the first in situ documentation of a stratified multiphase plume found at a depth of over 1000 m and along the continental slope (Diercks et al., 2010; Camilli et al., 2010; Hazen et al., 2010; Valentine et al., 2010; Kessler et al., 2011; Socolofsky et al., 2011). The evolution of this deep plume is the focus of this work. We review the knowledge acquired during and in the aftermath of the DWH oil spill and discuss critical issues yet unsolved that hinder development of better response strategies and improvement of forecast capabilities. With this goal in mind, our foci are the physical and biogeochemical processes that should be included or properly parameterized in models. We also discuss, although briefly, the current state and many open questions of the deepwater plume impact assessment.
Deep Plume: First Modeling Attempts
During and in the aftermath of any oil spill, it is essential to predict the dispersal pathways and the ultimate fate of the oil. However, at the time of the DWH blowout and subsequent uncontrolled spill most available models for oil dispersal were constrained to simulate processes occurring either at and near the ocean surface (Spaulding, 1988; Reed et al., 1999; Lehr et al., 2002; French-McCay, 2004; Beegle-Krause and Lynch, 2005), or in the immediate vicinity of the wellhead, in the so called near-field (Johansen, 2000; Chen and Yapa, 2003; Zheng et al., 2003; Yang and Chen, 2004). Moreover, despite the existence of near-surface models and near-field models, only few attempts had been made to integrate their results (Yapa et al., 1999, 2003; Beegle-Krause and Lynch, 2005). Most importantly, in 2010 an essential parameter for near-field models was not available: the size distribution of the gas bubbles and oil droplets formed at the jet break-up region. This critical initial condition could not be obtained from empirical equations based on experimental data since they are not scalable to the size of riser pipes (Chen and Yapa, 2007; Li et al., 2008; Boxall et al., 2012; Yapa et al., 2012). Given that larger droplets rise faster and dissolve more slowly than smaller ones, an inaccurate initial size distribution can lead to incorrect predictions of hydrocarbon concentrations (Lindo-Atichati et al., 2016; Vaz et al., 2020). Further limitations included the lack of understanding of biodegradation at high pressure and low temperature, and of the effects of SSDI on hydrocarbon partitioning and biodegradation rates.
Oil released at high hydrostatic pressure is remarkably different from oil released at the sea surface (Camilli et al., 2012; Paris et al., 2012; Zick, 2013a,b; Aman et al., 2015; Gros et al., 2016; Pesch et al., 2018). By 2010 it was understood that processes occurring within a few meters from the wellhead are better captured with near-field models, which can simulate the rising buoyant mixture of oil, gas, entrained seawater and formation of gas hydrate, and determine the plume evolution, including dissolution and formation of plumes by density stratification (Camilli et al., 2010; Diercks et al., 2010; Socolofsky et al., 2011; Paris et al., 2012). Once the liquid, gas-saturated hydrocarbon leaves the plume mixture in the form of individual droplets, advection by ocean currents controls their distribution and far-field models can track the individual movement and fate of each droplet (Paris et al., 2012; Le Hénaff et al., 2012; Spaulding et al., 2015; Perlin et al., 2020).
At the onset of the 2010 DWH spill, several research groups from academia, the government and industry developed new or modified existing models to track the oil dispersal. They ranged in complexity from 2D trajectory models to complete 3D concentration estimations (National Commission on the Bp Deepwater Horizon Oil Spill and Offshore Drilling, 2011; Deepwater Horizon Oil Spill Principal Investigator Workshop, 2012). Most forecasts, however, were not publicly available, due to their proprietary nature, confidentiality agreements or government oversight (National Commission on the Bp Deepwater Horizon Oil Spill and Offshore Drilling, 2010). This occurred despite the same forecasts were mentioned as tools for forecast or assessment of oil dispersal on Senate Hearing (Response efforts to the Gulf Coast oil spill, 2010), or final injury assessment (Deepwater Horizon Natural Resource Damage Assessment Trustees, 2016). In the immediate aftermath of the spill, the lack of official information on oil flow rate (National Commission on the Bp Deepwater Horizon Oil Spill and Offshore Drilling, 2010), amount of SSDI applied and chemical composition of Corexit limited the development of reliable parameterizations. This led to a delay in publication of peer-reviewed studies addressing the formation and fate of the deep hydrocarbon plume, although its existence was revealed by in situ measurements (Camilli et al., 2010; Diercks et al., 2010; Hazen et al., 2010; Valentine et al., 2010; Reddy et al., 2012). Adcroft et al. (2010) were the first to assess likely pathways of the deep plume dispersal, but they considered only dissolved and neutrally buoyant material, without accounting for the hydrocarbon concentrations, their buoyancy, chemistry or fate processes. Paris et al. (2012) presented the first full 3D multi-fraction oil model generating a deep plume with maximum oil concentration moving to the northwest between the 1000 and 1300 m isobaths, corroborating with dissolved oxygen anomalies (Kessler et al., 2011).
On the physical oceanographic side, six different modeling systems were implemented during the spill to track and forecast oil movement over time using virtual particles with the attention focused on the oil that was accumulating at the ocean surface, as summarized in Liu et al. (2011). In all cases, the horizontal resolution was 5 km or coarser and it was soon apparent that none of them could reproduce the surface frontal structures several tens of kilometers long and few hundreds of meters wide, inside which the oil preferentially propagated away from the main slick. These submesoscale structures were identified in SAR images and aerial photos and became prominent from the end of May 2010 onward (e.g., Figure 3 in Walker et al., 2011). Furthermore, most, if not all, of these modeling scenarios predicted that the oil would leave the GoM and spread into the Atlantic Ocean, while it remained contained within the northern portion of the Gulf. In relation to the deep plume, the paucity of observations available below the GoM surface strongly hindered the reliability of data-assimilative model hindcasts (Liu et al., 2011). It became clear in the aftermath of the spill that the lack of knowledge of the variability of near bottom currents along the continental slope of the GoM and of the amount of diapycnal mixing in the bottom boundary layer was crucially limiting any modeling exercise related to the deep plume.
What Has Been Learned: Modeling Requirements to Trace the Deep Plume
Physical Modeling
In relation to physical drivers, there remains a critical need to better constrain spatial and temporal evolution of multiscale flow interactions, especially in relation to the bottom turbulent boundary layer, as mentioned earlier. Indeed, it is not fully understood how processes at scales from few centimeters to few kilometers translate into large-scale impacts that can modify or modulate the fate and transport of hydrocarbons in the ocean in general and in the GoM in particular.
The lack of observations of mixing rates between water masses of different densities (diapycnal mixing) in the GoM motivated the deep tracer release experiment performed by the GISR (Gulf of Mexico Integrated Spill Response) Consortium in the summer of 2012. Ledwell and collaborators (Ledwell et al., 2016) injected a 25 km streak of trifluoromethyl sulfur pentafluoride (CF3SF5) in the vicinity of the DWH site at about 1100 m depth along the 1250 m isobath. They sampled the tracer 5–12 days after release, and then again 4 months and 1 year later.
The 4-month survey found the vertical distribution of the tracer to be considerably broader near the continental slope than in the interior. Thus, the stations were divided into two sets: those shoreward (boundary set) and those seaward (interior set) of the 1500 m isobath, a threshold which seemed to separate the broader profiles from the narrower, for the most part. The two sets of profiles were then averaged separately to place limits on the turbulent diffusivity. A turbulent diapycnal eddy diffusivity of 1.3 × 10–4 m2s–1 was estimated by applying a model of the one-dimensional diffusion equation to the initial profile and to the 4-month mean interior profile. The diffusivity required to bring the initial profile to the 4-month boundary profile, on the other hand, was estimated to be greater than 4 × 10–4 m2s–1. These results indicated that the diffusivity in the boundary region, Kboundary, is greater than the interior diffusivity Kinterior. However, since it could not be known how much time any of the tracer had spent in the interior versus the boundary region, it was not possible to assign an accurate value to the diffusivity in either region.
The mean vertical profile from the survey at 12 months (Ledwell et al., 2016, Figure 14 therein) was nearly indistinguishable from the mean interior profile at 4 months. Applying the model to the transition between these two profiles yielded a diffusivity of 0.15 ± 0.05 × 10–4 m2s–1, much smaller than either diffusivity from the first period. This must be an upper limit on Kinterior, since the dispersion of the tracer would still have been influenced by processes near the boundaries, although it had spent relatively little time there. The inference is that Kboundary must have been much greater than 1.3 × 10–4 m2s–1. While this begs a question of just how much, the literature points to eddy-topography interactions as the most likely candidate for supporting intense, localized mixing. Indeed, downslope jets and hydraulic transitions can induce effective drag coefficients an order of magnitude larger than in the absence of such topography, and the resulting diapycnal mixing appears in well stratified regions outside the bottom mixed layer (e.g., Nash and Moum, 2001).
Very few investigations using regional circulation models have focused on the representation of diapycnal mixing and near bottom circulation along the continental slope of the GoM in the context of the DWH spill. Bracco et al. (2016) examined the generation mechanisms and mixing implications of mesoscale and submesoscale circulations. They compared outputs from a regional model run at 5 km and 1.6 km horizontal resolution over the period January 2010 – December 2012 maintaining the bathymetry smoothed at 5 km in both simulations, and found that ageostrophic submesoscale eddies and vorticity filaments are continuously formed from horizontal shear layers at the edges of intermittent, bottom-intensified, along-slope boundary currents in the higher resolution case. In other words, submesoscale circulations occur also in the bottom boundary layer and impact mixing along the continental slope, and their relevance is not limited to the surface mixed-layer. Furthermore, the simulations showed that in the De Soto Canyon the mean currents are weaker and less variable than in the rest of the northern GoM, and intense, long-lived submesoscale eddies form preferentially along the Florida continental slope. To the west of the Mississippi Fan, on the other hand, the mean currents are stronger and the circulation is highly variable in time and space, with many short-lived submesoscale filaments and eddies being formed and destroyed by the interactions with the bathymetry. Bracco and co-authors used Lagrangian (particles) and Eulerian (dye) passive tracers to quantify mixing, and the diapycnal coefficient was found to increase whenever submesoscale processes were abundant, and therefore to be larger along the slope to the west of the Fan compared to De Soto.
A subsequent modeling exercise investigated how diapycnal mixing along the continental slope was represented in nine regional ocean model simulations for varying horizontal (from 1 to 9 km) and vertical (from 25 to 150 layers) resolution (Bracco et al., 2018). An Eulerian tracer mimicked the conditions of the deep release experiment by Ledwell et al. (2016) which in turn was comparable to the 2010 spill. This work highlighted how both horizontal and vertical resolutions contribute equally to the modeled diffusivities. Vertical resolution in particular was found to be key to a realistic representation of the passive tracer propagation and its confinement along the continental slope for a long (several months) time.
More recently, the diapycnal diffusivity coefficient quantified after 4 months from the dye release has been broadly reproduced in a GoM simulation at 1 km horizontal resolution and 50 vertical layers using Lagrangian tracers (Bracco et al., 2019; see also Figure 4). Submesoscale circulations in the form of eddies and vorticity filaments were found to contribute to the modeled diffusivity by entraining fluid in their interior, suggesting that the second mechanism of turbulent mixing may have played a key role for both the deep plume and the GISR dye. Submesoscale filaments may have also contributed to the localization of the deep plume, limiting its lateral extension. The simulations also support the conclusion that if a deep spill would occur to the west of the Mississippi Fan, such a spill would potentially impact the continental slope more extensively, by mixing faster and covering more effectively a larger area (Bracco et al., 2016; Rogener et al., 2018).
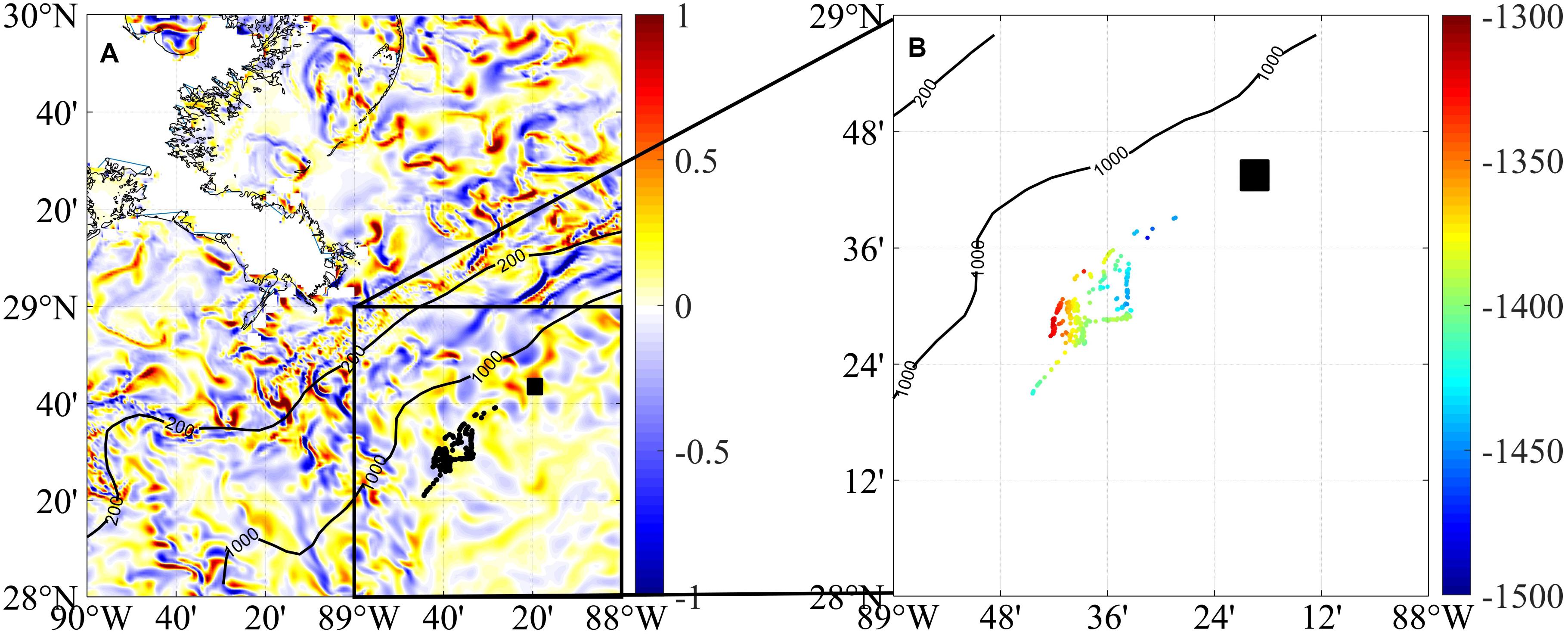
Figure 4. Physical-only modeling of advection around the DWH site. (A) Distribution of buoyancy neutral particles freely advected in three-dimensional space 1 month after their release at the Deepwater Horizon site. The particles are rendered in black and were released within the black box, then drifted to the southwest. The particle’s position is superimposed upon a map of the near-bottom vorticity field (ζ/f, estimated at the time of release). The particle dispersion at this 1-month time occurs following bathymetric and vorticity structures. (B) Zoom around the location of the particles with their depth indicated by color (Unit: m). Figure modified from Bracco et al. (2019).
Oil Transport and Fate Modeling
The 2010 DWH spill drove rapid advances in oil transport modeling. Improvements included better estimations of the initial size of droplets in the break up jet (Bandara and Yapa, 2011; Johansen et al., 2013; Zhao et al., 2014b, 2016; Li et al., 2017; Malone et al., 2018), and concerned the representation of both near-field (with respect to the DWH site) plume dynamics (Yapa et al., 2012; Gros et al., 2016, 2017; Spaulding et al., 2017), and far-field dispersal and fate (Le Hénaff et al., 2012; Paris et al., 2012; French-McCay et al., 2015; Lindo-Atichati et al., 2016; Spaulding et al., 2017; Perlin et al., 2020). The complexity of the processes included in the models increased, and it became possible to represent realistically the near-field processes in far-field models either by increasing parameterization accuracy, i.e., releasing droplets following observed lognormal droplet size distribution (DSD, from Spaulding et al., 2017; Malone et al., 2018) as done in Perlin et al. (2020), or by directly coupling near- and far-field models (French-McCay et al., 2016; Vaz et al., 2020).
Currently, two approaches can be used to estimate droplet and bubble sizes at the jet break-up. One is based on equilibrium models, which predict a characteristic bubble or droplet size at the end of the dynamic breakup region (e.g., Johansen et al., 2013; Li et al., 2017; Wang et al., 2018); the other is based on the so-called population models, which solve for the competing physical and chemical processes regulating breakup and coalescence, and allow for a dynamic solution of the entire bubble and droplet size distribution throughout the jet break-up area (Bandara and Yapa, 2011; Zhao et al., 2014b, 2015, 2017). Near-field models, which simulate the multiphase hydrocarbon plume following release, can be based on two different computational strategies. On one hand there are integral models, which solve the cross-sectionally averaged flow along the centerline trajectory of the plume, and were developed before the DWH spill (Yapa et al., 2001; Chen and Yapa, 2003; Johansen et al., 2003), and on the other there are three-dimensional computational fluid dynamics (CFD) models, based on Large Eddy Simulation (LES, Fraga et al., 2016; Olsen and Skjetne, 2016). The latter, however, remain computationally costly, and most applications developed during and post spill are based on integral models (Gros et al., 2017; Dissanayake et al., 2018).
Most current state-of-the-art far-field models represent the advection of individual droplets by integrating the deterministic velocity field from hydrodynamic models. This is commonly done using a spatio-temporal 4th order Runga-Kutta scheme together with accurate tri-cubic interpolation of the velocity field (Paris et al., 2013). Sub-grid scale turbulent diffusion is accounted for by adding a random displacement scaled by a diffusivity coefficient derived from observations (Okubo, 1987). The wind-induced drift, that is important to predict accurately the surface slicks at the ocean surface, is incorporated in the velocity field from the hydrodynamic model and explicitly considered (Reed et al., 1999; Le Hénaff et al., 2012; Perlin et al., 2020). The droplet terminal velocity is obtained from a relationship involving the droplet buoyancy as a function of density, size and shape (i.e., Reynolds number), and the viscosity and density of the ambient fluid (Clift et al., 1978; Chen and Yapa, 2003; Zheng et al., 2003; Yapa et al., 2010).
The dissolution and biodegradation of gas and oil droplets continue in the far-field. Biodegradation rates depend on the local microbial fauna, the composition of the petroleum fluids, and the environmental conditions (Chen et al., 2016; Joye et al., 2016). If droplets reach the ocean surface (which did not occur for those in the deep plume), evaporation affects low molecular components (Stout et al., 2013; Drozd et al., 2015; Romero et al., 2015) and they merge into continuous thin layers. During their ascent, droplets can aggregate with organic and inorganic matter, forming oil-particulate aggregates (OPA) and marine oil snow (MOS), both of which have a higher density than droplets, and subsequently settle on the seafloor (Khelifa et al., 2010; Daly et al., 2016; Zhao et al., 2016; Dissanayake et al., 2018; Vaz et al., 2020). Presently most far-field models account for the key processes that determine the oil fate (dissolution, biodegradation, evaporation, aggregation) using parameters that are constant, or at most dependent on ambient seawater variables, such as temperature, together with wind and wave fields for surface slicks, through a simple relationship (French-McCay et al., 2015; Perlin et al., 2020). Studies investigating the role of biodegradation, dissolution, and droplet size, however, have pointed to the importance of these parameter choices on the final distribution of liquid and dissolved hydrocarbon components (Paris et al., 2012; North et al., 2015; Lindo-Atichati et al., 2016; Perlin et al., 2020; Vaz et al., 2020). The next logical advancement in the oil modeling field may therefore be to include explicitly these processes as temporally and spatially variable. An example of a state-of-the-art oil transport model simulating the evolution of the DWH deep plume is provided in Figure 5.
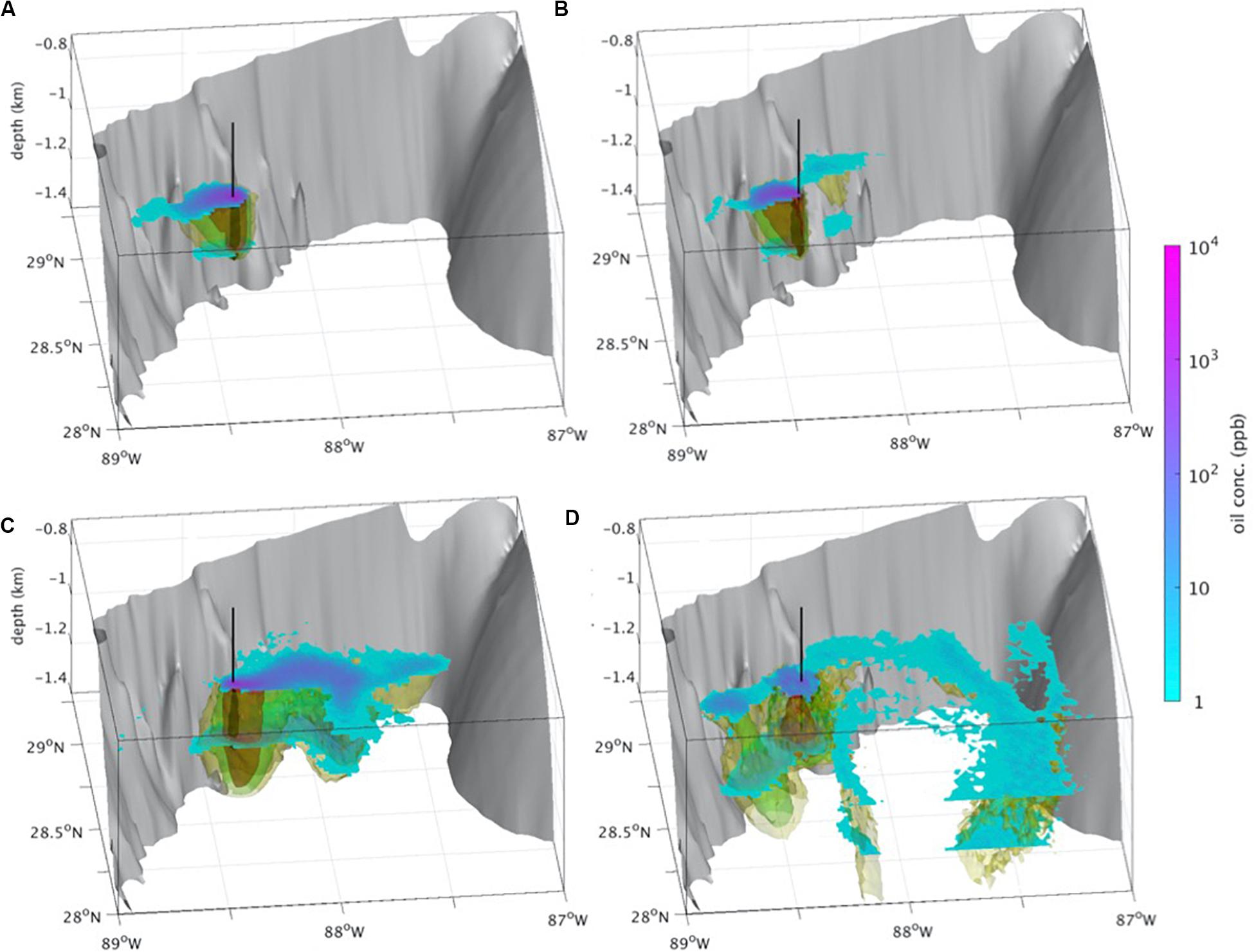
Figure 5. The Deepwater Horizon (DWH) deep plume model: 3-D evolution of the oil concentrations in the deep plume during (A) May 5th, 2010 (day 15); (B) May 30th, 2010 (day 41), (C) June 30th, 2010 (day 73), (D) July 29th, 2010 (day 100). Isosurfaces of the daily average subsea oil concentrations of 1000 ppb (black), 100 ppb (orange), 10 ppb (green), 1 ppb (yellow). Concentration layer gradients are plotted at 1,000 and 1,200 m, bounding the deep plume. Gray isosurface represents the bathymetry, and black line marks the axis of the Macondo well’s coordinates. The deep plume propagates initially toward the southwest until the end of May (A); it then oscillates and spreads along a southwest-northeast axis from Macondo, following bathymetric features (B); toward the end of June the core concentrations of the deep plume switch toward the northeast, and the plume penetrates the De Soto Canyon (C); through July the plume oscillates again and the core concentrations are transported to the southwest, while the northeast branch continues to disperse (D); this pattern was observed in Kessler et al. (2011). In addition, the 3D plume model shows strong subduction moving the plume high concentrations (i.e., 100 ppb) down to 1400 m. The simulations for this figure used the oil-CMS (Paris et al., 2012) algorithm, where 3,000 droplets were released every 2 h at the trap-height (i.e., 1222 m) above the wellhead from April 20th to July 15th, 2010, after which oil was tracked until July 28th (i.e., the simulation lasted 100 days). The initial DSD was simulated with a log-normal function (mean μ = 70μm and θ = 0.62), the pseudo-component composition and fate is from Perlin et al. (2020). Oil was dispersed using two implementations of GoM-HYCOM to better capture the vertical transport during the DWH (Paris et al., 2012): HyCOM expt. 20.1 until July 14th, and HyCOM expt. 31.0 from July 15th to October 31st. A random walk component simulates sub-gridscale processes with horizontal and vertical turbulent diffusivity coefficients set to 1 m2s–1 and 10–5 m2s–1, respectively.
Fate of Deep-Plume Hydrocarbons: Microbial Dynamics and Geochemistry
In terms of ecosystem impacts, the injection of hydrocarbons into the deep waters of the GoM initiated a dramatic shift in microbial community and in microbial activity. Within days to weeks, blooms of oil- and methane-degrading microorganisms occurred in the hydrocarbon infused waters (Hazen et al., 2010; Mason et al., 2012; Yang et al., 2016). Microbial diversity in the deepwater plumes decreased significantly, as specialist microbes bloomed in sequence taking advantage of local biogeochemical conditions (Hazen et al., 2010; Redmond and Valentine, 2012; Dubinsky et al., 2013; Kleindienst et al., 2015; Yang et al., 2016). Microbial methanotrophs (Rivers et al., 2013; Crespo-Medina et al., 2014) as well as microorganisms involved potentially in ethane and propane oxidation (Valentine et al., 2010) increased in response to the abundance of dissolved alkanes in solution. Microbial populations shifted rapidly and the altered distributions at the ecotype level (∼microbial species) were apparent for over a year (Kleindienst et al., 2015), suggesting a long-term impact on the GoM microbial ecology from the discharge.
While there is ample evidence describing the shifts in microbial community composition in surface and deep waters in response to the oil and gas infusion, the functional impacts of those population changes are difficult to constrain. Quantifying rates of methane oxidation is straightforward but robust rates of oil oxidation were not available at the time of the spill (e.g., Kleindienst et al., 2015; Sibert et al., 2016). As a consequence, methane and alkane oxidation rates are known but we do not have direct rates of oil oxidation for the immediate aftermath of the incident.
Methane oxidation rates increased rapidly compared to those previously reported (Wankel et al., 2010) and peaked about 6 weeks after the blowout began; methanotrophic activity patterns tracked changes in the abundance of novel methanotrophs as evidenced through quantitative Polymerase Chain Reaction or PCR (Crespo-Medina et al., 2014). While the observed high rates of methanotrophy were not maintained despite elevated methane concentrations, the potential for elevated methanotrophy persisted for almost 3 years after the incident (Rogener et al., 2018). The fall in methane oxidation rates observed in mid-June 2010 was unexpected, since methane concentrations were still well above background levels. Valentine et al. (2010) suggested that propane oxidation was most important at this time and that the propane oxidation stimulated methane oxidation only later. However, time series analysis of methane oxidation data suggests that methane oxidation rates had already peaked and subsequently dropped at the time of their sampling, leaving other alkane oxidation processes to dominate (Crespo-Medina et al., 2014).
The fact that the potential for methanotrophy was sustained for years after the incident suggests that once methanotrophic biomass is generated, it remains functionally viable and can be transported by the currents. This perpetuates the impact of a hydrocarbon infusion on the system (Rogener et al., 2018). The long-term methanotrophy potential may be linked to the peculiarity of the dynamical features, such as submesoscale eddies and filaments, that populate the continental slope in De Soto Canyon. These features may have trapped high concentrations of methane at their interior, releasing it slowly into the GoM interior (see left column in Figure 16 in Bracco et al., 2016). Similarly, natural methane seepage may stimulate local accumulations of methanotroph biomass that primes a system for methanotrophy and may facilitate rapid microbial responses to increased methane inputs. Importantly, the fate of methane in the deepwater plumes and in the Gulf system after the DWH oil well blowout was closely tied to the physical circulation (Crespo-Medina et al., 2014) that modulated biogeochemical dynamics and regulated the microbial response to methane inputs.
Oil oxidation rates are, on the other hand, very difficult to constrain. They were only determined indirectly (Hazen et al., 2010) and, as such, not much insight into the mechanisms, regulation and the potential fate of oil is available. The first reports of oil consumption in the deepwater plume presented conflicting estimates of biodegradation rates: Hazen et al. (2010) reported rapid oxidation rates on the time scale of weeks for alkanes, but Camilli et al. (2010) suggested extremely slow rates of oil degradation based on patterns of oxygen drawdown and oil concentrations. Oxygen drawdown patterns have also been linked, in hydrodynamical simulations that included passive tracers, to submesoscale coherent structures, such as vortices and filaments (Bracco et al., 2016). A more comprehensive estimate of hydrocarbon biodegradation rates was obtained by Du and Kessler (2012) tracking oxygen anomalies in the deepwater. They calculated that approximately 280,000 metric tons of hydrocarbons were oxidized. The fate of roughly 50% of the discharged hydrocarbon can be attributed to biological oxidation (28–34%) or sedimentation to the benthos (2–15%). Another 25% was recovered from the broken riser pipe (Joye et al., 2016). This leaves about 25–40% of the discharged oil and gas uncounted for in the hydrocarbon budget (Joye, 2015; Joye et al., 2016; see also Figure 6).
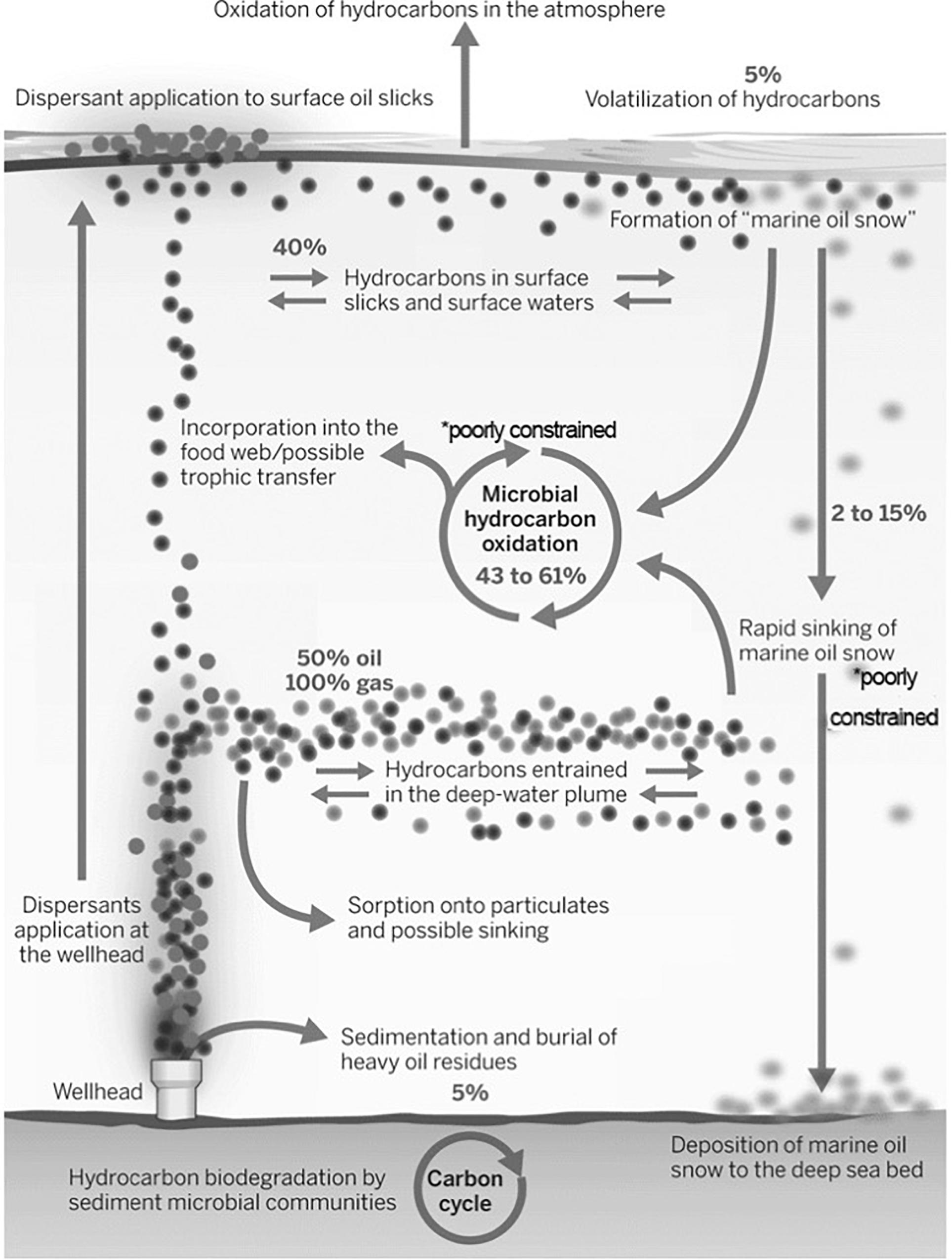
Figure 6. Approximate (likely) distribution of oil (darker circles) and gas (lighter circles) during the Deepwater Horizon well blowout; dispersant addition is also noted, and so is the deep plume contribution. Available data suggest that the long-term fate is known for only up to 76% of the discharged hydrocarbons. Oil that may have deposited in beaches and coastal marshes is not accounted in the figure. Modified from Joye (2015).
Impacts of the Deepwater Plume on Coldwater Corals and Upper Trophic Levels
The high concentrations of hydrocarbons and COREXIT at depth impacted coldwater corals, fish and mammal populations. In the case of the DWH spill, it is not possible to distinguish between impacts due to the deep plume and those from hydrocarbon accumulation at depth due to the formation, and subsequent sinking and sedimentation of marine oil snow (Daly et al., 2016). Large amounts of marine snow in the form of mucus-rich flocs were generated during the spill in the proximity of oil slicks as by-product of the microbial community bloom (Passow et al., 2012; Ziervogel et al., 2012; Gutierrez et al., 2013; Passow, 2016). Additionally, marine oil snow was generated by the sinking of diatom aggregates, as diatoms were present in exceptionally high concentrations following the blowout (Hu et al., 2011). These aggregates contributed to the oil sedimentation rates and to the redistribution of oil from the euphotic layer into the deeper water column (Passow et al., 2017).
Impacts on Coldwater Corals
The continental slope of the GoM hosts a variety of deep-sea, cold-water corals across a wide range of depths. Several known colonies are located in proximity (<30 km) of the DWH site. Deep-sea corals are foundational to the benthos, are commonly found on hard grounds, and support a biodiverse community of invertebrates and fishes. Logistical challenges prevent a direct quantification of their spatial distribution, abundance and essential factors that affect their life cycle. They grow very slowly, from just few mm to a couple of cm at most per year per colony, but they can live for hundreds to thousands of years (Roark et al., 2009; Risk et al., 2002; Prouty et al., 2016).
The first deep sea coral community impacted by the DWH spill was discovered in the fall of 2010 and was dominated by the octocoral Paramuricea biscaya (Grasshoff, 1977). It was found at about 11 km from the well, in the lease block Mississippi Canyon [MC] 294, at a depth of 1370 m (White et al., 2012). More impacted coral communities of P. biscaya were discovered in early 2011 at greater depths, 9 and 22 km from the well (Fisher et al., 2014). Visually, the corals appeared loosely covered in dark flocculent material that contained a mixture of Macondo oil and dispersant. Various pathologies and abnormalities, ranging from color to posture, were also observed in these corals in comparison with reference sites further away from the well and the deepwater plume (White et al., 2012; Fisher et al., 2014). Oil and dispersant were also abundant in the sediments nearby the coral communities. The most damaged deepwater corals (impacts on more than 90% of the colony) were found to be beneath the path of the deep plume (White et al., 2012). It is reasonable to assume that colonies with spotty and confined damage may have been affected by marine snow deposition, instead.
A yearly monitoring program that included both impacted and non-impacted communities started under the GoMRI auspices in 2011 (Fisher et al., 2014; Girard and Fisher, 2018). While the median level of impact appeared to decrease between 2010 and 2012, damage remained severe (Hsing et al., 2013) and the recovery process slow. Branch loss in the corals was found to be significantly higher than normal 7 years after the spill, suggesting a long-term impact (Girard and Fisher, 2018). For the most heavily impacted sites, recovery is expected to require up to 30 years, to be increase to 50 when accounting for size loss (Girard and Fisher, 2018; Girard et al., 2018). The recovery has been shown the be aided by the ophiuroid associate Asteroschema clavigerum, that is likely contributing to the “cleaning” of the coral from particles rich of polycyclic aromatic hydrocarbons (PAH) deposited on branches (Girard et al., 2016).
Impacts on Fish
Direct Effects of Oil Exposure
Laboratory exposure studies with early-life stage fishes have been conducted only for species that live in the upper water column. They all concur that oil exposure causes a characteristic suite of morphological abnormalities including pericardial and yolk sac edema, impaired cardiac development, spinal curvature, and craniofacial malformations (Incardona et al., 2014; Edmunds et al., 2015; Esbaugh et al., 2016; Khursigara et al., 2017). These observations are generally referred to as a cardiotoxic phenotype (Figure 7), which has been shown to correlate with reduced early life survival and cardiac performance (Esbaugh et al., 2016; Khursigara et al., 2017), as well as reduced swim performance and maximum metabolic rate in individuals that survive to later life stages (Hicken et al., 2011; Incardona et al., 2015; Mager et al., 2014). Analogous effects were observed in later life stages exposed to oil, with the cardiotoxic effects shown through reduced stroke volume and cardiac output (Nelson et al., 2016; Cox et al., 2017; Nelson et al., 2017). Consequences include impaired swimming, maximum metabolic rate and aerobic scope (Stieglitz et al., 2016; Johansen and Esbaugh, 2017; Pan et al., 2018). This latter measure represents the total energy available to the animal for ecologically important activities, such as exercise and reproduction. It is speculated that these impacts are depth-independent and apply to fishes in mid- and deep waters as well. As such, sub-lethal oil exposure in later life stage fish has important ecological implications, which is further compounded by the fact that the impairments following acute exposure (24–48 h) can be observed at least 6 weeks later (Johansen and Esbaugh, 2017; Mauduit et al., 2019), and in some cases on much longer time scales (Zhang et al., 2017). In addition to the typical cardiotoxic impairments, work on the DWH oil spill has highlighted a suite of emerging toxicity endpoints in fish, including: impaired eye development (Xu et al., 2017) with subsequent reductions in visual acuity (Magnuson et al., 2018); reduced brain size (Xu et al., 2017), evidence for impaired neural function (Xu et al., 2017, 2019 and altered behavior (Johansen et al., 2017; Rowsey et al., 2019); evidence for mitochondrial dysfunction (Johansen and Esbaugh, 2019; Kirby et al., 2019; Xu et al., 2017, 2019); altered control of embryonic buoyancy (Pasparakis et al., 2017); impaired cholesterol biosynthesis (McGruer et al., 2019); impaired immune function (Bayha et al., 2017; Jones et al., 2017; Rodgers et al., 2018); and impaired hypothalamus-pituitary-interrenal axis function (Reddam et al., 2017).
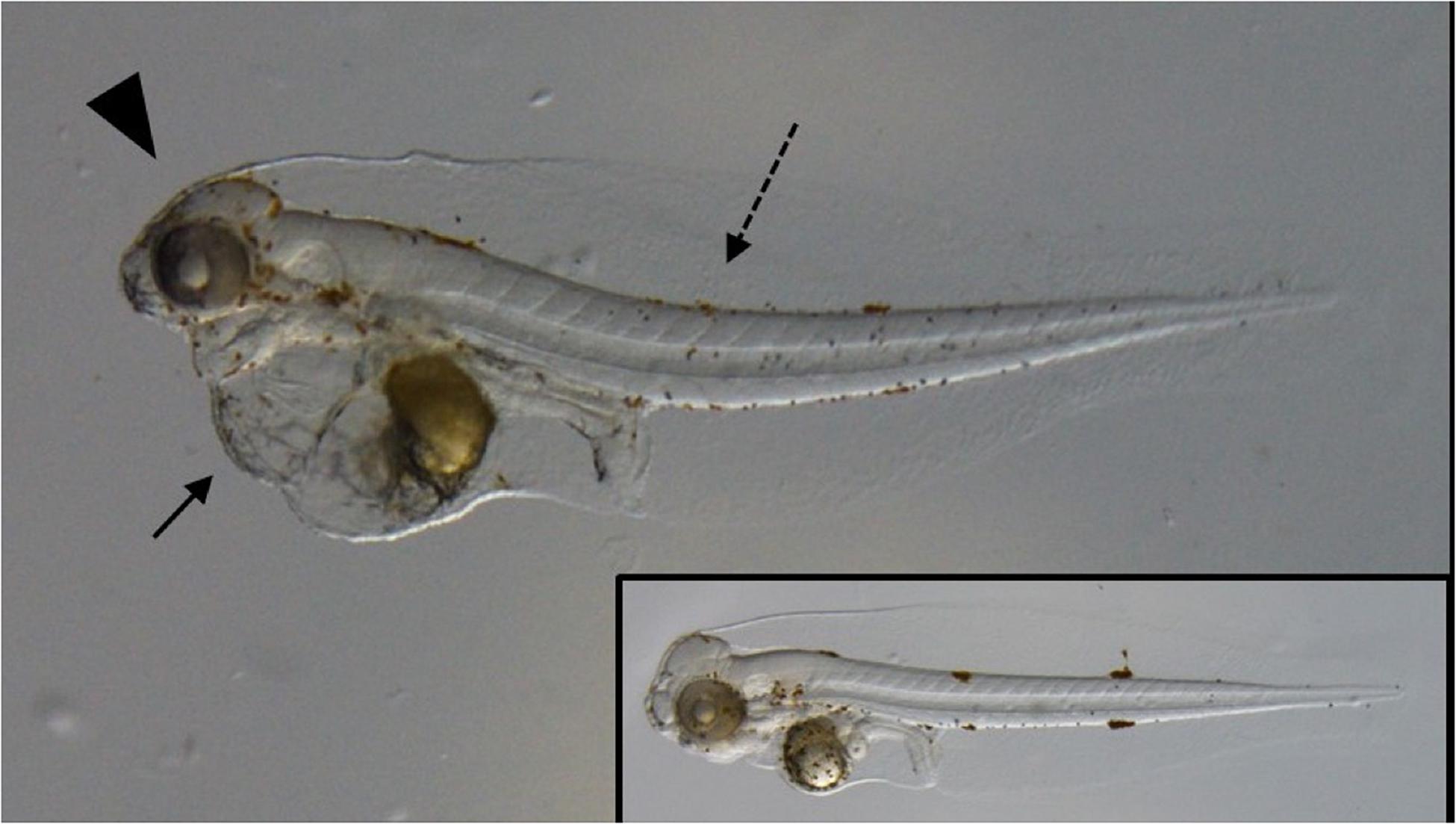
Figure 7. Representative image of a larval red drum displaying the typical effects of oil exposure on early life stage fishes. The image demonstrates the pericardial and yolk sac edema (arrow), spinal curvature (dashed arrow) and craniofacial malformations (arrowhead) that coincided with a 48 h exposure to 2.6 μg/L ΣPAH50 via a weathered oil water accommodated fraction. Insert shows control fish from the same experiment. Images courtesy of Khursigara et al. (2017).
Exposure in the Mesopelagic Zone
While the scope of oil-induced impairments on marine fish species are relatively well-known, the scale of toxicological impairments to species endemic to the mesopelagic zone (200–1000 m depth) is difficult to assess. This is largely because the majority of laboratory studies that define species sensitivity have been performed on coastal or epipelagic species, while the field studies that validate exposure have mostly focused on the epipelagic zones or demersal fishes. The best evidence of the extent of exposure for mesopelagic fishes comes from Romero et al. (2018) who described a significant increase in PAH metabolites in the muscle tissue of 7 species sampled that are endemic to the mesopelagic region of the Gulf of Mexico. Of particular note was the significant increase in the relative proportion of phenanthrene in the muscle PAH pool, as this PAH is thought to be a particularly strong driver of cardiotoxicity and limited embryonic survival (Incardona et al., 2004; Esbaugh et al., 2016; Brette et al., 2017). Time series sampling demonstrated that muscle PAHs were elevated in both 2010 and 2011 but had returned to baseline levels by 2015. Whether the exposures that resulted in these elevated muscle PAH concentrations were sufficient to induce sub-lethal toxicological effects remains an open question. The origin of the PAHs (marine oil snow versus deep plume) is also uncertain. These trends are generally consistent with the available information from demersal fishes, which have been more extensively studies through field collections beginning in 2011 (e.g., Snyder et al., 2015; Pulster et al., 2020). Species such as golden tilefish (Lopholatilus chamaeleonticeps) (Snyder et al., 2015) and a variety of grouper species (Pulster et al., 2020) sampled in the years immediately post-spill exhibited elevated biliary PAH metabolite concentrations as compared to pre-spill samples or those sampled from other regions of the Gulf of Mexico. Yet it important to note that other species, such as red snapper (Lutjanus campechanus) and king snake eel (Ophichtus rex), did not exhibit elevated biliary PAH metabolite concentrations when sampled from the same region over the same time frame (Snyder et al., 2015). This highlights the challenges of attempting to ascertain the breadth of species exposures, as these demersal species from the same general environment show different very patterns. This suggests that other unknown aspects of life history, ecology or physiology may affect the likelihood of species exposure.
The Deep Plume and the Fish Food Web
The route by which PAHs and other oil constituents enter the fish body is either through direct absorption across the gills, or via ingestion of contaminated prey and water. While both routes likely played a role in the aftermath of the Deepwater Horizon oil spill, the significance of ingestion via contaminated prey has been specifically highlighted in the context of the mesopelagic. Using carbon and nitrogen stable isotopes, Quintana-Rizzo et al. (2015) provided evidence of carbon intake from the oil spill in three mesopelagic fishes, which was attributed to dietary exposure. Similarly, Romero et al. (2018) demonstrated higher PAH concentrations in guts relative to the gills of exposed mesopelagic fishes, which they interpreted as evidence of dietary intake. However, this assertion should be viewed with caution owing to the high drinking rates of marine fish combined with the higher lipid content of intestinal tissue that would promote uptake of lipophilic PAHs in the gut. With respect to further trophic transfer, fish metabolize PAHs and other hydrocarbons through the aryl hydrocarbon receptor mediated pathway that ultimately leads to biotransformation and excretion of parent compounds. Yet bioaccumulation of PAHs will still occur when intake rates exceed biotransformation and excretion rates. PAH concentrations in muscle tissue of mesopelagic fishes sampled in the northern Gulf of Mexico were elevated in both 2010 and 2011, which would suggest that vertical migrations of predators (e.g., marine mammals and tuna species) and prey would result in trophic transfer of PAHs from the deepwater oil plume (Ryerson et al., 2012).
Impacts on Mammals
The deep waters of the GoM are inhabited by dispersed, highly mobile, oceanic populations of beaked whales, sperm whales, and a variety of delphinid species. Coastal waters and nearshore habitats are inhabited primarily by common bottlenose dolphins divided into many small, hyper-local stocks. Most of the research on DWH oil spill effects on marine mammals has focused on Bay, Sound and Estuary (BSE) and coastal bottlenose dolphins because they are far more easily observed than oceanic marine mammals. The degree to which oceanic GoM marine mammal individuals and populations were affected by the deep plume remains difficult to assess because potential pathways of exposure at depth are poorly understood, and odds of stranding are low for offshore species (Williams et al., 2011).
From February 1st, 2010 to July 31st, 2014, an “Unusual Mortality Event” (UME) was declared for marine mammals in the northern GoM. The UME began prior to the DWH oil spill, with common bottenose dolphin strandings triggered by cold temperatures and low coastal salinity (Venn-Watson et al., 2015). However, the largest increase in strandings occurred after May 2010, with over a thousand animals stranding, 86% of which were common bottlenose dolphins, likely from BSE, and coastal populations. It is widely accepted that the DWH oil spill was the underlying cause of the elevated stranding numbers following the spill. The low percentage of offshore species in the stranding record may reflect a low probability of carcasses reaching the shoreline (Williams et al., 2011).
The majority of known effects of oil exposure on marine mammals relate to surface interactions including contact with slicks, inhalation of fumes, and ingestion of oil with prey (Schwacke et al., 2013). These more observable types of exposure are linked to a range of effects including skin lesions, inhibited thermoregulation and death (Englehardt et al., 1977; Engelhardt, 1982). Surface observations following the DWH event and others suggest that marine mammals do not avoid oil in the environment (Wilkin et al., 2017), although they may be able to detect it (Geraci, 1990).
Data from a long-term passive acoustic monitoring program conducted from 2010 to 2016 in the GoM suggests continuous declines in Stenella dolphins and sperm whales at two monitored locations, one 10 km north of the wellhead, and the other 300 km west of the wellhead (Frasier et al., 2020), however pre-spill baselines do not exist, and the spatial extent over which these point observations apply is unknown. Beaked whale occurrence does not appear to have declined over the same period. It is not currently possible to discern whether observed trends reflect spatial population shifts or decreasing overall population sizes.
Differing dive depth distributions across marine mammal species dictate the likelihood of direct interactions with the deep plume. Sperm whales, beaked whales and short-finned pilot whales are known to conduct foraging dives to 1000 m or more (Tyack et al., 2006; Watwood et al., 2006; Aguilar Soto et al., 2008) in search of deep sea squid, therefore these species may have interacted directly with the deep plume. Potential impacts of exposure to subsurface oil at the concentrations associated with the deep plume are unknown. The remaining 13 or so common oceanic GoM marine mammal species include oceanic dolphins (Stenella spp., Risso’s dolphin, and rough-toothed dolphins, among others) and pygmy/dwarf sperm whales (Kogia spp.). These species typically dive to lesser depths (approximately 200–600 m) where they prey on midwater species, particularly myctophid fish and squid in the deep scattering layer (DSL). Dolphin species often forage at night when the DSL shoals to prey on surface plankton (Hopkins and Sutton, 1998). It is unlikely that oceanic dolphins directly interacted with the deep plume.
A likely pathway for deep plume impacts on mammals is via the food web, either through reduced prey quality and availability, or through exposure to oil-derived toxins accumulated in tissues of prey. Some evidence for deep food web disruption exists, including observations of elevated sedimentation rates associated with the DWH spill, which negatively affected deep sea coral communities and shifted microbial activity in sediments (Mason et al., 2014). Shifts in benthic foraminifera were also observed (Schwing et al., 2015). However, it remains unclear whether these benthic shifts lead to food web disruptions in the water column above. Kessler et al. (2011) reported a deep bloom of methanotrophic bacteria at depths associated with the deep plume, but, again, implications for the broader food web remain undetermined.
Changes in isotopic signatures and PAH content of mesopelagic fish indicate assimilation of oil-contaminated prey (Quintana-Rizzo et al., 2015; Romero et al., 2018), but since the mesopelagic community typically feeds in the epipelagic zone, the source of may have been surface oil rather than plume-derived oil. Cephalopods and fish, may bioaccumulate PAHs (Lacoue-Labarthe et al., 2016, Romero et al., 2018) creating a pathway for continued exposure for top predators. It is worth noting that Belugas exposed to PAHs in the St. Lawrence estuary (Quebec, Canada) were found to have high rates of cancer (27% of adults found dead) relative to marine mammal populations elsewhere (0.5% average for wild bottlenose dolphins at the time) (Martineau et al., 2002).
Open Questions
Many open questions remain in understanding formation, evolution, fate and impacts of the deepwater plume, with impacts being the least quantified and constrained. Here we summarize them in the context of physical processes and their representation in circulation models, oil spill modeling, biogeochemical and microbial processes, and repercussions on higher trophic levels.
Physics and Circulation Models
The dynamics governing the physical evolution of stratified rotating flows over complex topography represent an especially difficult problem. In general, existing literature that seeks a theoretical underpinning and robust model parameterizations deal with each class of forcing mechanisms independently, and typically using highly idealized models. For example, there is a wealth of knowledge within the atmospheric science community concerning stratified flows over topography. Such studies are usually performed for isolated topography (with length scale L) in a non-rotating limit, specifically U/fL >> 1, where U is the typical lateral flow velocity and f the Coriolis parameter. Extrapolating from this literature is hazardous to the following reasons:
• The oceanic paradigm is one in which topography is not isolated (the north western GoM provides a perfect example) and the scales of topography are such that rotation cannot be neglected [U/fL ∼ O(1)]; with rotation, the linear kinematics that link wave steepness to energetics are fundamentally altered.
• The presence of a large-scale slope alters the kinematics of the linear problem so that the lowest frequency internal wave has a wavenumber that points upslope (Thorpe, 1992; see Figure 2) rather than being parallel to the roughness wavenumber. This fundamental change in the steady linear solution modifies the blocking criteria in uncertain ways.
• Filtering of topography (commonly done in circulation models with a vertical and a horizontal discretization) is problematic because the bottom boundary condition that flow has to be parallel to the boundary is non-linear in topographic height. A simple filtering of the topography is implicitly equivalent to a linearization.
Similarly, within the third class (turbulent stresses associated with the viscous condition of no-flow at the bottom boundary), there is an extensive literature of highly idealized models of boundary layer structure for steady and oscillatory flows over a smooth inclined plane (Brink and Lentz, 2010a,b; Benthuysen and Thomas, 2012; Umlauf et al., 2015; Ruan and Thompson, 2016). A key parameter in such problems is the slope Berger number, α, where α represents the departure from the horizontal of an inclined plane, N is the Brunt–Väisälä frequency describing the flow stratification and f is the Coriolis parameter. Whenever the flow is oriented in the direction of Kelvin wave propagation, the steady solution to this problem (aka the arrested Ekman layer) consists of a weakly stratified near-boundary region with horizontal buoyancy gradients in thermal wind balance. If the stratification anomaly is large and if α is of order O(1), the Ertel potential vorticity can easily reverse sign at the bottom boundary. In a free shear flow, such sign reversals would indicate an unconditionally unstable conditions (Hoskins, 1974). Slope Burger numbers of order 1 are characteristic of significant portions of the continental slope in the northern GoM. Overall, the presence of roughness imprints its scales on the vertical velocity of a growing instability, and it remains unclear whether small amplitude bumps would enhance or limit instability growth. Additionally, the ocean, rather than resembling the paradigm of linear instability where perturbations grow from a white noise initial condition, may be closer to an externally forced system at finite amplitude. This narrative is suggested by sparse observations revealing that the response to quasi-stationary flow over rough topography may not be quasi-stationary: moored acoustic Doppler current profiler (ADCP) document that the vertical shear variance is modulated on eddy time scales but shear appears at near- and super-inertial Eulerian frequencies rather than being a quasi-stationary lee wave response (Brearley et al., 2013; Clément et al., 2016). Whether this time-dependence relates to the initial transients in the one-dimensional representation of Figure 3B or to submesoscale instabilities is unclear.
Submesoscale permitting simulations (Bracco et al., 2016, 2018) include both topography and friction, but convergence is not clearly achieved. In the vertical, increased model resolution is required to accurately represent the turbulent bottom boundary layer; in the horizontal, increased model resolution is required to accurately represent instabilities at the submesoscales. Further resolution increases, however, entail the adoption of much less cost-efficient non-hydrostatic models to insure an accurate representation of the vertical velocity field, especially for flows over complex bathymetries, such as in the GoM.
However, improving the prognostic capability of physical models for future deep spill releases may not require a major rise in computer processing power. The crux of the issue is that processes responsible for tracer dispersion are both spatially inhomogeneous and temporally intermittent, but much of the spatial inhomogeneity may be predictable for a given ocean “state.” For example, locations of flow separation and hydraulic transitions, where intense diapycnal mixing occurs, may depend upon model configuration and resolution, but are not random. The leading candidates for “state variables” are the time dependent near-bottom speed and direction. As a first step we advocate for creating maps of dispersion and boundary ventilation processes for given ocean state variables wherever spills could potentially occur, and then focus on the fidelity of regional models in predicting the required variables. This recommendation points to a key research gap articulated also in the report on the “Future of the U.S. Gulf Coast” by the National Academies of Sciences, Engineering, and Medicine (2018).
Additionally, spatial patterns of high-resolution nested models should undergo a ground truth verification against observations. In this regard, transport across isopycnals (diapycnal mixing) and along isopycnals (stirring) should be validated separately. The former (mixing) is likely quite sensitive to both resolved vertical velocities and sub-grid closures, and is highly intermittent in time, whereas the latter (stirring) depends upon the small-scale vorticity structures evidenced in Figure 4, and has a longer (days to week) persistence time scale. Both mixing and stirring act on a tracer whenever this is stirred into and out of intense mixing regions near the boundaries. Distinguishing between their relative contribution is a realistic objective as diagnostics to separate internal wave from vortical fields in quality fine-scale measurements already exist (e.g., Polzin et al., 2003).
Finally, substantial progress could be achieved with direct estimates of fluxes, and in particular of the spatial distribution of time varying form drag, as well as fluxes of density and momentum along the continental slope. This objective may be less onerous than it seems. Having a direct estimate of bed stress resulting from turbulence (Perlin et al., 2005) and estimates of reduced mixing efficiency with decreasing height above the boundary (Holleman et al., 2016) present indeed technical issues, but our ignorance largely lies within the internal wave and submesoscale frequency bands, and centers upon the question of how rotation alters the behavior of the system. These frequencies and question could be tackled with a focused numerical modeling effort, clarifying the possible coupling between class 2 and class 3 mechanisms.
Taken together, the efforts above would support the development of metrics concerning the spatial resolution of numerical efforts and may lead to a better understanding of when physical circulation models can be considered “good enough” for spill prediction purposes.
Oil Spill Modeling
To improve predictions of deep plume formation, a first requirement is to better refine the initial DSD estimations under different environmental and response scenarios. The droplet size distribution defines the sizes and the mass volumes range of the droplets, and is one of the most important characteristics to model the fate of uncontrolled deep-sea oil spills. It explains how much oil rises to the surface and helps determining the first response. DSD depends on the flow rate and the turbulent kinetic energy of the blowout, which ultimately depends on the riser-pipe diameter, the gas to oil ratio (GOR), and the pressure difference between the reservoir and the BOP (blowout preventer). Indeed, as gas suddenly expands with the pressure drop, the turbulent kinetic energy generated is high enough to atomize the oil in micro-droplets. When the oil is naturally dispersed by these thermodynamic processes, subsea dispersant injection (SSDI) which typically decreases the mean droplet size diameter, becomes largely ineffective since droplets are already small and entrained in a later plume (Paris et al., 2018). The outcome of such refinement needs then to be incorporated into models. Another process needing further investigation is internal degassing, which is the expansion of the gas in rising multiphase gas-saturated droplets (Pesch et al., 2018). This process, that depends on the pressure drop between the reservoir and the BOP (Griffiths, 2012) and the decrease of hypostatic pressure, changes the initial droplet size distribution, the plume buoyancy in the near-field and the rising speed of oil to the surface in the far-field (Pesch et al., 2018, 2020).
Despite the noted importance of making output of prediction models available to the public and wide academic community, only few groups currently have their 3D vertical concentrations of oil available on GRIID-C (Dissanayake et al., 2018; Paris et al., 2020; Perlin et al., 2020). Such models have been used for estimation of ecosystem impacts at different trophic levels (Ainsworth et al., 2018), and for the evaluation of fisheries closures (Berenshtein et al., 2020), but comparative work so far has been limited (Socolofsky et al., 2015) and more is required.
Fate: Microbial/Biogeochemistry
The fate of methane and oil in the deepwater plume can only be constrained using proxy data (Joye et al., 2011; Kessler et al., 2011) since rates of oil oxidation were not determined (Joye et al., 2014) and data documenting rates of methane oxidation were available for only limited periods of time (Crespo-Medina et al., 2014). While some authors suggested very sluggish oil oxidation rates (Camilli et al., 2010), others suggested very rapid oil oxidation rates (Hazen et al., 2010). Neither Hazen et al. (2010) or Camilli et al. (2010), used sensitive radiotracer methods to determine oil oxidation rates; in fact, no rates of oil degradation were determined utilizing radiotracers during the incident, making it near impossible to closely constrain rates of oil biodegradation.
A substantial debate arose regarding the fate of low molecular weight alkanes – namely methane – after the Macondo blowout (see for example Joye et al., 2014). While Valentine et al. (2010) and Kessler et al. (2011) suggested sluggish methanotrophy until the fall of 2010, Rivers et al. (2013) and Crespo-Medina et al. (2014) provide compelling support based on direct rate measurements of methane oxidation (May and June 2010) and metatranscriptomic evidence (May 2010) for extremely active methanotrophy early in the discharge when plume methane concentrations were hundreds of micromolar (Joye et al., 2011). The Rivers et al., Crespo-Medina et al., and Joye et al., papers together argue convincingly that methane oxidation peaked early in the spill and provide alternative explanations for the presence of methylotrophs in August 2010 (McCarren et al., 2010; Joye et al., 2014).
While some debate occurred initially over the temporal trajectory of methane oxidation activity (Valentine et al., 2010; Kessler et al., 2011; Crespo-Medina et al., 2014), in hindsight, the data were consistent with a dynamic time-dependent evolution of the methanotroph community. Crespo-Medina et al. (2014) documented increasing rates of methane oxidation in response to the hydrocarbon infusion beginning in early May 2010 with peak activity observed in late May 2010, after which rates decreased substantially to a low point in August 2010. Similarly, lower rates were observed by Valentine et al. (2010) and Kessler et al. (2011) in June and September 2010, respectively. Genomic data shows that both methanotrophs and oil degraders in the deepwater plume responded rapidly to the enrichment of hydrocarbons (Mason et al., 2012), consuming oxygen as they degraded oil and gas (Du and Kessler, 2012). The activity of methanotrophs appeared to be limited by either physiological and/or environmental factors after mid-June 2010, raising the possibility that methane may not have been consumed completely (Crespo-Medina et al., 2014). Du and Kessler (2012) observed that oxygen consumption in the deepwater plume had ceased by September 2010, suggesting that both methane and oil degradation rates had slowed substantially by that time. Whether the remainder of oil and gas were consumed in deepwaters remains unknown; Joye (2015) suggested that between 40 and 60% of the hydrocarbons were consumed while only 45–75% of the hydrocarbons could be accounted for in the overall oil budget.
The deepwater plume microbial response was impressive: oil and methane degrading taxa bloomed quickly and consumed a substantial amount of the discharged hydrocarbons. However, in the event of another deepwater blowout, it is imperative that rates of hydrocarbon oxidation be determined robustly across an appropriate spatial grid at high temporal resolution. Given the role of physical mixing in distributing hydrocarbons and biomass across the Gulf system, coordinating collection of biogeochemical, microbiological, and physical oceanographic data is necessary to fully describe and understand plume microbiological dynamics.
Impacts on Higher Trophic Levels
Effects of the deep plume on higher trophic levels remain unknown and unquantified. Deep oceanic food webs are poorly understood (Fisher et al., 2016), therefore potential pathways linking subsurface oil with offshore marine megafauna are largely hypothetical. On one hand, we need more frequent, higher precision estimates of megafauna population sizes in areas of concern to confidently assess whether and to what degree impacts may have occurred. On the other, we need minimally invasive methods for tracing pathways of indirect exposure, such as biological tracers and physiological indicators that could be measured from biopsies. Knowledge of impacts and long-term effects of plume exposure on prey populations, in particular on little-known deepwater squid, could fill in missing trophic links.
Identified Research Priorities
In the past 10 years the research community had an extraordinary opportunity to observe and study the northern continental slope of the Gulf of Mexico, its circulation and functionality. GoMRI funding and structure has allowed an integrated approach relying on both observations and modeling of the deep ocean, which is often under-sampled due to intrinsic logistical challenges and costs, and is overlooked by the modeling community. The sustained observations that many consortia were able to undertake, coupled with the urgency of predicting oil dispersion pathways, fate and impacts, fostered a step-function improvement in both understanding and modeling. While progress has been impressive and we have a much more integrated, holistic understanding of the physical controls on, and of the biological responses to, oil and gas across the coupled benthic – pelagic ecosystems, many open questions and research needs remain.
In Table 1, we outline few research priorities that could be tackled in the next decade or less to improve the ability to predict the fate of hydrocarbons and their impacts to the deep ocean another deepwater spill had to occur, whenever in the GoM or elsewhere. To address any of them, first and foremost a long-term observing system has to be sustained in the Gulf of Mexico or any region subject to deepwater drilling.
Summary
We have reviewed theoretical, observational and modeling advances related to the deepwater hydrocarbon plume that formed and evolved along the continental slope of the northern Gulf of Mexico following the Deepwater Horizon oil spill. We focused on the physical oceanography and oil modeling aspects and delved succinctly on the its fate and impacts on the Gulf’s ecosystem, from microbes to mammals.
Over the past decade, advances in understanding transport and mixing in the Gulf have been remarkable, but improvements have been significantly greater at the ocean surface, where multiple satellite products are available, than at depth, where observations remain limited and more expensive, and models can be more rarely validated. Notwithstanding these intrinsic limitations, we have acquired a much greater appreciation of the role that multiscale fluid movements at and near the bottom boundary layer and oil degradation processes play over a range temporal scales, from minutes to years.
Toward the end of this work, we outlined a number of remaining open questions that, if answered, will strengthen the scientific community ability to predict the movement of water and hydrocarbons, their impacts and the microbial response, in the event of another offshore deepwater spill, in the Gulf of Mexico or elsewhere. These efforts, if undertaken, will have broad, long-lasting ramification. Better physical and hydrocarbon models for continental margins have far-ranging applications from climate science to the prediction of the influence of methane seepage and gas hydrate systems, and from biogeochemical cycling on Earth and Icy Moons to the investigation of the origin of life.
Author Contributions
AB and CP coordinated the effort. All the authors contributed to the writing, read and approved the submitted version.
Funding
This review was made possible by funding from the Gulf of Mexico Research Initiative (GoMRI) and is a product of the Core Area 1 Synthesis workshop. The authors have contributed research on the Gulf deep circulation and the deep plume through GoMRI-funded consortia (ECOGIG for AB, SJ and GL, C-IMAGE for CP, AV and KF, and RECOVER for AE) and one of the RFP-5 grant (KP). KP was partially supported also by NSF OCE-1536779.
Conflict of Interest
The authors declare that the research was conducted in the absence of any commercial or financial relationships that could be construed as a potential conflict of interest.
Acknowledgments
We are grateful Dr. Natalie Perlin (UM) for providing post-processing codes for the completion of Figure 5 (deep plume figure), and to the three reviewers for their insightful comments and careful reading of the initial manuscript.
References
Adcroft, A., Hallberg, R., Dunne, J. P., Samuels, B. L., Galt, J. A., Barker, C. H., et al. (2010). Simulations of underwater plumes of dissolved oil in the Gulf of Mexico. Geophys. Res. Lett. 37:L18605. doi: 10.1029/2010GL044689
Aguilar Soto, N., Johnson, M. P., Madsen, P. T., Díaz, F., Domínguez, I., Brito, A., et al. (2008). Cheetahs of the deep sea: deep foraging sprints in short-finned pilot whales off Tenerife (Canary Islands). J. Anim. Ecol. 77, 936–947. doi: 10.1111/j.1365-2656.2008.01393.x
Ainsworth, C., Paris, C. B., Perlin, N., Dornberger, L., Patterson, W., Chancellor, E., et al. (2018). Impacts of the Deepwater Horizon oil spill evaluated using an end-to-end ecosystem model. PLoS One 13:e0190840. doi: 10.1371/journal.pone.0190840
Aliseda, A., Bommer, P., Espina, P., Flores, O., Lasheras, J. C., Lehr, B., et al. (2010). Deepwater Horizon release estimate of rate by PIV. Report to the Flow Rate Technical Group. Available online at: https://www.doi.gov/sites/doi.gov/files/migrated/deepwaterhorizon/upload/Deepwater_Horizon_Plume_Team_Final_Report_7-21-2010_comp-corrected2.pdf (accessed August 31, 2020).
Aman, Z., Paris, C. B., May, E. F., Johns, M. L., and Lindo-Atichati, D. (2015). High-Pressure visual experimental studies of oil-in-water dispersion droplet size: implication for oil transport. Chem. Eng. Sci. 127, 392–400. doi: 10.1016/j.ces.2015.01.058
ASCE (1996). State of the art review of modeling transport and fate of oil spills but the task committee on modeling of oil spills of the water resources engineering division, american society of civil engineers (ASCE). J. Hydraul. Eng. 122, 594–609. doi: 10.1061/(asce)0733-9429(1996)122:11(594)
Baines, P. G. (1995). Topographic Effects in Stratified Flows. Cambridge: Cambridge University Press.
Bandara, U. C., and Yapa, P. D. (2011). Bubble Sizes, Breakup, and Coalescence in Deepwater Gas/Oil Plumes. J. Hydr. Eng. 137, 729–738. doi: 10.1061/(ASCE)HY.1943-7900.0000380
Bayha, K. M., Ortell, N., Ryan, C. N., Griffitt, K. J., Krasnec, M., Sena, J., et al. (2017). Crude oil impairs immune function and increases susceptibility to pathogenic bacteria in southern flounder. PLoS One 12:e0176559. doi: 10.1371/journal.pone.0176559
Beegle-Krause, C. J., and Lynch, W. (2005). Combining modeling with response in potential deep well blowout: lessons learned from Thunder Horse. Int. Oil Spill Conf. Proc. 2005, 719–723. doi: 10.7901/2169-3358-2005-1-719
Benthuysen, J., and Thomas, L. N. (2012). Friction and diapycnal mixing at a slope: boundary control of potential vorticity. J. Phys. Oceanogr. 42, 1509–1523. doi: 10.1175/JPO-D-11-0130.1
Berenshtein, I., Paris, C. B., Perlin, N., Alloy, M., Murawski, S., and Joye, S. (2020). Invisible oil beyond the Deepwater Horizon satellite footprint. Sci. Adv. 6:eaaw8863. doi: 10.1126/sciadv.aaw8863
Boxall, J. A., Koh, C. A., Sloan, E. D., Sum, A. K., and Wu, D. T. (2012). Droplet size scaling of water-in-oil emulsions under turbulent flow. Langmuir 28, 104–110. doi: 10.1021/la202293t
Bp Gulf Science Data1 (2016). Chemical analysis of oil samples from the Gulf of Mexico and adjoining states from May 2010 to March 2014. Distributed by: Gulf of Mexico Research Initiative Information and Data Cooperative (GRIIDC), Harte Research Institute, Texas A&M University-Corpus Christi. doi: 10.7266/N7902251
Bracco, A., Choi, J., Joshi, K., Luo, H., and McWilliams, J. C. (2016). Submesoscale currents in the northern Gulf of Mexico: deep phenomena and dispersion over the continentalslope. Ocean Modell. 101, 43–58. doi: 10.1016/j.ocemod.2016.03.002
Bracco, A., Choi, J., Kurian, J., and Chang, P. (2018). Vertical and horizontal resolution dependency in the model representation of tracer dispersion in the northern Gulf ofMexico. Ocean Modell. 122, 13–25. doi: 10.1016/j.ocemod.2017.12.008
Bracco, A., Liu, G., and Sun, D. (2019). Mesoscale-submesoscale interactions in the Gulf of Mexico: from oil dispersion to climate. Chaos Solitons Fractals 119, 63–72. doi: 10.1016/j.chaos.2018.12.012
Brearley, J. A., Sheen, K. L., Naveira Garabato, A. C., Smeed, D. A., and Waterman, S. (2013). Eddy-induced modulation of turbulent dissipation over rough topography in the Southern Ocean. J. Phys. Ocean. 43, 2288–2308. doi: 10.1175/JPO-D-12-0222.1
Bretherton, F. P. (1969). Momentum transport by gravity waves. Q. J. R. Met. Soc. 95, 213–243. doi: 10.1002/qj.49709540402
Brette, F., Shiels, H. A., Galli, G. L., Cros, C., Incardona, J. P., Scholz, N. L., et al. (2017). A novel cardiotoxic mechanism for a pervasive global pollutant. Sci. Rep. 7:41476.
Brink, K. H., and Lentz, S. J. (2010a). Buoyancy arrest and bottom Ekman transport: part I. Steady flow. J. Phys. Oceanogr. 40, 621–635. doi: 10.1175/2009jpo4266.1
Brink, K. H., and Lentz, S. J. (2010b). Buoyancy arrest and bottom Ekman transport: part II. Oscillating flow. J. Phys. Oceanogr. 40, 636–655. doi: 10.1175/2009jpo4267.1
Camilli, R., Di Iorio, D., Bowen, A., Reddy, C. M., Techet, A. H., Yoerger, D. R., et al. (2012). Acoustic measurement of the Deepwater Horizon Macondo well flow rate. Proc. Natl. Acad. Sci. U.S.A. 109, 20235–20239. doi: 10.1073/pnas.1100385108
Camilli, R., Reddy, C. M., Yoerger, D. R., Van Mooy, B. A. S., Jakuba, M. V., Kinsey, J. C., et al. (2010). Tracking hydrocarbon plume transport and biodegradation at Deepwater Horizon. Science 330, 201–204. doi: 10.1126/science.1195223
Chalamalla, V. K., Gayen, B., Scotti, A., and Sarkar, S. (2013). Turbulence during the reflection of internal gravity waves at critical and near-critical slopes. J. Fluid Mech. 729, 47–68. doi: 10.1017/jfm.2013.240
Chen, B., Huang, J., Yuan, K., Lin, L., Wang, X., Yang, L., et al. (2016). Direct evidences on bacterial growth pattern regulating pyrene degradation pathway and genotypic dioxygenase expression. Mar. Pollut. Bull. 105, 73–80. doi: 10.1016/j.marpolbul.2016.02.054
Chen, F., and Yapa, P. D. (2003). A model for simulating deep water oil and gas blowouts - Part II: comparison of numerical simulations with “Deepspill” field experiments”. J. Hydr. Res. 41, 353–365. doi: 10.1080/00221680309499981
Chen, F., and Yapa, P. D. (2007). Estimating the oil droplet size distributions in deepwater oil spills. J. Hydr. Eng. 133, 197–207. doi: 10.1061/(ASCE)0733-94292007133:2(197)
Clément, L., Frajka-Williams, E., Sheen, K. L., Brearley, J. A., and Naveira Garabato, A. C. (2016). Generation of internal waves by eddies impinging on the western boundary of the North Atlantic. J. Phys. Ocean. 46, 1067–1079. doi: 10.1175/JPO-D-14-0241.1
Clift, R., Grace, J., and Weber, W. E. (1978). Bubbles, Drops, and Particles. Mineola, NY: Dover Publications Inc.
Cox, G. K., Crossley, D. A. II, Stieglitz, J. D., Heuer, R. M., Benetti, D. D., Grosell, M., et al. (2017). Oil exposure impairs in situ cardiac function in response to beta-adrenergic stimulation in Cobia (Rachycentron canadum). Environ. Sci. Technol. 51, 14390–14396. doi: 10.1021/acs.est.7b03820
Crespo-Medina, M., Meile, C. D., Hunter, K. S., Diercks, A.-R., Asper, V. L., Orphan, V. J., et al. (2014). The rise and fall of a methanotrophy following a deepwater oil-well blowout. Nat. Geosci. 7, 423–427. doi: 10.1038/ngeo2156
Crone, T. J., and Tolstoy, M. (2010). Magnitude of the 2010 Gulf of Mexico Oil Leak. Science 330, 634–634. doi: 10.1126/science.1195840
Daly, K. L., Passow, U., Chanton, J., and Hollander, D. (2016). Assessing the impacts of oil-associated marine snow formation and sedimentation during and after the Deepwater Horizon oil spill. Anthropocene 13, 18–33. doi: 10.1016/j.ancene.2016.01.006
Deepwater Horizon Natural Resource Damage Assessment Trustees (2016). Deepwater Horizon Oil Spill: Final Programmatic Damage Assessment and Restoration Plan and Final Programmatic Environmental Impact Statement. Available online at: http://www.gulfspillrestoration.noaa.gov/restoration-planning/gulf-plan (accessed August 31, 2020).
Deepwater Horizon Oil Spill Principal Investigator Workshop (2012). Deepwater Horizon Oil Spill Principal Investigator Workshop: Final Report. Washington, DC: National Science and Technology Council.
Diercks, A. R., Highsmith, R. C., Asper, V. L., Joung, D., Zhou, Z., Guo, L., et al. (2010). Characterization of subsurface polycyclic aromatic hydrocarbons at the deepwater horizon site. Geophys. Res. Lett. 37:L20602. doi: 10.1029/2010GL045046
Dissanayake, A. L., Gros, J., and Socolofsky, S. A. (2018). Integral models for bubble, droplet, and multiphase plume dynamics in stratification and crossflow. Environ. Fluid Mechanics 18, 1167–1202. doi: 10.1007/s10652-018-9591-y
Drozd, G. T., Worton, D. R., Aeppli, C., Reddy, C. M., Zhang, H., Variano, E., et al. (2015). Modeling comprehensive chemical composition of weathered oil following a marine spill to predict ozone and potential secondary aerosol formation and constrain transport pathways. J. Geophys. Res. Oceans 120, 7300–7315. doi: 10.1002/2015jc011093
Du, M., and Kessler, J. D. (2012). Assessment of the spatial and temporal variability of bulk hydrocarbon respiration following the deepwater horizon oil spill. Environ. Sci. Technol. 46, 10499–10507. doi: 10.1021/es301363k
Dubinsky, E. A., Conrad, M. E., Chakraborty, R., Bill, M., Borglin, S. E., Hollibaugh, J. T., et al. (2013). Succession of hydrocarbon-degrading bacteria in the aftermath of the deepwater horizon oil spill in the Gulf of Mexico. Environ. Sci. Technol. 47, 10860–10867.
Dutta, N. C. (1997). Pressure prediction from seismic data: implications for seal distribution and hydrocarbon exploration and exploitation in the deepwater Gulf of Mexico. Norwegian Petrol. Soc. Spec. Publ. 7, 187–199. doi: 10.1016/S0928-8937(97)80016-1
Edmunds, R. C., Gill, J. A., Baldwin, D. H., Linbo, T. L., French, B. L., Brown, T. L., et al. (2015). Corresponding morphological and molecular indicators of crude oil toxicity to the developing hearts of mahi mahi. Sci. Rep. 5:17326.
Engelhardt, F. R. (1982). Hydrocarbon metabolism and cortisol balance in oil-exposed ringed seals, Phoca hispida. Comp. Biochem. Physiol. C Comp. Pharmacol. 72, 133–136. doi: 10.1016/0306-4492(82)90219-2
Englehardt, F. R., Geraci, J. R., and Smith, T. G. (1977). Uptake and clearance of petroleum hydrocarbons in the ringed seal (Phoca hispida). J. Fish. Board Can. 34, 1143–1147. doi: 10.1139/f77-171
Eriksen, C. C. (1985). Implications of ocean bottom reflection for internal wave spectra and mixing. J. Phys. Oceanogr. 15, 1145–1156. doi: 10.1175/1520-0485(1985)015<1145:ioobrf>2.0.co;2
Esbaugh, A. J., Mager, E. M., Stieglitz, J. D., Hoenig, R., Brown, T. L., French, B. L., et al. (2016). The effects of weathering and chemical dispersion on Deepwater Horizon crude oil toxicity to mahi-mahi (Coryphaena hippurus) early life stages. Sci. Total Environ. 543, 644–651. doi: 10.1016/j.scitotenv.2015.11.068
Fisher, C. R., Demopoulos, A. W. J., Cordes, E. E., Baums, I. B., White, H. K., and Bourque, J. R. (2014). Coral communities as indicators of ecosystem-level impacts of the deepwater horizon spill. BioScience 64, 796–807. doi: 10.1093/biosci/biu129
Fisher, C. R., Montagna, P. A., and Sutton, T. T. (2016). How did the Deepwater Horizon oil spill impact deep-sea ecosystems? Oceanography 29, 182–195. doi: 10.5670/oceanog.2016.82
Fraga, B., Stoesser, T., Lai, C. C. K., and Socolofsky, S. A. (2016). A LES-based eulerian-lagrangian approach to predict the dynamics of bubble plumes. Ocean Modell. 97, 27–36. doi: 10.1016/j.ocemod.2015.11.005
Frasier, K. E., Solsona-Berga, A., Stokes, L., and Hildebrand, J. A. (2020). “Impacts of the deepwater horizon oil spill on marine mammals and sea turtles,” in Deep Oil Spills, eds S. Gilbert, M. Schlüter, and S. A. Murawski (Cham: Springer), 431–462. doi: 10.1007/978-3-030-11605-7_26
French-McCay, D., Li, Z., Horn, M., Crowley, D., Spaulding, M. L., Mendelsohn, D., et al. (2016). “Modeling oil fate and subsurface exposure concentrations from the deepwater horizon Oil Spill,” in Proceedings of the Thirty-ninth AMOP Technical Seminar, Environment and Climate Change Canada, Ottawa, ON, 115–150.
French-McCay, D. P. (2004). Oil spill impact modeling: development and validation. Environ. Toxicol. Chem. 23, 2441–2456. doi: 10.1897/03-382
French-McCay, D. P., Jayko, K., Li, Z., Horn, M., Kim, Y., Isaji, T., et al. (2015). Technical Reports for Deepwater Horizon Water Column Injury Assessment – WC_TR14: Modeling Oil Fate and Exposure Concentrations in the Deepwater Plume and Cone of Rising Oil Resulting from the Deepwater Horizon Oil Spill. DWH NRDA Water Column Technical Working Group Report. South Kingstown, RI: RPS ASA.
Garrett, C. (1979). Mixing in the ocean interior. Dyn. Atmos. Oceans 3, 239–265. doi: 10.1016/0377-0265(79)90011-3
Garrett, C. (2001). “Internal waves,” in Encyclopedia of Ocean Sciences, eds J. Steele, S. Thorpe, and K. Turekian (Cambridge, MA: Academic Press), 1335–1342.
Garrett, C., and Gilbert, D. (1988). “Estimates of vertical mixing by internal waves reflected off a sloping bottom,” in Small-Scale Turbulence and Mixing in the Ocean, Proceedings of the 19th International Liege Colloquium on Ocean Hydrodynamics, eds J. C. J. Nihoul and B. M. Jamart (New York: Elsevier), 405–424. doi: 10.1016/s0422-9894(08)70561-0
Garrett, C., MacCready, P., and Rhines, P. (1993). Boundary mixing and arrested ekman layers: rotating stratified flow near a sloping boundary. Annu. Rev. Fluid Mech. 25, 291–323. doi: 10.1146/annurev.fl.25.010193.001451
Geraci, J. R. (1990). “Physiologic and toxic effects on cetaceans,” in Sea Mammals and Oil: Confronting the Risks, eds J. R. Geraci and D. J. St. Aubin (San Diego, CA: Academic Press), 167–192. doi: 10.1016/b978-0-12-280600-1.50011-8
Girard, F., and Fisher, C. R. (2018). Long-term impact of the Deepwater Horizon oil spill on deep-sea corals detected after seven years of monitoring. Biol. Conserv. 225, 117–127. doi: 10.1016/j.biocon.2018.06.028
Girard, F., Fu, B., and Fisher, C. R. (2016). Mutualistic symbiosis with ophiuroids limited the impact of the Deepwater Horizon oil spill on deep-sea octocorals. Mar. Ecol. Prog. Ser. 549, 89–98. doi: 10.3354/meps11697
Girard, F., Shea, K., and Fisher, C. R. (2018). Projecting the recovery of a long-lived deep-sea coral species after the Deepwater Horizon oil spill using state-structured models. J. Appl. Ecol. 55, 1812–1822. doi: 10.1111/1365-2664.13141
Grasshoff, M. (1977). Die Gorgonarien des östlichen Nordatlantik und des Mittelmeeres III. Die Familie Paramuriceidae (Cnidaria, Anthozoa). “Meteor”. Forsch Ergeb Sec. D 27, 5–76.
Griffiths, S. K. (2012). Oil release from macondo well MC252 following the deepwater horizon accident. Environ. Sci. Technol. 46, 5616–5622. doi: 10.1021/es204569t
Gros, J., Reddy, C. M., Nelson, R. K., Socolofsky, S. A., and Arey, J. S. (2016). Simulating gas-liquid-water partitioning and fluid properties of petroleum under pressure: implications for deep-sea blowouts. Environ. Sci. Technol. 50, 7397–7408. doi: 10.1021/acs.est.5b04617
Gros, J., Socolofsky, S. A., Dissanayake, A. L., Jun, I., Zhao, L., Boufadel, M. C., et al. (2017). Petroleum dynamics in the sea and influence of subsea dispersant injection during Deepwater Horizon. Proc. Nat. Acad. Sci. U.S.A. 114, 10065–10070. doi: 10.1073/pnas.1612518114
Gutierrez, T., Singleton, D. R., Berry, D., Yang, T., Aitken, M. D., and Teske, A. (2013). Hydrocarbon-degrading bacteria enriched by the Deepwater Horizon oil spill identified by cultivation and DNA-SIP. ISME J. 7, 2091–2104. doi: 10.1038/ismej.2013.98
Harvey, G. R., Requejo, A. G., McGillivary, P. A., and Tokar, J. M. (1979). Observation of a subsurface oil-rich layer in the open ocean. Science 205, 999–1001. doi: 10.1126/science.205.4410.999
Hazen, T. C., Dubinsky, E. A., DeSantis, T. Z., Andersen, G. L., Piceno, Y. M., Singh, N., et al. (2010). Deep-sea oil plume enriches indigenous oil-degrading bacteria. Science 330, 204–208. doi: 10.1126/science.1195979
Hicken, C. E., Linbo, T. L., Baldwin, D. H., Willis, M. L., Myers, M. S., Holland, L., et al. (2011). Sublethal exposure to crude oil during embryonic development alters cardiac morphology and reduces aerobic capacity in adult fish. Proc. Natl. Acad. Sci. U.S.A. 108, 7086–7090. doi: 10.1073/pnas.1019031108
Holleman, R. C., Geyer, W. R., and Ralston, D. K. (2016). Stratified turbulence and mixing efficiency in a salt wedge estuary. J. Phys. Oceanogr. 46, 1769–1783. doi: 10.1175/JPO-D-15-0193.1
Hopkins, T. L., and Sutton, T. T. (1998). Midwater fishes and shrimps as competitors and resource partitioning in low latitude oligotrophic ecosystems. Mar. Ecol. Prog. Ser. 164, 37–45. doi: 10.3354/meps164037
Hoskins, B. J. (1974). The role of potential vorticity in symmetric stability and instability. Q. J. R. Met. Soc. 100, 480–482. doi: 10.1002/qj.49710042520
Hsing, P.-Y., Fu, B., Larcom, E. A., Berlet, S. P., Shank, T. M., Govindarajan, A. F., et al. (2013). Evidence of lasting impact of the Deepwater Horizon oil spill on a deep Gulf of Mexico coral community. Elementa 1:000012. doi: 10.12952/journal.elementa.000012
Hu, C., Weisberg, R. H., Liu, Y., Zheng, L., Daly, K. L., English, D. C., et al. (2011). Did the northeastern Gulf of Mexico become greener after the Deepwater Horizon oil spill? Geophys. Res. Lett. 38:L09601.
Incardona, J. P., Carls, M. G., Holland, L., Linbo, T. L., Baldwin, D. H., Myers, M. S., et al. (2015). Very low embryonic crude oil exposures cause lasting cardiac defects in salmon and herring. Sci. Rep. 5:13499.
Incardona, J. P., Collier, T. K., and Scholz, N. L. (2004). Defects in cardiac function precede morphological abnormalities in fish embryos exposed to polycyclic aromatic hydrocarbons. Toxicol. Appl. Pharmacol. 196, 191–205. doi: 10.1016/j.taap.2003.11.026
Incardona, J. P., Gardner, L. D., Linbo, T. L., Brown, T. L., Esbaugh, A. J., Mager, E. M., et al. (2014). Deepwater Horizon crude oil impacts the developing hearts of large predatory pelagic fish. Proc. Natl. Acad. Sci. U.S.A. 111, E1510–E1518.
Ji, Z.-G., Johnson, W. R., Marshall, C. F., and Lear, E. M. (2004). Oil-Spill Risk Analysis: Contingency Planning Statistics for Gulf of Mexico OCS Activities. OCS Report 2004-026. Herndon, VA: U.S. Dept. of the Interior.
Johansen, J. L., Allan, B. J. M., Rummer, J. L., and Esbaugh, A. J. (2017). Oil exposure disrupts early life-history stages of coral reef fishes via behavioural impairments. Nat. Ecol. Evol. 1, 1146–1152. doi: 10.1038/s41559-017-0232-5
Johansen, J. L., and Esbaugh, A. J. (2017). Sustained impairment of respiratory function and swim performance following acute oil exposure in a coastal marine fish. Aquat. Toxicol. 187, 82–89. doi: 10.1016/j.aquatox.2017.04.002
Johansen, J. L., and Esbaugh, A. J. (2019). Oil-induced responses of cardiac and red muscle mitochondria in red drum (Sciaenops ocellatus). Comp. Biochem. Physiol. C 219, 35–41. doi: 10.1016/j.cbpc.2019.02.003
Johansen, Ø (2000). Deepblow- a Lagrangian plume model for deep water blowouts. Spill Sci. Technol. Bull. 6, 103–111. doi: 10.1016/s1353-2561(00)00042-6
Johansen, Ø, Brandvik, P. J., and Farooq, U. (2013). Droplet breakup in subsea oil releases - Part 2: predictions of droplet size distributions with and without injection of chemical dispersants. Mar. Pollut. Bull. 73, 327–335. doi: 10.1016/j.marpolbul.2013.04.012
Johansen, Ø, Rye, H., and Cooper, C. (2003). Deep Spill-Field study of a simulated oil and gas blowout in deep water. Spill Sci. Technol. Bull. 8, 433–443. doi: 10.1016/S1353-2561(02)00123-8
Jones, E. R., Martyniuk, C. J., Morris, J. M., Krasnec, M. O., and Griffitt, R. J. (2017). Exposure to Deepwater Horizon oil and Corexit 9500 at low concentrations induces transcriptional changes and alters immune transcriptional pathways in sheepshead minnows. Comp. Biochem. Physiol. D Genomics Proteomics 23, 8–16. doi: 10.1016/j.cbd.2017.05.001
Joye, S. B. (2015). Deepwater Horizon, 5 years on. Science 349, 592–593. doi: 10.1126/science.aab4133
Joye, S. B., Bracco, A., Özgökmen, T. M., Chanton, J. P., Grosell, J. P., MacDonald, J. P., et al. (2016). The Gulf of Mexico ecosystem, six years after the Macondo oil well blowout. Deep Sea Res. Part II 129, 4–19. doi: 10.1016/j.dsr2.2016.04.018
Joye, S. B., MacDonald, I. R., Leifer, I., and Asper, V. A. (2011). Magnitude and oxidation potential of hydrocarbon gases released from the BP oil well blowout. Nat. Geosci. 4, 160–164. doi: 10.1038/ngeo1067
Joye, S. B., Teske, A. P., and Kostka, J. E. (2014). Microbial Dynamics Following the Macondo Oil Well Blowout across Gulf of Mexico Environments. BioScience 64, 766–777. doi: 10.1093/biosci/biu121
Kessler, J. D., Valentine, D. L., Redmond, M. C., Du, M., Chan, E. W., Mendes, S. D., et al. (2011). A persistent oxygen anomaly reveals the fate of spilled methane in the deep Gulf of Mexico. Science 331, 312–315. doi: 10.1126/science.1199697
Khelifa, A., Fingas, M., and Brown, C. (2010). Effects of Dispersants on Oil-SPM Aggregation and Fate in US Coastal Waters. Durham, NH: University of NH Coastal Response and Research Center.
Khursigara, A. J., Perrichon, P., Martinez Bautista, N., Burggren, W. W., and Esbaugh, A. J. (2017). Cardiac function and survival are affected by crude oil in larval red drum, Sciaenops ocellatus. Sci. Total Environ. 579, 797–804. doi: 10.1016/j.scitotenv.2016.11.026
Kirby, A. R., Cox, G. K., Nelson, D., Heuer, R. M., Stieglitz, J. D., Benetti, D. D., et al. (2019). Acute crude oil exposure alters mitochondrial function and ADP affinity in cardiac muscle fibers of young adult Mahi-mahi (Coryphaena hippurus). Comp. Biochem. Physiol. C. 218, 88–95. doi: 10.1016/j.cbpc.2019.01.004
Kleindienst, S., Grim, S., Sogin, M., Bracco, A., Crespo-Medina, M., and Joye, S. B. (2015). Diverse, rare microbial taxa responded to the Deepwater Horizon deep-sea hydrocarbon plume. ISME J. 10, 400–415. doi: 10.1038/ismej.2015.121
Lacoue-Labarthe, T., Le Pabic, C., and Bustamante, P. (2016). Ecotoxicology of early-life stages in the common cuttlefish Sepia officinalis: review and perspectives. Life Environ. 66, 65–79.
Le Hénaff, M., Kourafalou, V. H., Paris, C. B., Helgers, J., Aman, Z. M., Hogan, P. J., et al. (2012). Surface evolution of the deepwater horizon oil spill patch: combined effects of circulation and wind-induced drift. Environ. Sci. Technol. 46, 7267–7273. doi: 10.1021/es301570w
Ledwell, J. R., He, R., Xue, Z., DiMarco, S. F., Spencer, L., and Chapman, P. (2016). Dispersion of a tracer in the deep northern Gulf of Mexico. J. Geophys. Res. 121:11101132. doi: 10.1002/2015JC011405
Lehr, W., Jones, R., Evans, M., Simecek-Beatty, D., and Overstreet, R. (2002). Revisions of the ADIOS oil spill model. Environ. Modell. Softw. 17, 191–199.
Leitch, A. M., and Baines, W. D. (1989). Liquid volume flux in a weak bubble plume. J. Fluid Mech. 205, 77–98. doi: 10.1017/s0022112089001953
Li, Z., Lee, T., King, T., Boufadel, M. C., and Venosa, A. D. (2008). Oil droplet size distribution as a function of energy dissipation rate in an experimental wave tank. Int. Oil Spill Conf. Proc. 2008, 621–626. doi: 10.7901/2169-3358-2008-1-621
Li, Z., Spaulding, M. L., and French-McCay, D. (2017). An algorithm for modeling entrainment and naturally and chemically dispersed oil droplet size distribution under surface breaking wave conditions. Mar. Pollut. Bull. 119, 45–152. doi: 10.1016/j.marpolbul.2017.03.048
Lindo-Atichati, D., Paris, C. B., Le Hénaff, M., Schedler, M., Valladares Juárez, A. G., and Müller, A. (2016). Simulating the effects of droplet size, high-pressure biodegradation, and variable flow rate on the subsea evolution of deep plumes from the Macondo blowout. Deep Sea Res. II Top. Stud. Oceanogr. 129, 301–310. doi: 10.1016/j.dsr2.2014.01.011
Liu, Y., Weisberg, R. H., Hu, C., and Zheng, L. (2011). Tracking the deepwater horizon oil spill: a modeling perspective. EOS Trans. 92, 45–46. doi: 10.1029/2011eo060001
Lubchenco, J., McNutt, M. K., Dreyfus, G., Murawski, S. A., Kennedy, D. M., Anastas, P. T., et al. (2012). Science in support of the Deepwater Horizon response. Proc. Nat. Acad. Sci. U.S.A. 109, 20212–20221. doi: 10.1073/pnas.1204729109
Mager, E. M., Esbaugh, A. J., Stieglitz, J. D., Hoenig, R., Bodinier, C., Incardona, J. P., et al. (2014). Acute embryonic or juvenile exposure to Deepwater Horizon crude oil impairs the swimming performance of mahi-mahi (Coryphaena hippurus). Environ. Sci. Technol. 48, 7053–7061. doi: 10.1021/es501628k
Magnuson, J. T., Khursigara, A. J., Allmon, E. B., Esbaugh, A. J., and Roberts, A. P. (2018). Effects of Deepwater Horizon crude oil on ocular development in two estuarine fish species, red drum (Sciaenops ocellatus) and sheepshead minnow (Cyprinodon variegatus). Ecotoxicol. Environ. Saf. 166, 186–191. doi: 10.1016/j.ecoenv.2018.09.087
Malone, K., Pesch, S., Schlüter, M., and Krause, D. (2018). Oil droplet size distributions in deep-sea blowouts: influence of pressure and dissolved gases. Environ. Sci. Technol. 52, 6326–6333. doi: 10.1021/acs.est.8b00587
Martineau, D., Lemberger, K., Dallaire, A., Labelle, P., Lipscomb, T. P., Michel, P., et al. (2002). Cancer in wildlife, a case study: beluga from the St. Lawrence estuary, Québec, Canada. Environ. Health Perspect. 110, 285–292. doi: 10.1289/ehp.02110285
Mason, O. U., Hazen, T. C., Borglin, S., Chain, P. S., Dubinsky, E. A., Fortney, J. L., et al. (2012). Metagenome, metatranscriptome and single-cell sequencing reveal microbial response to Deepwater Horizon oil spill. ISME J. 6, 1715–1727. doi: 10.1038/ismej.2012.59
Mason, O. U., Scott, N. M., Gonzalez, A., Robbins-Pianka, A., Bælum, J., Kimbrel, J., et al. (2014). Metagenomics reveals sediment microbial community response to Deepwater Horizon oil spill. ISME J. 8:1464. doi: 10.1038/ismej.2013.254
Mauduit, F., Farrell, A. P., Domenici, P., Lacroix, C., Le Floch, S., Lemaire, P., et al. (2019). Assessing the long-term effect of exposure to dispersant-treated oil on fish health using hypoxia tolerance and temperature susceptibility as ecologically relevant biomarkers. Environ. Toxicol. Chem. 38, 210–221. doi: 10.1002/etc.4271
McCarren, J., Becker, J. W., Repeta, D. J., Shi, Y., Young, C. R., Malmstrom, R. R., et al. (2010). Microbial community transcriptomes reveal microbes and metabolic pathways associated with dissolved organic matter turnover in the sea. PNAS 107, 16420–16427. doi: 10.1073/pnas.1010732107
McGruer, V., Pasparakis, C., Grosell, M., Stieglitz, J. D., Benetti, D. D., Greer, J. B., et al. (2019). Deepwater Horizon crude oil exposure alters cholesterol biosynthesis with implications for developmental cardiotoxicity in larval mahi-mahi (Coryphaena hippurus). Comp. Biochem. Physiol. C 220, 31–35. doi: 10.1016/j.cbpc.2019.03.001
McNutt, M., Camilli, R., Crone, T. J., Guthrie, G. D., Hsieh, P. A., Ryerson, T. B., et al. (2012). Review of flow rate estimates of the Deepwater Horizon oil spill. Proc. Nat. Acad. Sciences U.S.A. 109, 20260–20267. doi: 10.1073/pnas.1112139108
Müller, P., and Liu, X. (2000). Scattering of internal waves at finite topography in two dimensions. Part I: theory and case studies. J. Phys. Oceanogr. 30, 532–549. doi: 10.1175/1520-0485(2000)030<0532:soiwaf>2.0.co;2
Murawski, S. A., Schlüter, M., Paris, C. B., and Aman, Z. M. (2020). “Chapter 28: summary of contemporary research on the use of chemical dispersants for deep-sea oil spills,” in Deep Oil Spills – Facts, Fate and Effects, eds S. A. Murawski, C. Ainsworth, S. Gilbert, D. Hollander, C. B. Paris, M. Schlüter, et al. (Berlin: Springer), 494–512. doi: 10.1007/978-3-030-12963-7_28
Nash, J. D., and Moum, J. N. (2001). Internal hydraulic flows on the continental shelf: high drag states over a small bank. J. Geoph. Res. Oceans 106, 4593–4611. doi: 10.1029/1999jc000183
National Academies of Sciences, Engineering, and Medicine (2018). Understanding the Long-Term Evolution of the Coupled Natural-Human Coastal System: The Future of the U.S. Gulf Coast. Washington, DC: The National Academies Press.
National Commission on the Bp Deepwater Horizon Oil Spill and Offshore Drilling (2010). The Amount and Fate of the Oil. Staff working paper. no. 3. Available online at: https://cybercemetery.unt.edu/archive/oilspill/20130215212051/http://www.oilspillcommission.gov/sites/default/files/documents/Updated%20Amount%20and%20Fate%20of%20the%20Oil%20Working%20Paper.pdf (accessed August 31, 2020).
National Commission on the Bp Deepwater Horizon Oil Spill and Offshore Drilling (2011). Deep Water. The Gulf Oil Disaster and the Future of Offshore Drilling. Available online at:: https://www.nrt.org/sites/2/files/GPO-OILCOMMISSION.pdf (accessed August 31, 2020).
Nelson, D., Heuer, R. M., Cox, G. K., Stieglitz, J. D., Hoenig, R., Mager, E. M., et al. (2016). Effects of crude oil on in situ cardiac function in young adult mahi-mahi (Coryphaena hippurus). Aquat. Toxicol. 180, 274–281. doi: 10.1016/j.aquatox.2016.10.012
Nelson, D., Stieglitz, J. D., Cox, G. K., Heuer, R. M., Benetti, D. D., Grosell, M., et al. (2017). Cardio-respiratory function during exercise in the cobia, Rachycentron canadum: the impact of crude oil exposure. Comp. Biochem. Physiol. C 201, 58–65. doi: 10.1016/j.cbpc.2017.08.006
Nissanka, I. D., and Yapa, P. D. (2016). Calculation of oil droplet size distribution in an underwater oil well blowout. J. Hydr. Res. 54, 307–320. doi: 10.1080/00221686.2016.1144656
North, E. W., Adams, E. E., Thessen, A. E., Schlag, Z., He, R., Socolofsky, S. A., et al. (2015). The influence of droplet size and biodegradation on the transport of subsurface oil droplets during the Deepwater Horizon spill: a model sensitivity study. Environ. Res. Lett. 10:024016. doi: 10.1088/1748-9326/10/2/024016
Okubo, A. (1987). “Fantastic voyage into the deep: marine biofluid mechanics,” in Mathematical Topics in Population Biology, Morphogenesis and Neurosciences. Lecture Notes in Biomathematics, Vol. 71, eds E. Teramoto and M. Yumaguti (Berlin: Springer).
Olsen, J. E., and Skjetne, P. (2016). Current understanding of subsea gas release: a review. Can. J. Chem. Eng. 94, 209–219. doi: 10.1002/cjce.22345
Pan, Y. K., Khursigara, A. J., Johansen, J. L., and Esbaugh, A. J. (2018). The effects of oil induced respiratory impairment on two indices of hypoxia tolerance in Atlantic croaker (Micropogonias undulates). Chemosphere 200, 143–150. doi: 10.1016/j.chemosphere.2018.02.028
Paris, C. B., Berenshtein, I., Trillo, M. L., Faillettaz, R., Olascoaga, M. J., Aman, Z. M., et al. (2018). BP gulf science data reveals ineffectual sub-sea dispersant injection for the Macondo Blowout. Front. Mar. Sci. Sect. Mar. Pollut. 5:389. doi: 10.3389/fmars.2018.00389
Paris, C. B., Helgers, J., van Sebille, E., and Srinivasan, A. (2013). Connectivity modeling system: a probabilistic modeling tool for the multi-scale tracking of biotic and abiotic variability in the ocean”. Environ. Model. Softw. 42, 47–54. doi: 10.1016/j.envsoft.2012.12.006
Paris, C. B., Le Hénaff, M., Aman, Z. M., Subramaniam, A., Helgers, J., Wang, D. P., et al. (2012). Evolution of the Macondo well blowout: simulating the effects of the circulation and synthetic dispersants on the subsea oil transport. Environ. Sci. Technol. 46, 13293–13302. doi: 10.1021/es303197h
Paris, C. B., Vaz, A. C., Berenshtein, I., Perlin, P., Faillettaz, R., Aman, Z. M., et al. (2020). “Simulating deep oil spills beyond the Gulf of Mexico,” in Scenarios and Responses to Future Deep Oil Spills: Fighting the Next War, Chap. 19, eds S. A. Murawski, C. Ainsworth, S. Gilbert, D. Hollander, C. B. Paris, M. Schlüter, et al. (Berlin: Springer), 315–336. doi: 10.1007/978-3-030-12963-7_19
Pasparakis, C., Sweet, L. E., Stieglitz, J. D., Benetti, D., Casente, C. T., Roberts, A. P., et al. (2017). Combined effects of oil exposure, temperature and ultraviolet radiation on buoyancy and oxygen consumption of embryonic mahi-mahi, Coryphaena hippurus. Aquat. Toxicol. 191, 113–121. doi: 10.1016/j.aquatox.2017.07.021
Passow, U. (2016). Formation of rapidly-sinking, oil-associated marine snow. Deep Sea Res. II Top. Stud. Oceanogr. 19, 232–240. doi: 10.1016/j.dsr2.2014.10.001
Passow, U., Sweet, J., and Quigg, A. (2017). How the dispersant Corexit impacts the formation of sinking marine oil snow. Mar. Pollut. Bull. 125, 139–145. doi: 10.1016/j.marpolbul.2017.08.015
Passow, U., Ziervogel, K., Asper, V., and Diercks, A. (2012). Marine snow formation in the aftermath of the Deepwater Horizon oil spill in the Gulf of Mexico. Environ. Res. Lett. 7:035301. doi: 10.1088/1748-9326/7/3/035301
Perlin, A., Moum, J. N., Klymak, J. M., Levine, M. D., Boyd, T., and Kosro, P. M. (2005). A modified law-of-the-wall applied to oceanic bottom boundary layers. J. Geophys. Res. 110:C10S10. doi: 10.1029/2004JC002310
Perlin, N., Berenshtein, I., Vaz, A. C., Faillettaz, R., Schwing, P. T., Romero, P. T., et al. (2020). “Far-field modeling of deep-sea blowout: sensitivity studies of initial conditions, biodegradation, sedimentation and SSDI on surface slicks and oil plume concentrations,” in Deep Oil Spills: Facts, Fate, Effects, eds S. A. Murawski, C. Ainsworth, S. Gilbert, D. Hollander, C. B. Paris, M. Schlüter, et al. (Cham: Springer).
Pesch, S., Jaeger, P., Jaggi, A., Malone, K., Hoffmann, M., Krause, D., et al. (2018). Rise velocity of live-oil droplets in deep-sea oil spills. Environ. Eng. Sci. 35, 289–299. doi: 10.1089/ees.2017.0319
Pesch, S., Schlüter, M., Aman, Z. M., Malone, K., Krause, D., and Paris, C. B. (2020). “Behavior of rising droplets and bubbles: impact on the physics of deep-sea blowouts and oil fate,” in Deep Oil Spills, eds S. Gilbert, M. Schlüter, and S. A. Murawski (Cham: Springer), 65–82. doi: 10.1007/978-3-030-11605-7_5
Polzin, K. L., Kunze, E., Toole, J. M., and Schmitt, R. W. (2003). The partition of finescale energy into internal waves and subinertial motions. J. Phys. Oceanogr. 33, 234–248. doi: 10.1175/1520-0485(2003)033<0234:tpofei>2.0.co;2
Prouty, N. G., Fisher, C. R., Demopoulos, A. W. J., and Druffel, E. R. M. (2016). Growth rates and ages of deep-sea corals impacted by the deepwater horizon oil spill. Deep Sea Res. II Top. Stud. Ocean 129, 196–212. doi: 10.1016/j.dsr2.2014.10.021
Pulster, E. L., Gracia, A., Armenteros, M., Carr, B. E., Mrowicki, J., and Murawski, S. A. (2020). Chronic PAH exposures and associated declines in fish health indices observed for ten grouper species in the Gulf of Mexico. Sci. Total Environ. 703:135551. doi: 10.1016/j.scitotenv.2019.135551
Quintana-Rizzo, E., Torres, J. J., Ross, S. W., Romero, I., Watson, K., Goddard, E., et al. (2015). δ13C and δ15N in deep-living fishes and shrimps after the Deepwater Horizon oil spill, Gulf of Mexico. Mar. Pollut. Bull. 94, 241–250. doi: 10.1016/j.marpolbul.2015.02.002
Reddam, A., Mager, E. M., Grosell, M., and McDonald, M. D. (2017). The impact of acute PAH exposure on the toadfish glucocorticoid stress response. Aquat. Toxicol. 192, 89–96. doi: 10.1016/j.aquatox.2017.08.014
Reddy, C. M., Arey, J. C., Seewald, J. S., Sylva, S. P., Lemkau, K. L., Nelson, R. K., et al. (2012). Composition and fate of gas and oil released to the water column during the Deepwater Horizon oil spill. Proc. Natl. Acad. Sci. U.S.A. 109, 20229–20234. doi: 10.1073/pnas.1101242108
Redmond, M. C., and Valentine, D. L. (2012). Natural gas and temperature structured a microbial community response to the Deepwater Horizon oil spill. Proc. Natl. Acad. Sci. U.S.A. 109, 20292–20297. doi: 10.1073/pnas.1108756108
Reed, M., Johansen, O., Brandvik, P. J., Daling, P., Lewis, A., Fiocco, R., et al. (1999). Oil spill modeling towards the close of the 20th century: overview of the state of the art. Spill Sci. Technol. Bull. 5, 3–16. doi: 10.1016/s1353-2561(98)00029-2
Response efforts to the Gulf Coast oil spill (2010). Hearing before the Committee on Commerce, Science, and Transportation, Senate, 111th Cong. Gulf of Mexico: Gulf Coast oil spill, 1011–1013.
Risk, M. J., Heikoop, J. M., Snow, M. G., and Beukens, R. (2002). Lifespans and growth patterns of two deep-sea corals: Primnoa resedaeformis and Desmophyllum cristagalli. Hydrobiologia 471, 125–131.
Rivers, A. R., Sharma, S., Tringe, S. G., Martin, J., Joye, S. B., and Moran, M. A. (2013). Transcriptional response of bathypelagic marine bacterioplankton to the Deepwater Horizon oil spill. ISME J. 7, 2315–2329. doi: 10.1038/ismej.2013.129
Roark, E. B., Guilderson, T. P., Dunbar, R. B., Fallon, S. J., and Mucciarone, D. A. (2009). Extreme longevity in proteinaceous deep-sea corals. Proc. Nat. Acad. Sci. U.S.A. 106, 5204–5208. doi: 10.1073/pnas.0810875106
Rodgers, M. L., Takeshita, R., and Griffitt, R. J. (2018). Deepwater Horizon oil alone and in conjunction with Vibrio anguillarum exposure modulates immune response and growth in red snapper (Lutjanus campechanus). Aquat. Toxicol. 204, 91–99. doi: 10.1016/j.aquatox.2018.09.002
Rogener, M. K., Bracco, A., Hunter, K. S., Saxton, M. A., and Joye, S. B. (2018). Impact of the Deepwater Horizon oil well blowout on methane oxidation dynamics in the Northern Gulf of Mexico. Elementa 6:73. doi: 10.1525/elementa.332
Romero, I. C., Schwing, P. T., Brooks, G. R., Larson, R. A., Hastings, D. W., Ellis, G., et al. (2015). Hydrocarbons in deep-sea sediments following the 2010 Deepwater Horizon blowout in the northeast Gulf of Mexico. PLoS One 10:e0128371. doi: 10.1371/journal.pone.0128371
Romero, I. C., Sutton, T., Carr, B., Quintana-Rizzo, E., Ross, S. W., Hollander, D. J., et al. (2018). Decadal assessment of polycyclic aromatic hydrocarbons in mesopelagic fishes from the gulf of mexico reveals exposure to oil-derived sources. Environ. Sci. Technol. 52, 10985–10996. doi: 10.1021/acs.est.8b02243
Rowsey, L. E., Johansen, J. L., Khursigara, A. J., and Esbaugh, A. J. (2019). Oil exposure impairs predator–prey dynamics in larval red drum (Sciaenops ocellatus). Mar. Freshw. Res. 71, 99–106. doi: 10.1071/MF18263
Ruan, X., and Thompson, A. F. (2016). Bottom boundary potential vorticity injection from an oscillating flow: a PV pump. J. Phys. Ocean. 46, 3509–3526. doi: 10.1175/jpo-d-15-0222.1
Ryerson, T. B., Camilli, R., Kessler, J. D., Kujawinski, E. B., Reddy, C. M., Valentine, D. L., et al. (2012). Chemical data quantify Deepwater Horizon hydrocarbon flow rate and environmental distribution. Proc. Nat. Acad. Sci. U.S.A. 109, 20246–20253. doi: 10.1073/pnas.1110564109
Satter, A., and Iqbal, G. M. (2016). Reservoir Engineering. Waltham, Ma: Gulf professional publishing.
Schwacke, L. H., Smith, C. R., Townsend, F. I., Wells, R. S., Hart, L. B., Balmer, B. C., et al. (2013). Health of common bottlenose dolphins (tursiops truncatus) in barataria bay, louisiana, following the deepwater horizon oil spill. Environ. Sci. Technol. 48, 93–103.
Schwing, P. T., Romero, I. C., Brooks, G. R., Hastings, D. W., Larson, R. A., and Hollander, D. J. (2015). A decline in benthic foraminifera following the Deepwater Horizon event in the northeastern Gulf of Mexico. PLoS One 10:e0120565. doi: 10.1371/journal.pone.0120565
Sibert, R. J., Harrison, S. J., and Joye, S. B. (2016). “Protocols for radiotracer estimation of primary hydrocarbon oxidation in oxygenated seawater,” in Hydrocarbon and Lipid Microbiology Protocols, eds T. J. Mcgenity, K. N. Timmis, and B. Nogales (Totowa, NJ: Humana press), 263–276. doi: 10.1007/8623_2016_227
Snyder, S. M., Pulster, E. L., Wetzel, D. L., and Murawski, S. A. (2015). Pah exposure in gulf of mexico demersal fishes, post-deepwater horizon. Environ. Sci. Technol. 49, 8786–8795. doi: 10.1021/acs.est.5b01870
Socolofsky, S. A., and Adams, E. E. (2002). Multi-phase plumes in uniform and stratified crossflow. J. Hydr. Res. 40, 661–672. doi: 10.1080/00221680209499913
Socolofsky, S. A., and Adams, E. E. (2005). Role of slip velocity in the behavior of stratified multiphase plumes. J. Hydr. Eng. 131, 273–282. doi: 10.1061/(asce)0733-9429(2005)131:4(273)
Socolofsky, S. A., Adams, E. E., Boufadel, M. C., Aman, Z. M., Johansen, Ø, Konkel, W. J., et al. (2015). Intercomparison of oil spill prediction models for accidental blowout scenarios with and without subsea chemical dispersant injection. Mar. Pollut. Bull. 96, 110–126. doi: 10.1016/j.marpolbul.2015.05.039
Socolofsky, S. A., Adams, E. E., Paris, C., and Yang, D. (2016). How do oil, gas, and water interact near a subsea blowout? Oceanography 29, 64–75. doi: 10.5670/oceanog.2016.63
Socolofsky, S. A., Adams, E. E., and Sherwood, C. R. (2011). Formation dynamics of subsurface hydrocarbon intrusions following the Deepwater Horizon blowout. Geophys. Res. Lett. 38:L09602. doi: 10.1029/2011GL047174
Spaulding, M. S. (1988). A state-of-the-art review of oil spill trajectory and fate modeling. Oil Chem. Pollut. 4, 39–55. doi: 10.1016/s0269-8579(88)80009-1
Spaulding, M. S., Li, Z., Mendelsohn, D., Crowley, D., French-McCay, D., and Bird, A. (2017). Application of an integrated blowout model system, OILMAP DEEP, to the deepwater horizon (DWH) spill. Mar. Pollut. Bull. 120, 37–50. doi: 10.1016/j.marpolbul.2017.04.043
Spaulding, M. S., Mendelsohn, D., Crowley, D., Li, Z., and Bird, A. (2015). Technical Reports for Deepwater Horizon Water Column Injury Assessment – WC_TR.13: Application of OILMAP DEEP to the Deepwater Horizon Blowout. South Kingstown, RI: RPS ASA.
Stieglitz, J. D., Mager, E. M., Hoenig, R. H., Benetti, D. D., and Grosell, M. (2016). Impacts of Deepwater Horizon crude oil exposure on adult mahi-mahi (Coryphaena hippurus) swim performance. Environ. Toxicol. Chem. 35, 2613–2622. doi: 10.1002/etc.3436
Stout, S. A., Payne, J. R., Emsbo-Mattingly, S. D., and Baker, G. (2013). Weathering of field-collected floating and stranded Macondo oils during and shortly after the Deepwater Horizon oil spill”. Mar. Pollut. Bull. 105, 7–22. doi: 10.1016/j.marpolbul.2016.02.044
Thorpe, S. A. (1992). The generation of internal waves by flow over the rough topography of a continental slope. Proc. R. Soc. Lond. A 439, 115–130. doi: 10.1098/rspa.1992.0137
Tyack, P. L., Johnson, M., Soto, N. A., Sturlese, A., and Madsen, P. T. (2006). Extreme diving of beaked whales. J. Exp. Biol. 209, 4238–4253. doi: 10.1242/jeb.02505
Umlauf, L., Smyth, W. D., and Moum, J. N. (2015). Energetics of bottom Ekman layers during buoyancy arrest. J. Phys. Oceanogr. 45, 3099–3117.
Valentine, D. L., Kessler, J. D., Redmond, M. C., Mendes, S. D., Heintz, M. B., Farwell, C., et al. (2010). Propane respiration jump-starts microbial response to a deep oil spill. Science 330, 208–211.
Vaz, A. C., Paris, C. B., Dissanayake, A. L., Socolofsky, S. A., Gros, J., and Boufadel, M. C. (2020). “Dynamic coupling of near-field and far-field models,” in Deep Oil Spills: Facts, Fate, Effects, eds S. A. Murawski, C. Ainsworth, S. Gilbert, D. Hollander, C. B. Paris, M. Schlüter, et al. (Cham: Springer).
Venn-Watson, S., Garrison, L., Litz, J., Fougeres, E., Mase, B., Rappucci, G., et al. (2015). Demographic clusters identified within the Northern Gulf of Mexico common bottlenose dolphin (Tursiops truncatus) Unusual Mortality Event: January 2010-June 2013. PLoS One 10:e0117248. doi: 10.1371/journal.pone.0117248
Walker, N. D., Pilley, C. T., Raghunathan, V. V., D’Sa, E. J., Leben, R. R., Hoffmann, N. G., et al. (2011). “Impacts of loop current frontal cyclonic eddies and wind forcing on the 2010 Gulf of Mexico oil spill,” in Monitoring and Modeling the Deepwater Horizon Oil Spill: A Record-Breaking Enterprise. Geophysical Monograph Series 195, eds Y. Liu, A. MacFadyen, Z. G. Ji, and R. H. Weisberg (Washington, D.C: American Geophysical Union).
Wang, B., Socolofsky, S. A., Lai, C. C. K., Adams, E. E., and Boufadel, M. C. (2018). Behavior and dynamics of bubble breakup in gas pipeline leaks and accidental subsea oil well blowouts. Mar. Pollut. Bull. 131, 72–86. doi: 10.1016/j.marpolbul.2018.03.053
Wankel, S. D., Joye, S. B., Samarkin, V. A., Shah, S. R., Friederich, G., Melas-Kyriazi, J., et al. (2010). New constraints on methane fluxes and rates of anaerobic methane oxidation in a Gulf of Mexico brine pool via in situ mass spectrometry. Deep Sea Res. Part II 57, 2022–2029. doi: 10.1016/j.dsr2.2010.05.009
Watwood, S. L., Miller, P. J., Johnson, M., Madsen, P. T., and Tyack, P. L. (2006). Deep-diving foraging behaviour of sperm whales (Physeter macrocephalus). J. Anim. Ecol. 75, 814–825.
White, H. K., Hsing, P.-Y., Cho, W., Shank, T. M., Cordes, E. E., and Quattrini, A. M. (2012). Impact of the Deepwater Horizon oil spill on a deep-water coral community in the Gulf of Mexico. Proc. Nat. Acad. Sci. U.S.A. 109, 20303–20308. doi: 10.1073/pnas.1118029109
Wilkin, S. M., Rowles, T. K., Stratton, E., Adimey, N., Field, C. L., and Wissmann, S. (2017). Marine mammal response operations during the Deepwater Horizon oil spill. Endang. Spec. Res. 33, 107–118.
Williams, R., Gero, S., Bejder, L., Calambokidis, J., Kraus, S. D., and Lusseau, D. (2011). Underestimating the damage: interpreting cetacean carcass recoveries in the context of the Deepwater Horizon/BP incident. Conserv. Lett. 4, 228–233.
Xu, E. G., Khursigara, A. J., Li, S., Esbaugh, A. J., Dasgupta, S., Volz, D., et al. (2019). mRNA-miRNA-Seq reveals neuro-cardio mechanisms of crude oil toxicity in red drum (Sciaenops ocellatus). Environ. Sci. Technol. 53, 3296–3305. doi: 10.1021/acs.est.9b00150
Xu, E. G., Khursigara, A. J., Magnuson, J., Hazard, E. S., Hardiman, G., Esbaugh, A. W., et al. (2017). Larval red drum (Sciaenops ocellatus) sublethal exposure to weathered deepwater horizon crude oil: developmental and transcriptomic consequences. Environ. Sci. Technol. 51, 10162– 10172.
Yang, D., and Chen, B. (2004). Three-dimensional visualization of multi-phase (oil/gas/hydrate) plumes. Environ. Model. Softw. 19, 751–760.
Yang, T., Nigro, L. M., Gutierrez, T., D’Ambrosio, L., Joye, S. B., Highsmith, R., et al. (2016). Pulsed blooms and persistent oil-degrading bacterial populations in the water column during and after the Deepwater Horizon blowout. Deep Sea Res. Part II 129, 282–291. doi: 10.1016/j.dsr2.2014.01.014
Yapa, P. D., Chen, F., and Beegle-Krause, C. J. (2003). “Integration of the CDOG deep water oil and gas blowout model with the NOAA GNOME trajectory model,” in Proceedings of the AMOP 2003, Victoria, BC.
Yapa, P. D., Dasanayaka, L. K., Bandara, U. C., and Nakata, K. (2010). A model to simulate the transport and fate of gas and hydrates released in deepwater”. J. Hydr. Res. 48, 559–572.
Yapa, P. D., Wimalaratne, M. R., Dissanayake, A. L., and DeGraff, J. A. Jr. (2012). How does oil and gas behave when released in deepwater? J. Hydro Environ. Res. 6, 275–285.
Yapa, P. D., and Zheng, L. (1997). Simulation of oil spills from underwater accidents I: model development. J. Hydr. Res. 35, 673–687.
Yapa, P. D., Zheng, L., and Chen, F. H. (2001). A model for deepwater oil/gas blowouts. Mar. Pollut. Bull. 43, 234–241.
Yapa, P. D., Zheng, L., and Nakata, K. (1999). Modeling underwater oil/gas jets and plumes. J. Hydr. Eng. 125, 481–491.
Zhang, Y., Mauduit, F., Farrell, A. P., Chabot, D., Ollivier, H., Rio-Cabello, A., et al. (2017). Exposure of European sea bass (Dicentrarchus labrax) to chemically dispersed oil has a chronic residual effect on hypoxia tolerance but not aerobic scope. Aquat. Toxicol. 191, 95–104.
Zhao, L., Boufadel, M. C., Adams, E. E., Socolofsky, S. A., King, T., Lee, K., et al. (2015). Simulation of scenarios of oil droplet formation from the Deepwater Horizon blowout. Mar. Pollut. Bull. 101, 304–319.
Zhao, L., Boufadel, M. C., King, T., Robinson, B., Gao, F., Socolofsky, S. A., et al. (2017). droplet and bubble formation of combined oil and gas releases in subsea blowouts. Mar. Pollut. Bull. 120, 203–216. doi: 10.1016/j.marpolbul.2017.05.010
Zhao, L., Shaffer, F., Robinson, B., King, T., D’ambrose, C., Pan, Z., et al. (2016). underwater oil jet: hydrodynamics and droplet size distribution. Chem. Eng. J. 299, 292–303.
Zhao, L., Boufadel, M. C., Socolofsky, S. A., Adams, E., King, T., and Lee, K. (2014a). Evolution of droplets in subsea oil and gas blowouts: development and validation of the numerical model VDROP-J. Mar. Pollut. Bull. 83, 58–69. doi: 10.1016/j.marpolbul.2014.04.020
Zhao, L., Torlapati, J., Boufadel, M. C., King, T., Robinson, B., and Lee, K. (2014b). VDROP: a comprehensive model for droplet formation of oils and gases in liquids - Incorporation of the interfacial tension and droplet viscosity. Chem. Eng. J. 253, 93–106. doi: 10.1016/j.cej.2014.04.082
Zheng, L., and Yapa, P. D. (2000). Buoyant velocity of spherical and nonspherical bubbles/droplets. J. Hydr. Eng. 126, 852–854. doi: 10.1061/(ASCE)0733-94292000126:11(852)
Zheng, L., Yapa, P. D., and Chen, F. (2003). A model for simulating deepwater oil and gas blowouts - Part I: theory and model formulation. J. Hydr. Res. 41, 339–351. doi: 10.1080/00221680309499980
Zick, A. A. (2013a). Equation-of-State Fluid Characterization and Analysis of the Macondo Reservoir Fluids; Expert Report Prepared On Behalf of the United States; TREX-011490R. Washington, DC: United States of America.
Zick, A. A. (2013b). Expert Rebuttal Report; Prepared on Behalf of the United States; TREX-011491R. Washington, DC: United States of America.
Keywords: Deepwater Horizon, deepwater plume, ocean modeling, oil modeling, transport and mixing processes, active tracer
Citation: Bracco A, Paris CB, Esbaugh AJ, Frasier K, Joye SB, Liu G, Polzin KL and Vaz AC (2020) Transport, Fate and Impacts of the Deep Plume of Petroleum Hydrocarbons Formed During the Macondo Blowout. Front. Mar. Sci. 7:542147. doi: 10.3389/fmars.2020.542147
Received: 11 March 2020; Accepted: 24 August 2020;
Published: 11 September 2020.
Edited by:
Elva G. Escobar-Briones, National Autonomous University of Mexico, MexicoReviewed by:
Andreas Teske, University of North Carolina at Chapel Hill, United StatesIan MacDonald, Florida State University, United States
Melbourne G. Briscoe, Independent Researcher, Alexandria, United States
Copyright © 2020 Bracco, Paris, Esbaugh, Frasier, Joye, Liu, Polzin and Vaz. This is an open-access article distributed under the terms of the Creative Commons Attribution License (CC BY). The use, distribution or reproduction in other forums is permitted, provided the original author(s) and the copyright owner(s) are credited and that the original publication in this journal is cited, in accordance with accepted academic practice. No use, distribution or reproduction is permitted which does not comply with these terms.
*Correspondence: Annalisa Bracco, YWJyYWNjb0BnYXRlY2guZWR1; Claire B. Paris, Y3BhcmlzQHJzbWFzLm1pYW1pLmVkdQ==