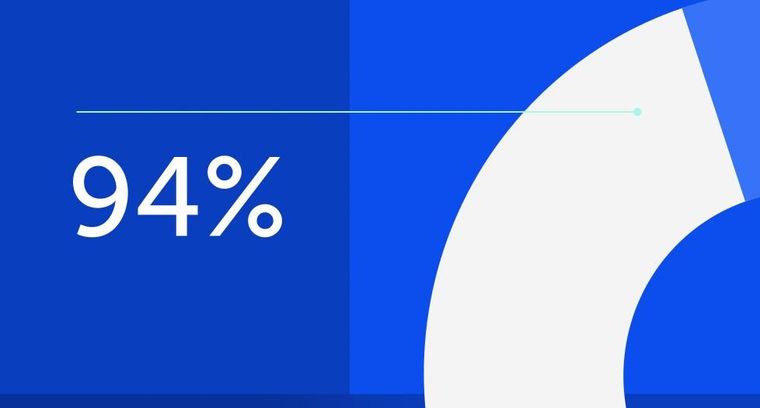
94% of researchers rate our articles as excellent or good
Learn more about the work of our research integrity team to safeguard the quality of each article we publish.
Find out more
ORIGINAL RESEARCH article
Front. Mar. Sci., 11 August 2020
Sec. Marine Molecular Biology and Ecology
Volume 7 - 2020 | https://doi.org/10.3389/fmars.2020.00598
This article is part of the Research TopicMarine Environmental EpigeneticsView all 14 articles
Eastern oysters in the northern Gulf of Mexico are routinely infected with the protistan parasite Perkinsus marinus, the cause of the disease commonly known as dermo. Recent experimental challenges among Atlantic coast populations have identified both resistant and susceptible genotypes using comparative transcriptomics. While controlled experimental challenges are essential first assessments, expanding this analysis to field reared individuals provides an opportunity to identify key genomic signatures of infection that appear both in the laboratory and in the field. In this study we combined reduced representation bisulfite sequencing with 3′ RNA sequencing (Tag-seq) to describe two molecular phenotypes associated with infection in oysters outplanted at a common garden field site. These combined approaches allowed us to examine changes in DNA methylation and gene expression for a large number of individuals (n = 40) that developed infections during the course of a common garden outplant experiment. Our epigenetic analysis of DNA methylation identified significant changes in gene body methylation associated with increasing infection intensity, across genes associated with immune responses. There was a smaller transcriptomic response to increasing infection intensities with 32 genes showing differential expression; however, only 40% of these genes were found to also be differentially methylated. While there was no clear pattern between direction of differential methylation and gene expression, there was a significant effect of percent methylation on gene-by-gene expression levels and the coefficient of variation in gene body methylation between treatments. These results show that in C. virginica, heavily methylated genes have high levels of gene expression with low levels of variation. Comparing our differential expression results with previously published experimental P. marinus challenges identified overlapping expression patterns for genes associated with C1q-domain-containing and V-type proton ATPase proteins. Through our comparative transcriptomic approach using field reared individuals and co-expression network analysis we have also been able to identify a network of genes that change in expression in response to infection. These combined analyses provide evidence for a conserved response to P. marinus infections across infection intensities and suggest that DNA methylation may not be a reliable predictor of differential gene expression in long-term infections.
The relationship between tissue-specific DNA methylation and gene expression is an area of interest to a broad research community as these two molecular phenotypes have been proposed as mechanisms of environmental responsiveness and used as biomarkers to describe interactions between organisms and their environment (Roberts and Gavery, 2012; Dixon et al., 2014; Putnam et al., 2016; Hawes et al., 2018; Schmid et al., 2018; Eirin-Lopez and Putnam, 2019; Rubi et al., 2019). The utility of these metrics relies heavily on a well-developed understanding of how a stimulus will modulate either gene expression or DNA methylation levels, and how methylation could in turn modulate gene expression. As a result, the last decade has seen a dramatic increase in the number of studies using whole transcriptome sequencing to describe how non-model species modify their transcriptomes in response to developmental cues, diseases, and shifts in the abiotic environment (Alvarez et al., 2015; De Wit et al., 2018; Jones et al., 2019; Proestou and Sullivan, 2020). Interest in the functional role of DNA methylation has also grown in recent years due to its potential contributions to transgenerational plasticity (Kappeler and Meaney, 2010; Herman and Sultan, 2016; Gavery and Roberts, 2017; Ryu et al., 2018). These studies together have further fueled interest in the mechanisms that control transcriptome variation, with the majority of studies focusing on either DNA methylation or chromatin modifications (Clark et al., 2018; Weinhold, 2018; Eirin-Lopez and Putnam, 2019).
Oysters in the genus Crassostrea are both an ecologically and environmentally important keystone species distributed across broad geographic ranges. These widely dispersed species inhabit highly variable environments leading to species with a high degree of phenotypic plasticity (Li et al., 2017). As such, oysters are an ideal organism for exploring how DNA methylation influences phenotypic plasticity. Recent research on this genus has focused on describing the degree to which gene expression contributes to phenotype or how DNA methylation directs gene expression levels. These studies have found strong evidence that environmental variation and/or disease state can have a predictable influence on gene expression (Yan et al., 2017; Jones et al., 2019; Proestou and Sullivan, 2020). In a similar manner, a growing body of literature has provided ample evidence that DNA methylation can shape the transcriptomic phenotype (Rivière et al., 2013; Olson and Roberts, 2014; Song et al., 2017). In the Pacific oyster (C. gigas) high levels of methylation in gene bodies are associated with elevated expression, while lowly methylated genes showed higher levels of transcriptomic plasticity (Gavery and Roberts, 2013; Olson and Roberts, 2014). In the eastern oyster (C. virginica) DNA methylation is more divergent between populations than single nucleotide polymorphism-based estimates of divergence, suggesting a non-genetic (i.e., environmental) influence on DNA methylation (Johnson and Kelly, 2020). Together, these studies provide some evidence of an association between DNA methylation and transcriptomic expression levels; however, the functional consequences of changes in DNA methylation are still largely unknown in bivalves.
In a similar manner, shifts in gene expression are often essential for responding to infectious diseases in bivalves (Renault et al., 2011; Moreira et al., 2012; Rosani et al., 2015). Previous research investigating the transcriptomic responses of C. virginica challenged with Perkinsus marinus found significant changes in the regulation of genes involved in immune defense (Tanguy et al., 2004; Wang et al., 2010). Although previous studies have shown that C. virginica regulates gene expression in response to P. marinus, a major gap remains regarding whether there is a population-specific transcriptomic response to dermo in the northern Gulf of Mexico. Previous research has shown that oyster populations vary in mortality rates in response to dermo, though the molecular basis for this is still unclear (Casas et al., 2017; Leonhardt et al., 2017; La Peyre et al., 2019). A recent study looking at the effect of P. marinus infection on the global gene expression pattern of resistant vs susceptible C. virginica families found strong acute responses to infection with over 3,000 differentially expressed transcripts with resistant oysters upregulating genes involved with peptidase inhibitor activity and regulation of proteolysis (Proestou and Sullivan, 2020). This response diminished over 28 days after which only 21 differentially expressed genes were observed within the dermo resistant family while the dermo susceptible family differentially expressed over 2,000 genes. These large differences in response to infection in controlled settings provide a base to begin exploring how responses to infections differ in the field.
To this end we present a comparison of 40 individuals with differing levels of P. marinus infections following 14 months of outplant at a common-garden field site. We investigated both transcriptomic and epigenetic signatures of these infections to better understand how light vs heavy intensity infections influence molecular phenotypes. Using a combination of reduced representation bisulfite sequencing and 3′ RNA sequencing (TAGseq) we are able to describe two molecular responses to infection intensity in a common garden study. These results also allow us to compare the molecular phenotype of farm-raised oysters with P. marinus infections to recent results from a controlled infection (Proestou and Sullivan, 2020). Through a common garden outplant of hatchery reared juveniles we were able to compare changes in DNA methylation and gene expression for a large number of individuals (n = 40) that were identified as infected with P. marinus, but at varying intensities. This allowed us to use a weighted gene co-expression network analysis to identify a collection of genes positively correlated with infection. Furthermore, we were able to explore how changes in DNA methylation related to changes in gene expression on a gene-by-gene basis.
In May 2016, adult oysters (C. virginica) were collected by dredging from each of two estuaries; Vermilion Bay, LA (29° 35′ 7.26″ N, 91° 0\2′ 33.92″ W) and Calcasieu Lake, LA (29° 50′ 58.02″ N, 93° 17′ 1.32″ W). These oysters were transported to the Louisiana Department of Wildlife and Fisheries Michael C. Voisin Oyster Hatchery in Grand Isle, LA (29° 14′ 20.3″ N, 90° 00′ 11.2″ W) and placed into off-bottom mesh cages for common garden acclimation. In October 2016, after 5 months of acclimation, oysters were spawned at the MCV oyster hatchery. Oyster spat were reared in an upwelling system, individually tagged, and outplanted in one of three adjustable long-line mesh bags at the Grand Isle Hatchery farm on February 20, 2017. Oysters within each bag were monitored for mortality and cleaned of epibionts approximately every 3 months over a 14-month period.
On April 24, 2018, after 14 months at the Grand Isle outplant site, 50 individuals were haphazardly sampled. Shell height of each individual was measured from shell umbo to distal edge using a digital caliper (ABS Coolant Proof Calipers, Mituyoto Corporation, Japan). Approximately 1 cm2 piece of gill tissue was sampled in the field from each individual and preserved with either Invitrogen RNAlater (gene expression) or 95% ethanol (DNA methylation). The remaining whole animal was placed in a pre-weighed 50 ml test tube in order to measure wet meat weight. Infection intensities were enumerated by adding 0.22 μm filtered seawater (20 ppt) at a concentration of ∼0.4 g ml–1 and homogenizing the oyster meat in each 50 ml test tube. One ml of the oyster homogenate was used to measure the number of P. marinus hypnospores g–1 oyster wet tissue using the whole-oyster procedure (La Peyre et al., 2018). Oyster infections were classified as either very-light (≤1,000 parasites g–1 wet tissue), light (1,001–10,000 parasites g–1 wet tissue), moderate (10,001–100,000 parasites g–1 wet tissue) or moderate-heavy (>100,000 parasites g–1 wet tissue). Of the 50 individuals sampled we sequenced 40 individuals that spanned the four different infection categories (Table 1).
Table 1. Distribution of the number of individuals sequenced for each infection category, with both mean infections and range of infections for each category.
DNA was extracted using the OMEGA E.Z.N.A. Tissue DNA Kit (D3396-01; Omega bio-tek) with a 2 min RNase A digestion to remove co-purified RNA. DNA purity was assessed based on 260/280 and 260/230 ratios using a nanodrop spectrophotometer (ND1000; Thermofisher Scientific). Presence of high molecular weight DNA was confirmed using a 1.5% agarose gel, and DNA concentration was verified using a Qubit 3.0 Fluorometric dsDNA BR assay kit (Q32850; Life Technologies). The epiGBS library preparation followed previously published methods (Van Gurp et al., 2016; Johnson and Kelly, 2020). Briefly, a total of 500 ng of purified genomic DNA was double digested using the two frequent cutter enzymes AseI and NsiI (NEB-R0127L and NEB-R0526L; Van Gurp 2016). Digested DNA was ligated to custom y-yoked methylated sequencing adapters using a T4 DNA ligase (B9000S; New England Biolabs) with additional rATP to ensure ligation of custom adapters (Glenn et al., 2019). The adapter ligated DNA was bisulfite converted in a 96 well plate using the Zymo Research EZ DNA Methylation-Lightning kit (D5031; Zymo Research) with a 15 min L-desulphonation step. This bisulfite converted DNA was tagged and amplified with Illumina adapters using 16 cycles of PCR. Amplified libraries were size selected to 300–600 base-pairs (bp) using the Zymo Research Select-A-Size DNA clean & concentrator (D4080; Zymo Research). Size selection was confirmed using the Agilent Bioanalyzer DNA high sensitivity chip (5067-4626; Agilent Technologies). Libraries were pooled and sequenced by NovoGene Inc. (R) with a 10% PhiX spike-in on a full flow cell of the Illumina HiseqX with 100 bp paired-end reads.
The epiGBS sequencing reads were adapter trimmed and base pairs with a phred score less than 30 were removed using Trimmomatic (version 0.39) (Bolger et al., 2014). Trimmed reads were mapped to the reference genome (NCBI GCF_002022765.2) and CpG methylation was called using the software package bismark (v0.19.0) (Krueger and Andrews, 2011). The bismark commands used in the mapping allowed for 1 mismatch in a seed alignment of 10 with a minimum alignment score setting of −0.6 (–score_min L, 0, −0.6). These settings having previously been used in this species and were selected to account for genomic variations between C. virginica collected from the northern Gulf of Mexico (nGOM, this study) and the disease-resistant inbred line from the United States East Coast used for the construction of the reference genome (Gómez-Chiarri et al., 2015; Johnson and Kelly, 2020). CpG methylation was extracted from the non-deduplicated mapped reads using the bismark command bismark_methylation_extractor with the following commands; –ignore_r2 2, –bedGraph, –zero_based, –no_overlap, –cytosine_report, and –report. Differential methylation was conducted on CpG features using the bismark coverage files with methylation across both strands merged and analyzed using the R program MethylKit (v.1.2.4) (Akalin et al., 2012). Methylated regions were identified using a tiled window approach with a tile size of 1,000 bp (1 kb) and a step size of 1 kb. The 1 kb regions were filtered using the filterByCoverage command to require coverage greater than 10× in at least 8 of the 20 individuals.
Pair-wise differential methylation was measured between individuals with moderate or moderate-heavy (parasites g−1>10,000, n = 15) and very-light (parasites g−1≤1,000, n = 16) infection intensities. For these analysis, one sample in the high infection group was not included as a result of poor sequence quality. Significantly differentially methylated regions (DMRs) were identified based on a minimum percent methylation difference between groups of 20% and an adjusted P-value (q-value) less than or equal to 0.05 (Mathers et al., 2019). Functional enrichment of methylation was assessed using two methods. The first approach explored enrichment in methylation amongst all samples tested using a Mann-Whitney U-test to identify enriched ontologies between highly and lowly methylated genes. This test used mean methylation among all individuals for each gene feature with at least a single 1 kb region overlapping a gene’s promoter, gene body or downstream region; and, calculated enrichment across each gene ontology (GO) category (MF, Molecular Function; BP, Biological Process, and CC, Cellular Component). The second approach tested for functional enrichment between genes that showed differentially methylated regions using a Fisher’s Exact test for each GO category. For both analyses we used a background list consisted of all genes with GO annotations for which DNA methylation was measured (n = 8,624 of genes passing filter with GO annotations).
Total RNA was extracted using a E.Z.N.A.® Total RNA Kit I (Omega BIO-TEK Inc., Norcross, GA, United States) following the manufacturer’s instructions. The yield and quantity were initially assessed using a NanoDrop 2000 spectrophotometer. Total RNA extracted from the 40 individuals was sent to the University of Texas at Austin’s Genomic Sequencing and Analysis Facility where RNA quality control was confirmed using a 2100 Agilent Bioanalyzer on a Eukaryote Total RNA Nano chip and libraries were produced using the 3′ poly-A-directed mRNA-sequencing (TAGseq) method (Meyer et al., 2011). The resulting 40 libraries were sequenced on two lanes of an Illumina HiSeq 2500 platform, with 100 base pair single-end reads. Sequencing reads were trimmed of adapter sequences using Trimmomatic (version 0.39) (Bolger et al., 2014) and base pairs with quality scores below 30 were removed. The trimmed reads were then mapped to the published C. virginica reference genome (Gómez-Chiarri et al., 2015) using the single pass option for STAR RNA-seq aligner (version 2.6.0a) (Dobin et al., 2012). We used the default of 10 allowed mismatches for filtering and allowed for multi-mapping. After mapping, we used HTSeq (version 0.11.2) (Anders et al., 2015) to obtain the number of reads mapped to each gene and allowed for multiple alignments to be counted (–non-unique = all). The counts were sorted by alignment position and based on gene features obtained from the C. virginica genome assembly on NCBI (version GCF_002022765.2).
Changes in gene expression associated with P. marinus infection intensity were tested with two approaches. The first approach assessed pairwise changes in gene expression between individuals with moderate-heavy and very-light infection using the package edgeR (version 3.24.2) (Robinson et al., 2010). For this analysis genes with fewer than three counts per million mapped reads across 50% (n = 20) of all samples were removed. The remaining read counts were distributed across 17,439 gene features and were normalized using the trimmed mean of M-values (TMM) normalization method (Robinson and Oshlack, 2010). Broad changes in gene expression were first assessed using a principal coordinate analysis (PCoA) conducted using the R program vegan with Euclidean distances calculated from log2 + 1 transformed normalized counts obtained from the cpm() function in edgeR. These log-transformed counts were also used to test for any significant interactions between gene expression and infection intensity interactions using a PERMANOVA with 1e6 permutations. Pairwise differential expression was measured using the genewise negative binomial generalized linear model implemented in the edgeR function glmFit and significantly differentially expressed genes (DEGs) were identified based on FDR rates calculated using benjamini-hochberg method (Benjamini and Hochberg, 1995). Comparisons were made between the lowest infection group (very-light, n = 16) and a combination of the two highest infection groups (moderate and moderate-high, n = 16). Functional enrichment of differential gene expression for each comparison was tested using a rank-based gene ontology analysis with adaptive clustering that uses a Mann-Whitney U-Test to identify enriched ontologies (Wright et al., 2015). For these tests, we tested for enrichment using the log-transformed and signed p-value for each gene in each comparison contrasted using all of the genes that passed the expression filtering for the analysis and had annotated GO terms (n = 11,057).
The second approach used to assess changes in gene expression associated with P. marinus infection intensity was a Weighted Gene Network Analysis (WGCNA). For this component of the analysis we restricted the number of genes to remove lowly expressed features retaining only samples with greater than five counts per million in 75% of all samples (n = 30). This additional filtering was included to remove genes with low counts prior to network analysis. WGCNA was run using the 11,998 genes that passed this filter, a soft-threshold of 12, a minimum module size of 30, a signed adjacency matrix, and was correlated to shell height, meat weight, and infection intensity (a continuous variable of counts/g). Functional enrichment was assessed across 7,398 of the 11,998 genes that had annotated GO terms for the five modules that showed significant correlation with any of the five traits using the GO_MWU methods for WGCNA using eigengene-based module connectivity (kME) as a continuous variable. This method calculates significant enrichment across each gene ontology (GO) category (MF, BP, and CC) for each module, and identifies functional categories in the modules using the Fisher’s Exact test. These functional categories were further tested for an association with higher kME values using a within-module MWU test. The product of the resulting p-values from the Fisher’s Exact and MWU test was then used to calculate false discovery rates with ten permutations of randomly shuffled significance measures among genes.
An important component of this study was the opportunity to examine the interaction between DNA methylation and transcript expression across many individuals. We tested the relationship between percent methylation for moderate-heavy infected individuals vs very-lightly infected individuals and changes in gene expression, as measured by the pairwise differential gene expression analysis. To do this, we summarized each 1 kb region into three categories: 1 kb regions that were located in (i) gene promoter regions (within 2 kb upstream of the first exon), (ii) gene body regions (first exon to last exon), or (iii) downstream regions (within 2 kb downstream of the last exon). For all comparisons if multiple 1 kb regions were present within the annotated feature (promoter, gene body, or downstream region) the mean p-value, mean q-value, and mean percent difference in methylation was calculated. For each of these three gene regions we explored the association of mean methylation with the log-transformed cpm expression data. For these analyses cpm expression data was distributed into 10 deciles using the decile function available in the R package StatMeasures (v.1.0). Significance of the association between mean methylation and expression level deciles was assessed using a Kruskal–Wallis test along with a Dunn post hoc test and corrected for multiple comparisons using the Benjamini-Hochberg method.
RRBS sequencing produced a total of 878 million reads with an average of 21.4 million reads per sample. Trimming of these reads led to an average of 18.1 million reads per sample, and mapping resulted in an average of 84.6% of reads mapping to the reference genome (range: 77.3–89.1%). Genome tiling in methylkit distributed these reads across 43,085 1-kb regions with a minimum count of 10 in a minimum of 9 individuals in either of the 2 groups (very-light or moderate and moderate-heavy). Of these, 10,741 regions were located along intergenic regions (i.e., greater than 2 kb from any gene feature). The remaining 32,344 1-kb regions were distributed across 15,543 annotated gene features with 13.1% (n = 2,364 genes) found within promoter regions (i.e., 2 kb upstream of first exon), 71.3% (n = 12,781 genes) found within gene bodies, and 15.6% (n = 2,791 genes) found within 2 kb downstream of a gene’s final exon. Functional enrichment testing using the rank-based gene ontology analysis with adaptive clustering among all 40 individuals based on mean methylation identified enriched gene functions among both heavily- and lightly methylated genes (relative to the genome-wide average) in each of the three gene ontology categories (14 MF, 6 BP, and 11 CC). We observed higher methylation of key molecular processes such as RNA metabolism, chromosome organization, and general protein binding. While lightly methylated genes were associated with environmentally responsive categories such as enzyme regulation, immune processes and oxidoreductase activity (Table 2).
Table 2. Top results from Mann Whitney U-Test enriched gene ontology (GO) terms tested using percent methylation of gene body.
Our pair-wise assessments of differential methylation between the moderately and moderate-heavily and lightly infected groups identified 913 significantly differentially methylated 1-kb regions (DMRs), distributed across 846 genes. Among these, 86 DMRs (10.1%) were found within promoter regions, 666 DMRs (78.8%) were found within gene bodies, and 66 DMRs (8%) were found in downstream regions. Functional enrichment using a Fisher’s Exact test for each region (i.e., promoter, gene body, and downstream) did not identify any significant enrichment for hyper/hypo-methylated genes or gene body regions. Examining genes that showed the strongest change in methylation between infection categories identified significant hypomethylation of a uncharacterized long non-coding RNA (−58%), a complement C1q tumor necrosis factor-related protein 4-like gene (−36.5%), a heat shock 70 kDa protein 12A-like isoform (−29.25%), and a caspase-8-like gene (−27.65%) in individuals with higher levels of infection. Gene-body hypermethylation in more infected individuals occurred in UPF0600 protein c5orf51 homolog (+65.1%), two uncharacterized long non-coding RNAs (+52.2%; +48.1%), an organic cation transporter protein (+44.9%), and an ankyrin-2-like isoform (+44.9%).
Transcriptome sequencing using TAGseq produced a total of 189 million reads, with 4.7 million reads per sample. Trimming of those reads led to a final read count of 4.5 million per sample, which is sufficient for this method (Meyer et al., 2011). Star mapping resulted in 80.20% of reads mapping to the reference genome (range: 75.7–83.1%). Filtering mapped reads based on expression resulted in a total of 17,439 gene features with more than 3 counts per million reads in 20 of the 40 samples. This stringent level of filtering was chosen to avoid including too many genes with low count totals. Principle coordinate analysis revealed significant overlap with the three low-infection groups with separation of these from the single high infection group (Figure 1). The PERMANOVA testing expression ∼ infection (parasites g−1 wet tissue wet) found a non-significant interaction between infection and gene expression (p-value = 0.09).
Figure 1. Biplot of the first two principle components from principle coordinate analysis using the gene expression data.
Pairwise differential gene expression analysis identified modest levels of differential gene expression between infection intensities. Gene expression changes associated with moderate-heavy vs very-light infections, identified 31 genes up-regulated and 8 genes down-regulated in the moderate-heavy infected individuals (Figure 2). A Mann-Whitney U-Test of the 11,057 genes in the analysis (regardless of DE status) was run using the log-transformed and signed p-values for genes that passed filtering for the analysis and had annotated GO terms (n = 11,057). This analysis identified 19 enriched categories associated with infection intensity including G protein-coupled receptor activity, ribonucleoprotein complex biogenesis, and peptide metabolic process (Table 3). There were three genes significantly differentially expressed (FDR < 0.05) that had predicted enzyme annotations, these genes include a GTP-binding protein GEM-like enzyme (logFC = −1.6; gene18819), a gene coding for a hepatic-like arginase (logFC = + 0.7; gene13511), and a gene coding for uncharacterized protein LOC111128066 that has been functionally annotated as an endonuclease (logFC = +1.5; gene13511). There were five additional genes that were differentially expressed and have previously been found to respond to infection in marine invertebrates (de Lorgeril et al., 2005; Proestou and Sullivan, 2020). These included nijurin 1-like (logFC = +2.1;gene24478); phytanoyl-CoA dioxygenase domain-containing protein 1-like (logFC = +3.0; gene6975), C1q tumor necrosis factor protein 6-like (logFC = −2.6; gene30362), C1q tumor necrosis factor protein protein 7-like (logFC = +2.6; gene10222), a GATA zinc finger domain containing protein 14 (logFC = −6.0; gene 5837), and a nuclear polyadenylated RNA-binding protein 3-like gene (logFC = −3.2; gene5505).
Figure 2. Heatmap and hierarchical clustering of the 39 differentially expressed transcripts between heavily and lightly infected individuals. Top color-coded bar provides individual infection levels with: light blue = very-light infection, blue = light infection, pink = moderate infection, and red = moderate-heavy infection.
Table 3. Top enriched GO terms for each GO from genes that were differentially expressed between the moderate-heavy and very-light infection intensities.
The WGCNA analysis identified a total of 12 gene modules; of these, only 1 module was significantly correlated with dermo infection intensity (Figure 3A). Below we discuss the significance of module pink as this module was the only one significantly associated with infection.
Figure 3. (A) Weighted Gene Correlated Network Analysis, showing significant relationships between module eigengenes (rows) and oyster traits (columns) in which the color scale (red-blue) represents the strength of the correlation (1 to −1). Only modules with significant correlations to one of the traits are listed. (B) Mean eigengene expression levels for genes in module pink across the four infection categories, showing increase expression of module pink among the moderate-heavy infected individuals. (C) Enriched gene ontology terms for genes in module pink. Percent of reference shows the number of genes identified as enriched when compared to the total number of genes for that GO term in the background reference list.
Module Pink was comprised of 385 transcripts and was significantly associated with increased intensities of dermo infection. These genes showed a positive correlation between dermo infection and expression levels with the highest infection group showing the strongest association with the module (Figure 3B). Of the 24 genes with the highest module membership scores (KmE > 0.75), 2 have been identified in other studies as being involved in immunity in oysters (Wang et al., 2010; Li et al., 2017). In addition, module pink was found to also contain isoform 1 of the ninjurin-1-like gene that was also identified as differentially expressed in the pairwise comparison. Functional enrichment of this module identified enrichment of supramolecular fiber organization, intracellular, and cellular carbohydrate metabolic process. Comparing the list of genes in module Pink and genes identified as differentially expressed in Proestou and Sullivan (2020) identified 16 genes in both datasets. These genes included two isoforms of a multimerin-2-like gene, a calmodulin-2/4-like gene, a HSP70 12A-like gene, and a proline-rich transmembrane protein. This module was also found to have significant GO enrichment for 8 MF terms, 13 BP terms, and 8 CC terms (Figure 3C).
We examined the relationship between gene expression and methylation for gene promoters (2 kb up-stream of first exon), gene bodies, downstream regions (2 kb down stream of last exon), and all intragenic regions combined (e.g., region covered by promoter, gene body, and downstream region). After breaking the expression into deciles, we used a Kruskal–Wallis test to compare mean methylation of each decile. This analysis identified a near-significant association between increasing expression and increased promoter methylation (p-value = 0.065), a significant positive interaction association between expression and gene body methylation (p-value < 2.2e–16; Figure 4A), and no interaction association between expression and downstream methylation (p-value = 0.11). We also explored the relationship between the mean methylation and the coefficient of gene expression variation for each gene calculated across all samples. This analysis identified a significant relationship between decreasing methylation and increasing variation in gene expression (p-value < 2.2e–16; Figure 4B). However, there was only significant overlap between 2 DMRs and 2 DEGs and a Fisher’s Exact test revealed no significant overlap between differential expression and differential methylation (p-value > 0.05).
Figure 4. Violin plots of interaction between gene body methylation and gene expression for 5,295 genes with both gene body methylation and expression data. Panel (A) plots mean methylation vs normalized counts per million (CPM) expression levels broken into 10 equal sized deciles, and panel (B) plots mean methylation vs the coefficient of variation (CV) in expression also broken into 10 equal sized deciles. Letters represent significant differences in mean methylation between deciles calculated separately for CPM and CV of expression.
Our study described how P. marinus infection intensities influence DNA methylation and gene expression in the eastern oyster C. virginica. Through a combination of reduced representation DNA methylation sequencing and 3′-RNA sequencing (TAGseq) we identified significant changes in DNA methylation and subtle changes in gene expression that support previous findings of transcriptomic responses to infection and confirms the relationship between percent DNA methylation and the magnitude and variation in gene expression. The ability to assess these changes across multiple individuals (n = 40) strengthened these observations and encourages the use of gene body percent methylation as a proxy for expression and reinforces a potential role for plasticity. However, this study did not find compelling evidence for a relationship between differential methylation and differential expression. This lack of overlap suggests that the majority of changes in DNA methylation in response to infection might occur at any level of infection (i.e., all individuals are exhibiting abroad infection methylome); or, that these changes in DNA methylation are only necessary for acute responses that gradually return to either a seasonally or environmentally responsive methylation pattern. The lack of evidence for this direct connection may derive from the limited knowledge of when infection was initiated, and mechanisms governing changes to gene body methylation. These data suggest much of the observed variation in methylation does not reliably predict the direction of differential gene expression. This observation however, may also be a reflection of the TAGseq approach that is unable to identify changes in splice variant expression. It is therefore quite possible that the changes in DNA methylation are not associated with changes in expression at the gene level, but these changes could be influencing expression at the isoform level.
When we then look at the ontologies enriched in the genes that were differentially methylated between the moderate-heavy vs very-light infection categories, we see the regions that were hypomethylated in more heavily infected individuals were enriched for immune response genes, oxidative stress genes, and enzyme regulator activity. The hypomethylation of these regions may increase the plasticity of these genes given that lower methylation was also associated with greater variability in expression in our genome-wide analysis. That this occurs as a function of infection provides some evidence that the plasticity of these genes is being promoted. In contrast, regions hypermethylated in infected individuals were enriched for broad organizational categories associated with cellular structure and replication. If hypermethylation leads to canalization of expression, then it is possible that the increase in percent methylation of the gene bodies reinforces the stability of expression among these genes that may otherwise be altered by the activation of immune responses. The hypomethylation in heavily infected individuals of genes involved in apoptosis (caspase-8), pathogen clearance (C1q tumor necrosis factor-related protein 4), and molecular chaperones (HSP 70 12A-like), suggests that moderate-heavy infections may be promoting higher plasticity of immune response genes (de Lorgeril et al., 2011; Wang et al., 2018). These potential roles are still largely based on the hypothesis that large changes in DNA methylation will drive phenotypic responses. As this still remains untested in bivalves, the community would greatly benefit from controlled studies investigating changes in DNA methylation across time in the same individuals.
Differential gene expression analysis identified only 39 genes that showed differential expression between the moderate-heavy (>100,000 parasites g−1) and very-light infected (≤1,000 parasites g−1) individuals (Figure 2). This low level of differential gene expression is comparable to the dampened response (21 genes DEG) observed in a dermo resistant family when measured 28-days post-exposure in a controlled experiment (Proestou and Sullivan, 2020). The genes that were differentially expressed in both studies (day 28; Proestou and Sullivan, 2020) included 2 forms of the complement C1q tumor necrosis factor (protein 6-like and protein 7-like) and a nuclear polyadenylated RNA-binding protein 3-like. The fact that these two datasets provide similar findings of a small number of genes being differentially expressed suggests two major takeaways; (1) that the individuals observed in this study were likely suffering from long-term infections of P. marinus; and, (2) that the shared differential expression of genes associated with tumor necrosis factors (complement system C1q) means these genes in particular are good candidates for further investigation as potential markers of disease resistance. Assuming that the individuals were suffering from long-term infections is consistent with other observed infection rates at this out-plant site (La Peyre et al., 2018). For the genes identified by both our study and the previous controlled exposure (complement C1q tumor necrosis factor-related protein 7-like and multimerin-2-like) we see an approximate sixfold increase in expression between the moderate-heavy and very-light infection categories. The role of these genes in immune responses is further supported by recent assessments of the functional role of these gene families in bivalve innate immunity (Gerdol et al., 2019). We also observed two versions of a ninjurin 1-like gene that were both up-regulated in the moderate-heavy infected individuals. Ninjurin-like genes have been found to be upregulated in Pacific Blue Shrimp (Litopenaeus stylirostris) that survive infection with Vibrio penaeicidia (de Lorgeril et al., 2005). We also found strong upregulation of two forms of a phytanoyl-CoA dioxygenase domain-containing protein 1-like that is putatively involved in peroxisomal lipid metabolism, a potential signal of a prolonged immune response in Manila clams (Venerupis philippinarum) responding to Perkinsus olseni (Romero et al., 2015). Together, these differential expression data highlight potential gene targets for future selective breeding programs aimed at increasing disease resistance.
WGCNA identified 1 module that was significantly correlated with dermo infection (module pink). Comparing the genes in module pink with the results from Proestou and Sullivan (2020) found 18 genes that were identified as DE after 28 days of infection in the dermo susceptible group and 3 genes identified as DE after 28 days in the dermo resistant group in their study. Of these genes the multimerin-2-like gene appears to be associated with angiogenesis and tumor formation (Khan et al., 2017). The positive association of infection of this gene within module Pink may suggest a role of angiogenesis in healing tissues degraded by P. marinus secreted proteases (La Peyre et al., 1995). In addition, multimerin-2-like genes have been proposed to have similar domains to complement component C1q genes (Gerdol et al., 2019) that were significantly differentially expressed in the above pairwise analysis of gene expression. In addition, two genes within this module, Thymosin beta-4 and kelch-like protein, have been associated with immune defense against pathogens, Thymosin beta-4 is also involved in antibacterial activity in C. gigas (Nam et al., 2015) and was found to facilitate the clearance of Vibrio alginolyticus in C. hongkongensi (Li et al., 2017). Kelch-like proteins have also been shown to be differentially regulated in C. virginica when challenged with dermo (Wang et al., 2010). Our results show that both of these genes are up-regulated as P. marinus infection intensity increases. These findings suggest a potential role for both genes in the immune response of C. virginica infected with dermo. However, further studies are needed to clarify the role these genes play in defending against dermo infection in oysters. This overlap between groups further supports the module pink as a gene network involved in immune responses and provides additional gene targets for better understanding the variability in susceptibility to P. marinus across oyster families. These results also further support the use of weighted gene co-expression network analysis for exploring genomic patterns of expression for disease resistance in marine invertebrates.
Global levels of DNA methylation across gene features revealed some unexpected associations, with limited support for a role of methylation among promoter and downstream regions on gene expression levels. Previous research in the Pacific oyster has found evidence for a putative role of promoter methylation in regulating gene expression during development (Rivière et al., 2013; Rivière, 2014). In our study, we tested this directly and found a nearly significant association (p-value = 0.065) between gene expression and mean promoter methylation. This lack of significant association is surprising, and it is possible that these differences in observations maybe associated with sampling during development (Rivière et al., 2013) or that only some genes are influenced by promoter methylation leading to a nearly significant result. Other more recent studies have reported that methylation increases at the start of the gene body and not upstream in promoter regions (Song et al., 2017). Rather, gene body methylation showed a strong positive correlation with gene expression (Figure 4), a finding that has been previously reported in the Pacific oyster (Gavery and Roberts, 2013; Olson and Roberts, 2014). In our study we found that genes with higher mean methylation have higher overall expression and a lower coefficient of variation of expression. When comparing mean methylation with gene expression, we found that there were four categories (Figure 4A) of mean methylation associated with increasing gene expression, but, eight categories (Figure 4B) of mean methylation associated with increasing variation in expression. This suggests that mean methylation is a better predictor of gene variation than it is of the magnitude of gene expression. These results align well with previous research in Pacific oyster where it has been proposed that higher levels of gene body methylation are associated with constitutively expressed genes while lower levels of gene body methylation are associated with “environmentally responsive” gene categories (Roberts and Gavery, 2012; Song et al., 2017). As such, it is plausible that genes showing a decrease in gene body methylation (hypomethylated) are modified in a manner that may lead to an increase in plasticity of that gene. This potential increase in plasticity will require additional studies, ideally consisting of time-course observations of a small number of individuals in order to determine if an environmentally induced change in methylation will lead to future changes in the plasticity of that gene. In addition, future studies should further investigate the role of differential methylation on alternative splicing events. Regardless, these data suggest a relatively minor role of differential DNA methylation on observed differences in gene expression among individuals experiencing chronic exposure to infections.
This study sought to understand how chronic exposure to sub-lethal infections with the protistan parasite P. marinus influenced DNA methylation and gene expression in eastern oysters. Our results have confirmed a relationship between DNA methylation and gene expression such that lower levels of DNA methylation are found among genes with higher variations in expression, while highly methylated genes are found to have higher but less variable constitutive levels of expression. Genes with low gene body methylation included those involved in immune system process and enzyme regulator activity. Gene expression analyses found a limited response to infection, similar to the differential expression levels seen among dermo resistant families after 28 days of exposure (Proestou and Sullivan, 2020). The concordant differential expression results between ours and this previous study for C1q tumor necrosis factor genes suggests additional studies should explore the function of these genes in infection resistance. Finally, the significant but limited overlap in genes showing differential methylation and differential expression is a potential indicator of a limited influence of changes in methylation on changes in expression.
The datasets generated for this study can be found in the NCBI SRA database under BioProject PRJNA604121.
MK and JL conceived of and designed the outplant study. KJ, MK, JL, SC, and KS sampled the animals for this study. SC and JL measured infection intensities. KJ prepared RRBS libraries and performed differential methylation analysis. KS and KJ analyzed the transcriptomic data and wrote the manuscript with input from SC, JL, and MK. All authors contributed to the article and approved the submitted version.
This research was supported by NSF-BioOCE 1731710 to MK and JL, Louisiana Sea Grant award NA14OAR4170099 to MK and JL, and the Alfred P. Sloan Foundation research fellowship awarded to MK. During the project, KJ was supported by an NSF Postdoctoral Fellowship in Biology under Grant No. 1711319; and, KS was supported by an NSF Graduate Research Fellowship under Grant No. 00001414. Portions of this research were conducted with high performance computing resources provided by Louisiana State University (http://www.hpc.lsu.edu).
The authors declare that the research was conducted in the absence of any commercial or financial relationships that could be construed as a potential conflict of interest.
We thank the Louisiana Department of Fisheries and Wildlife, Dr. John Supan, and Dr. Brian Callam for collecting and spawning the oysters used in this study. We would also like to thank Bennett Thomas and Michelle Gautreaux for their assistance in RNA extractions, Joanna Griffiths for feedback on data analysis. Finally, we thank Alicia Reigel and Megan Guidry for assistance in sampling the oysters used in this study.
The Supplementary Material for this article can be found online at: https://www.frontiersin.org/articles/10.3389/fmars.2020.00598/full#supplementary-material
Akalin, A., Kormaksson, M., Li, S., Garrett-Bakelman, F. E., Figueroa, M. E., Melnick, A., et al. (2012). MethylKit: a comprehensive R package for the analysis of genome-wide DNA methylation profiles. Genome Biol. 13, R87–R87. doi: 10.1186/gb-2012-13-10-r87
Alvarez, M., Schrey, A. W., and Richards, C. L. (2015). Ten years of transcriptomics in wild populations: what have we learned about their ecology and evolution? Mol. Ecol. 24, 710–725. doi: 10.1111/mec.13055
Anders, S., Pyl, P. T., and Huber, W. (2015). HTSeq–a Python framework to work with high-throughput sequencing data. Bioinformatics 31, 166–169. doi: 10.1093/bioinformatics/btu638
Benjamini, Y., and Hochberg, Y. (1995). Controlling the false discovery rate: a practical and powerful approach to multiple testing. J. R. Stat. Soc. Ser. B 57, 289–300. doi: 10.1111/j.2517-6161.1995.tb02031.x
Bolger, A. M., Lohse, M., and Usadel, B. (2014). Trimmomatic: a flexible trimmer for Illumina sequence data. Bioinformatics 30, 2114–2120. doi: 10.1093/bioinformatics/btu170
Casas, S., Walton, W., Chaplin, G., Rickards, S., Supan, J., and La Peyre, J. (2017). Performance of oysters selected for dermo resistance compared to wild oysters in northern Gulf of Mexico estuaries. Aquac. Environ. Interact. 9, 169–180. doi: 10.3354/aei00222
Clark, M. S., Thorne, M. A. S., King, M., Hipperson, H., Hoffman, J. I., and Peck, L. S. (2018). Life in the intertidal: cellular responses, methylation and epigenetics. Funct. Ecol. 32, 1982–1994. doi: 10.1111/1365-2435.13077
de Lorgeril, J., Saulnier, D., Janech, M. G., Gueguen, Y., and Bachere, E. (2005). Identification of genes that are differentially expressed in hemocytes of the Pacific blue shrimp (Litopenaeus stylirostris) surviving an infection with Vibrio penaeicida. Physiol. Genom. 21, 174–183. doi: 10.1152/physiolgenomics.00281.2004
de Lorgeril, J., Zenagui, R., Rosa, R. D., Piquemal, D., and Bachère, E. (2011). Whole transcriptome profiling of successful immune response to Vibrio infections in the oyster Crassostrea gigas by digital gene expression analysis. PLoS One 6:e23142. doi: 10.1371/journal.pone.0023142
De Wit, P., Durland, E., Ventura, A., and Langdon, C. J. (2018). Gene expression correlated with delay in shell formation in larval Pacific oysters (Crassostrea gigas) exposed to experimental ocean acidification provides insights into shell formation mechanisms. BMC Genom. 19:160. doi: 10.1186/s12864-018-4519-y
Dixon, G. B., Bay, L. K., and Matz, M. V. (2014). Bimodal signatures of germline methylation are linked with gene expression plasticity in the coral Acropora millepora. BMC Genom. 15:1109. doi: 10.1186/1471-2164-15-1109
Dobin, A., Davis, C. A., Schlesinger, F., Drenkow, J., Zaleski, C., Jha, S., et al. (2012). STAR: ultrafast universal RNA-seq aligner. Bioinformatics 29, 15–21. doi: 10.1093/bioinformatics/bts635
Eirin-Lopez, J. M., and Putnam, H. M. (2019). Marine environmental epigenetics. Annu. Rev. Mar. Sci. 11, 335–368. doi: 10.1146/annurev-marine-010318-095114
Gavery, M. R., and Roberts, S. B. (2013). Predominant intragenic methylation is associated with gene expression characteristics in a bivalve mollusc. PeerJ 1:e215. doi: 10.7717/peerj.215
Gavery, M. R., and Roberts, S. B. (2017). Epigenetic considerations in aquaculture. PeerJ 5:e4147. doi: 10.7717/peerj.4147
Gerdol, M., Greco, S., and Pallavicini, A. (2019). Extensive tandem duplication events drive the expansion of the C1q-domain-containing gene family in bivalves. Mar. Drugs 17:583. doi: 10.3390/md17100583
Glenn, T. C., Nilsen, R. A., Kieran, T. J., Sanders, J. G., Bayona-Vásquez, N. J., Finger, J. W., et al. (2019). Adapterama I: universal stubs and primers for 384 unique dual-indexed or 147,456 combinatorially-indexed Illumina libraries (iTru & iNext). PeerJ 7:e7755. doi: 10.7717/peerj.7755
Gómez-Chiarri, M., Warren, W. C., Guo, X., and Proestou, D. (2015). Developing tools for the study of molluscan immunity: the sequencing of the genome of the eastern oyster, Crassostrea virginica. Fish Shellf. Immunol. 46, 2–4. doi: 10.1016/j.fsi.2015.05.004
Hawes, N. A., Fidler, A. E., Tremblay, L. A., Pochon, X., Dunphy, B. J., and Smith, K. F. (2018). Understanding the role of DNA methylation in successful biological invasions: a review. Biol. Invasions 20, 2285–2300. doi: 10.1007/s10530-018-1703-6
Herman, J. J., and Sultan, S. E. (2016). DNA methylation mediates genetic variation for adaptive transgenerational plasticity. Proc. R. Soc. B Biol. Sci. 283:20160988. doi: 10.1098/rspb.2016.0988
Johnson, K. M., and Kelly, M. W. (2020). Population epigenetic divergence exceeds genetic divergence in the Eastern oyster Crassostrea virginica in the Northern Gulf of Mexico. Evolut. Appl. 13, 945–959. doi: 10.1111/eva.12912
Jones, H. R., Johnson, K. M., and Kelly, M. W. (2019). Synergistic effects of temperature and salinity on the gene expression and physiology of Crassostrea virginica. Integr. Comparat. Biol. 59, 306–319. doi: 10.1093/icb/icz035
Kappeler, L., and Meaney, M. J. (2010). Epigenetics and parental effects. Bioessays 32, 818–827. doi: 10.1002/bies.201000015
Khan, K., Naylor, A., Khan, A., and Mohammed, F. (2017). Multimerin-2 is a ligand for group 14 family C-type lectins CLEC14A, CD93 and CD248 spanning the endothelial pericyte interface. Oncogene 36, 6097–6108. doi: 10.1038/onc.2017.214
Krueger, F., and Andrews, S. R. (2011). Bismark: a flexible aligner and methylation caller for Bisulfite-Seq applications. Bioinformatics 27, 1571–1572. doi: 10.1093/bioinformatics/btr167
La Peyre, J. F., Casas, S. M., Richards, M., Xu, W., and Xue, Q. G. (2019). Testing plasma subtilisin inhibitory activity as a selective marker for dermo resistance in eastern oysters. Dis. Aquat. Organ. 133, 127–139. doi: 10.3354/dao03344
La Peyre, J. F., Casas, S. M., and Supan, J. E. (2018). Effects of controlled air exposure on the survival, growth, condition, pathogen loads and refrigerated shelf life of eastern oyster. Aquac. Res. 49, 19–29. doi: 10.1111/are.13427
La Peyre, J. F., Schafhauser, D. Y., Rizkalla, E. H., and Faisal, M. (1995). Production of serine proteases by the oyster pathogen Perkinsus marinus (Apicomplexa) in vitro. J. Eukaryot. Microbiol. 42, 544–551. doi: 10.1111/j.1550-7408.1995.tb05903.x
Leonhardt, J. M., Casas, S., Supan, J. E., and La Peyre, J. F. (2017). Stock assessment for eastern oyster seed production and field grow-out in Louisiana. Aquaculture 466, 9–19. doi: 10.1016/j.aquaculture.2016.09.034
Li, A., Li, L., Song, K., Wang, W., and Zhang, G. (2017). Temperature, energy metabolism, and adaptive divergence in two oyster subspecies. Ecol. Evol. 7, 6151–6162. doi: 10.1002/ece3.3085
Mathers, T. C., Mugford, S. T., Percival-Alwyn, L., Chen, Y., Kaithakottil, G., Swarbreck, D., et al. (2019). Sex-specific changes in the aphid DNA methylation landscape. Mol. Ecol. 28, 4228–4241. doi: 10.1111/mec.15216
Meyer, E., Aglyamova, G. V., and Matz, M. V. (2011). Profiling gene expression responses of coral larvae (Acropora millepora) to elevated temperature and settlement inducers using a novel RNA-Seq procedure. Mol. Ecol. 20, 3599–3616.
Moreira, R., Balseiro, P., Planas, J. V., Fuste, B., Beltran, S., Novoa, B., et al. (2012). Transcriptomics of in vitro immune-stimulated hemocytes from the Manila clam Ruditapes philippinarum using high-throughput sequencing. PLoS One 7:e35009. doi: 10.1371/journal.pone.0035009
Nam, B. H., Seo, J. K., Lee, M. J., Kim, Y. O., Kim, D. G., An, C. M., et al. (2015). Functional analysis of Pacific oyster (Crassostrea gigas) β-thymosin: focus on antimicrobial activity. Fish Shellf. Immunol. 45, 167–174. doi: 10.1016/j.fsi.2015.03.035
Olson, C. E., and Roberts, S. B. (2014). Genome-wide profiling of DNA methylation and gene expression in Crassostrea gigas male gametes. Front. Physiol. 5:224. doi: 10.3389/fphys.2014.00224
Proestou, D. A., and Sullivan, M. E. (2020). Variation in global transcriptomic response to Perkinsus marinus infection among eastern oyster families highlights potential mechanisms of disease resistance. Fish Shellf. Immunol. 96, 141–151. doi: 10.1016/j.fsi.2019.12.001
Putnam, H. M., Davidson, J. M., and Gates, R. D. (2016). Ocean acidification influences host DNA methylation and phenotypic plasticity in environmentally susceptible corals. Evolut. Appl. 9, 1165–1178. doi: 10.1111/eva.12408
Renault, T., Faury, N., Barbosa-Solomieu, V., and Moreau, K. (2011). Suppression substractive hybridisation (SSH) and real time PCR reveal differential gene expression in the Pacific cupped oyster, Crassostrea gigas, challenged with Ostreid herpesvirus 1. Dev. Comp. Immunol. 35, 725–735. doi: 10.1016/j.dci.2011.02.004
Rivière, G. (2014). Epigenetic features in the oyster Crassostrea gigas suggestive of functionally relevant promoter DNA methylation in invertebrates. Front. Physiol. 5:129. doi: 10.3389/fphys.2014.00129
Rivière, G., Wu, G. C., Fellous, A., Goux, D., Sourdaine, P., and Favrel, P. (2013). DNA methylation is crucial for the early development in the Oyster C. gigas. Mar. Biotechnol. 15, 739–753. doi: 10.1007/s10126-013-9523-2
Roberts, S. B., and Gavery, M. R. (2012). Is there a relationship between DNA methylation and phenotypic plasticity in invertebrates? Front. Physiol. 2:116. doi: 10.3389/fphys.2011.00116
Robinson, M. D., and Oshlack, A. (2010). A scaling normalization method for differential expression analysis of RNA-seq data. Genome Biol. 11:R25. doi: 10.1186/gb-2010-11-3-r25
Robinson, M. D., McCarthy, D. J., and Smyth, G. K. (2010). edgeR: a Bioconductor package for differential expression analysis of digital gene expression data. Bioinformatics 26, 139–140. doi: 10.1093/bioinformatics/btp616
Romero, A., Forn-Cuní, G., Moreira, R., Milan, M., Bargelloni, L., Figueras, A., et al. (2015). An immune-enriched oligo-microarray analysis of gene expression in Manila clam (Venerupis philippinarum) haemocytes after a Perkinsus olseni challenge. Fish Shellf. Immunol. 43, 275–286. doi: 10.1016/j.fsi.2014.12.029
Rosani, U., Varotto, L., Domeneghetti, S., Arcangeli, G., Pallavicini, A., and Venier, P. (2015). Dual analysis of host and pathogen transcriptomes in ostreid herpesvirus 1-positive Crassostrea gigas. Environ. Microbiol. 17, 4200–4212. doi: 10.1111/1462-2920.12706
Rubi, T. L., Knowles, L. L., and Dantzer, B. (2019). Museum epigenomics: characterizing cytosine methylation in historic museum specimens. Mol. Ecol. Resour. 2019, 1–10. doi: 10.1111/1755-0998.13115
Ryu, T., Veilleux, H. D., Donelson, J. M., Munday, P. L., and Ravasi, T. (2018). The epigenetic landscape of transgenerational acclimation to ocean warming. Nat. Clim. Chang. 8, 504–509. doi: 10.1038/s41558-018-0159-0
Schmid, M. W., Heichinger, C., Coman Schmid, D., and Grossniklaus, U. (2018). Contribution of epigenetic variation to adaptation in Arabidopsis. Nat. Commun. 9:4446. doi: 10.1038/s41467-018-06932-5
Song, K., Li, L., and Zhang, G. (2017). The association between DNA methylation and exon expression in the Pacific oyster Crassostrea gigas. PLoS One 12:e0185224. doi: 10.1371/journal.pone.0185224
Tanguy, A., Guo, X., and Ford, S. E. (2004). Discovery of genes expressed in response to Perkinsus marinus challenge in Eastern (Crassostrea virginica) and Pacific (C. gigas) oysters. Gene 338, 121–131. doi: 10.1016/j.gene.2004.05.019
Van Gurp, T. P., Wagemaker, N. C., Wouters, B., Vergeer, P., Ouborg, J. N., and Verhoeven, K. J. (2016). EpiGBS: reference-free reduced representation bisulfite sequencing. Nat. Methods 13, 322–324. doi: 10.1038/nmeth.3763
Wang, L., Song, X., and Song, L. (2018). The oyster immunity. Dev. Comparat. Immunol. 80, 99–118. doi: 10.1016/j.dci.2017.05.025
Wang, S., Peatman, E., Liu, H., Bushek, D., Ford, S. E., Kucuktas, H., et al. (2010). Microarray analysis of gene expression in eastern oyster (Crassostrea virginica) reveals a novel combination of antimicrobial and oxidative stress host responses after dermo (Perkinsus marinus) challenge. Fish Shellfish Immun. 29, 921–929. doi: 10.1016/j.fsi.2010.07.035
Weinhold, A. (2018). Transgenerational stress-adaption: an opportunity for ecological epigenetics. Plant Cell Rep. 37:3. doi: 10.1007/s00299-017-2216-y
Wright, R. M., Aglyamova, G. V., Meyer, E., and Matz, M. V. (2015). Gene expression associated with white syndromes in a reef building coral, Acropora hyacinthus. BMC Genom. 16:371. doi: 10.1186/s12864-015-1540-2
Keywords: DNA methylation, TagSeq, eastern oyster Crassostrea virginica, Perkinsus marinus disease, transcriptomics, weighted gene co-expression network analyses
Citation: Johnson KM, Sirovy KA, Casas SM, La Peyre JF and Kelly MW (2020) Characterizing the Epigenetic and Transcriptomic Responses to Perkinsus marinus Infection in the Eastern Oyster Crassostrea virginica. Front. Mar. Sci. 7:598. doi: 10.3389/fmars.2020.00598
Received: 31 January 2020; Accepted: 29 June 2020;
Published: 11 August 2020.
Edited by:
Hollie Putnam, University of Rhode Island, United StatesReviewed by:
Guillaume Rivière, Université de Caen Normandie, FranceCopyright © 2020 Johnson, Sirovy, Casas, La Peyre and Kelly. This is an open-access article distributed under the terms of the Creative Commons Attribution License (CC BY). The use, distribution or reproduction in other forums is permitted, provided the original author(s) and the copyright owner(s) are credited and that the original publication in this journal is cited, in accordance with accepted academic practice. No use, distribution or reproduction is permitted which does not comply with these terms.
*Correspondence: Kevin M. Johnson, ZHIua2V2aW4ubS5qb2huc29uQGdtYWlsLmNvbQ==
Disclaimer: All claims expressed in this article are solely those of the authors and do not necessarily represent those of their affiliated organizations, or those of the publisher, the editors and the reviewers. Any product that may be evaluated in this article or claim that may be made by its manufacturer is not guaranteed or endorsed by the publisher.
Research integrity at Frontiers
Learn more about the work of our research integrity team to safeguard the quality of each article we publish.