- 1Department of Marine Sciences, University of Connecticut, Groton, CT, United States
- 2Bermuda Institute of Ocean Sciences, St. George’s, Bermuda
- 3Department of Marine and Coastal Sciences, Rutgers University, New Brunswick, NJ, United States
- 4Institute of Marine Science, University of Alaska Fairbanks, Fairbanks, AK, United States
The dramatic warming of the Arctic Ocean will impact pelagic ecosystems in complex ways, including shifting patterns of species distribution and abundance, and altering migration pathways and population connectivity. Species of the Phylum Chaetognatha (arrow worms) are abundant in the zooplankton assemblage and are highly effective predators, with key roles in pelagic food webs. They are useful indicator species for impacts of climate change on marine ecosystems. This study examined the population genetic diversity, structure and connectivity of the chaetognath, Eukrohnia hamata, based on sampling from six regions defined by geography, bathymetry, and major currents flowing through the Arctic Ocean. A 528-base pair sequenced region of mitochondrial cytochrome oxidase I (mtCOI) analyzed for 131 specimens resulted in 78 haplotypes and very high haplotype diversity. Analysis of mtCOI haplotype frequencies provided no evidence of population genetic structure. Genomic Single Nucleotide Polymorphisms (SNPs) detected from the same specimens by double-digest Restriction-site Associated Digestion (ddRAD) confirmed high levels of gene flow among the regions, but supported the genetic distinctiveness of two population clusters: Atlantic–Arctic versus Pacific–Arctic. Removal of SNPs subject to selection resulted in slightly higher probability of three clusters, and suggested the possibility of local adaptation of regional populations of E. hamata. Comparative analysis revealed evidence that random selection of subsets of SNPs, perhaps impacted by different ecological and (micro) evolutionary drivers, can result in marked differences in numbers and distributional patterns of clusters and associated variation in F-statistics. Analysis of population connectivity using SNPs supported the primary migration pathway via flow from the Atlantic to the Pacific Arctic regions.
Introduction
The Arctic Ocean Ecosystem
The flow of major currents throughout the Arctic Ocean is determined by geography and bathymetry, including deep basins and undersea mountain ridges (Rudels et al., 1994, 2000). Exchange between the Arctic Ocean and other ocean regions occurs through a limited number of passageways defined by land masses (Figure 1). Cold, relatively fresh water flows through the Bering Strait from the Pacific Ocean, and is swept into the Beaufort Gyre in the Pacific Arctic (Weingartner et al., 2005; Woodgate et al., 2007). Under some conditions, the gyre rotation weakens and freshwater is transported via the Transpolar Current toward the North Atlantic Ocean. The North Atlantic Current carries warmer and relatively salty water northward, with one branch passing to the East and the other to the West of Svalbard. Due to the salinity, this water is denser and sinks below Arctic waters, where it is trapped beneath a density barrier to mixing, created in part by cold, fresh water resulting from melting ice (Rudels et al., 2004). These deep currents form smaller gyres within the Eurasian and Amerasian Basins near the North Pole (Figure 1).
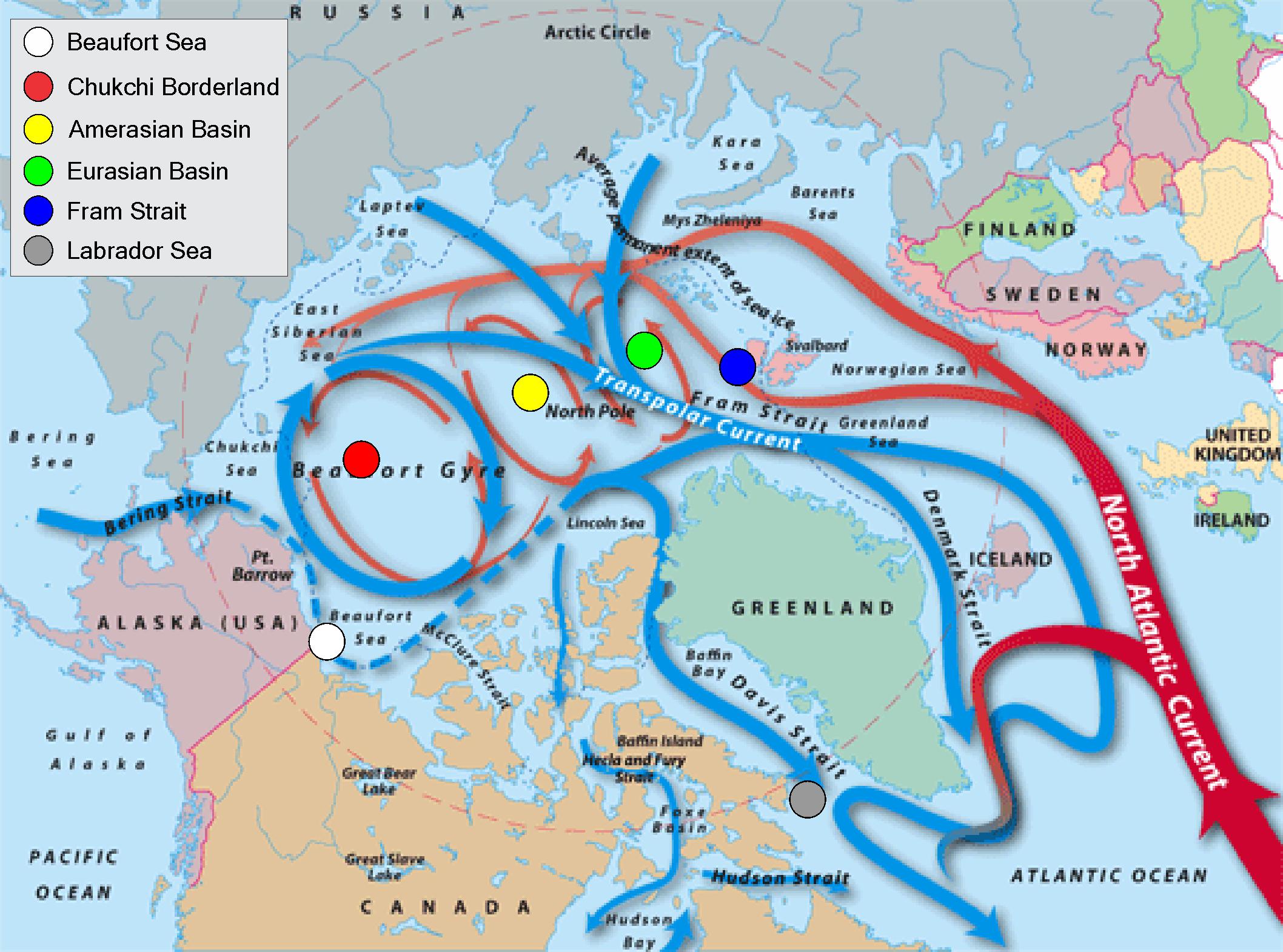
Figure 1. Major ocean currents in the Arctic Ocean, with approximate locations of the geographic regions from which samples were collected for this study. Figure courtesy of Woods Hole Oceanographic Institution. (See https://www.whoi.edu/main/topic/arctic-ocean-circulation).
The Arctic is one of the fastest-warming regions throughout the global ocean, and patterns of circulation in the Arctic Ocean are being dramatically impacted by climate change, including increased sea ice melt, reduced and thinner sea ice cover, and associated changes in water column density structure. Predicted changes include a weakening of the Beaufort Gyre circulation system, changes in water column structure, and increased outflow of Arctic waters into the North Atlantic Ocean (Proshutinsky et al., 2015). Notably, predicted increasing inflow of Atlantic waters into the Eurasian Basin, referred to “atlantification,” is expected to reduce ice cover, impact water column structure, and alter transport pathways (Polyakov et al., 2017). These changes can disrupt the Arctic Ocean ecosystem by decreasing abundance and biodiversity of native Arctic species by shifting species biogeographical ranges and decreasing energy transfer efficiency (Gamfeldt et al., 2015).
Changes in Arctic current systems will also impact pathways of dispersal and transport of zooplankton, which will be reflected in population genetic diversity, structure, and connectivity of species with broad geographic distributions throughout Arctic Ocean regions. Examination of population genetic/genomic characteristics of key species can provide insights into possible ecological and evolutionary impacts of the changing climate, including likelihood of local adaptation, changes in biogeographic distributions, and consequences for pelagic food webs and the ocean ecosystem of the Arctic.
Phylum Chaetognatha
Patterns of abundance and biomass of zooplankton have been found to be consistent across the Arctic basins sampled for this study (Kosobokova et al., 2011). Both abundance and biomass are highest in surface waters, where copepods and chaetognaths are the predominant groups. Throughout the water column, the two groups represent ∼75 and ∼13% of biomass, respectively, on average (Kosobokova and Hopcroft, 2010). In contrast, species composition does differ between Atlantic and Pacific Arctic regions, most notably in the presence of expatriate species, which tend to be restricted to Arctic regions adjacent to their home biogeographic ranges (Kosobokova and Hopcroft, 2010; Kosobokova et al., 2011). Pacific expatriate zooplankton species are found frequently in the Chukchi Sea and adjacent Chukchi Borderland (Nelson et al., 2009; Hopcroft et al., 2010; Questel et al., 2013) and occasionally in the Canada Basin and Beaufort Sea (Hopcroft et al., 2005; Smoot and Hopcroft, 2017a, b).
Chaetognaths are found in abundance throughout the global ocean (Pierrot-Bults and Nair, 1991; Pierrot-Bults, 2008). As a group, chaetognaths are abundant, make up a large portion of zooplankton biomass, and play key roles in pelagic food webs as highly effective predators, frequently targeting copepods (Terazaki, 2000). In the Arctic, chaetognaths may significantly impact patterns of seasonal and geographic variability in food web functioning, which has been best studied at lower trophic levels. In particular, relationships between seasonal ice cover, primary productivity, and trophic relationships, including grazing of zooplankton and consumption of sinking organic matter have been examined (Grigor et al., 2014, 2017) to characterize the impact and consequences of variation in predator distribution and abundance.
Eukrohnia hamata is the species of chaetognath most commonly found in the Arctic Ocean (Kosobokova and Hopcroft, 2010; Kosobokova et al., 2011). The species has been considered to exhibit a global distribution (Pierrot-Bults and Nair, 1991; Baranova et al., 2009), including reports throughout the Antarctic and Atlantic (Alvarino, 1964) and Pacific regions (Terazaki and Miller, 1986). Notably, the life history of E. hamata is quite variable based on geographic location: temperate populations have shorter life spans and are found in the deep ocean, while polar populations can have a lifespan of multiple years and live in the surface ocean (Bone et al., 1991).
Phylogeography and Population Connectivity
Phylogeography integrates biogeography and population genetics to allow examination of the evolutionary history of a species in relation to its environment (Avise, 2000). Patterns of migration and gene flow can be revealed by examination of population genetic diversity, structure, and connectivity. For marine zooplankton, such analyses can provide useful indicators of community responses to environmental conditions and climate change.
The characteristically broad biogeographical distributions typical of many chaetognath species have made them interesting subjects for examining population structure and connectivity (Peijnenburg et al., 2005, 2006). The mitochondrial cytochrome oxidase I (mtCOI) gene, which has been widely used as a barcode for identification and discrimination of zooplankton species (Bucklin et al., 2010), has also been used to identify and discriminate species of chaetognaths (e.g., Jennings et al., 2010). The accurate and reliable identification of chaetognath species based on morphological characters remains challenging, and the routine use of mtCOI barcodes for species identification is a useful first step and best practice for any ecological or population genetic study (Peijnenburg et al., 2005, 2006). Many marine zooplankton species exhibit sufficient intraspecific sequence variation of mitochondrial genes to allow analysis of large-scale population genetic diversity and structure based on mtCOI and 16S rRNA (Bucklin, 2000; Bucklin et al., 2011, 2018; Peijnenburg and Goetze, 2013). Genetic divergence of chaetognath populations has been documented in European seas and in the NE Atlantic (Peijnenburg et al., 2005). MtCOI has been used for examination of phylogeography and population connectivity of copepods in Arctic and sub-Arctic waters (Aarbakke et al., 2014; Questel et al., 2016; Weydmann et al., 2016, 2018). Population genetic diversity and structure of E. hamata have been examined using mtCOI sequence variation, where both studies revealed evidence of significant genetic differentiation of biogeographically defined populations, usually at the scale of ocean basins, suggesting the possible presence of cryptic species (Miyamoto et al., 2012; Kulagin et al., 2014).
In recent years, with widespread application of high throughput DNA sequencing, the discovery and simultaneous genotyping of thousands of single nucleotide polymorphisms (SNPs) has become possible using various genotyping-by-sequencing (GBS) approaches that entail high throughput sequencing of a targeted fraction of the genome. Importantly, these approaches do not require pre-existing genomic reference data, making them particularly useful for non-model organisms (McCormack et al., 2013; Schlötterer et al., 2014). One GBS approach is restriction-site associated DNA sequencing (RAD; Miller et al., 2007; Davey and Blaxter, 2010; Reitzel et al., 2013), which allows detection of hundreds to hundreds of thousands of SNPs across a genome (Schweyen et al., 2014). Population genomic studies of a number of zooplankton species have used RAD detection of SNPs (see review by Bucklin et al., 2018), including the copepods, Centropages typicus (Blanco-Bercial and Bucklin, 2016), Tigriopus californicus (Foley et al., 2011), Calanus sinicus (Yang et al., 2014), and Calanus finmarchicus (Choquet et al., 2019); and the euphausiid, Euphausia superba (Deagle et al., 2015), among other marine organisms (see review by Crawford and Oleksiak, 2016). RAD has been applied to a broad spectrum of questions, including tests of selection (e.g., Hohenlohe et al., 2011) and phylogeographic studies (e.g., Emerson et al., 2010). Several cost-effective modifications of the original RAD protocol have been developed, including double-digest RAD (ddRAD; Peterson et al., 2012), which uses two restriction enzymes to reduce genomic complexity and produce a more even distribution of the sequenced DNA fragments across the genome. Phylogeographic analysis of the widely distributed chaetognath, E. hamata, can provide useful insights into how a warming Arctic may affect population structure and connectivity of the pelagic assemblage. This study uses both mtCOI sequences and genomic SNPs detected by ddRAD to examine population genetic/genomic diversity, structure, and connectivity of E. hamata with respect to Pan-Arctic circulation patterns. The overarching goal is to provide new insights into potential impacts of climate change on this abundant predatory zooplankton species, which is an important trophic link in the pelagic food web and a key species in the Arctic Ocean ecosystem.
Materials and Methods
Collection and Preservation of Samples
Zooplankton samples containing E. hamata were collected from six regions across the Arctic Ocean during oceanographic research cruises from 2013 to 2016 (Table 1 and Figure 2). Samples from the central Arctic Basins (Eurasian and Amerasian) were collected during a 2011 cruise of the R/V Polarstern (PS11); samples from the Beaufort Sea were collected during 2013 and 2014 as part of the United States–Canadian Transboundary program aboard the R/V Norseman II (TB13/14); samples from the Chukchi Borderland region were collected during a 2016 cruise of the USCGC Healy (HLY2016); samples from Fram Strait were collected during a 2015 cruise of the R/V Helmer Hanssen (HH2015), as part of the SI_Arctic Initiative led by the Institute of Marine Research, Norway; samples from the Labrador Sea were collected during a 2013 cruise of the R/V GO Sars (GOSARS 2013), as part of the EuroBASIN Program.
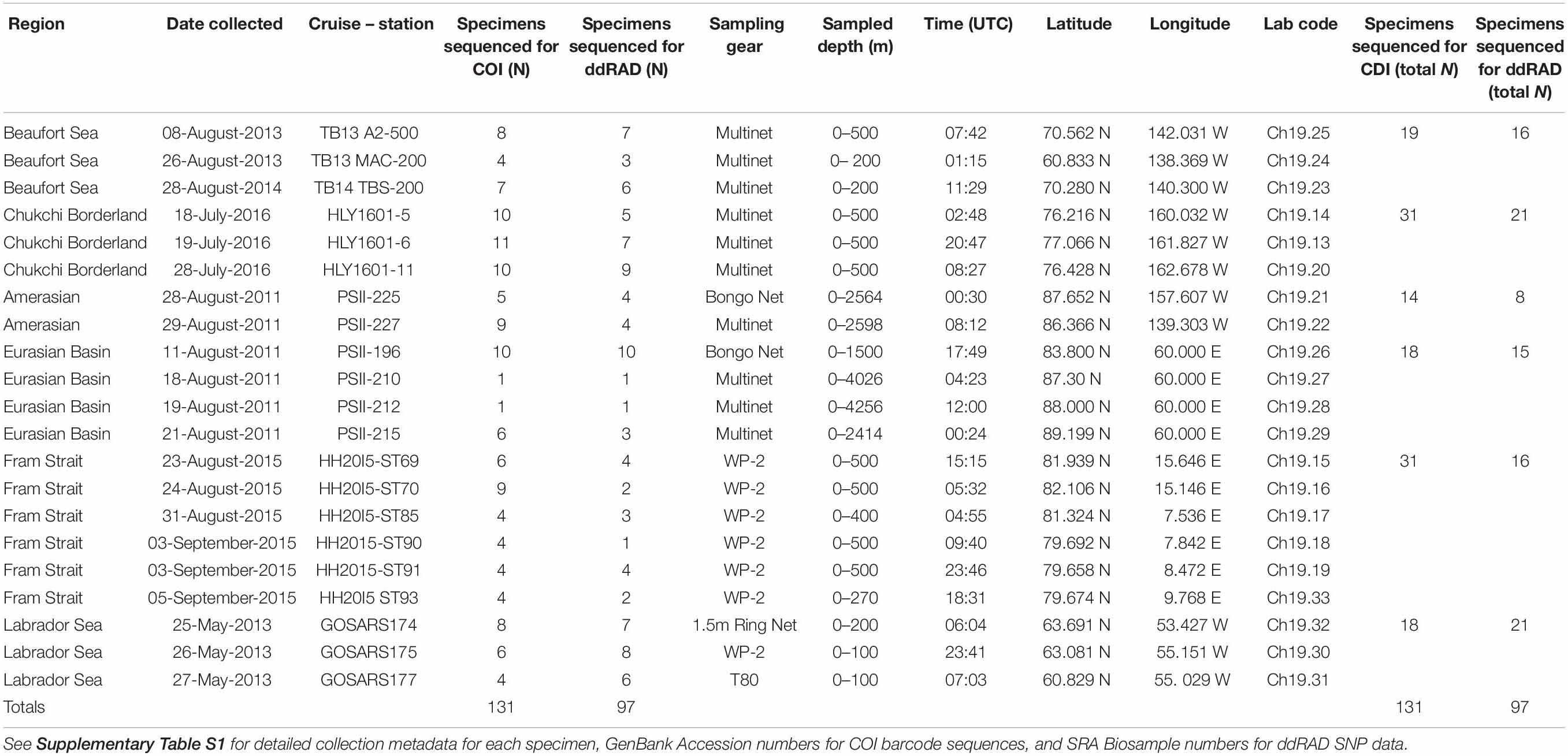
Table 1. Collection metadata for samples from which specimens of Eukrohnia hamata were identified for analysis in this study.
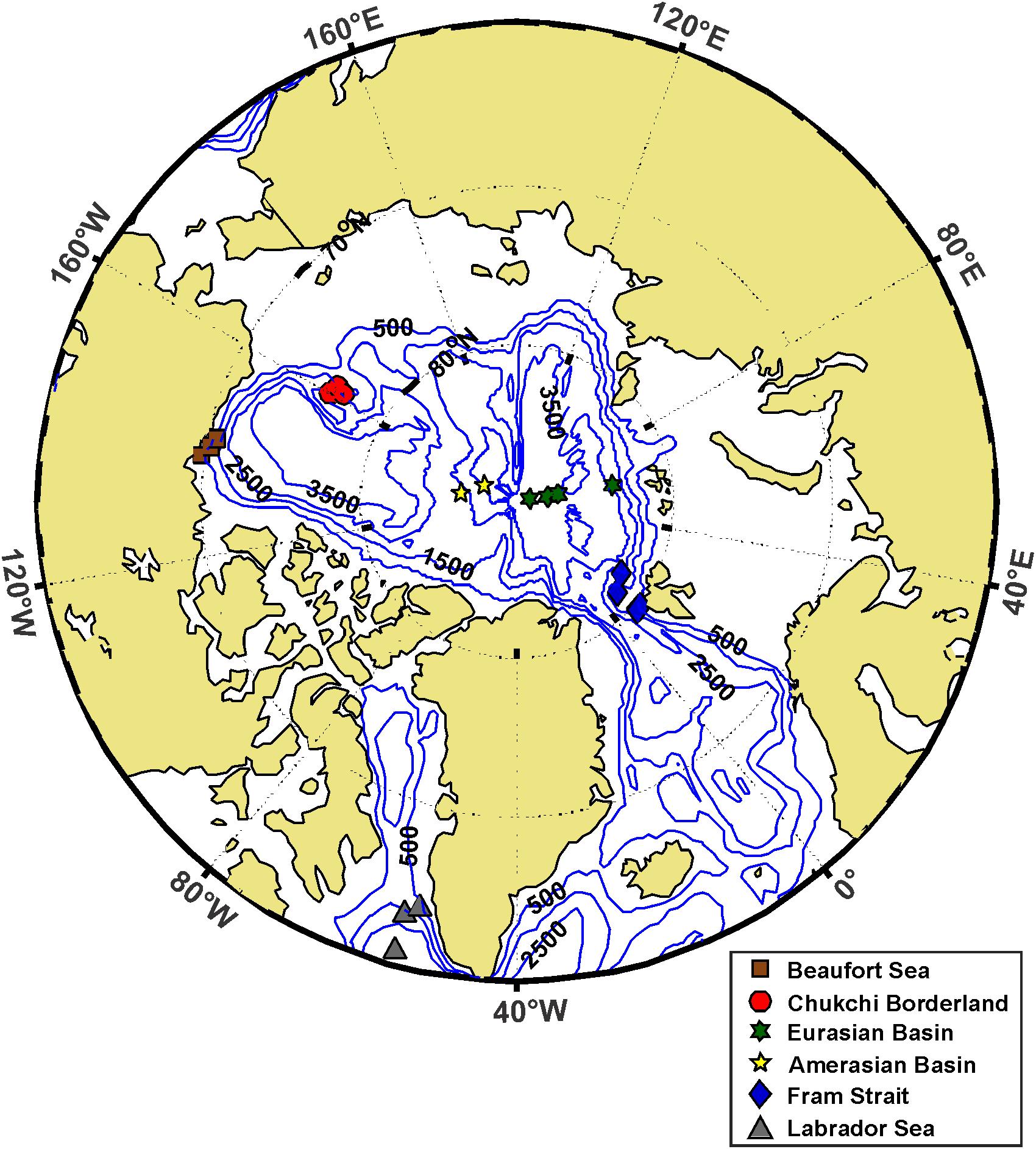
Figure 2. Collection locations of samples from which Eukrohnia hamata was identified and analyzed for mtCOI barcode sequencing and ddRAD SNP detection for this study. Symbol shape and color indicate regional designation: Eurasian Basin, Amerasian Basins, Beaufort Sea, Chukchi Borderland, Fram Strait, and Labrador Sea. Numbers indicate depths (in meters) of the bathymetric contour lines. See Table 1 for collection metadata.
Samples were preserved immediately after collection in either 95% undenatured ethanol or RNAlater Stabilization SolutionTM (Thermo Fisher Scientific). Chaetognaths were removed from each sample and examined using a Leica MZ16 dissecting microscope. Diagnostic characteristics for species identification and measurements of each individual were recorded as described in Fowler (1996), but positive identification was not possible in all cases due to the degradation of fins and corona cilia and distortion to the body from preservation.
DNA Extraction, PCR Amplification, and Sequencing of mtCOI
Specimens were rinsed in sterile MilliQ water to remove traces of ethanol or RNAlaterTM prior to DNA extraction. Genomic DNA from individual specimens was extracted using the DNeasy Blood and Tissue Kit (Qiagen). Final elution occurred in 100–200 μL of elution buffer (Qiagen). DNA was quantified using the Qubit dsDNA HS Assay (Invitrogen) and low concentration samples (<100 ng total DNA) were concentrated using vacuum centrifugation. Extracted DNA was analyzed using two different approaches, including DNA sequencing of the barcode region of mitochondrial cytochrome oxidase subunit I (mtCOI) and detection of genomic SNPs using double-digest restriction-site associated DNA sequencing (ddRAD; see below).
Polymerase Chain Reaction (PCR) amplification of a 710-base pair (bp) fragment of the mtCOI gene was achieved using 12.2 μL MilliQ water, 5 μL 5X GoTaq® Flexi Buffer, 0.3 μM dNTPs, 2.7 mM MgCl2, 0.25 μL GoTaq® Flexi DNA Polymerase, and 0.4 μM forward and reverse primer, and 2.5 μL DNA template, for a total reaction volume of 25 μL. Consensus primers were used (Folmer et al., 1994): LCO1490, 5′-GGTCAACAAATCATAAAGATATTGG-3′ and HCO2198, 5′-TAAACTTCAGGGTGACCAAAAAATCA-3′. The PCR protocol was adapted from Miyamoto et al. (2012): 94°C for 3 min; 35 cycles of 94°C for 45 s, 50°C for 45 s, and 72°C for 45 s; and 1 cycle of 72°C for 7 min. Amplicons resulting from successful PCR reactions were cleaned using ExoSAP-ITTM Express PCR cleanup (Thermo Fisher Scientific) and sequenced by Eurofins Genomics1.
Analysis of mtCOI Sequences
Forward and reverse mtCOI sequences were aligned with CLUSTAL-W (Thompson et al., 1994, 1997) using BioEdit Ver. 7.1.9 to create a consensus sequence. Confirmation of species identification as E. hamata was based on mtCOI sequences using BLAST searches of the NCBI GenBank database (Altschul et al., 1997) and matches to E. hamata records with E-values = 0, where E is the expected number of matches by chance (Karlin and Altschul, 1990). Sequences were aligned using the Muscle algorithm (Edgar, 2004) and trimmed to 528 bp for analysis using the Molecular Evolutionary Genetics Analysis (MEGA ver. 7; Kumar et al., 2016) software package. Nucleotide (π) and haplotype (Hd) diversity, as well as neutrality tests (Fu’s and Li’s F statistics), for the COI gene were calculated using the software DnaSP Ver. 5 (Librado and Rozas, 2009). A hierarchical Analysis of MOlecular VAriance (AMOVA) was used to examine population genetic structure using the software Arlequin 3.5 (Excoffier and Lischer, 2010). Samples were grouped by geographic region (Table 1). Variance partitions were tested for significance under 10,100 permutations, with α = 0.05, after sequential Bonferroni correction (Holm, 1979). All negative ΦST values obtained were assumed to be zero.
Maximum Parsimony (MP) gene trees were analyzed using the Tamura best–fit nucleotide substitution model (Tamura, 1992) as determined by MEGA Ver. 7 (Kumar et al., 2016). The significance of the substitution model was estimated through 10,000 coalescence simulations using a bootstrap test of 1,000 replicates. Haplotype networks were determined using Haploviewer (Center for Integrative Bioinformatics2).
Gene flow between regions for E. hamata was modeled using the coalescent–based program Migrate–N Ver. 3.6.11 (Beerli, 2012). Migrate–N uses ratios of maximum likelihood or Bayesian inference to estimate migration rates and effective population size (NE) under the assumption of asymmetrical migration rates at different subpopulation sizes (Beerli and Felsenstein, 1999, 2001; Beerli, 2004, 2006, 2012). Custom migration models were chosen based on known pathways of current flow in the Arctic Ocean (Figure 1). Four model scenarios were tested using Migrate–N: Pan-Arctic or Full model (i.e., all known current pathways among sampled regions, considering restrictions due to geographical constraints), Chukchi-to-Fram Strait, Fram-Strait-to-Chukchi, and bi-directional between Chukchi and Fram Strait.
Parameters for each Migrate–N model run were kept at the default settings, with the following exceptions: parameter start settings for theta (θ) and migration rates (M) used the Mode values from the posterior distributions of an initial run’s FST–based θ and M; and long–chain values (1–3) were tested for optimal posterior distributions. Bayesian factor predictions for the four model scenarios are reported.
Detection by ddRAD and Analysis of SNPs
A total of 97 libraries (one per individual) were prepared for analysis using ddRAD protocols based on Peterson et al. (2012). Protocol modifications described by Etter et al. (2011) to optimize protocols originally developed for single RAD protocols were implemented at the University of Connecticut Center for Genome Innovation3. Two high-fidelity restriction enzymes, NsiI and SphI, were selected based on in silico digestion of simulated genomes of 1 GB with 40.3% GC content, derived from published transcriptomes available in GenBank, using the R package SimRAD (Lepais and Weir, 2014). Several combinations of commonly used restriction enzymes were tested; the pair selected offered an intermediate number of fragments expected to provide both good coverage and sufficient levels of variation. Digestion took place with 100–200 ng starting DNA in a 50 μL total volume with 1 μL (20 units) of each enzyme and 5 μL of CutSmart Buffer (New England Biolabs). Samples were digested for 2 h at 60°C and then heat-killed for 20 min at 80°C following manufacturer protocol. Single-strand individually labeled NsiI adapters and degenerated SphI adapters were hybridized to 40 μM using 20 μL of each single-strand oligonucleotide, 10 μL 10× annealing buffer, and 50 μL water at the following thermal profile: 97.5°C for 2.5 min, then decreasing at 1.2°C every 30 s until reaching a temperature of 21°C. Adapters were ligated overnight. Adapter ligations were carried out with 1 μL 10× NEB Buffer 2, 0.6 μL rATP, 0.5 μL concentrated T4 DNA ligase, 4 μL of each adapter, and 50 μL double-digested DNA.
Samples were combined in 3 equimolar pools of 27, 33, and 37 individuals and cleaned using a 1.5× AMPure® XP bead cleanup (Beckman Coulter) eluted in 30 μL nuclease-free water. Pools were then size selected from 350–550 bp in 80–100 μL using the Pippin Prep DNA size selector (Sage Science) with a pause at 450 bp. Size-selected libraries were cleaned using a QIAquick PCR Purification Kit (Qiagen) in 30 μL of nuclease-free water. Illumina flow cell adapters and index primers were added to each pooled library using 15 PCR cycles in the following reaction volumes: 12.5 μL Phusion® High-Fidelity PCR Master Mix (New England Biolabs), 0.5 μL reverse indexed primer, 0.5 μL universal forward primer, 2 μL pooled libraries, and 9.5 μL nuclease-free water. The PCR protocol was: 98°C for 30 s; 15 cycles of 98°C for 10 s, 65°C for 30 s, 72°C for 30 s; and 1 cycle of 72°C for 5 min. Successful adapter ligation and amplification were inspected on the Agilent 2200 TapeStation Automated Electrophoresis system with the High Sensitivity DNA ScreenTape. PCR products were cleaned using a 1X AMPure® XP bead cleanup. Amplified libraries were sequenced at Macrogen (Seoul, South Korea) using a paired end, 100 bp kit on an Illumina HiSeq 2500 system. Numbers of reads obtained for each sample averaged 509,783, SD = 119,906 (see Supplementary Table S1).
After demultiplexing, RAD tags were analyzed using STACKS ver. 2.2 (Catchen et al., 2013). Since overlapping was not always attained, analyses included only the forward read, and length was limited to 99 bp. Definitive STACKS parameters were set after following the procedures as recommended in Paris et al. (2017), with final parameters set for M (number of mismatches allowed between putative alleles to merge them into a putative locus) = 2 and n (number of mismatches allowed between putative loci during construction of the catalog) = 2. All parameters tested are shown in Supplementary Figure S1. Populations parameters were set to select loci that had a MAF (minimum minor allele frequency) = 0.05, and were present in all populations in at least 80% of the individuals of each population. A distribution of the pairwise Φ-statistics (FST, F′ST, and ΦST) from the populations package in the STACKS software was obtained after 1,000 iterations. At each iteration, a different set of SNPs per tag was randomly selected among markers with a minor allele frequency (MAF) above 0.05. The potential number of genetic clusters and the membership of each individual were estimated using STRUCTURE Ver. 2.3.4 (Pritchard et al., 2000), which can identify subsets within the data based on detecting allele frequency differences and assign individuals to those sub-populations based on analysis of likelihood (Porras-Hurtado et al., 2013). The model choice criterion implemented in STRUCTURE to detect the number of sub-populations (K) is an estimate of the posterior probability of the data, referred to as L(K), which is calculated for a given K by computing the log likelihood of the data at each step of a Markov Chain Monte Carlo (MCMC); the average of these values is computed and half their variance is subtracted to the mean (Evanno et al., 2005). In cases where the distribution of L(K) does not clearly identify the true K, an ad hoc quantity based on the second-order rate of change of the likelihood function with respect to K (ΔK) can show a clear peak or break in slope at the true value of K (Evanno et al., 2005).
For this study, analyses were run on 100 different combinations of one SNP per RAD tag, rather than running multiple replicates of a single selection of SNPs, with the numbers of genetic clusters (values of K) ranging from 1 to 8. The MCMC simulation was run for 100,000 repetitions, after a burn-in period of 50,000. No admixture model was assumed, and the location information was included as a prior to facilitate detection of the true structure despite a weak signal (Hubisz et al., 2009). The optimal K-value was selected based on the L(K) values and the individual assignment patterns, and also using STRUCTURE HARVESTER Ver. 0.6.94 (Earl and vonHoldt, 2012) to assess the likelihood values at each K and select the optimal value using the ad hoc statistic, ΔK (Evanno et al., 2005). Results from 800 runs (100 for each run) were graphically summarized using the online version of CLUMPAK (Kopelman et al., 2015) with default parameters: LargeKGreedy algorithm, random input order, 2,000 repeats, dynamic threshold for similarity scores, and minimal cluster size threshold = 0.1.
The same analyses were run after markers under selection were identified using two different analyses. The first was BayeScan Ver. 2.1 (Foll and Gaggiotti, 2008), which uses a Bayesian approach to estimate the probability that each locus is under selection, taking into account populations and incorporating uncertainties in allele frequencies due to small sample sizes. This analysis has been shown to be more reliable than alternative methods in detecting outliers and reducing the number of false positives (Pérez-Figueroa et al., 2010; Narum and Hess, 2011). BayeScan was run on all SNPs from all tags from the dataset under default settings, except that prior odds for the neutral model were set at 100 (i.e., the probability of a model under selection for each SNP site is 100 times less likely than a neutral model); and the False Discovery Rate (FDR) was set at 0.05. The second analysis was done keeping the prior odds for the neutral model to 10, and the FDR to 0.1, which probably resulted in several neutral markers being identified as subject to selection. In either case, only markers considered to be neutral were used for calculation of Φ statistics using the populations program in STACKS and for generation of input files for STRUCTURE, using a single SNP per tag. Tags that were identified as candidates for selection were compared to the GENBANK database using BLAST (Altschul et al., 1990), but reliable annotation of the markers was not possible, and these results are not shown or discussed. All scripts used for these procedures are available at: https://github.com/blancobercial/Eukrohnia.
Results
Analysis of mtCOI Sequence Variation
Based on comparison with the mtCOI sequences available in the NCBI GenBank database, all 131 specimens analyzed for this study were confirmed to be E. hamata. The mtCOI sequences clustered within clades described from Arctic regions by Kulagin et al. (2011, 2014) and Miyamoto et al. (2012). The mtCOI sequences also clustered with significant bootstrap values with two barcoded specimens (GenBank Acc. Nos. FJ602472 and FJ602473) reported by Bucklin et al. (2010).
The Arctic population of E. hamata analyzed for this study exhibited high levels of sequence variation: based on the 528 bp (excluding sites with gaps/missing data) region of mtCOI analyzed, there were 78 unique haplotypes among 131 specimens, with haplotype diversity, Hd = 0.939 (SD = 0.017) and nucleotide diversity; Pi = 0.01031 (SD < 0.001); and average number of nucleotide differences, k = 5.44416. The mtCOI haplotype frequencies did not conform to neutral theory expectations: Tajima’s D = −2.16711 (p < 0.01); Fu and Li’s D∗ = −5.28736 (p < 0.02); Fu and Li’s F∗ = −4.70272 (p < 0.02). These statistics suggested genetic changes or lack of equilibrium conditions within the sampled population.
A mtCOI haplotype network constructed using mtCOI data for E. hamata showed that the most common haplotype (31 sequences) occurred among individuals from all sampling sites, whereas the second most common haplotype (8 sequences) occurred in only 3 locations (Chukchi Borderland, Fram Strait, and Eurasian Basin) (Figure 3). AMOVA analysis of spatial patterns of population genetic variation within and among samples based on mtCOI haplotypes revealed lack of geographic structure based on mtCOI, with no significant genetic differences between regions (results not shown).
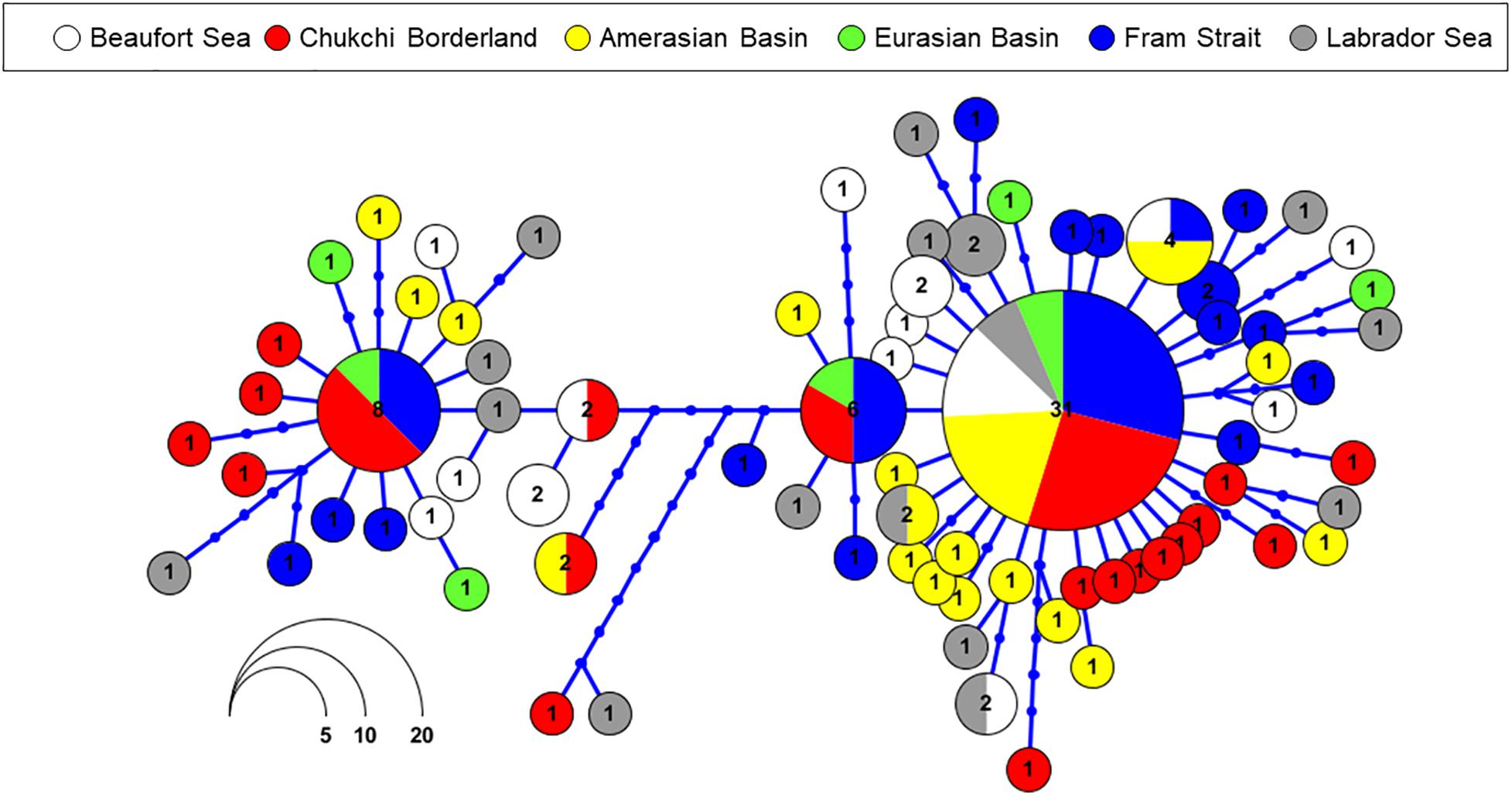
Figure 3. Haplotype network for E. hamata based on mtCOI sequences. Each circle represents a unique haplotype. Circle size and color correspond to the number of haplotypes and geographic location, respectively. Cross-bars represent number of mutations between haplotypes. Color key: white: Beaufort Sea; red: Chukchi Borderland; yellow: Amerasian Basin; green: Eurasian Basin; blue: Fram Strait; gray: Labrador Sea.
The mtCOI sequence data were also analyzed using Migrate-N software to determine Bayesian statistics of relative probabilities of hypothesized patterns and pathways of connectivity, which were based on the major Pan-Arctic currents (Figure 1). The Migrate-N results based on mtCOI did not reliably identify Arctic connectivity pathways for E. hamata (results not shown). In general, posterior distributions for each parameter were not consistently recovered between parallel runs, and often showing a flat posterior distribution.
Analysis of Genomic SNPs Detected by ddRAD
After all filtering and quality control, 1,957 RADtags that were present in at least 80% of the individuals across the 6 regional populations were retained for analysis, with a MAF > 0.05. Of these, 1,120 had only one SNP site, 489 had two SNP sites, 185 had three SNP sites, and 163 had four or more SNP sites, totaling 3,455 SNP sites overall. BayeScan analyses identified two of these SNPs as candidates for selection, with prior odds for a neutral model set to 100 and FDR set to 0.05. When prior odds for a neutral model were set to 10 and FDR to 0.1, then 17 SNPs were flagged as candidates for selection (Supplementary Figure S2).
Pairwise distances based on F-statistics calculated for single SNPs per RADtag were much lower within the Pacific Arctic (between Beaufort Sea and Chukchi Borderland) and within the Atlantic Arctic (between Fram Strait and Labrador Sea), than between the Atlantic and Pacific Arctic regions (Figure 4). The central Arctic Amerasian and Eurasian Basins were notably divergent for FST calculations based on single-SNP markers, while higher FST and ΦST most notably distinguished the Eurasian Basin from the Chukchi Borderland (Figure 4). Regarding each metric, in all cases FST > 0, meanwhile ϕST and FST values were in some cases < 0. Removal of more SNPs identified as candidates for selection by lowering probability threshold markedly lowered the differences between samples, however, the major patterns were retained (Supplementary Figure S3).
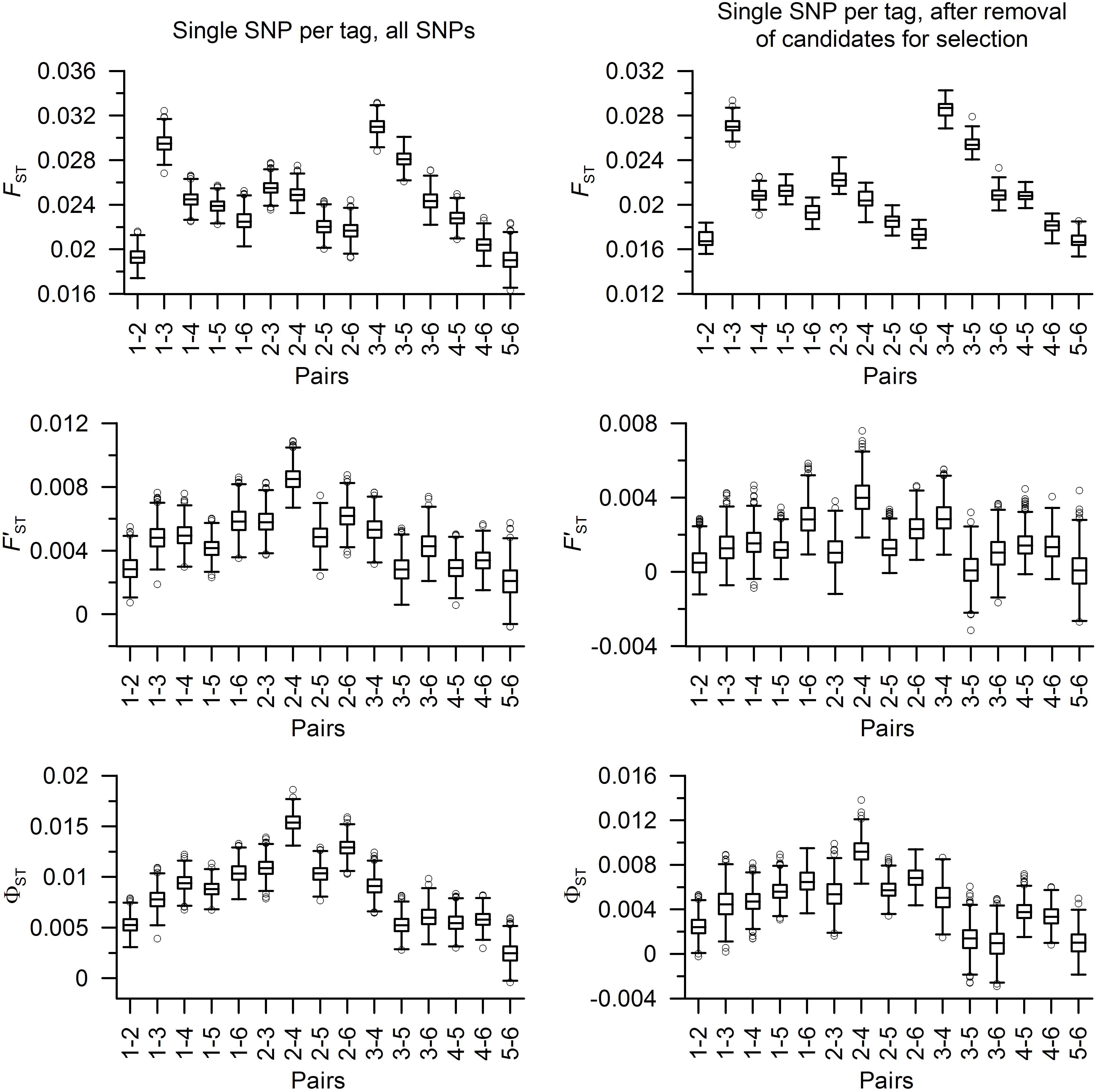
Figure 4. Box and whiskers plot showing quartiles (box), 95% confidence interval (whiskers) and outliers (circles) of F-statistics between regions, derived from 1,000 iterations using randomly chosen sets of SNPs. The three F-statistics indicate closer relationships between the Chukchi Borderland and Beaufort Sea samples (Pacific Arctic), and between the other four regional samples (Eurasian and Amerasian Basins, Fram Strait, and Labrador Sea). After removal of markers deemed to be candidates for selection, the pattern was less clear, although there remained clear differences. NB: Y-axis units differ between statistics. Key: 1: Beaufort Sea; 2: Chukchi Borderland; 3: Amerasian Basin; 4: Eurasian Basin; 5: Fram Strait; 6: Labrador Sea.
Clustering analysis based on all SNPs differed from patterns using only SNPs identified as neutral, and resulted in a change in the relative likelihood of the number of clusters (K) revealed by the Evanno procedure (Evanno et al., 2005). Combinations from all SNPs available resulted in the selection of K = 2, while inclusion of only neutral markers resulted in K = 3 (Figure 5). Other useful indicators, e.g., the likelihood of each clustering level, L(K), also yielded K = 2 based on all SNPs, however, results were unclear for analysis of only SNPs identified as neutral, with higher likelihoods for K = 1 and K = 3 (Figure 5). Analysis based on two hypothesized clusters (K = 2) in STRUCTURE revealed marked differentiation between E. hamata populations in the Beaufort Sea and Chukchi Borderland versus genetically distinct populations spanning the Eurasian and Amerasian Basins, Fram Strait, and Labrador Sea (Figure 6A). Removal of SNPs identified as candidates for selection only slightly reduced the level of genetic differentiation observed between these two clusters of populations. Removal of SNPs identified as likely to be under selection also provided support for three population clusters (K = 3), including one comprising the Eurasian Basin, Fram Strait, and Labrador Sea, a second comprising the Chukchi Borderland and Beaufort Sea, with the Amerasian Basin comprised of a mixture of all 3 clusters (Figure 6B). This pattern with 3 clusters was observed in 93 of the 100 runs, with no other pattern being consistent among the other runs. Removal of additional SNPs under possible selection did not alter the results further (data not shown); results of all clustering patterns up to K = 8, including minor clustering patterns, are shown in Supplementary Figure S4.
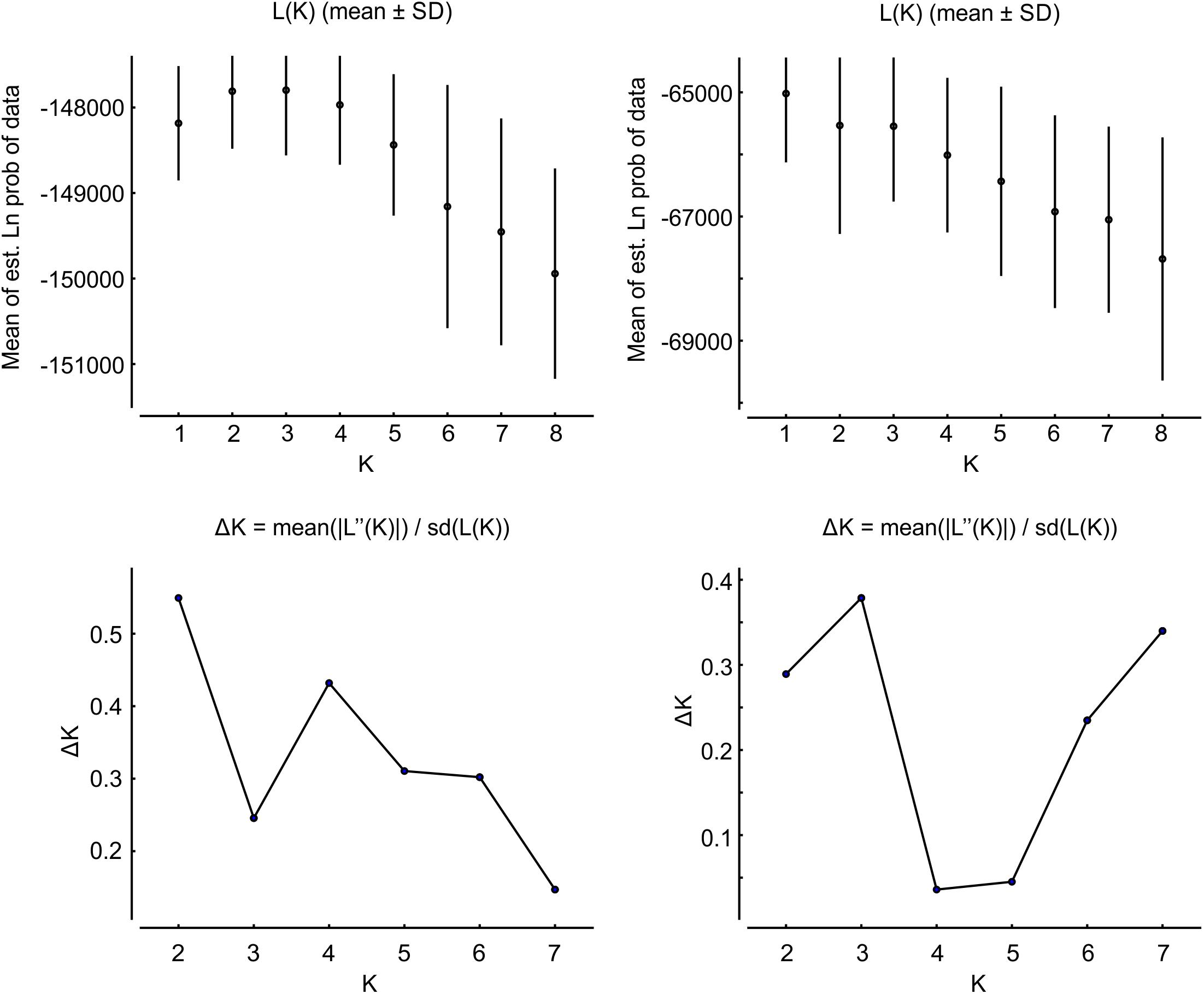
Figure 5. Graphs representing L(K) and ΔK following Evanno et al. (2005) using combinations of one SNP per tag from all SNPs, and after removing candidates for selection. The most likely clustering level is indicated by the modal value of ΔK, in these cases K = 2 for all SNPs, and K = 3 after removal of those candidate for selection as indicated by BAYESCAN. Other indicator, like L(K), did not fully agreed with the clustering level indicated. Note that x-axis scales differ between graphs [1–8 for L(K); 2–8 for ΔK].
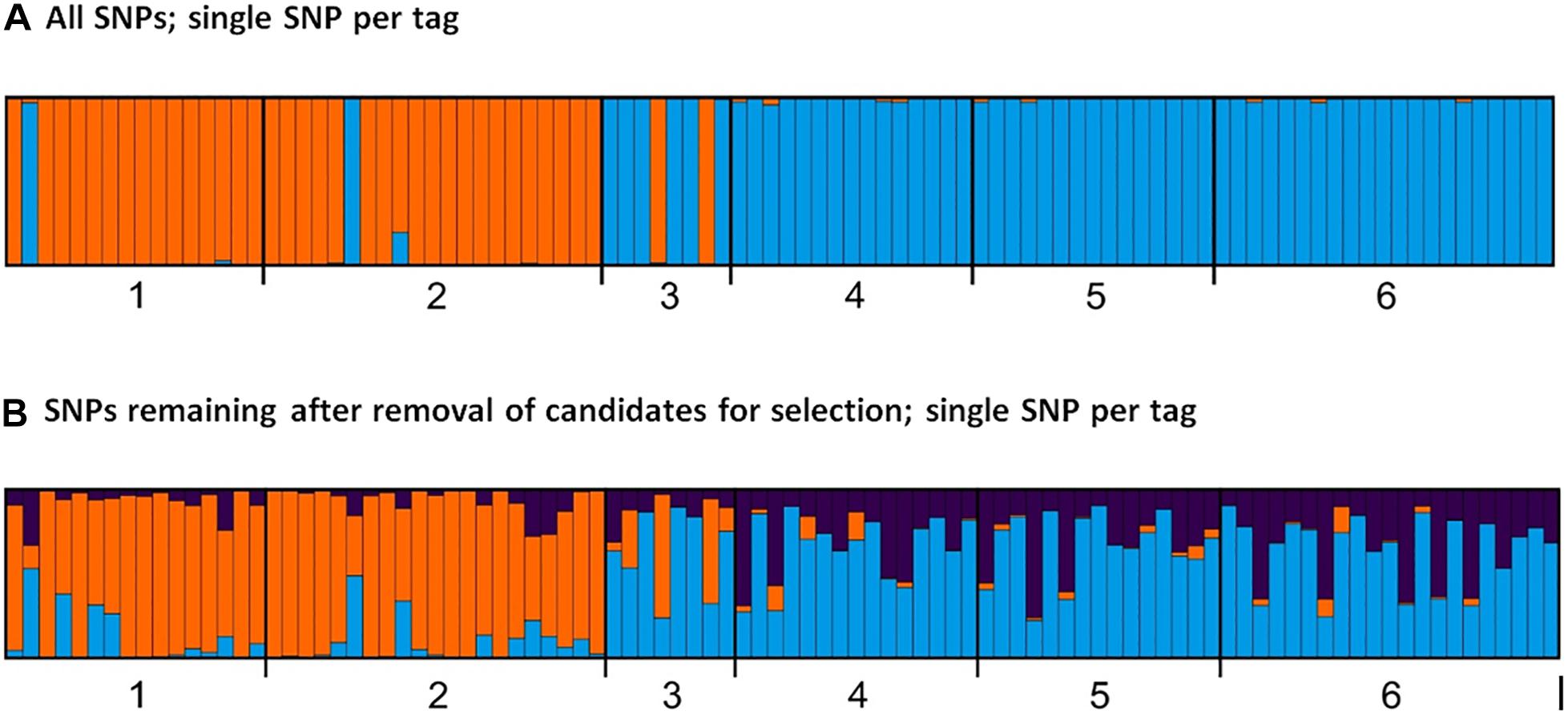
Figure 6. Bar plots from STRUCTURE showing with genetic clustering of sub-populations based on ddRAD SNP analysis, with one SNP selected per RADtag for each run, for numbers of clusters, K = 2 and K = 3. (A) Analysis of all 3,455 SNPs from 1,957 RADtags. (B) Analysis of SNPs deemed to be neutral, including 3,438 SNPs from 1955 RADtags. Genetic clusters are represented by colors; bar graphs show average probability of membership (y-axis) of each individual; regions are defined by thick vertical lines. There is a strong differentiation between the Pacific Arctic (Beaufort Sea and Chukchi Borderland) and the Atlantic Arctic (Fram Strait and Labrador Sea) in both graphs. Key: 1: Beaufort Sea; 2: Chukchi Borderland; 3: Amerasian Basin; 4: Eurasian Basin; 5: Fram Strait; 6: Labrador Sea.
Analysis of population connectivity using SNPs supported the primary migration pathway via flow from the Atlantic to the Pacific, consistent with the population genetic analysis using STRUCTURE. The results are shown as the relative likelihood from Bayesian analysis (Table 2) of pathways hypothesized based on circulation patterns shown in Figure 1.
Discussion
Population Genetic Diversity, Structure, and Connectivity Based on mtCOI
All E. hamata specimens collected from diverse Arctic regions yielded mtCOI sequences that clustered in one mtCOI lineage (Eh-3) reported by Kulagin et al. (2014), who described the collection locations of this clade as including two areas in the Arctic Ocean: Nansen Basin (north of Svalbard) and Kara Sea. Based on global phylogeographic analysis using mtCOI, Kulagin et al. (2014) concluded that one panmictic population of E. hamata was distributed throughout Arctic and North Atlantic regions. The two most frequent haplotypes observed in our analyses were identical to two sequences (NCBI GenBank Acc. Nos. FJ602472 and FJ602473) from E. hamata collected from the Arctic Canada Basin and reported by Bucklin et al. (2010).
The cosmopolitan-but-disjunct distribution of E. hamata has been examined using mtCOI sequence variation (Jennings et al., 2010; Kulagin et al., 2011, 2014; Miyamoto et al., 2012). Miyamoto et al. (2012) also noted the difficulty of discriminating E. hamata from Eukrohnia bathypelagica based on mtCOI, which complicated interpretation of their results. Kulagin et al. (2014) found evidence of five distinct mtCOI lineages of E. hamata, largely defined by biogeographical distribution, and concluded that the lineages represent distinct, cryptic species. Evidence for species-level differences was based on Kimura-2-Parameter (K2P) genetic distances between lineages (K2P = 0.043–0.135; Kulagin et al., 2014) that were higher than the intraspecific mean for 14 chaetognath species (K2P = 0.015) analyzed by Jennings et al. (2010). Genetic divergence between geographic populations has been reported for several chaetognath species (Peijnenburg et al., 2006; Marlétaz et al., 2008; Miyamoto et al., 2010), suggesting the possibility of cryptic species within their wide-spread or global biogeographical distributions.
The high levels of mtCOI haplotype diversity observed for E. hamata in this study, with 78 unique haplotypes from 130 specimens (Hd = 0.939), are comparable to previous observations in diverse populations of this species throughout the global ocean (Miyamoto et al., 2012; Kulagin et al., 2014), although this is in contrast to findings of low diversity for allozymic loci for the species by Thuessen et al. (1993). High haplotype diversity may increase resilience of E. hamata to Arctic-wide environmental changes.
Patterns of population genetic diversity and structure have been used to infer evolutionary potential and demographic history of marine zooplankton species (e.g., Aarbakke et al., 2014; Questel et al., 2016; see review by Peijnenburg and Goetze, 2013). For E. hamata, mtCOI revealed high haplotype diversity and low nucleotide diversity, with haplotype frequencies that did not conform to neutral theory expectations (Fu’s F statistic = −4.88; p < 0.02). These findings suggest that E. hamata may have undergone a recent population expansion, perhaps similar to that hypothesized for the Pan-Arctic copepod, Calanus glacialis (Weydmann et al., 2018). In contrast, Peijnenburg et al. (2005) concluded that patterns of haplotype diversity for mitochondrial cytochrome oxidase II, with high diversity that did not conform to neutral theory expectations, provided evidence that the chaetognath species, Sagitta setosa, was impacted by population bottlenecks from biogeographic range shifts caused perhaps by climatic perturbations during the Pleistocene era.
Population Genetic Diversity, Structure, Connectivity Based on Genomic SNPs
Genomic SNPs can provide markedly improved resolution of population genetic structure over mitochondrial gene markers (Helyar et al., 2011; Reitzel et al., 2013; Crawford and Oleksiak, 2016), and are especially useful for high gene flow species, such as most marine zooplankton (Blanco-Bercial and Bucklin, 2016; Bucklin et al., 2018; Choquet et al., 2019).
In this study, the finding of limited exchange between Atlantic Arctic and Pacific Arctic populations of a cosmopolitan – if not circumglobal – species of zooplankton warrants careful and thorough consideration. The migration patterns for E. hamata found to be most probable based on SNP markers are notably different from those inferred and predicted based on Pan-Arctic assessments of ecosystems and ocean circulation (e.g., Wassmann, 2015), as well as recent studies of the population genetics and connectivity of Arctic Ocean zooplankton. Questel et al. (2016) found no evidence of population structure based on mtCOI for copepod species of Pseudocalanus, and recent studies using mitochondrial and microsatellite makers concluded that Arctic populations of the copepod C. glacialis are panmictic (Weydmann et al., 2016, 2018), but see Nelson et al. (2009). Patterns of COI haplotype diversity also supported Pan-Arctic dispersal of the pteropod Limacina helicina, with evidence of population expansion during Pleistocene glaciation (Abyzova et al., 2018).
Genomic SNPs that show evidence of selection can provide markers of micro-evolution and local adaptation to environmental conditions (Gagnaire et al., 2015). After removal of SNPs showing impacts of selection, a similar pattern of two primary population genetic clusters of E. hamata in the Arctic was observed, although more mixing was detected and, in particular, the distinctiveness of the Amerasian and Eurasian Basins was less clear (Figure 6). The exclusion of SNPs under selection in particular resulted in higher likelihood of three genetic clusters across Arctic populations of E. hamata (Figure 5), with the third cluster distributed primarily in the Atlantic Arctic regions, especially the Labrador Sea (Figure 6). Analyses including combinations of all SNPs most frequently yielded results with only one genetic cluster (ranging from 91 to 100% of probability, with the exception of the Amerasian region). In contrast, the maximum probability for a single cluster for each population dropped to 41–81% after removal of candidates for selection. Such comparative analyses suggest the possibility of local adaptation of regional populations of E. hamata throughout the Arctic.
Additional Factors Determining Population Genetic Diversity and Structure
Patterns of distribution and abundance of Arctic zooplankton may be explained in large part by differences in their vertical distributions (Kosobokova et al., 2011). Arctic Ocean water mass structure and circulation patterns in the Arctic are highly dependent on water depth, with wind-driven surface currents and density-driven currents at depth (i.e., >1,000 m). In the Pacific Arctic, Atlantic Water predominates between 200 and 1,000 m, which almost certainly contributes to the observed connectivity of the central Arctic with the Atlantic. In addition, surface flow in the Beaufort Gyre would transport zooplankton from the Chukchi Borderland and Beaufort Sea into the Amerasian Basin, and may restrict flow from the Pacific to the central Arctic. However, deeper current flow is in the reverse direction, and would carry zooplankton from Fram Strait, into the Eurasian Basin, and potentially toward Pacific Arctic waters.
Chaetognaths are exceptional among marine zooplankton in their active swimming behavior and position-keeping capabilities (Kosobokova et al., 2011). Chaetognath species, including E. hamata, are most abundant in the mesopelagic zone (200–1,000 m) of the Arctic Ocean, and are major contributors to biomass of the zooplankton assemblage of the deep-sea ecosystem below 1,000 m (Kosobokova and Hopcroft, 2010). Eukrohnia hamata is reported to occur over a broad depth range (0–3,000 m) and is one of the top 10 species contributing to patterns observed in Arctic zooplankton communities over a wide range of depth zones, including 50–100, 100–200, 200–300, and 500–1,000 m (Kosobokova et al., 2011). Ulloa et al. (2000) described the annual cycle of E. hamata off the coast of Chili, where the species is found in surface waters from August to December, descending in May to 400–900 m (the maximum depth sampled). Another study (Gjøsæter et al., 2017) reported that chaetognaths exhibited diel vertical migration in the Arctic. The limited number of samples analyzed and the lack of vertically stratified sampling for this study makes it impossible to determine sub-regional scale patterns of dispersal or the impacts of water column stratification on migration pathways of E. hamata. However, published reports of the wide vertical distribution and active swimming behaviors, including likely vertical migration, of E. hamata provide a basis for confidence that our analyses accurately reflect the overall patterns of connectivity among geographic regions of the Arctic Ocean. In addition, the similarity of zooplankton assemblages among Arctic regions suggests effective exchange and lack of faunal barriers (Kosobokova et al., 2011).
This study provided evidence of the complexity of population genomic inferences from SNPs. Comparative analysis of different combinations of randomly selected unique SNPs per RADtag, revealed overall similarities in general patterns of population genetic diversity and structure. However, in some instances (7 of 100 runs) there were notable differences in numbers and distributional patterns of clusters, with associated variation in F-statistic values and significance. This finding reveals an important issue for studies using SNP markers detected by various RAD protocols. In particular, random selection of subsets of SNPs impacted by different ecological and (micro) evolutionary drivers can result in misleading or erroneous results (e.g., false positives or negatives). If by chance the SNPs selected correspond to a minor clustering pattern, this may bias F-statistic values. In this study, the 100 different runs selected for analysis by STRUCTURE, and even the 1,000 combinations used for calculation of F-statistics, helped minimize the probability of selecting a biased set of SNPs. Here >50% of the SNPs were analyzed in all calculations, since they were the only variant in our dataset of nearly 2,000 different stacks. However, there were significant differences among the results in 7 of the 100 runs, due presumably to the SNPs that were allowed to change. This finding should urge caution in the interpretation of results and encourage analysis of multiple combinations of SNPs, rather than replicate analyses of one set of single SNP sites per tag. In fact, addressing this issue is computationally relatively straightforward, as demonstrated in the scripts used in this study4.
Conclusion
Direct comparisons between results of population genetic analyses using single gene markers and genomic SNPs are key to accurate understanding of patterns of population genetic structure and pathways of population connectivity. Studies of marine zooplankton are particularly needed, given their widespread biogeographical distributions, possible occurrence of cryptic species, and complex patterns and pathways of population connectivity.
The large populations and relatively short lifespans of these species suggest their usefulness as rapid-responders to environmental perturbations driven by climate change. Analysis of genomic adaptation and micro-evolutionary changes in response to environmental variation and global climate change are key elements in improved understanding and prediction of alterations of species distribution and abundance, and consequences for food webs and ecosystems of the Arctic Ocean.
A primary goal of this study was to carry out fully parallel investigations, based on analysis of DNA sequence variation of the barcode region of mtCOI and allelic variation of genomic SNPs detected by ddRAD, of the population genetic diversity, structure, and connectivity of an important member of the Arctic Ocean zooplankton assemblage, the chaetognath E. hamata. Analysis of the same specimens in samples collected from six regions across the Arctic Ocean allowed direct comparison of the results from the different molecular markers in terms of detection and resolution of patterns and pathways of genetic exchange via the major currents flowing through the region. Importantly, this study allows further examination of previous studies using mtCOI to examine global-scale patterns of species diversity, distribution, and phylogeography of E. hamata based on the mtCOI barcode region, including evidence for a distinct population of the species in Arctic regions (Miyamoto et al., 2010; Kulagin et al., 2014). A primary finding of the study is that genomic SNPs detected by ddRAD provided clear evidence of genetic differentiation of Pacific Arctic and Atlantic Arctic populations of E. hamata, although mtCOI haplotype frequencies revealed no genetic differentiation among regional populations of E. hamata across the Arctic Ocean. Of the 131 analyzed specimens, mtCOI sequences and genomic SNPs detected by RAD-seq identified two genetically distinct populations between the Atlantic Arctic and Pacific Arctic regions, with evidence of some mixing across geographic boundaries. After removal of SNPs shown to be under selection, there was additional evidence of mixing, suggesting the possibility of local adaptation of populations of E. hamata among regions of the Arctic Ocean.
Data Availability Statement
The original contributions presented in this study are publicly available. This data can be found at MtCOI barcode sequences: GenBank Accession Numbers MN117728–MN117858 and SNP ddRAD sequences: NCBI SRA BioProject Accession: PRJNA576675.
Author Contributions
AB, LB-B, and JQ designed the research. HD and MP performed the research. HD, LB-B, and JQ analyzed the data. AB, HD, LB-B, JQ, and MP wrote the manuscript.
Funding
Zooplankton samples analyzed for this study were collected during Arctic cruises sponsored and funded through various programs, including: Chukchi Sea Environmental Studies Program (funded by ConocoPhillips, Shell Exploration and Production, and Statoil USA); United States–Canadian Transboundary Fish and Lower Trophic Communities Project (Bureau of Ocean Energy Management); SI_Arctic Initiative (Institute of Marine Research, Tromsø, Norway); and the EuroBASIN Program (Institute of Marine Research, Bergen, Norway). Funding for this study was provided by the NOAA Office of Exploration and Research (Chukchi Borderland Project, # NA15OAR0110209); and the NSF Research Experiences for Undergraduate (REU) Program at Mystic Aquarium and University of Connecticut (Grants # 1658663 and 1658742).
Conflict of Interest
The authors declare that the research was conducted in the absence of any commercial or financial relationships that could be construed as a potential conflict of interest. The reviewer MC declared a past co-authorship with several of the authors AB, JQ, and LB-B to the handling Editor.
Acknowledgments
We thank the captains and crews of the R/V Polarstern (PS11); USCGC Healy (HLY2016); R/V Helmer Hanssen (HH2015); R/V Westward Wind (CSESP); R/V Norseman II (TB); and R/V GO Sars (GOSARS 2013). The chief scientists of the cruises helped ensure successful sample processing; we are grateful to Russell R. Hopcroft (University of Alaska Fairbanks) and Ksenia Kosobokova (Russian Academy of Sciences, Moscow) for their leadership at sea. Expert technical assistance was provided by Bo Reese (Center for Genomic Innovation, University of Connecticut). We owe a warm acknowledgement to Peter H. Wiebe (Woods Hole Oceanographic Institution) for preparation of map figures. Bioinformatics and statistical analyses were carried out using resources of the Computational Biological Core (Institute of Systems Genomics, University of Connecticut). This manuscript has benefitted from the comments of the peer reviewers.
Supplementary Material
The Supplementary Material for this article can be found online at: https://www.frontiersin.org/articles/10.3389/fmars.2020.00396/full#supplementary-material
Footnotes
- ^ https://www.eurofinsgenomics.com
- ^ http://www.cibiv.at/~greg/haploviewer
- ^ https://cgi.uconn.edu/
- ^ https://github.com/blancobercial/Eukrohnia
References
Aarbakke, O. N. S., Bucklin, A., Halsband, C., and Norrbin, F. (2014). Comparative phylogeography and demographic history of five sibling species of Pseudocalanus (Copepoda: Calanoida) in the North Atlantic Ocean. J. Exp. Mar. Biol. Ecol. 461, 479–488.
Abyzova, G. A., Nikitin, M. A., Popova, O. V., and Pasternak, A. F. (2018). Genetic population structure of the pelagic mollusk Limacina helicina in the Kara Sea. PeerJ 6:e5709. doi: 10.7717/peerj.5709
Altschul, S. F., Gish, W., Miller, W., Myers, E. W., and Lipman, D. J. (1990). Basic local alignment search tool. J. Mol. Biol. 215, 403–410.
Altschul, S. F., Madde, N. T. L., Schäffer, A. A., Zhang, J., Zhang, Z., Miller, W., et al. (1997). Gapped BLAST and PSI-BLAST: a new generation of protein database search programs. Nuclic Acids Res. 25, 3389–3402. doi: 10.1093/nar/25.17.3389
Avise, J. (2000). Phylogeography: The History and Formation of Species. Cambridge, MA: Harvard University Press.
Baranova, O. K., O’Brien, T. D., Boyer, T. P., and Smolyar, I. V. (2009). “Plankton data,” in World Ocean Database, NOAA Atlas NESDIS 66, ed. S. Levitus (Washington, DC: U.S. Government Printing Office), 216.
Beerli, P. (2004). Effect of unsampled populations on the estimation of population sizes and migration rates between sampled populations. Mol. Ecol. 13, 827–836. doi: 10.1111/j.1365-294x.2004.02101.x
Beerli, P. (2006). Comparison of Bayesian and maximum-likelihood inference of population genetic parameters. Bioinformatics 22, 341–345. doi: 10.1093/bioinformatics/bti803
Beerli, P. (2012). Migrate Documentation Version 3.2.1. Tallahassee, FL: Florida State University Press, 119.
Beerli, P., and Felsenstein, J. (1999). Maximum-likelihood estimation of migration rates and effective population numbers in two populations using a coalescent approach. Genetics 152, 763–773.
Beerli, P., and Felsenstein, J. (2001). Maximum likelihood estimation of a migration matrix and effective population sizes in n subpopulations by using a coalescent approach. Proc. Nat. Acad. Sci. U.S.A. 98, 4563–4568. doi: 10.1073/pnas.081068098
Blanco-Bercial, L., and Bucklin, A. (2016). New view of population genetics of zooplankton: RAD-seq analysis reveals population structure of the North Atlantic planktonic copepod Centropages typicus. Mol. Ecol. 25, 1566–1580. doi: 10.1111/mec.13581
Bone, Q., Kapp, H., and Pierrot-Bults, A. C. (1991). The Biology of Chaetognaths. Oxford: Oxford University Press, 184.
Bucklin, A. (2000). “Methods for population genetic analysis of zooplankton,” in The Zooplankton Methodology Manual eds R. P. Harris, P. H. Wiebe, J. Lenz, H.-R. Skjoldal, and M. Huntley. (London, UK: International Council for the Exploration of the Sea, Academic Press) 533–570.
Bucklin, A., DiVito, K., Smolina, I., Choquet, M., Questel, J. M., Hoarau, G., et al. (2018). “Population genomics of marine zooplankton,” in Population Genomics: Marine Organisms, eds O. P. Rajora and M. Oleksiak (Cham: Springer).
Bucklin, A., Hopcroft, R. R., Kosobokova, K. N., Nigro, L. M., Ortman, B. D., Jennings, R. M., et al. (2010). DNA barcoding of Arctic Ocean holozooplankton for species identification and recognition. Deep Sea Res. II 57, 40–48.
Bucklin, A., Steinke, D., and Blanco-Bercial, L. (2011). DNA barcoding of marine metazoa. Ann. Rev. Mar. Sci. 2011, 471–508.
Catchen, J., Hohenlohe, P. A., Bassham, S., Amores, A., and Cresko, W. A. (2013). STACKS: an analysis tool set for population genomics. Mol. Ecol. 22, 3124–3140. doi: 10.1111/mec.12354
Choquet, M., Smolina, I., Dhanasiri, A. K. S., Blanco-Bercial, L., Kopp, M., Jueterbock, A., et al. (2019). Towards population genomics in non-model species with large genomes: a case study of the marine zooplankton Calanus finmarchicus. R. Soc. Open Sci. 6:180608. doi: 10.1098/rsos.180608
Crawford, D. L., and Oleksiak, M. F. (2016). Ecological population genomics in the marine environment. Brief. Funct. Genomics 15, 342–351. doi: 10.1093/bfgp/elw008
Davey, J. L., and Blaxter, M. W. (2010). RADseq: next-generation population genetics. Brief. Funct. Genomics 9, 416–423. doi: 10.1093/bfgp/elq031
Deagle, B. E., Faux, C., Kawaguchi, S., Meyer, B., and Jarman, S. N. (2015). Antarctic krill population genomics: apparent panmixia, but genome complexity and large population size muddy the water. Mol. Ecol. 24, 4943–4959. doi: 10.1111/mec.13370
Earl, D., and vonHoldt, B. (2012). STRUCTURE HARVESTER: a website and program for visualizing STRUCTURE output and implementing the Evanno method. Conser. Genet. Resour. 4, 359–361.
Edgar, R. C. (2004). Muscle: a multiple sequence alignment method with reduced time and space complexity. BMC Bioinformatics 5:113. doi: 10.1186/1471-2105-5-113
Emerson, K. J., Merz, C. R., Catchen, J. M., Hohenlohe, P. A., Cresko, W. A., Bradshaw, W. E., et al. (2010). Resolving postglacial phylogeography using high-throughput sequencing. Proc. Nat. Acad. Sci. U.S.A. 107, 16196–16200. doi: 10.1073/pnas.1006538107
Etter, P. D., Bassham, S., Hoenlohe, P. A., Johnson, E. A., and Cresko, W. A. (2011). SNP discovery and genotyping for evolutionary genetics using RAD sequencing. Methods Mol. Biol. 722, 157–178. doi: 10.1007/978-1-61779-228-1_9
Evanno, G., Regnaut, S., and Goudet, J. (2005). Detecting the number of clusters of individuals using the software structure: a simulation study. Mol. Ecol. 14, 2611–2620. doi: 10.1111/j.1365-294X.2005.02553.x
Excoffier, L., and Lischer, H. E. L. (2010). Arlequin suite ver 3.5: a new series of programs to perform population genetics analyses under Linux and Windows. Mol. Ecol. Res. 10, 564–567. doi: 10.1111/j.1755-0998.2010.02847.x
Foley, B. R., Rose, C. G., Rundle, D. E., Leong, W., Moy, G. W., Burton, R. S., et al. (2011). A gene-based SNP resource and linkage map for the copepod Tigriopus californicus. BMC Genomics 12:568. doi: 10.1186/1471-2164-12-568
Foll, M., and Gaggiotti, O. (2008). A Genome-Scan method to identify selected loci appropriate for both dominant and codominant markers: a Bayesian perspective. Genetics 180, 977–993. doi: 10.1534/genetics.108.092221
Folmer, O., Black, M., Hoeh, W., Lutz, R., and Vrijenhoek, R. (1994). DNA primers for amplification of mitochondrial cytochrome c oxidase subunit I from diverse metazoan invertebrates. Mol. Mar. Biol. Biotech. 3, 294–299.
Fowler, G. H. (1996). Contributions to our knowledge of the plankton of the Faroe Channel. Proc. Zool. Soc. London 896, 991–999.
Gagnaire, P. A., Broquet, T., Aurelle, D., Viard, F., Souissi, A., Bonhomme, F., et al. (2015). Using neutral, selected, and hitchhiker loci to assess connectivity of marine populations in the genomic era. Evol. Appl. 8, 769–786. doi: 10.1111/eva.12288
Gamfeldt, L., Lefcheck, J. S., Byrnes, J. E., Cardinale, B. J., Duffy, J. E., and Griffin, J. N. (2015). Marine biodiversity and ecosystem functioning: what’s known and what’s next? Oikos 124, 252–265.
Gjøsæter, H., Wiebe, P. H., Knutsen, T., and Ingvaldsen, R. B. (2017). Evidence of diel vertical migration of mesopelagic sound-scattering organisms in the Arctic. Front. Mar. Sci. 4:332. doi: 10.3389/fmars.2017.00332
Grigor, J. J., Schmid, M. S., and Fortier, L. (2017). Growth and reproduction of the chaetognaths Eukrohnia hamata and Parasagitta elegans in the Canadian Arctic Ocean: capital breeding versus income breeding. J. Plankton Res. 39, 910–929.
Grigor, J. J., Søreide, J. E., and Varpe, Ø (2014). Seasonal ecology and life history strategy of the high-latitude predatory zooplankter Parasagitta elegans. Mar. Ecol. Prog. Ser. 499, 77–88.
Helyar, S. J., Hemmer-Hansen, J., Bekkevold, D., Taylor, M. I., Ogden, R., Limborg, M. T., et al. (2011). Application of SNPs for population genetics of nonmodel organisms: new opportunities and challenges. Mol. Ecol. Res. 11, 123–136. doi: 10.1111/j.1755-0998.2010.02943.x
Hohenlohe, P. A., Amish, S. J., Catchen, J. M., Allendorf, F. W., and Luikart, G. (2011). Next-generation RAD sequencing identifies thousands of SNPs for assessing hybridization between rainbow and westslope cutthroat trout. Mol. Ecol. Res. 11, 117–122. doi: 10.1111/j.1755-0998.2010.02967.x
Hopcroft, R. R., Clarke, C., Nelson, R. J., and Raskoff, K. A. (2005). Zooplankton communities of the Arctic’s Canada Basin: the contribution by smaller taxa. Polar Biol. 28, 197–206.
Hopcroft, R. R., Kosobokova, K. N., and Pinchuk, A. I. (2010). Zooplankton community patterns in the Chukchi Sea during summer 2004. Deep Sea Res. II 57, 27–39.
Hubisz, M. J., Falush, D., Stephens, M., and Pritchard, J. K. (2009). Inferring weak population structure with the assistance of sample group information. Mol. Ecol. Res. 9, 1322–1332. doi: 10.1111/j.1755-0998.2009.02591.x
Jennings, R. M., Bucklin, A., and Pierrot-Bults, A. (2010). Barcoding of arrow worms (Phylum Chaetognatha) from three oceans: genetic diversity and evolution within an enigmatic phylum. PLoS One 5:e9949. doi: 10.1371/journal.pone.0009949
Karlin, S., and Altschul, S. F. (1990). Methods for assessing the statistical significance of molecular sequence features by using general scoring schemes. Proc. Nat. Acad. Sci. U.S.A. 87, 2264–2268. doi: 10.1073/pnas.87.6.2264
Kopelman, N. M., Mayzel, J., Jakobsson, M., Rosenberg, N. A., and Mayrose, I. (2015). Clumpak: a program for identifying clustering modes and packaging population structure inferences across K. Mol. Ecol. Res. 15, 1179–1191. doi: 10.1111/1755-0998.12387
Kosobokova, K. N., and Hopcroft, R. R. (2010). Diversity and vertical distribution of mesozooplankton in the Arctic’s Canada Basin. Deep Sea Res. II 57, 96–110.
Kosobokova, K. N., Hopcroft, R. R., and Hirche, H. J. (2011). Patterns of zooplankton diversity through the depths of the Arctic’s central basins. Mar. Biodivers. 41, 29–50.
Kulagin, D. N., Stupnikova, A. N., Neretina, T. V., and Mugue, N. S. (2011). Genetic diversity of Eukrohnia hamata (Chaetognatha) in the South Atlantic: analysis of gene mtCO1. Invert. Zool. 8, 127–136.
Kulagin, D. N., Stupnikova, A. N., Neretina, T. V., and Mugue, N. S. (2014). Spatial genetic heterogeneity of the cosmopolitan chaetognath Eukrohnia hamata (Mobius, 1875) revealed by mitochondrial DNA. Hydrobiol. 721:197.
Kumar, S., Stecher, G., and Tamura, K. (2016). MEGA7: molecular evolutionary genetics analysis version 7.0 for bigger datasets. Mol. Biol. Evol. 33, 1870–1874. doi: 10.1093/molbev/msw054
Lepais, O., and Weir, J. T. (2014). SimRAD: an R package for simulation-based prediction of the number of loci expected in RADseq and similar genotyping by sequencing approaches. Mol. Ecol. Res. 14, 1314–1321. doi: 10.1111/1755-0998.12273
Librado, P., and Rozas, J. (2009). DNASP V5: a software for comprehensive analysis of DNA polymorphism data. Bioinformatics 25, 1451–1452. doi: 10.1093/bioinformatics/btp187
Marlétaz, F., Gilles, A., Caubit, X., Perez, Y., Dossat, C., Samain, S., et al. (2008). Chaetognath transcriptome reveals ancestral and unique features among bilaterians. Genome Biol. 9:R94. doi: 10.1186/gb-2008-9-6-r94
McCormack, J. E., Hird, S. M., Zellmer, A. J., Carstens, B. C., and Brumfield, R. T. (2013). Applications of next generation sequencing to phylogeography and phylogenetics. Mol. Phylogenet. Evol. 66, 526–538. doi: 10.1016/j.ympev.2011.12.007
Miller, M. R., Dunham, J. P., Amores, A., Cresko, W. A., and Johnson, E. A. (2007). Rapid and cost-effective polymorphism identification and genotyping using restriction site associated DNA (RAD) markers. Genome Res. 17, 240–248. doi: 10.1101/gr.5681207
Miyamoto, H., Machida, R. J., and Nishida, S. (2010). Genetic diversity and cryptic speciation of the deep sea chaetognath Caecosagitta macrocephala (Fowler, 1904). Deep Sea Res. II 57, 2211–2219.
Miyamoto, H., Machida, R. J., and Nishida, S. (2012). Global phylogeography of the deep-sea pelagic chaetognath Eukrohnia hamata. Prog. Oceanogr. 104, 99–109.
Narum, S. R., and Hess, J. E. (2011). Comparison of FST outlier tests for SNP loci under selection. Mol. Ecol. Res. 11, 184–194. doi: 10.1111/j.1755-0998.2011.02987.x
Nelson, R. J., Carmack, E. C., Mclaughlin, F. A., and Cooper, G. A. (2009). Penetration of Pacific zooplankton into the western Arctic Ocean tracked with molecular population genetics. Mar. Ecol. Prog. Ser. 381, 129–138.
Paris, J. R., Stevens, J. R., and Catchen, J. M. (2017). Lost in parameter space: a road map for stacks. Methods Ecol. Evol. 8, 1360–1373.
Peijnenburg, K. T. C. A., Fauvelot, C., Breeuwer, A. J., and Menken, S. (2006). Spatial and temporal genetic structure of the planktonic Sagitta setosa (Chaetognatha) in European seas as revealed by mitochondrial and nuclear DNA markers. Mol. Ecol. 15, 3319–3338. doi: 10.1111/j.1365-294X.2006.03002.x
Peijnenburg, K. T. C. A., and Goetze, E. (2013). High evolutionary potential of marine zooplankton. Trends Ecol. Evol. 3, 2765–2783. doi: 10.1002/ece3.644
Peijnenburg, K. T. C. A., van Haastrecht, E. K., and Fauvelot, C. (2005). Present day genetic composition suggests contrasting demographic histories of two dominant chaetognaths of the North-East Atlantic, Sagitta elegans and S. setosa. Mar. Biol. 147, 1279–1289.
Pérez-Figueroa, A., García-Pereira, M. J., Saura, M., Rolán-Alvarez, E., and Caballero, A. (2010). Comparing three different methods to detect selective loci using dominant markers. J. Evol. Biol. 23, 2267–2276. doi: 10.1111/j.1420-9101.2010.02093.x
Peterson, B. K., Weber, J. N., Kay, E. H., Fisher, H. S., and Hoekstra, H. E. (2012). Double digest RADseq: an inexpensive method for de novo SNP discovery and genotyping in model and non-model species. PLoS One 7:e37135. doi: 10.1371/journal.pone.0037135
Pierrot-Bults, A. C. (2008). A short note on the biogeographic patterns of the Chaetognatha fauna in the North Atlantic. Deep Sea Res. II 55, 137–141.
Pierrot-Bults, A. C., and Nair, V. R. (1991). “Distribution patterns in Chaetognatha,” in The Biology of Chaetognaths, eds Q. Bone, H. Kapp, and A. C. Pierrot-Bults (Oxford: Oxford University Press), 86–116.
Polyakov, I. V., Pnyushkov, A. V., Alkire, M. B., Ashik, I. M., Baumann, T. M., Carmack, E. C., et al. (2017). Greater role for Atlantic inflows on sea-ice loss in the Eurasian Basin of the Arctic Ocean. Science 356, 285–291. doi: 10.1126/science.aai8204
Porras-Hurtado, L., Ruiz, Y., Santos, C., Phillips, C., Carracedo, A., and Lareu, M. V. (2013). An overview of STRUCTURE: applications, parameter settings, and supporting software. Front. Genetics 4:98. doi: 10.3389/fgene.2013.00098
Pritchard, J. K., Stephens, M., and Donnelly, P. (2000). Inference of population structure using multilocus genotype data. Genetics 155, 945–959. doi: 10.1111/j.1471-8286.2007.01758.x
Proshutinsky, A., Dukhovskoy, D., Timmermans, M.-L., Krishfield, R., and Bamber, J. L. (2015). Arctic circulation regimes. Philos. Trans. A Math. Phys. Eng. Sci. 373:20140160.
Questel, J. M., Blanco-Bercial, L., Hopcroft, R. R., and Bucklin, A. (2016). Phylogeography and connectivity of the Pseudocalanus (Copepoda: Calanoida) species complex in the eastern North Pacific and the Pacific Arctic Region. J. Plankton Res. 38, 610–623. doi: 10.1093/plankt/fbw025
Questel, J. M., Clarke, C., and Hopcroft, R. R. (2013). Seasonal and interannual variation in the planktonic communities of the northeastern Chukchi Sea during the summer and early fall. Continent. Shelf Res. 67, 23–41.
Reitzel, A. M., Herrera, S., Layden, M. J., Martindale, M. Q., and Shank, T. M. (2013). Going where traditional markers have not gone before: utility of and promise for RAD sequencing in marine invertebrate phylogeography and population genomics. Mol. Ecol. 22, 2953–2970. doi: 10.1111/mec.12228
Rudels, B., Jones, E. P., Anderson, L. G., and Kattner, G. (1994). “On the intermediate depth waters of the Arctic Ocean,” in The Polar Oceans and Their Role in Shaping the Global Environment, eds O. M. Johannessen, R. D. Muench, and J. E. Overland (Washington, DC: American Geophysical Union), 33–46.
Rudels, B., Jones, E. P., Schauer, U., and Eriksson, P. (2004). Atlantic sources of the Arctic Ocean surface and halocline waters. Polar Res. 23, 181–208. doi: 10.1021/acs.est.7b00788
Rudels, B., Muench, R. D., Gunn, J., Schauer, U., and Friedrich, H. J. (2000). Evolution of the Arctic Ocean Boundary current north of the Siberian Shelves. J. Mar. Syst. 25, 77–99.
Schlötterer, C., Tobler, R., Kofler, R., and Nolte, V. (2014). Sequencing pools of individuals–mining genome-wide polymorphism data without big funding. Nat. Rev. Gen. 15, 749–763. doi: 10.1038/nrg3803
Schweyen, H., Rozenberg, A., and Leese, F. (2014). Detection and removal of PCR duplicates in population genomic ddRAD studies by addition of a degenerate base region (DBR) in sequencing adapters. Biol. Bull. 227, 146–160. doi: 10.1086/BBLv227n2p146
Smoot, C. A., and Hopcroft, R. R. (2017a). Cross-shelf gradients of epipelagic zooplankton communities of the Beaufort Sea and the influence of localized hydrographic features. J. Plankton Res. 39, 65–78.
Smoot, C. A., and Hopcroft, R. R. (2017b). Depth-stratified community structure of Beaufort Sea slope zooplankton and its relations to water masses. J. Plankton Res. 39, 79–91.
Tamura, K. (1992). Estimation of the number of nucleotide substitutions when there are strong transition-transversion and G+C-content biases. Mol. Biol. Evol. 9, 678–687. doi: 10.1093/oxfordjournals.molbev.a040752
Terazaki, M. (2000). “Feeding of carnivorous zooplankton, chaetognaths in the Pacific,” in Dynamics and Characterization of Marine Organic Matter, eds N. Handa, E. Tanoue, and T. Hama (Dordrecht: Springer), 257–276.
Terazaki, M., and Miller, C. B. (1986). Life history and vertical distribution of pelagic chaetognaths at Ocean station P in the subarctic Pacific. Deep Sea Res. 33, 323–337.
Thompson, J. D., Gibson, T. J., Plewniak, F., Jeanmougin, F., and Higgins, D. G. (1997). The ClustalX windows interface: flexible strategies for multiple sequence alignment aided by quality analysis tools. Nuclic Acids Res. 25, 4876–4882. doi: 10.1093/nar/25.24.4876
Thompson, J. D., Higgins, D. G., and Gibson, T. J. (1994). CLUSTAL W: improving the sensitivity of progressive multiple sequence alignment through sequence weighting, position-specific gap penalties and weight matrix choice. Nuclic Acids Res. 22, 4673–4680. doi: 10.1093/nar/22.22.4673
Thuessen, E. V., Numachi, K., and Nemoto, T. (1993). Genetic variation in the planktonic chaetognaths Parasagitta elegans and Eukrohnia hamata. Mar. Ecol. Prog. Ser. 101, 243–251.
Ulloa, R., Palma, S., and Silva, N. (2000). Bathymetric distribution of chaetognaths and their association with water masses off the coast of Valparaiso. Chile Deep Sea Res. 47, 2009–2027.
Wassmann, P. (2015). Overarching perspectives of contemporary and future ecosystems in the Arctic Ocean. Prog. Oceanogr. 139, 1–12.
Weingartner, T., Aagaard, K., Woodgate, R., Danielson, S., Sasaki, Y., and Cavalieri, D. (2005). Circulation on the north central Chukchi Sea shelf. Deep Sea Res. II 52, 3150–3174.
Weydmann, A., Coelho, N. C., Serrão, E. A., Burzyński, A., and Pearson, G. A. (2016). Pan-Arctic population of the keystone copepod Calanus glacialis. Polar Biol. 39, 2311–2318.
Weydmann, A., Przyłucka, A., Lubośny, M., Walczyńska, K. S., Serrão, E. A., Pearson, G. A., et al. (2018). Postglacial expansion of the Arctic keystone copepod Calanus glacialis. Mar. Biodivers. 48, 1027–1035.
Woodgate, R. A., Aagaard, K., Swift, J. H., Smethie, W. M., and Falkner, K. K. (2007). Atlantic water circulation over the Mendeleev Ridge and Chukchi Borderland from thermohaline intrusions and water mass properties. J. Geophys. Res. 112:C02005.
Keywords: zooplankton, population connectivity, Arctic Ocean, single nucleotide polymorphisms, DNA barcodes, Chaetognatha
Citation: DeHart HM, Blanco-Bercial L, Passacantando M, Questel JM and Bucklin A (2020) Pathways of Pelagic Connectivity: Eukrohnia hamata (Chaetognatha) in the Arctic Ocean. Front. Mar. Sci. 7:396. doi: 10.3389/fmars.2020.00396
Received: 15 November 2019; Accepted: 07 May 2020;
Published: 03 June 2020.
Edited by:
Haiwei Luo, The Chinese University of Hong Kong, ChinaCopyright © 2020 DeHart, Blanco-Bercial, Passacantando, Questel and Bucklin. This is an open-access article distributed under the terms of the Creative Commons Attribution License (CC BY). The use, distribution or reproduction in other forums is permitted, provided the original author(s) and the copyright owner(s) are credited and that the original publication in this journal is cited, in accordance with accepted academic practice. No use, distribution or reproduction is permitted which does not comply with these terms.
*Correspondence: Ann Bucklin, ann.bucklin@uconn.edu
†Present address: Hayley M. DeHart, Computational Biology Institute, The George Washington University, Washington, DC, United States