- School of Natural Sciences, Ryan Institute, National University of Ireland Galway, Galway, Ireland
With the increased uncertainty introduced through climate change and fishing pressure, having accurate estimates of fish biomass is essential for global ecosystem and economic health. Acoustic surveys are an efficient way to determine population size for pelagic species in the Northeast Atlantic (NEA), but acoustic population estimates still contain uncertainty and are difficult for some species. For example, Atlantic mackerel (Scomber scombrus) is one of the most valuable fisheries in the NEA and is not monitored acoustically, as mackerel lack the swim bladder that provides the strongest acoustic echo (target strength) at common assessment frequencies. For all pelagic species, and especially for mackerel, behavior is a source of variation in acoustic measurements and therefore in population estimates. Behavior is mediated by both extrinsic and intrinsic factors, such as the environment and the life history of the fish. In turn, behavior affects the density of the shoal and the tilt angle of the fish relative to the survey vessel, affecting their target strength, which affects the biomass estimate. Some fish may also undergo an anti-predator response to survey vessels, changing their behavior in response to the survey. Understanding these behaviors and incorporating them into acoustic stock assessment methods can improve the accuracy of population estimates. Individual-based models (IBM) of fish shoals provide a pathway for incorporating behavior into acoustic methods. IBMs have been used extensively to build theoretical models of fish shoals, but few have been successfully tested in lab or field conditions. As computational power and monitoring technology improve, modeling the collective behavior of pelagic fishes will be possible. Novel, interdisciplinary approaches to data collection and analysis will help translate theoretical IBMs to the fisheries science domain. Beyond acoustic stock assessments, this approach can be used to investigate knowledge gaps in the effects of fisheries-induced evolution and the potential for range shifts under climate change. Further work to synthesize existing models and incorporate field data will help determine how environmental, ecological, physiological, and anthropogenic factors, often affecting both behavior and acoustic surveying, are interconnected. Moving from theoretical models to practical applications will be a valuable tool in tackling the uncertainty that accompanies further fisheries exploitation and warming oceans.
Introduction
As fishing pressure and climate change intensify, so does the need for well-informed and standardized fisheries management. Ineffective management could lead to ecosystem collapse and widespread food shortages (Scheffer et al., 2005; Srinivasan et al., 2010). Effective management, in addition to well-crafted legislation and incentives, must be based on the most accurate possible estimation of fisheries stock size (Burgess et al., 2017; Zimmermann and Werner, 2019). In the Northeast Atlantic (NEA), small pelagic fishes, such as Atlantic mackerel (Scomber scombrus), Atlantic herring (Clupea harengus), blue whiting (Micromesistius poutassou), horse mackerel (Trachurus trachurus), and capelin (Mallotus villosus) are high-value catches, representing 37% of global fisheries landings (Alder et al., 2008). Fished for both human consumption and animal feed, their large shoals mean low effort for commercial fisheries (Biseau, 1998). They are also essential to the pelagic and benthic ecosystems, transferring energy from low-trophic plankton to predators such as larger fish, seabirds, and marine mammals (Essington et al., 2015).
The population size for the majority of these species is assessed with acoustic surveys, an efficient and effective method for estimating stock size, especially when combined with trawls to determine the finer-scale population dynamics such as age and condition (Massé, 1996; Georgakarakos et al., 2011). All acoustic stock estimates are, however, an estimate, and while huge advances in technology and fisheries science have reduced the bias inherent in the method, there are limits to how much information can be gleaned from an acoustic survey. The behavior of fish, and how that affects the density recorded by the echosounder, can complicate the final biomass estimate (Fréon et al., 1993).
Behavior affects all assessments for gregarious fishes and models of shoaling behavior can be adapted to any of these species. Including behavior is especially important for developing an acoustic protocol for monitoring Atlantic mackerel, which form large shoals and lack the swim bladder that provides the strongest acoustic echo at the commonly-used frequencies (Simmonds and MacLennan, 2005; Korneliussen, 2010). Mackerel is one of the most valuable, and most controversial, pelagic fisheries species. Mackerel distributions are largely mediated by temperature. Therefore, their range may expand poleward and into deeper waters with increasing temperatures (Dulvy et al., 2008; Hughes et al., 2015; van der Kooij et al., 2016; Ólafsdóttir et al., 2019). The shift in mackerel distributions precipitated a dispute over the total allowable catch (TAC) for the NEA, a region shared by the European Union, Norway, Iceland, and the Faroe Islands (Hughes et al., 2015; Jensen, 2015; Spijkers and Boonstra, 2017). These disputes were worsened by decreases in other fish catches, such as blue whiting (Jensen, 2015). With climate change, the ranges of pelagic fishes may shift (Pecl et al., 2014), potentially creating conflict (Spijkers and Boonstra, 2017) and economic impacts (Hughes et al., 2015).
Reducing the uncertainty in mackerel stock assessments will require extensive and standardized evaluation of their spatial distributions, population size, and behavior. The estimates of NEA population size are based on surveys of mackerel egg biomass, catch-at-age data, and tagging and recapture, all of which are subject to biases and error (Tenningen et al., 2011). For example, a 2005 International Council for Exploration of the Seas (ICES) report on acoustic and aerial surveys for mackerel in the North Sea produced an abundance estimate that was lower than the fishery catch (ICES, 2005; Scoulding et al., 2017). Until 2014, there was no standardized quantitative assessment criteria between the agencies assessing the NEA stock, contributing to uncertainty in the calculation of the total stock biomass (ICES, 2014). Because of this uncertainty, ICES, who reviewed the NEA mackerel stock, recommended a 20% reduction in catch levels as a “precautionary buffer” (ICES, 2014).
Improvements in acoustic techniques and novel approaches incorporating individual behavior, however, are providing a pathway to monitoring mackerel acoustically (Scoulding et al., 2017), reducing the possibility that the stock will be overfished before monitoring catches up with the current state of the population. While there are many avenues for improving acoustic monitoring, one possibility is to use models of collective fish behavior to inform density estimates. While this is a theoretical approach, it provides ample opportunities for research that bridges theory and practice and brings the broad bodies of work in ethology and acoustics to bear on a new problem. This approach could inform all species management processes, with the most impact for species like mackerel.
Fisheries Acoustics and Behavior
Acoustic Survey Techniques
Acoustic surveys serve as the basis for population estimates and stock assessments for many species, informing effective fisheries management. The population size of gregarious fishes is difficult to estimate because of their large geographic ranges and dense shoals. Before 1960, fish abundance estimates relied on catch per unit effort (CPUE) data collected from commercial fisheries, which was biased by the constant improvements in commercial fishery technology and the lack of an accurate relationship between CPUE and stock size (Gunderson, 1993). In the 1970s, catch-at-age and cohort analyses based on scientific trawl data became more popular, initiating the move to fisheries-independent assessments. These methods were more accurate, but were subject to bias from erroneous age estimates and the mortality coefficients used in the analysis (Gunderson, 1993). There is still uncertainty in fisheries-independent assessments, however, and they are entirely dependent on the data collected during scientific surveys (Mesnil et al., 2009).
Acoustic surveys, conducted along transect lines, are an expedient option for estimating populations, as they can cover large areas in short timeframes and estimate density with low effort (Figure 1). They also provide information on species absence, highlighting distribution changes and migration patterns (Georgakarakos et al., 2011). Acoustic surveys are carried out with echosounders, which consist of a transmitter that produces a burst of energy at a particular frequency, passed through a transducer that converts this energy to acoustic energy (sound). This acoustic pulse is emitted in a focused beam that can propagate through water (Simmonds and MacLennan, 2005).
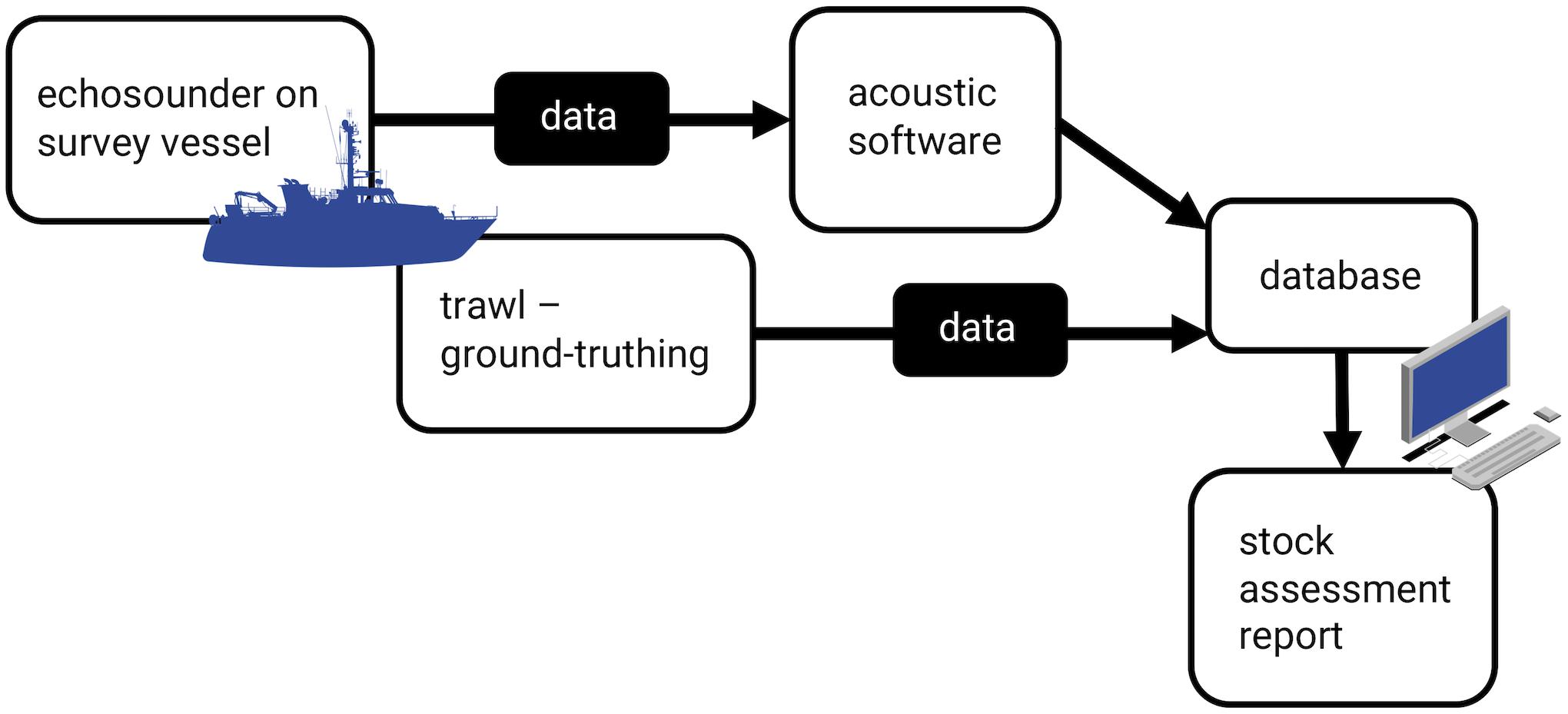
Figure 1. Acoustic stock assessment workflow, from data collection with an echosounder and ground-truthing with trawl data to data processing and report generation.
Modern fisheries survey vessels are equipped with echosounders that run on one or multiple frequencies (commonly from 18 to 333 kHz) and are used for estimating fish biomass and distribution, along with zooplankton populations, oceanographic processes, and other applications (Demer et al., 2017). The data from the echosounder are processed with software that infers the species present and the approximate biomass from shoal shape, location, and behavior (Reid and Simmonds, 1993; Scalabrin, 1996). Species identification is based on descriptive factors for each species, determined by their acoustic resonance on various frequencies (target strength) in various orientations and conditions, determined experimentally (Nakken and Olsen, 1977; Simmonds and MacLennan, 2005; Korneliussen, 2018). The echosounder software uses algorithms based on predictive neural networks and Bayesian networks to identify species (Georgakarakos et al., 2011). These identifications are ground-truthed by fishing a target shoal and recording species present and relative and total biomass (Georgakarakos et al., 2011). The trawls also provide data on the size, sex, life stage, and condition of the fish. The accuracy of these algorithms determines the accuracy of the density estimates. Simulated backscatter from virtual shoals indicates how bias in estimates of shoal properties can vary with an interaction of detection range and shoal density (Trygonis and Kapelonis, 2018). Hence, algorithms can be confounded by shoaling behavior (Fréon et al., 1992; Scalabrin, 1996; Godø et al., 2004; Hensor et al., 2005; Lopez et al., 2012) and physiological differences between fish species (Blaxter and Batty, 1990; Misund, 1993; Misund and Beltestad, 1996; Scalabrin, 1996).
The essence of collective behavior is that the group is more than just a collection of individuals and fisheries acoustics perceive the behavior of the shoal as a whole, rather than an aggregate of separate individual signals. Collective behavior facilitates feeding, reproduction, and predator avoidance and can vary between species and with environmental conditions (Rieucau et al., 2014; Handegard et al., 2017). Shoals of fish, defined as a group engaged in social behavior beyond resource exploitation and following one direction, and schools of fish, the same following one speed and approximately less than a body length between individuals, are common in the open ocean (Pitcher, 1983; Delcourt and Poncin, 2012; Rieucau et al., 2014). These dense groups introduce variation and therefore uncertainty into acoustic population estimates (Fréon et al., 1993). The echosounder software performs a series of calculations that are based on “single target detector” algorithms that sort through the acoustic echoes of the group to filter out the echoes from individuals (Georgakarakos et al., 2011). These algorithms perform best in low-density, monospecific conditions. Therefore, larger shoals, where the signal-to-noise ratio is lower, can introduce bias where the algorithms can fail to reject multiple echoes from the same individual (Georgakarakos et al., 2011). Density varies both between shoals and within individual groups, with holes and density nuclei shifting in time (Lopez et al., 2012). While ground-truthing with trawls can confirm species composition and provide other biological details, especially important for multi-species shoals (Massé, 1996), overall density is hard to verify, as much of the backscatter from fish depends on their distance from and angle relative to the acoustic beam, in addition to numbers (De Robertis and Handegard, 2013; Trygonis and Kapelonis, 2018).
Incorporating Behavior Into Acoustic Assessments
Though predation risk is often seen as the main driver for fish aggregations, shoal dimensions and intra- and inter-shoal density can depend on multiple other behavioral and environmental factors. These include the geographical distances traversed, motivation of the fish (seeking food or seeking safety), reproductive stage, position within the water column, time of day, oxygen levels and consumption, and water flow (Tables 1, 2). The factors affecting individual behavior and hence shoal structure can be considered as imposed on the shoal (extrinsic variables like time of day) or originating from the biology of the fish (e.g., feeding state). Incorporating these variables into surveys of fish abundance is difficult because some of the same variables that affect fish behavior also affect the acoustic surveying process (Table 3).
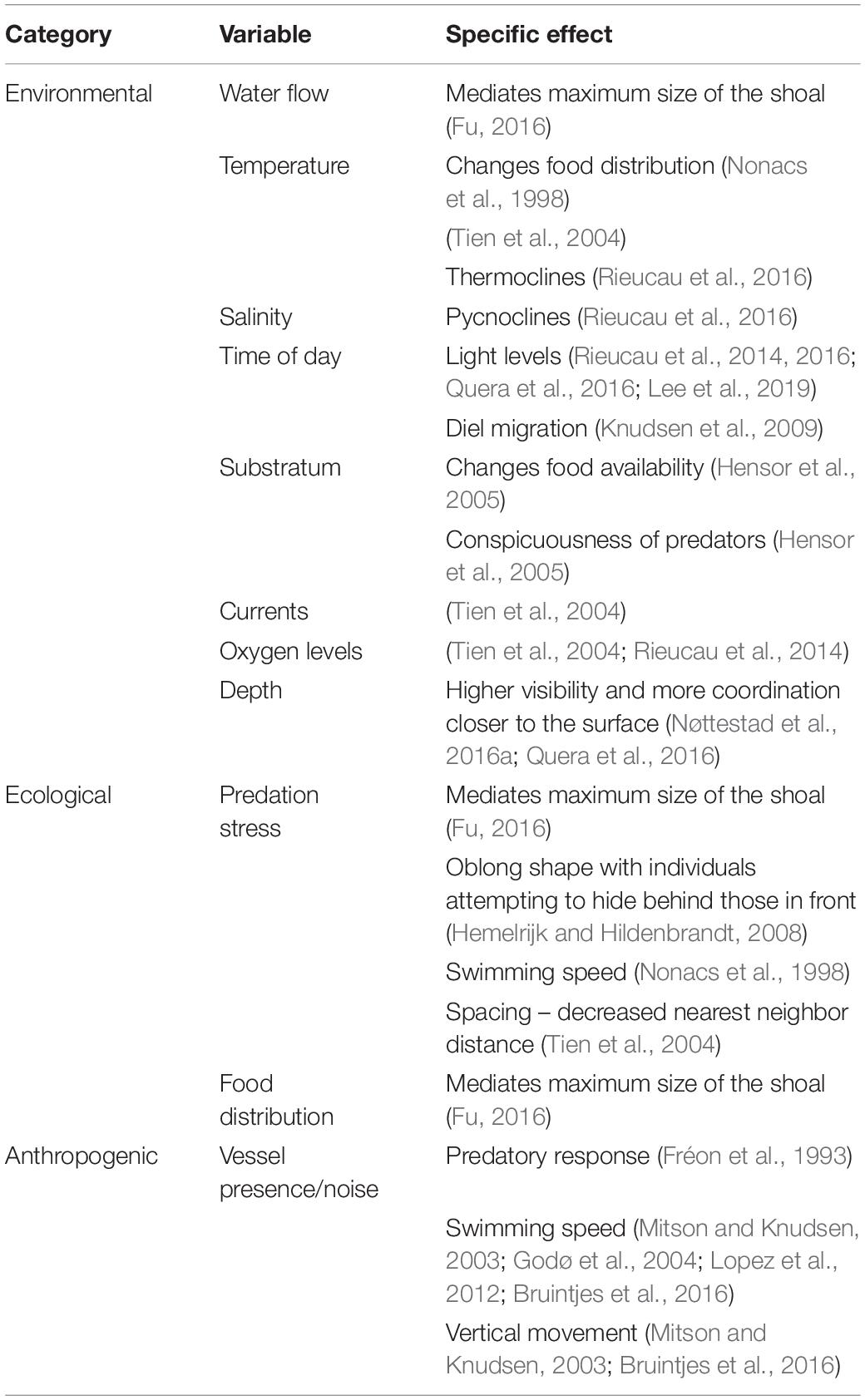
Table 1. Extrinsic sources of variability in individual behavior that may affect the overall shape and density of a shoal and therefore acoustic measurements, where they are mentioned in the literature, and the specific effects of these variables, if stated.
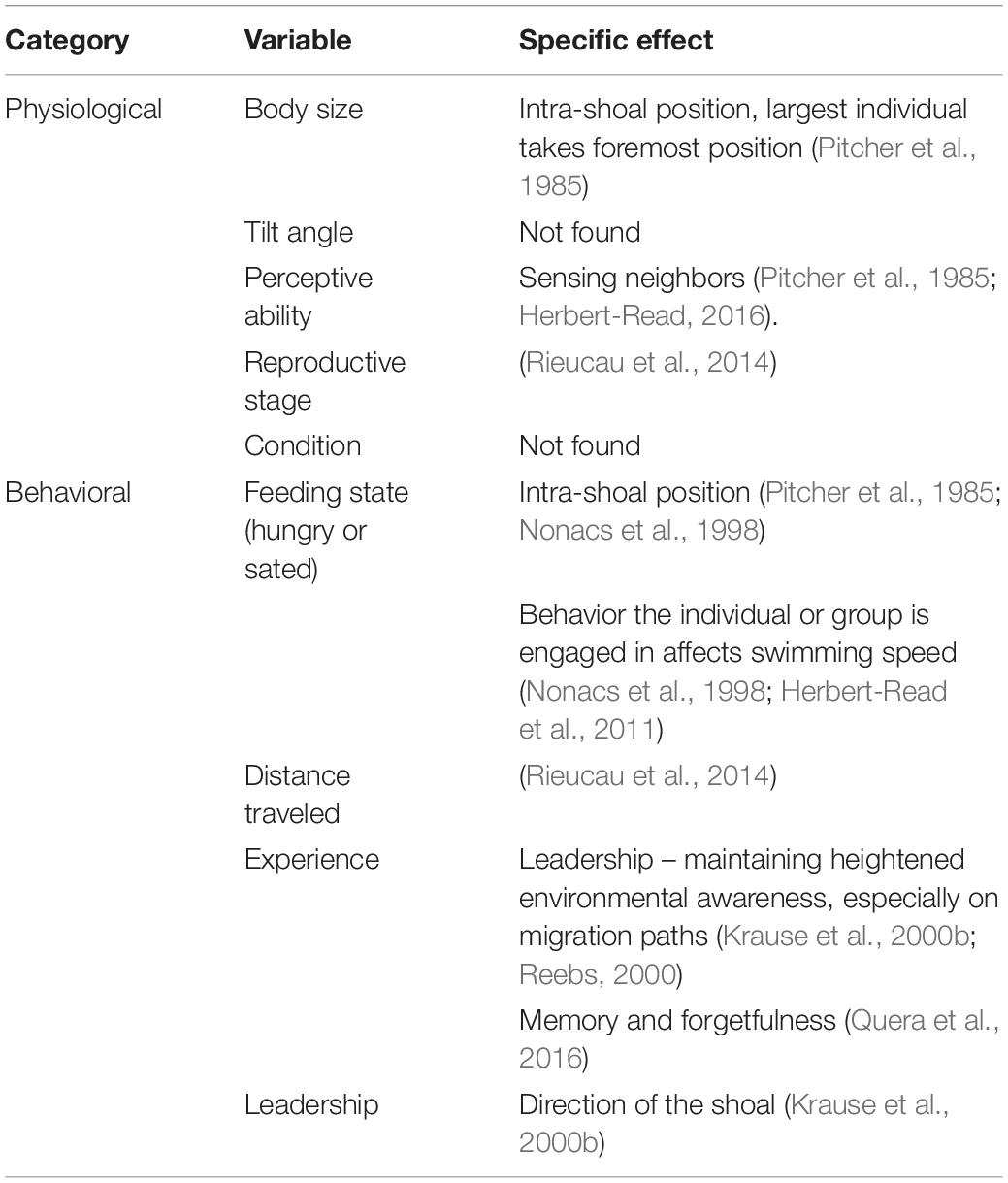
Table 2. Intrinsic sources of variability in individual behavior that may affect the overall shape and density of a shoal and therefore acoustic measurements, where they are mentioned in the literature, and the specific effects of these variables, if stated.
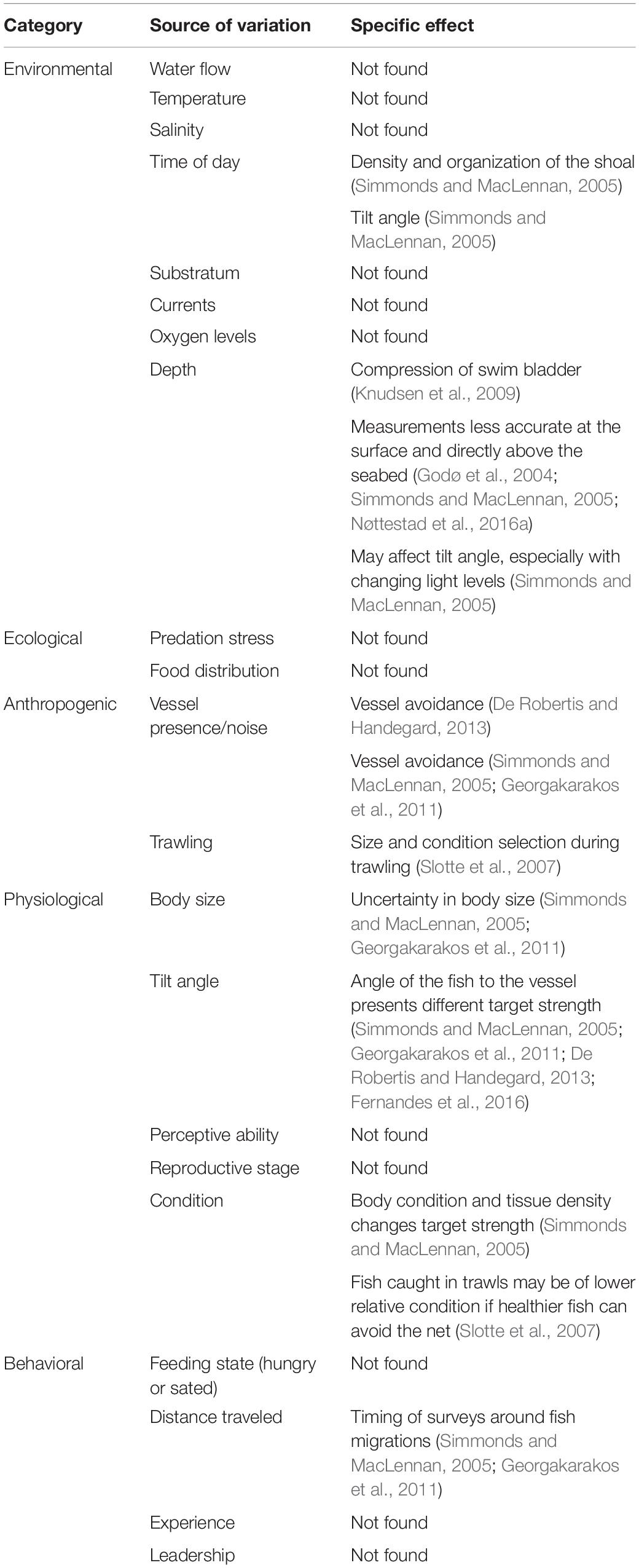
Table 3. Potential sources of variability in the acoustic survey process (beyond standard calibration settings accounting for local conditions and vessel/echosounder capacity), if found in the literature, and the specific effect, if stated.
Acoustic survey technology and analysis are continually improved, but still include assumptions about behavior that introduce bias into the abundance estimates. Target strength calculations assume even distributions within shoals and often only account for variation in the length of the fish (Hazen and Horne, 2003). In reality, further variation introduced from uneven densities within the shoal and biased recording of the edge of a larger group can lead to incorrect abundance estimates (Simmonds and MacLennan, 2005). Just as intra-shoal density is not consistent, the body size and condition of fish within the shoal may not be evenly distributed. The fish on the edge of a shoal may differ physiologically and behaviorally in important ways. Some fish have been shown to sort themselves by body size (Pitcher et al., 1985) and individuals on the edge may be hungry and seeking food, rather than seeking safety in the center of the shoal (Rieucau et al., 2014).
In addition to boundary effects and physiological variation, behavior affects the orientation of the fish relative to the echosounder beam. This tilt angle of the fish affects the target strength, as the orientation of the fish affects which tissues are along the acoustic beam and different tissues have different target strengths. Tilt angle is especially important for species without swim bladders, where other tissues (i.e., the backbone) provide the best acoustic echo (Hazen and Horne, 2003; Simmonds and MacLennan, 2005). Experimental determinations of target strength from scenarios where the fish are held in a fixed-position or in fish cages cannot replicate the complex conditions of the open ocean and large shoals. Therefore, tilt angle in situ remains an important and elusive behavioral determinant of acoustic abundance estimate accuracy (Reid and Simmonds, 1993; Fernandes et al., 2016).
Vessel Avoidance
Beyond more static environmental and behavioral factors, some research suggests that fish perceive the survey vessel as a threat and undergo avoidance behaviors, though improvements in the acoustic profile of vessels has mitigated this issue. Fréon et al. (1993) reported that the low-frequency noise from some vessels causes avoidance behavior that can reduce shoal density by 40–90% in some studies, but only weak or no reaction in others. There is continued evidence to both support and refute avoidance behavior in various species and scenarios (Fernandes et al., 2000; Mitson and Knudsen, 2003; Knudsen et al., 2009). High noise can disrupt the social behaviors that fish rely on for survival and reproduction and can affect individuals’ ability to coordinate their movements by interrupting the transfer of information through the lateral line, such as their neighbors’ position. Noise can also affect other sensory inputs, such as vision and olfaction (Herbert-Read et al., 2017). This communication interference, combined with possible effects on stress, injury rate, feeding rate, predator avoidance, and swimming behavior, can lead to lower catch rates, especially when bottom trawling.
Fish may avoid the path of the vessel, horizontally or vertically, changing their tilt angle relative to the acoustic beam (Mitson and Knudsen, 2003; De Robertis and Handegard, 2013; Bruintjes et al., 2016; Brehmer et al., 2019). Changes in tilt angle from diving or surfacing to avoid the vessel affects the backscatter of the shoal, causing the signal to change with the orientation of the fish to the vessel, rather than a change in shoal density or distribution (Fréon et al., 1993; De Robertis and Handegard, 2013). In addition, the expulsion of air from the swim bladder when diving can affect the backscatter; in herring, a dive from 50 to 90 m results in an 11% reduction in target strength (Knudsen et al., 2009; De Robertis and Handegard, 2013). Laterally, vessel avoidance can also reduce the likelihood of a shoal being detected by the echosounder, the magnitude of which depends on whether the change in position happens before or after the fish have been measured by the echosounder (De Robertis and Handegard, 2013). Trawl avoidance may also affect the biological sampling during research cruises; Slotte et al. (2007) found that research trawls contained smaller, more immature, and possibly “weaker” fish than the catch from commercial trawlers. Therefore, the biological data used to determine the shoal composition and therefore population structure may also be biased by avoidance behavior (Slotte et al., 2007).
The effect that vessel avoidance has on population estimates depends on how stock assessments are used. While vessel avoidance introduces bias into the stock assessment pipeline, this only becomes an issue when the assessment is used as an absolute representation of population, or if the inclusion of bias varies between years (De Robertis and Handegard, 2013). The magnitude of response also depends on the type of vessel and the hydrographic conditions, such as time of day, physiological state, and predation risk. The introduction of noise-reduced vessels has lessened the impact of noise (Fernandes et al., 2000), though vessel avoidance remains a source of bias (Georgakarakos et al., 2011; De Robertis and Handegard, 2013).
Difficult Fish to Sample Acoustically – Northeast Atlantic Mackerel
While swim bladders provide the strongest acoustic echo for most fishes, Atlantic mackerel (Scomber scombrus) lack a swim bladder, meaning that acoustically measuring their population size is difficult at the most common acoustic frequency (i.e., 38 kHz; Korneliussen, 2010). While the large shoals that mackerel form mean they are easily found, determining density is difficult. The mackerel egg survey was the only source of fisheries-independent data for the NEA mackerel stock for a long time, but changes in the distribution of mackerel eggs and in the timing of spawning affect the survey’s accuracy (Slotte et al., 2007; van der Kooij et al., 2016). Acoustic or sonar monitoring is the preferred way to carry out fisheries-independent surveying, so research has been carried out in an effort to update and parameterize the existing framework to work for mackerel.
Multifrequency acoustics can be used to identify mackerel, combining frequencies ranging from 18 to 364 kHz. The backbone provides the highest target strength at 200 kHz, instead of the standard 38 kHz (Gorska et al., 2005; Korneliussen, 2010; Fernandes et al., 2016). Even with an expanded frequency range, the target strength of mackerel is low and, therefore, correct identification of species is even more important. For example, the incorrect identification of herring as mackerel at these frequencies would overestimate the abundance by 20 times (Fernandes et al., 2016). Additionally, while estimating the correct tilt angle is important for all fish species, in mackerel, it is essential for correctly estimating abundance (Hazen and Horne, 2003; Fernandes et al., 2016). The difference in target strength for mackerel with a tilt angle of 1° vs. 15°can double the abundance estimate (Scoulding et al., 2017). When expanded over the survey area, this can have a considerable effect on the total estimates of density, as in 2005, when the mackerel abundance estimate for the North Sea was lower than the catch for the fishery (ICES, 2005; Scoulding et al., 2017). For all pelagic fishes, but especially mackerel, novel techniques to determine how behavior affects density and orientation are essential to improving stock assessment techniques and therefore estimates of population size.
Along with multifrequency acoustic surveys, the algorithms for differentiating mackerel from other species, such as capelin and sandeel (Ammodytes marinus) have been successful (Korneliussen et al., 2016). With these improvements, estimates for the stock in Norway are determined acoustically while fish are aggregating in the feeding grounds before migrating south to spawn (Korneliussen and Ona, 2004; Slotte et al., 2007). A method for the acoustic monitoring of mackerel has not, however, been implemented across the NEA region. Issues with vessel avoidance due to mackerel’s swimming speed may introduce bias into the sample of mackerel in research trawls. Slotte et al. (2007) suggest that mackerel exhibit size-dependent trawl avoidance, as the age, length, and condition of mackerel caught in the slower and smaller research trawls was significantly lower than the fish caught during purse seining in the same area. The acoustic surveys carried out in the Norwegian Sea may also be possible because of the behavior of the mackerel, grouped into schools, predominately in the top 40 m, whereas their behavior changes and may be more difficult to measure acoustically at other points in their migration (Godø et al., 2004; van der Kooij et al., 2016). While some opportunistic sampling has been successful in identifying mackerel, such as the work conducted by van der Kooij et al. (2016) during the North Sea International Bottom Trawl Survey, there is still no overarching method. Developing a protocol will require extensive work to determine how the behavior of mackerel varies across their migration route and how these behaviors affect their acoustic properties.
Modeling Collective Behavior
Determining How Fish Move and Behave With Individual-Based Models
Many commercial species are gregarious, forming aggregations to serve a particular purpose, such as facilitating mating, increasing foraging efficiency, or mitigating risk from predators (Rieucau et al., 2014; Reuter et al., 2016). One method for improving stock assessments is to decrease the assumptions made about the behavior of individual fish within the shoal. Though predation risk is often considered the main driver of aggregations, changes in the dimensions and density of the school can depend on a variety of factors (Table 1).
Developing a quantitative description of how individual fish interact within a group is essential for understanding how animals behave (Gautrais et al., 2012), yet there are few species whose collective behavior has been effectively quantified and the definitions of behaviors and modeling methods vary across the literature. Modeling collective behavior depends on quantifying the relationships between individuals, balancing biological reality and theoretical simplicity. Quantification is generally based on measuring the basic dimensions of the group and determining the degree of cohesion. Measurements of cohesion can be the mean distance to the center of the aggregation or the distance between individuals (Delcourt and Poncin, 2012).
Incorporating behavior into models of fish density can be achieved with individual-based models (IBMs)1, which model the interactions of individual agents within an environment with a simple set of rules. In addition to modeling collective behavior, IBMs and particle models have been used to model fish distributions with environmental factors, such as for mackerel (Boyd et al., 2018) and capelin (Barbaro et al., 2009a, b). Before IBMs, most movement ecology used “state-variable models,” which do not incorporate location and assume that each individual has equal influence on the others (Huston et al., 1988). With IBMs, the interactions between individuals are local; an individual is affected only by the other individuals and the environment with which it comes in contact, facilitating modeling of variance on the small scale (Huston et al., 1988). IBMs also include individual variability and stochastic processes, allowing the actions of individuals to build the behavior of the group as a whole (Reuter et al., 2016).
An IBM consists of three aspects: (1) the individual agents, including their attributes and behavior, (2) the relationships between the agents: how they are connected and how they interact, and (3) the environment (Macal and North, 2010). The fundamental assumption of an IBM is that all individuals are self-contained and uniquely identifiable. Older versions of IBMs required the individuals to be identical, but newer methods allow for heterogeneous individuals whose goals, behaviors, and resource loads can vary (Huston et al., 1988; Macal and North, 2010). Additionally, the individuals must be autonomous: programmed behaviors must not curtail their independent decision-making. Individuals also have a state that can vary over time and that is subject to their environment (Macal and North, 2010).
Fish shoal IBMs have generally been based on three rules: attraction to, separation at a minimum distance from, and alignment with neighbors to establish collective behavior (Figure 2; Couzin et al., 2002; Tien et al., 2004; Hensor et al., 2005; Hemelrijk and Hildenbrandt, 2008; Quera et al., 2016). These models are based on the Boids model, developed by Reynolds (1987), which follows this three parameter framework. While these models create a theoretical, cohesive shoal, they include huge assumptions, such as constant speed, large perception ranges, and small school sizes (Couzin et al., 2002; Hemelrijk and Hildenbrandt, 2008).
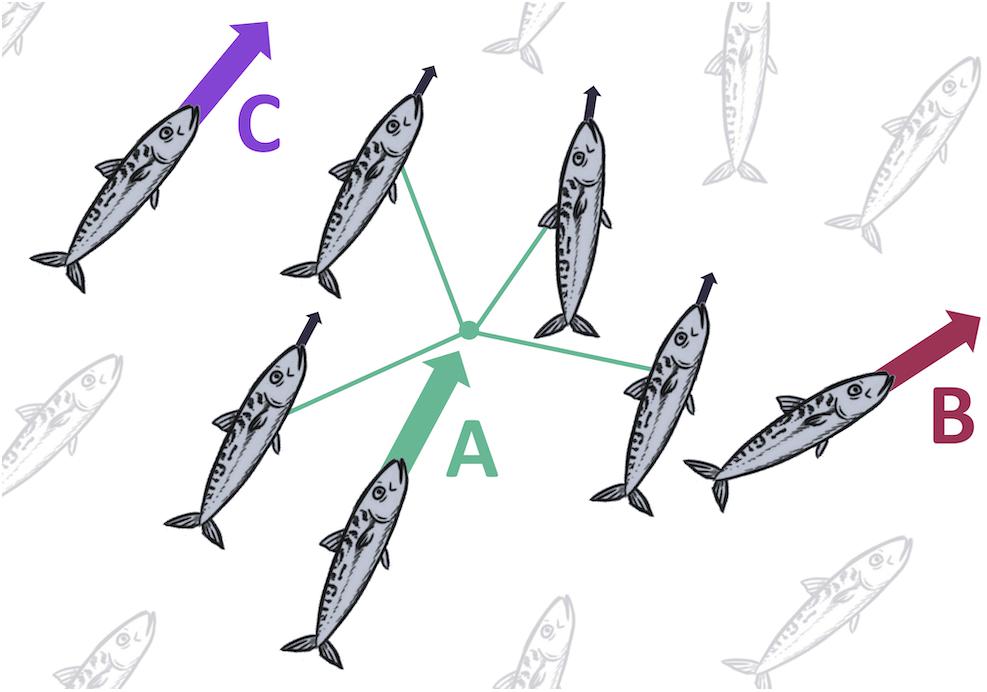
Figure 2. Interaction rules that form the basis of a “Boids” model (Reynolds, 1987), often used in collective behavior IBMs. Rules are (A) attraction to mean position of nearest neighbors; (B) avoidance of other individuals at close proximity; (C) alignment with neighbors.
Improvements to this basic model have included changing the rules to be based on cohesion, separation, and alignment zones instead of set limits (Tien et al., 2004; Hemelrijk and Hildenbrandt, 2008), and incorporating a blind zone behind each “fish” to represent the sensory capabilities of vision and the lateral line (Hemelrijk and Hildenbrandt, 2008; Rountree and Sedberry, 2009). These models can be expanded to include different behavioral states, such as food-seeking or safety-seeking (Pitcher et al., 1985; Nonacs et al., 1998; Krause et al., 2000a, b; Reebs, 2000), presence of a threat (Tien et al., 2004), leadership (Huth and Wissel, 1992; Krause et al., 2000b), methods for information transfer through the shoal (Ward et al., 2011; Rieucau et al., 2016), and environmental conditions such as thermoclines, pycnoclines, and light levels (Fu, 2016; Rieucau et al., 2016). While the specific effects of these variables on collective behavior in situ are not fully-understood, IBMs are a useful tool for modeling shoaling behavior, even with spatial heterogeneity (Reuter et al., 2016).
Validating IBMs for Fish Behavior
Many shoaling IBMs are purely theoretical, and while there have been a number of studies of collective behavior under lab and controlled field conditions, such as aquaculture pens, few studies have attempted to validate their models using open field data. These in situ studies are necessary to fully understand collective behavior, but moving from theoretical models to a replication of real-world systems is difficult to undertake (Krause et al., 2000a; Lopez et al., 2012). First, while humans are adept at distinguishing collective patterns in both simulated and real video, machines are not always as accurate (Butail et al., 2013). Additionally, most research has focused on a single species, outside of its ecological context. That context can be important; for example, species from high-predation habitats have been shown to form larger, tighter groups, evidence that predation pressure may shape collective behavior (Ioannou, 2017). There is ample evidence that factors such as predation affect group dynamics, but it remains unclear how these behaviors emerge from the group’s decision-making process. For example, Ioannou (2017) modeled killifish behavior in the lab and the field and found that their shoaling model could not handle the thousands individuals in naturally-occurring shoals, let alone explain their behavior. Ioannou (2017) also found that the substratum likely had an effect on the fishes’ behavior because the type of substrata was tied to food availability and the visibility of predators. The number of extraneous biotic and abiotic factors in the field made predicting movement difficult (Hensor et al., 2005).
Many emergent group behaviors can be understood and modeled without a thorough understanding of the underlying rules. However, comprehension of the rules governing individual interactions is important for understanding the evolution of social behaviors and the conditions that affect them (Mann, 2011). It is well-accepted that complex group behaviors derive from simple rules, but the details of the interactions, i.e., whether there is a leader or the number of neighbors an individual interacts with, are unknown for most groups (Lukeman et al., 2010; Herbert-Read et al., 2011). It is not enough to simulate collective behavior; any model needs to be compared to real behavior (Lukeman et al., 2010). Inferring interaction rules from data is often done by correlating measurable aspects of individual behavior and interactions with neighbors (Mann et al., 2013).
One of the complications with comparing a theoretical model to empirical data is that the theoretical models rarely incorporate the environment and also rarely represent the entirety of a fish shoal. Many others focus on either individual behaviors, such as speed and direction changes, or collective phenomena, rather than both. Various studies have determined quality-of-fit and model parameters through Bayesian statistics (Mann, 2011; Lopez et al., 2012; Mann et al., 2013; van der Vaart et al., 2015). This method requires the central assumption that fine-scale behavior leads to group behavior and therefore one can be used to test the other. Different individual rules can result in the same collective behavior, deemed IBMs’ “Achilles heel” by Eriksson et al. (2010), and therefore small-scale dynamics can be more useful in determining interaction rules (Mann, 2011). Bayesian paradigms are best-suited to deal with the uncertainty inherent to IBMs because Bayesian inference uses a probability distribution to determine the model parameters, rather than absolute values (Mann, 2011). Additionally, Bayesian methods allow researchers to examine many competing hypotheses, while avoiding over-fitting (Mann et al., 2013). Other methods for assessing theoretical models are through artificial neural networks, which were used by Herbert-Read et al. (2011) to tease out the influence of social cues vs. environmental topography on individual movement. The authors found that while their model may have represented the experimental data effectively, it would not necessarily accurately depict group behavior under all circumstances, a point also made by Katz et al. (2011) when looking at the effect of predator detection on collective behavior. Katz et al. (2011) found that while the model could reproduce the patterns observed, the response in the lab is likely less dynamic than in the real system.
Balancing Complexity and Parsimony in Collective Behavior Models
Replicating an open ocean scenario requires the incorporation of both behavioral and environmental variables, but there is a balance between the most “accurate” model and an effective one. While leadership (Huth and Wissel, 1992; Krause et al., 2000b; Lopez et al., 2012) and memory (Huth and Wissel, 1992; Couzin et al., 2002; Lopez et al., 2012; Biro et al., 2016) are important aspects of collective behavior and determining decision-making pathways, does their inclusion in a collective behavior model have an impact on the practical applications for collective behavior models, i.e., in the acoustic monitoring of fish shoals? Lopez et al. (2012) state that while IBMs have had some experimental success in linking individual behaviors to school-wide properties, they are unlikely to accurately reproduce experimental data because they fail to produce experimental speed distributions. The applicability of models based on experimental conditions may also depend on the size of the shoal. For example, Huth and Wissel (1994) successfully reproduced the basic structure of a fish school for 20 and 100 fish, concluding that the same model would be applicable to schools of 1000 or more. Is that a valid assumption?
Additionally, there are biological and environmental factors to consider when comparing models to data in and ex situ. On a fundamental level, not all fish have the same sensory capabilities, and there are effects of time of day, water dynamics, prey availability, predation risk, etc. in the open ocean. Random variation as a stand-in for some of these variables can be included in the model, as in Huth and Wissel (1994), but this approach may introduce further bias. With the inherent complexity, the “best” model answers the question asked; as we know, while all models may be wrong, some are useful (Wasserstein, 2010).
An important area for research would be to reduce the complexity of the variables listed in Tables 1, 2. It should be possible for several research programs to replicate the response of shoals to extrinsic and intrinsic factors. It is plausible that the mechanisms that affect shoal characteristics have aspects in common across environmental and biological drivers. Further research would be to reduce the apparent complexity to a limited number of empirically justified processes. For example, the responses to temperature, flow and salinity could all be special cases of a response based on (for example) leadership. An individual-based framework, linked to field data, has the potential to make these types of syntheses, moving toward an understanding of what extrinsic and intrinsic processes affect acoustic data collection. For example, Trygonis and Kapelonis (2018) use a static, spatially random representation of shoal density, but recognize that dynamic, realistic descriptions of the internal structure of shoals are needed to refine signal algorithms.
Implications of Behavior for Acoustics – Atlantic Mackerel
For mackerel, the in situ conditions that affect collective behavior include their ontogeny, foraging ecology, and reaction to predators. Mackerel are gregarious forage fish, at high risk for predation (Nonacs et al., 1998), and environmental factors govern the route of foraging and spawning migrations, as well as the dynamics of the shoal. Foraging mackerel are usually found close to the surface, with their geographical and vertical movements mediated by temperature (Hughes et al., 2015; Nøttestad et al., 2016a). Nøttestad et al. (2016a) found all mackerel schools in water above 6°C, with the majority found between 7 and 11°C, a temperature tolerance lower than the 8°C found in previous studies. Mackerel stay above the thermocline where warmer, stabilized water masses trap nutrients, phytoplankton, and zooplankton. The higher light levels may also help them detect prey and the warmer waters may facilitate efficient swimming (Nøttestad et al., 2016a). As mackerel are temperature-sensitive, their distributions have shifted with climate change, following increased thermally-acceptable habitat, but the relationship between mackerel and temperature is not straight-forward. The fisheries catch has also become more dispersed, moving further offshore to areas of deeper water (Hughes et al., 2015). Temperature and plankton concentrations affect swimming speed as well, with areas with high plankton concentrations and low temperatures found to be home to the largest fish, swimming the slowest (Nøttestad et al., 2016a). Mackerel migratory behavior has also been shown to be affected by currents; rheotaxis is likely due to the influence on the lateral line and changes in the inertia of the shoal (Godø et al., 2004).
Another consideration for field studies of mackerel behavior is their predator avoidance strategy, if the shoal senses the survey vessel or trawler as a threat. One fisher stated that after trawlers had passed through an area, the mackerel were “all flighty,” that “they lie on the bottom to feed instead of swimming in midwater,” making them harder to fish (Purvis, 2002). Within the literature, however, there is conflicting evidence about whether mackerel respond to vessels as predators, as with most fisheries species (Fréon et al., 1993; Fernandes et al., 2000; Slotte et al., 2007). Nøttestad et al. (2016b) found no change in mackerel acoustic readings before and after trawling, but Godø et al. (2004) had previously found that vessels may affect their behavior and suggest reducing vessel speed when surveying. Mackerel may also respond to the net while trawling, with some evidence that they dive to avoid it, mixing with herring near the seabed (Fernandes et al., 2000; Slotte et al., 2007). With their weak target strength, correct identification of a mackerel shoal is essential, and this mixing could have major effects on the total biomass estimate. Further investigations into their behavior are necessary.
Possible Future Research
Parameterizing IBMs for Acoustic Research
Much of the work on modeling collective behavior has been theoretical, but the insights from these models, when validated with empirical data, demonstrate that they are a useful tool for determining overall shoal characteristics such as density and orientation and how these characteristics change depending on conditions. No studies, however, have attempted to apply these models to acoustic surveys or to how the collective behavior of pelagic fish may change along their migration path. This is a rich avenue for research, as behavior is a major source of variation in acoustic stock assessments (Table 3). Properly parameterized and applied, IBMs could be used to reduce sources of bias such as uncertainty in tilt angle and density variations, especially for mackerel and other gregarious species. IBMs may also provide more information on whether behavior changes in response to the survey vessel, or between acoustic sampling and trawling, by providing statistical descriptors of shoal shape that can be related to individual behaviors such as nearest neighbor distance and polarization (Huth and Wissel, 1992; Gautrais et al., 2012).
IBMs provide a framework for testing environmental and behavioral factors that would be near impossible to replicate in situ. As the fundamental rules governing collective behavior are well-understood, there is scope to test both intrinsic and extrinsic effects on shoals to determine what changes to the environment or to the animals may affect shoal shape (Tables 1, 2) and acoustic surveys (Table 3) and whether these effects are independent or linked. A clear taxonomy of these factors may not be possible, but focusing on the connections between individual behavior and acoustic surveying will help build hypotheses that can be tested with theoretical models and limited data collection. There is a wealth of empirical data to be collected on whether the same conditions, such as bathymetry and oceanography, change behavior to a degree that is reflected in acoustic surveys (Tables 1, 3). Similarly, experimental work is needed to test if behavioral conditions such as leadership and the differences between foraging and evasive behaviors affect shoal shape in acoustic surveys, even at a small scale (Tables 2, 3). IBMs have been used successfully to model the spatial and temporal variation in mackerel populations (Boyd et al., 2018). This research could be expanded further by incorporating behavioral changes across migration, i.e., between foraging, spawning, and traveling between grounds, to predict the approximate vertical position and density of shoals along the migratory path.
Improvements in Technology for Testing Theoretical Models
The practical side of testing theoretical models is also increasingly feasible, as monitoring, tracking, and computing resources improve and become more accessible. Investigating the individual behavior of fish in the open ocean and efficient and accurate analysis of the data collected requires novel uses of and advancements in these technologies. Technology for monitoring and assessing pelagic fish in the field ranges from consumer-level cameras to 3-D sonar and does not need to be highly technical to be effective. Fernandes et al. (2016) used small video cameras to determine the tilt angle of mackerel during surveys. The small cameras did not disturb the mackerel and the fish were shallow enough to be clearly visible on video. More advanced technologies are also being used, such as multibeam sonar, used by Tenningen et al. (2015) to determine the backscatter and density of herring and mackerel during purse-seine capture to determine how they responded to the net.
Further improvements in data collection include three-dimensional video equipment and analysis techniques and automated tracking technology (Butail et al., 2013; Nath et al., 2019) for individuals in video. The vanguard of collective behavior research are using approaches seemingly out of science fiction, such as creating photorealistic virtual environments for zebrafish (Danio rerio) to test leadership and social interactions (Stowers et al., 2017). Additionally, cloud computing is increasingly accessible for individuals and institutions, allowing for more robust models and further parameterization and testing (Michener and Jones, 2012). With these technological improvements come myriad opportunities to turn theoretical models into practical tools for fisheries assessment.
Applying Behavioral Modeling to Other Important Questions
IBMs may also be useful in approaching more complex and theoretical questions about how behavior affects fisheries science, such as whether fish behavior has evolved with fishing pressure. There is evidence to suggest that humans have exerted considerable selective pressure on fisheries species through direct intervention, such as the escape of selectively-bred fishes from aquaculture (Hutchings and Kuparinen, 2020). While the evidence for indirect intervention through fishing is less clear, the theoretical basis for genetic change under “predator” and prey interactions is clearer (Hutchings and Kuparinen, 2020). For example, shoals facilitate information transfer and Macdonald et al. (2018) found that the age of individual herring in a shoal can affect the migration path and organization of the shoal. Further, Hollins et al. (2019) found that the vulnerability of minnows to trawling depended on anaerobic capacity when the fish were swimming with familiar conspecifics, but there was no effect in an unfamiliar shoal. These studies suggest that collective behavior and experience has an additional effect on both spatial distribution and fisheries capture. While collective memory and information transfer have been questions explored in theoretical modeling studies (Couzin et al., 2002; Giardina, 2008; Lopez et al., 2012; Ioannou, 2017), the applications to fisheries and stock assessments have not been clearly laid out.
Another important avenue for behavioral research is the effect of climate change on shoaling. Environmental conditions such as temperature, water flow and currents, salinity, and oxygen levels can affect shoaling behavior (Table 1; Nonacs et al., 1998; Tien et al., 2004; Rieucau et al., 2014, 2016) and the influence of these factors is likely to vary under climate change. While shifts in the spatial distribution of important fisheries species, such as mackerel, has been investigated (Hughes et al., 2015; Boyd et al., 2018; Ólafsdóttir et al., 2019), there has not been an overall synthesis of how climate change may affect behavior and, in turn, how those behavioral changes will affect larger-scale spatial distributions.
Conclusion
The body of theoretical modeling works spans over four decades and many disciplines, including mathematics, physics, medicine, ecology, and cognitive science. From models of pathogen transmission across aquaculture sites (Alaliyat et al., 2019), to testing theories of democratic consensus with fish shoals (Couzin et al., 2011), collective behavior modeling has been hugely useful. Simple theoretical concepts have been effectively applied to complex, real-world problems. The same approach is possible for fish behavior in stock assessments. Making predictions on how extrinsic conditions affect shoal shape and density will require further synthesis of existing models and deliberate inclusion of variables that affect acoustic monitoring, as many of the factors affecting behavior are likely interdependent. This research can improve our knowledge of and therefore capacity to manage the commercially-valuable pelagic species in the Northeast Atlantic, especially for mackerel. With climate change and increasing global demands for fish, improvements to fisheries assessment are imperative if we want to ensure a stable and productive future for our oceans.
Author Contributions
SW drafted the manuscript. SW and MJ revised the manuscript.
Funding
SW was funded by an Irish Research Council Government of Ireland Postgraduate Scholarship.
Conflict of Interest
The authors declare that the research was conducted in the absence of any commercial or financial relationships that could be construed as a potential conflict of interest.
Acknowledgments
We gratefully acknowledge the financial support from the Irish Research Council. We thank the reviewers for their helpful comments that have greatly improved the manuscript.
Footnotes
- ^ Individual-based models are also called agent-based models and are related to particle models.
References
Alaliyat, S., Yndestad, H., and Davidsen, P. I. (2019). An agent-based approach for predicting patterns of pathogen transmission between aquaculture sites in the Norwegian fjords. Aquaculture 505, 98–111. doi: 10.1016/j.aquaculture.2019.02.044
Alder, J., Campbell, B., Karpouzi, V., Kaschner, K., and Pauly, D. (2008). Forage fish: from ecosystems to markets. Annu. Rev. Environ. Resour. 33, 153–166.
Barbaro, A. B. T., Taylor, K., Trethewey, P. F., Youseff, L., and Birnir, B. (2009a). Discrete and continuous models of the dynamics of pelagic fish: application to the capelin. Math. Comput. Simul. 79, 3397–3414. doi: 10.1016/j.matcom.2008.11.018
Barbaro, A. B. T., Einarsson, B., Birnir, B., Sigurðsson, S., Valdimarsson, H., Pálsson, Ó. K., et al. (2009b). Modelling and simulations of the migration of pelagic fish. ICES J. Mar. Sci. 66, 826–838. doi: 10.1093/icesjms/fsp067
Biro, D., Sasaki, T., and Portugal, S. J. (2016). Bringing a time–depth perspective to collective animal behaviour. Trends Ecol. Evol. 31, 550–562. doi: 10.1016/j.tree.2016.03.018
Biseau, A. (1998). Definition of a directed fishing effort in a mixed-species trawl fishery, and its impact on stock assessments. Aquat. Living Resour. 11, 119–136. doi: 10.1016/S0990-7440(98)80109-5
Blaxter, J. H. S., and Batty, R. S. (1990). Swimbladder “behaviour” and target strength. Rapp. P.-V. Réun. Cons. Int. Explor. Mer 189, 233–244.
Boyd, R., Roy, S., Sibly, R., Thorpe, R., and Hyder, K. (2018). A general approach to incorporating spatial and temporal variation in individual-based models of fish populations with application to Atlantic mackerel. Ecol. Model. 382, 9–17. doi: 10.1016/j.ecolmodel.2018.04.015
Brehmer, P., Sarré, A., and Guenne, Y. (2019). Vessel avoidance response: a complex tradeoff between fish multisensory integration and environmental variables. Rev. Fish. Sci. Aquac. 27, 380–391. doi: 10.1080/23308249.2019.1601157
Bruintjes, R., Purser, J., Everley, K. A., Mangan, S., Simpson, S. D., and Radford, A. N. (2016). Rapid recovery following short-term acoustic disturbance in two fish species. R. Soc. Open Sci. 3:150686. doi: 10.1098/rsos.150686
Burgess, M. G., Fredston-Hermann, A., Pinsky, M. L., Gaines, S. D., and Tilman, D. (2017). Reply to Le Pape et al.: management is key to preventing marine extinctions. Proc. Natl. Acad. Sci. U.S.A. 114, E6275–E6276.
Butail, S., Bollt, E. M., and Porfiri, M. (2013). Analysis and classification of collective behavior using generative modeling and nonlinear manifold learning. J. Theor. Biol. 336, 185–199. doi: 10.1016/j.jtbi.2013.07.029
Couzin, I. D., Ioannou, C. C., Demirel, G., Gross, T., Torney, C. J., Hartnett, A., et al. (2011). Uninformed individuals promote democratic consensus in animal groups. Science 334, 1578–1580. doi: 10.1126/science.1210280
Couzin, I. D., Krause, J., James, R., Ruxton, G. D., and Franks, N. R. (2002). Collective memory and spatial sorting in animal groups. J. Theor. Biol. 218, 1–11. doi: 10.1006/yjtbi.3065
De Robertis, A., and Handegard, N. O. (2013). Fish avoidance of research vessels and the efficacy of noise-reduced vessels: a review. ICES J. Mar. Sci. 70, 34–45. doi: 10.1093/icesjms/fss155
Delcourt, J., and Poncin, P. (2012). Shoals and schools: back to the heuristic definitions and quantitative references. Rev. Fish Biol. Fish. 22, 595–619. doi: 10.1007/s11160-012-9260-z
Demer, D. A., Andersen, L. N., Bassett, C., Berger, L., Chu, D., Condiotty, J., et al. (2017). 2016 USA-Norway EK80 Workshop Report: Evaluation of a Wideband Echosounder for Fisheries and Marine Ecosystem Science. ICES Cooperative Research Report No. 336. Available online at: http://doi.org/10.17895/ices.pub.2318 (accessed October 15, 2019).
Dulvy, N. K., Rogers, S. I., Jennings, S., Stelzenmüller, V., Dye, S. R., and Skjoldal, H. R. (2008). Climate change and deepening of the North Sea fish assemblage: a biotic indicator of warming seas. J. Appl. Ecol. 45, 1029–1039. doi: 10.1111/j.1365-2664.2008.01488.x
Eriksson, A., Nilsson Jacobi, M., Nyström, J., and Tunstrøm, K. (2010). Determining interaction rules in animal swarms. Behav. Ecol. 21, 1106–1111. doi: 10.1093/beheco/arq118
Essington, T. E., Moriarty, P. E., Froehlich, H. E., Hodgson, E. E., Koehn, L. E., Oken, K. L., et al. (2015). Fishing amplifies forage fish population collapses. Proc. Natl. Acad. Sci. U.S.A. 112, 6648–6652. doi: 10.1073/pnas.1422020112
Fernandes, P. G., Brierley, A. S., Simmonds, E. J., Millard, N. W., McPhail, S. D., Armstrong, F., et al. (2000). Fish do not avoid survey vessels. Nature 404, 35–36. doi: 10.1038/35003648
Fernandes, P. G., Copland, P., Garcia, R., Nicosevici, T., and Scoulding, B. (2016). Additional evidence for fisheries acoustics: small cameras and angling gear provide tilt angle distributions and other relevant data for mackerel surveys. ICES J. Mar. Sci. 73, 2009–2019. doi: 10.1093/icesjms/fsw091
Fréon, P., Gerlotto, F., and Misund, O. A. (1993). Consequences of fish behaviour for stock assessment. ICES Mar. Sci. Symp. 196, 190–195.
Fréon, P., Gerlotto, F., and Soria, M. (1992). Changes in school structure according to external stimuli: description and influence on acoustic assessment. Fish. Res. 15, 45–66. doi: 10.1016/0165-7836(92)90004-D
Fu, S. (2016). Effects of group size on schooling behavior in two cyprinid fish species. Aquat. Biol. 25, 165–172. doi: 10.3354/ab00667
Gautrais, J., Ginelli, F., Fournier, R., Blanco, S., Soria, M., Chaté, H., et al. (2012). Deciphering interactions in moving animal groups. PLoS Comput. Biol. 8:e1002678. doi: 10.1371/journal.pcbi.1002678
Georgakarakos, S., Trygonis, V., and Haralabous, J. (2011). “Accuracy of acoustic methods in fish stock assessment surveys,” in Sonar Systems, ed. N. Kolev (Rijeka: InTech). doi: 10.5772/18631
Giardina, I. (2008). Collective behavior in animal groups: theoretical models and empirical studies. HFSP J. 2, 205–219. doi: 10.2976/1.2961038
Godø, O. R., Hjellvik, V., Iversen, S. A., Slotte, A., Tenningen, E., and Torkelsen, T. (2004). Behaviour of mackerel schools during summer feeding migration in the Norwegian Sea, as observed from fishing vessel sonars. ICES J. Mar. Sci. 61, 1093–1099. doi: 10.1016/j.icesjms.2004.06.009
Gorska, N., Ona, E., and Korneliussen, R. (2005). Acoustic backscattering by Atlantic mackerel as being representative of fish that lack a swimbladder. Backscattering by individual fish. ICES J. Mar. Sci. 62, 984–995. doi: 10.1016/j.icesjms.2005.03.010
Handegard, N. O., Tenningen, M., Howarth, K., Anders, N., Rieucau, G., and Breen, M. (2017). Effects on schooling function in mackerel of sub-lethal capture related stressors: crowding and hypoxia. PLoS One 12:e0190259. doi: 10.1371/journal.pone.0190259
Hazen, E. L., and Horne, J. K. (2003). A method for evaluating the effects of biological factors on fish target strength. ICES J. Mar. Sci. 60, 555–562. doi: 10.1016/S1054-3139(03)00053-5
Hemelrijk, C. K., and Hildenbrandt, H. (2008). Self-organized shape and frontal density of fish schools. Ethology 114, 245–254. doi: 10.1111/j.1439-0310.2007.01459.x
Hensor, E., Couzin, I. D., James, R., Krause, J., and Persson, L. (2005). Modelling density-dependent fish shoal distributions in the laboratory and field. Oikos 110, 344–352.
Herbert-Read, J. E. (2016). Understanding how animal groups achieve coordinated movement. J. Exp. Biol. 219, 2971–2983. doi: 10.1242/jeb.129411
Herbert-Read, J. E., Kremer, L., Bruintjes, R., Radford, A. N., and Ioannou, C. C. (2017). Anthropogenic noise pollution from pile-driving disrupts the structure and dynamics of fish shoals. Proc. R. Soc. B 284:20171627. doi: 10.1098/rspb.2017.1627
Herbert-Read, J. E., Perna, A., Mann, R. P., Schaerf, T. M., Sumpter, D. J. T., and Ward, A. J. W. (2011). Inferring the rules of interaction of shoaling fish. Proc. Natl. Acad. Sci. U.S.A. 108, 18726–18731. doi: 10.1073/pnas.1109355108
Hollins, J. P. W., Thambithurai, D., Van Leeuwen, T. E., Allan, B., Koeck, B., Bailey, D., et al. (2019). Shoal familiarity modulates effects of individual metabolism on vulnerability to capture by trawling. Conserv. Physiol. 7:coz043. doi: 10.1093/conphys/coz043
Hughes, K. M., Dransfeld, L., and Johnson, M. P. (2015). Climate and stock influences on the spread and locations of catches in the northeast Atlantic mackerel fishery. Fish. Oceanogr. 24, 540–552. doi: 10.1111/fog.12128
Huston, M., DeAngelis, D., and Post, W. (1988). New Computer models unify ecological theory. Bioscience 38, 682–691.
Hutchings, J. A., and Kuparinen, A. (2020). Implications of fisheries-induced evolution for population recovery: refocusing the science and refining its communication. Fish Fish. 21, 453–464. doi: 10.1111/faf.12424
Huth, A., and Wissel, C. (1992). The simulation of the movement of fish schools. J. Theor. Biol. 156, 365–385. doi: 10.1016/S0022-5193(05)80681-2
Huth, A., and Wissel, C. (1994). The simulation of fish schools in comparison with experimental data. Ecol. Model. 75–76, 135–146. doi: 10.1016/0304-3800(94)90013-2
ICES (2005). Report of the Planning Group on Aerial and Acoustic Surveys for Mackerel (PGAAM). Bergen: ICES.
Ioannou, C. C. (2017). Swarm intelligence in fish? The difficulty in demonstrating distributed and self-organised collective intelligence in (some) animal groups. Behav. Process. 141, 141–151. doi: 10.1016/j.beproc.2016.10.005
Jensen, F. (2015). Game theory and fish wars: the case of the Northeast Atlantic mackerel fishery. Fish. Res. 172, 7–16.
Katz, Y., Tunstrøm, K., Ioannou, C. C., Huepe, C., and Couzin, I. D. (2011). Inferring the structure and dynamics of interactions in schooling fish. Proc. Natl. Acad. Sci. U.S.A. 108, 18720–18725. doi: 10.1073/pnas.1107583108
Knudsen, F. R., Hawkins, A., McAllen, R., and Sand, O. (2009). Diel interactions between sprat and mackerel in a marine lough and their effects upon acoustic measurements of fish abundance. Fish. Res. 100, 140–147. doi: 10.1016/j.fishres.2009.06.015
Korneliussen, R. J. (2010). The acoustic identification of Atlantic mackerel. ICES J. Mar. Sci. 67, 1749–1758. doi: 10.1093/icesjms/fsq052
Korneliussen, R. J. (ed.) (2018). Acoustic target classification. ICES Cooperative Research Report. Available online at: http://doi.org/10.17895/ices.pub.4567 (accessed October 16, 2019).
Korneliussen, R. J., Heggelund, Y., Macaulay, G. J., Patel, D., Johnsen, E., and Eliassen, I. K. (2016). Acoustic identification of marine species using a feature library. Methods Oceanogr. 17, 187–205. doi: 10.1016/j.mio.2016.09.002
Korneliussen, R. J., and Ona, E. (2004). Verified acoustic identification of Atlantic mackerel. Paper Presented at the ICES ASC 2004 Theme Session R: New Developments in Fisheries Acoustics, Gdynia, 14.
Krause, J., Butlin, R. K., Peuhkuri, N., and Pritchard, V. L. (2000a). The social organization of fish shoals: a test of the predictive power of laboratory experiments for the field. Biol. Rev. Camb. Philos. Soc. 75, 477–501. doi: 10.1111/j.1469-185X.2000.tb00052.x
Krause, J., Hoare, D., Krause, S., Hermelrijk, C. K., and Rubenstein, D. I. (2000b). Leadership in fish shoals. Fish Fish. 1, 82–89. doi: 10.1111/j.1467-2979.2000.tb00001.x
Lee, D., Oh, W., Gim, B.-M., Lee, J. S., Yoon, E., and Lee, K. (2019). Investigating the effects of different LED wavelengths on aggregation and swimming behavior of chub mackerel (Scomber japonicus). Ocean Sci. J. 54, 573–579. doi: 10.1007/s12601-019-0034-6
Lopez, U., Gautrais, J., Couzin, I. D., and Theraulaz, G. (2012). From behavioural analyses to models of collective motion in fish schools. Interface Focus 2, 693–707. doi: 10.1098/rsfs.2012.0033
Lukeman, R., Li, Y.-X., Edelstein-Keshet, L., and Levin, S. A. (2010). Inferring individual rules from collective behavior. Proc. Natl. Acad. Sci. U.S.A. 107, 12576–12580. doi: 10.1073/pnas.1001763107
Macal, C. M., and North, M. J. (2010). Tutorial on agent-based modelling and simulation. J. Simul. 4, 151–162. doi: 10.1057/jos.2010.3
Macdonald, J. I., Logemann, K., Krainski, E. T., Sigurðsson, Þ., Beale, C. M., Huse, G., et al. (2018). Can collective memories shape fish distributions? A test, linking space-time occurrence models and population demographics. Ecography 41, 938–957. doi: 10.1111/ecog.03098
Mann, R. P. (2011). Bayesian inference for identifying interaction rules in moving animal groups. PLoS One 6:e22827. doi: 10.1371/journal.pone.0022827
Mann, R. P., Perna, A., Strömbom, D., Garnett, R., Herbert-Read, J. E., Sumpter, D. J. T., et al. (2013). Multi-scale Inference of interaction rules in animal groups using bayesian model selection. PLoS Comput. Biol. 9:e1002961. doi: 10.1371/journal.pcbi.1002961
Massé, J. (1996). Acoustic observations in the Bay of Biscay: schooling, vertical distribution, species assemblages and behaviour. Sci. Mar. 60, 227–234.
Mesnil, B., Cotter, J., Fryer, R. J., Needle, C. L., and Trenkel, V. M. (2009). A review of fishery-independent assessment models, and initial evaluation based on simulated data. Aquat. Living Resour. 22, 207–216. doi: 10.1051/alr/2009003
Michener, W. K., and Jones, M. B. (2012). Ecoinformatics: supporting ecology as a data-intensive science. Trends Ecol. Evol. 27, 85–93. doi: 10.1016/j.tree.2011.11.016
Misund, O., and Beltestad, A. (1996). Target-strength estimates of schooling herring and mackerel using the comparison method. ICES J. Mar. 53, 281–284. doi: 10.1006/jmsc.1996.0035
Misund, O. A. (1993). Dynamics of moving masses: variability in packing density, shape, and size among herring, sprat, and saithe schools. ICES J. Mar. Sci. 50, 145–160.
Mitson, R. B., and Knudsen, H. P. (2003). Causes and effects of underwater noise on fish abundance estimation. Aquat. Living Resour. 16, 255–263. doi: 10.1016/S0990-7440(03)00021-4
Nakken, O., and Olsen, K. (1977). Target strength measurements of fish. Rapp. P.-v. Réun. Cons. Int. Explor. Mer 170, 52–69.
Nath, T., Mathis, A., Chen, A. C., Patel, A., Bethge, M., and Mathis, M. W. (2019). Using DeepLabCut for 3D markerless pose estimation across species and behaviors. Nat. Protoc. 14, 2152–2176. doi: 10.1038/s41596-019-0176-0
Nonacs, P., Smith, P. E., and Mangel, M. (1998). Modeling foraging in the northern anchovy (Engraulis mordax): individual behavior can predict school dynamics and population biology. Can. J. Fish. Aquat. Sci. 55, 1179–1188. doi: 10.1139/cjfas-55-5-1179
Nøttestad, L., Diaz, J., Pena, H., Søiland, H., Huse, G., and Fernö, A. (2016a). Feeding strategy of mackerel in the Norwegian Sea relative to currents, temperature, and prey. ICES J. Mar. Sci. 73, 1127–1137. doi: 10.1093/icesjms/fst034
Nøttestad, L., Utne, K. R., Óskarsson, G. J., Jónsson, S. Þ., Jacobsen, J. A., Tangen, Ø., et al. (2016b). Quantifying changes in abundance, biomass, and spatial distribution of Northeast Atlantic mackerel (Scomber scombrus) in the Nordic seas from 2007 to 2014. ICES J. Mar. Sci. 73, 359–373. doi: 10.1093/icesjms/fsv218
Ólafsdóttir, A. H., Utne, K. R., Jacobsen, J. A., Jansen, T., Óskarsson, G. J., Nøttestad, L., et al. (2019). Geographical expansion of Northeast Atlantic mackerel (Scomber scombrus) in the Nordic Seas from 2007 to 2016 was primarily driven by stock size and constrained by low temperatures. Deep Sea Res. Part II Top. Stud. Oceanogr. 159, 152–168. doi: 10.1016/j.dsr2.2018.05.023
Pecl, G. T., Ward, T. M., Doubleday, Z. A., Clarke, S., Day, J., Dixon, C., et al. (2014). Rapid assessment of fisheries species sensitivity to climate change. Clim. Change 127, 505–520. doi: 10.1007/s10584-014-1284-z
Pitcher, T., Magurran, A., and Edwards, J. (1985). Schooling mackerel and herring choose neighbours of similar size. Mar. Biol. 86, 319–322.
Purvis, A. (2002). One Boat, One Line, No Bait. This Man has the Future of Fishing in His Hands. The Guardian. Available online at: https://www.theguardian.com/lifeandstyle/2002/nov/10/foodanddrink.features6 (accessed January 14, 2020).
Quera, V., Beltran, F. S., and Gimeno, E. (2016). Modelling the emergence of coordinated collective motion by minimizing dissatisfaction. Math. Biosci. 271, 154–167. doi: 10.1016/j.mbs.2015.11.007
Reebs, S. (2000). Can a minority of informed leaders determine the foraging movements of a fish shoal? Anim. Behav. 59, 403–409. doi: 10.1006/anbe.1999.1314
Reid, D. G., and Simmonds, E. J. (1993). Image analysis techniques for the study of fish school structure from acoustic survey data. Can. J. Fish. Aquat. Sci. 50, 886–893. doi: 10.1139/f93-102
Reuter, H., Kruse, M., Rovellini, A., and Breckling, B. (2016). Evolutionary trends in fish schools in heterogeneous environments. Ecol. Model. 326, 23–35. doi: 10.1016/j.ecolmodel.2015.09.008
Reynolds, C. W. (1987). Flocks, herds and schools: a distributed behavioral model. Comput. Graph. 21, 25–34. doi: 10.1145/37402.37406
Rieucau, G., Fernö, A., Ioannou, C. C., and Handegard, N. O. (2014). Towards of a firmer explanation of large shoal formation, maintenance and collective reactions in marine fish. Rev. Fish Biol. Fish. 25, 21–37. doi: 10.1007/s11160-014-9367-5
Rieucau, G., Holmin, A. J., Castillo, J. C., Couzin, I. D., and Handegard, N. O. (2016). School level structural and dynamic adjustments to risk promote information transfer and collective evasion in herring. Anim. Behav. 117, 69–78. doi: 10.1016/j.anbehav.2016.05.002
Rountree, R. A., and Sedberry, G. R. (2009). A theoretical model of shoaling behavior based on a consideration of patterns of overlap among the visual fields of individual members. Acta Ethol. 12, 61–70. doi: 10.1007/s10211-009-0057-6
Scalabrin, C. (1996). Narrowband acoustic identification of monospecific fish shoals. ICES J. Mar. Sci. 53, 181–188. doi: 10.1006/jmsc.1996.0020
Scheffer, M., Carpenter, S., and de Young, B. (2005). Cascading effects of over?shing marine systems. Trends Ecol. Evol. 20, 579–581.
Scoulding, B., Gastauer, S., MacLennan, D. N., Fässler, S. M. M., Copland, P., and Fernandes, P. G. (2017). Effects of variable mean target strength on estimates of abundance: the case of Atlantic mackerel (Scomber scombrus). ICES J. Mar. Sci. 74, 822–831. doi: 10.1093/icesjms/fsw212
Simmonds, J., and MacLennan, D. (2005). Fisheries Acoustics: Theory and Practice, 2nd Edn. Oxford: Blackwell Science Ltd.
Slotte, A., Skagen, D., and Iversen, S. A. (2007). Size of mackerel in research vessel trawls and commercial purse-seine catches: implications for acoustic estimation of biomass. ICES J. Mar. Sci. 64, 989–994. doi: 10.1093/icesjms/fsm070
Spijkers, J., and Boonstra, W. J. (2017). Environmental change and social conflict: the northeast Atlantic mackerel dispute. Reg. Environ. Change 17, 1835–1851. doi: 10.1007/s10113-017-1150-4
Srinivasan, U. T., Cheung, W. W. L., Watson, R., and Sumaila, U. R. (2010). Food security implications of global marine catch losses due to overfishing. J. Bioecon. 12, 183–200. doi: 10.1007/s10818-010-9090-9
Stowers, J. R., Hofbauer, M., Bastien, R., Griessner, J., Higgins, P., Farooqui, S., et al. (2017). Virtual reality for freely moving animals. Nat. Methods 14, 995–1002. doi: 10.1038/nmeth.4399
Tenningen, M., Peña, H., and Macaulay, G. J. (2015). Estimates of net volume available for fish shoals during commercial mackerel (Scomber scombrus) purse seining. Fish. Res. 161, 244–251. doi: 10.1016/j.fishres.2014.08.003
Tenningen, M., Slotte, A., and Skagen, D. (2011). Abundance estimation of Northeast Atlantic mackerel based on tag recapture data—A useful tool for stock assessment? Fish. Res. 107, 68–74. doi: 10.1016/j.fishres.2010.10.009
Tien, J. H., Levin, S. A., and Rubenstein, D. I. (2004). Dynamics of fish schools: identifying key decision rules. Evol. Ecol. Res. 6, 555–565.
Trygonis, V., and Kapelonis, Z. (2018). Corrections of fish school area and mean volume backscattering strength by simulation of an omnidirectional multi-beam sonar. ICES J. Mar. Sci. 75, 1496–1508. doi: 10.1093/icesjms/fsy009
van der Kooij, J., Fässler, S. M. M., Stephens, D., Readdy, L., Scott, B. E., and Roel, B. A. (2016). Opportunistically recorded acoustic data support Northeast Atlantic mackerel expansion theory. ICES J. Mar. Sci. 73, 1115–1126. doi: 10.1093/icesjms/fsv243
van der Vaart, E., Beaumont, M. A., Johnston, A. S. A., and Sibly, R. M. (2015). Calibration and evaluation of individual-based models using Approximate Bayesian Computation. Ecol. Model. 312, 182–190. doi: 10.1016/j.ecolmodel.2015.05.020
Ward, A. J. W., Herbert-Read, J. E., Sumpter, D. J. T., and Krause, J. (2011). Fast and accurate decisions through collective vigilance in fish shoals. Proc. Natl. Acad. Sci. U.S.A. 108, 2312–2315. doi: 10.1073/pnas.1007102108
Keywords: Atlantic mackerel, pelagic fisheries, fish shoaling, fisheries acoustics, individual-based models (IBM), collective behavior
Citation: Wassermann SN and Johnson MP (2020) The Potential to Improve the Sustainability of Pelagic Fisheries in the Northeast Atlantic by Incorporating Individual Fish Behavior Into Acoustic Sampling. Front. Mar. Sci. 7:357. doi: 10.3389/fmars.2020.00357
Received: 20 January 2020; Accepted: 27 April 2020;
Published: 21 May 2020.
Edited by:
Maria Grazia Pennino, Oceanographic Center of Vigo, Spanish Institute of Oceanography, SpainReviewed by:
Angelo Bonanno, National Research Council (CNR), ItalyLuis A. Cubillos, University of Concepcion, Chile
Copyright © 2020 Wassermann and Johnson. This is an open-access article distributed under the terms of the Creative Commons Attribution License (CC BY). The use, distribution or reproduction in other forums is permitted, provided the original author(s) and the copyright owner(s) are credited and that the original publication in this journal is cited, in accordance with accepted academic practice. No use, distribution or reproduction is permitted which does not comply with these terms.
*Correspondence: Sophia N. Wassermann, s.wassermann1@nuigalway.ie