- 1Fisheries College, Ocean University of China, Qingdao, China
- 2Sea Around Us, Institute for the Oceans and Fisheries, University of British Columbia, Vancouver, BC, Canada
To assess the changes that fisheries have imposed on the functioning of coastal marine ecosystems in China, 19 published Ecopath models were used to construct particle size distribution (PSD) spectra. The results show that high biomass of jellyfish from ranching operation impacted almost all of the ecosystems studied here. As well, an increasing impact of fisheries was demonstrated, via steeper PSD slopes, for ecosystems with models covering two or more periods. Models of nearshore areas, i.e., bays and estuaries, exhibited steeper PSD slopes than models of offshore areas. The PSD slopes were also correlated with total catch (TC), mean trophic level of catch, and the Shannon–Wiener diversity index (H′), which can be computed from Ecopath models. A multiple regression predicting the PSD slopes from year and mean trophic level of the catch explained 38.5% of the variance in the slopes. Overall, this study confirmed the status of depletion in China's marine fisheries resources. In addition, it established that including a PSD while constructing an Ecopath model, which is straightforward, will improve it, and allow more insights to be obtained from it regarding the impact of fishing on marine ecosystems.
Introduction
Marine ecosystems provide habitats to marine organisms and supply them with their nutrients (Odum and Barrett, 1971); however, the diversity of species and the multitude of interactions between them are difficult to identify and represent in models (Limburg et al., 2002; Fulton et al., 2003). Nevertheless, the Ecopath with Ecosim (EwE) modeling approach has become widely used, notably because of the relative ease with which its parameters can be estimated (Christensen and Pauly, 1992; Pauly et al., 2000; Coll et al., 2015; Colléter et al., 2015) and its ability to realistically represent the structure of food web within a given period (with Ecopath), then to simulate over time the changes of trophic interactions between species or group thereof (with Ecosim).
China has a long coastline, often divided into three large marine ecosystems (LMEs), i.e., the Yellow Sea (including a marginal sea, the Bohai Sea), the East China Sea, and the South China Sea (Wang and Aubrey, 1987; Sherman and Hempel, 2008). The structure and functioning of these ecosystems have been impacted by climate change (Jiao et al., 2015; Liang et al., 2018), ocean acidification (Liu and He, 2012), and especially overfishing (Wang et al., 2008; Liang and Pauly, 2017a,b; Liang et al., 2018; Pauly and Liang, 2019; Zhai and Pauly, 2019).
The depletion of fisheries resources in China's coastal seas was partly mitigated by the development of mariculture. Thus, in 2018, the total coastal areas devoted to marine aquaculture was above 20,000 km2, and production was more than 2 million tonnes (Anonymous, 2019). Indeed, the high density of mariculture enterprises and the escape of farmed animals exacerbate coastal pollution (Cao et al., 2007).
Another issue is worldwide proliferation of jellyfish (Brotz et al., 2012). While jellyfish are almost everywhere perceived as nuisance species (e.g., Lynam et al., 2005), several species are sought after in China, and some jellyfish are even farmed, or rather “ranched” (Dong et al., 2008). The increase in jellyfish in China's coastal seas in recent years are well-documented (Jiang et al., 2008; Dong et al., 2010) as is the negative impacts of jellyfish blooms on fishery and ecosystem functioning (Xian et al., 2005). For these reasons, Pauly et al. (2008) pointed out the important role of jellyfish in many ecosystems and the need to include them in food web models, which may have inspired the authors of subsequent Ecopath food web models to explicitly include jellyfish in their food webs (Lamb et al., 2019).
While the South China Sea, whose living resources are shared between numerous countries (Pauly and Liang, 2019), was modeled in the early 1990s (Pauly and Christensen, 1993), the first Ecopath model of a uniquely Chinese marine ecosystem was that of Tong et al. (2000), which sought to represent the structure of the food web in the Bohai Sea in 1982–1983. Since this pioneering effort, all of China's LMEs have been modeled by Chinese authors, i.e., the Yellow Sea (Lin et al., 2013), the East China Sea (Jiang et al., 2008), and the South China Sea (Cheung, 2007). However, many due to a perceived “lack of data,” none of these authors have used Ecosim, the dynamic routine of EwE.
Here, we use 19 extant Ecopath models of Chinese coastal ecosystems for inferences on the impact of fisheries on such ecosystems using particle size distribution spectra (Sheldon et al., 1972; Guiet et al., 2016), based on these 19 models, but after they were modified such as to generate clear linear spectra. The resulting particle size distributions (PSD) spectra were then used to describe and quantify the effect of fisheries and environmental effects.
Materials and Methods
Sources of Materials
The Ecopath models used in this study were sourced from the EcoBase (http://ecobase.ecopath.org/) and the scientific literature (Table 1). The geographical distribution of modeled ecosystems ranged from northern to southern China (Figure 1) and involves major main types of marine ecosystems, i.e., LMEs, a marginal sea, shelves, gulfs and bays, and estuaries. In addition, the models cover the 1960s to the 2010s, which encompass a period of huge changes in China's economy, including fisheries.
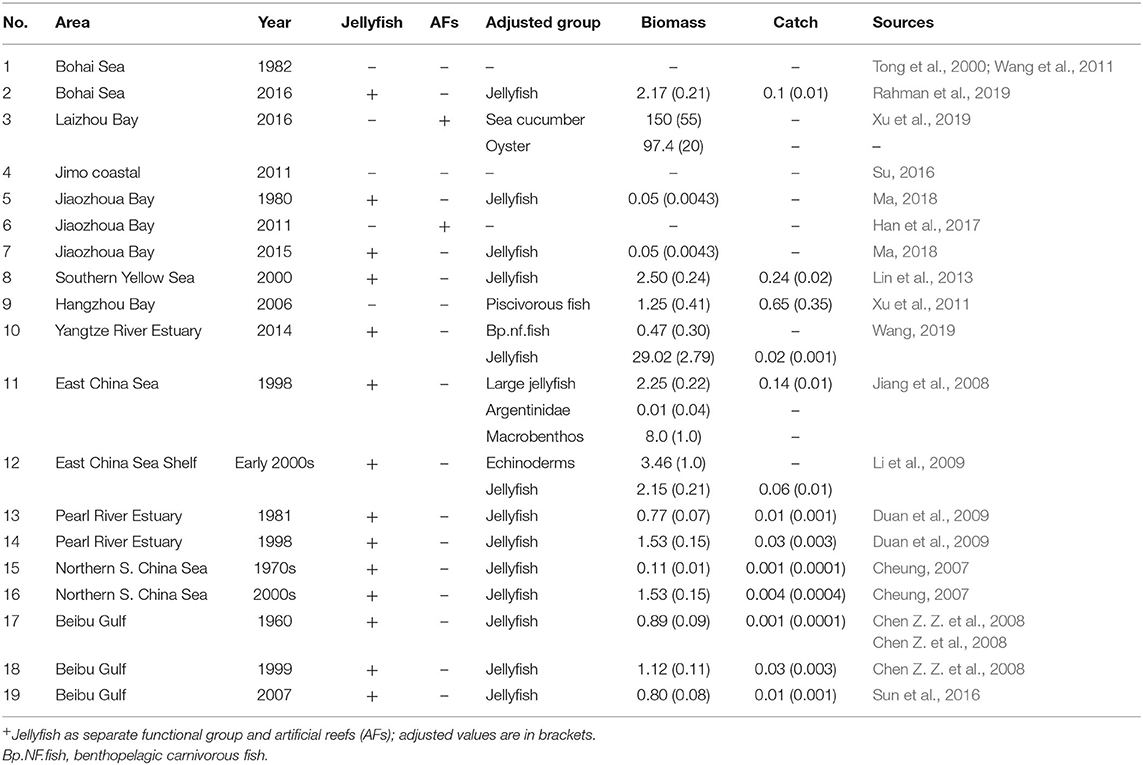
Table 1. Summary and adjustment of 19 adjusted Ecopath models in China's seas (biomass in tonnes km−2; catch in tonnes km−2 year−1).
Basic Principles of Ecopath Modeling
Ecopath was initially conceived by Polovina (1984) as a food web model with a set of functional groups linked by biomass flows according to the principle of mass balance, and further developed by Christensen and Pauly (1992) and Pauly et al. (1993). The model is structured by a master equation, which applies to all consumer groups in the models:
where Bi and Bj are the biomass (here in tonnes wet weight by km−2) of prey i and predator j, respectively; P/Bi is the production per biomass (tonnes km−2 year−1) of prey I; Q/Bi is the consumption per biomass (tonnes km2 year−1) of prey i; DCij is the proportion of prey i in the diet of predator j; EEi is the ecotrophic efficiency, i.e., the fraction of i's production that is consumed within the system; Yi is the fishery catch (tonnes km−2 year−1); Ei is the net migration rate (i.e., emigration–immigration; in tonnes km−2 year−1); and BAi is the biomass accumulation rate (year−1).
Another key equation of Ecopath is
where Qi is the consumption (tonnes km−2 year−1), Pi is the production (tonnes km−2 year−1), Ri is the respiration (tonnes km−2 year−1), and UAi is the unassimilated food of group i (tonnes km−2 year−1). The model assumes that the mass and energy input and output must be balanced within the time period covered by the model.
Particle Size Distribution
Particle size distribution (PSD) or size spectra within aquatic ecosystems are a powerful tool to show how much human activities and environmental-driven factors impact on aquatic ecosystem (Blanchard et al., 2017) and are usually based on sampling plankton (Hunt et al., 2015; Wallis et al., 2016) or fish (Boudreau and Dickie, 1992; Bianchi et al., 2000) using net gears that are adequate for these groups only. Irrespective of the way they are presented—which is usually as log(biomass) vs. log(body weight)—the resulting spectra frequently cover only plankton and/or or fish while regularly omitting marine mammals, i.e., they mostly cover a relatively narrow range of sizes, and thus their slopes are probably biased downward. Pauly and Christensen (2002) demonstrated that Ecopath models, which necessarily include small and large organisms with biomasses that are realistic, and mutually compatible, can be used to construct PSD, whose slopes can be compared between systems and/or with the slope of PSD estimated through field sampling.
In order to be able to use the groups' biomasses of an Ecopath model in a size spectrum, two issues must be considered:
(1) Many groups (e.g., large teleosts, which start as planktonic larvae) have ontogenies that span several (log) sizes classes or “bins,” and thus, their biomass must be allocated to several bins in accordance to their relative abundances.
(2) Different size/age groups (e.g., in large species) have vastly different growth rates, as do different species occurring in the same ecosystems, and thus, they remain in bins of given sizes for periods that vary among and between the species that define a group.
Version 6.6 of EwE includes a routine based on Pauly and Christensen (2002), which account for these two items using, for each of the groups, the parameter of a von Bertalanffy growth function (VBGF) to express the growth of the animal therein across a range of size bins. For length, the VBGF is
where Lt is the mean length at age t of the species in question; Linf is the asymptotic size, i.e., the mean length attained after an infinitely long time; K is growth coefficient (here in year−1); and t0 (usually negative) is the age they would have had at a size of zero if they had always grown in the manner predicted by the equation (which they have not; see e.g., Pauly, 1998).
However, given that we are dealing with biomass, the VBGF here is combined with a length–weight relationship (LWR) of the form W = a·Lb to obtain a version of the VBGF suitable for growth in weight, i.e.,
where Winf is the mean weight attained after an infinitely long time, and the other parameters are as defined previously.
Recall that, in fisheries science and in Ecopath, it is expressed by
where Nt is the number at time t, Nt−Δt is the number at time t – Δt, and Z is the instantaneous rate of total mortality, which happens to be equal to the P/B ratio, a parameter of Ecopath models, when growth follows the VBGF (Allen, 1971). Thus, the biomass contribution Bt of a species (or group, see below) in a given size bin can be computed from
where Δt is the time the group takes to grow through a bin, or weight class, with Wt as defined above; Bt is scaled over all weight classes so as to sum up to the total biomass of the group.
As for Δt, it is estimated as the time difference between the relative ages (t′) computed from the inverse of the VBGF, i.e.,
where t′ is the relative age corresponding to the lower or upper limit of the weight interval, and all other parameters are as defined previously. Note that t0, which allows absolute ages to be computed, is not required because Δt is computed as a difference between relative ages.
Thus, to construct a PSD when a parameterized and balanced Ecopath model is available, all that is needed are Linf (or Winf) and K values to deal with the ontogeny of each group (when Linf only is available, a LWR must be also provided, but its exponent b can be assumed = 3; Froese, 2006; Hay et al., 2020).
The growth parameters of the species reported or assumed to contribute most of the biomass in each group were used to describe the growth of each functional group. These growth parameters originated from FishBase (www.fishbase.org), SeaLifeBase (www.sealifebase.org), and the scientific literature. When several sets of growth parameters were available, those originating from China or nearby countries were selected. For species without growth parameters, similar size species from the same genus or family were taken as substitutes (see Table SI). This applied also to zooplankton, of which an increasing number of species have their growth described by the VBGF (see, e.g., Wang et al., 2017).
For Ecopath models in which jellyfish were listed as a separate group, the input biomasses and catches of jellyfish were adjusted by reducing their values to the ones they would have if they had the same water content as fish, i.e., from an average of 98% to an approximate value of 75% (Palomares and Pauly, 2008). These and other minor adjustments of the 19 Ecopath models (see Table 1) were done for each model separately, such as avoid artificially introducing common features.
Multivariate Analyses
Nine of the indicators output by Ecopath were used for comparisons between the 19 models at hand, i.e., the slope of PSDs, mean year of coverage, mean latitude (ML) of area covered, total system throughput (TST, in tonnes km−2 year−1), mean trophic level of the catch (MTLc), mean trophic level of the ecosystem (MTLe), total biomass (TB), total catch (TC), and Shannon–Wiener diversity index (H′). MTL and H′ values were calculated based on functional groups instead of species. All indices except mean year and mean latitude were calculated by the program EwE 6.6 (Christensen et al., 2000).
Among these nine indicators, year, slope, MTLc, MTLe, and TC are the factors that can be related to fishery impacts on ecosystems. Four indicators, ML, TST, TB, and H′ are environmental factors; TST is the total annual biomass flux through all direct trophic interactions, which indicate the size of ecosystems; TB and H′ define the size and complexity of ecosystems from different perspectives; and ML deals with the impacts on ecosystems of different geographic locations (e.g., via temperature). These are assumed to be the most representative indicators of impacts on the ecosystems by both human and environmental factors.
Regression analysis was used to explore the relationships between the slopes of the PSD, and these indicators and multivariate analyses were performed to compare the structure and function of the ecosystems represented by the 19 models. Multidimensional scaling (MDS; Borg and Groenen, 2003) and H-clustering (Johnson, 1967; Bishop and Tipping, 1998; Fraley, 1998) were used to classify the 19 models based on the 9 indicators above, and the differences between the groups were tested by an analysis of similarities (ANOSIM). Similarity percentage analysis (SIMPER) was applied to identify the indicator associated with the difference between models. These analyses were conducted using Microsoft Excel and the Rstudio software.
Results
Adjustments to the Ecopath Models
Fifteen models contained jellyfish as explicit group. They were all adjusted for water contents, i.e., there biomass was reduced (see above). In addition, their estimates of ecotrophic efficiency (EE) was increased from an average of 0.37–0.77, as suggested by a negative correlation (p < 0.01) between EE and biomass (see Table 3). This implies that their production is consumed at about twice the rate assumed by the original authors.
PSD Slopes
Figure 2, pertaining to the Bohai Sea, illustrates the model adjustments that were performed to obtain the PSD slope summarized in Table 2, while the adjustments required for the other models are illustrated in Figures SI, SII. For eight of the models, the adjustments lead to the PSD exhibiting markedly closer correlation, while the remainder of the models was not affected much.
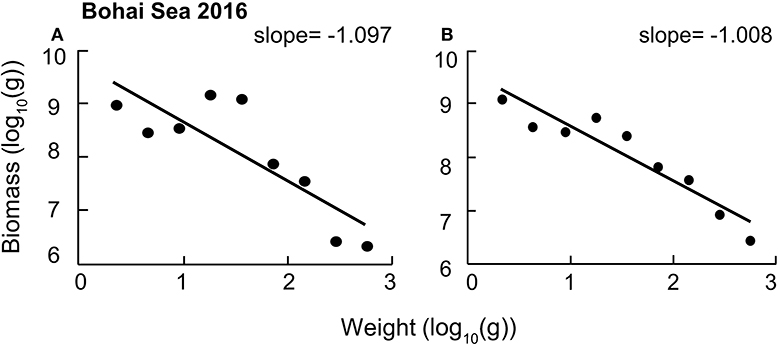
Figure 2. Results of particle size distribution (PSD) for the model of Bohai Sea (2016) in China. (A) Before adjusting the biomass of jellyfish. (B) After adjusting the biomass of jellyfish.
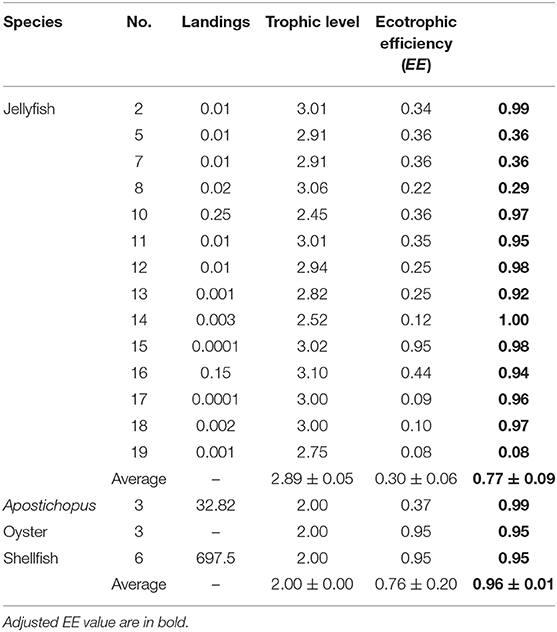
Table 3. Ecotrophic parameters for jellyfish and farmed species in China's seas (landings in tonnes km−2 year−1).
The slopes of 19 models in Chinese coastal seas are shown in Figure 3. The mean slope and standard error were −0.89 ± 0.069, and their range was −0.38 to −1.61. One of the main results is that, for all models representing 2 or more years of the same ecosystems, there was a clear steepening of the slopes with time (Figure 4), suggesting that, in recent years, the upper higher trophic levels of costal food webs have disappeared.
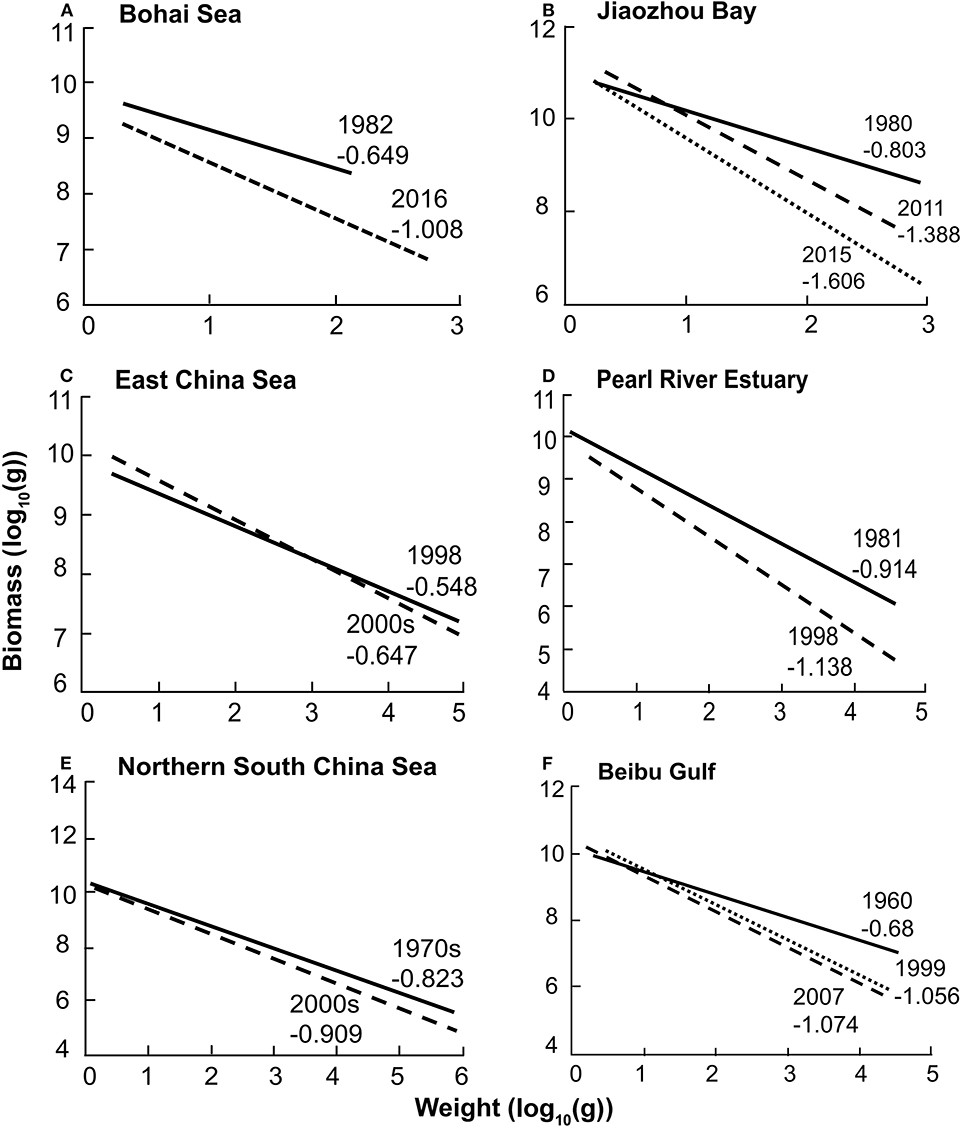
Figure 4. Comparison of particle size distribution (PSD) slopes for 6six paired models in China's seas from 1960s to 2010s. (A) Bohai Sea; (B) Jiaozhou Bay; (C) East China Sea; (D) Pearl River Estuary; (E) Northern South China Sea; (F) Beibu Gulf.
Multivariate Analyses
The MDS analyses and the H-clustering produced similar results, i.e., they both divided the 19 models into 5 clusters (Figure 5). One cluster consisted of northern South China Sea (1970s and 2000s) and another three models consisted of Jiaozhou Bay (1980, 2011, and 2015). The “stress” of MDS is an indicator of the fit of the graph to the available data. Here, the MDS result had an “excellent” fit, with a stress value of 0.01(<2.5%; Kruskal, 1964).
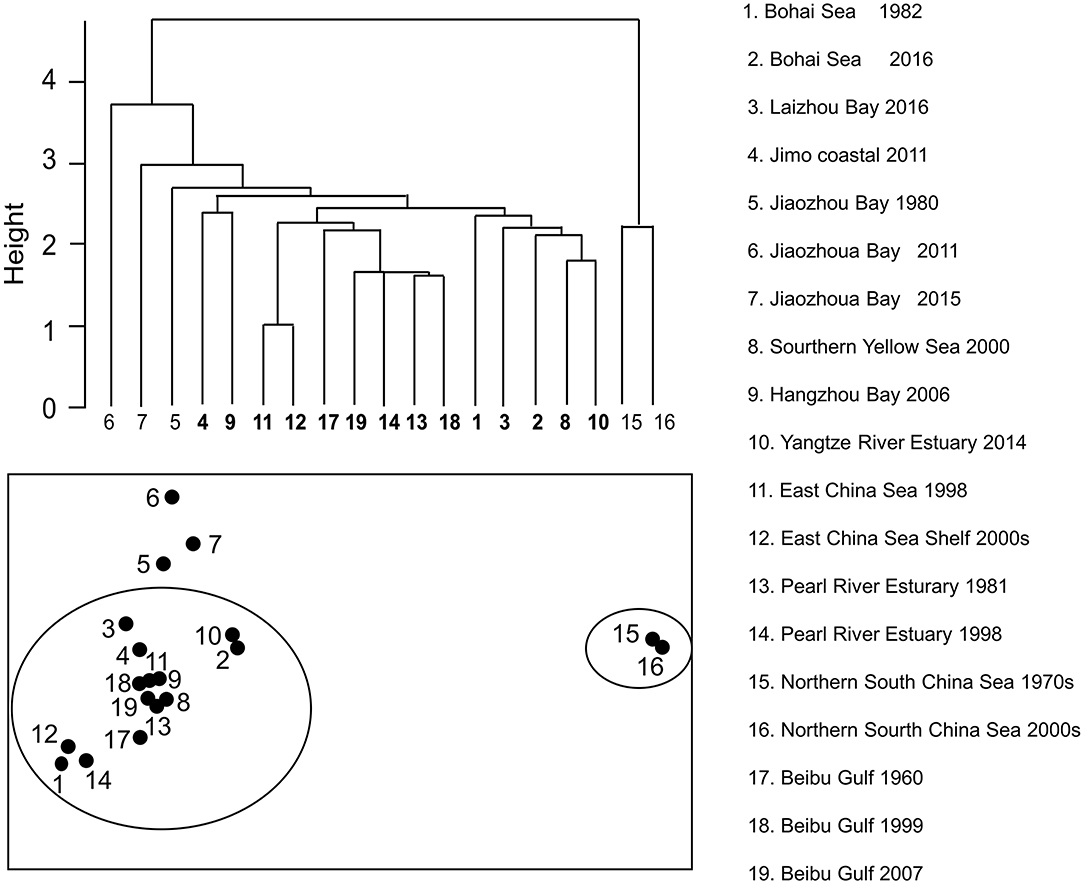
Figure 5. H-clustering and multidimensional scaling (MDS) groups for 19 Ecopath models in China's coastal seas.
The ANOSIM yielded a global R of 0.70 > 0 (p < 0.001), implying that the difference between groups was significant. The SIMPER analysis indicated that the main reason for the difference between the models was TST, i.e., the scale of the ecosystems; the second reason is TC.
The slopes were significantly correlated with TC, H′, and TC × MTLc when all 19 models were included (see Figures 6A–C). In addition, the slopes have significant relationships with year and MTLc for the 14 models representing the same areas in different years (see Figures 6D,E). A multiple regression predicting the PSD slopes from year and mean trophic level of the catch had a multiple coefficient of variation (R2) of 0.385, implying that year and mean trophic level explain 38.5% of the variance in the slopes (see Figure 6F).
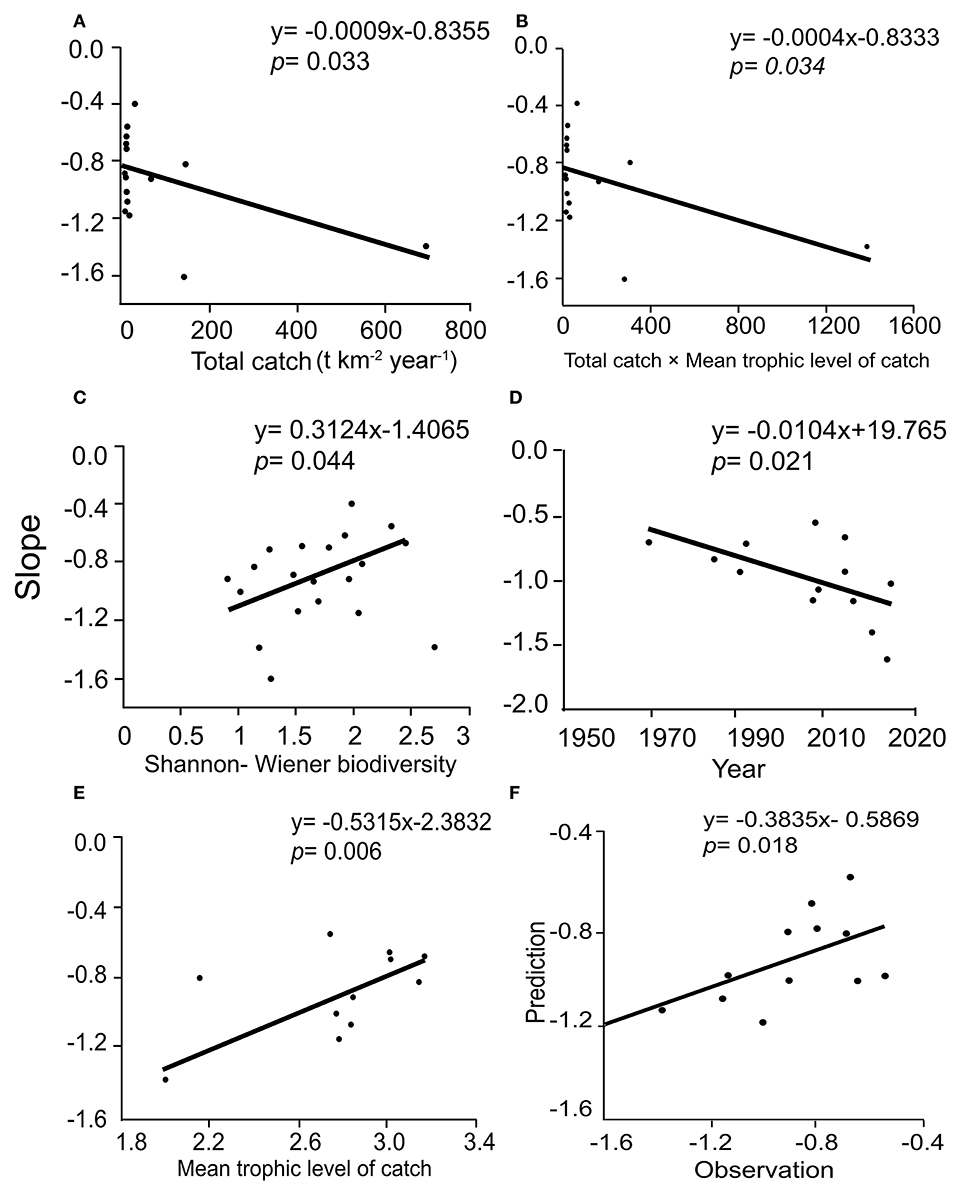
Figure 6. Regression of the slopes of particle size distributions in 19 Ecopath models of Chinese coastal ecosystems against explanatory factors. (A) Total catch; (B) Total catch × mean trophic level; (C) Shannon-Wiener biodiversity index; (D) Year; (E) Mean trophic level of catch; (F) Predicted vs observed slope (see text).
Discussion
The key insights emanating from this study are, in our opinion, that:
(1) Constructing PSD plots from balanced Ecopath models is straightforward;
(2) but jellyfish and mariculture require special treatment;
(3) PSD do reflect fishing pressure on ecosystems, and thus,
(4) PSD should become part of the completion and verification of Ecopath models.
We elaborate on these four points.
The routine of EwE 6.6 that can be downloaded from www.ecopath.org which allows for deriving PSD from Ecopath models is, as mentioned above, very easy to use. Particularly useful is a graph that shows the biomass that each group contributes to different body weight bins and which was used for the model adjustments described above.
Moreover, contrary to the situation prevailing only a few years ago, growth parameters and LWRs are increasingly available for fish in FishBase (Froese and Pauly, 2019; Hay et al., 2020), SeaLifeBase (Palomares and Pauly, 2019), and online literature (e.g., Palomares and Pauly, 2008) such that even groups for which other growth models had been used now have the VBGF to describe their growth, a trend likely to continue.
The results obtained here suggest that one of main reasons for imbalance in Chinese coastal ecosystems is due to jellyfish, whose biomass must be adjusted—if it was no done in the original models—for their extremely high water content (Palomares and Pauly, 2008) and the short duration of the period during which their biomass is high—particularly in jellyfish species that are ranched (You et al., 2007; Dong et al., 2008). In these species, the high biomass period may last only 1 or 2 months, and thus, their ecosystem impact (i.e., food consumption) must be reduced accordingly; such temporal adjustments were not done by the authors of all models.
Although not used here, temporal adjustments may also have to be performed in some cases for short-lived farmed species, e.g., those on artificial reefs, deployed along coastline to simulate the natural reefs environment, to provide a replacement for lost natural habitats to marine fisheries resources (Baine, 2001). Thus, the models for artificial reefs in Laizhou Bay showed serious impacts on the trophic flows by individual farmed species, notably by a high biomass of sea cucumber and oyster that had spilled over from a nearby farm (Xu et al., 2019). Such adjustment can be done by multiplying the mean biomass of the group while it is in the system by the fraction of the year (often <<1) that it is in the system.
Another adjustment that might have to be considered in some cases (though it was not used here) is to reduce the biomass in the smallest size group (phytoplankton and microzooplankton), when its size range is smaller than the bin size of the PSD plots (such adjustments are required for reason of arithmetic and do not imply that the Ecopath models in question are in error).
As expected, different models covering the same area exhibit PSD slopes that became steeper over time, implying more fishing pressure on these areas, which is in line with what is known of fisheries along China's coastline (Ling et al., 2006; Li et al., 2015; Teh et al., 2017). The PSD for the Bohai Sea, Jiaozhou Bay, Pearl River Estuary, Beibu Gulf, and other coastal and nearshore ecosystems had steeper slope than the PSD for the wider East China Sea and the northern South China Sea, which is consistent with the result of Liang and Pauly (2017b), who demonstrated a strong fishing down effect (which induces strongly negative PSD) along the coast of the East China Sea, followed by the development of an offshore fishery, which induces another fishing down trend.
The TST index was the main reason why the northern South China Sea model is different from others, while the TC index was the main reason why the models of Jiaozhou Bay (1980, 2011, and 2015) formed a distinct group. Jiaozhou Bay is the most important shellfish mariculture site in the northern China, and its high TC values are mostly due to shellfish farming and fishing for escapees from farms. Therefore, it appears that mariculture has more impacts on the ecosystems than (over)fishing.
The steepness of the PSD slopes, which expresses fishing pressure, was significantly negatively correlated with the year and TC variables and was significantly positively correlated with H′ and MTLc. This implies that the marine ecosystems that have a high biodiversity and MTLc would be in better shape than the others. Given a background of generalized overfishing, this would be reversed with higher TC, indicating that reducing the fishery catch is conducive to the balance of the marine ecosystems' structure and function. Moreover, the impacts of year variable is not direct, rather mainly associated with TC and the depletion of fishery resources, which increase through time. Thus, in China, if the high fishing pressure is maintained, or even increase, the PSD slope of coastal marine ecosystems will continue to decline. In addition, the slopes were positively correlated with MTLc, implying that an ecosystem with a less negative PSD slope will have more high-trophic level fish that can be caught, which is another definition of “fishing down” (Pauly et al., 1998, 2001; Liang and Pauly, 2019).
High level of diversity is conducive to the stability of ecosystem (Elmqvist et al., 2003), and thus, models should represent that biodiversity ought to be represented. Several of the models considered here lacked large marine mammals, sharks, and/or seabirds; while many of these larger animals do not occur along China's coast, some do, if in small numbers, and models should include them. Some PSD slopes were too steep because of the omissions.
Overall, this contribution, besides further confirming that China's coastlines are strongly exploited by fisheries, also confirmed that PSDs allow for numerous insights and inference on food web models and the ecosystems they represent. Moreover, PSDs, which are easily constructed, help identify problems with the parametrization of Ecopath models. Thus, while Ecopath-derived PSDs are not common so far (but see Palomares et al., 2009), we suggest that they should be, in China and elsewhere.
Author Contributions
LZ was responsible for the data collection, formal analysis, and writing of the original draft. DP was responsible for the conceptualization, methodology, and supervision.
Funding
LZ research was funded by China Scholarship Council (CSC). DP research was supported by the Sea Around Us, which receives funding from the Oak Foundation, the Marisla Foundation, the Paul M. Angell Family Foundation, the David and Lucile Packard Foundation, the Minderoo Foundation, and the Bloomberg Philanthropies through RARE.
Conflict of Interest
The authors declare that the research was conducted in the absence of any commercial or financial relationships that could be construed as a potential conflict of interest.
Acknowledgments
LZ acknowledges China Scholarship Council (CSC) for the support. DP acknowledges support for the Sea Around Us, a research initiative funded by several philanthropic foundations, notably the Oak, Marisla, and Minderoo Foundations.
Supplementary Material
The Supplementary Material for this article can be found online at: https://www.frontiersin.org/articles/10.3389/fmars.2020.00298/full#supplementary-material
References
Allen, K. R. (1971). Relation between production and biomass. J. Fish. Res. Board Can. 28, 1573–1581. doi: 10.1139/f71-236
Baine, M. (2001). Artificial reefs, a review of their design, application, management and performance. Ocean Coast. Manag. 44, 241–259. doi: 10.1016/S0964-5691(01)00048-5
Bianchi, G., Gislason, H., Graham, K., Hill, L., Jin, X., Koranteng, K., et al. (2000). Impact of fishing on size composition and diversity of demersal fish communities. ICES J. Mar. Sci. 57, 558–571. doi: 10.1006/jmsc.2000.0727
Bishop, C. M., and Tipping, M. E. (1998). A hierarchical latent variable model for data visualization. IEEE Trans. Pattern Anal. Mach. Intell. 20, 281–293. doi: 10.1109/34.667885
Blanchard, J. L., Heneghan, R. F., Everett, J. D., Trebilco, R., and Richardson, A. J. (2017). From bacteria to whales, using functional size spectra to model marine ecosystems. Trends Ecol. Evol. 32, 174–186. doi: 10.1016/j.tree.2016.12.003
Borg, I., and Groenen, P. J. F. (2003). Modern multidimensional scaling, theory and applications. J. Educ. Measur. 40, 277–280. doi: 10.1111/j.1745-3984.2003.tb01108.x
Boudreau, P. R., and Dickie, L. M. (1992). Biomass spectra of aquatic ecosystems in relation to fisheries yield. Can. J. Fish. Aquat. Sci. 49, 1528–1538. doi: 10.1139/f92-169
Brotz, L., Cheung, W. W. L., Kleisner, K., Pakhomov, E., and Pauly, D. (2012). “Increasing jellyfish populations, trends in large marine ecosystems,” in Jellyfish Blooms, Series IV. eds J. E. Purcell, H. Mianzan, and J. R. Frost (Dordrecht: Springer, 3–20 doi: 10.1007/978-94-007-5316-7_2
Cao, L., Wang, W. M., Yang, Y., Yang, C. T., Yuan, Z. H., Xiong, S. B., et al. (2007). Environmental impact of aquaculture and countermeasures to aquaculture pollution in China. Environ. Sci. Pollut. Res. Int. 14, 452–462. doi: 10.1065/espr2007.05.426
Chen, Z., Qiu, Y. S., Jia, X. P., and Xu, S. N. (2008). Using an ecosystem modeling approach to explore possible ecosystem impacts of fishing in the Beibu Gulf, northern South China Sea. Ecosystems 11, 1318–1334. doi: 10.1007/s10021-008-9200-x
Chen, Z. Z., Qiu, Y. S., Jia, X. P., and Zhong, Z. H. (2008). Effects of fishing on the marine ecosystem of Beibu Gulf. Chin. J. Appl. Ecol. 19, 1604–1610. doi: 10.7666/d.y1291216
Cheung, W. W. L. (2007). Vulnerability of Marine Fishes to Fishing, From Global Overview to the Northern South China Sea (Doctoral thesis), University of British Columbia, Vancouver, Canada.
Christensen, V., and Pauly, D. (1992). ECOPATH II-a software for balancing steady-state ecosystem models and calculating network characteristics. Ecol. Model. 61, 169–185. doi: 10.1016/0304-3800(92)90016-8
Christensen, V., Walters, C. J., and Pauly, D. (2000). Ecopath with Ecosim: a user's guide. Vancouver, BC: University of British Columbia, Fisheries Centre, Canada and ICLARM, Penang, Malaysia, 154.
Coll, M., Akoglu, E., Arreguin-Sanchez, F., Fulton, E. A., Gascuel, D., Heymans, J. J., et al. (2015). Modelling dynamic ecosystems, venturing beyond boundaries with the Ecopath approach. Rev. Fish Biol. Fish. 25, 413–424. doi: 10.1007/s11160-015-9386-x
Colléter, M., Valls, A., Guitton, J., Gascuel, D., Pauly, D., and Christensen, V. (2015). Global overview of the applications of the Ecopath and Ecosim modeling approach using the ecobase model repository. Ecol. Model. 302, 42–53. doi: 10.1016/j.ecolmodel.2015.01.025
Dong, J., Jiang, L. X., Tan, K. F., Liu, H. Y., Purcell, J. E., Li, P. J., et al. (2008). “Stock enhancement of the edible jellyfish (Rhopilema esculentum Kishinouye) in Liaodong Bay, China, a review,” in Jellyfish Blooms, Causes, Consequences, and Recent Advances, 2008, eds K. A. Pitt and J. E. Purcell (Dordrecht: Springer), 113–118. doi: 10.1007/978-1-4020-9749-2_8
Dong, Z. J., Liu, D. Y., and Keesing, J. K. (2010). Jellyfish blooms in China, dominant species, causes and consequences. Mar. Pollut. Bull. 60, 954–963. doi: 10.1016/j.marpolbul.2010.04.022
Duan, L. J., Li, S. Y., Liu, Y., Moreau, J., and Christensen, V. (2009). Modeling changes in the coastal ecosystem of the pearl river estuary from 1981 to 1998. Ecol. Model. 220, 2802–2818. doi: 10.1016/j.ecolmodel.2009.07.016
Elmqvist, T., Folke, C., Nyström, M., Peterson, G., Bengtsson, J., Walker, B., et al. (2003). Response diversity, ecosystem change, and resilience. Front. Ecol. Environ. 1, 488–494. doi: 10.1890/1540-9295(2003)001[0488:RDECAR]2.0.CO;2
Fraley, C. (1998). Algorithms for model-based Gaussian hierarchical clustering. SIAM J. Sci. Comput. 20, 270–281. doi: 10.1137/S1064827596311451
Froese, R. (2006). Cube law, condition factors and weight-length relationships, history, meta-analysis and recommendations. J. Appl. Ichthyol. 22, 241–253. doi: 10.1111/j.1439-0426.2006.00805.x
Froese, R., and Pauly, D. (2019). FishBase. Species list, World Wide Web electronic publication. Available online at: www.fishbase.org, version: 08/2019.
Fulton, E. A., Smith, A. D., and Johnson, C. R. (2003). Effect of complexity on marine ecosystem models. Mar. Ecol. Prog. Series 253, 1–16. doi: 10.3354/meps253001
Guiet, J., Poggiale, J. C., and Maury, O. (2016). Modelling the community size-spectrum, recent developments and new directions. Ecol. Model. 337, 4–14. doi: 10.1016/j.ecolmodel.2016.05.015
Han, D. Y., Xue, Y., Zhang, C. L., and Ren, Y. P. (2017). A mass balanced model of trophic structure and energy flows of a semi-closed marine ecosystem. Acta Oceanol Sinica 36, 60–69. doi: 10.1007/s13131-017-1071-6
Hay, A., Xian, W., Bailly, N., Liang, C., and Pauly, D. (2020). The why and how of determining length-weight relationships of fish from preserved museum specimens. J. Appl. Ichyol. 36, 376–382. doi: 10.1111/jai.14014
Hunt, B. P., Allain, V., Menkès, C., Lorrain, A., Graham, B., Rodier, M., et al. (2015). A coupled stable isotope-size spectrum approach to understanding pelagic food-web dynamics, a case study from the southwest sub-tropical Pacific. Deep Sea Res. Part II Top. Stud. Oceanogr. 113, 208–224. doi: 10.1016/j.dsr2.2014.10.023
Jiang, H., Cheng, H. Q., Xu, H. G., Arreguín-Sánchez, F., Zetina-Rejón, M. J., Luna, P. D. M., et al. (2008). Trophic controls of jellyfish blooms and links with fisheries in the East China Sea. Ecol. Model. 212, 492–503. doi: 10.1016/j.ecolmodel.2007.10.048
Jiao, N. Z., Chen, D. K., Luo, Y. M., Huang, X. P., Zhang, R., Zhang, H. B., et al. (2015). Climate change and anthropogenic impacts on marine ecosystems and countermeasures in China. Adv. Clim. Change Res. 6, 118–125. doi: 10.1016/j.accre.2015.09.010
Johnson, S. C. (1967). Hierarchical clustering schemes. Psychometrika 32, 241–254. doi: 10.1007/BF02289588
Kruskal, J. B. (1964). Multidimensional scaling by optimizing goodness of fit to a nonmetric hypothesis. Psychometrika 29, 1–27. doi: 10.1007/BF02289565
Lamb, P. D., Hunter, E., Pinnegar, J. K., Doyle, T. K., Creer, S., and Taylor, M. I. (2019). Inclusion of jellyfish in 30+ years of Ecopath and Ecosim models. ICES J. Mar. Sci. 76, 1941–1950. doi: 10.1093/icesjms/fsz165
Li, Q. C., Zou, Y., Zhang, S. C., Wang, Y. J., Zhao, D., and Song, A. H. (2015). Changes in main fish stocks in Bohai Sea and Shandong Province. Fish. Sci. 34, 647–651. doi: 10.16378/j.cnki.1003-1111.2015.10.009
Li, Y., Chen, Y., Olson, D., Yu, N., and Chen, L. (2009). Evaluating ecosystem structure and functioning of the East China sea shelf ecosystem, China. Hydrobiologia. 636:331. doi: 10.1007/s10750-009-9964-9
Liang, C., and Pauly, D. (2017a). Growth and mortality of exploited fishes in China's coastal seas and their uses for yield-per-recruit analyses. J. Appl. Ichthyol. 33, 746–756. doi: 10.1111/jai.13379
Liang, C., and Pauly, D. (2017b). Fisheries impacts on China's coastal ecosystems, unmasking a pervasive ‘fishing down' effect. PLoS ONE. 12:e0173296. doi: 10.1371/journal.pone.0173296
Liang, C., and Pauly, D. (2019). Masking and unmasking fishing down effects, the Bohai Sea (China) as a case study. Ocean Coast. Manag. 184:105033. doi: 10.1016/j.ocecoaman.2019.105033
Liang, C., Xian, W. W., and Pauly, D. (2018). Impacts of ocean warming on china's fisheries catch, application of the 'mean temperature of the catch'. Front. Mar. Sci. 5:26. doi: 10.3389/fmars.2018.00026
Limburg, K. E., O'Neill, R. V., Costanza, R., and Farber, S. (2002). Complex systems and valuation. Ecol. Econ. 41, 409–420. doi: 10.1016/S0921-8009(02)00090-3
Lin, Q., Jin, X., and Zhang, B. (2013). Trophic interactions, ecosystem structure and function in the southern Yellow Sea. Chin. J. Oceanol. Limnol. 31, 46–58. doi: 10.1007/s00343-013-2013-6
Ling, J. Z., Li, S. F., and Yan, L. P. (2006). Analysis on the utilization of main fishery resources in the East China Sea. Mar. Fish. 28, 111–116. doi: 10.13233/j.cnki.mar.fish.2006.02.005
Liu, W., and He, M. (2012). Effects of ocean acidification on the metabolic rates of three species of bivalve from southern coast of China. Chin. J. Oceanol. Limnol. 30, 206–211. doi: 10.1007/s00343-012-1067-1
Lynam, C. P., Heath, M. R., Hay, S. J., and Brierley, A. S. (2005). Evidence for impacts by jellyfish on North Sea herring recruitment. Mar. Ecol. Prog. Series 298, 157–167. doi: 10.3354/meps298157
Ma, M. L. (2018). The structure and function of the typical semi-enclosed by ecosystem based on Ecopath model. (Master's thesis), Shanghai Ocean University, Shanghai, China.
Palomares, M. L. D., Bailly, N., and Pauly, D. (2009). “FishBase, SeaLifeBase and database-driven ecosystem modeling,” in Ecopath 25 Years Conference Proceedings, Extended Abstracts, eds M. L. D. Palomares, L. Cisneros-Montemayor, A. Morissette, D. Varkey, M. Coll, C. Piroddi (Fisheries Centre Research Reports), Vancouver, BC: Fisheries Centre, University of British Columbia, 156–158.
Palomares, M. L. D., and Pauly, D. (2008). “The growth of jellyfishes,” in Jellyfish Blooms, Causes, Consequences, and Recent Advances, eds K. A. Pitt and J. E. Purcell (Dordrecht: Springer), 11–21. doi: 10.1007/978-1-4020-9749-2_2
Palomares, M. L. D., and Pauly, D. (2019). SeaLifeBase. World Wide Web electronic publication. www.sealifebase.org, version: 12/2019.
Pauly, D. (1998). Beyond our original horizons, the tropicalization of Beverton and Holt. Rev. Fish Biol. Fish. 8, 307–334. doi: 10.1023/A:1008863215253
Pauly, D., and Christensen, V. (1993). “Stratified models of large marine ecosystems, a general approach and an application to the South China Sea,” in, Stress, Mitigation and Sustainability of Large Marine Ecosystems, eds K. Sherman., L. M. Alexander and B. D. Gold (Washington, DC: Gold American Association for the Advancement of Science, 148–174.
Pauly, D., and Christensen, V. (2002). “Chapter 10. Ecosystem models,” in, Handbook of Fish Biology and Fisheries, Vol. 2, eds P. J. B. Hart and J. D. Reynolds (Oxford: Blackwell Science Ltd). 211–227. doi: 10.1002/9780470693919.ch10
Pauly, D., Christensen, V., Dalsgaard, J., Froese, R., and Torres, F. (1998). Fishing down marine food webs. Science 279, 860–863. doi: 10.1126/science.279.5352.860
Pauly, D., Christensen, V., and Walters, C. J. (2000). Ecopath, Ecosim and Ecospace as tools for evaluating ecosystem impact of fisheries. ICES J. Mar. Sci. 57, 697–706. doi: 10.1006/jmsc.2000.0726
Pauly, D., Graham, W., Libralato, S., Morissette, L., and Palomares, M. L. D. (2008). “Jellyfish in ecosystems, online databases and ecosystem models,” in Jellyfish Blooms, Causes, Consequences, and Recent Advances. Developments in Hydrobiology, Vol. 206, eds K. A. Pitt and J. Purcell (Dordrecht: Springer), 67–85. doi: 10.1007/978-1-4020-9749-2_5
Pauly, D., and Liang, C. (2019). The fisheries of the South China Sea, major trends since 1950. Mar. Policy 32, 816–822. doi: 10.1016/j.marpol.2019.103584
Pauly, D., Palomares, M. L. D., Froese, R., Sa-a, P., Vakily, M., Preikshot, D., et al. (2001). Fishing down Canadian aquatic food webs. Can. J. Fish. Aquat. Sci. 58, 51–62. doi: 10.1139/f00-193
Pauly, D., Soriano-Bartz, M. L., and Palomares, M. L. D. (1993). “Improved construction, parametrization and interpretation of steady-state ecosystem models,” in Trophic Models of Aquatic Ecosystems, eds V. Christensen and D. Pauly (Manila: ICLARM Conference Proceedings, 1–13.
Polovina, J. J. (1984). Model of a coral reef ecosystem. Coral Reefs 3, 1–11. doi: 10.1007/BF00306135
Rahman, M. F., Lin, Q., Shan, X. J., Chen, Y. L., Ding, X. S., and Liu, Q. (2019). Temporal changes of structure and functioning of the Bohai Sea ecosystem, insights from Ecopath models. Thalas. Int. J. Mar. Sci. 35, 625–641. doi: 10.1007/s41208-019-00139-1
Sheldon, R. W., Prakash, A., and Sutcliffe, W. H. Jr. (1972). The size distribution of particles in the ocean. Limnol. Oceanogr. 17, 327–340. doi: 10.4319/lo.1972.17.3.0327
Sherman, K., and Hempel, G., (eds.). (2008). The UNEP Large Marine Ecosystem Report, A Perspective on Changing Conditions in LMEs of the World's Regional Seas. Nairobi, Kenya, UNEP Regional Seas Reports and Studies No. 182, p.852.
Su, M. (2016). Preliminary analysis of the Jimo coastal ecosystem with the Ecopath model. J. Ocean Univ. China 15, 1059–1066. doi: 10.1007/s11802-016-3078-6
Sun, L. Q., Lin, Y. S., Chen, L. X., Cao, W. Q., and Zheng, L. M. (2016). Analysis of ecosystem structure and function the northern Beibu Gulf VII, nutrition structure and keystone species selection based on Ecopath with Ecosim. J. Trop. Oceanogr. 35, 51–62. doi: 10.1016/j.ecss.2006.10.013
Teh, L. S., Witter, A., Cheung, W. W., Sumaila, U. R., and Yin, X. (2017). What is at stake? Status and threats to South China Sea marine fisheries. Ambio 46, 57–72. doi: 10.1007/s13280-016-0819-0
Tong, L., Tang, Q. S., and Pauly, D. (2000). A preliminary approach on mass-balance Ecopath model of the Bohai Sea. J. Appl. Ecol. 11, 435–440. doi: 10.13287/j.1001-9332.2000.0109
Wallis, J. R., Swadling, K. M., Everett, J. D., Suthers, I. M., Jones, H. J., Buchanan, P. J., et al. (2016). Zooplankton abundance and biomass size spectra in the East Antarctic sea-ice zone during the winter - spring transition. Deep Sea Res. Part II Top. Stud. Oceanogr. 131, 170–181. doi: 10.1016/j.dsr2.2015.10.002
Wang, B., Yu, G., Huang, J., Wang, T., and Hu, H. Y. (2011). Probabilistic ecological risk assessment of DDTs in the Bohai Bay based on a food web bioaccumulation model. Sci. Total Environ. 409, 495–502. doi: 10.1016/j.scitotenv.2010.10.039
Wang, K., Hunt, B. P. V., Liang, C., Pauly, D., and Pakhomov, E. A. (2017). Reassessment of the life cycle of the pteropod Limacina helicina from a high resolution interannual time series in the temperate North Pacific. ICES J. Mar. Sci. 74, 1906–1920. doi: 10.1093/icesjms/fsx014
Wang, Y., and Aubrey, D. G. (1987). The characteristics of the China coastline. Contin. Shelf Res. 7, 329–349. doi: 10.1016/0278-4343(87)90104-X
Wang, Y. C. (2019). Modelling the structure and interannual dynamics of energy flows in Yangtze Estuary and its adjacent waters (Master's thesis), Institute of Oceanology, Chinese Academy of Sciences, Qingdao, China, 103.
Wang, Y. S., Lou, Z. P., Sun, C. C., and Sun, S. (2008). Ecological environment changes in Daya Bay, China, from 1982 to 2004. Mar. Pollut. Bull. 56, 1871–1879. doi: 10.1016/j.marpolbul.2008.07.017
Xian, W. W., Kang, B., and Liu, R. Y. (2005). Jellyfish blooms in the Yangtze Estuary. Science 307:41. doi: 10.1126/science.307.5706.41c
Xu, M., Qi, L., Zhang, L. B., Zhang, T., Yang, H. S., and Zhang, Y. L. (2019). Ecosystem attributes of trophic models before and after construction of artificial oyster reefs using Ecopath. Aquacult. Environ. Inter. 11, 111–127. doi: 10.3354/aei00284
Xu, S. N., Chen, Z. Z., Li, S. Y., and He, P. M. (2011). Modeling trophic structure and energy flows in a coastal artificial ecosystem using mass-balance Ecopath model. Estuaries Coasts 34, 351–363. doi: 10.1007/s12237-010-9323-0
You, K., Ma, C., Gao, H., Li, F., Zhang, M., Qiu, Y., et al. (2007). Research on the jellyfish (Rhopilema esculentum Kishinouye) and associated aquaculture techniques in China, current status. Aquacult. Int. 15, 479–488. doi: 10.1007/s10499-007-9114-1
Keywords: Ecopath food web model, farmed jellyfish, fishing down food web, China's marine ecosystems, PSD spectra
Citation: Zhai L and Pauly D (2020) Construction and Interpretation of Particle Size Distribution Spectra From 19 Ecopath Models of Chinese Coastal Ecosystems. Front. Mar. Sci. 7:298. doi: 10.3389/fmars.2020.00298
Received: 27 January 2020; Accepted: 14 April 2020;
Published: 26 May 2020.
Edited by:
António V. Sykes, University of Algarve, PortugalReviewed by:
Valeria Mamouridis, Independent Researcher, Rome, ItalyHui Zhang, Chinese Academy of Sciences, China
Copyright © 2020 Zhai and Pauly. This is an open-access article distributed under the terms of the Creative Commons Attribution License (CC BY). The use, distribution or reproduction in other forums is permitted, provided the original author(s) and the copyright owner(s) are credited and that the original publication in this journal is cited, in accordance with accepted academic practice. No use, distribution or reproduction is permitted which does not comply with these terms.
*Correspondence: Lu Zhai, zhailu0708@hotmail.com