- 1Deltares, Delft, Netherlands
- 2Institute for Environmental Studies (IVM), Vrije Universiteit Amsterdam, Amsterdam, Netherlands
- 3Danish Meteorological Institute, Copenhagen, Denmark
- 4Delft University of Technology, Delft, Netherlands
The world’s coastal areas are increasingly at risk of coastal flooding due to sea-level rise (SLR). We present a novel global dataset of extreme sea levels, the Coastal Dataset for the Evaluation of Climate Impact (CoDEC), which can be used to accurately map the impact of climate change on coastal regions around the world. The third generation Global Tide and Surge Model (GTSM), with a coastal resolution of 2.5 km (1.25 km in Europe), was used to simulate extreme sea levels for the ERA5 climate reanalysis from 1979 to 2017, as well as for future climate scenarios from 2040 to 2100. The validation against observed sea levels demonstrated a good performance, and the annual maxima had a mean bias (MB) of -0.04 m, which is 50% lower than the MB of the previous GTSR dataset. By the end of the century (2071–2100), it is projected that the 1 in 10-year water levels will have increased 0.34 m on average for RCP4.5, while some locations may experience increases of up to 0.5 m. The change in return levels is largely driven by SLR, although at some locations changes in storms surges and interaction with tides amplify the impact of SLR with changes up to 0.2 m. By presenting an application of the CoDEC dataset to the city of Copenhagen, we demonstrate how climate impact indicators derived from simulation can contribute to an understanding of climate impact on a local scale. Moreover, the CoDEC output locations are designed to be used as boundary conditions for regional models, and we envisage that they will be used for dynamic downscaling.
Introduction
The world’s coastal areas are increasingly at risk of coastal flooding due to sea-level rise (SLR). By 2100, global mean sea-levels are projected to be 30–60 cm higher than today, even with a sharp reduction of greenhouse gas emissions (Church et al., 2013; Oppenheimer et al., 2019). In regions with little sea level variability, even an SLR of 10 cm could double the flooding frequency (Vitousek et al., 2017). Without mitigation and adaptation, the expected economic annual losses due to flooding may come close to 10% of the global gross domestic product (Hinkel et al., 2014). Raising flood defenses is therefore critical in densely populated and economically important coastal areas, and is cost effective for 13% of the global coastline (Lincke and Hinkel, 2018). The stabilization of global temperatures to 1.5°C, as agreed upon in the Paris Agreement, will lead to a strong reduction of the economic impact of SLR (Nicholls et al., 2018).
Accurate high-resolution projections of extreme sea levels and coastal flooding can support decision makers in identifying which regions will face the strongest increases in flood risk, and in prioritizing mitigation and adaptation efforts (Ward et al., 2015). Recent years have seen rapid progress in the application of hydrodynamic models for large-scale risk assessments (Wahl, 2017; Bouwer, 2018). With its spatially varying grid resolution, the Global Tide and Surge Model (GTSM) has sufficient resolution in coastal areas at relatively low computational costs (Verlaan et al., 2015), and as such has been leading in global hydrodynamic modeling. In recent years, GTSMv2.0 has been used to simulate extreme sea levels worldwide, with applications in operational forecasting (Verlaan et al., 2015), multi-decadal hindcasting (Muis et al., 2016), and projecting changes for future climate scenarios (Vousdoukas et al., 2018b).
The performance of the global simulations of extreme sea levels is controlled by the accuracy of the meteorological forcing, as well as by the hydrodynamic model. The previous generation of global climate models and climate reanalysis datasets typically had a resolution higher than 0.75° (approximately 79 km). While data with such a resolution performs reasonably for extra-tropical storms (Dullaart et al., 2019), it strongly underestimates the intensity of tropical cyclones (Schenkel and Hart, 2012) and associated storm surges (Bloemendaal et al., 2017; Muis et al., 2019). In 2019, the European Centre for Medium-Range Weather Forecasts (ECWMF) released the ERA5 climate reanalysis dataset. With a resolution of 0.25° (approximately 31 km), ERA5 is much better at representing the strong intensities of tropical cyclones (Belmonte Rivas and Stoffelen, 2019). Moreover, GTSMv2.0’s accuracy for global tidal modeling was insufficient, and previous large-scale studies used separate models to simulate tides, surges, and changes in mean sea level (MSL; Hinkel et al., 2014; Muis et al., 2016; Vitousek et al., 2017; Vousdoukas et al., 2017, 2018a; Brown et al., 2018; Jevrejeva et al., 2018). The individual sea level components were linearly superimposed, which means non-linear interaction effects are ignored. Tide-surge interaction is important in shallow seas with a large tidal range (Horsburgh and Wilson, 2007; de Lima Rego and Li, 2010; Mawdsley et al., 2015), and tides can significantly change in response to SLR (Pickering et al., 2012, 2017; Wilmes et al., 2017). It has been shown that climate change and SLR could lead to changes in non-linear interactions (Arns et al., 2017). The next generation Global Tide and Surge Model Version 3.0 (GTSMv3.0) was recently developed. Comparison against observations indicates that GTSMv3.0 has a tidal performance comparable to other global tidal models (Stammer et al., 2014). Hence, GTSMv3.0 can now be used to dynamically simulate tides, storm surges, and changes in MSL, including interaction effects. Moreover, GTSMv3.0 has an unprecedented high resolution along the coast, with a grid size of 2.5 km globally (and 1.25 km in Europe). This is expected to further increase the model’s accuracy, especially in areas with a complex geometry.
We use these developments in order to present a novel global dataset of extreme sea levels, namely, the Coastal Dataset for the Evaluation of Climate Impact (CoDEC). The CoDEC dataset consists of extreme sea levels (MSL, tides, and storm surges), and covers both the historical climate for the period 1979–2017 and future climates for different scenarios for the period 2040–2100. In this paper, we evaluate the performance of the dataset for the historical periods and analyze the changes in extreme sea levels for the future climate scenarios. Finally, we discuss how the CoDEC dataset can enhance the understanding of climate impact on the local scale.
Data and Methods
Global Tide and Surge Model Version 3.0
The GTSMv3.0 is a depth-averaged hydrodynamic model with global coverage that dynamically simulates tides and storm surges. GTSMv3.0 uses the unstructured Delft3D Flexible Mesh software (Kernkamp et al., 2011) and, as such, employs an efficient distribution of resolution. The model has no open boundaries, and tides are induced by including tide generating forces using a set of 60 frequencies. Surges are caused by gradients in the surface pressure of the atmosphere and the transfer of momentum from the wind to the water. We use the relation of Charnock (1955), with a drag coefficient of 0.0041, to estimate the wind stress at the ocean surface. A combination of different datasets is used for the bathymetry: EMODnet at 250 m resolution in Europe (Consortium EMODnet Bathymetry, 2018) and General Bathymetric Chart of the Ocean with a 30 arc seconds resolution for the rest of the globe (GEBCO, 2014). The bathymetry under the permanent ice shelves in Antarctica is represented by Bedmap2 (Fretwell et al., 2013).
In comparison to GTSMv2.0, which was used in previous studies (Muis et al., 2016; Vousdoukas et al., 2018b; Bloemendaal et al., 2019), the resolution of GTSMv3.0 is increased from 5 km along the coast (50 km in the deep ocean) to 2.5 km along the coast (25 km in the deep ocean). The coastal resolution was further increased to 1.25 km for Europe. The improved resolution increased the total number of grid cells from 2.0 to 5.0 million. In addition to the improved resolution, the model performance for tides was improved by the implementation of additional physical processes. This included the implementation of self-attraction and loading, and the improved parameterization of internal tides (Irazoqui Apecechea et al., 2017). To enable the dissipation of barotropic energy through the generation of internal tides, the grid resolution is further refined in areas in deep ocean that have a steep topography.
Simulations, Scenarios, and Forcing Data
Climate Reanalysis and Future Climate Scenarios
Table 1 provides an overview of the simulations and scenarios, including the acronyms used to refer to the different scenarios. For the simulation of extreme sea levels for the historical period (1979–2017), GTSMv3.0 is forced with 10 m wind speed and atmospheric pressure from the ERA5 climate reanalysis of ECMWF Copernicus Climate Change Service [C3S], 2017. ERA5 is the successor of the ERA-Interim climate reanalysis and has hourly fields with a spatial resolution of 0.25° × 0.25° (∼31 km). For the projections of extreme sea levels for climate change scenarios for the future period (2040–2100), we use meteorological fields from EC-Earth simulations developed within the Climate Model Intercomparion Phase 5 (CMIP5) initiative (Taylor et al., 2012). The EC-Earth dataset has three hourly fields with a spatial resolution of 1.125° × 1.125° (∼125 km). For Europe, we use the downscaled projections developed within the World Climate Research Program Coordinated Regional Downscaling Experiment (EURO-CORDEX) initiative (Jacob et al., 2014). We use the DMI-HIRHAM5 regional climate simulations (Christensen et al., 2007), which were nested in the global EC-Earth simulations. The DMI-HIRHAM5 dataset had three hourly fields, with a spatial resolution of 0.11° × 0.11° (∼12.5 km).
For the future period, we appy two climate change scenarios, namely, Representative Concentration Pathways (R) 4.5 and 8.5, corresponding to a radiative forcing of 4.5 and 8.5 W/m2 by the year 2100, respectively (Moss et al., 2010). The RCP8.5 pathway was developed in order to explore an unlikely high-risk future. While global carbon emissions have been tracking just above RCP8.5 (Peters et al., 2013), it is argued that recent climate policies, such as the Paris Agreement, have caused the RCP8.5 pathway to become increasingly implausible for the end of this century (Hausfather and Peters, 2020). Given computational constrains and the user requirements of the CoDEC project, it was decided to select the RCPs that we considered most plausible. Therefore, RCP8.5 is considered for the mid-century (i.e., 2041–2070), while RCP4.5 is considered for the end of the century (i.e., 2071–2100). In order to obtain a baseline simulation that would enable us to compute changes, we also simulate the historical period 1976–2005, using the same climate models.
For each scenario, we apply SLR projections that corresponded to the same RCPs. We use the mean ensemble from IPCC AR5 (Church et al., 2013), which is based on the CMIP5 models and distributed by the Integrated Climate Data Center of the University of Hamburg. The yearly SLR fields are referenced to the mean level over the period 1986–2005, with a spatial resolution of 1° × 1°.
The vertical reference of GTSMv3.0 is MSL. To make the definition of MSL consistent with the vertical reference used in the SLR fields, the mean sea-level pressure field (MSLP) over 1986–2005 is removed. The MSLP calculation is based on the ERA-Interim because ERA5 was not available at the time.
Simulations and Data Processing
We produce time series of the total water levels, surge levels, tides, and MSLs for each simulation. In order to be able to decompose the total water levels into the different sea level components, we run a tide-only simulation for each scenario. For the future climate scenarios, the tide-only simulation also includes SLR. Subsequently, the time series of surge level are computed by subtracting the tides from the total water level. The total number of simulated years is 230 years. Each year is simulated separately, using a spin-up time of 15 days. All the simulations are run on the Deltares computing cluster. Using a parallel setup with 4 cores, each 1-year simulation took 21 days to complete.
The time series are stored at a 10-min temporal resolution for 44,734 output locations. It is unfeasible to save the time series at all 5 million grid cells, as this would have generated huge amounts of data. In previous studies with GTSM (Muis et al., 2016, 2018), the output locations were based on the DIVA coastal segmentation, which included 16,611 locations (Vafeidis et al., 2008). For the CoDEC project, we develop a new set of output locations (Figure 1) based on the following procedure. First, we smooth the Natural Earth 1:10 m coastline at 5 km by applying a buffer and subsequently an inverse buffer in ArcGIS. This removes any estuaries and bays with an inlet less than 5 km wide. Second, we apply equidistant sampling along the coastline to generate points at every 50 km along the smoothed coastline. We use 10 km for Europe due to the higher resolution there. In addition, we add the locations of the tide gauge stations. In addition to the approximately 18,000 coastal output locations, we also include the output locations in the ocean that could be used as boundary conditions for regional hydrodynamic models. The output resolution is based on a regular grid that increases from the deep ocean toward the coast, that is, from 5.0 to 2.5 to 1.0° for, respectively, further than 500 km away from land, from 100 to 500 km from land, and closer than 100 km from land. The resolution is 1.0°, 0.5°, and 0.25°, respectively, for Europe. Output locations in high-latitude regions are excluded because the model performance is expected to be insufficient. This is because GTSM does not include sea ice physics and because the bathymetry in the Arctic areas is generally poor.
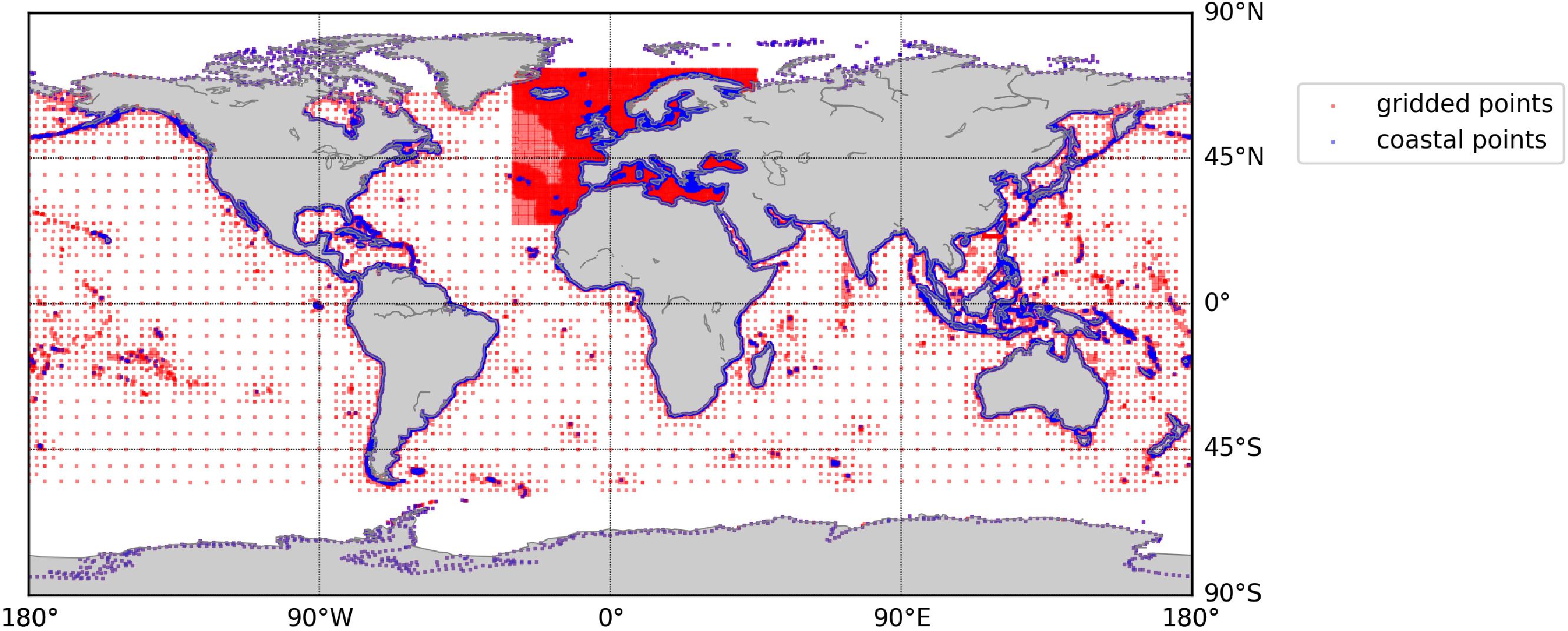
Figure 1. Overview of the output locations used for the CoDEC dataset, including the coastal locations, tide gauge stations, and gridded points.
Post-processing and Extreme Value Statistics
All the output is stored in NetCDF4 file format. The processing and analysis is done with Python 3.0 using the xarray package (Hoyer and Hamman, 2017), which allows for label-based analysis of multi-dimensional arrays and supports parallel computations on datasets that do not fit into memory. In addition, we use the Climate Data Operators (CDOs) to compute the annual maxima (Schulzweida, 2019).
To obtain extreme sea levels for the various return periods, we fit a Gumbel distribution to the annual maxima using the maximum-likelihood method. We are aware of its limitations (see section “Future Research Directions”), but the annual maxima method is computationally less demanding than the peaks-over-threshold method. Moreover, the application of the annual maxima method allows for easy comparison with the previous Global Tide and Surge Reanalysis (GTSR) dataset (see section “Evaluation of the CoDEC Dataset”). While this study focuses on the annual maxima and return periods, the CoDEC dataset also includes several other indicators, such as tidal water levels and annual percentiles for total water levels and surge levels.
Evaluation of the CoDEC Dataset
To validate CoDEC-ERA5, we compare the modeled sea levels with observed sea levels from the tide gauge stations in the Global Extreme Sea Level Analysis (GESLA) dataset (Woodworth et al., 2016). The GESLA dataset has an hourly resolution, but we use annual maxima obtained from Wahl et al. (2017). The water levels are referenced to the present-day MSL by removing the annual average sea level for each year, and subsequently also by subtracting the mean over the last 19 years from the (de-trended) time series. This dataset contains 591 tide gauge stations. Over the period 1979–2005, 327 stations have more than 20 years of data and 476 stations have more than 10 years of data. In a similar way to the modeled sea levels, we fit a Gumbel distribution to the annual maxima in order to estimate return periods. We use the tide gauge stations with more than 10 years of data and only used overlapping years.
The accuracy of the CoDEC-ERA5 sea levels is evaluated using various indicators, including Pearson’s correlation coefficient r, the root-mean-squared error (RMSE), the mean absolute error (MAE) in meters, and the mean absolute percentage error (MAPE) in percentages. The modeled and observed 1 in 10-year water levels (RP10) are validated using the mean bias (MB) in meters, the MAE in meters, and the MAPE in percentages. We show the average values across all the tide gauge stations, together with the standard deviations (SDs).
In addition to evaluating against observed sea levels, we also evaluated CoDEC-ERA5 against the previous GTSR dataset (Muis et al., 2016). CoDEC-ERA5 is the successor of GTSR and is based on the next generation climate and hydrodynamic models. Table 2 summarizes the main improvements.
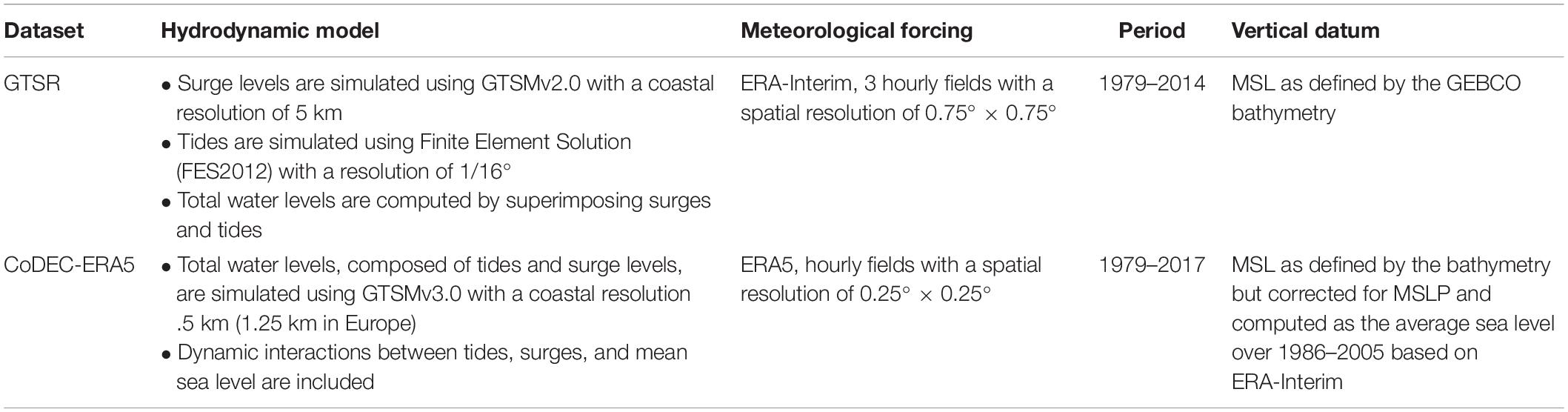
Table 2. Overview of the main differences between the CoDEC-ERA5 dataset and the previous GTSR dataset.
CoDEC-HIST is validated by comparing the 1 in 10-year water levels against both the observations and CoDEC-ERA5. While the performance of CoDEC-HIST in comparison to the observations is influenced by the model performance of the GTSMv3.0, the comparison of CoDEC-HIST against CODEC-ERA5 exposes any bias between the baseline climate simulation and the observed historical climate. A good performance provides confidence that the model setup can be used to assess changes in water levels under future climate scenarios.
Results and Discussion
Evaluation of CoDEC-ERA5 on the Basis of Annual Maxima
The comparison between the observed annual maxima from GESLA and the modeled annual maxima from CoDEC-ERA5 indicates that the average value for Pearson’s r was 0.54 (SD 0.28) across all the tide gauge stations, while the MB was -0.04 m (SD 0.32 m) (Table 3). The absolute bias is smaller than 0.2 m for 75% of the tide gauge stations. The MAPE indicates a relative error of 14.0% (SD 13.4%) across all the tide gauge stations. As the relatively high SDs indicate, the model performance varies spatially. Figures 2A,B show that CoDEC-ERA5 generally performs best in mid-latitude regions, such as northwestern Europe, southern Africa, and southern Australia. Along the coasts of islands in the Pacific Ocean, the Mediterranean Sea, and Indonesia, the correlation coefficients are generally lower than 0.5. At the same time, the absolute biases are also less than 0.1 m. This indicates a low variability in extremes in some regions, which may be due to few storms and a small tidal range, which make it difficult to capture the variability correctly. Moreover, in some regions steric processes, which are not included in the GTSM, may add to the sea level variability (Muis et al., 2018).
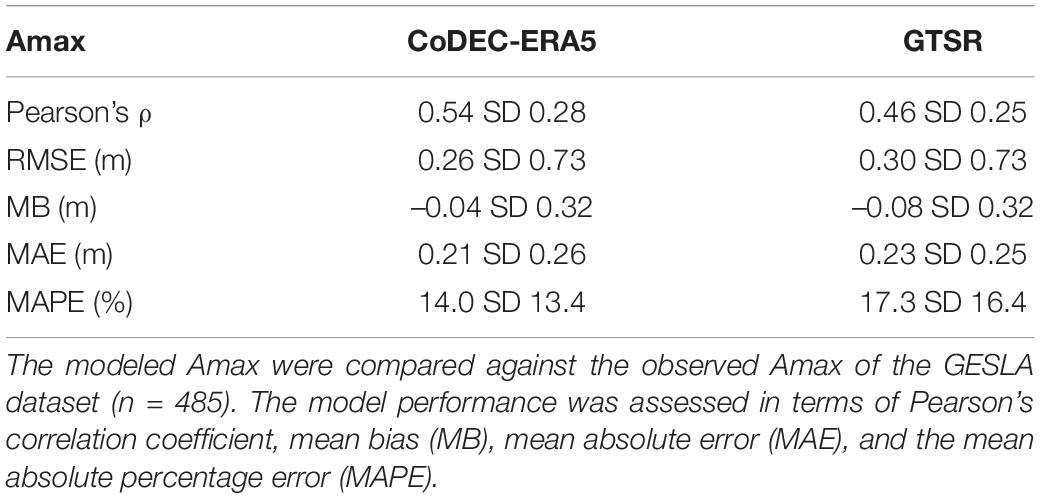
Table 3. Model performance of the annual maxima (Amax) of the CoDEC-ERA5 dataset and the GTSR dataset.
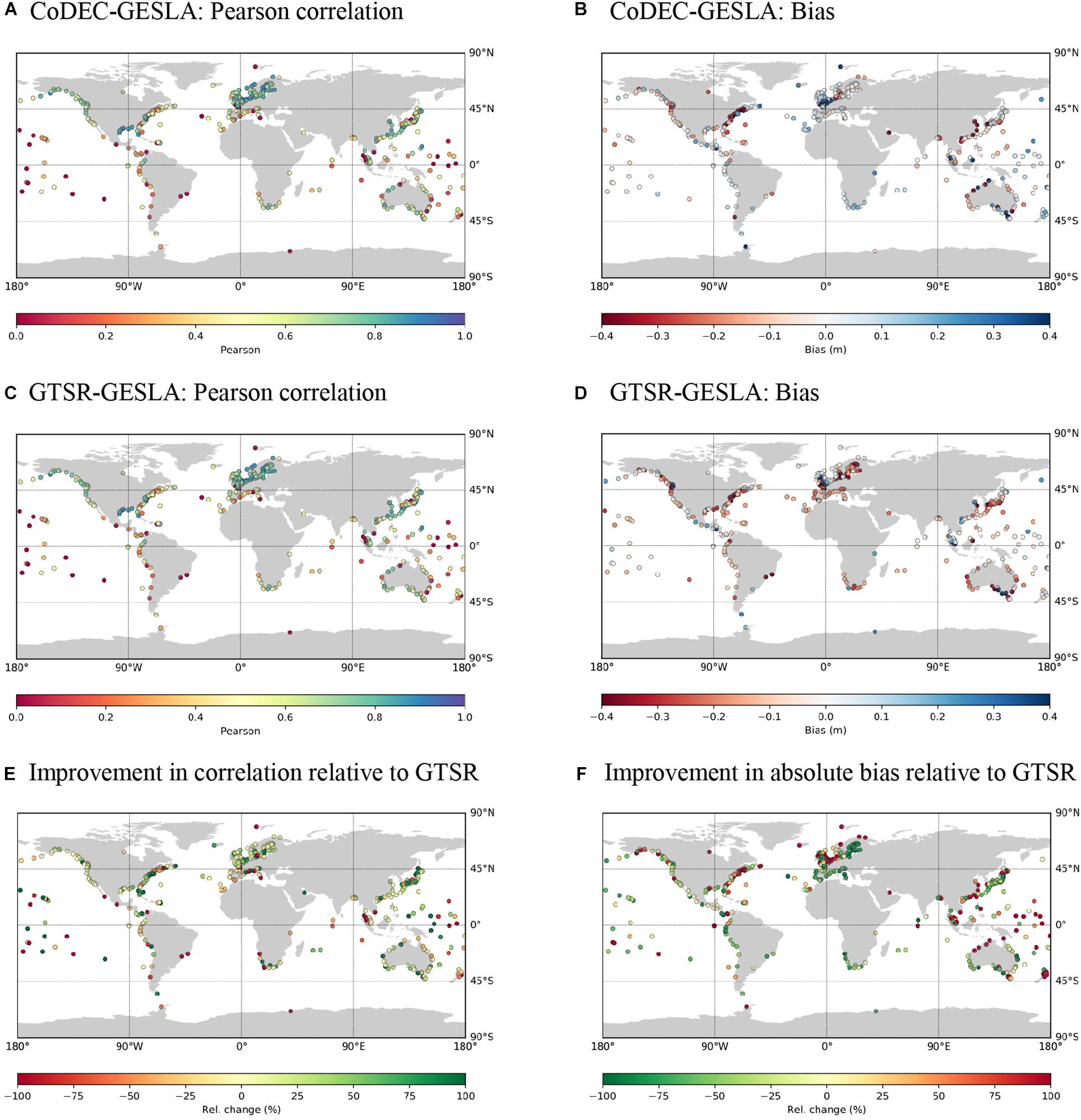
Figure 2. Model performance of the modeled annual maxima evaluated against observed annual maxima from the GESLA dataset. We show Pearson’s correlation coefficient of the annual maxima for (A) CoDEC-ERA5 and (C) GTSR, as well as (E) the relative changes in correlation coefficients between the two datasets. In addition, we show the mean bias over the annual maxima for (B) CoDEC-ERA5 and (D) GTSR, as well as (F) the relative changes in the mean bias between the two datasets.
There is a clear improvement when comparing the performance of CoDEC-ERA5 against GTSR (see Figures 2B,D). The correlation coefficients increase for 90% of the tide gauge stations (yellow to green dots in Figure 2E), while the absolute biases reduce for 95% of the tide gauge stations (yellow to green dots in Figure 2F). With an MB of -0.04 m for CoDEC-ERA5 and -0.08 m for GTSR, the errors across all the tide gauge stations are reduced by 50% (Table 3). At the same time, there are also regions where the model performance worsens. It appears that biases increase in regions with a high tidal range, such as the North Sea, northern Australia, and the northwest of the United States and Canada, which could indicate that GTSM is outperformed by the FES2012 model that was used to develop the GTSR dataset. There was no clear spatial pattern for the correlation coefficients, although they appear to mainly decrease in tropical regions. Figure 3 presents scatter density plots for the modeled and observed annual maxima for all the GESLA tide gauge stations combined. For water levels larger than 6 m, CoDEC-ERA5 tends to overestimate the water levels, while GTSR shows an underestimation. This is probably caused by the higher resolution of the meteorological forcing, which resolves extreme storms better. Overall, the comparison of the performance of CoDEC-ERA5 and GTSR demonstrates a significant improvement due to model development and improved forcing.
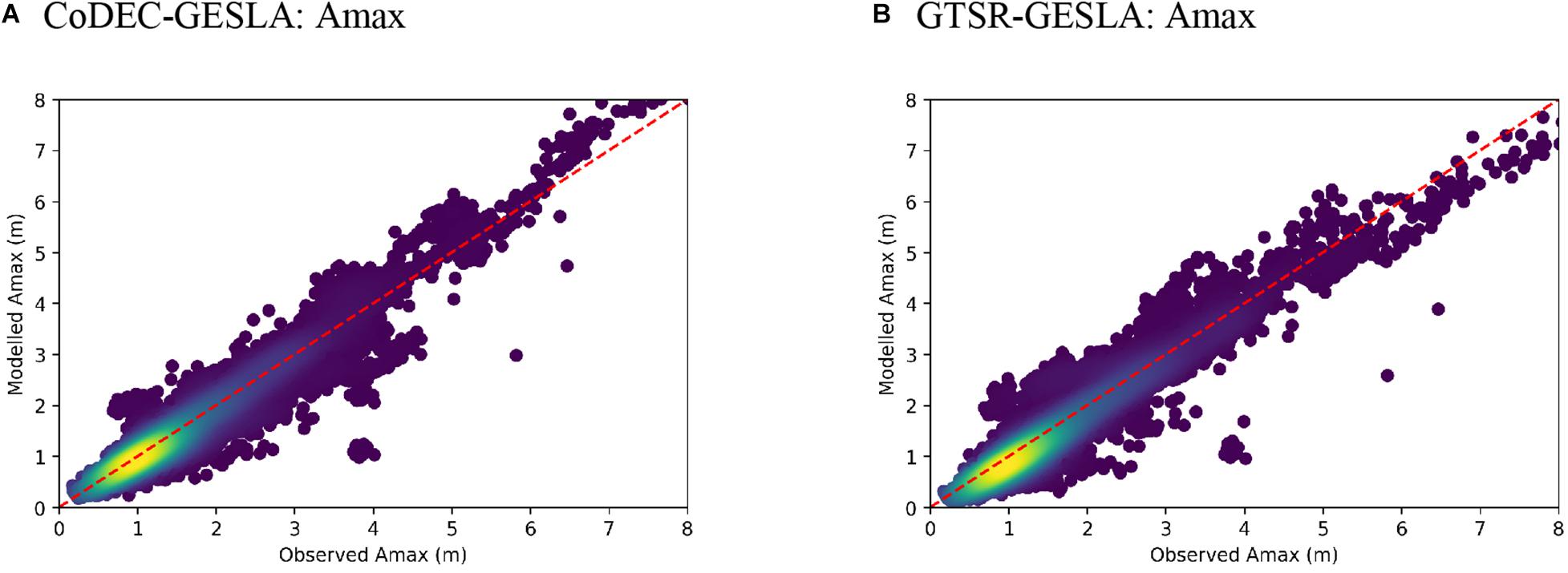
Figure 3. Scatter density plots of the modeled and observed annual maxima (Amax) for the GESLA dataset for (A) the CoDEC-ERA5 dataset, and (B) the GTSR dataset. The data for all the tide gauge stations were combined. The red dotted line indicates the perfect fit line.
Evaluation of CoDEC-ERA5 on the Basis of Return Periods
Figure 4A maps the 1 in 10-year water levels. When averaged across all the tide gauge stations, the modeled 1 in 10-year water levels for CoDEC-ERA5 are underestimated by -0.10 m (SD is 0.32), while the MAPE was 12.1% (SD 12.8) (Table 4). Overall, the absolute biases were lower than 0.3 m for 75% of the tide gauge stations. The relative errors were within -30% and + 15% for 75% of the tide gauge stations. Due to the poor global coverage of the tide gauge stations, it is difficult to identify a spatial pattern in the model performance (Figure 4B). But in general, there is a slight overestimation along the European coastline and an underestimation elsewhere. The difference in model performance in Europe could be linked to the fact that the GTSM resolution is more refined there, as well as the use of high-resolution bathymetry. In some regions, such as northern Australia and the northeastern United States, the biases are relatively large. These regions typically experience large sea level extremes due to a large tidal range and the occurrence of tropical cyclones.
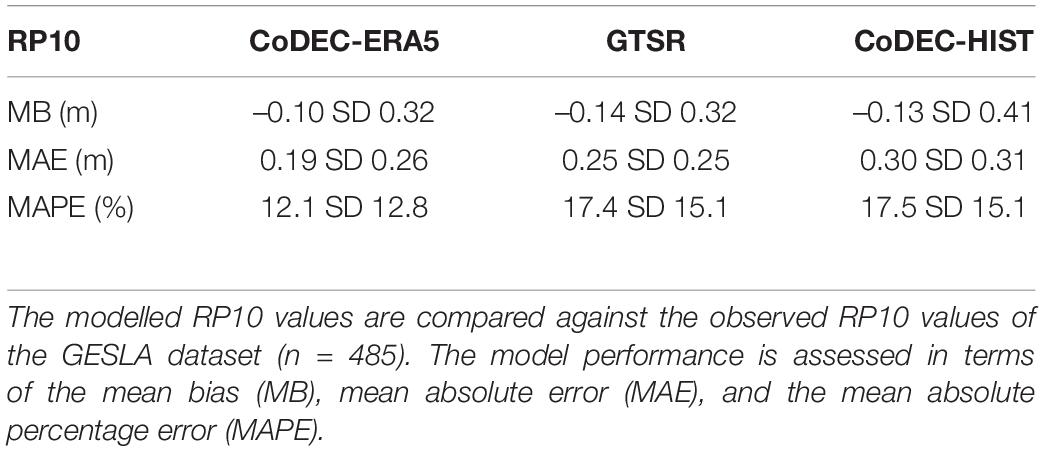
Table 4. Model performance of the 1 in 10-year water levels (RP10) of the CoDEC-ERA, GTSR, and CoDEC-HIST dataset.
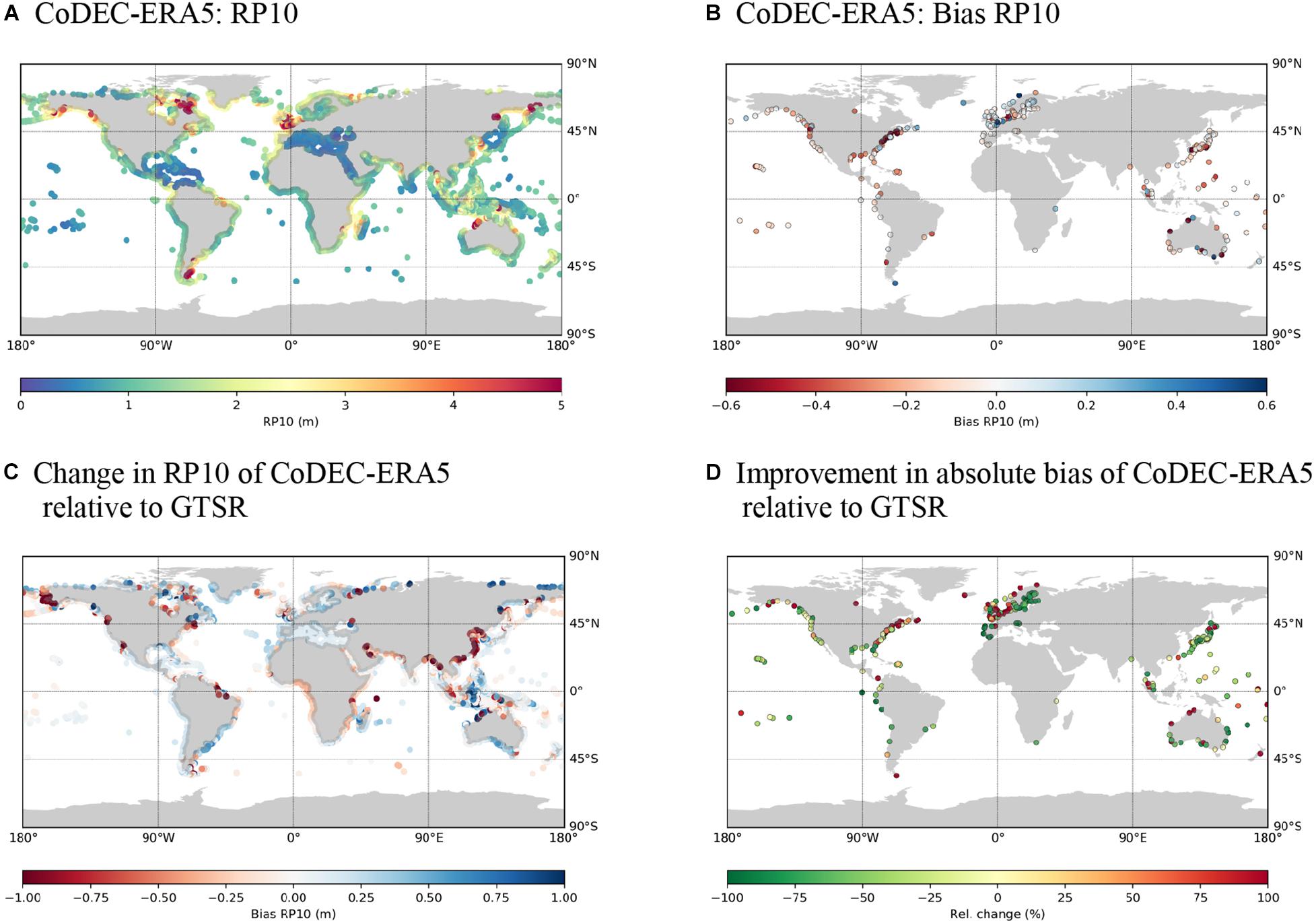
Figure 4. Modeled water levels with (A) 1 in 10-year return periods (RP10) for (A) the CoDEC-ERA5 dataset, as well as (B) the bias of the 1 in 10-year water levels compared against observations from the GESLA dataset, (C) difference between the 1 in 10-year water levels derived from the CoDEC-ERA5 dataset and the GTSR dataset, and (D) Improvement in absolute bias of CoDEC-ERA5.
There is a clear improvement in performance of the 1 in 10-year water levels from CoDEC-ERA5 to GTSR. Table 4 indicates that the average MAE drops by 25%, from 0.25 m for GTSR to 0.19 m for CoDEC-ERA5, while the MAPE is reduced by 29%. For CoDEC-ERA5, 60% of the tide gauge stations have an MAE smaller than 0.2 m for CoDEC-ERA5, in comparison to 40% for GTSR. Figure 4C indicates that the largest changes between the two datasets are up to 1 m. This is rather high, and could be partly caused by the correction of the vertical datum by the MSLP, which has a magnitude up to 0.2 m. Moreover, the use of another tidal model could explain the large differences. In general, the 1 in 10-year water levels for CoDEC-ERA5 are higher than those for GTSR (blue points in Figure 4C). This could be linked to the higher resolution of CoDEC-ERA5 (i.e., model resolution as well as the resolution of the meteorological forcing), which leads to an improved accuracy of the most extreme water levels. However, there are also regions where the 1 in 10-year water levels in GTSR are higher those in CoDEC-ERA5 (red points in Figure 4C). For 66% of the tide gauge stations, the MAE was reduced when comparing CoDEC-ERA5 against GTSR (Figure 4D).
To illustrate the performance of the individual locations, Figure 5 shows the ranked annual maxima and the fitted Gumbel distributions for four selected tide gauge stations. It shows a general good fit of the Gumbel distribution to the annual maxima. The plots also illustrate that for some locations, such as Aberdeen, the difference in performance between GTSR and CoDEC-ERA5 is negligible, while for other locations, such as Brisbane, the improvement in model performance is relatively large.
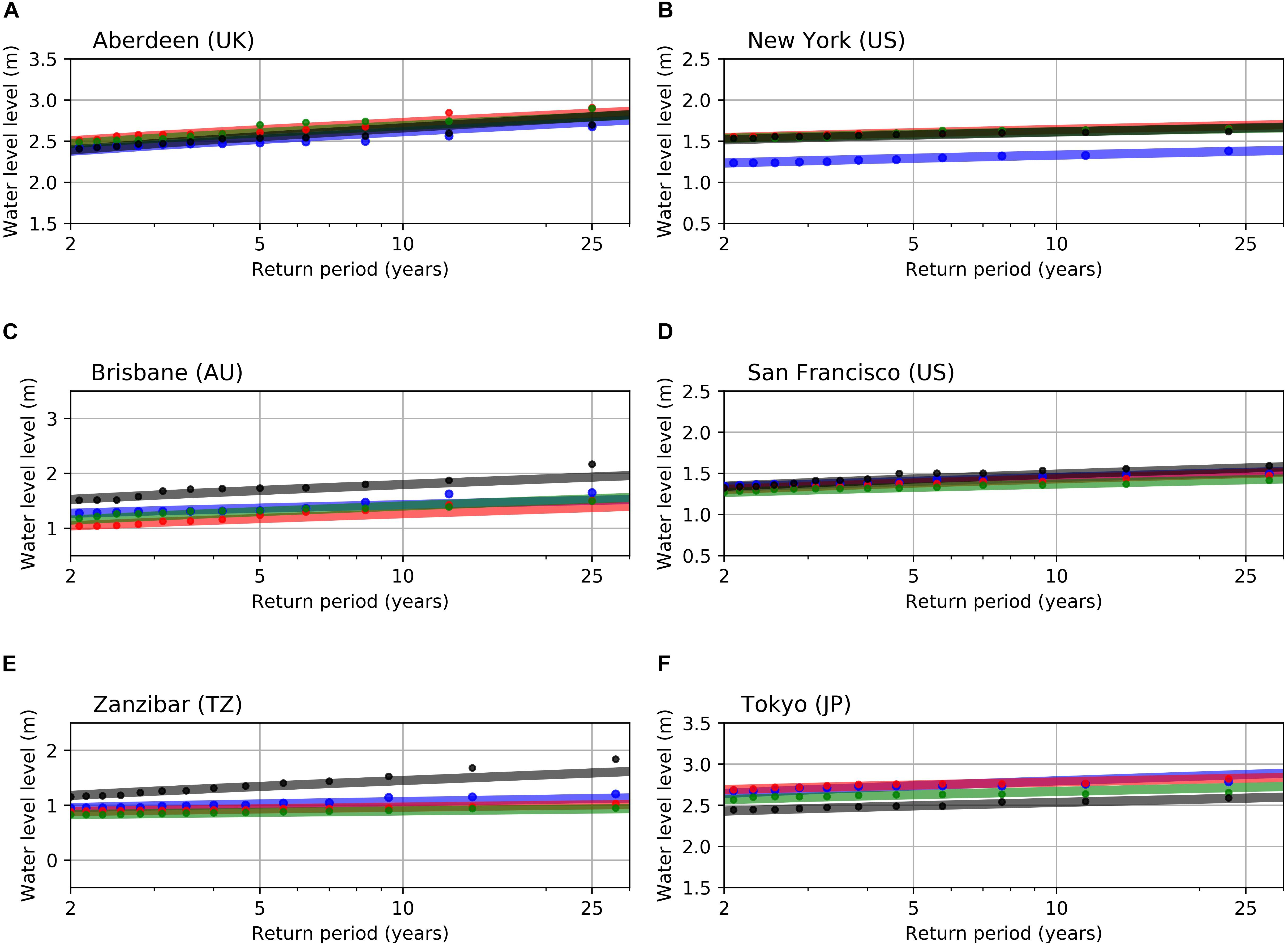
Figure 5. Plots showing the Gumbel extreme value distribution fitted to the modeled and observed annual maxima at selected stations. The selected stations are (A) Aberdeen (UK), (B) New York, United States, (C) Brisbane, Australia, (D) San Fransisco, United States, (E) Zanzibar, Tanzania, and (F) Tokyo, Japan. The black line indicates the observed maxima, whereas GTSR, CoDEC-ERA5, and CoDEC-HIST are plotted as a blue, red, and green line, respectively. We also plot the empirical probabilities of the annual maxima (dots) based on the Weibull plotting positions.
Evaluation of CoDEC-HIST to Assess Spatial Bias of the Climate Model
Before analyzing how extreme sea levels may change under future climate change scenarios, we evaluate how the performance of CoDEC-HIST return periods. The validation of the 1 in 10-year water levels shows an MB of -0.13 m, a mean MAE of 0.30 m, and a MAPE of 17.5% across all the tide gauge stations (Table 4). Mapping the bias of the 1 in 10-year water levels shows that they are generally overestimated in Europe and underestimated elsewhere (Figure 6A). The poor global coverage of the tide gauge stations makes it difficult to assess such a spatial bias. However, comparison of the 1 in 10-year water levels against those derived from the CoDEC-ERA5 simulations confirms the spatial bias (Figure 6A). While the bias compared to tide gauge stations is affected by the performance of GTSM, the bias between CoDEC-HIST and CoDEC-ERA5 is only affected by the difference in meteorological forcing. The bias between CoDEC-HIST and CoDEC-ERA5 is generally less than 0.2 m. Regions that are prone to tropical cyclones, such as Mozambique and the east coast of the United States, show a larger than average difference (>0.3 m). This appears to be an effect of the higher spatial resolution of the ERA5 forcing over the CMIP5 forcing (i.e., 0.5° × 0.5°, 3 hourly vs. 0.25° × 0.25°, hourly). In general, the 1 in 10-year water levels from CoDEC-HIST are lower than those from CoDEC-ERA5 (red points in Figure 6B). Some regions, including Europe (the Baltic Sea, the North Sea, and the Mediterranean Sea) and Alaska (Bering Sea), the 1 in 10-year water levels are higher in CoDEC-HIST (blue points in Figure 6B). The overestimation in Europe could be linked to the downscaling of EC-EARTH by the regional climate model DMI-HIRHAM that we applied for forcing for Europe. This regional model has a higher resolution than ERA5, and wind speeds will generally increase with a higher model resolution (Pryor et al., 2012). Moreover, the surface extreme wind fields in HIRHAM (25 and 50 km resolutions, downscaled from the reanalysis data) were evaluated by Donat et al. (2010), who concluded that HIRHAM simulated higher wind speed than the ERA-40 reanalysis data. The HIRHAM simulations (12 km resolution) used in this project have the same tendency (Yan et al., 2019). In general, the CMIP5 simulations are capable of capturing the spatial variability of the annual maximum wind speeds, while the historical temporal trends in annual maximum wind speeds are not well represented (Kumar et al., 2015). Since our focus is primarily on the average statistics over the entire period, this should not affect performance.
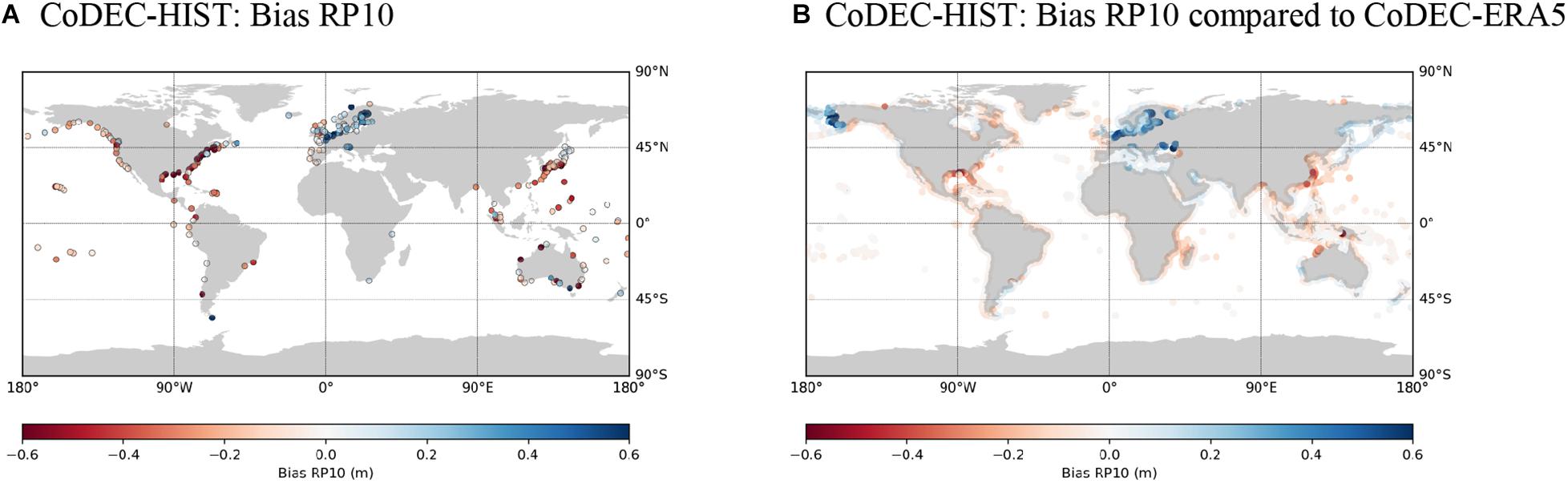
Figure 6. Model performance of 1 in 10-year water levels for the CoDEC-HIST simulation shows as (A) the bias of the 1 in 10-year water levels compared against observations from the GESLA dataset and (B) the difference between the 1 in 10-year return periods derived from CoDEC-HIST and CoDEC-ERA5.
The overall performance of CoDEC-HIST is lower than the performance of CoDEC-ERA5. CoDEC-HIST tends to overestimate extreme sea levels in Europe. However, the differences in performance are rather small, and we conclude that CoDEC-HIST is sufficiently accurate to support large-scale assessments when used as baseline and for comparison against simulations for future climate scenarios with the same climate model.
Changes in 1 in 10-Year Water Levels in Response to Climate Change
Figure 7 maps the change in the 1 in 10-year water levels for the mid-century on the basis of CoDEC-RCP8.5 for the mid-century (2041–2070) and on the basis for CoDEC-RCP4.5 (2070–2100) for the end of the century, using CoDEC-HIST as a baseline. The average change across all output locations is 0.25 m (SD 0.15) and 0.34 m (SD 0.23) for CoDEC-RCP8.5 and CoDEC-RCP4.5, respectively. These relatively high SDs indicate a strong spatial heterogeneity. The most remarkable are the sea-level changes along the coastlines of the Baltic Sea and Hudson Bay, which experience sea-level fall rather than SLR due to the glacio-isostatic adjustment of land. When removing SLR, the average change across all output locations becomes negligible for both CoDEC-RCP8.5 and CoDEC-RCP4.5. It shows the change in 1 in 10-year water levels is largely driven by SLR. There are a few outliers that show large changes (the red dots in Figures 7C,D), which are probably due to spurious output when a grid cell falls dry (SLR may change when that occurs), leading to large changes in comparison to the baseline. For both RCPs, only 5% of the output locations show a change larger than 0.1 m when the SLR signal is removed. Figures 7C,D show some spatial coherency regarding the projected change in the 1 in 10-year water levels. However, Figure 8 indicates that aggregation of the changes at continental scales does not indicate significant changes in climate extremes. Europe shows the largest spread in changes (both negative and positive). For example, there are places along the Atlantic coasts of Spain, France, and Great Britain where the CoDEC projections indicate an increase in 1 in 10-year water levels of up to 0.2 m, in addition to SLR. This requires further analysis, but could possibly be linked to the northward movement of tropical cyclone tracks in the Atlantic (Haarsma et al., 2013). By contrast, the CoDEC projections indicate a decrease of the 1 in 10-year water levels near Denmark. It should be noted that changes in water level without SLR include both a change in storm surges and interaction effects in response to SLR. The tidal propagation and tide–surge interaction may respond to changes in water depth due to SLR (Arns et al., 2017; Haigh et al., 2019). This can also lead to changes in extreme sea levels, in addition to climatic changes.
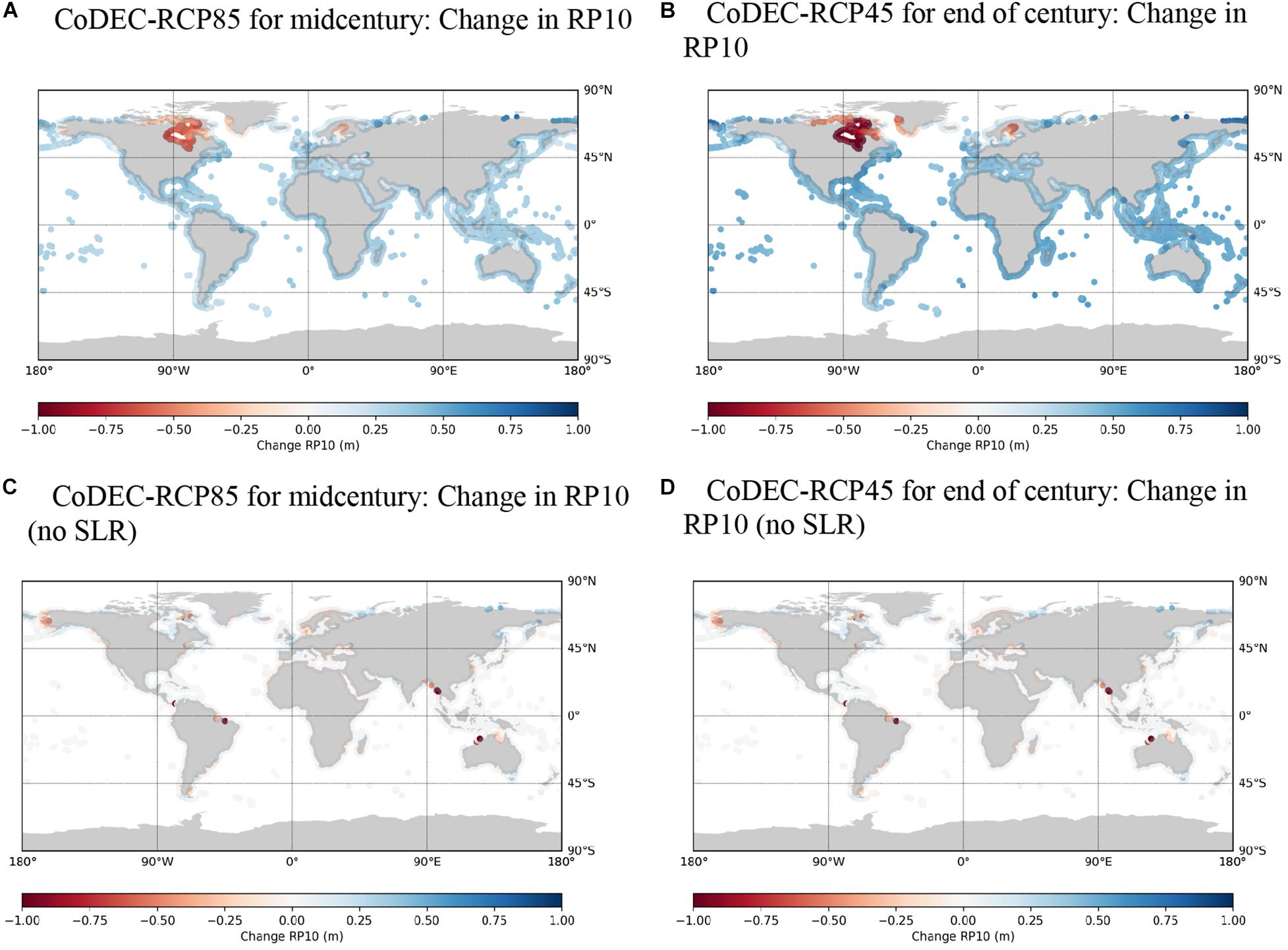
Figure 7. Changes in the 1 in 10-year water levels (RP10) for (A) mid-century (2041–2070 with SLR of 2055) based on CoDEC-RCP8.5 compared to CoDEC-HIST and for (B) the end of the century (2071–2100 with SLR of 2085) based on CoDEC-RCP4.5 compared to CoDEC-HIST. We also show the change when SLR is not taken into account (C,D). Note the different color scales.
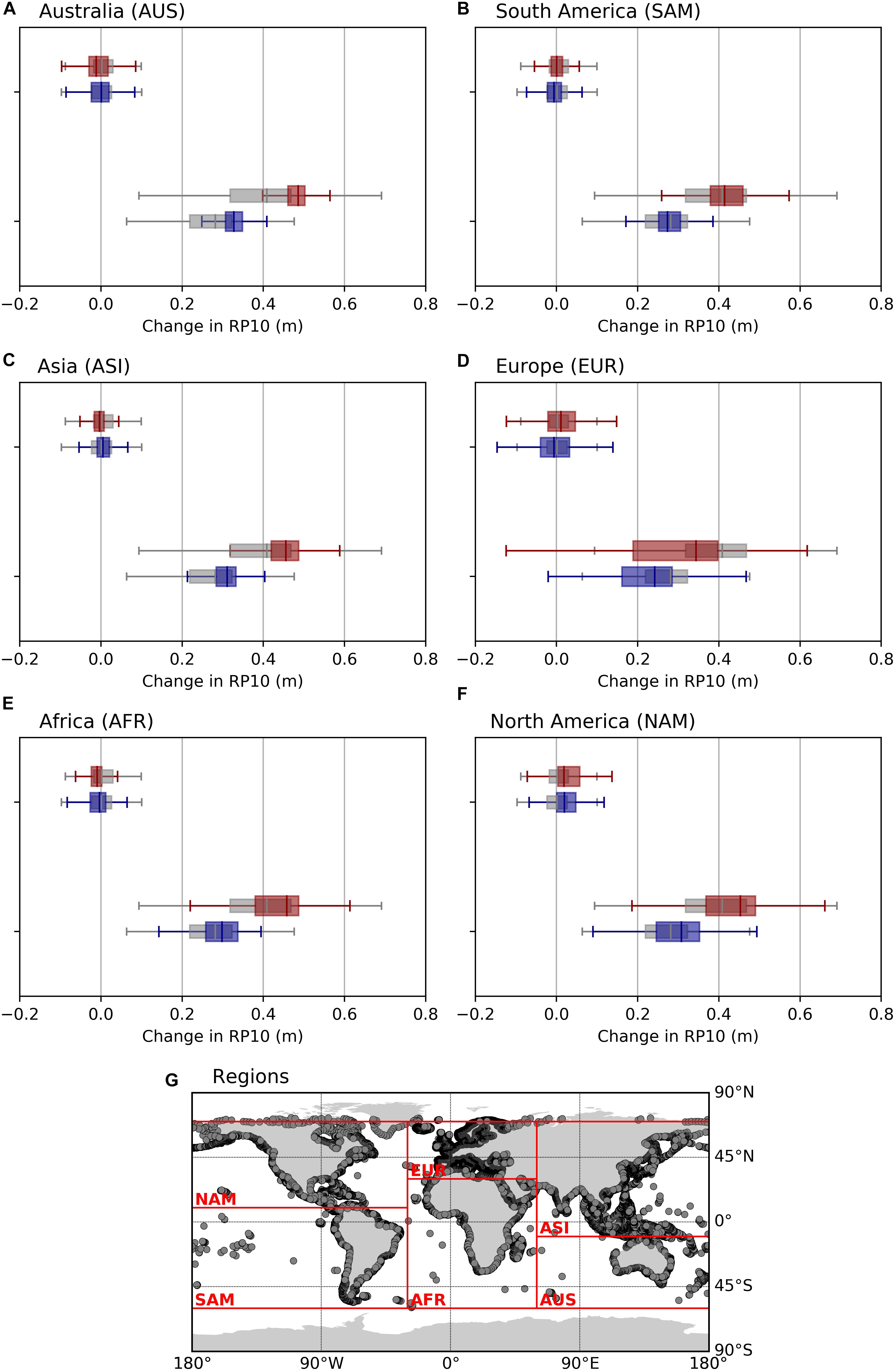
Figure 8. Changes in the 1 in 10-year water levels (RP10) for different geographical regions, which are indicated in panel G. The regions shown are (A) Australia, (B) South America, (C) Asia, (D) Europe, (E) Africa, and (F) North America. We show the changes for the mid-century (2041–2070 with SLR of 2055) based on CoDEC-RCP8.5 compared to CoDEC-HIST (blue bars) and for the end of the century (2071–2100 with SLR of 2085) based on CoDEC-RCP4.5 compared to CoDEC-HIST (red bars). The gray bars indicate the global average. The box plots extend from the lower to upper quartile values of the data, with a line at the median. The whiskers indicate the 5th–95th percentiles.
On a global scale, our findings are largely in agreement with Vousdoukas et al. (2018b), who also concluded that changes in extreme sea levels are mainly driven by SLR. In contrast to our findings, they reported a very weak increasing trend for the projected global average of changes in storm surge in combination with wind-waves. While Vousdoukas et al. (2018b) did not differentiate between the contribution of wind-waves and storm surges to the water level change, wind-waves are probably more sensitive to climatic changes than storm surges. Similarly to our findings, Vousdoukas et al. (2018b) found strong spatial heterogeneity regarding the sign of water levels changes (increase vs. decrease) that tend to cancel each other when averaged over larger areas. When comparing our results to regional studies, the water level changes that exclude SLR appear to differ between studies. Like Colberg et al. (2019), who investigated extreme sea levels around Australia, we found an increase of near Tasmania and a decrease for most of the southern coastline, but our results for the rest of the coastline are in contrast to theirs. Also in Europe there are many places where our results differ from Vousdoukas et al. (2016). The potential reasons for the discrepancies between different studies are wide-ranging. They could be due to the different climate models used, which show different climate signals. At the local scale, the differences could also be due to interaction effects, which are explicitly included in the CoDEC simulations (see section “Future Research Directions”).
Use of the Data for Assessing Climate Impact at Local Scale
As part of the project, the usability of the CoDEC dataset to assess climate impact for specific sectors was showcased by five use cases covering different parts of the European coastline. They considered a variety of coastal sectors and issues, such as flooding due to severe storm surge and wave overtopping, industrial sectors such as offshore wind, harbors, and ports, as well as coastal erosion and dune safety. One of these five use cases was on flood risk in Copenhagen, Denmark. Copenhagen is located on a connecting strait between the North Sea and the Baltic Sea in Northern Europe. While the strait is relatively sheltered during average weather conditions, the city has experienced storm surges. The historical city center is centered on the harbor, and the urban and industrial areas are located on the shoreline and include important infrastructure. The risk of flooding from the sea, especially due to storm surges, threatens economic assets, and this risk is projected to greatly increase with climate change (Hallegatte et al., 2011).
Therefore, climate adaptation is a focus point for the municipalities in the area, and a solid knowledge basis regarding coastal climate change is key to proper adaptation planning. European and global climate information services, such as the C3S portal, provide input for local use cases and national information regarding future climate change at the municipality level. Information has recently been collected into a Danish “Climate Atlas”1, which contains information on temperature, precipitation, sea level, and storm surges.
To determine the needs for coastal climate change information, representatives of the expert technical staff of five municipalities in the Copenhagen area, together with the Danish Coastal Authority, were interviewed (Madsen et al., 2019). These experts identified information on changes in sea level, storm surges, and wave conditions as key needs. The idea of a “gate index” emerged during the interviews, which would provide information on the necessary number of closures of a potential new storm surge gate. Five indicators were formulated on the basis of the interviews (Table 5). The indicators were computed by downscaling the CoDEC simulations of MSL, together with regional simulations of waves and ocean current variability, using the WAM Cycle 4.5 wave model and the HBM-DKSS2013 3D ocean model, both of which are nested to have an approximately 1 km resolution in the study area (Günther et al., 1992; Berg and Poulsen, 2012). Wave simulations for the case study were only performed for the RCP8.5 scenario (Table 5).
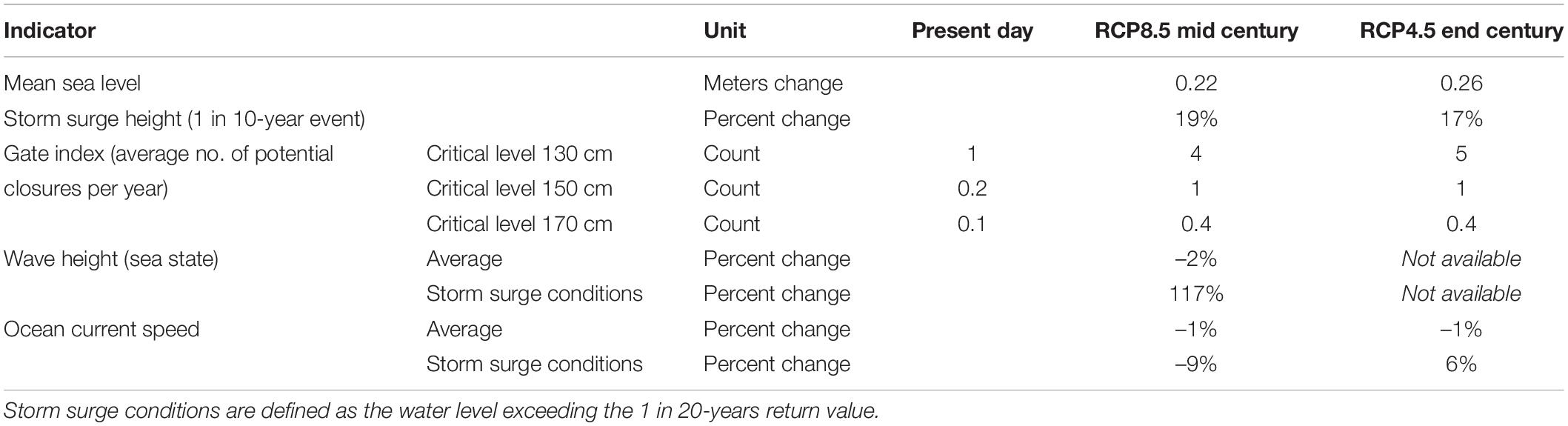
Table 5. Climate change indicators for the Copenhagen use case for the RCP8.5 mid-century (2041–2070) and RCP 4.5 end-century (2071–2100) periods relative to the present day (1977–2005).
Table 5 provides an overview of the results for the indicators. For MSL changes, the simulations show comparable values for the two RCPs. This indicates that, if the RCP8.5 scenario is followed, the study area will experience approximately the same sea level rise in the mid-century as it will for RCP4.5 at the end of the century. It should be noted that under RCP8.5, there is a continued acceleration of SLR, which will cause greater sea-level changes toward the end of the century and beyond. The changes in storm surge heights reflect the sea-level changes and indicate a risk of changes in the wind causing additional contributions to storm surges. However, further studies are needed, as changes in extreme wind in this region are rather uncertain. The gate index largely depends on the critical sea level to determine where the gate will be closed. Both RCPs show an increased number of closures with future SLR. Closures of potential gates will be required four to five times more frequently with a sea-level change in the range of 20–30 cm. The indicators on sea level and storm surge have been further detailed and derived for all the coastal stretches of Denmark in the Danish Climate Atlas.
Conclusion
Aiming to contribute to the accurate global mapping of the impact of climate change on coastal regions, we have presented a novel global dataset of extreme sea levels, the CoDEC. This dataset is based on the next generation GTSMv3.0, which has a coastal resolution of 2.5 km (1.25 km in Europe). We have provided extreme sea levels for the period 1979–2017 that are based on the ERA5 climate reanalysis (CoDEC-ERA5), together with future climate projections from 2040 to 2100 (CoDEC-RCP4.5 and RCP8.5) and a baseline simulation (CoDEC-HIST). The validation of CoDEC-ERA5 against observed sea levels demonstrates a good performance. The annual maxima have a global MB of -0.04 m, which is 50% lower than the MB of the previous GTSR dataset. The validation of CoDEC-HIST shows a spatial bias, with higher sea levels in Europe and Alaska and lower sea levels elsewhere, but the overall performance is comparable, although slightly worse than CoDEC-ERA5. The average change in 1 in 10-year water levels across all the output locations was 0.25 and 0.34 m for CoDEC-RCP8.5 and CoDEC-RCP4.5, respectively. This change was largely driven by SLR, although at certain locations the change in water level was amplified by changes in climatic extremes, or by changes in the interaction effects in response to SLR.
The CoDEC dataset can be used for climate impact studies. CoDEC-ERA5 could, for example, be used to assess present-day flood damage, and to investigate the different aspects of coastal flooding, including climate variability and trends, or the cultural heritage and global infrastructure that is threatened by flooding (e.g., Muis et al., 2018; Reimann et al., 2018; Koks et al., 2019). The future projections can be used to assess how coastal flooding may change in response to climate change. In addition to the large-scale analysis of extreme sea levels, we have demonstrated how the CoDEC dataset can contribute to the understanding of climate impact on the local scale by presenting a use case for the city of Copenhagen. In comparison to the previous GTSR dataset, a promising innovation is that, in addition to the coastal points, the CoDEC dataset includes output on a regular grid in the ocean. We envisage that this way the CoDEC dataset can provide boundary conditions for regional models, which will facilitate dynamic downscaling from the global scale to the local scale.
Future Research Directions
In addition to the use of the CoDEC dataset for climate impact studies, there are various directions for future research. For example, it would be interesting to extend the evaluation of the model’s performance. This could be done by also including a comparison with previous studies, both in Europe and worldwide (Cid et al., 2014; Vousdoukas et al., 2016; Paprotny and Terefenko, 2017; Fernández-Montblanc et al., 2019). Moreover, satellite altimetry could be used to evaluate the model’s performance in areas where tide gauge data are unavailable (Cid et al., 2014). We have presented a first analysis of the sea-level changes in the CoDEC dataset based on the 1 in 10-year water levels, but this could be explored further. Decomposing the total water level to tides and storm surges would allow further investigation of the mechanisms driving the sea-level changes, and whether the interaction effects are important to include in large-scale assessments. Moreover, future research could quantify how the sea-level changes affect return periods (Vitousek et al., 2017; Frederikse et al., 2020). This could include an estimation of the uncertainty in the return periods due to fitting the extreme value distribution. In addition, recent studies have shown that fitting a Gumbel distribution to the annual maxima may lead to an overestimation of extreme sea levels, especially with lower probabilities (Buchanan et al., 2017; Wahl et al., 2017). Furthermore, the application of non-stationary approaches has several advantages in comparison to the stationary approaches used in this study (e.g., Menéndez and Woodworth, 2010; Mentaschi et al., 2016), which is worth exploring. A limitation of the current approach is that the return levels in regions prone to tropical cyclones may be underestimated. Tropical cyclones are relatively rare and generally affect a small stretch of coast. As a result, the length of the simulations is insufficient to estimate the return periods of the most extreme events. Studies have therefore relied on statistical methods to extend the historical period of several decades to thousands of years and to generate databases with synthetic tropical cyclones (Emanuel et al., 2006). Until now, studies that analyzed extreme sea levels based on synthetic tropical cyclones have been carried out on the continental to regional scale (Lin et al., 2012; Haigh et al., 2013; Marsooli et al., 2019). In the future, however, we aim to force GTSMv3.0 with a global dataset comprised of synthetic tropical cyclone tracks (Bloemendaal et al., 2020) and to develop reliable probabilities of storm surge generated by tropical cyclones. Users of the CoDEC dataset should be aware that the future projections are based on one climate model of the CMIP5 multi-model ensemble and on the CMIP5 ensemble MSL change. Previous studies have shown that future projections of extreme sea levels can have wide uncertainties, with a large spread between model ensemble members (Vousdoukas et al., 2018b; Colberg et al., 2019). The discrepancies between future projections from different studies highlight these uncertainties, and we cannot estimate the full uncertainties related to the future projections on the basis of one climate model. We have used a physically based approach to model the tides, surges, and changes in MSL, thereby dynamically including any interactions between them. Such a dynamic approach comes with large computational costs, and it was not feasible to run more than one model from the CMIP5 multi-model ensemble within the scope of the present study. However, having now developed the entire modeling framework, we see opportunities for addressing this limitation in the future.
Data Availability Statement
The Gumbel parameters and the various return periods for the global coastline are available at the Zenodo repository with identifier 10.5281/zenodo.3660927. Time series, including storm surges, tides, and total water levels, together with the climate change indicators for Europe, will become available at the Climate Data Store (CDS) of the Copernicus Climate Change Service (C3S) at the following url: https://cds.climate.copernicus.eu/.
Author Contributions
MA and SM were responsible for conducting the model runs and the post-processing. KY led the project and wrote the proposal together with MA and MV. SM wrote the manuscript, with contributions from KY and input from KM and JS for the Copenhagen case study. All the authors were involved in the writing of the manuscript, and discussed the results and the implications.
Conflict of Interest
The authors declare that the research was conducted in the absence of any commercial or financial relationships that could be construed as a potential conflict of interest.
The reviewer MV declared a past co-authorship with one of the authors MV to the handling Editor.
Funding
The research leading to these results was funded by Contract C3S-422-Lot2-Deltares European Services of the Copernicus Climate Change Service (11200665-003). Additional funding was received from the Deltares Strategic Research Program, Grant No. 11204672 and Grant No. l1000210-012). SM received additional funding from the MOSAIC research program (Grant No. ASDI.2018.036), which is financed by the Dutch Research Council (NWO). The Copernicus Climate Change Service (C3S) is funded by the European Union and aims to:
• Become an authoritative source of climate information for Europe.
• Build upon national investments and complement national climate service providers.
• Support the market for climate services in Europe.
• Provide free public access to climate change information.
Acknowledgments
The authors would like to thank Thomas Wahl and Marta Marcos for providing the observed annual maxima from the GESLA dataset.
Footnotes
References
Arns, A., Dangendorf, S., Jensen, J., Talke, S., Bender, J., and Pattiaratchi, C. (2017). Sea-level rise induced amplification of coastal protection design heights. Sci. Rep. 7:40171. doi: 10.1038/srep40171
Belmonte Rivas, M., and Stoffelen, A. (2019). Characterizing ERA-interim and ERA5 surface wind biases using ASCAT. Ocean Sci. Discuss. 15, 831–852. doi: 10.5194/os-2018-160
Berg, P., and Poulsen, W. J. (2012). Implementation details for HBM. DMI Technical Report No. 12-11. Copenhagen: Danish Meteorological Institute, 149.
Bloemendaal, N., Haigh, I. D., de Moel, H., Muis, S., Haarsma, R. J., and Aerts, J. C. J. H. (2020). Generation of a global synthetic tropical cyclone hazard dataset using STORM. Sci. Data 7:40. doi: 10.1038/s41597-020-0381-2
Bloemendaal, N., Muis, S., Haarsma, R. J., Verlaan, M., Apecechea, M. I., de Moel, H., et al. (2019). Global modeling of tropical cyclone storm surges using high-resolution forecasts. Clim. Dyn. 52, 5031–5044. doi: 10.1007/s00382-018-4430-x
Bloemendaal, N., Muis, S., Haarsma, R., Apechchea, M. I., Verlaan, M., Ward, P. J., et al. (2017). “Horizontal resolution effects on tropical cyclone storm surges,” in Proceedings of the 6th Summit on Hurricanes and Climate Change: from Hazard to Impact, Heraklion.
Bouwer, L. M. (2018). Next-generation coastal risk models. Nat. Clim. Chang. 10:2010. doi: 10.1038/s41558-018-0262-2
Brown, S., Nicholls, R. J., Goodwin, P., Haigh, I. D., Lincke, D., Vafeidis, A. T., et al. (2018). Quantifying land and people exposed to sea-level rise with no mitigation and 1.5°C and 2.0°C rise in global temperatures to year 2300. Earths Future 6, 583–600. doi: 10.1002/2017EF000738
Buchanan, M. K., Oppenheimer, M., and Kopp, R. E. (2017). Amplification of flood frequencies with local sea level rise and emerging flood regimes. Environ. Res. Lett. 12:064009. doi: 10.1088/1748-9326/aa6cb3
Charnock, H. (1955). Wind stress on a water surface. Q. J. R. Meteorol. Soc. 81, 639–640. doi: 10.1002/qj.49708135027
Christensen, O. B., Drews, M., Christensen, J. H., Dethloff, K., Ketelsen, K., Hebestadt, I., et al. (2007). The HIRHAM Regional Climate Model Version 5 (beta). Technical Report No. 06-17. Copenhagen: Danish Meteorological Institute.
Church, J. A., Clark, P. U., Cazenave, A., Gregory, J. M., Jevrejeva, S., Levermann, A., et al. (2013). “Sea level change,” in Climate Change 2013: The Physical Science Basis. Contribution of Working Group I to the Fifth Assessment Report of the Intergovernmental Panel on Climate Change, eds T. F. Stocker, D. Qin, G.-K. Plattner, M. Tignor, S. K. Allen, J. Boschu, et al. (Cambridge: Cambridge University Press), doi: 10.1017/CB09781107415315.026
Cid, A., Castanedo, S., Abascal, A. J., Menéndez, M., and Medina, R. (2014). A high resolution hindcast of the meteorological sea level component for Southern Europe: the GOS dataset. Clim. Dyn. 43, 2167–2184. doi: 10.1007/s00382-013-2041-0
Colberg, F., McInnes, K. L., O’Grady, J., and Hoeke, R. (2019). Atmospheric circulation changes and their impact on extreme sea levels around Australia. Nat. Hazards Earth Syst. Sci. 19, 1067–1086. doi: 10.5194/nhess-19-1067-2019
Consortium EMODnet Bathymetry (2018). EMODnet Digital Bathymetry (DTM). Available online at: https://sextant.ifremer.fr/record/18ff0d48-b203-4a65-94a9-5fd8b0ec35f6/ (accessed March, 2018).
Copernicus Climate Change Service [C3S] (2017). ERA5: Fifth Generation of ECMWF Atmospheric Reanalyses of the Global Climate. Available online at: https://cds.climate.copernicus.eu/cdsapp#!/home (accessed July, 2018).
de Lima Rego, J., and Li, C. (2010). Nonlinear terms in storm surge predictions: effect of tide and shelf geometry with case study from Hurricane Rita. J. Geophys. Res. 115:C06020. doi: 10.1029/2009JC005285
Donat, M. G., Leckebusch, G. C., Wild, S., and Ulbrich, U. (2010). Benefits and limitations of regional multi-model ensembles for storm loss estimations. Clim. Res. 44, 211–225. doi: 10.3354/cr00891
Dullaart, J. C. M., Muis, S., Bloemendaal, N., and Aerts, J. C. J. H. (2019). Advancing global storm surge modelling using the new ERA5 climate reanalysis. Clim. Dyn. 54, 1007–1021. doi: 10.1007/s00382-019-05044-0
Emanuel, K. A., Ravela, S., Vivant, E., and Risi, C. (2006). A statistical deterministic approach to hurricane risk assessment. Bull. Am. Meteorol. Soc. 87, 299–314. doi: 10.1175/BAMS-87-3-299
Fernández-Montblanc, T., Vousdoukas, M. I., Ciavola, P., Voukouvalas, E., Mentaschi, L., Breyiannis, G., et al. (2019). Towards robust pan-European storm surge forecasting. Ocean Model. 133, 129–144. doi: 10.1016/j.ocemod.2018.12.001
Frederikse, T., Buchanan, M. K., Lambert, E., Kopp, R. E., Oppenheimer, M., Rasmussen, D. J., et al. (2020). Antarctic Ice Sheet and emission scenario controls on 21st-century extreme sea-level changes. Nat. Commun. 11:390. doi: 10.1038/s41467-019-140496
Fretwell, P., Pritchard, H. D., Vaughan, D. G., Bamber, J. L., Barrand, N. E., Bell, R., et al. (2013). Bedmap2: improved ice bed, surface and thickness datasets for Antarctica. Cryosphere 7, 375–393. doi: 10.5194/tc-7-375-2013
GEBCO (2014). General Bathymetric Chart of the Oceans (GEBCO) 2014 Grid. Available online at: https://www.gebco.net/
Günther, H., Hasselmann, S., and Janssen, P. A. E. M. (1992). The WAM Model Cycle 4.0. Technical Report No. 4. Hamburg: Deutsches Klimarechenzentrum.
Haarsma, R. J., Hazeleger, W., Severijns, C., de Vries, H., Sterl, A., Bintanja, R., et al. (2013). More hurricanes to hit Western Europe due to global warming. Geophys. Res. Lett. 40, 1783–1788. doi: 10.1002/grl.50360
Haigh, I. D., Pickering, M. D., Green, J. A. M., Arbic, B. K., Arns, A., Dangendorf, S., et al. (2019). The Tides They Are a-Changin’: a comprehensive review of past and future non-astronomical changes in tides, their driving mechanisms and future implications. Rev. Geophys. 58:e2018RG000636. doi: 10.1029/2018rg000636
Haigh, I. D., Wijeratne, E. M. S., MacPherson, L. R., Pattiaratchi, C. B., Mason, M. S., Crompton, R. P., et al. (2013). Estimating present day extreme water level exceedance probabilities around the coastline of Australia: tides, extra-tropical storm surges and mean sea level. Clim. Dyn. 42, 121–138. doi: 10.1007/s00382-012-1652-1
Hallegatte, S., Ranger, N., Mestre, O., Dumas, P., Corfee-Morlot, J., Herweijer, C., et al. (2011). Assessing climate change impacts, sea level rise and storm surge risk in port cities: A case study on Copenhagen. Clim. Change 104, 113–137. doi: 10.1007/s10584-010-9978-3
Hausfather, Z., and Peters, G. P. (2020). Emissions – the ‘business as usual’ story is misleading. Nature 577, 618–620. doi: 10.1038/d41586-020-001773
Hinkel, J., Lincke, D., Vafeidis, A. T., Perrette, M., Nicholls, R. J., Tol, R. S. J., et al. (2014). Coastal flood damage and adaptation costs under 21st century sea-level rise. Proc. Natl. Acad. Sci. U.S.A. 111, 3292–3297. doi: 10.1073/pnas.1222469111
Horsburgh, K. J., and Wilson, C. (2007). Tide-surge interaction and its role in the distribution of surge residuals in the North Sea. J. Geophys. Res. 112:C08003. doi: 10.1029/2006JC004033
Hoyer, S., and Hamman, J. J. (2017). xarray: N-D labeled Arrays and Datasets in Python. J. Open Res. Softw. 5:10. doi: 10.5334/jors.148
Irazoqui Apecechea, M., Verlaan, M., Zijl, F., Le Coz, C., and Kernkamp, H. W. J. (2017). Effects of self-attraction and loading at a regional scale: a test case for the Northwest European Shelf. Ocean Dyn. 67, 729–749. doi: 10.1007/s10236-017-1053-4
Jacob, D., Petersen, J., Eggert, B., Alias, A., Christensen, O. B., Bouwer, L. M., et al. (2014). EURO-CORDEX: New high-resolution climate change projections for European impact research. Reg. Environ. Chang. 14, 563–578. doi: 10.1007/s10113-013-0499-2
Jevrejeva, S., Jackson, L. P., Grinsted, A., Lincke, D., and Marzeion, B. (2018). Flood damage costs under the sea level rise with warming of 1.5∘C and 2∘C. Environ. Res. Lett. 13:074014. doi: 10.1088/1748-9326/aacc76
Kernkamp, H. W., Van Dam, A., Stelling, G. S., and de Goede, E. D. (2011). Efficient scheme for the shallow water equations on unstructured grids with application to the Continental Shelf. Ocean Dyn. 61, 1175–1188. doi: 10.1007/s10236-011-0423-6
Koks, E. E., Rozenberg, J., Zorn, C., Tariverdi, M., Vousdoukas, M., Fraser, S. A., et al. (2019). A global multi-hazard risk analysis of road and railway infrastructure assets. Nat. Commun. 10:2677. doi: 10.1038/s41467-019-10442-3
Kumar, D., Mishra, V., and Ganguly, A. R. (2015). Evaluating wind extremes in CMIP5 climate models. Clim. Dyn. 45, 411–453. doi: 10.1007/s00382-014-2306-2
Lin, N., Emanuel, K. A., Oppenheimer, M., and Vanmarcke, E. (2012). Physically based assessment of hurricane surge threat under climate change. Nat. Clim. Chang. 2, 462–467. doi: 10.1038/nclimate1389
Lincke, D., and Hinkel, J. (2018). Economically robust protection against 21st century sea-level rise. Glob. Environ. Chang. 51, 67–73. doi: 10.1016/j.gloenvcha.2018.05.003
Madsen, K. S., Murawski, J., Blokhina, M., and Su, J. (2019). Sea level change: mapping Danish municipality needs for climate information. Front. Earth Sci. 7:81. doi: 10.3389/feart.2019.00081
Marsooli, R., Lin, N., Emanuel, K., and Feng, K. (2019). Climate change exacerbates hurricane flood hazards along US Atlantic and Gulf Coasts in spatially varying patterns. Nat. Commun. 10:3785. doi: 10.1038/s41467-019-11755-z
Mawdsley, R. J., Haigh, I. D., and Wells, N. C. (2015). Global secular changes in different tidal high water, low water and range levels. Earths Future 3, 1–16. doi: 10.1002/2014EF000282
Menéndez, M., and Woodworth, P. L. (2010). Changes in extreme high water levels based on a quasi-global tide-gauge data set. J. Geophys. Res 115:C10011. doi: 10.1029/2009JC005997
Mentaschi, L., Vousdoukas, M., Voukouvalas, E., Sartini, L., Feyen, L., Besio, G., et al. (2016). The transformed-stationary approach: a generic and simplified methodology for non-stationary extreme value analysis. Hydrol. Earth Syst. Sci. 20, 3527–3547. doi: 10.5194/hess-20-3527-2016
Moss, R. H., Edmonds, J. A., Hibbard, K. A., Manning, M. R., Rose, S. K., Van Vuuren, D. P., et al. (2010). The next generation of scenarios for climate change research and assessment. Nature 463, 747–756. doi: 10.1038/nature08823
Muis, S., Haigh, I. D., Nobre, G. G., Aerts, J. C. J. H., and Ward, P. J. (2018). Influence of El Niño–Southern Oscillation on global coastal flooding. Environ. Res. Lett. 6, 1311–1322.
Muis, S., Lin, N., Verlaan, M., Winsemius, H. C., Ward, P. J., and Aerts, J. C. J. H. (2019). Spatiotemporal patterns of extreme sea levels along the western North-Atlantic coasts. Sci. Rep. 9:3391. doi: 10.1038/s41598-019-40157-w
Muis, S., Verlaan, M., Winsemius, H. C., Aerts, J. C. J. H. J. H., and Ward, P. J. (2016). A global reanalysis of storm surges and extreme sea levels. Nat. Commun. 7:11969. doi: 10.1038/ncomms11969
Nicholls, R. J., Brown, S., Goodwin, P., Wahl, T., Lowe, J., Solan, M., et al. (2018). Stabilization of global temperature at 1.5°C and 2.0°C: implications for coastal areas. Philos. Trans. R. Soc. A Math. Phys. Eng. Sci. 376:20160448. doi: 10.1098/rsta.2016.0448
Oppenheimer, M., Glavovic, B., Hinkel, J., van de Wal, R., Magnan, A. K., Abd-Elgawad, A., et al. (2019). “Sea level rise and implications for low lying islands, coasts and communities,” in PCC Special Report on the Ocean and Cryosphere in a Changing Climate, eds H.-O. Pörtner, D. C. Roberts, V. Masson-Delmotte, P. Zhai, M. Tignor, E. Poloczanska, et al. (Cambridge: Cambridge University Press).
Paprotny, D., and Terefenko, P. (2017). New estimates of potential impacts of sea level rise and coastal floods in Poland. Nat. Hazards 85, 1249–1277. doi: 10.1007/s11069-016-2619-z
Peters, G. P., Andrew, R. M., Boden, T., Canadell, J. G., Ciais, P., Le Quéré, C., et al. (2013). The challenge to keep global warming below 2C. Nat. Clim. Chang. 3, 4–6. doi: 10.1038/nclimate1783
Pickering, M. D. D., Horsburgh, K. J. J., Blundell, J. R. R., Hirschi, J. J.-M. J. M., Nicholls, R. J. J., Verlaan, M., et al. (2017). The impact of future sea-level rise on the global tides. Cont. Shelf Res. 142, 50–68. doi: 10.1016/j.csr.2017.02.004
Pickering, M. D. D., Wells, N. C., Horsburgh, K. J., and Green, J. A. M. A. M. (2012). The impact of future sea-level rise on the European Shelf tides. Cont. Shelf Res. 35, 1–15. doi: 10.1016/j.csr.2011.11.011
Pryor, S. C., Nikulin, G., and Jones, C. (2012). Influence of spatial resolution on regional climate model derived wind climates. J. Geophys. Res. Atmos. 117:D03117. doi: 10.1029/2011JD016822
Reimann, L., Vafeidis, A. T., Brown, S., Hinkel, J., and Tol, R. S. J. (2018). Mediterranean UNESCO World Heritage at risk from coastal flooding and erosion due to sea-level rise. Nat. Commun. 9:4161. doi: 10.1038/s41467-018-06645-9
Schenkel, B. A., and Hart, R. E. (2012). An examination of tropical cyclone position, intensity, and intensity life cycle within atmospheric reanalysis datasets. J. Clim. 25, 3453–3475. doi: 10.1175/2011JCLI4208.1
Schulzweida, U. (2019). CDO User Guide Version 1.9.6. Available online at: https://doi.org/10.5281/zenodo.2558193 (accessed July, 2019).
Stammer, D., Ray, R. D., Andersen, O. B., Arbic, B. K., Bosch, W., Carrère, L., et al. (2014). Accuracy assessment of global barotropic ocean tide models. Rev. Geophys. 52, 243–282. doi: 10.1002/2014RG000450
Taylor, K. E., Stouffer, R. J., and Meehl, G. A. (2012). An overview of CMIP5 and the experiment design. Bull. Am. Meteorol. Soc. 93, 485–498. doi: 10.1175/BAMS-D-11-00094.1
Vafeidis, A. T., Nicholls, R. J., McFadden, L., Tol, R. S. J., Hinkel, J., Spencer, T., et al. (2008). A new global coastal database for impact and vulnerability analysis to sea-level rise. J. Coast. Res. 244, 917–924. doi: 10.2112/06-0725.1
Verlaan, M., De Kleermaeker, S., and Buckman, L. (2015). “GLOSSIS: global storm surge forecasting and information system,” in Proceedings of the 22nd Australasian Coastal and Ocean Engineering Conference and the 15th Australasian Port and Harbour Conference (Auckland: Engineers Australia and IPENZ), 1–6.
Vitousek, S., Barnard, P. L., Fletcher, C. H., Frazer, N., Erikson, L., and Storlazzi, C. D. (2017). Doubling of coastal flooding frequency within decades due to sea-level rise. Sci. Rep. 7:1399. doi: 10.1038/s41598-017-01362-7
Vousdoukas, M. I., Mentaschi, L., Voukouvalas, E., Bianchi, A., Dottori, F., and Feyen, L. (2018a). Climatic and socioeconomic controls of future coastal flood risk in Europe. Nat. Clim. Chang. 8, 776–780. doi: 10.1038/s41558-018-0260-4
Vousdoukas, M. I., Mentaschi, L., Voukouvalas, E., Verlaan, M., and Feyen, L. (2017). Extreme sea levels on the rise along Europe’s coasts. Earths Future 5, 304–323. doi: 10.1002/2016EF000505
Vousdoukas, M. I., Mentaschi, L., Voukouvalas, E., Verlaan, M., Jevrejeva, S., Jackson, L. P., et al. (2018b). Global probabilistic projections of extreme sea levels show intensification of coastal flood hazard. Nat. Commun. 9:2360. doi: 10.1038/s41467-018-04692-w
Vousdoukas, M. I., Voukouvalas, E., Annunziato, A., Giardino, A., and Feyen, L. (2016). Projections of extreme storm surge levels along Europe. Clim. Dyn. 47, 1–20. doi: 10.1007/s00382-016-3019-5
Wahl, T. (2017). Sea-level rise and storm surges, relationship status: complicated! Environ. Res. Lett. 12:111001. doi: 10.1088/1748-9326/aa8eba
Wahl, T., Haigh, I. D., Nicholls, R. J., Arns, A., Dangendorf, S., Hinkel, J., et al. (2017). Understanding extreme sea levels for broad-scale coastal impact and adaptation analysis. Nat. Commun. 8:16075. doi: 10.1038/ncomms16075
Ward, P. J., Jongman, B., Salamon, P., Simpson, A., Bates, P. D., De Groeve, T., et al. (2015). Usefulness and limitations of global flood risk models. Nat. Clim. Chang. 5, 712–715. doi: 10.1038/nclimate2742
Wilmes, S.-B., Green, J. A. M., Gomez, N., Rippeth, T. P., and Lau, H. (2017). Global tidal impacts of large-scale ice-sheet collapses. J. Geophys. Res. Ocean 122, 8354–8370. doi: 10.1002/2017JC013109
Woodworth, P. L., Hunter, J. R., Marcos, M., Caldwell, P., Menéndez, M., and Haigh, I. (2016). Towards a global higher-frequency sea level dataset. Geosci. Data J. 3, 50–59. doi: 10.1002/gdj3.42
Keywords: climate change, global model, extreme sea levels, sea-level rise, coastal flooding
Citation: Muis S, Irazoqui Apecechea M, Dullaart J, de Lima Rego J, Madsen KS, Su J, Yan K and Verlaan M (2020) A High-Resolution Global Dataset of Extreme Sea Levels, Tides, and Storm Surges, Including Future Projections. Front. Mar. Sci. 7:263. doi: 10.3389/fmars.2020.00263
Received: 18 November 2019; Accepted: 01 April 2020;
Published: 29 April 2020.
Edited by:
Roshanka Ranasinghe, IHE Delft Institute for Water Education, NetherlandsReviewed by:
Michalis Vousdoukas, European Commission, Joint Research Centre (JRC), ItalyKathleen Lynne McInnes, CSIRO Oceans and Atmosphere (O&A), Australia
Copyright © 2020 Muis, Irazoqui Apecechea, Dullaart, de Lima Rego, Madsen, Su, Yan and Verlaan. This is an open-access article distributed under the terms of the Creative Commons Attribution License (CC BY). The use, distribution or reproduction in other forums is permitted, provided the original author(s) and the copyright owner(s) are credited and that the original publication in this journal is cited, in accordance with accepted academic practice. No use, distribution or reproduction is permitted which does not comply with these terms.
*Correspondence: Sanne Muis, sanne.muis@deltares.nl; sanne.muis@vu.nl