- 1Muséum National d’Histoire Naturelle, UMR BOREA, MNHN-CNRS-UCN-UPMC-IRD-UA, Station Marine de Concarneau, Concarneau, France
- 2UMR 7245, Unité Molécules de Communication et Adaptation des Micro-organismes, Muséum National d’Histoire Naturelle, Paris, France
Microphytobenthic (MPB) biofilms in intertidal muddy sediments play important ecological functions in coastal ecosystems. These biofilms are mainly composed of epipelic diatoms but also prokaryotes, with a dominance of bacteria, which excrete diverse extracellular polymeric substances (EPS) according to their environment. While numerous studies have investigated the main components of these EPS matrices via traditional colorimetric assays, their fine composition, notably in specialized metabolites, is still largely unknown. A better chemical characterization of these MPB biofilms is necessary, especially regarding the numerous functions their chemical components play for microorganisms (e.g., motility, cell protection, defense mechanisms, and chemical communication), but also for coastal systems (e.g., primary production, sediment stabilization, larval settlement of some invertebrates with high economical value). An alternative approach to traditional analyses is the use of untargeted metabolomic techniques, which have not yet been applied to such MPB biofilms. The objectives of the present study were to (a) propose a protocol for metabolic fingerprinting by LC-MS and GC-MS for metabolites analysis in polar and non-polar fractions in MPB biofilms extracted from mudflat sediment and to (b) apply this protocol to a case study: the effect of light exposure on the metabolomic fingerprint of the MPB biofilm community. We compared three extraction methods using different mixes of solvents and selected a methanol/chloroform mix (1:1), which gave better results for both techniques and fractions. We then applied the selected protocol to our case study using a short-term light exposure experiment in aquaria (7 days). The present study is the first using a detailed untargeted metabolomic approach on MPB biofilms from mudflat sediment and will provide a solid baseline for further work in this area.
Introduction
Intertidal mudflats are key areas, forming the transition between terrestrial and aquatic environments, playing important ecological roles in estuarine ecosystems (Underwood and Kromkamp, 1999; Stal, 2003; Haro et al., 2019). These mudflats support extensive microphytobenthic (MPB) biofilm developing at the sediment/water interface in shallow water environments (e.g., estuarine, intertidal areas, and sandy beach) (Pierre et al., 2014; Hubas et al., 2018). The species composition of MPB is diverse and often dominated by epipelic diatoms (Perkins et al., 2010), and composed of other eukaryotic (e.g., euglenids) and procaryotic (e.g., cyanobacteria and archaea) organisms. These biofilms contribute to the high productivity of intertidal mudflats and provide various ecosystem services such as nutrient recycling (carbon and nitrogen), sediment stabilization and larval settlement for invertebrates of high commercial value (Decho, 2000; Toupoint et al., 2012; Bohórquez et al., 2017). The microorganisms forming the MBP biofilm are entangled in a matrix of hydrated extracellular polymeric substances (EPS) exuded by the microphytobenthos, mainly by benthic diatoms (Pierre et al., 2014; Passarelli et al., 2015). These EPS constitute the cement holding cells in close proximity, allowing interaction, communication, metabolic cooperation or competition (Flemming and Wingender, 2010; Elias and Banin, 2012; Sutherland, 2017). EPS also play diverse fundamental roles in biofilms (e.g., motility of the pennate diatoms; Underwood and Paterson, 2003; Hanlon et al., 2006). Numerous studies have investigated the main components of these EPS matrices via traditional colorimetric assays, notably in their carbohydrate fraction (e.g., Underwood and Paterson, 2003; Hanlon et al., 2006; Pierre et al., 2010, 2014) but the chemical characterization of MPB biofilms, notably in small compounds (metabolites; typically < 1,500 Da) is still largely unknown. The biofilm matrix is able to absorb diverse small compounds and ions (Wotton, 2004; Hubas et al., 2018), increasing the chemical diversity of the ‘dark matter of biofilms’ (Flemming and Wingender, 2010; Flemming, 2016). Due to the complexity of microbial species assemblages in mudflat biofilms, the chemical analysis of synthesized compounds is challenging. A better chemical characterization of these MPB biofilms is therefore necessary, especially regarding the numerous functions their chemical compounds play for microorganisms and coastal areas. It is also crucial to better understand microbial interactions within natural MPB biofilms.
Metabolites are the end products of cellular regulatory processes (Fiehn, 2002). Traditionally, we distinguish primary metabolites, implied in metabolic pathways required for cell maintenance, survival, development and growth, from secondary or specialized metabolites, which are considered to be non-essential for the life of the producer organism but provide survival advantages in various ways (e.g., by improving nutrient availability, protecting against environmental stressors, and enhancing competitive interactions with other organisms or acting as a defense mechanism) (Kliebenstein, 2004; Kooke and Keurentjes, 2011). The production of specialized metabolites is strongly impacted by environmental signals, such as pH, light, carbon, and nitrogen sources or by organisms living in the same habitat. Accordingly, the metabolome (i.e., the set of metabolites) can provide a ‘snapshot’ of the physiological state of an organism at a given time (Fiehn, 2002; Kooke and Keurentjes, 2011).
The use of metabolomic techniques, notably through metabolomic fingerprinting approaches, allows the simultaneous analysis of a large set of metabolites and can thus be an alternative (or complementary) approach to traditional analyses for the study of MPB biofilms. In marine sciences, metabolomics is an emerging discipline that can bring useful information on the responses of marine organisms to environmental changes or stressors (Bundy et al., 2009), to assess health status (Dove et al., 2012) and to explore chemical communication between organisms (Gaillard and Potin, 2014).
Several studies explored the metabolomic response of marine microorganisms, such as diatoms or bacteria, to different factors. For example, a metabolomic approach has been used to study the metabolomic changes associated with the sexual reproduction in the marine diatom Seminavis robusta and to further isolate the sex pheromone implied (Gillard et al., 2013). Metabolite profiling was undertaken on 13 diatom cultures to assess their lipid diversity and to explore their metabolomic adaptation to nitrogen limitation (Bromke et al., 2015). Metabolomics has also been used to study chemically mediated interactions between bacteria and diatoms (Paul et al., 2013; Lépinay et al., 2018). However, studies of chemical profiles/metabolomic responses on complex assemblages such as natural biofilms are rare [Elias and Banin, 2012, an exception being the work of Chung et al. (2010) using GC-MS to study the chemical profile of subtidal biofilms according to substrata and age; and Bourke et al. (2017) using GC-MS and LC-MS to explore microalgae metabolism on permeable sediments]. Metabolomics could be a useful tool to better understand microbial interactions and communication in complex microphytobenthic communities of mudflat biofilms.
The objectives of the present study were to (a) propose a protocol for metabolomic fingerprinting by LC-MS and GC-MS for metabolites analysis in mid-polar and apolar fractions of MPB biofilms extracted from mudflat sediments and to (b) apply this protocol to a case study: the effect of light exposure on the metabolomic fingerprint of the MPB biofilm community, using a short-term aquaria experiment (7 days). Light dose and quality is an important environmental factor, notably for photosynthetic activity, microphytobenthic movement, and metabolites biosynthesis (Perkins et al., 2001; Li et al., 2014; Juneau et al., 2015). We thus explored the significant changes in metabolic production of MPB biofilms as a response to changes in light exposure. Identification of metabolites was also tentatively performed by using NIST 2017 database.
Materials and Methods
Sampling
General Procedure and Site Description
Surface samples of mud sediment (depth of ∼1–2 cm) presenting dense microphytobenthic (MPB) biofilm (Supplementary Figure S1) were collected during low tide at the Marine Station of Concarneau (France; 47°52.5804′N; 3°55.026′W) in an empty breeding pond, supplied by the surrounding seawater from the Concarneau bay. Due to the particularity of this breeding pond, the biofilm growing at the sediment surface is never fully emerged during low tide (minimum 4–5 cm of seawater). A subsample of MPB biofilm was prepared for Scanning Electron Microscopy (SEM) observation. Briefly, the sample was cleaned in saturated potassium permanganate followed by concentrated HCl. After acid cleaning, the sample was filtered on a polycarbonate membrane filter (Millipore GTTP, 0.2 μm), coated with gold and observed with a Sigma 300 (Zeiss) field-emission SEM equipped with a conventional Everhart–Thornley and in-lens detectors of secondary electrons at 1.5 kV.
Protocol Optimization for Metabolite Extraction
For the optimization of metabolite extraction, surface samples of mud sediment were collected in February 2019, placed in a tray and the MBP biofilm (top 2–5 mm depth) was collected with a spatula after sediment stabilization (15 samples). Samples were immediately frozen at −20°C until chemical extraction.
Short-Term Light Exposure Experiment
For the short-term light exposure experiment, surface samples of mud sediment were collected on the 13th March 2019 and directly randomly assigned to ten 1 L experimental tanks. The tanks were filled with seawater (around 4 cm of seawater layer on the sediment surface) and left overnight for sediment and biofilm stabilization. During the next morning, t0 samples of MBP biofilm (top 2–5 mm depth) were collected with a spatula and frozen at −20°C (10 samples). Five of the tanks were exposed to natural irradiance (NI; around 1 m from the window; mean irradiance of ca. 102 ± 19 μmol photon m–2 s–1). The five remaining tanks were placed in an opaque box covered in the inside with foiled and exposed to higher artificial irradiance (AI). Light was provided by two LED sources (12 W, V-Lumtech®) supplying an irradiance of ca. 167 ± 23 μmol photon m–2 s–1 over a 11 h:13 h light:dark cycle, corresponding to the photoperiod at this time of the year. Experimental conditions (natural/higher artificial irradiance) were maintained during 7 days. At the end of the experiment (t7), MBP biofilm samples were collected and frozen at −20°C until chemical extraction (n = 5 per condition).
Sample Preparation
Extraction and Fractionation
MBP biofilm samples were freeze-dried prior to extraction. A mass of 200 mg was extracted three times with 3 mL of solvent in an ultrasonic bath during 30 min. Three mixtures of solvent were tested for metabolite extraction in terms of reproducibility and metabolites detection (n = 5 samples per test): M1 = MeOH (methanol)/CHCl3 (chloroform) (1:1); M2 = H20/MeOH/CHCl3 (1:1:1); and M3 = H20/MeOH/CHCl3 (1.5:3.5:5) (see Table 1). Samples from the short-term light exposure experiment were treated with the mixture 1.
The organic phase was collected after centrifugation (1,800 g, 10 min). These steps were repeated three times and the organic phases were pooled and dried under N2 at room temperature. The dried extracts were then resuspended in 1 mL of MeOH and fractioned by Solid Phase Extraction (Strata C18-E, 500 mg/6 mL, Phenomenex®) after cartridges cleaning (6 mL MeOH) and conditioning (6 mL H2O), via three successive elutions: 6 mL of H2O, 6 mL of MeOH and 6 mL of CHCl3. The MeOH (mid-polar) and CHCl3 (apolar) fractions were dried under N2 before derivatization and were further analyzed separately to reduce the complexity of the extracts. Due to the high concentration in salts, which affects MS-based metabolomics analysis and can damage syringe and column, H2O fractions were not analyzed. SPE also permitted to fractionate samples in two phases that could be analyzed separately: one expected to contain mostly polar to mid-polar metabolites (MeOH fraction) and the other to mostly consist of non-polar metabolites (CHCl3 fraction).
Derivatization
Compounds were derivatized in order to be stable and volatile according to a standard protocol. First, 10 μL of ribitol (0.5 mg.mL–1 in dH2O) were added in MeOH fractions and 3 μL of tricosanoic acid (5 mg.mL–1 in chloroform) were added in CHCl3 fractions. Fractions were dried under N2 prior to derivatization. Polar functional groups (e.g., -OH, -COOH, and -NH2; Liebeke and Puskás, 2019) are routinely transformed to TMS-derivatives via the well-establish two-step derivatization procedure involving methoxymation followed by trimethylsilylation (Roessner et al., 2000; Sogin et al., 2019). Recently, a modification of this standard procedure has been applied on different human, terrestrial and marine samples (e.g., urine, yeast, seagrass, corallines, and mangrove sediments) and has shown an increase of sensitivity (i.e., increase in metabolite signal intensity) (Liebeke and Puskás, 2019; Sogin et al., 2019). This method improvement includes a drying step between the methoxymation and trimethylsilylation and was thus employed on our MeOH fractions. First, 80 μL of methoxyamine hydrochloride dissolved in pyridine (20 mg.mL–1) were added on the dried MeOH fractions. The mixture was ultrasonicated for 10 min and incubated for 90 min at 37°C in a thermal rotating incubator (120 rpm). The samples were then evaporated under N2 to remove pyridine. Secondly, 100 μL of BSTFA + 1% TMCS were added and the samples were ultrasonicated for 10 min, briefly vortexed and incubated again for 30 min at 37°C in the thermal rotating incubator. The samples were evaporated again under N2 to remove the BSTFA/TMCS and resuspended in MeOH for GC-MS analyses. Fatty acids are classically analyzed after transesterification to their corresponding fatty acid methyl esters (FAMEs) (e.g., Beale et al., 2018) the method presently used to derivatize compounds in our CHCl3 fractions. One milliliter of BF3-MeOH was added on the dried CHCl3 fractions. The mixture was heated at 80°C for 10 min and cooled down at room temperature. Then, 1 mL of deionized water and 1 mL of CHCl3 were added and vortexed before centrifugation at 1,800 g during 5 min. The lower phase was collected and used for GC-MS analyses.
Metabolomic Analyses
GC-MS
The MeOH and CHCl3 fractions were analyzed on a gas chromatograph (7890B GC System- G1513A autosampler, Agilent Technologies®) coupled to a mass selective detector (5977B MSD, Agilent Technologies®) and a flame ionization detector (FID). Separation of metabolites was performed on an HP-5ms Ultra Inert column (30 m, 0.25 mm, and 0.25 μm, Agilent Technologies®) with helium as mobile phase. A volume of 1 μL of each sample was injected in splitless mode at 250°C. The injector temperature was set to 280°C and the FID detector to 300°C. Mass spectra were acquired in electron ionization mode at 70 eV between 35 and 600 m/z at a scan rate of 1.3 scan.s–1. A constant flow rate was set to 1 mL.min–1.
For the CHCl3 fractions, the run started at 100°C for 1 min and increased by 15°C min–1 up to 215°C, by 5°C min–1 from 215 to 285°C and by 15°C min–1 from 285 to 325°C, followed by 3 min of post-run at 100°C. The total runtime was 28.33 min. For the MeOH fractions, the run started at 80°C for 1 min and increased by 10°C min–1 up to 325°C, holding 1 min at the final temperature. The run was followed by 3 min of post-run at 80°C for a total runtime of 26.5 min.
For both fractions, a solution with a mix of C8-C20 and C21-C40 alkanes (Fluka Analytical) was also injected for the determination of compound retention index. The identification of fatty acid methyl esters (FAMEs) was confirmed by comparison with a standard mixture (SupelCo 37 FAME mix). For each experiment and fraction, a quality control sample (QC) was prepared with 25 μL of each sample. It was used to monitor MS shift over time and to normalize data according to injection order. The run started with two blank injections, followed by 5 injections of the QC. Samples were then randomly injected, inserting one QC every five samples and two final blanks.
UHPLC-QToF
As LC-MS is more appropriate for polar, weakly polar and neutral compounds (Wang et al., 2015), only the MeOH fractions were analyzed with this technique. Metabolomic fingerprints of MeOH fractions were recorded on a Dionex Ultimate 3000 HPLC system coupled with a Maxis IITM QTOF mass spectrometer (Bruker, MA, United States) fitted with an electrospray ionization (ESI) source. Metabolite separation was performed on a C18 AcclaimTM RSLC Polar Advantage II (2.1 mm × 100 mm, 2.2 μm pore size) column (Thermo Scientific, MA, United States) at 40°C. The mobile phase consisted in a mix of H2O + 0.1% formic acid (solvent A) and acetonitrile + 0.1% formic acid (solvent B). Injection volume was set to 2 μL and elution flow to 0.3 mL min–1. The elution gradient profile was programmed as follows: 5% B during 2 min, increased up to 50% B from 2 to 9 min and to 90% B from 9 to 15 min, followed by an isocratic step of 90% B during 2 min. The initial conditions were gradually recovered from 17 to 19 min, and hold 3 min for column equilibration for a total runtime of 21 min. In the first half minute of each run, a sodium formate solution was injected directly as an internal reference for calibration. The acquisitions parameters of the ESI source were set as follows: electrospray voltage for the ESI source: 3,500 V, nebulizing gas (N2) pressure: 35 psi, drying gas (N2) flow: 8 mL min–1, and drying temperature: 200°C. Mass spectra were recorded in positive ionization mode over the m/z range 100–1,300 at a frequency of 2 Hz. For MS/MS analysis, the cycle time was of 3 s. A quality control sample (QC) was prepared with 25 μL of each sample. It was used to check MS shift over time and to normalize data according to injection order. The run started with two blank injections, followed by 8 injections of the QC for mass spectrometer stabilization. Samples were then randomly injected, inserting one QC every five samples. A final blank was injected to check any memory effect of the compounds on the column.
Data Treatment and Statistical Analyses
GC-MS
Agilent data files acquired from GC-MS analyses were exported into mzXML files using MSconvert (Chambers et al., 2012). mzXML files were then processed using the eRah package (version 1.1.0; Domingo-almenara et al., 2016) under R performing preprocessing, peak deconvolution [min.peak.width = 2.5 (1.5 for MeOH fractions), min.peak.height = 2,500 (1,500 for MeOH fractions), noise. threshold = 500, avoid. processing.mz = c (73,149,207)], peak alignment [min.spectra.cor = 0.90, max. time.dist = 5 (3 for MeOH fractions), mz. range = 40:600] and missing compounds recovery (recMissComp function, minimum number of samples was set at 6). The matrix of compounds obtained was then filtered: peaks present in blanks (signal/noise ratio > 10) and those with higher coefficient of variation in pools (CV < 25%) were removed from the dataset. Finally, compounds annotation was performed by comparing mass spectra with NIST 2017 library completed with the calculation of Kovats’ index (Van Den Dool and Kratz, 1963).
LC-MS
LC–MS raw data files were converted to mzXML files with MSconvert. mzXML files were then processed using the package XCMS for R software (R version 3.3.2, XCMS version 3.2.0). Optimized parameters for XCMS were used as follows: peak picking [method = “centwave”, peakwidth = c(5,20), ppm = 10], retention time correction (method = “obiwarp”, plottype = “deviation”) with final grouping parameters (bw = 2, mzwid = 0.015) and filling in missing peaks.
Other parameters were set to default values. A matrix of compounds with peak intensity, m/z value and retention time was generated. The latter was filtered according to blanks and QC to remove technical variability using in-house R scripts [1-Filtering the matrix according to peaks present in blanks relative to pools (signal/noise ratio > 10), 2-filtering the matrix according to peaks coefficient of variation (CV) calculated on pool (CV < 20%) and 3-filtering the matrix according to autocorrelation between peaks]. Metabolites were annotated with constructor software (Bruker Compass DataAnalysis 4.4). Molecular network based on LC-MS/MS spectra were constructed with GNPS (M. Wang et al., 2016) using the following settings: precursor ion mass tolerance: 2 Da, fragment ion mass tolerance: 0.5 Da, min pairs cos: 0.7, minimum matched fragment ion: 6, node topK: 10 and minimum cluster size: 2. Resulting networks were observed under Cytoscape 3.5.0 (Shannon et al., 2003). Metlin1, MassBank, SIRIUS 4.0 (Böcker and Dührkop, 2016) and In-Silico MS/MS DataBase (ISDB) (Allard et al., 2016) were also used for putative annotation.
Data from LC-MS and GC-MS were normalized by log-transformation before statistical analyses. The relative standard deviations (%RSD = standard deviation/mean∗100) were calculated for each metabolite (Parsons et al., 2009) to characterize measurement variability according to the solvent extraction mixtures. The percentage of total detected metabolites per sample was also calculated for each mixture used for metabolites extraction, for each dataset (i.e., MeOH fractions analyzed by GC-MS, MeOH fractions analyzed by LC-MS and CHCl3 fractions analyzed by GC-MS) on the final matrix (after data analyses and filtering according to blanks and QC). The normality of the data distribution (%RSD and %compounds detected) was tested using the Shapiro–Wilk test but not confirmed. The non-parametric Kruskal–Wallis’ test was thus used to identify differences between the percentages of RSD and metabolites detected according to the method, followed by post hoc Conover’s test. To identify which significant factors were linked to the metabolites diversity, we used Permutational Multivariate Analysis of Variance using distance matrices (PERMANOVA, 9999 permutations, vegan package for R). Principal component analysis (PCA) was used to visualize the metabolome variation according to the irradiance condition and time (ade4 package for R). Powered Partial Least-Squares-Discriminant Analysis (PPLS-DA) were used to find the maximum covariance between our data set and their class membership. Permutational tests based on cross model validation (MVA.test and pairwise.MVA.test) were applied to test differences between groups (RVAideMemoire package) and correlation circles were drown to identify discriminating compounds (RVAideMemoire package). Wilcoxon signed-rank tests were used to identify differences in normalized intensities of discriminating compounds between sampling time (t0 vs. t7 samples) and Mann–Whitney–Wilcoxon tests to identify those between light treatments (NI vs. AI).
Results
The MPB biofilm was dominated by diatoms, mainly by Pleurosigma formosum (Figure 1A), followed by Gyrosigma balticum (Figure 1B). Other abundant genera were characterized: Entomoneis, Cocconeis, Falacia, and Campylodiscus.
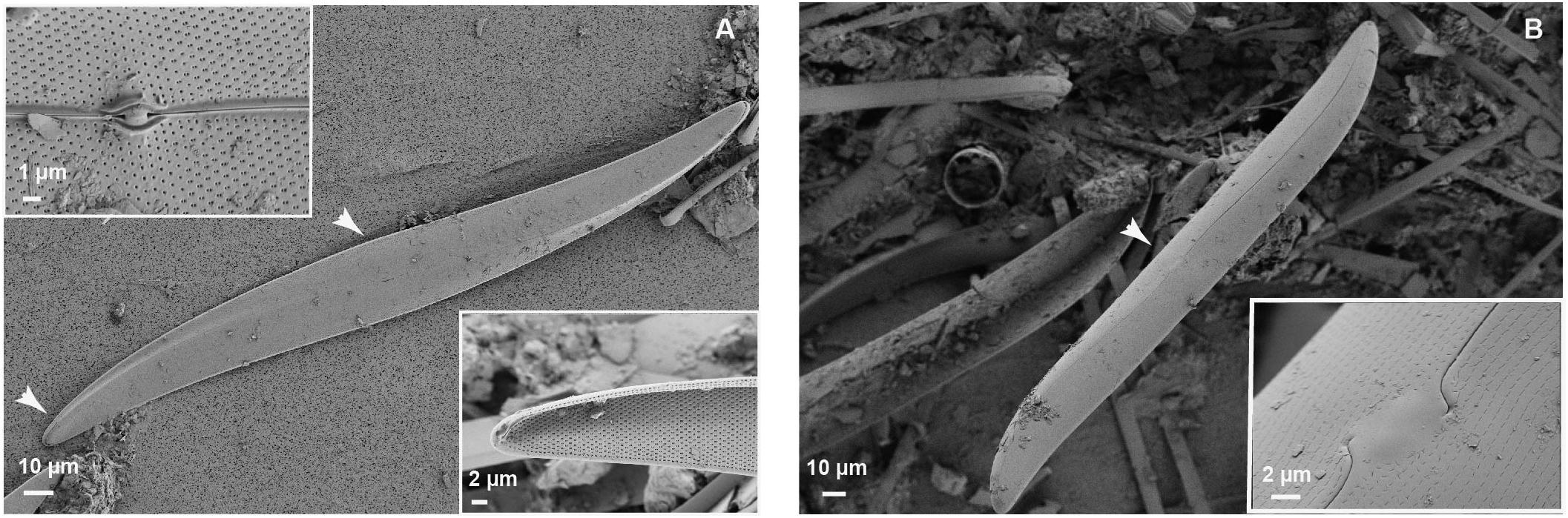
Figure 1. Scanning electron microscopy (SEM) images of (A) Pleurosigma formosum with a focus on the central nodule structure (top left) and apical end structure (bottom right) and (B) Gyrosigma balticum with a focus on the central nodule structure (for all images: detector SE2, diaph 30.00).
Protocol Selection for Metabolite Extraction
The resulting CHCl3 and MeOH fractions obtained with M1, M2 or M3 were compared for extracting metabolites from MPB biofilms present in mudflat sediments.
In the CHCl3 fractions analyzed by GC-MS, the RSDs were low and not significantly different according to the solvent mixtures used (median RSDs of 1.25, 1.15, and 1.47% for M1, M2, and M3, respectively; Figure 2A; KW = 1.13, p = 0.57). All three mixtures allowed to detect the same number of metabolites in these fractions (100% of total compounds detected, Figure 2B).
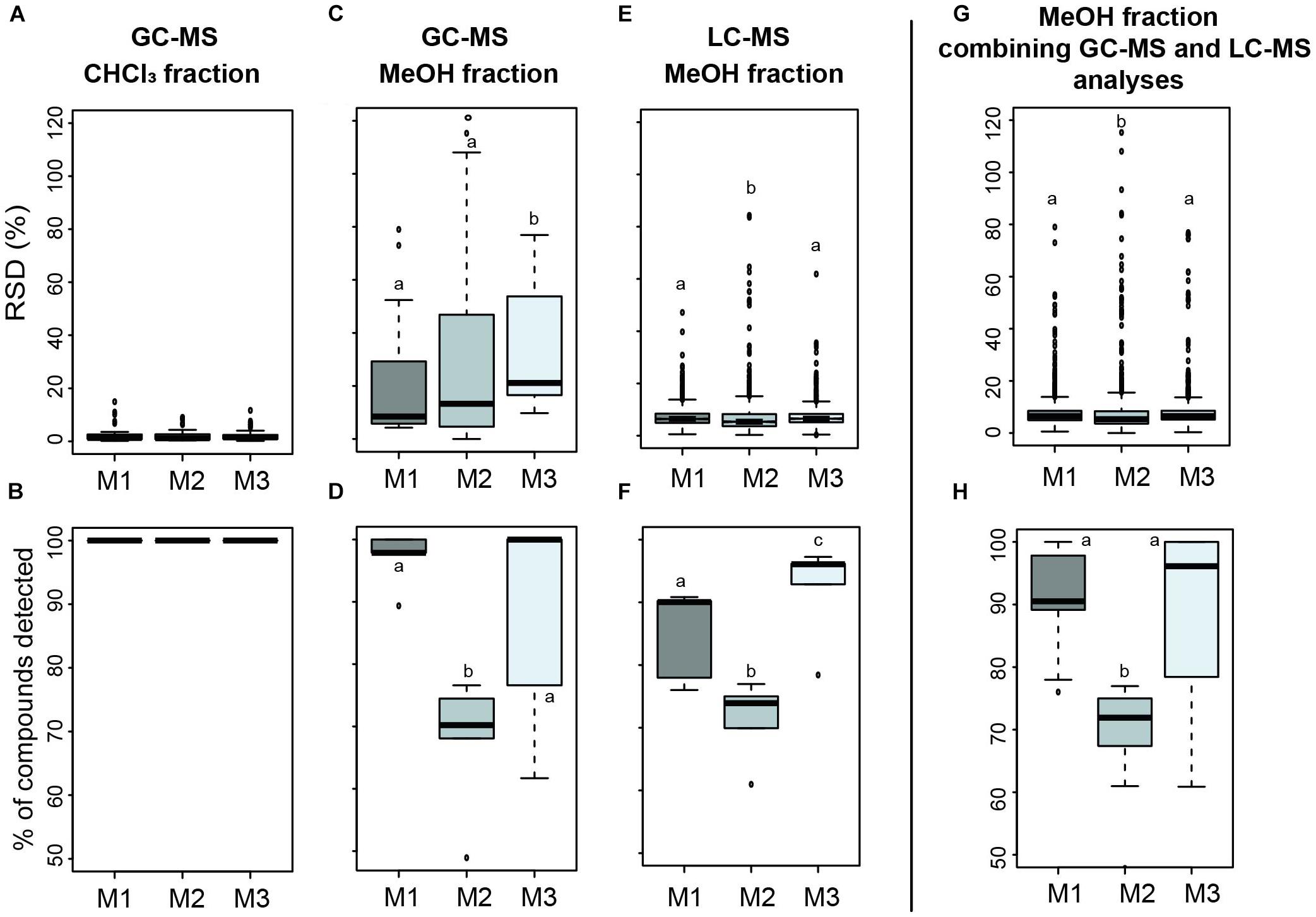
Figure 2. Boxplots of the relative standard deviation (% RSD; A,C,E,G) and percentage of total detected metabolites (B,D,F,H) comparing the reproducibility associated with three solvent extraction mixtures according to the fraction (CHCl3/MeOH) and the technique (GC-MS/LC-MS) used (M1 = MeOH/CHCl3 (1:1), M2 = H20/MeOH/CHCl3 (1:1:1), and M3 = H20/MeOH/CHCl3 (1.5:3.5:5). Statistical analyses were performed using Kruskal–Wallis followed by post hoc Conover’s test. Letters indicate distinct groupings based on post hoc pairwise comparisons among solvent mixtures (p < 0.05).
However, significant differences in reproducibility and number of detected metabolites were observed in the MeOH fractions analyzed by GC-MS and LC-MS (Figures 2C,E). In these fractions analyzed by GC-MS, a higher reproducibility was obtained with M1 and M2 (median RSDs of 8.47 and 13.28%, respectively, Figure 2C) while M3 gave significantly higher RSD (median RSD of 21.12%; post hoc p < 0.05). A higher number of metabolites were detected with M1 and M3 (96.96 ± 4.51 and 87.39 ± 18.08% of total detected metabolites, respectively; Figure 2D) but the variability in M3 appeared superior (standard deviation of 18.08%, four times higher compared to M1). A lower number of metabolites was detected in the same dataset with M2 (66.95 ± 11.23%; post hoc p < 0.05). In the same MeOH fractions analyzed by LC-MS (Figure 2E), low RSDs were obtained for all mixtures but M2 gave the lowest (median RSD 5.29%) compared to M1 and M3 which were not significantly different regarding the reproducibility (median RSDs of 6.42 and 6.45%, respectively; post hoc p = 0.58). A higher number of metabolites were detected with M3 (92.2 ± 7.8%), followed by M1 (85 ± 7.4%; Figure 2F). As for GC-MS analyses in these MeOH fractions, less metabolites were significantly detected in the same dataset with M2 (71.3 ± 6.3%). Combining results obtained with both techniques on the MeOH fractions (Figures 2G,H), we got a higher number of metabolites detected with M1 and M3 (91 ± 8.5 and 89.8 ± 13.4%, respectively) with a lower variability for M1, while not statistically supported [Figure 2H; KW = 14, p < 0.05, post hoc p(M1 vs. M3) = 0.98].
Collectively, we determined that solvent mixture 1 was more appropriate to reflect the chemical diversity of MPB biofilm and was used for the light exposure experiment.
Short-Term Light Exposure Experiment
CHCl3 Fractions Analyzed by GC-MS
After GC-MS data analyses and the exclusion of artifactual and irreproducible peaks (coefficient of variation > 25%), a total of 56 features were highlighted in the CHCl3 fraction (see Supplementary Table S1 and Supplementary Figure S2). These features were tentatively annotated using NIST 2017 library and by comparison with a standard mixture for fatty acid methyl esters. Around 77% of the compounds could be annotated while 23% remain unknown. Among the annotated compounds (Table 2 and Figure 3), a majority of fatty acids (FA) were displayed (53.5%), with a dominance of saturated FA (SFA; 30.2%) followed by monounsaturated FA (MUFA; 11.6%) and polyunsaturated FA (PUFA; 11.6%). Other families of molecules were highlighted such as alkenes (9.3%), alkanes (9.3%), fatty esters (7%), terpenes (7%), carboxylic acids (4.7%), phtalic acids (4.7%), a lactone (4,8,12-trimethyltridecan-4-olide; 2.3%), and an heterocyclic compound [3-(3,7-dimethyloct-6-enoxy)oxane; 2.3%]. Two compounds were assigned as plastic pollutants (ethyl 4-ethoxybenzoate and diethyl phtalate).
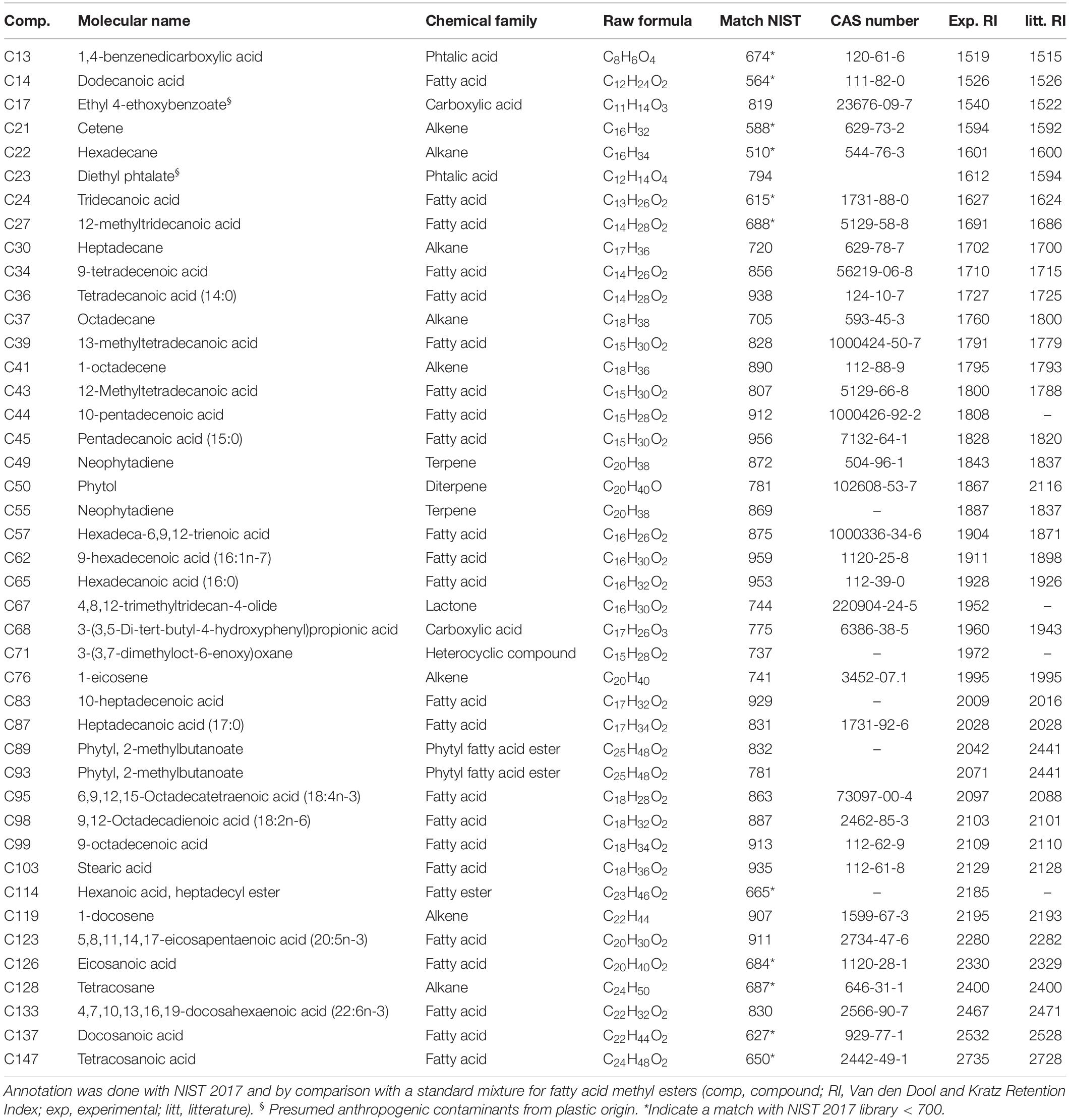
Table 2. Annotated compounds in the CHCl3 fraction of the MPB biofilm during the light exposure experiment (after data analyses and filtering).
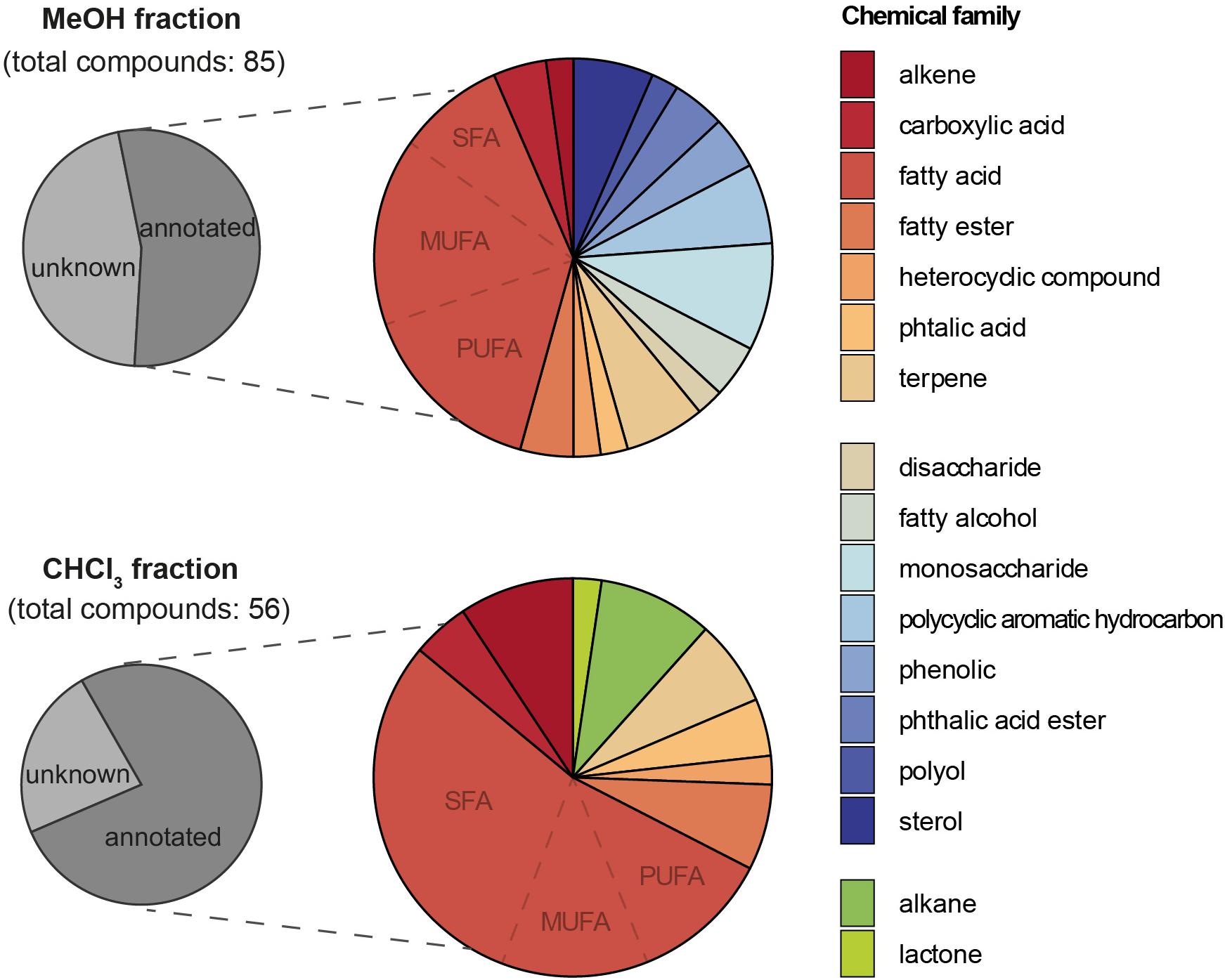
Figure 3. Pie charts showing the proportions (from the total number of detected compounds) of annotated compounds and their chemical family in the MeOH and CHCl3 fractions analyzed by GC-MS.
The effects of irradiance condition (natural: NI versus higher artificial: AI) and time on the metabolomic fingerprint of MPB biofilm were then investigated in this CHCl3 fraction obtained by GC-MS. An unsupervised analysis (PCA) allowed to explain 53.47% of variance on the two first components (Figure 4A). The supervised analysis (PPLS-DA) highlighted the influence of time and light exposure on the metabolome of the MPB biofilm. Indeed, different metabolomic fingerprints were observed between t0 and t7 samples independently of the irradiance condition (PPLS-DA, CER = 0.39, p = 0.003, post hoc p < 0.05 for each pair, Figure 4B and Supplementary Table S2), except for t0 NI versus t7 AI for which metabolomic changes were not statistically supported (post hoc p = 0,108, Supplementary Table S2). The irradiance condition was correlated with metabolomic changes after 7 days of treatment, between samples exposed to natural (t7 NI) and higher artificial irradiance [t7 AI; post hoc p(t7 AI vs. t7 NI) = 0.036; Figure 4B, Supplementary Table S2].
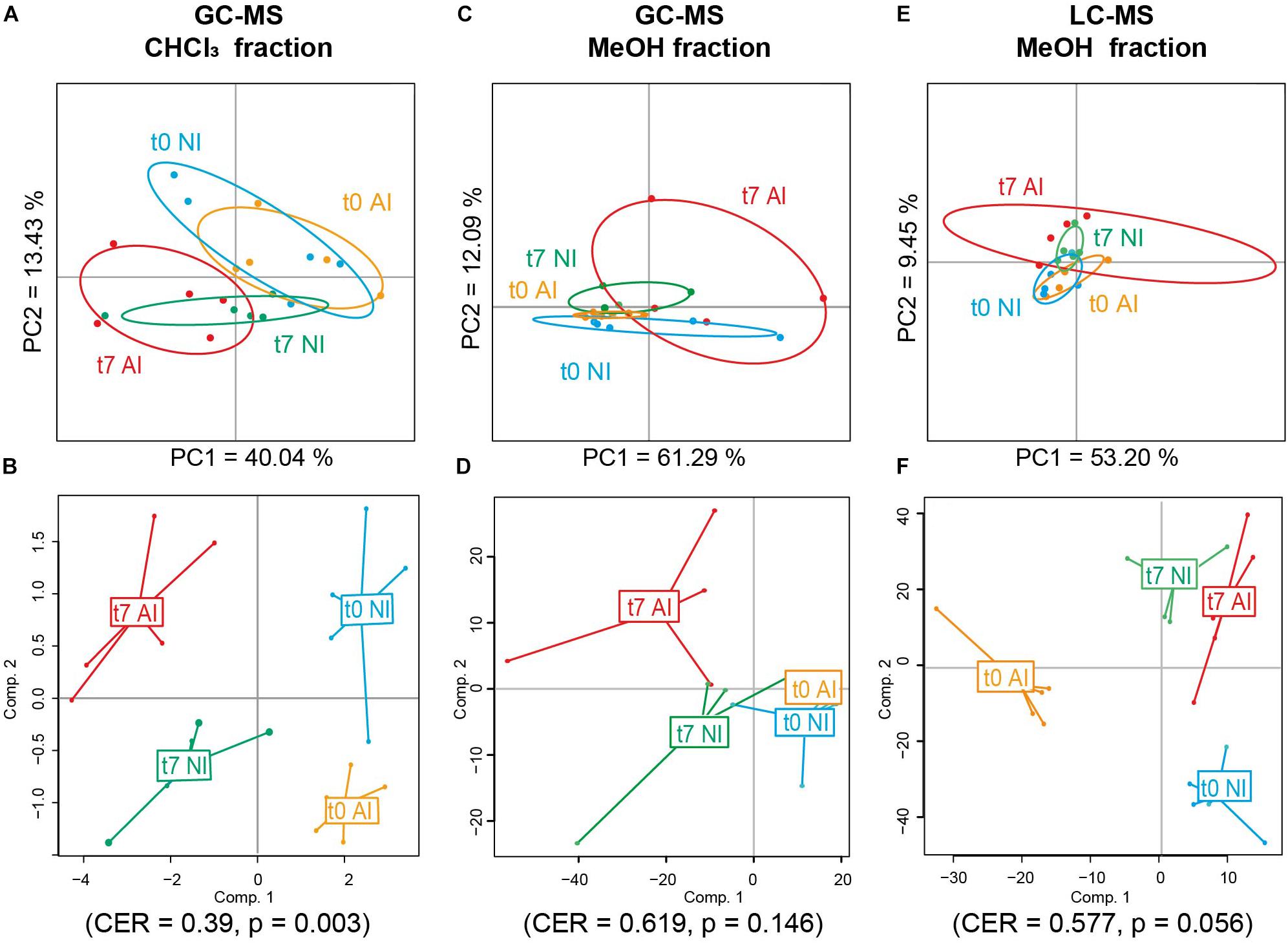
Figure 4. Metabolomic fingerprint analyses of the MPB biofilm at the beginning (t0) and the end (t7) of the experiment depending of the light exposure conditions via unsupervised (Principal Component Analysis, PCA, A,C,E) and supervised discriminant (Powered Partial Least-Squares-Discriminant Analysis, PPLS-DA, B,D,F) analyses. The metabolome was analyzed by (A,B) GC-MS for CHCl3 fractions, (C,D) GC-MS for MeOH fractions, and (E,F) LC-MS for MeOH fractions (NI: natural irradiance; AI: higher artificial irradiance). CER, classification error rate with p-value after double cross model validation.
The metabolites significantly impacted by time or light exposure conditions were thus investigated (Figure 5A). Four of them majorly contributed to the metabolomic discrimination according to time (threshold 0.8; plastic pollutants were not considered): C39, C43, C56, and C93. All these compounds were significantly decreased at the end of the experiment (Figure 5B). Two of them could be annotated as branched-chain fatty acids: 13- methyltetradecanoic acid (C39) and 12- methyltetradecanoic acid (43), one as a phytol derivative (C93, phytyl, 2-methylbutanoate) and C56 remained unknown. Other compounds significantly contributing to the metabolomic variation with time were found (with threshold = 0.7 on PPLS-DA loading plot, Figure 5A) and majorly tend toward a decrease by the end of the experiment (C14: dodecanoic acid, C19: unknown, C27: 12-methyltridecanoic acid, C36: tetradecanoic acid, C62: 9-hexadecenoic acid, C67: 4,8,12-trimethyltridecan-4-olide, C74: unknown, C75: unknown, C83: 10-heptadecenoic acid and C114: hexanoic acid, heptadecyl ester), excepted one metabolite which increased at t7 (C128: tetracosane) (see Supplementary Figure S3). Two metabolites correlated with the irradiance condition at t7 were also highlighted (threshold = 0.7, Figure 5C): C12 (unknown) and C30 (heptadecane), which are both decreased by the end of the experiment in samples exposed to higher artificial irradiance compared to those exposed to natural irradiance.
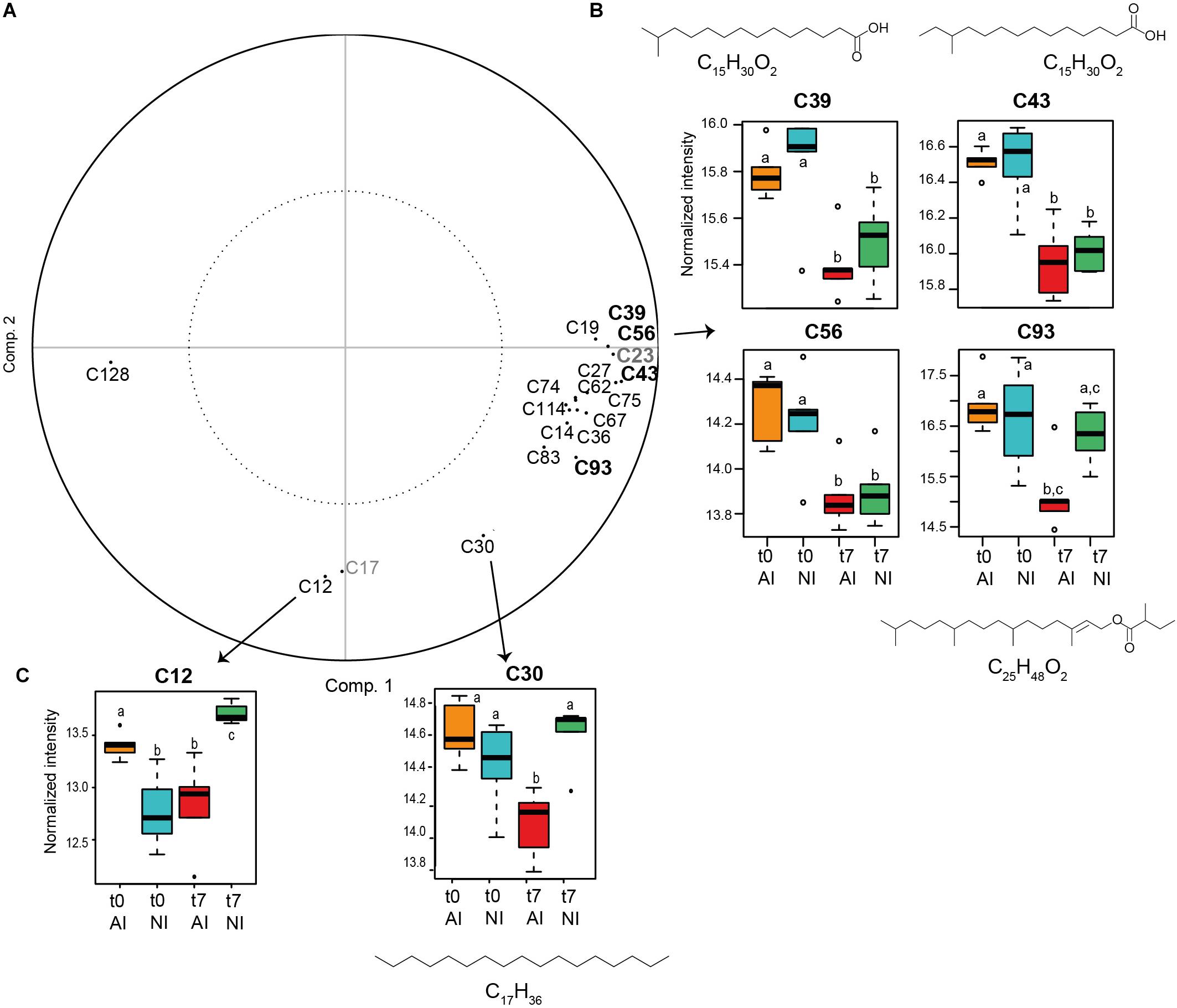
Figure 5. (A) PPLS-DA loading in the CHCl3 fraction (compounds in bold were selected with threshold = 0.8 and the others with threshold = 0.7. The two compounds in gray are plastic pollutants and were not considered). (B) Box plots of the compounds annotated in the CHCl3 fraction responsible for metabolomic differences according to time (threshold = 0.8) and (C) to the light exposure condition at t7 (threshold = 0.7) (t0: beginning and t7: end of the experiment; NI: natural irradiance; AI: higher artificial irradiance). Ion intensities of metabolites are expressed as mean normalized intensities ± SD (log-transformed data, n = 5 per group). Statistical analyses were performed using Wilcoxon signed-rank tests to compare t0 vs. t7 samples and Mann–Whitney–Wilcoxon tests to compare light treatments. Letters indicate distinct groupings based on these tests for each compounds (p < 0.05). Chemical formulas are displayed for annotated compounds.
MeOH Fractions Analyzed by GC-MS and LC-MS
After GC-MS data treatment and filtering, 85 features were isolated in the MeOH fraction (see Supplementary Table S3 and Supplementary Figure S4). As for the CHCl3 fraction, the NIST 2017 library allowed us to annotated some compounds present in the MeOH fraction of MPB biofilm (around 54%) after data analyses and filtering (Table 3 and Figure 3). A majority of fatty acids (39.1%) was also highlighted, dominated by PUFA and MUFA (15.2% each), followed by SFA (8.7%). Other compounds in smaller quantity were found and included mono- and disaccharides (8.7 and 2.2%), sterols (6.5%), terpenes (6.5%), fatty alcohols (4.3%), fatty esters (4.3%), carboxylic acids (4.3%), phenolics (4.3%), phtalic acid and phtalic acid esters (2.2 and 4.3%), a polyol (2.2%), an heterocyclic (2.2%) and an alkene (2.2%). Presumed anthropogenic contaminants from plastic origin were also identified among them, including some belonging to Polycyclic Aromatic Hydrocarbons (PAH, 6.5%).
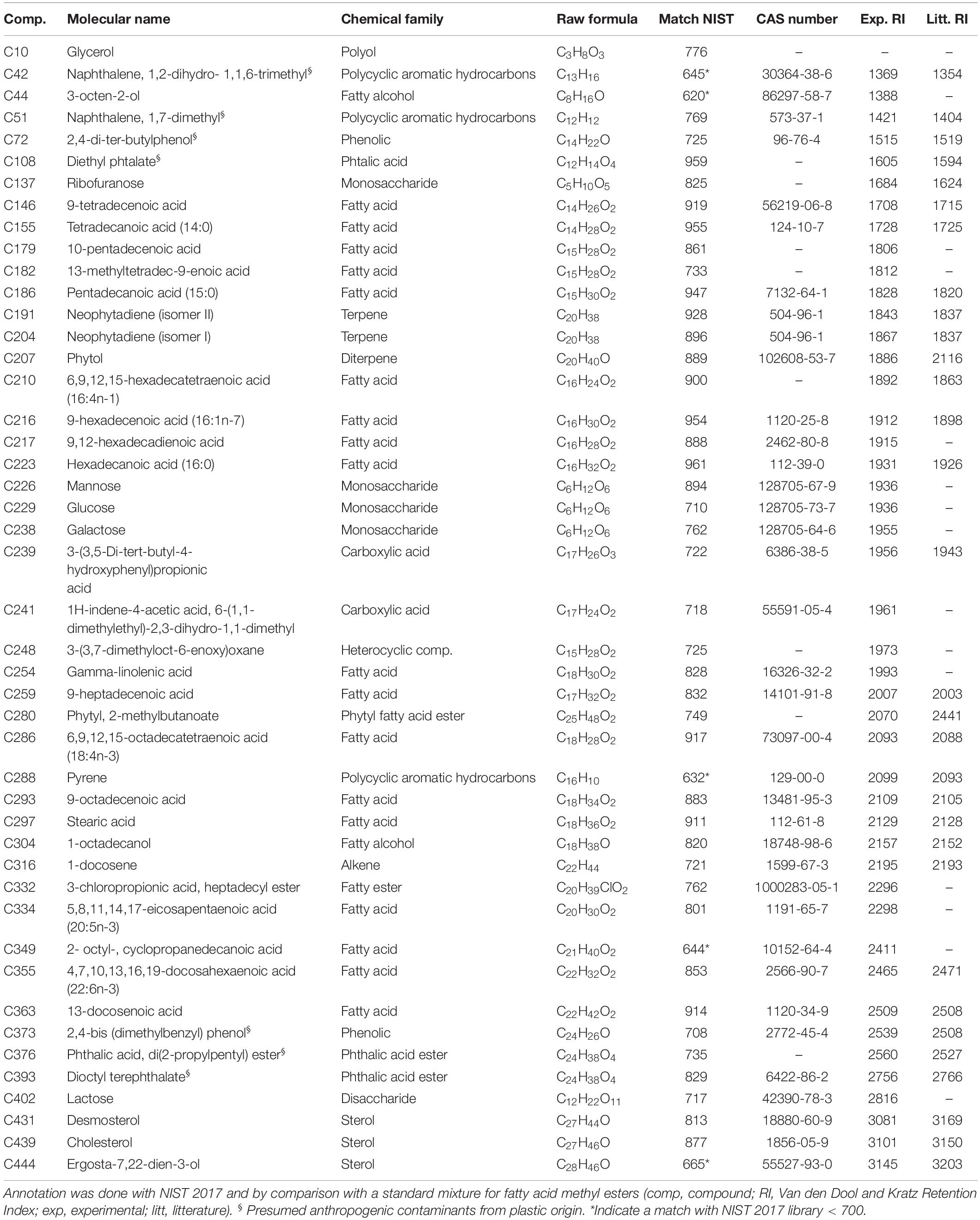
Table 3. Annotated compounds in the MeOH fraction of the MPB biofilm during the light exposure experiment (after data analyses and filtering).
The effects of light exposure and time on the metabolomic fingerprint of this MeOH fraction analyzed by GC-MS were then explored. The variance on the two first components of the PCA was explained by 73.4% (Figure 4C) and mainly due to a high intra-group variation in samples collected after 7 days of exposure to higher artificial irradiance (t7 AI). The irradiance condition was not statistically correlated with metabolomic changes in the MPB biofilm (PERMANOVA, F = 0.49, p = 0.89) neither to the time or their combination [PERMANOVA, F(time) = 2.14, p(time) = 0.06; F(time∗irradiance) = 1.70, p(time∗irradiance) = 0.13; PPLS-DA, CER = 0.619, p = 0.146, Figure 4D].
The effects of experimental conditions on the metabolomic fingerprint of MPB biofilms were finally explored in the same MeOH fraction analyzed by LC-MS. After data analyses and filtering, 2,547 features were considered in this fraction. The explained variance on axis 1–2 of the PCA was 62.65% (Figure 4E) and mainly due to a high intra-group variation in samples collected at t7 after exposure to higher artificial irradiance (t7 AI), as observed in the PCA for the same fraction analyzed by GC-MS. Only the time was correlated with metabolomic changes in this fraction (PERMANOVA, F = 2.48, p = 0.019; PPLS-DA, CER = 0.105; p = 0.001), neither the effect of irradiance or the combination of factors was statistically supported [PERMANOVA, F(irradiance) = 0.96, p(irradiance) = 0.46; F(time∗irradiance) = 0.8, p(time∗irradiance) = 0.71; PPLS-DA, CER = 0.577, p = 0.056, Figure 4F]. Unfortunately, most probable raw formula of compounds correlated to time (Supplementary Table S4) did not match with any known compounds after the construction of a molecular network with GNPS and were not unambiguously identified by annotation against ISDB, MassBank and SIRIUS 4.0.
Discussion
In this study, we detailed a protocol for untargeted metabolomic fingerprinting in MPB biofilms from mudflats. We selected a sonication-assisted extraction using organic solvents, a popular and easy to reproduce technique that has been widely applied on different types of marine samples (e.g., Fernandez-Varela et al., 2015; Bourke et al., 2017; Wilkinson et al., 2018; Gaubert et al., 2019). Three solvent extraction mixtures using different proportions of the commonly used methanol and chloroform (e.g., Kruger et al., 2008; Cajka and Fiehn, 2016; Kumar et al., 2016) have been tested. Using a biphasic mixture with a polar (MeOH + 0–33% H2O) and a non-polar (CHCl3) solvent, a wide range of compounds has been extracted, both hydrophilic and lipophilic, also increasing the molecular complexity of the MPB extracts. A good reproducibility (median RSDs < 1.5%) and the same high number of detected metabolites were equivalently obtained in the CHCl3 fraction with all mixtures. Thus, we could not use this fraction to select the most appropriate solvent mixture for metabolite extraction. Based on the MeOH fraction analyzed by GC-MS and LC-MS, the mixture 1 (MeOH/CHCl3 1:1) has been retained as it gave a large number of detected metabolites with a good reproducibility. This is in accordance with the objective of the untargeted metabolomic fingerprinting approach. The mixture 3 showed similar results but the number of metabolites detected was more variable (13.4% for M3 vs. 8.5% for M1) while not statistically supported. The mixture 2 was dismissed as the number of metabolites detected was distinctly lower compared to other mixtures. The presently described experimental set up was then applied and validated on a case study: the effect of light exposure condition on the metabolome of MBP biofilms from mudflat sediments.
MPB biofilm samples collected at t0 and t7, under natural or higher artificial irradiance, were processed and the metabolite composition was analyzed by GC-MS. Among them, 46 and 43 features (in MeOH and CHCl3 fractions, respectively) were putatively annotated based on a combinatorial matching of mass spectra and retention index. Both fractions displayed a majority of fatty acids (FA) with 12 to 24 carbon atoms among annotated compounds. This is not surprising as diatoms, one of the main components of MPB biofilm, are known for their richness in lipids (Nappo et al., 2009; Cointet et al., 2019). The presence and combination of some FA were characteristic of diatoms: 14:0, 16:0 and its metabolic derivatives 16:1n-7 and 16:4n-1 and the two PUFA 20:5n-3 (eicosapentaenoic acid, EPA) and 22:6n-3 (docosahexaenoic acid, DHA) (Dalsgaard et al., 2003; Kelly and Scheibling, 2012). The detection of 22:6n-3 and 18:4n-3 could reflect the presence of dinoflagellates (Budge and Parrish, 1998; Dalsgaard et al., 2003; Kelly and Scheibling, 2012). Fatty acids biomarkers of heterotrophic bacteria were also identified. They are composed of odd-numbered and iso- and anteiso-branched SFA and MUFA such as 15:0, 17:0, and 15:1 (e.g., 12-methyltetradecanoic acid; 13-methyltetradec-9-enoic acid; 13-methyltetradec-9-enoic acid), as well as cyclopropyl FA (Dalsgaard et al., 2003; Kelly and Scheibling, 2012).
Apart from FA, our study showed the high molecular diversity of the MPB biofilm, with numerous classes of compounds represented: alkenes, alkanes, fatty esters, terpenes, carboxylic acids, phtalic acids, heterocyclic compounds, lactones, mono- and disaccharides, sterols, fatty alcohols, phenolics and polyols. In the CHCl3 fraction, hydrocarbons (alkenes and alkanes) were the second most represented groups among annotated features. Hydrocarbons are commonly found in diatoms, bacteria and cyanobacteria (e.g., Rontani and Volkman, 2005) and are products of the biodecarboxylation of fatty acids (Stonik and Stonik, 2015). The molecular diversity of the MeOH fraction was higher, with classes of compounds ranging from polar (e.g., monosacharides) to apolar compounds (e.g., alkenes). Interestingly, we annotated a short-chained oxylipin (3-octen-2-ol) closely similar to a self-stimulating oxylipin messenger (1-octen-3-ol) inducing defense in marine algae (Chen et al., 2019). Some marine diatoms are also known to possess volatile oxylipins belonging to unsaturated and polyunsaturated aldehydes (D’Ippolito et al., 2002; Ianora et al., 2004) but we did not find any in our study. A longer fatty alcohol with 18 carbons was also found in this fraction (1-octadecanol), indicator of an algal or bacterial contribution (Shiea et al., 1991; Rontani and Volkman, 2005). The presence of the terpene phytol in both fractions was not surprising as this compound is ubiquitous. Phytol has been found in cyanobacterial mats and photosynthetic bacterial mats (Shiea et al., 1991), diatoms (Stonik and Stonik, 2015), macroalgae (Santos et al., 2015), microalgae (Mendiola et al., 2008), and coccolithophorid (Riebesell et al., 2000). Phytol is generally considered to be the most abundant acyclic isoprenoid on earth as it represents the side chain of the chlorophyll, mainly chlorophyll a (Rontani et al., 1999; Rontani and Volkman, 2003; Kraub and Vetter, 2018). In our samples, phytol may arise from the hydrolysis of chlorophyll or bacteriochlorophyll. It may also originate from diatom chloroplasts where it can be biosynthesized by the methylerythritol (MEP) pathway (Masse et al., 2004; Stonik and Stonik, 2015). Another terpene, neophytadiene, was also found in both fractions. This terpene may be a phytol degradation product (Rontani and Volkman, 2003) and has been reported in some microalgae (López-Rosales et al., 2019) or macroalgae (Santos et al., 2015) and antimicrobial properties have been associated to this compound (e.g., Ahn et al., 2016).
Moreover, we found presumed anthropogenic contaminants from plastic origin in our samples, including some belonging to Polycyclic Aromatic Hydrocarbons (PAH). This is not surprising as these compounds, notably PAH, are ubiquitous and persistent environmental contaminants found in sediments and associated waters of urbanized estuaries and coastal areas (J. Wang et al., 2016; Baali and Yahyaoui, 2019). PAH can also come from natural sources through biodegradation by microorganisms (Baali and Yahyaoui, 2019).
Focusing on the chemical changes induced by the experimental conditions, we were able to highlight some compounds specifically correlated to the light exposure condition or time in the CHCl3 fraction. Indeed, significant variations in the metabolomic fingerprinting were observed at the end of the experiment in samples exposed to natural vs. higher artificial irradiance. Only two metabolites driving these changes were highlighted, the hydrocarbon heptadecane and another unknown metabolite. The n-heptadecane is usually among the predominant hydrocarbons in cyanobacteria and cyanobacterial mats (Shiea et al., 1991; Grimalt et al., 1992; Dembitsky et al., 2001; Rontani and Volkman, 2005). It is also found in benthic diatoms, such as Cocconeis scutellum (Nappo et al., 2009). Some microalgae such as Chlamydomonas variabilis (Chlorophyceae) or Nannochloropsis sp. (Eustigmatophyceae) have the ability to synthesize heptadecanes and heptadecenes from the corresponding C18 FA by a light dependent way (Sorigué et al., 2016). As heptadecane was decreased by the end of the experiment in biofilms under AI, we may suppose that its conversion from C18 fatty acids was somehow down-regulated by our higher artificial irradiance treatment. While the function of these hydrocarbons is unknown, roles in regulating membrane properties or as cell signaling have been suggested (Sorigué et al., 2016).
Some metabolites were also correlated to metabolomic changes according to time and showed a decreased at t7. They mainly consisted of FA. Two of them were putatively annotated as branched-chain fatty acids with 15 carbons, which are, along with 15:0 and 17:0, typical of bacteria. Their decrease at t7 compared to t0 may be explained by a decrease of bacteria or their grazing by other organisms, such as bacterivorous nematodes (Hubas et al., 2010). The decrease of these compounds may also be explained by their degradation. One branched-chained SFA with 14 carbons was also identified, along with two SFA with 12 and 14 carbon atoms and two MUFA, including the 16:1n-7. An isoprenoid wax ester derived from phytol (phytyl fatty acid ester) was also decreased at t7 in higher artificial irradiance. In terrestrial plants, a large proportion of phytol and fatty acids is converted into fatty acid phytyl esters during stress or senescence in chloroplasts, to protect plant cell as free phytol shows membrane toxic properties (Lippold et al., 2014; Kraub and Vetter, 2018). In marine microorganisms, phytyl esters have been reported in dinoflagellates (Withers and Nevenzel, 1977), some microalgae species and bacteria (Rontani et al., 1999) and may serve as a potential energy storage (Rontani et al., 1999). We may therefore hypothesize that the decrease in this phytyl ester may reflect the consumption of some energy reserves. Another metabolite potentially derivated from phytol was putatively annotated as 4,8,12-trimethyltridecan-4-olide. This lactone may be a phytol degradation by-product metabolite, formed after lactonization of the isoprenoid metabolite 4-hydroxy-4,8,12-trimethyl-tridecanoic acid (Rontani et al., 1999). Only one metabolite correlated to the experimentation time increased at t7 (with the chosen threshold 0.7). This metabolite was annotated as tetracosane, a common long-chained saturated alkane found in marine microorganisms (Grimalt et al., 1992; Nappo et al., 2009; López-Rosales et al., 2019).
No significant effect of the light exposure condition was recorded in the metabolomic fingerprinting of the MeOH fraction with both GC-MS and LC-MS analyses. This result might be explained in part by our experimental conditions. Indeed, our higher artificial irradiance treatment (167 ± 23 μmol photon m–2 s–1) was relatively low when compared to the natural conditions (102 ± 19 μmol photon m–2 s–1) and compared with solar irradiance experienced in the natural habitat (up to 2,000 μmol photon m–2 s–1). Moreover, the photon flux of the natural light treatment was not constant compared to the AI condition as it depended on the natural environmental variations. This parameter might slightly influence the metabolomic variation of the biofilm and may be further taken into consideration. Only a metabolomic variation according to time was observed after LC-MS analyses in this fraction. This might be explained by the different nature of the compounds observed by LC-MS vs. GC-MS. As the experiment was short (7 days), some chemical changes may also take longer to take place in the mid-polar fraction of the MPB biofilm. It would be interesting to extent this preliminary experiment in order to get multiple time points and to test more irradiance conditions (with higher values).
Conclusion
This paper represents the first report about the metabolomic fingerprinting of MPB biofilms from mudflat sediments using an untargeted GC-MS and LC-MS metabolomic approach and will provide a baseline for further work in this area. Of the three extraction solvent mixtures tested, we concluded that using a MeOH:CHCl3 (1:1) mixture provided the best compromise. The proposed protocol, detailing steps from sample collection to data analyses, was successfully applied to a case study: the impact of light exposure condition on the metabolome of MPB biofilm. While no metabolomic change was recorded in the MeOH fraction according to light exposure conditions, significant variations in the metabolomic fingerprinting of MPB biofilm were highlighted in the apolar fraction, according to the light exposure or time. Some metabolites correlated to these changes were identified and annotated. Our study demonstrated the interest of the metabolomic approach introduced here for rapid and simultaneous detection of metabolites from various groups and their respective chemical identification using available GC-MS databases. Both selected techniques are relevant to be used in combination for a broader analysis of metabolites. With its rich database, GC-MS allows a better identification of compounds and is particularly suitable for non-polar fractions. LC-MS, highly sensitive, is more appropriate for polar, weakly polar and neutral compounds (Wang et al., 2015). The metabolomic workflow introduced here on MPB biofilms has the potential to be adapted to further ecological studies on MPB biofilms in mudflat areas and would complete classical approaches on these biofilms. We focused on a global metabolomic study of the complex MPB biofilm (i.e., with no distinction between intra- and extracellular compounds, or between the endo- and exo-metabolome), but this workflow could be applied on the EPS fractions extracted through classical approach [Dowex resin (Jahn and Nielsen, 1995) or any other extraction methods (Takahashi et al., 2009)]. As numerous studies already investigated the EPS matrices composition (notably the carbohydrate fraction, highly polar) of the MPB biofilm, our study focused on mid-polar to apolar fractions. Metabolomics could help us to further understand the influence of various environmental factors on the MPB biofilm community and to explore the chemical communication between organisms. This approach would notably be pertinent to explore the diatom migration through the sediment. While this migration is known to take place in response to tidal and endogenous rhythms (Smith and Underwood, 1998), but also in response to environmental stress, this phenomenon still remained not fully understood. We cannot exclude that this diatom migration is, at least partially, coordinated through chemical communication, an hypothesis that could be further investigated via a metabolomic approach.
Data Availability Statement
Metabolomics data have been deposited to the EMBL-EBI MetaboLights database (doi: 10.1093/nar/gks1004.PubMed) with the identifier MTBLS1378 (for LC-MS data) and MTBLS1379 (for GC-MS data). The complete LC-MS dataset can be accessed here https://www.ebi.ac.uk/metabolights/MTBLS1378 and https://www.ebi.ac.uk/metabolights/MTBLS1379 for the GC-MS dataset.
Author Contributions
CH and JG-B designed the experiments and performed MPB biofilm sample collections. JG-B carried out extractions and fractionations, analyzed metabolomic fingerprints by GC-MS and LC-MS (with SP), performed data treatment and statistical analyses, and drafted the manuscript with input from CH and SP.
Funding
This study was supported by the BIO-Tide project, funded through the 2015–2016 BiodivERsA COFUND call for research proposals, with the national funders BelSPO, FWO, ANR, and SNSF. LC-MS fingerprints were acquired at the MNHN Bioorganic Mass Spectrometry Platform. The post-doctoral grant of JG-B was supported by the Regional Council of Brittany, SAD program and the META-Tide project. The Regional Council of Brittany, the General Council of Finistère, the urban community of Concarneau Cornouaille Agglomération and the European Regional Development Fund (ERDF) are acknowledged for the funding of the Sigma 300 FE-SEM of the Concarneau Marine Biology Station. MEB images were acquired thanks to the HULK project (FCT grant number 143255).
Conflict of Interest
The authors declare that the research was conducted in the absence of any commercial or financial relationships that could be construed as a potential conflict of interest.
Acknowledgments
We thank Elisabeth Nezan (Ifremer Concarneau) for the identification of Gyrosigma balticum and Pleurosigma formosum.
Supplementary Material
The Supplementary Material for this article can be found online at: https://www.frontiersin.org/articles/10.3389/fmars.2020.00250/full#supplementary-material
Footnotes
References
Ahn, H. M., Kim, S., Hyun, S., Lim, S. R., Kim, H., Oh, J., et al. (2016). Effects of the timing of a culture temperature reduction on the comprehensive metabolite profiles of Chlorella vulgaris. J. Appl. Phycol. 28, 2641–2650. doi: 10.1007/s10811-016-0817-4
Allard, P., Péresse, T., Bisson, J., Gindro, K., Pham, V. C., Roussi, F., et al. (2016). Integration of molecular networking and In-Silico MS/MS fragmentation for natural products dereplication. Anal. Chem. 88, 3317–3323. doi: 10.1021/acs.analchem.5b04804
Baali, A., and Yahyaoui, A. (2019). Polycyclic Aromatic Hydrocarbons (PAHs) and Their Influence to Some Aquatic Species. London: IntechOpen, doi: 10.5772/intechopen.86213
Beale, D. J., Pinu, F. R., Kouremenos, K. A., Poojary, M. M., Narayana, V. K., Boughton, B. A., et al. (2018). Review of recent developments in GC – MS approaches to metabolomics-based research. Metabolomics 14:152. doi: 10.1007/s11306-018-1449-2
Böcker, S., and Dührkop, K. (2016). Fragmentation trees reloaded. J. Cheminform. 8:5. doi: 10.1186/s13321-016-0116-8
Bohórquez, J., McGenity, T. J., Papaspyrou, S., García-Robledo, E., Corzo, A., and Underwood, G. J. C. (2017). Different types of diatom-derived extracellular polymeric substances drive changes in heterotrophic bacterial communities from intertidal sediments. Front. Microbiol. 8:245. doi: 10.3389/fmicb.2017.00245
Bourke, M. F., Marriott, P. J., Glud, R. N., Hasler-Sheetal, H., Kamalanathan, M., Beardall, J., et al. (2017). Metabolism in anoxic permeable sediments is dominated by eukaryotic dark fermentation. Nat. Geosci. 10, 30–35. doi: 10.1038/ngeo2843
Bromke, M. A., Sabir, J. S., Alfassi, F. A., Hajarah, N. H., Kabli, S. A., Al-Malki, A. L., et al. (2015). Metabolomic profiling of 13 diatom cultures and their adaptation to nitrate-limited growth conditions. PLoS One 10:e0138965. doi: 10.1371/journal.pone.0138965
Budge, S. M., and Parrish, C. C. (1998). Lipid biogeochemistry of plankton, settling matter and sediments in Trinity Bay, Newfoundland. II. Fatty acids. Org. Geochem. 29, 1547–1559. doi: 10.1016/s0146-6380(98)00177-6
Bundy, J. G., Davey, M. P., Viant, M. R., Davey, ÆM. P., and Viant, M. R. (2009). Environmental metabolomics: a critical review and future perspectives. Metabolomics 5, 3–21. doi: 10.1007/s11306-008-0152-0
Cajka, T., and Fiehn, O. (2016). Toward merging untargeted and targeted methods in mass spectrometry-based metabolomics and lipidomics. Anal. Chem. 88, 524–545. doi: 10.1021/acs.analchem.5b04491
Chambers, M. C., Maclean, B., Burke, R., Amodei, D., Ruderman, D. L., Neumann, S., et al. (2012). A cross-platform toolkit for mass spectrometry and proteomics. Nat. Biotechnol. 30, 918–920. doi: 10.1038/nbt.2377
Chen, H., Yang, R., Chen, J., Luo, Q., Cui, X., Yan, X., et al. (2019). 1-Octen-3-ol, a self-stimulating oxylipin messenger, can prime and induce defense of marine alga. BMC Plant Biol. 19:37. doi: 10.1186/s12870-019-1642-0
Chung, H. C., Lee, O. O., Huang, Y., Mok, S. Y., and Kolter, R. (2010). Bacterial community succession and chemical profiles of subtidal biofilms in relation to larval settlement of the polychaete Hydroides elegans. ISME J. 4, 817–828. doi: 10.1038/ismej.2009.157
Cointet, E., Wielgosz-collin, G., Méléder, V., and Gonçalves, O. (2019). Lipids in benthic diatoms: a new suitable screening procedure. Algal Res. 39:101425. doi: 10.1016/j.algal.2019.101425
Dalsgaard, J., St John, M., Kattner, G., Müller-Navarra, D., and Hagen, W. (2003). Fatty acid trophic markers in the pelagic marine environment. Adv. Mar. Biol. 46, 225–340. doi: 10.1016/s0065-2881(03)46005-7
Decho, A. W. (2000). Microbial biofilms in intertidal systems: an overview. Cont. Shelf Res. 20, 1257–1273. doi: 10.1016/s0278-4343(00)00022-4
Dembitsky, V. M., Dor, I., Shkrob, I., and Aki, M. (2001). Branched alkanes and other apolar compounds produced by the Cyanobacterium Microcoleus vaginatus from the Negev Desert. Russ. J. Bioorganic Chem. 27, 110–119.
D’Ippolito, G., Iadicicco, O., Romano, G., and Fontana, A. (2002). Detection of short-chain aldehydes in marine organisms: the diatom Thalassiosira rotula. Tetrahedron Lett. 43, 6137–6140. doi: 10.1016/s0040-4039(02)01283-2
Domingo-almenara, X., Brezmes, J., Vinaixa, M., Samino, S., Ramirez, N., Ramon-, M., et al. (2016). eRah: a computational tool integrating spectral deconvolution and alignment with quantification and identification of metabolites in GC-MS-based metabolomics. Anal. Bioanal. Chem. 88, 9821–9829. doi: 10.1021/acs.analchem.6b02927
Dove, A. D. M., Leisen, J., Zhou, M., Byrne, J. J., Lim-hing, K., Webb, H. D., et al. (2012). Biomarkers of whale shark health: a metabolomic approach. PLoS One 7:e49379. doi: 10.1371/journal.pone.0049379
Elias, S., and Banin, E. (2012). Multi-species biofilms: living with friendly neighbors. FEMS Microbiol. Rev. 36, 990–1004. doi: 10.1111/j.1574-6976.2012.00325.x
Fernandez-Varela, R., Tomasi, G., and Christensen, J. (2015). An untargeted gas chromatography mass spectrometry metabolomics platform for marine polychaetes. J. Chromatogr. A 1384, 133–141. doi: 10.1016/j.chroma.2015.01.025
Fiehn, O. (2002). Metabolomics–the link between genotypes and phenotypes. Plant Mol. Biol 48, 155–171. doi: 10.1007/978-94-010-0448-0_11
Flemming, H. (2016). Biofilms: an emergent form of bacterial life. Nat. Publ. Gr. 14, 563–575. doi: 10.1038/nrmicro.2016.94
Flemming, H. C., and Wingender, J. (2010). The biofilm matrix. Nat. Rev. Microbiol. 8, 623–633. doi: 10.1038/nrmicro2415
Gaillard, F., and Potin, P. (2014). “Proteomics and metabolomics of marine organisms: current strategies and knowledge Fanny Gaillard and Philippe Potin abstract,” in Outstanding Marine Molecules: Chemistry, Biology, Analysis, eds S. La Barre and J. M. Kornprobst (Weinheim: Wiley-VCH Verlag GmbH & Co. KGaA), 457–472. doi: 10.1002/9783527681501.ch21
Gaubert, J., Greff, S., Thomas, O. P., and Payri, C. E. (2019). Metabolomic variability of four macroalgal species of the genus Lobophora using diverse approaches. Phytochemistry 162, 165–172. doi: 10.1016/j.phytochem.2019.03.002
Gillard, J., Frenkel, J., Devos, V., Sabbe, K., Paul, C., Rempt, M., et al. (2013). Metabolomics enables the structure elucidation of a diatom sex pheromone. Angew. Chemie Int. Ed. 52, 854–857. doi: 10.1002/anie.201208175
Grimalt, J., Wit, R., Teixidor, P., and Albaiges, J. (1992). Lipid biogeochemistry of Phormidium and Microcoleus mats. Adv. Org. Geochem. 19, 509–530. doi: 10.1016/0146-6380(92)90015-p
Hanlon, A. R. M., Bellinger, B., Haynes, K., Xiao, G., and Hofmann, T. A. (2006). Dynamics of extracellular polymeric substance (EPS) production and loss in an estuarine, diatom-dominated, microalgal biofilm over a tidal emersion – immersion period. Limnol. Oceanogr. 51, 79–93. doi: 10.4319/lo.2006.51.1.0079
Haro, S., Brodersen, K. E., and Papaspyrou, S. (2019). Radiative energy budgets in a microbial mat under different irradiance and tidal conditions. Microb. Ecol. 77, 852–865. doi: 10.1007/s00248-019-01350-6
Hubas, C., Passarelli, C., and Paterson, D. M. (2018). “Microphytobenthic biofilms: composition and interactions,” in Mudflat Ecology. Aquatic Ecology Series, Vol. 7, ed. P. G. Beninger (Cham: Springer), 63–90. doi: 10.1007/978-3-319-99194-8_4
Hubas, C., Sachidhanandam, C., Rybarczyk, H., Lubarsky, H. V., Rigaux, A., Moens, T., et al. (2010). Bacterivorous nematodes stimulate microbial growth and exopolymer production in marine sediment microcosms. Mar. Ecol. Prog. Ser. 419, 85–94. doi: 10.3354/meps08851
Ianora, A., Miralto, A., Poulet, S. A., Carotenute, Y., Buttino, T., Romano, G., et al. (2004). Aldehyde suppression of copepod recruitment in blooms of a ubiquitous planktonic diatom. Nature 429, 403–407. doi: 10.1038/nature02565.1
Jahn, A., and Nielsen, P. H. (1995). Extraction of extracellular polymeric substances (EPS) from biofilms using a cation exchange resin. Pergamon 32, 157–164. doi: 10.2166/wst.1995.0287
Juneau, P., Barnett, A., Méléder, V., Dupuy, C., and Lavaud, J. (2015). Combined effect of high light and high salinity on the regulation of photosynthesis in three diatom species belonging to the main growth forms of intertidal fl at inhabiting microphytobenthos. J. Exp. Mar. Bio. Ecol. 463, 95–104. doi: 10.1016/j.jembe.2014.11.003
Kelly, J. R., and Scheibling, R. E. (2012). Fatty acids as dietary tracers in benthic food webs. Mar. Ecol. Prog. Ser. 446, 1–22. doi: 10.3354/meps09559
Kliebenstein, D. J. (2004). Secondary metabolites and plant / environment interactions: a view through Arabidopsis thaliana tinged glasses. Plant Cell Environ. 27, 675–684. doi: 10.1111/j.1365-3040.2004.01180.x
Kooke, R., and Keurentjes, J. J. B. (2011). Multi-dimensional regulation of metabolic networks shaping plant development and performance. J. Exp. Bot. 63, 3353–3365. doi: 10.1093/jxb/err373
Kraub, S., and Vetter, W. (2018). Phytol and phytyl fatty acid esters: occurrence, concentrations and relevance. Eur. J. Lipid Sci. Technol. 120:1700387. doi: 10.1002/ejlt.201700387
Kruger, N. J., Troncoso-ponce, M. A., and Ratcliffe, R. G. (2008). 1H NMR metabolite fingerprinting and metabolomic analysis of perchloric acid extracts from plant tissues. Nat. Protoc. 3, 1001–1012. doi: 10.1038/nprot.2008.64
Kumar, M., Kuzhiumparambil, U., Pernice, M., Jiang, Z., and Ralph, P. J. (2016). Metabolomics: an emerging frontier of systems biology in marine macrophytes. Algal Res. 16, 76–92. doi: 10.1016/j.algal.2016.02.033
Lépinay, A., Turpin, V., Mondeguer, F., Grandet-Marchant, Q., Capiaux, H., Baron, R., et al. (2018). First insight on interactions between bacteria and the marine diatom Haslea ostrearia: algal growth and metabolomic fingerprinting. Algal Res. 31, 395–405. doi: 10.1016/j.algal.2018.02.023
Li, H., Lu, Y., Zheng, J., Yang, W., and Liu, J. (2014). Biochemical and genetic engineering of diatoms for polyunsaturated fatty acid biosynthesis. Mar. Drugs 12, 153–166. doi: 10.3390/md12010153
Liebeke, M., and Puskás, E. (2019). Drying enhances signal intensities for global GC – MS metabolomics. Metabolites 9:68. doi: 10.3390/metabo9040068
Lippold, F., Dorp, K., Abraham, M., Hölzl, G., Wewer, V., Yilmaz, L., et al. (2014). Fatty acid phytyl ester synthesis in chloroplasts of Arabidopsis. Plant Cell 24, 2001–2014. doi: 10.1105/tpc.112.095588
López-Rosales, A. R., Ancona-Canché, K., Chavarria-Hernandez, J. C., Barahona-Pérez, F., Toledano-Thompson, T., Garduño-Solórzano, G., et al. (2019). Fatty acids, hydrocarbons and terpenes of nannochloropsis and nannochloris isolates with potential for biofuel production. Energies 12:130. doi: 10.3390/en12010130
Masse, G., Belt, S. T., Rowland, S. J., and Rohmer, M. (2004). Isoprenoid biosynthesis in the diatoms Rhizosolenia setigera (Brightwell) and Haslea ostrearia (Simonsen). Proc. Natl. Acad. Sci. U.S.A. 101, 4413–4418. doi: 10.1073/pnas.0400902101
Mendiola, J. A., Santoyo, S., Cifuentes, A., Reglero, G., and Sen, F. J. (2008). Antimicrobial activity of sub- and supercritical CO 2 extracts of the green alga Dunaliella salina. J. Food Prot. 71, 2138–2143. doi: 10.4315/0362-028x-71.10.2138
Nappo, M., Berkov, S., Codina, C., Avila, C., Messina, P., Zupo, V., et al. (2009). Metabolite profiling of the benthic diatom Cocconeis scutellum by GC-MS. J. Appl. Phycol. 21, 295–306. doi: 10.1007/s10811-008-9367-8
Parsons, H. M., Ekman, D. R., Collette, W., and Viant, M. R. (2009). Spectral relative standard deviation: a practical benchmark in metabolomics. Analyst 134, 478–485. doi: 10.1039/b808986h
Passarelli, C., Meziane, T., Thiney, N., Boeuf, D., Jesus, B., Ruivo, M., et al. (2015). Seasonal variations of the composition of microbial biofilms in sandy tidal flats: focus of fatty acids, pigments and exopolymers. Estuar. Coast. Shelf Sci. 153, 29–37. doi: 10.1016/j.ecss.2014.11.013
Paul, C., Mausz, M. A., and Pohnert, G. (2013). A co-culturing/metabolomics approach to investigate chemically mediated interactions of planktonic organisms reveals influence of bacteria on diatom metabolism. Metabolomics 9, 349–359. doi: 10.1007/s11306-012-0453-1
Perkins, R. G., Lavaud, J., Serôdio, J., Mouget, J. L., Cartaxana, P., Rosa, P., et al. (2010). Vertical cell movement is a primary response of intertidal benthic biofilms to increasing light dose. Mar. Ecol. Prog. Ser. 416, 93–103. doi: 10.3354/meps08787
Perkins, R. G., Underwood, G. J. C., Brotas, V., Snow, G. C., Jesus, B., and Ribeiro, L. (2001). Responses of microphytobenthos to light: primary production and carbohydrate allocation over an emersion period. Mar. Ecol. Prog. Ser. 223, 101–112. doi: 10.3354/meps223101
Pierre, G., Graber, M., Orvain, F., Dupuy, C., and Maugard, T. (2010). Biochemical characterization of extracellular polymeric substances extracted from an intertidal mudflat using a cation exchange resin. Biochem. Syst. Ecol. 38, 917–923. doi: 10.1016/j.bse.2010.09.014
Pierre, G., Zhao, J. M., Orvain, F., Dupuy, C., Klein, G. L., Graber, M., et al. (2014). Seasonal dynamics of extracellular polymeric substances (EPS) in surface sediments of a diatom-dominated intertidal mudflat (Marennes-Oléron, France). J. Sea Res. 92, 26–35. doi: 10.1016/j.seares.2013.07.018
Riebesell, U., Revill, A. T., Holdsworth, D. G., and Volkman, J. K. (2000). The effects of varying CO 2 concentration on lipid composition and carbon isotope fractionation in Emiliania huxleyi. Geochim. Cosmochim. Acta 64, 4179–4192. doi: 10.1016/s0016-7037(00)00474-9
Roessner, U., Wagner, C., Kopka, J., Trethewey, R. N., and Willmitzer, L. (2000). Simultaneous analysis of metabolites in potato tuber by gas chromatography-mass spectrometry. Plant J. 23, 131–142. doi: 10.1046/j.1365-313x.2000.00774.x
Rontani, J., Bonin, P. C., and Volkman, J. K. (1999). Production of wax esters during aerobic growth of marine bacteria on isoprenoid compounds. Appl. Environ. Microbiol. 65, 221–230. doi: 10.1128/aem.65.1.221-230.1999
Rontani, J., and Volkman, J. K. (2003). Phytol degradation products as biogeochemical tracers in aquatic environments. Org. Geochem. 34, 1–35. doi: 10.1016/s0146-6380(02)00185-7
Rontani, J. F., and Volkman, J. K. (2005). Lipid characterization of coastal hypersaline cyanobacterial mats from the Camargue (France). Org. Geochem. 36, 251–272. doi: 10.1016/j.orggeochem.2004.07.017
Santos, S. A. O., Vilela, C., Freire, C. S. R., Abreu, M. H., Rocha, S. M., and Silvestre, A. J. D. (2015). Chlorophyta and Rhodophyta macroalgae: a source of health promoting phytochemicals. Food Chem. 183, 122–128. doi: 10.1016/j.foodchem.2015.03.006
Shannon, P., Markiel, A., Ozier, O., Baliga, N. S., Wang, J. T., Ramage, D., et al. (2003). Cytoscape: a software environment for integrated models of biomolecular interaction networks. Genome Res. 13, 2498–2504. doi: 10.1101/gr.1239303.metabolite
Shiea, J., Brassell, S., and Ward, D. (1991). Comparative analysis of extractable lipids in hot spring microbial mats and their component photosynthetic bacteria. Org. Geochem. 17, 309–319. doi: 10.1016/0146-6380(91)90094-z
Smith, D. J., and Underwood, G. J. C. (1998). Exopolymer production by intertidal epipelic diatoms. Limnol. Oceanogr. 43, 1578–1591. doi: 10.4319/lo.1998.43.7.1578
Sogin, E. M., Puskás, E., Dubilier, N., and Liebeke, M. (2019). Marine metabolomics: measurement of metabolites in seawater by gas chromatography mass spectrometry. bioRxiv [Preprint]. doi: 10.1101/528307
Sorigué, D., Légeret, B., Cuiné, S., Morales, P., Mirabella, B., Guédeney, G., et al. (2016). Microalgae synthesize hydrocarbons from long-chain fatty acids via a light-dependent pathway 1 [OPEN]. Plant Physiol. 171, 2393–2405. doi: 10.1104/pp.16.00462
Stal, L. J. (2003). Microphytobenthos, their extracellular polymeric substances, and the morphogenesis of intertidal sediments. Geomicrobiol. J. 20, 463–478. doi: 10.1080/713851126
Stonik, V., and Stonik, I. (2015). Low-molecular-weight metabolites from diatoms: structures. Biological roles and biosynthesis. Mar. Drugs 13, 3672–3709. doi: 10.3390/md13063672
Sutherland, I. W. (2017). “EPS – a complex mixture,” in The Perfect Slime: Microbial Extracellular Polymeric Substances (EPS), eds H. Flemming, T. Neu, and J. Wingender (London: IWA Publishing), 15–24.
Takahashi, E., Ledauphin, J., Goux, D., and Orvain, F. (2009). Optimising extraction of extracellular polymeric substances (EPS) from benthic diatoms: comparison of the efficiency of six EPS extraction methods. Mar. Freshw. Res. 60, 1201–1210. doi: 10.1071/MF08258
Toupoint, N., Mohit, V., Linossier, I., Bourgougnon, N., Olivier, F., Lovejoy, C., et al. (2012). Effect of biofilm age on settlement of Mytilus edulis. Biofouling 28, 985–1001. doi: 10.1080/08927014.2012.725202
Underwood, G. J. C., and Kromkamp, J. (1999). “Primary production by phytoplankton and microphytobenthos in Estuaries,” in Advances in Ecological Research Estuaries, eds A. H. Fitter and D. G. Raffaelli (Cambridge, MA: Academic Press), 93–139.
Underwood, G. J. C., and Paterson, M. D. (2003). The importance of extracellular carbohydrate production by marine epipelic diatoms. Adv. Bot. Res. 40, 183–240. doi: 10.1016/s0065-2296(05)40005-1
Van Den Dool, H., and Kratz, P. D. (1963). A generalization of the retention index system including linear temperature programmed gas-liquid partition chromatography. J. Chromatogr. 11, 463–471. doi: 10.1016/s0021-9673(01)80947-x
Wang, J., Tan, Z., Peng, J., Qiu, Q., and Li, M. (2016). The behaviors of microplastics in the marine environment. Mar. Environ. Res. 113, 7–17. doi: 10.1016/j.marenvres.2015.10.014
Wang, M., Carver, J. J., Phelan, L. M., Sanchez, L. M., Garg, N., and Al, E. (2016). Sharing and community curation of mass spectrometry data with global natural products social molecular networking. Nat. Biotechnol. 34, 828–837. doi: 10.1038/nbt.3597
Wang, Y., Liu, S., Hu, Y., Li, P., and Wan, J. B. (2015). Current state-of-the-art of mass spectrometry-based metabolomics studies – a review focusing on wide coverage, high throughput and easy identification. R. Soc. Chem. 5, 78728–78737. doi: 10.1039/C5RA14058G
Wilkinson, J. L., Hooda, P. S., Swinden, J., Barker, J., and Barton, S. (2018). Spatial (bio) accumulation of pharmaceuticals, illicit drugs, plasticisers, perfluorinated compounds and metabolites in river sediment, aquatic plants and benthic organisms. Environ. Pollut. 234, 864–875. doi: 10.1016/j.envpol.2017.11.090
Withers, N., and Nevenzel, J. (1977). Phytyl esters in a marine dinoflagellate. Lipids 12, 989–993. doi: 10.1007/bf02533324
Keywords: microphytobenthos, biofilms, mudflats, metabolomics, diatoms
Citation: Gaubert-Boussarie J, Prado S and Hubas C (2020) An Untargeted Metabolomic Approach for Microphytobenthic Biofilms in Intertidal Mudflats. Front. Mar. Sci. 7:250. doi: 10.3389/fmars.2020.00250
Received: 19 November 2019; Accepted: 30 March 2020;
Published: 22 April 2020.
Edited by:
João Serôdio, University of Aveiro, PortugalReviewed by:
Carina Rafaela Faria Da Costa Félix, Polytechnic Institute of Leiria, PortugalEtelvina Figueira, University of Aveiro, Portugal
Copyright © 2020 Gaubert-Boussarie, Prado and Hubas. This is an open-access article distributed under the terms of the Creative Commons Attribution License (CC BY). The use, distribution or reproduction in other forums is permitted, provided the original author(s) and the copyright owner(s) are credited and that the original publication in this journal is cited, in accordance with accepted academic practice. No use, distribution or reproduction is permitted which does not comply with these terms.
*Correspondence: Julie Gaubert-Boussarie, anVsaWVnMTkwN0BnbWFpbC5jb20=