- 1Tvärminne Zoological Station, University of Helsinki, Hanko, Finland
- 2Baltic Sea Centre, Stockholm University, Stockholm, Sweden
Functional diversity (FD) experiments are highly effective for investigating how a community interacts with its environment. However, such experiments using morphological and chemical traits have not been conducted for submerged aquatic plants and their insights would be highly valuable for understanding the ecology of these communities. We conducted a 15-week field experiment in the Baltic Sea where we manipulated the species composition of aquatic plant communities to investigate functional diversity. We constructed artificial triculture communities with different species compositions to change the Community Weighted Means (CWMs) and variability of traits. We measured nine plant traits and tested how community productivity (CP) was related to FD, trait CWMs and community trait ranges. CP varied by more than four times across treatments and functional richness was significantly related to CP. Functional evenness and functional divergence were not significantly related to CP. Height, leaf area and δ13C were significantly related to CP. Leaf δ13C trends with CP suggested that the carbon supply is not replete, yet species composition was partly responsible for the relationship. Plant height likely had multifaceted benefits to CP because there was evidence of a competitive height interaction between the tallest and 2nd tallest species, therefore the effects of plant height to CP would have been disproportionally large. The height of the tallest species significantly drove the variability of the community height range, which was significantly related to CP and it had a relatively large influence on the calculation of FD indices. Leaf area, which was strongly correlated to plant height, was also significantly related to CP. The significant relationship between functional richness and CP was most likely driven by the presence of taller plants. FD likely enhanced CP, by selecting for extreme trait values which enhanced production (selection effect), while niche complementarity effects were not observed. This study provides experimental evidence and mechanistic insights into the role of FD and specific traits for CP in submerged aquatic plant communities. To conclude, FD was significantly related to CP of temperate aquatic plant communities likely by selecting for traits which enhanced light capture, with consequences for carbon supply.
Introduction
Functional diversity experiments are powerful tools for understanding how an ecosystem works (Tilman et al., 1997; Díaz and Cabido, 2001; Hooper et al., 2005; Mouillot et al., 2013; Gagic et al., 2015). Not only do they inform us about functional diversity indices, but the volume of traits measured allows us to explore multiple trait–process and trait–trait relationships (McGill et al., 2006; Cadotte et al., 2011). This provides an in-depth understanding of how the study organisms interact with their environment (Hooper and Vitousek, 1997; Tilman et al., 1997; Díaz and Cabido, 2001; Hooper et al., 2005; Gagic et al., 2015).
Plant functional traits are measurable morpho-physio-phenological characteristics of a plant which can improve its performance of processes linked to its fitness, for example increased biomass production (Violle et al., 2007). It is likely that functional traits strongly influence ecosystem properties, as suggested by a consensus of literature (Hooper et al., 2005). They can support the biomass production of plants by many potential mechanisms (Pérez-Harguindeguy et al., 2013), for example by enhancing their ability to capture light (Díaz et al., 2004; Gustafsson and Norkko, 2019), to compete for resources against other plants (Gaudet and Keddy, 1988; Cadotte, 2017), or by increasing their access to nutrient pools (Campbell et al., 1991; Kembel et al., 2008; Angove et al., 2018). These traits constitute the foundation of functional diversity, because their measurements are combined to calculate functional diversity indices. Indeed, the definition of functional diversity is the performance variability of different functions (Garnier et al., 2016), such as the combined performance of light capture and nutrient uptake. It is important to investigate functional diversity because it provides mechanistic insights into the relationships between plant diversity and ecosystem processes, which contributes to the practical management of vegetated ecosystems (Díaz and Cabido, 2001). Functional diversity can increase primary productivity by, for example, selecting for extreme trait values which enhance productivity (selection effects) or by a complementarity of fundamental niches (complementarity effects) (Loreau and Hector, 2001). Functional ecology is an enrichment to traditional taxonomic ecology because it allows us to investigate the underlying mechanisms for diversity effects to processes (e.g., Violle et al., 2007). For example, species richness affects productivity in temperate submerged aquatic plant meadows (Salo et al., 2009; Gustafsson and Boström, 2011), as can functional traits (Gustafsson and Norkko, 2019) and perhaps these functional traits can be used to explain species effects (e.g., Hillebrand and Matthiessen, 2009).
Aquatic plant meadows of the northern Baltic Sea are temperate communities with typical seasonal patterns of pronounced summer growth (Boström et al., 2004; Attard et al., 2019). The brackish seawater conditions allow for marine, brackish and limnic species to coincide within the same meadow (Kautsky, 1988), therefore meadows can be highly diverse communities with a variety of different plant traits (Kautsky, 1988; Gustafsson and Norkko, 2016, 2019; Angove et al., 2018). Such a diversity of traits provides an opportunity to conduct a functional diversity experiment to understand how aquatic plants interact with their environment. Previous functional diversity research for aquatic plants has consisted of surveys along environmental gradients (e.g., Fu et al., 2014; Gustafsson and Norkko, 2019) and controlled mesocosm experiments (e.g., Engelhardt and Ritchie, 2002). However, to our knowledge, no experiment has yet manipulated species composition to investigate functional diversity in situ. We conducted a functional diversity experiment in situ to investigate how the functional diversity of submerged aquatic plants can be linked to their primary production. When conducting functional diversity experiments, it is necessary to understand the environmental context to select plant traits which are most likely to affect the process being measured (Petchey and Gaston, 2006). Two of the most limiting resources conventionally known to affect aquatic plant growth are light and nutrient availability (Lee et al., 2007). Light intensity can affect the depth limit of aquatic plants (Ralph et al., 2007). Accordingly, the distribution of plants can shift from turbid, nutrient-rich waters to clearer, relatively nutrient-poor waters to favor light capture (Krause-Jensen et al., 2008). Indeed, plant height is significantly related to productivity in temperate plant meadows (Gustafsson and Norkko, 2019). A reduction of light availability, such as by shading, can lead to reduced growth, changes in the biomass investment for light capture, mortality and/or changes in community properties such as shoot density (Longstaff and Dennison, 1999; Ruiz and Romero, 2001; Boström et al., 2004; Gustafsson and Boström, 2013; Salo et al., 2015). Nutrient availability can also affect the growth of aquatic plants (Perez et al., 1991; Ferdie and Fourqurean, 2004; Armitage et al., 2011), as well as the expansion of meadows (Furman et al., 2017) and it can dictate how much biomass plants invest for capturing light (Maurer and Zedler, 2002). Aquatic plants absorb nutrients from the sediment porewater and the water column (Touchette and Burkholder, 2000), and the sediment nutrient source is spatio-temporally variable and finite (Angove et al., 2018). Even in eutrophic environments such as the northern Baltic Sea (Andersen et al., 2009; Gustafsson et al., 2012), plants can deplete nutrients from their surrounding sediments in response to biomass-driven demands (Angove et al., 2018). Therefore, both light and nutrient availability are viable candidates which could limit productivity in temperate aquatic plant communities (Boström et al., 2004; Lee et al., 2007). Resultantly, traits which are linked to light capture and nutrient uptake could be influential to biomass production by submerged aquatic plants.
We investigated the role of plant traits and functional diversity for community biomass production by conducting a 15-week transplant experiment in situ. By comparing the relationships between plant traits, functional diversity and biomass production, we could provide novel mechanistic insights about the role of different traits and drivers for the productivity of submerged aquatic plant communities. Previous evidence shows that communities with taller plants are significantly more productive (Fu et al., 2014; Gustafsson and Norkko, 2019). Evidence also shows that resource partitioning might allow submerged aquatic plants to utilize the sediment nutrient pool more effectively (Gustafsson and Boström, 2011). In such conditions, traits which represent nutrient metabolism and sourcing by plants, e.g., leaf tissue δ15N, might be superseded by morphological traits to access nutrients; such as root length (Aerts, 1999). Therefore, we hypothesized that aquatic plant community biomass production would be closely related to plant height and root length range, but not to leaf chemical traits such as leaf tissue δ15N and δ13C (H1). Because of such trait variability effects, we hypothesized that plant communities with a higher functional diversity would produce more biomass (H2).
Materials and Methods
Study Area and Field Experiment
We conducted the experiment in the shallow archipelago areas of the northern Baltic Sea; our experimental site, Kyan (59.827415, 23.209903 WGS84), is a brackish-water (salinity ca. 5.6 PSU, Gammal et al., 2019) semi-exposed lagoon located on the island Vindskären, Tvärminne archipelago, southern Finland. It is a coarse-sand environment with a relatively low organic matter (OM) content [silt fraction (<0.63 μm) = 0.43%, OM = 0.54%; Gammal et al., 2019]. It is protected from the open Baltic Sea by a partly-submerged rock covered by bladderwrack, Fucus vesiculosus. Its moderate levels of wave exposure allowed submerged plant communities to thrive (Worm and Reusch, 2000; Gustafsson and Norkko, 2019). The lagoon is inhabited by a diverse and mixed community of submerged aquatic plants (Gustafsson and Norkko, 2019, Figure 1A) and we selected naturally bare sand patches (4 m × 8 m) amongst these communities at approximately 2.5 m depth to conduct the experiment. We conducted a transplant experiment in situ by SCUBA diving (e.g., Salo et al., 2009; Gustafsson and Boström, 2011). We defined six experimental zones (8 m × 4 m) within the pre-selected bare patches. Within these patches, we cleared the sand of lone shoots and buried rhizomes then marked out plots, which were evenly spaced and separated by at least 1 m. In each bare patch, we planted three experimental functional diversity communities (total 18 communities). We commenced the experiment on 01/06/2016.
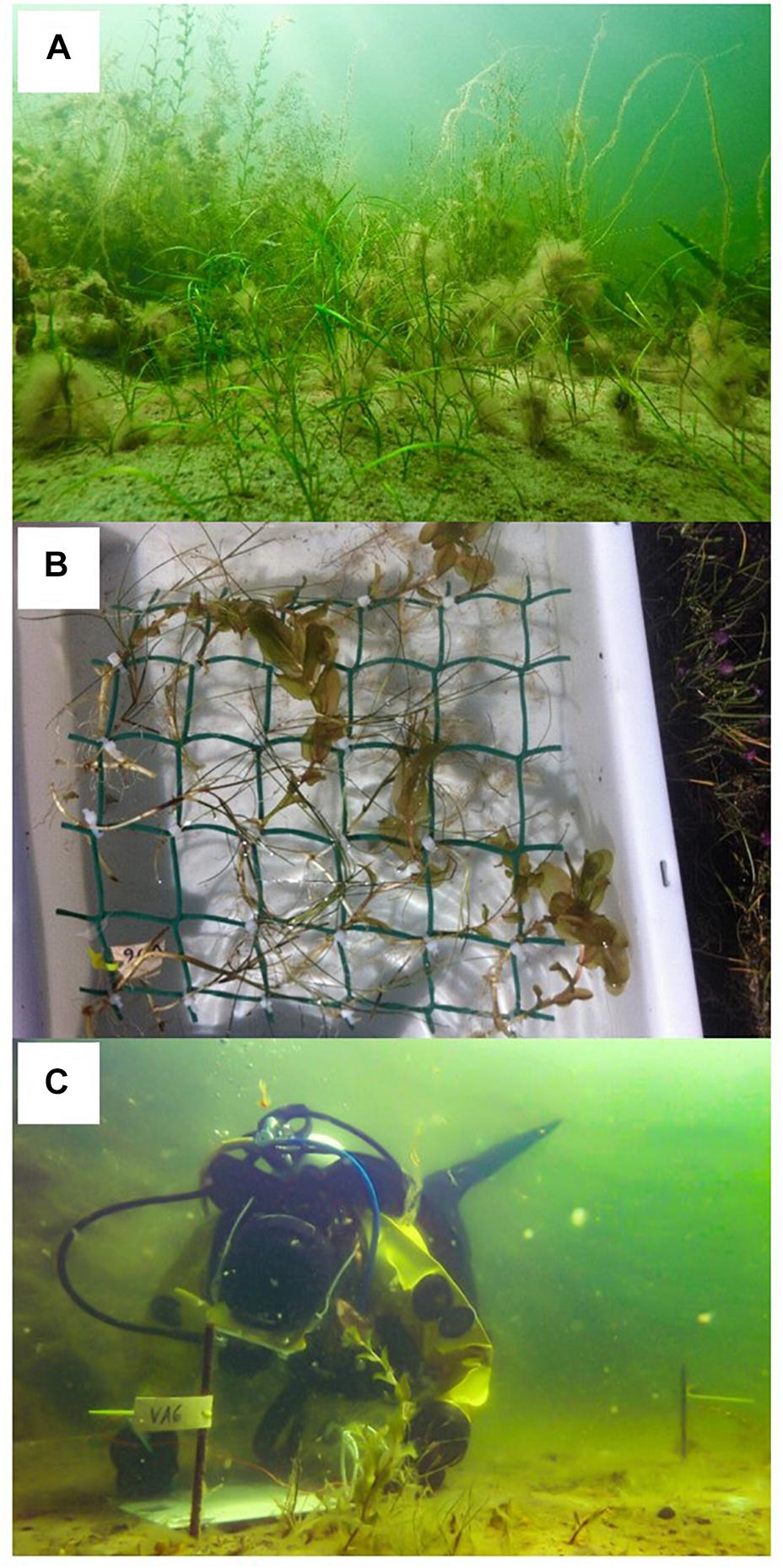
Figure 1. (A) Natural mixed species aquatic plant community in the northern Baltic Sea (image credit Alf Norkko). (B) Artificial triculture community ready to be transplanted (image credit Charlotte Angove). (C) Regular monitoring of experimental communities (image credit Tiina Salo).
We collected shoots of the six most abundant species which were already existing in the lagoon; these species were Zostera marina L., Potamogeton perfoliatus L., Zannichellia major Boenn. ex Reichenb., Ruppia cirrhosa (Petagna) Grande, Stuckenia pectinata (L.) Böerner and Myriophyllum spicatum L. (based on preliminary checks in 2016 and pre-existing data; Gustafsson and Norkko, 2019). At this time of year, the growth season for these plants had only recently begun (personal observation). We created experimental triculture communities where we manipulated the species composition to change the community weighted means (CWMs) of traits. Thus, our experimental treatment was the mean and variability of heritable traits manifested by different species within a community. We selected each species assemblage using a random number generator, and experimental treatments varied along a continuous scale (total 18 communities, Supplementary Table S1). The random selection process could have selected identical communities at random, and resultantly there were 11 unique community assemblages amongst the 18 communities. There were no selection criteria amongst the six experimental species, however we removed one species M. spicatum from the random selection process after its maximum possible occurrence (2 experimental plots, 16 shoots) because it was found in lower abundances than the other experimental species. We constructed the communities by randomly assembling 8 individuals of each 3 species onto a 30∗30 cm plastic grid using cable ties (total 24 shoots, Figure 1B) while keeping the plants submerged. The individuals used to assemble communities were standardized by approximate biomass and we randomly collected 10 individuals of each species to estimate the starting biomass (mg DW).
We carefully planted the experimental communities and secured the grids into the sand using two stainless steel hooks. Two weeks after planting we revisited the communities to survey the plant survival rate after the transplant procedure (Figure 1B). While most communities had been successfully transplanted, if an individual was lost then we corrected the starting biomass value of the community accordingly. We re-visited the communities every 2–3 weeks to monitor their growth and remove the shoots of non-experimental plants which approached or intruded the plots (Figure 1C) until the experiment was terminated after 15 weeks on 14/09/2016, when all the plots were harvested. After harvesting, we collected subsamples of new leaf tissues for nutrient analysis (Table 1) then froze the samples (−18°C) for future processing. During the experimental period, we monitored the temperature and light continuously using loggers (HOBO Pendant® Temperature/Light Data Logger 64K, Onset, United States) and the daily maximum temperature varied between 9.1 and 19.6°C ( = 15.3°C). We converted the light intensity data (lum ft–2) to photosynthetically-active radiation (PAR, μmol m–2 s–1) using the methods described by Gustafsson and Norkko (2016). The daily maximum PAR ranged from 168 to 555 μmol m–2 s–1 ( = 419 μmol m–2 s–1). While light irradiance and temperature varied throughout the experiment (Supplementary Figure S1), conditions were approximately similar across treatments because communities were transplanted at a consistent depth (approximately 2.5 m).
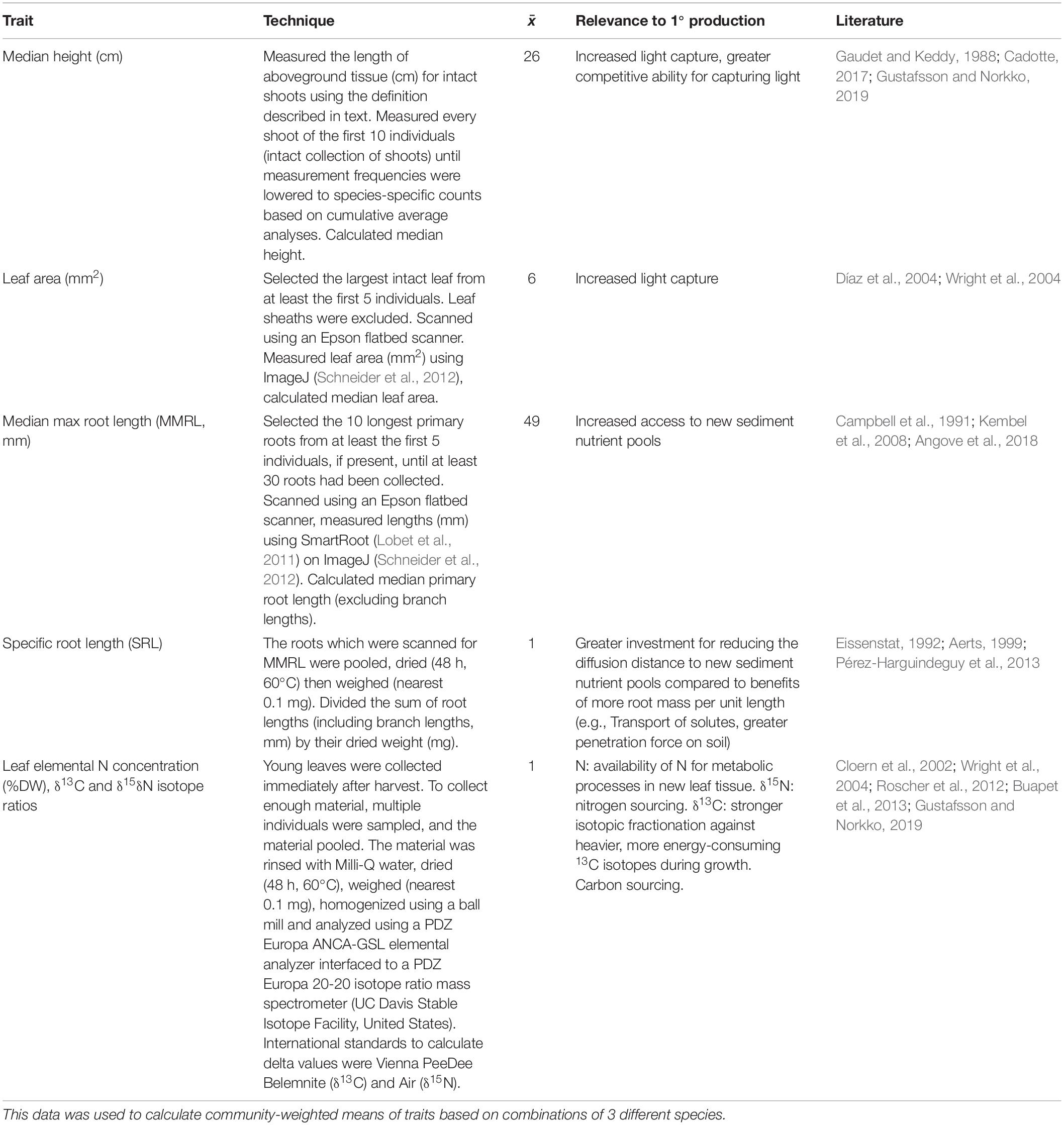
Table 1. Description of the traits which were measured for each species in each community; their abbreviations, the technique used to quantify them, the average frequency of measurements for each species trait value, the relevance of the trait to primary production in aquatic plant communities and the literature from which these relationships are based.
Trait Selection and Measurement
We measured the variability of traits manifested by the plants at harvest (e.g., Roscher et al., 2012). We defrosted the samples and counted the total number of shoots for each species in a community. To define the difference between shoots and branches, we used a guideline to separate biomass between aboveground and belowground material; aboveground biomass began where tissues became photosynthetic. One species could not be classified using this technique (Zannichellia major) because its rhizomes were often unpigmented. For this species, we separated aboveground biomass at the location of the last rhizome internode with roots before aboveground material. This adjustment did not affect height measurements because in comparison to the variability of shoot heights it was negligible. We measured traits which were potentially influential for community biomass production (Table 1), based on guidance by previous literature and the trait handbook by Pérez-Harguindeguy et al. (2013). We calculated the molar ratio between C and nitrogen (N, C:N ratio) to indicate changes in the availability and investment of C relative to N and vice versa.
We dried the samples at 60°C for at least 48 h and measured the biomass of different plant parts. Biomass measurements were pooled for each species in each plot to collect the value of the total biomass. Primary productivity (mg Dry Weight [DW] d–1) was calculated using the increase in biomass (mg DW) throughout the experimental period (105 days) for each species in each community. Community productivity (CP) was its sum of all species productivities. We removed data for one plot because its growth had noticeably failed, and we suspect that the plants suffered from something which was not included in our experimental treatment.
Functional Diversity Indices
We conducted all analyses using R (version 3.5.2, R Core Team, 2018). We calculated CWMs based on the relative shoot frequencies of each species in a community (Garnier et al., 2004; Gustafsson and Norkko, 2019). These and the CP were calculated manually from communities with no missing values, therefore there were fewer replicates than originally present and their frequency varied between analyses. While CWMs are criticized for inferring trait–environment relationships (Peres-Neto et al., 1991), this criticism does not apply to our experiment because we examined trait–productivity relationships and we sampled the entire experimental population. We calculated trait ranges for communities by subtracting the 10th percentile trait value of the species with the lowest trait value from the 90th percentile trait value from the species with the highest trait value (Schleuter et al., 2010). We did this to calculate height range (cm) and the range of root lengths (mm).
We used Spearman’s Rank correlation to check for significant relationships between traits (Table 1) and incorporated a False Discovery Rate (Benjamini and Hochberg, 1995) to reduce the likelihood of Type I errors which arise from multiple comparisons. Following this, we excluded leaf area from calculations for functional diversity indices, because it had a strong significant positive correlation to median height (Supplementary Figure S2). We used the remaining traits listed in Table 1 and the “FD” package (Laliberté et al., 2014) to calculate Functional Richness (FRic), Functional Evenness (FEve) and Functional Divergence (FDiv) (Mouchet et al., 2010; Schleuter et al., 2010). FRic describes the volume of functional space occupied by the mixture of traits, while FEve and FDiv describe the distribution of traits within the trait space (Mason et al., 2005; Villéger et al., 2008; Laliberté and Legendre, 2010; Schleuter et al., 2010). Indeed, FEve describes the regularity of the distribution of mean species traits within the trait space for a community, while FDiv describes the position of species’ trait clusters within the trait space (Schleuter et al., 2010). To calculate these indices, we estimated the relative weights of different traits for productivity using findings from a spatial survey conducted in the same region which examined the role of temperate aquatic plant traits for primary production across environmental gradients (Gustafsson and Norkko, 2019). When traits were significantly related to primary production across communities, we used standardized versions of their coefficient estimates to represent their relative weights for functional diversity indices (Height = 33, δ15N = 13, δ13C = 11) (Petchey and Gaston, 2006). When traits were not significantly linked to productivity across communities, we ranked their weight as 1. The FRic and FDiv estimates represented the variability of traits poorly (quality = 0.28) because of the corrective process to include more traits than species; while FRic indices must traditionally have more species than traits, the model which we used incorporated a corrective process (Laliberté et al., 2014).
Statistical Analysis
We conducted linear regressions between functional diversity indices and CP, then between CWMs of traits and CP to examine the relative relationships between different traits and productivity in this experiment. When necessary, we transformed variables to meet the criteria for parametric analyses; in these cases, the transformations are shown on graphs when present. If data was non-normally distributed by a marginal amount, we maintained the use of parametric regression and used White’s test for homogeneous variances in addition to the widely-used Breusch-Pagan test to validate that variances were homogeneous (Godfrey and Orme, 1999).
We tested whether the correlation between leaf area and height was reflected at community level using Pearson’s correlation analysis. Additionally, we further investigated the relationship between community δ13C and CP using a generalized linear model (GLM); we tested whether trends were due to species differences or whether there was a valid independent trend between δ13C and productivity. For this analysis, we removed one species Myriophyllum spicatum because its occurrence in the experimental communities was much lower than other species (2 out of 18 communities). There was collinearity between species identity and δ13C, therefore we centered δ13C to species identity by subtracting mean species δ13C from their corresponding δ13C values to reduce collinearity. We repeated this process for C:N ratios and productivity, however we used factor ceiling analysis (Thomson et al., 1996; Thrush et al., 2003) and non-parametric regression to investigate C:N-productivity trends. Finally, we conducted a permutation-based multiple regression analysis to summarize the combined effect of different traits for productivity (Table 1). For this analysis, we included the estimated start biomass for each community to examine its relative effect to biomass production compared to other traits measured. We used Euclidean distances and we implemented a backward-selection process to select a model by its AICc. The main findings were consistent when the backward-selection process was replaced by a forward-selection process, indicating model stability.
Results
By the time of harvest, there was a large variability in growth across our experiment; with overall biomass per community ranging from 1.854 to 8.145 g DW. CP ranged from 11.9 to 57.2 mg DW d–1 (tenth and ninetieth percentiles, respectively) which meant that the amount of biomass produced in each community varied by more than four times. Overall, functional diversity metrics were weakly related to biomass production (Table 2). While there was a significant relationship between FRic and community biomass production (Figure 2 and Table 2), there was not a significant relationship between community biomass production and FEve (0.78) or FDiv (0.78) (Table 2).
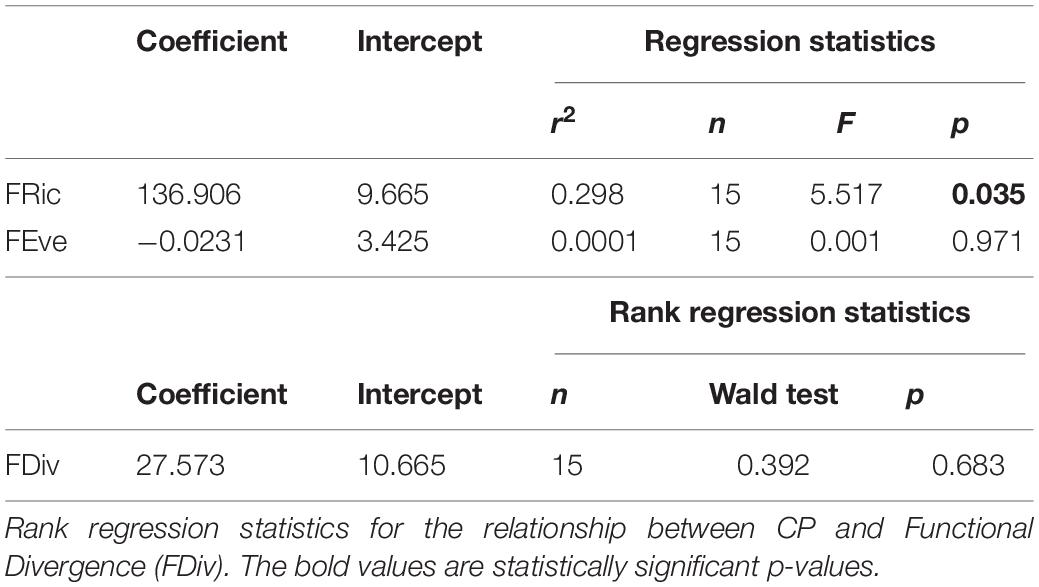
Table 2. Linear regression statistics for the relationship between CP (mg DW d–1) and functional diversity indices; Functional Richness (FRic) and Functional Evenness (FEve).
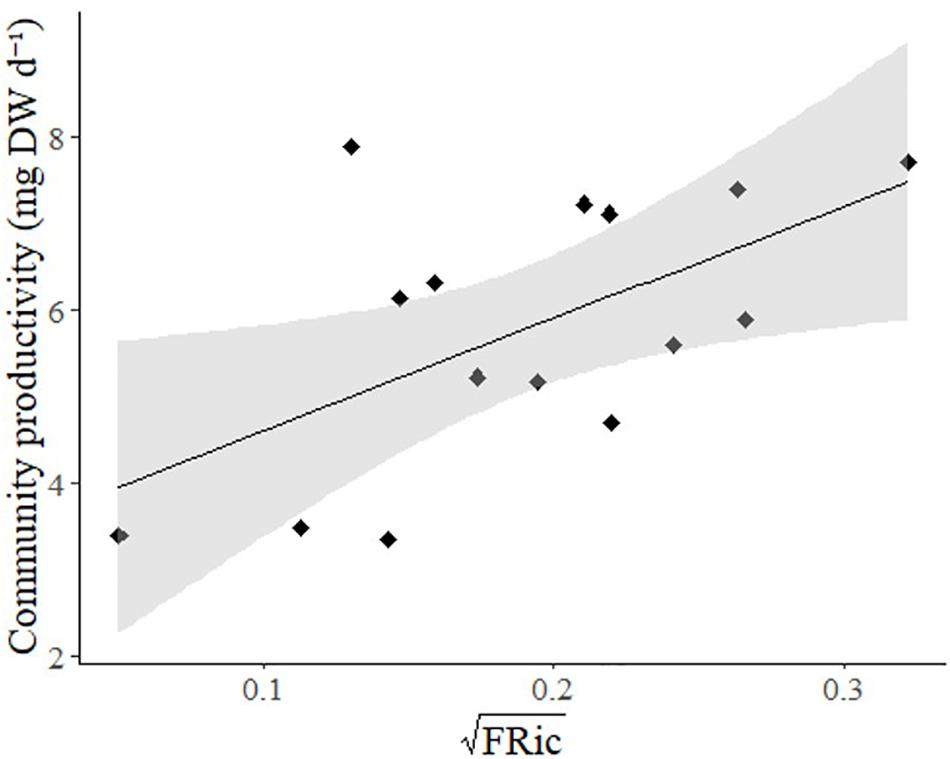
Figure 2. Relationship between Functional Richness (FRic, square root transformed) and community productivity (mg DW d–1). Solid line: line of best fit, shaded area: 95% confidence intervals.
Linear Regression Analyses
Linear regression analyses identified two types of traits which were relatively strongly related to CP; these were traits that characterized light capture (height and leaf area) and some traits that described nutrient concentrations in the leaves (Table 3). Other traits were not significantly related to productivity in these analyses (p > 0.05, Supplementary Table S2). These included mean community maximum root length (MMRL, mm), specific root length (SRL), leaf tissue nitrogen (% DW) and δ15N concentrations, as well as the range of root lengths within a community (mm).
Height was strongly related to CP by a variety of mechanisms. Firstly, taller communities were significantly more productive (Figure 3A and Table 4).
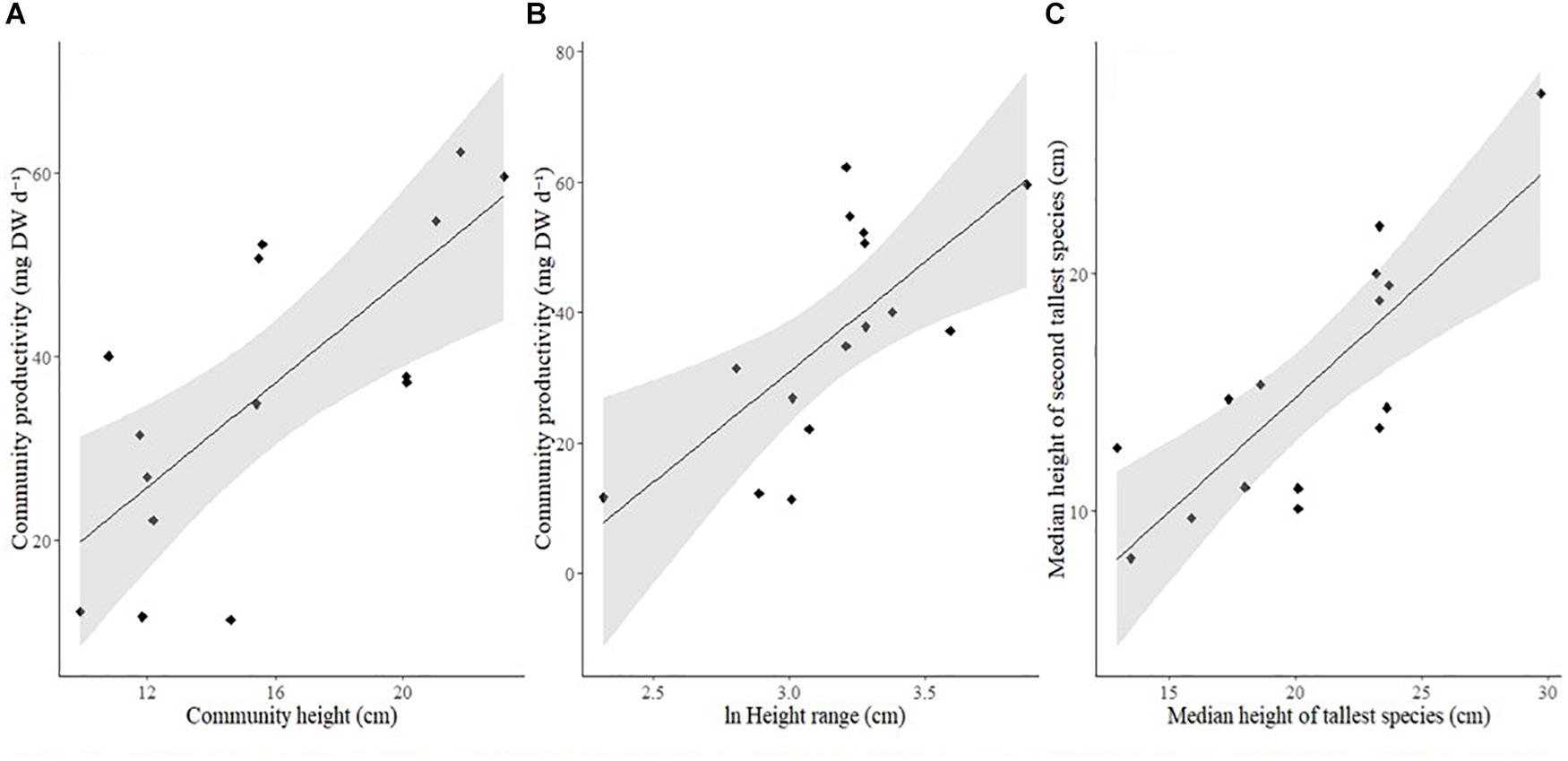
Figure 3. Relationship between (A) Community height (cm) and community productivity (mg DW d–1), (B) Community height range (ln-transformed, cm) and community productivity (mg DW d–1), (C) Median height of tallest species (cm) and median height of second tallest species (cm). Solid line: line of best fit, shaded area: 95% confidence intervals.
Secondly, the upper height limit of a community drove the height range (Table 3 and Supplementary Figure S3) which was also significantly related to primary production (Figure 3B and Table 3). This is partly owing to the tallest species being more productive in response to its own increase in height (Table 3 and Supplementary Figure S4). However, it is also a result of the second tallest species being more productive as the community height range increased (Table 3 and Supplementary Figure S4). This is because the second tallest species was significantly more likely to grow taller as the tallest species increased in height (Figure 3C, Pearson correlation; r = 0.8038, p < 0.001). The productivity of the third tallest species was not significantly related to community height range (Table 3).
Taller communities were significantly more likely to have larger leaf areas (Figure 4A, Pearson correlation, r = 0.8441, p < 0.001), and leaf area was significantly related to the productivity of a community (Figure 4B and Table 3).
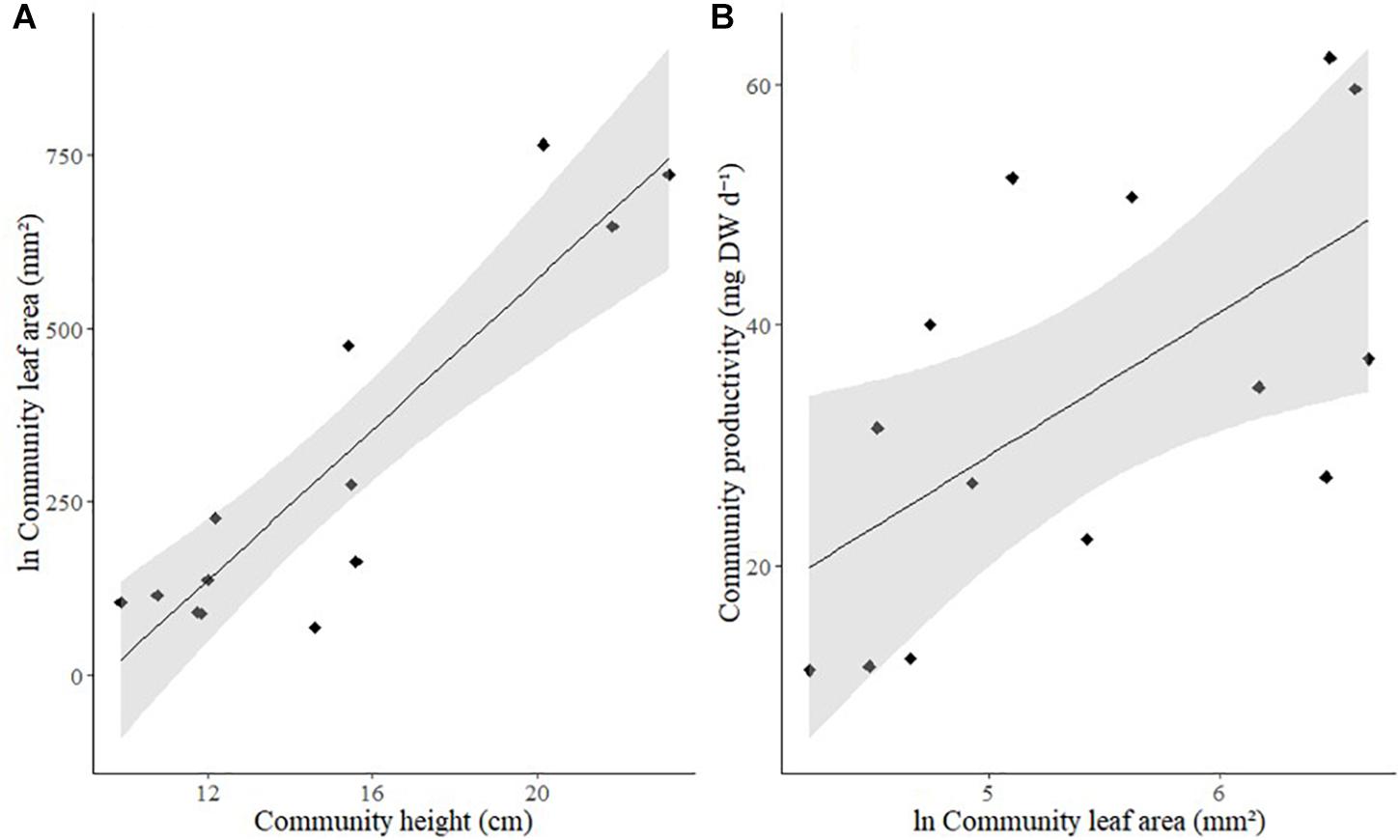
Figure 4. Relationship between (A) Community height (cm) and community leaf area (ln-transformed, mm2), (B) Community leaf area (ln-transformed, mm2) and community productivity (mg DW d–1). Solid line: line of best fit, shaded area: 95% confidence intervals.
With increasing productivity of communities, δ13C was significantly likely to be less negative (Figure 5A and Table 3). There was also a weak, negative relationship between leaf tissue C:N and community production which was marginally non-significant (Figure 5C and Table 3).
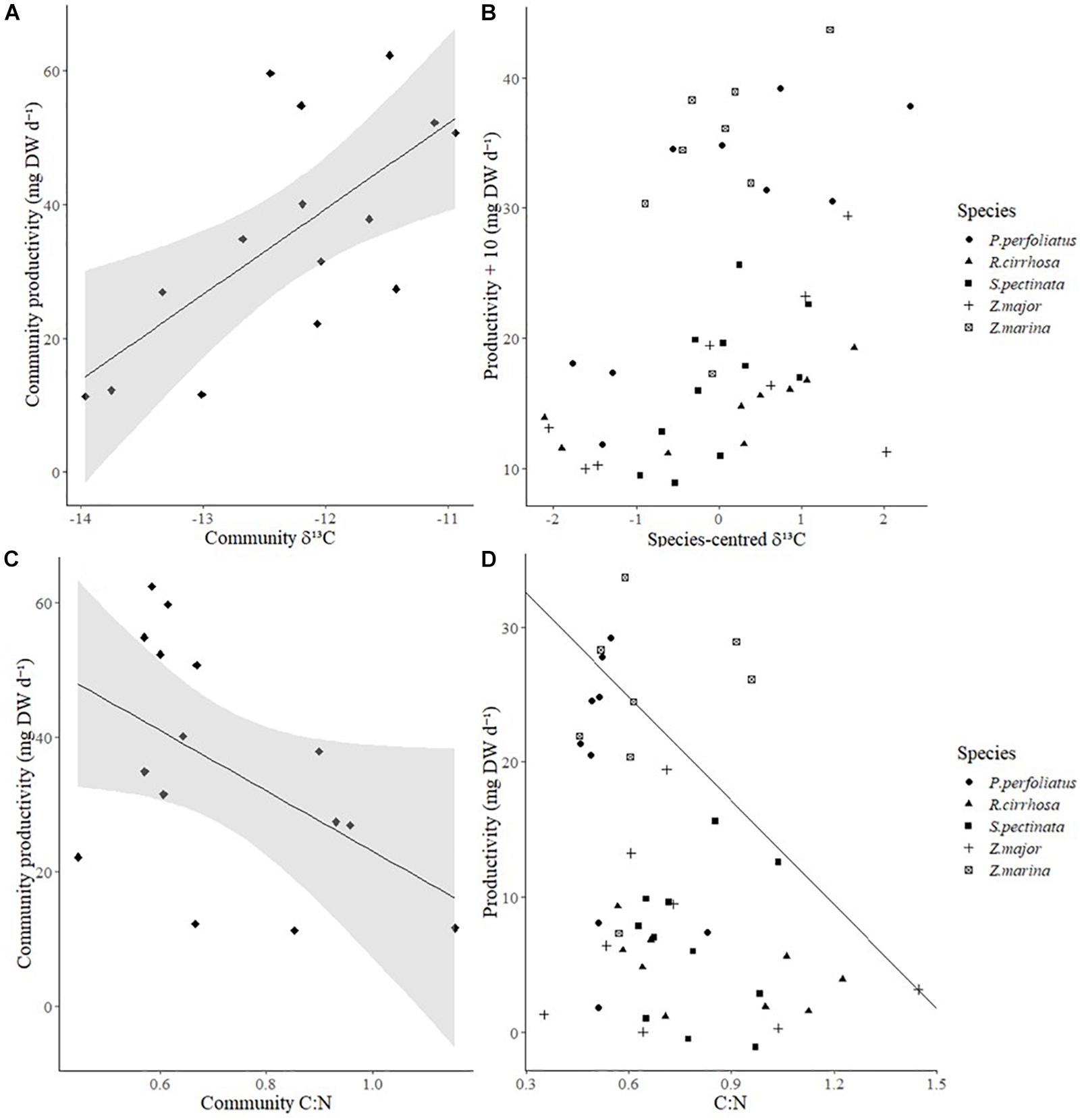
Figure 5. (A) Relationship between community leaf tissue δ13C and community productivity (mg DW d–1); solid line: line of best fit, shaded area: 95% confidence intervals. (B) Relationship between species-centered leaf tissue δ13C of each species in each community and their productivity (+ 10 in accordance to its entry in a generalized linear model with error family Gamma, mg DW d–1). (C) Relationship between community leaf tissue carbon: nitrogen (C:N) ratios and community productivity (mg DW d–1); solid line: line of best fit, shaded area: 95% confidence intervals. (D) Relationship between leaf tissue C:N of each species in each community and their productivity (mg DW d–1); solid line: 85th percentile of productivity with changes in C:N.
Post hoc Nutrient Analyses
Post hoc analyses showed that the relationship between δ13C and productivity was affected by species identity, because there was collinearity between species identity and δ13C (VIF > 100). However, once the collinearity was remediated by centering δ13C by species identity, a significant relationship between δ13C and productivity persisted [Figure 5B, GLM; F(1,43) = 21.099, p < 0.0001]. Indeed, while species had significantly different rates of primary productivity [Figure 5B, GLM; F(4,39) = 17.691, p < 0.0001] species identity did not significantly affect the relationship between δ13C and productivity [Figure 5D, GLM; F(4,35) = 0.794, p > 0.05]. Overall, there appeared to be a validly independent trend between δ13C and productivity.
For each species in each community, there was a marginally non-significant relationship between the 85th percentile of C:N and productivity (Figure 5D, quantile regression; p = 0.0598). These trends between C:N and productivity were likely caused by species identity effects because C:N ratios exhibited potential collinearity to species identity (VIF > 10) and once such collinearity was remediated by centering C:N ratios by species identity, the factor ceiling effect was not present (p > 0.05) nor was rank C:N significantly related to rank productivity (Overall Wald test = 0.391, p > 0.05).
Summary Model for Community Trait Relationships to Productivity
The summary model which included the variability of multiple traits (Table 1) supported the main findings of the single linear regression analyses; community height, leaf area and leaf tissue δ13C were the most related traits to community productivity (Table 4). Also, there were other traits included in the summary model which were not significantly related to productivity when the variability of other traits was not included in the analysis (Table 4). Indeed, these traits included in the model were the biomass at the start of the experiment and community leaf tissue δ15N (Table 4).
Discussion
Overview
We found FRic to be significantly related to primary production, unlike FEve and FDiv. Also, primary production was more strongly related to specific traits than FD indices, and these traits characterized light capture and nutrient concentrations in the leaves. The summary model supported the main findings of the single linear regression analyses, because it included community height, leaf area, and leaf tissue δ13C. It also included traits which were not significantly related to primary production on an individual basis in the model (Table 4) but apparently their variability became relevant after the variability of other traits had been considered. Such traits were the biomass of the community at the start of the experiment and the leaf tissue δ15N; indicative of different N sourcing (e.g., Cloern et al., 2002; Roscher et al., 2012). Overall, the R2 of the model was very high and indicative of overfitting, however such a high R2 is also plausible because it included many traits which were strongly related to community productivity. Thus, our results indicated that the traits which we measured in the experiment were strongly linked to primary production.
Some traits had multifaceted benefits for primary production, for example plant height was not only strongly related to community production, but it also could have facilitated a competitive response in the community because the heights of the tallest and second tallest species were correlated. Taller plants were also more likely to have larger leaf areas, and leaf area was also significantly related to productivity. Therefore, these plants were likely to have invested in capturing light more effectively (Figures 3, 4 and Table 1) and communities with such plants were more productive. Indeed, both height and leaf area were strongly related to community productivity. However, because both traits (height and leaf area) were correlated it is difficult to discern whether productivity was enhanced by both traits independently or only one trait was functionally important for productivity. Many other traits were not significantly related to primary production in the many linear regressions (Supplementary Table S2) including those which characterized root properties, leaf tissue N (% DW) concentrations and community δ15N (Supplementary Table S2). Trends in δ13C suggested that the ambient carbon supply was likely not replete, because more productive communities were richer in 13C compared to 12C. In this case, they had likely expended more energy to acquire carbon because 13C is heavier and more energy-consuming to metabolize compared to 12C, which is perhaps a consequence of limited carbon availability (Hu et al., 2012). This suggests that carbon availability could have inhibited the increased photosynthesis facilitated to plants (Kiswara et al., 2005; Hu et al., 2012) which had an increased ability to capture light. Therefore, δ13C is likely to have responded to primary productivity, and it is possible that in our experiment, δ13C represented environmental carbon availability in response to productivity rather than affecting it. Thus, an opportunity for further study would be to manipulate, for example, the nutrient availability to experimental communities to explore the dynamics between effect and response traits. With this information, there would be potential to construct an informed and predictive response-effect trait framework for aquatic plants.
Functional Diversity Enhanced Productivity by Selecting for Extreme Trait Values to Capture Light
The relationship between FRic and primary production was likely driven by the variability of height, because height was heavily weighted for the FRic calculation and height range was significantly related to productivity. However, results also showed that the height range of communities was driven by the height of the tallest species. Therefore, FRic was likely related to biomass production due to the presence of taller plants. This was supported by indices which incorporated the distribution of species abundances within the trait space; FDiv and FEve, because their results suggested that it was not the distribution of traits within a community but instead the expression of specific traits. Perhaps also, FEve and FDiv would become more informative in more species-rich communities or in an experiment which accounts for intraspecific variability in the indices. It is apparent from our findings that here, functional diversity could have benefited productivity by selecting for extreme trait values for light capture (selection effect) rather than a complementarity of fundamental niches (complementarity effect) (Loreau, 2000). Cadotte (2017) hypothesized that the selection effect was strongest for plants with similar trait values, which is partly supported by our findings because the two tallest species competed for light, however in this case it did not appear that the shortest species benefited from a complementarity effect with an increased height range. Thus, the benefit of light capture for these submerged aquatic plant communities appears to be unidirectional. Perhaps for emergent plants its effects are not unidirectional once plants reach the water-air interface. Indeed, plant biomass investment in height can change with water depth (Fu et al., 2012).
Plant height is renowned to be a fundamental trait for influencing community productivity (Díaz et al., 2004; Cadotte, 2017; Gustafsson and Norkko, 2019). This study has provided evidence that the benefits of aquatic plant height to community production are multifaceted, because the height of the tallest species was significantly related to community productivity, and its height likely stimulated a competitive height response in the community (Figure 3, Hector et al., 1997). Therefore, there is a disproportional increase in productivity in response to the manifestation of this trait (Hector et al., 1997; Cardinale et al., 2002). Such findings show that while it is arbitrary that larger plants (taller plants with larger leaf areas) are strongly related to biomass production, the manifestation of height is clearly related to biomass production for more reasons than its facilitation of plant size.
Increased Productivity Had Carbon Consequences
Temperate aquatic plant meadows are unlikely to have a replete supply of C (Buapet et al., 2013), and C depletion can limit their productivity (Hellblom and Björk, 1999; Buapet et al., 2013). Indeed, it may have been limiting to the more productive communities in this experiment because they had become enriched with the heavy isotope δ13C. Their increased ability to capture light, thus photosynthesize, and uptake carbon at higher rates (Kiswara et al., 2005; Hu et al., 2012), could have facilitated energy-consuming consequences because of their increased demand for C (Kiswara et al., 2005) which led to their enrichment with the heavy isotope 13C. It is noteworthy to recognize that while N and P can be the most limiting to growth in terrestrial communities (Güsewell, 2004), it is also important to incorporate C availability in aquatic environments as a possible limiting factor for growth (Beer and Rehnberg, 1997; Zimmerman et al., 1997; Buapet et al., 2013; Campbell and Fourqurean, 2013). At community level, species differences in δ13C also contributed to the relationship between community δ13C and productivity; which could be related to, for example, their ability to use bicarbonate () as a carbon source (Lepoint et al., 2004). Leaf C:N ratios were largely affected by species identity, which is accordant to findings by Li et al. (2015) that species differences in the allocation of nutrients are likely to be more important for the variability of C:N rather than growth rates of aquatic plants. The marginally non-significant relationship between leaf tissue C:N and productivity indicated that there could have been an underlying role for relative species abundances to affect productivity. Indeed, it is clear from these results that species identity was likely to have had an integral role to the results of this experiment; results depended on whether species could manifest certain trait values. However, this was expected because we manipulated the species composition to change the variability of plant traits.
Generalizing Local Findings to Larger Spatial Scales
Gustafsson and Norkko (2019) surveyed aquatic plant meadows which included the natural meadow next to this experimental site. They found that aquatic plant height was the only plant trait with a strong significant relationship to productivity across environmental scales, and the location of this study was included in their range. Our findings complement this survey because it provides experimental evidence that height is likely to be one of the most related traits to primary productivity. Fu et al. (2014) also found that height was significantly related to primary production along a water depth gradient in freshwater lakes, amongst other traits. In our study, we found that leaf area was also significantly related to primary production and that it was significantly correlated with height, strengthening inferences for the potential generality of our findings. The strong link between leaf area and height indicates that the interaction between closely related traits likely contributes to the overall functioning of aquatic plant communities. For example, in our experiment, the taller plants could have invested in strategies to optimize light capture so that they could facilitate high productivity. This may have catalyzed the increase in productivity relative to height. The relationship between δ13C and productivity were much less variable in our experiment than as observed by Gustafsson and Norkko (2019), and in the local region of this site the relationship was reverse to our observations. This could have been due to the environmental variability in carbon sources across different communities at a larger spatial scale. However, we found no significant relationship between δ15N and productivity like the local region by Gustafsson and Norkko (2019), and potentially the source of nitrogen is not as closely related to productivity compared to other local factors. Whereas, Gustafsson and Norkko (2019) found that δ15N was more closely related to productivity at larger spatial scales, maybe because δ15N represented the influence of N sourcing at larger spatial scales, or it became more influential in other conditions. It was included in our summary model which examined the overall relationship between traits and productivity; therefore, perhaps it is also related to productivity for a reason that we have not yet identified, which is linked to the variability of other traits. Overall, Gustafsson and Norkko (2019) suggested that while height is strongly related to productivity across communities, the combination of plant traits which are related to productivity is highly context-dependent. Following our mechanistic study, we build on this to hypothesize that traits linking to light capture are prioritized (i.e., Height and leaf area) (Cadotte, 2017). These findings show that CWMs have been related to productivity so strongly that their changes led to a relationship between functional diversity and productivity. Such findings are accordant to those by Fu et al. (2014) which show that CWMs are likely more related to aquatic plant productivity than a metric for functional diversity. Our results provide further evidence that the dynamics for plants in aquatic environments are significantly different to those in the terrestrial realm (e.g., Carbon availability, water availability and light-nutrient dynamics; Hemminga and Duarte, 2000; Peterson and Heck, 2001; Lee et al., 2007). Furthermore, it is important to acknowledge the ecological independence of aquatic plants to the terrestrial realm and to conduct an informed evaluation. Indeed, with this approach the studies of aquatic plants are not only exploring a relatively new environment, but it also challenges the paradigms for terrestrial environments by exploring how they interact with extremely different environmental conditions (e.g., Refraction of light in the water column which is related to increased nutrient availability; Krause-Jensen et al., 2008). Further studies which investigate the underlying mechanisms of function for aquatic plants would greatly benefit our understanding of the ecology of aquatic and perhaps even terrestrial plants.
Conclusion
This is, to our knowledge, the first experiment to manipulate species composition to investigate functional diversity in aquatic plant meadows in situ. It shows how functional diversity experiments can be conducted in situ in ecosystems beyond terrestrial grasslands, also for those which have a relatively low species-diversity. We found that functional richness was related to community productivity, likely because of a selection effect which enhanced community light capture (height and leaf area). Indeed, there were multifaceted benefits for communities with taller species, because of observed competition between species. It appeared that biomass production came at a consequence to carbon supply, and species identity participated an integral role for the relationship between carbon and productivity. An opportunity for further study would be to explore plant trait responses and build a predictive plant response-effect trait framework.
Data Availability Statement
The raw data supporting the conclusions of this article will be made available by the authors, without undue reservation, to any qualified researcher.
Author Contributions
CG and CA conceived the ideas and designed methodology. CA, CG, and AN conducted the experiment in the field, analyzed the data, and wrote the manuscript. CA led laboratory processing of the samples.
Funding
This work was funded by the Walter and Andrée de Nottbeck Foundation (CA and CG), Societas pro Fauna et Flora Fennica (CA), Academy of Finland (AN, grant number 294853 and CG, grant number 295443), and Svenska Kulturfonden (CG).
Conflict of Interest
The authors declare that the research was conducted in the absence of any commercial or financial relationships that could be construed as a potential conflict of interest.
Acknowledgments
This work was conducted at Tvärminne Zoological Station (University of Helsinki). We are very thankful to Albert Palomino Gonzàlez, Camille Domy, and Denise Ong Rui Ying for helping us to process the large volume of samples from this experiment, and to Albert for his assistance in the field. We also thank Hanna Halonen, Samu Elovaara, Laura Kauppi, and Iván Rodil for their assistance in the field, and we are grateful to Onni Talas interns Pirita Anttila, Salla Ahonen, and Ella von Weissenberg for their assistance in the field and laboratory. Thank you to Johanna Gammal, Judi Hewitt, and Anna Villnäs for their analytical advice and to Anna for her comments on the manuscript.
Supplementary Material
The Supplementary Material for this article can be found online at: https://www.frontiersin.org/articles/10.3389/fmars.2020.00140/full#supplementary-material
References
Aerts, R. (1999). Interspecific competition in natural plant communities: mechanisms, trade-offs and plant-soil feedbacks. J. Exp. Bot. 50, 29–37. doi: 10.1093/jxb/50.330.29
Andersen, J. H., Laamanen, M., Aigars, J., Axe, P., Blomqvist, M., Carstensen, J., et al. (2009). “Eutrophication in the Baltic Sea-An integrated thematic assessment of the effects of nutrient enrichment in the Baltic Sea region,” in Baltic Sea Environment Proceedings, (Washington, DC: Helsinki Commission).
Angove, C., Norkko, A., and Gustafsson, C. (2018). Assessing the efficiencies and challenges for nutrient uptake by aquatic plants. J. Exp. Mar. Biol. Ecol. 507, 23–30. doi: 10.1016/j.jembe.2018.07.005
Armitage, A. R., Frankovich, T. A., and Fourqurean, J. W. (2011). Long-term effects of adding nutrients to an oligotrophic coastal environment. Ecosystems 14, 430–444. doi: 10.1007/s10021-011-9421-2
Attard, K. M., Rodil, I. F., Glud, R. N., Berg, P., Norkko, J., and Norkko, A. (2019). Seasonal ecosystem metabolism across shallow benthic habitats measured by aquatic eddy covariance. Limnol. Oceanogr. Lett. 4, 79–86. doi: 10.1002/lol2.10107
Beer, S., and Rehnberg, J. (1997). The acquisition of inorganic carbon by the seagrass Zostera marina. Aqu. Bot. 56, 277–283. doi: 10.1016/s0304-3770(96)01109-6
Benjamini, Y., and Hochberg, Y. (1995). Controlling the false discovery rate: a practical and powerful approach to multiple testing. J. R. Stat. Soc. Ser. B 57, 289–300. doi: 10.1111/j.2517-6161.1995.tb02031.x
Boström, C., Roos, C., and Rönnberg, O. (2004). Shoot morphometry and production dynamics of eelgrass in the northern Baltic Sea. Aqu. Bot. 79, 145–161. doi: 10.1016/j.aquabot.2004.02.002
Buapet, P., Rasmusson, L. M., Gullstrom, M., and Bjork, M. (2013). Photorespiration and carbon limitation determine productivity in temperate seagrasses. PLoS One 8:e83804. doi: 10.1371/journal.pone.0083804
Cadotte, M. W. (2017). Functional traits explain ecosystem function through opposing mechanisms. Ecol. Lett. 20, 989–996. doi: 10.1111/ele.12796
Cadotte, M. W., Carscadden, K., and Mirotchnick, N. (2011). Beyond species: functional diversity and the maintenance of ecological processes and services. J. Appl. Ecol. 48, 1079–1087. doi: 10.1111/j.1365-2664.2011.02048.x
Campbell, B., Grime, J., and Mackey, J. (1991). A trade-off between scale and precision in resource foraging. Oecologia 87, 532–538. doi: 10.1007/bf00320417
Campbell, J. E., and Fourqurean, J. W. (2013). Effects of in situ CO2 enrichment on the structural and chemical characteristics of the seagrass Thalassia testudinum. Mar.Biol. 160, 1465–1475. doi: 10.1007/s00227-013-2199-3
Cardinale, B. J., Palmer, M. A., and Collins, S. L. (2002). Species diversity enhances ecosystem functioning through interspecific facilitation. Nature 415:426. doi: 10.1038/415426a
Cloern, J., Canuel, E., and Harris, D. (2002). Stable carbon and nitrogen isotope composition of aquatic and terrestrial plants of the San Francisco Bay estuarine system. Limnol. Oceanogr. 47, 713–729. doi: 10.4319/lo.2002.47.3.0713
Díaz, S., and Cabido, M. (2001). Vive la difference: plant functional diversity matters to ecosystem processes. Trends Ecol. Evol. 16, 646–655. doi: 10.1016/s0169-5347(01)02283-2
Díaz, S., Hodgson, J., Thompson, K., Cabido, M., Cornelissen, J. H., Jalili, A., et al. (2004). The plant traits that drive ecosystems: evidence from three continents. J. Veg. Sci. 15, 295–304.
Eissenstat, D. (1992). Costs and benefits of constructing roots of small diameter. J. Plant Nutr. 15, 763–782. doi: 10.1080/01904169209364361
Engelhardt, K. A., and Ritchie, M. E. (2002). The effect of aquatic plant species richness on wetland ecosystem processes. Ecology 83, 2911–2924. doi: 10.1890/0012-9658(2002)083[2911:teoaps]2.0.co;2
Ferdie, M., and Fourqurean, J. W. (2004). Responses of seagrass communities to fertilization along a gradient of relative availability of nitrogen and phosphorus in a carbonate environment. Limnol. Oceanogr. 49, 2082–2094. doi: 10.4319/lo.2004.49.6.2082
Fu, H., Yuan, G., Cao, T., Ni, L., Zhang, M., and Wang, S. (2012). An alternative mechanism for shade adaptation: implication of allometric responses of three submersed macrophytes to water depth. Ecol. Res. 27, 1087–1094. doi: 10.1007/s11284-012-0991-z
Fu, H., Zhong, J., Yuan, G., Xie, P., Guo, L., Zhang, X., et al. (2014). Trait−based community assembly of aquatic macrophytes along a water depth gradient in a freshwater lake. Freshw. Biol. 59, 2462–2471. doi: 10.1111/fwb.12443
Furman, B. T., Jackson, L. J., and Peterson, B. J. (2017). Edaphic resource foraging by Zostera marina (Linnaeus) patches. J. Exp. Mar. Biol. Ecol. 486, 214–221. doi: 10.1016/j.jembe.2016.10.013
Gagic, V., Bartomeus, I., Jonsson, T., Taylor, A., Winqvist, C., Fischer, C., et al. (2015). Functional identity and diversity of animals predict ecosystem functioning better than species-based indices. Proc. R. Soc. B Biol. Sci. 282:20142620. doi: 10.1098/rspb.2014.2620
Gammal, J., Järnström, M., Bernard, G., Norkko, J., and Norkko, A. (2019). Environmental context mediates biodiversity–ecosystem functioning relationships in coastal coft-sediment habitats. Ecosystems 22, 137–151. doi: 10.1007/s10021-018-0258-9
Garnier, E., Cortez, J., Billès, G., Navas, M., Roumet, C., Debussche, M., et al. (2004). Plant functional markers capture ecosystem properties during secondary succession. Ecology 85, 2630–2637. doi: 10.1890/03-0799
Garnier, E., Navas, M., and Grigulis, K. (2016). Plant Functional Diversity: Organism Traits, Community Structure, and Ecosystem Properties. Oxford: Oxford University Press.
Gaudet, C., and Keddy, P. (1988). A comparative approach to predicting competitive ability from plant traits. Nature 334, 242–243. doi: 10.1038/334242a0
Godfrey, L. G., and Orme, C. D. (1999). The robustness, reliability and power of heteroskedasticity tests. Econ. Rev. 18, 169–194. doi: 10.1080/07474939908800438
Güsewell, S. (2004). N: P ratios in terrestrial plants: variation and functional significance. N. Phytol. 164, 243–266. doi: 10.1111/j.1469-8137.2004.01192.x
Gustafsson, B. G., Schenk, F., Blenckner, T., Eilola, K., Meier, H. M., Müller-Karulis, B., et al. (2012). Reconstructing the development of Baltic Sea eutrophication 1850–2006. Ambio 41, 534–548. doi: 10.1007/s13280-012-0318-x
Gustafsson, C., and Boström, C. (2011). Biodiversity influences ecosystem functioning in aquatic angiosperm communities. Oikos 120, 1037–1046. doi: 10.1111/j.1600-0706.2010.19008.x
Gustafsson, C., and Boström, C. (2013). Influence of neighboring plants on shading stress resistance and recovery of eelgrass, Zostera marina L. PLoS One 8:e64064. doi: 10.1371/journal.pone.0064064
Gustafsson, C., and Norkko, A. (2016). Not all plants are the same: Exploring metabolism and nitrogen fluxes in a benthic community composed of different aquatic plant species. Limnol. Oceanogr. 61, 1787–1799. doi: 10.1002/lno.10334
Gustafsson, C., and Norkko, A. (2019). Quantifying the importance of functional traits for primary production in aquatic plant communities. J. Ecol. 107, 154–166. doi: 10.1111/1365-2745.13011
Hector, A., Schmid, B., Beierkuhnlein, C., Caldeira, M. C., Diemer, M., Dimitrakopoulos, P. G., et al. (1997). The effects of plant composition and diversity on ecosystem processes. Science 277, 1302–1305. doi: 10.1126/science.277.5330.1302
Hellblom, F., and Björk, M. (1999). Photosynthetic responses in Zostera marina to decreasing salinity, inorganic carbon content and osmolality. Aqu. Bot. 65, 97–104. doi: 10.1016/s0304-3770(99)00034-0
Hillebrand, H., and Matthiessen, B. (2009). Biodiversity in a complex world: consolidation and progress in functional biodiversity research. Ecol. Lett. 12, 1405–1419. doi: 10.1111/j.1461-0248.2009.01388.x
Hooper, D. U., Chapin, F. S., Ewel, J. J., Hector, A., Inchausti, P., Lavorel, S., et al. (2005). Effects of biodiversity on ecosystem functioning: a consensus of current knowledge. Eco. Monogr. 75, 3–35.
Hooper, D. U., and Vitousek, P. M. (1997). The effects of plant composition and diversity on ecosystem processes. Science 277, 1302–1305.
Hu, X., Burdige, D. J., and Zimmerman, R. C. (2012). δ13C is a signature of light availability and photosynthesis in seagrass. Limnol. Oceanogr. 57, 441–448. doi: 10.4319/lo.2012.57.2.0441
Kembel, S. W., De Kroon, H., Cahill, J. F. Jr., and Mommer, L. (2008). Improving the scale and precision of hypotheses to explain root foraging ability. Ann. Bot. 101, 1295–1301. doi: 10.1093/aob/mcn044
Kiswara, W., Huiskes, A. H., and Herman, P. M. (2005). Uptake and allocation of 13C by Enhalus acoroides at sites differing in light availability. Aqu. Bot. 81, 353–366. doi: 10.1016/j.aquabot.2005.02.002
Krause-Jensen, D., Sagert, S., Schubert, H., and Boström, C. (2008). Empirical relationships linking distribution and abundance of marine vegetation to eutrophication. Ecol. Indic. 8, 515–529. doi: 10.1016/j.ecolind.2007.06.004
Laliberté, E., and Legendre, P. (2010). A distance-based framework for measuring functional diversity from multiple traits. Ecology 91, 299–305. doi: 10.1890/08-2244.1
Laliberté, E., Legendre, P., and Shipley, B. (2014). FD: Measuring Functional Diversity From Multiple Traits, and Other Tools for Functional Ecology. R Package Version 1.0-12.
Lee, K., Park, S. R., and Kim, Y. K. (2007). Effects of irradiance, temperature, and nutrients on growth dynamics of seagrasses: a review. J. Exp. Mar. Biol.Ecol. 350, 144–175. doi: 10.1016/j.jembe.2007.06.016
Lepoint, G., Dauby, P., and Gobert, S. (2004). Applications of C and N stable isotopes to ecological and environmental studies in seagrass ecosystems. Mar. Pollut. Bull. 49, 887–891. doi: 10.1016/j.marpolbul.2004.07.005
Li, W., Cao, T., Ni, L., Zhu, G., Zhang, X., Fu, H., et al. (2015). Size-dependent C, N and P stoichiometry of three submersed macrophytes along water depth gradients. Environ. Earth Sci. 74, 3733–3738. doi: 10.1007/s12665-015-4295-9
Lobet, G., Pages, L., and Draye, X. (2011). A novel image-analysis toolbox enabling quantitative analysis of root system architecture. Plant Physiol. 157, 29–39. doi: 10.1104/pp.111.179895
Longstaff, B. J., and Dennison, W. C. (1999). Seagrass survival during pulsed turbidity events: the effects of light deprivation on the seagrasses Halodule pinifolia and Halophila ovalis. Aqu. Bota. 65, 105–121. doi: 10.1016/s0304-3770(99)00035-2
Loreau, M. (2000). Biodiversity and ecosystem functioning: recent theoretical advances. Oikos 91, 3–17. doi: 10.1034/j.1600-0706.2000.910101.x
Loreau, M., and Hector, A. (2001). Partitioning selection and complementarity in biodiversity experiments. Nature 412:72. doi: 10.1038/35083573
Mason, N. W., Mouillot, D., Lee, W. G., and Wilson, J. B. (2005). Functional richness, functional evenness and functional divergence: the primary components of functional diversity. Oikos 111, 112–118. doi: 10.1111/j.0030-1299.2005.13886.x
Maurer, D. A., and Zedler, J. B. (2002). Differential invasion of a wetland grass explained by tests of nutrients and light availability on establishment and clonal growth. Oecologia 131, 279–288. doi: 10.1007/s00442-002-0886-8
McGill, B. J., Enquist, B. J., Weiher, E., and Westoby, M. (2006). Rebuilding community ecology from functional traits. Trends Ecol. Evol. 21, 178–185. doi: 10.1016/j.tree.2006.02.002
Mouchet, M. A., Villéger, S., Mason, N. W., and Mouillot, D. (2010). Functional diversity measures: an overview of their redundancy and their ability to discriminate community assembly rules. Funct. Ecol. 24, 867–876. doi: 10.1111/j.1365-2435.2010.01695.x
Mouillot, D., Graham, N. A., Villéger, S., Mason, N. W., and Bellwood, D. R. (2013). A functional approach reveals community responses to disturbances. Trends Ecol.Evol. 28, 167–177. doi: 10.1016/j.tree.2012.10.004
Peres-Neto, P. R., Dray, S., and Ter Braak, C. J. (2017). Linking trait variation to the environment: critical issues with community-weighted mean correlation resolved by the fourth-corner approach. Ecography 40, 806–816. doi: 10.1111/ecog.02302
Perez, M., Romero, J., Duarte, C. M., and Sand-Jensen, K. (1991). Phosphorus limitation of Cymodocea nodosa growth. Mar. Biol. 109, 129–133.
Pérez-Harguindeguy, N., Díaz, S., Garnier, E., Lavorel, S., Poorter, H., Jaureguiberry, P., et al. (2013). New handbook for standardised measurement of plant functional traits worldwide. Austr. J. Bot. 61, 167–234.
Petchey, O., and Gaston, K. (2006). Functional diversity: back to basics and looking forward. Ecol. Lett. 9, 741–758. doi: 10.1111/j.1461-0248.2006.00924.x
Peterson, B. J., and Heck, K. L. Jr. (2001). An experimental test of the mechanism by which suspension feeding bivalves elevate seagrass productivity. Mar. Ecol. Progr. Ser. 218, 115–125. doi: 10.3354/meps218115
R Core Team (2018). R: A Language and Environment for Statistical Computing. 3.5, 2 Edn. Vienna: R Foundation for Statistical Computing.
Ralph, P., Durako, M. J., Enriquez, S., Collier, C., and Doblin, M. (2007). Impact of light limitation on seagrasses. J. Exp. Mar. Biol.Ecol. 350, 176–193. doi: 10.1016/j.jembe.2007.06.017
Roscher, C., Schumacher, J., Gubsch, M., Lipowsky, A., Weigelt, A., Buchmann, N., et al. (2012). Using plant functional traits to explain diversity–productivity relationships. PLoS One 7:e36760. doi: 10.1371/journal.pone.0036760
Ruiz, J. M., and Romero, J. (2001). Effects of in situ experimental shading on the Mediterranean seagrass Posidonia oceanica. Mar. Ecol. Prog. Ser. 215, 107–120. doi: 10.3354/meps215107
Salo, T., Gustafsson, C., and Boström, C. (2009). Effects of plant diversity on primary production and species interactions in brackish water angiosperm communities. Mar. Ecol. Progr. Ser. 396, 261–272. doi: 10.3354/meps08325
Salo, T., Reusch, T. B., and Boström, C. (2015). Genotype-specific responses to light stress in eelgrass Zostera marina, a marine foundation plant. Mar. Ecol.Progr.Ser. 519, 129–140. doi: 10.3354/meps11083
Schleuter, D., Daufresne, M., Massol, F., and Argillier, C. (2010). A user’s guide to functional diversity indices. Ecol. Monogr. 80, 469–484. doi: 10.1890/08-2225.1
Schneider, C. A., Rasband, W. S., and Eliceiri, K. W. (2012). Nih Image to ImageJ: 25 years of image analysis. Nat. Methods 9:671. doi: 10.1038/nmeth.2089
Thomson, J. D., Weiblen, G., Thomson, B. A., Alfaro, S., and Legendre, P. (1996). Untangling multiple factors in spatial distributions: lilies, gophers, and rocks. Ecology 77, 1698–1715. doi: 10.2307/2265776
Thrush, S. F., Hewitt, J. E., Norkko, A., Nicholls, P. E., Funnell, G. A., and Ellis, J. I. (2003). Habitat change in estuaries: predicting broad-scale responses of intertidal macrofauna to sediment mud content. Mar. Ecol.Progr. Ser. 263, 101–112. doi: 10.3354/meps263101
Tilman, D., Knops, J., Wedin, D., Reich, P., Ritchie, M., and Siemann, E. (1997). The influence of functional diversity and composition on ecosystem processes. Science 277, 1300–1302. doi: 10.1126/science.277.5330.1300
Touchette, B. W., and Burkholder, J. M. (2000). Review of nitrogen and phosphorus metabolism in seagrasses. J. Exp. Mar. Biol Ecol. 250, 133–167. doi: 10.1016/s0022-0981(00)00195-7
Villéger, S., Mason, N. W., and Mouillot, D. (2008). New multidimensional functional diversity indices for a multifaceted framework in functional ecology. Ecology 89, 2290–2301. doi: 10.1890/07-1206.1
Violle, C., Navas, M., Vile, D., Kazakou, E., Fortunel, C., Hummel, I., et al. (2007). Let the concept of trait be functional! Oikos 116, 882–892. doi: 10.1111/j.0030-1299.2007.15559.x
Worm, B., and Reusch, T. B. (2000). Do nutrient availability and plant density limit seagrass colonization in the Baltic Sea? Mar. Ecol. Progr. Ser. 200, 159–166. doi: 10.3354/meps200159
Wright, I. J., Reich, P. B., Westoby, M., Ackerly, D. D., Baruch, Z., Bongers, F., et al. (2004). The worldwide leaf economics spectrum. Nature 428, 821–827.
Keywords: Baltic Sea, BEF, carbon cycling, functional diversity, functional traits, primary production, Zostera marina
Citation: Angove C, Norkko A and Gustafsson C (2020) The Fight to Capture Light: Functional Diversity Is Related to Aquatic Plant Community Productivity Likely by Enhancing Light Capture. Front. Mar. Sci. 7:140. doi: 10.3389/fmars.2020.00140
Received: 13 November 2019; Accepted: 24 February 2020;
Published: 20 March 2020.
Edited by:
Dongyan Liu, East China Normal University, ChinaReviewed by:
Nianjun Xu, Ningbo University, ChinaKun-Seop Lee, Pusan National University, South Korea
Copyright © 2020 Angove, Norkko and Gustafsson. This is an open-access article distributed under the terms of the Creative Commons Attribution License (CC BY). The use, distribution or reproduction in other forums is permitted, provided the original author(s) and the copyright owner(s) are credited and that the original publication in this journal is cited, in accordance with accepted academic practice. No use, distribution or reproduction is permitted which does not comply with these terms.
*Correspondence: Charlotte Angove, charlotte.angove@helsinki.fi