- 1Demersal Division, Marine and Freshwater Research Institute, Reykjavik, Iceland
- 2Department of Marine Research in Developing Countries, Institute of Marine Research, Bergen, Norway
- 3Research Group on Bethic Communities, Institute of Marine Research, Bergen, Norway
- 4Demersal Division, Faroe Marine Research Institute, Tórshavn, Faroe Islands
- 5Department of Oceanography, Institute of Marine Research, Bergen, Norway
In the deep waters of the Nordic Seas and adjacent areas, several benthic habitats such as cold-water coral reefs, coral gardens, and deep-sea sponge aggregations have been classified as vulnerable marine ecosystems (VMEs), due to their uniqueness, limited spatial extent, physical fragility, and slow recovery rate. In the last decade observations carried out by habitat mapping programmes in Norway, Iceland, and more recently in the Faroe Islands have substantially increased knowledge on the distribution of VMEs in the Nordic Seas. Nevertheless, large areas have not been explored due to the cost and logistics of obtaining observations in the deep-sea. Species distribution models can be used to predict the distribution of VMEs and their indicator species. Here we present the predicted distribution of 44 VME indicator taxa including 20 sponges, 17 cold-water corals, and 7 seapens in the Nordic Seas based on data compiled and models developed by the NovasArc project (2016–2018). Models for 44 VME indicator species were developed using the maximum entropy algorithm MaxEnt, using an extensive database compiled from habitat mapping surveys, by-catch data from bottom fish surveys, and records from reports and peer reviewed publications. Modeled distributions showed good agreement with observations. Niche overlap measures were used to identify seven groups and four subgroups of VME indicator taxa that co-occur. These were consistent with the species composition of known biotopes in the study area. A VME Index that combine the predictions for all VME indicator species was computed to identify particularly valuable and vulnerable ecosystems that should be targets of further exploration and conservation efforts. Such areas were identified at shelf break and slope off Iceland, the Faroe Islands, and central Norway, and the continental shelf off southern Greenland. The predicted distribution of VMEs in Arctic and sub-Arctic waters allows for the evaluation of interactions with fisheries and other anthropogenic activities and provides an important input for managers.
1. Introduction
In the deep sea, bottom trawling is the main source of anthropogenic impacts (Benn et al., 2010; Ramirez-Llodra et al., 2011). Of particular concern are the effects of bottom trawling on Vulnerable Marine Ecosytems (VMEs), ecosystems dominated by large epibenthic organisms (e.g., corals or sponges) which are likely to experience substantial alterations, and where recovery occurs very slowly (Wheeler et al., 2005; Clark et al., 2010; Williams et al., 2010; Buhl-Mortensen et al., 2013). In addition, VMEs are increasingly being threatened by pollution (Fisher et al., 2014) and by the effects of climate change including increasing water temperatures and ocean acidification (Guinotte et al., 2006; Levin and Le Bris, 2015).
The recognition that some deep-sea ecosystems are particularly susceptible to bottom trawling led the United Nations General Assembly (UNGA) to adopt resolutions 59/25, 61/105, and 64/72, calling for member states and regional fisheries management organisations (RFMOs) to identify areas beyond national jurisdiction (ABNJ) where VMEs occur, or are likely to occur, and to prevent significant negative impacts from damaging fishing practices. The Food and Agriculture Organisation of the United Nations (FAO) defined a set of criteria to identify VMEs, including their uniqueness or rarity, the functional significance of the habitat they form, structural complexity, fragility, and life history traits that make recovery difficult (e.g., slow growth, limited mobility; FAO, 2009). In general VMEs are identified by the presence of indicator taxa (e.g., stony corals, sponges), although merely detecting the presence of a VME element (species, habitats or features) is not sufficient to identify a VME (FAO, 2009). In the north-east Atlantic several benthic habitats have been classified by the Convention for the Protection of the Marine Environment of the North East Atlantic (OSPAR) and by the North East Atlantic Fisheries Commission (NEAFC) as vulnerable to human impacts (VMEs). In the sub-Arctic waters of the Nordic seas these habitats include seapen fields, cold-water coral reefs, coral gardens, and deep-sea sponge aggregations.
Vulnerable marine ecosystems are by definition susceptible to low levels of fishing pressure, and some types of spatial management have been shown to be effective to protect VMEs in the deep-sea (Clark and Dunn, 2012; Schlacher et al., 2014). These include confining the bottom trawling effort to already established footprints and establishing spatial closures to protect vulnerable species and habitats (Hourigan, 2014; Clark et al., 2015). In the North East Atlantic several closures have been established by NEAFC and OSPAR in areas beyond national jurisdiction (NEAFC, 2014). In addition, spatial closures to protect VMEs from fishery impacts have been established within the territorial waters of Norway (Fossåand Skjoldal, 2010), Iceland (Ólafsdóttir and Burgos, 2012), and the Faroe Islands (Anonymous, 2014).
A fundamental requirement for spatial management is detailed information on the distribution of VMEs. The optimal and non-destructive methodology to identify the occurrence of VMEs is through the use of underwater imagery, which allows an accurate description of the species composition and the abundance or density of organisms (e.g., Fabri et al., 2014; Anderson et al., 2016a; Beisiegel et al., 2017; Buhl-Mortensen et al., 2017). Nevertheless, the collection of underwater images in deep-sea environments is an expensive and complex endeavor, and therefore in most areas only a limited proportion of the seabed has been mapped visually. Often, the only available information is from the occurrence of VME indicator species in by-catch from fishery surveys and commercial trawls (Murillo et al., 2011; Durán Muñoz et al., 2012; Jørgensen et al., 2014). The lack of information on the distribution of VMEs in the deep sea is hampering the development and application of measures to protect these habitats from impacts of anthropogenic activities (Weaver et al., 2011).
Given the lack of extensive biological data on VMEs in most offshore environments and their presumed wide distribution, species distribution models (SDMs), also known as habitat suitability models or environmental niche models, are increasingly recognized as an effective way to obtain knowledge on the likely distribution of VMEs (Hourigan, 2014; Vierod et al., 2014; Clark et al., 2015). SDMs are models that predict the potential distribution of a species or a group of species in a given area using environmental variables as suitability predictors. Several studies have used SDMs to predict the distribution of VMEs (Howell et al., 2011, 2016) and of VME indicator species (e.g., Rengstorf et al., 2013; Ross and Howell, 2013; Guinotte and Davies, 2014; Anderson et al., 2016b). The use of these models has been recommended for designing management plans to protect VMEs from fishing impacts (Ardron et al., 2014; Vierod et al., 2014). This includes the evaluation of the risk of fishing impacts (Penney and Guinotte, 2013) and the selection of areas for spatial closures (Lagasse et al., 2015; Rowden et al., 2019).
Within the Nordic Seas, the presence of VMEs has been documented by visual habitat mapping programmes in Norway and Iceland (Ólafsdóttir and Burgos, 2012; Buhl-Mortensen et al., 2015b), and records of VME indicator taxa have been obtained from commercial fisheries and scientific surveys. But predictive models of the distribution of VMEs and VME indicator taxa have not been developed in this area, except for Howell et al. (2016), who modeled the distribution of deep-sea sponge aggregations, and the predicted distribution of biotopes produced by the MAREANO programme in several regions in Norwegian waters (Elvenes et al., 2014; Buhl-Mortensen et al., 2015b; Gonzalez-Mirelis and Buhl-Mortensen, 2015). Here we present predictive models for a suite of indicator taxa of the most important VMEs in the Nordic Seas and adjacent areas.
2. Materials and Methods
2.1. Study Area
The study area ranged between 56°N and 80°N and was centered in the Nordic Seas, including the Norway Sea, the Greenland Sea, and the Icelandic Sea, and a portion of the Barents Sea west of 38°E (Figure 1). The area includes the Exclusive Economic Zone (EEZ) of Iceland and Norway, most of Svalbard's Fisheries Protection Zone, part of the EEZ of Greenland, the United Kingdom, and Ireland, and the Exclusive Fisheries Zone of the Faroe Islands. The study area also includes the entire NEAFC's Regulatory Area 2 (known as the “Banana Hole”) and part of Regulatory Area 1. The study area is encompassed mostly in region I of OSPAR (e.g., the Arctic), but includes also small proportions of regions II, III, and V.
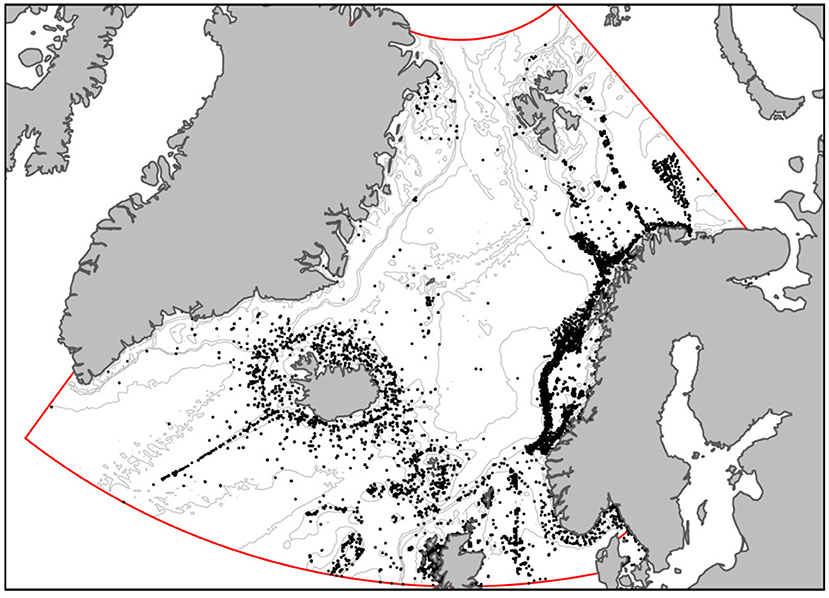
Figure 1. Map of the study area, indicated by the red line. Black dots indicate the location of records of VME indicator taxa. Gray lines are the 100, 500, 1,000, and 2,500 m depth contours.
This area can be divided into three main basins separated by the northern extension of the mid Atlantic Ridge and the Greenland-Iceland-Scotland Ridge (GISR). The oceanography of the area is characterized by relatively warm surface water supplied from the south by the North Atlantic Drift, an extension of the Gulf Stream, overlying colder water masses (Norwegian Sea Deep Water, Arctic Intermediate Water) supplied from deep-water formation in Arctic areas (Buhl-Mortensen et al., 2015c). In coastal areas, the water is influenced by run-off from land. The seasonal variation is much less in the deeper waters than in the upper layers. Current velocities are controlled by the flow of the water masses and the tide, modified by the seabed topography. The GISR has a major impact on the distribution of water masses. The main pathway of water crossing this ridge is through the Wyville-Thomson Ridge between the Faroe Islands and Scotland. Here, the warm NAD passes into the Norwegian Sea above a sill of approximately 500 m. South of the Wyville-Thomson Ridge, the NAD water extends deeper and overlies a watermass characterized by water from the Mediterranean Ocean (the Mediterranean Outflow Water). The ridge system from Greenland to Scotland represents a major geographic barrier with great implications for distribution of marine species (Brix and Svavarsson, 2010; Dauvin et al., 2012; Omarsdottir et al., 2013).
2.2. Biological Data
Records of VME indicator species in the Nordic Seas were compiled from an extensive set of sources. Data were extracted from several databases in Norway, Iceland, and the Faroe Islands, including data from the Benthic Invertebrates of Icelandic waters (BIOICE) and the Marine Benthic Fauna of the Faroe Islands (BIOFAR) projects, and the Institute of Marine Research (IMR) coral database. In addition we used unpublished data from habitat mapping surveys by the Marine and Freshwater Research Institute (MFRI) in Iceland (Ólafsdóttir and Burgos, 2012) and the MAREANO project in Norway (Buhl-Mortensen et al., 2015b), and recent video observations carried out in the Faroe Islands (Buhl-Mortensen et al., 2019). We included by-catch data from the Joint Annual Norwegian-Russian Ecosystem Surveys in the Barents Sea (Jørgensen et al., 2015), and from the MFRI autumn surveys. We also extracted data from the ICES VME database (Morato et al., 2018), and the Ocean Biogeographic Information System (OBIS, Grassle, 2000). Finally, records were extracted from the literature including published data from the early expeditions, and the more recent work by Copley et al. (1996), Klitgaard and Tendal (2004), Mortensen et al. (1995, 2001), Cárdenas and Rapp (2015), and Hestetun et al. (2017). A complete list of the publications used to obtain records of VME indicator species can be found in Buhl-Mortensen et al. (2015b) and as an appendix in Buhl-Mortensen et al. (2019).
2.3. Selection of VMEs and Indicator Species
For the selection of the relevant vulnerable marine ecosystems and their indicator species for the Arctic and sub-Arctic area of this study, we considered: the VME classifications made by the Convention for the Protection of the Marine Environment of the North East Atlantic (OSPAR, 2010b); the list of VMEs compiled by the North East Atlantic Fisheries Commission (NEAFC, 2014) and the Northwest Atlantic Fisheries Organization (NAFO, Fuller et al., 2008); and the revised list of deep-water VMEs with characteristic taxa for ICES/NAFO waters by the ICES Workshop on Vulnerable Marine Ecosystem Database (WKVME, ICES, 2016). In addition we used recent experience gathered by national mapping projects in the study region. We selected 44 indicator taxa of 11 VME types and sub-types in the study area (Table 1). In total, 21 models were at the species level (e.g., Acanella arbusculla). The remaining 23 models were fitted at the genus level, either because most of the compiled record originated from video observations and the species could not be identified (e.g., Stryphnus sp.), or because there were several species of the same genus and the number of records was too low to allow modeling of individual species (e.g., Cladorhiza sp.). The VME types included in this study are the following:
2.3.1. Soft Bottom Sponge Aggregationse
In the Nordic Seas, demosponges of the order Tetractinellida form dense aggregations commonly known as “ostur” or “cheese bottom.” These species can occur at depths between 150 and 1,700 m, on gravel and coarse-sand bottoms (Klitgaard and Tendal, 2004; Murillo et al., 2012; Maldonado et al., 2015). Two main types of ostur assemblages were recognized by Klitgaard and Tendal (2004) : the boreal “ostur” and the cold water “ostur.” The boreal “ostur” is, according to Klitgaard and Tendal (2004), characterized by Geodia barretti, G. macandrewii, G. phlegraei, G. atlantica, Stelletta normaini, and Strypnhus ponderosus, although more recently it has been suggested that the latter species correspond to Strypnhus fortis (Cárdenas and Rapp, 2015; Maldonado et al., 2015). Boreal “ostur” assemblages were observed on some areas of the western Barents Sea, the Norwegian shelf (Kutti et al., 2013; Gonzalez-Mirelis and Buhl-Mortensen, 2015) and Faroese shelf (Klitgaard et al., 1997; Davison et al., 2019), and off southern Iceland (Klitgaard and Tendal, 2004). The cold water “ostur” is characterized by G. hentscheli, G. parva, and Stelletta raphidiophora, and it is found off northern Iceland, the Denmark Strait, off East Greenland, and north of Spitzbergen (Klitgaard and Tendal, 2004). The models of the distribution of species of the genus Geodia in the North Atlantic made by Howell et al. (2016) agreed with the observed distribution patterns. We fitted eight models of sponges considered indicators of soft bottom sponge aggregations: six models based on the Geodia species, and two models for sponges of the genera Stryphnus and Stelletta.
2.3.2. Hard Bottom Sponge Aggregations
A range of medium- to large-sized sponges occur on hard substrates including bedrock, lithified crust, and rocks. In the study area these include various axinellid sponges from the genera Axinella and Phakellia, and the demosponges Antho dichotoma and Mycale lingua. Off northern Norway, hard bottom demospongiae represents a single community (Gonzalez-Mirelis and Buhl-Mortensen, 2015). In addition to these four taxa, the ICES WGDEC (ICES, 2016) considered the family Tetillidae (genera Crainella and Tetilla), as well as sponges of the genera Polymastia and Tethya also to be indicators of hard bottom sponge aggregations, and these are frequently recorded in the Nordic seas (Buhl-Mortensen et al., 2012, 2015b). Models were fitted to seven indicator taxa of this VME.
2.3.3. Deep Arctic Sponge Aggregations
Several species of hexactinellid sponges are found in relatively high densities in deep cold (<0 °C) waters. One of the most common species in the Norwegian Sea is Caulophacus arcticus, which is generally found on hard bottoms at the lower part of the continental slope (Tendal and Barthel, 1993; Buhl-Mortensen et al., 2015b), and has been observed on the base of the Schultz Massif Seamount, at depths below 1,400 m (Roberts et al., 2018). Hexactinellid sponges of the genus Asconema can also constitute sponge grounds, although in restricted geographical settings (ICES, 2008). Asconema foliata has been observed on seamounts (Roberts et al., 2018), and is considered as a main habitat builder associated to cold water “ostur” habitats (Maldonado et al., 2015). In addition, poecilosclerid demosponges of the family Cladorhizidae become numerous at depths below 400 m, and at greater depths they constitute a large fraction of the sponge fauna (Hestetun et al., 2017). Several species of these carnivorous sponges of the genera Chrondocladia, Cladorhiza, and Lycopodina have been reported in the Nordic Seas (Hestetun et al., 2017). They are usually found in low densities, although aggregations of Chondrocladia grandis and Cladorhiza sp. have been observed off northern Iceland. Models were fitted to five indicator taxa of this VME.
2.3.4. Soft Bottom Coral Gardens
The term “coral garden” refers to relatively dense aggregations of colonies or individuals of one or several coral species (OSPAR, 2010a). They can be classified by substrate type (soft and hard bottoms) and the main representative taxa (ICES, 2016). Soft bottom coral gardens can be comprised by gorgonians of the families Isididae and Chrysogorgiidae, which can form dense aggregations on sandy mud (Buhl-Mortensen et al., 2015d). Among these, Isidella lofotensis is found almost exclusively off Norway (Buhl-Mortensen et al., 2015c), although it has been reported off east Greenland (Mayer and Piepenburg, 1996). Radicipes sp. aggregations have been observed off Norway only on the area known as the Bjørnøya slide, but it seems to be more widely distributed south of Iceland (Buhl-Mortensen et al., 2015c). In the warmer waters off southern Iceland the bamboo coral Acanella arbuscula is also relatively common. Soft-bottom coral gardens can also be comprised of solitary scleractinean corals of the genus Caryophyllia and Flabellum aggregated in relatively high densities forming what is known as “cup coral fields” (Baker et al., 2012; Buhl-Mortensen et al., 2015d). Models were produced for six indicator taxa of this VME.
2.3.5. Hard Bottom Coral Gardens
Hard-bottom coral gardens often occur in locations with strong currents. In the study area three of the subtypes from the ICES VME classification (ICES, 2016) are relevant: hard bottom gorgonian gardens, stylasterid corals on hard bottom, and cauliflower coral fields. In the Nordic Seas, the main indicator taxa of gorgonian gardens are Paragorgia arborea, Paramuricea sp., and Primnoa resedaeformis. In addition, Anthomastus sp. is also frequent south of Iceland. Hydrocorals from the family Stylasteridae are not commonly observed in large aggregations but form part of mixed coral communities. Cauliflower corals of the family Nephtheidae (Duva florida, Drifa glomerata, and Gersemia sp.) are widely distributed and dense aggregations have been observed in video surveys off NW and SE of Iceland at 500–600 m (Buhl-Mortensen et al., 2019), and off northern Norway (ICES, 2011).
2.3.6. Reef-Forming Scleractineans
In Nordic waters only three species of scleractinean corals are reef building: Lophelia pertussa, Madrepora oculata, and Solenosmilia variabilis. Among them, L. pertusa is the most common and has been recorded frequently on the Norwegian shelf, around the Faroe Islands and off southern Iceland. M. oculata is less abundant, has a more limited framework-building capacity, and it often co-occurs with L. pertusa (Roberts et al., 2009). In our study area, S. variabilis has been observed deep on the Reykjanes Ridge south of Iceland (Copley et al., 1996). Reef forming scleractineans do not always form reefs. For example, on vertical solid substrates coral debris cannot aggregate and reefs do not develop (Buhl-Mortensen et al., 2015d). In the North Atlantic reef-forming scleractineans can also form densely-packed “thickets,” as part of hard bottom coral gardens, or as isolated colonies (Mortensen and Buhl-Mortensen, 2004, 2005; Davies et al., 2017). These growth forms are usually considered to represent different habitats. For example, the ICES VME classification distinguishes between cold-water coral reefs, non-reefal scleractineans, and colonial scleractineans on rocky outcrops (ICES, 2016). Because the growth form is seldom known or reported all observations of each taxon were grouped under a single VME type. Three models were fitted using presence data of each of the three reef-forming scleractineans.
2.3.7. Shallow Sea Pen Communities
Sea pen communities are usually defined as areas of bioturbated fine sediments with relatively high densities of sea pens. In OSPAR's list of threatened and/or declining habitats, this biotope is termed “sea-pens and burrowing megafauna communities” (Curd, 2010). This biotope is found in the relatively warm Atlantic water shallower than 700 m. The most common sea pen species are Funiculina quadrangularis, Virgularia mirabilis, Pennatula phosphorea, and Kophobelemnon stelliferum. Here we fitted four models based on records of these four species.
2.3.8. Deep-Sea Sea Pen Communities
The sea pen species Umbellula spp. and Anthoptilum spp. occur in deep waters (below 700 m) in an environment with colder temperatures and less anthropogenic activities than shallow water sea pens, and therefore should be regarded as a separate sea pen VME or at least a distinct sub-type (Buhl-Mortensen et al., 2019). High densities of Umbellula encrinus are found in deep waters north of Iceland and on the Norwegian slope, at depths below 800 m in the Norwegian Sea-Arctic Intermediate water. This large sea pen can reach a height of three meters. Off southern Iceland, sea pens of the genus Anthoptilum are also found in deep, albeit warmer waters. Models were fitted for both species.
2.4. Environmental Predictors
Bathymetry data for the study area was obtained from the General Bathymetric Chart of the Oceans (GEBCO version 20150318, Weatherall et al., 2015), a global relief model with a resolution of 30 arc-seconds. The data was projected using a Lamberts Equal Area projection centered at 69°N and 4°W and bilinearly interpolated to obtain a grid with a resolution of 500 m. All other environmental data sets were adjusted to match the same projection and resolution using bilinear interpolation.
The seabed morphology was characterized following Lecours et al. (2017), using the following parameters derived from the 500 m bathymetry grid: local mean depth, slope, aspect (divided into northness and eastness), bathymetric position index (BPI), and vector roughness. The BPI indicates if a particular pixel forms part of a positive or negative feature of the surrounding terrain (Wilson et al., 2007). Vector roughness on the other hand measures the topographic surface roughness by quantifying the local variability in slope and aspect (McKean and Roering, 2004). Terrain analysis variables were calculated using a moving window of 3 cells, corresponding to a scale of 1,500 m, by fitting a bivariate quadratic polynomial to each window size using least squares. In addition BPI and vector roughness were also calculated using a moving window of 21 cells, corresponding to a scale of 10,500 m.
Temperature and salinity profiles for the study area were obtained from the NISE project (Norwegian Iceland Seas Experiment; Nilsen, 2008). Near-bottom temperature and salinity gridded fields were estimated following the methodology described in Jochumsen et al. (2016). The data going into the gridding corresponded to the deepest observation point. To avoid including shallow profiles, we only used observations obtained deeper than 80 % and within 80 m of the bottom depth. The gridding was then performed on a spatial resolution of 0.2° longitudinal by 0.1° latitudinal using an objective analysis and with an influence radius of 50 km. Minimum and maximum temperature, temperature difference (the difference between the lowest and highest temperature values), and mean salinity were calculated by interpolating along topography following Davis (1998) using a topography length scale of 300 km (Voet et al., 2010; Skagseth and Mork, 2012).
The aragonite saturation state for the study area was obtained from data provided by Jiang et al. (2015), and interpolated using a similar methodology to the temperature and salinity. Since the aragonite data is much more limited, the criteria for data to be included in the gridding was relaxed. We only required the observation depth to be within 80% of the bottom depth, and further we increased the influence radius to 200 km.
Monthly averages of mean net primary productivity (mg C m−2 day−1, NPP) estimated from MODIS data using the carbon-based Production Model (CbPM) (Behrenfeld et al., 2005; Westberry et al., 2008) were obtained from the Ocean Productivity site1. Data was downloaded for the period 2006-2015 with a resolution of 5 arc min. Particulate organic carbon flux to the sea bottom (POC flux; g C m−2 year−1) was estimated from the bottom depth and the seasonal variation in NPP, defined as the ratio between the standard deviation and the mean of monthly NPP values (Lutz et al., 2002, 2007).
Data on near-bottom average current speed and concentrations of nitrate, phosphate, and silicate were obtained from the Bio-ORACLE v2.0 database using the package “sdmpredictors” (Assis et al., 2018) in the R statistical environment (R Core Team, 2019), which provides layers of near-bottom physical and chemical parameters. Current velocity data (m.s−1) was produced by the Global Ocean Physics Reanalysis (ECMWF) using the OCEAN5 system at a native resolution of 0.25° in the horizontal and 75 levels in the vertical, with the separation between vertical levels increasing with depth. Nutrient concentrations (mmol.m−3) were derived from the Global Ocean Biogeochemistry Non-assimilative Hindcast (PISCES). In both cases, data was obtained from the E.U. Copernicus Marine Service Information2, and statistically downscaled to a resolution of 5 arcmin using a kriging model (Assis et al., 2018).
Collinearity among environmental layers was explored by computing the Variance Inflation Factor (VIF) (Dormann et al., 2013). Variables with high collinearity were eliminated through a stepwise procedure in which the VIF was calculated for all variables, the variable with highest VIF was removed, and VIFs were recalculated until all variables had a VIF value lower than 10 (Naimi et al., 2014). This procedure selected maximum temperature, nitrate and phosphate as variables causing collinearity, therefore these parameters were not included as predictors. The correlation among remaining variables is shown in Figure 2. In the case of MaxEnt, it has been suggested that variables should be selected based on previous knowledge on the biology and ecology of the modeled species, but that stricter selection of variables is unlikely to improve models (Elith and Graham, 2009; Elith et al., 2011). Therefore we did not attempted to select particular sets of predictor variables for individual taxa, and all models were constructed using all available variables.
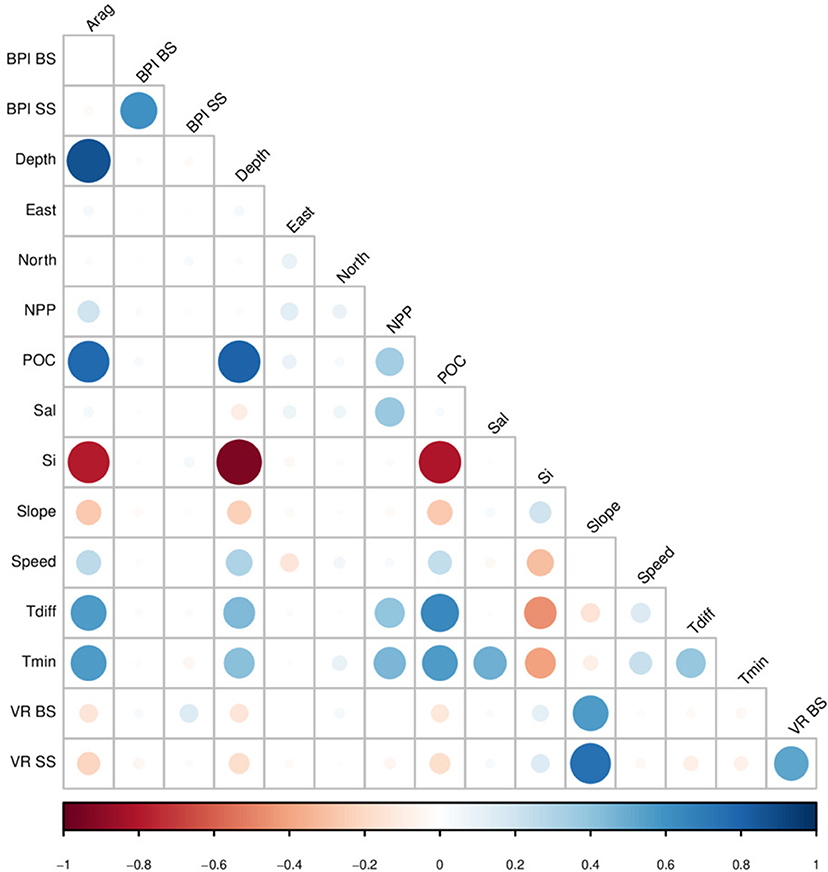
Figure 2. Correlation matrix among the environmental variables used as predictor for the species distribution models: Aragonite saturation state (Arag), broad-scale bathimetric position index (BPI BS), small-scale bathimetric position index (BPI SS), bottom depth (Depth), eastness (East), northness (North), mean net primary productivity (NPP), particulate organic carbon (POC), mean salinity (Sal), silica concentration (Si), bottom slope (Slope), near-bottom current speed (Speed), temperature difference (Tdiff), minimum temperature (Tmin), broad-scale vector roughness, and small-scale vector roughness. Colors and size of circles indicate correlation values, from 1 (blue) to –1 (red).
2.5. Modeling Approach
In this study, rather than modeling the distribution of a VME by using the combined records of all indicator taxa (as done e.g., by Buhl-Mortensen et al., 2019), we opted to model each taxon individually. The rationale for this approach is that some of the VMEs include indicator taxa with different environmental requirements. For example, the corals Isidella lofotensis and Radicipes sp. are both considered indicators of soft-bottom coral gardens, but I. lofotensis is almost exclusively found on the Norwegian shelf, while Raicipes sp. is much more common off southern Iceland (Buhl-Mortensen et al., 2015c). A single model with records of both taxa will overestimate their distribution because a wider range of environmental settings would be considered suitable. To avoid this, we choose to (a) model individually each of the 44 taxon, (b) measure the similarity among the predicted distributions using the “I” similarity statistic (Warren et al., 2008), (c) use cluster analysis to identify groups of taxa with similar predicted distributions, and (d) combine the predictions of the taxa of each group using a stacked species distribution approach in order to obtain a predictive map of the distribution of each group. Finally, a VME index was computed to map the distribution and relative vulnerability of VMEs in the Nordic Seas.
2.6. Models of Individual Taxa
The distribution of VME indicator taxa was predicted using species distribution models (SDMs), which predict the geographic distribution of a species by identifying the combinations of environmental variables where they are observed to be more prevalent and then mapping that combination of variables into geographic space. We predicted the distribution of suitable habitats for VME indicator taxa using the maximum entropy algorithm MaxEnt (version 3.4.1). MaxEnt is a machine learning model that uses a presence-only approach to quantify the relationship between environmental variables at locations where a species has been observed vs. background locations in the study region (Phillips et al., 2006). MaxEnt uses transformations of the original environmental variables named “feature classes” (FC), namely the linear, product, quadratic, hinge, threshold, and categorical feature classes (Elith et al., 2011). Different combinations of feature classes allow the construction of very flexible models. By default, MaxEnt selects the number of feature classes based on the number of presence observations, increasing the number of feature classes with the number of records. To avoid overfitting, MaxEnt uses regularization, which penalizes the inclusion of parameters that produce small improvements in the model (Merow et al., 2013). Regularization is controlled by a parameter termed regularization multiplier (RM, default value = 1). Higher RM values reduce the flexibility in the relationships between species presence and environmental predictor variables. The performance of SDM models is sensible to model specifications (Elith et al., 2011; Merow et al., 2013; Warren et al., 2014). Recent studies have shown that the default MaxEnt options (i.e., the RM value and feature classes used) can produce models that perform poorly (Radosavljevic and Anderson, 2014). To select model settings that approximate optimal levels of model complexity, models were fitted to each VME indicator taxon using different combinations of feature classes and regularization multiplier values using the “ENMeval” (Muscarella et al., 2014) and “dismo” (Hijmans et al., 2017) packages in the R statistical environment (R Core Team, 2019). Each model was fitted using k-fold validation with five bins. To predict the distribution of each VME indicator taxon we selected the model with the highest average test AUC (area under the curve of the receiver operating characteristic plot), averaged across the 5-folds. This was the model with the best capability to successfully discriminate occurrence from background localities (Muscarella et al., 2014). In addition, we evaluated the Symmetric Extremal Dependence Index (SEDI, Wunderlich et al., 2019). SEDI is analogous to the widely used True Skill Statistic (TSS, Allouche et al., 2006) but better behaved in presence-background models because its error weighting reflects the low confidence in the pseudo-absence data, in particular in models with low prevalence and a high number of background points as the models in this study (Wunderlich et al., 2019). The SEDI for each model was estimated using a confusion matrix obtained after converting the model prediction into a binary presence-absence raster using the threshold that maximizes the sum of sensitivity and specificity (maxSSS), minimizing commission and omission errors (Liu et al., 2016). This threshold is commonly used to transform the output of SDMs into a binary output (Liu et al., 2005; Elith et al., 2006).
To evaluate if the models were overfitting we examined two metrics. The first is the difference between training and testing AUC, averaged across the 5 random folds (Warren and Seifert, 2011). This metric is expected to be high if models are overfitting the data (Muscarella et al., 2014). The second is the values of the 10% training omission rate (OR10). This is a threshold-dependent metric equivalent to the proportion of test localities with predicted suitability values lower than excluding the 10% of training localities with the lowest predicted suitability. Omission rates higher that the expected value of 10% typically indicate model overfitting (Muscarella et al., 2014; Radosavljevic and Anderson, 2014). Higher values are sometimes used to distinguish between degrees of overfitting. Here we followed Kivlin et al. (2017) by considering values below 0.2 as indicators of models with relatively low degrees of overfitting.
The model selected was used to indicate the distribution of VME indicator taxa in the study area based on the predicted occurrence of suitable habitat. Predictions were obtained for each cell in the same 500 m grid used for the environmental predictors. Model predictions were exported in the cloglog scale, which under specific conditions can be approximated to a probability of presence (Phillips et al., 2017). In each model we computed the permutation importance of each predictor variable, which is the drop in AUC resulting from randomly permuting the values of the predictor variable on the training and background data sets and reevaluating the model (Phillips et al., 2006). Finally, models were examined visually to evaluate that high suitability areas corresponded to the locations of the majority of observations (Radosavljevic and Anderson, 2014).
2.7. Target Group Background
The spatial distribution of the available records of VME indicator species showed a strong sampling bias within the study area. In some areas the sampling intensity was high, in particular on areas of the Norwegian shelf and in the Barents Sea that have been mapped by the MAREANO programme (Buhl-Mortensen et al., 2015b), but also to some degree the Icelandic and Faroese shelves. In other areas like the eastern Greenland shelf and the deep basins the sampling effort has been very low or non-existent, or existing data is unavailable. Sampling bias can strongly influence the reliability of predictions of presence-background modeling. One way to reduce the effect of sampling bias in presence-only models is to use a set of background points with the same bias as the sampling effort (Phillips et al., 2009; Fourcade et al., 2014). To do this we modeled the sampling effort in the study area by fitting a kernel density estimate (KDE) to the locations of all indicator taxa compiled in the database, an approach known as target-group sampling (Elith et al., 2011; Merow et al., 2013). The KDE produced an estimation of the density of samples in each cell of the 500 m grid. These estimates were normalized so the sum of all cells was equal to one. These values were used to select 50,000 background points using the normalized kernel density values as a probability grid.
2.8. Niche Similarity
Niche overlap among all VME indicator taxa was estimated using the “I” similarity statistic (Warren et al., 2008), which ranges between 0 (no overlap) and 1 (niches are identical). To verify if VME classes currently used (e.g., ICES, 2016) consisted of indicator taxa with similar predicted distribution we carried out a cluster analysis using the Ward method, which defines groups by minimizing the within-group sum of squares (Legendre and Legendre, 1998). As a measure of dissimilarity between predicted distributions we used the complement of the “I” similarity statistic (1 - I). Groups of VME indicator taxa with similar distributions were identified from a dendrogram using a dissimilarity cutoff value of 0.4. This value was selected to produce groups roughly similar to known VME classifications.
2.9. Stacked Species Distribution Models
To map patterns of environmental suitability for VMEs, we merged the predicted distribution of VME indicator taxa to form stacked species distribution models (S-SDMs, Ferrier and Guisan, 2006; D'Amen et al., 2017; Wiltshire et al., 2018). S-SDMs is an approach that follows the “predict first, assemble latter” strategy in which the distribution of each individual taxon is modeled first, and then the predictions are combined (or “stacked") to produce a community prediction (D'Amen et al., 2017). This approach allows for the use of presence-only data where the presence records originated from different sources and where records of species of the same community or habitat are not usually collected at the same location. This is important when modeling deep-sea benthic megafauna in areas where the majority of records originated from fisheries by-catch and from scientific surveys using gear with relatively low sampling efficiency like dredges or bottom trawls (as opposed to underwater video surveys, which provide a more complete description of the benthic megafauna in a particular location). The S-SDMs approach also provides the flexibility of letting different environmental variables influence the distribution of individual species with distinct species-environment relationships (Ferrier and Guisan, 2006).
Here we produced S-SDMs for groups of VME indicator taxa with similar predicted habitat suitability, as defined by the cluster analysis of the “I” similarity statistic. SDMs were produced by averaging the predictions of individual VME indicator taxa (Calabrese et al., 2014; D'Amen et al., 2015; Wiltshire et al., 2018), and scaling the resulting average to a range of values between 0 and 1.
In the absence of knowledge on species prevalence, the output of presence-only models like MaxEnt is monotonically, but not proportionally, related to the relative probability of presence (Elith et al., 2011; Wiltshire et al., 2018), and cannot be interpreted as a measure of abundance or compared between species (Elith et al., 2011; Merow et al., 2013). As a result, the average suitability in each cell is likely to be related to the relative species richness in that location, although the relationship is not necessarily directly proportional (Aranda and Lobo, 2011; Guillera-Arroita et al., 2015; Wiltshire et al., 2018). Therefore, rather than attempting to predict species richness or community composition, we considered the average suitability as a tool to examine patterns of habitat suitability in each VME and to highlight areas of high average suitability that should be examined more closely and targeted for conservation measurements.
2.10. VME Index
To obtain a general overview of the distribution and relative vulnerability of the VMEs in the Nordic Seas, we computed a SDM-based VME Index analogous to the VME index developed for the ICES VME database (Morato et al., 2018). This index is a combination of a set of indicator scores, which quantifies in very broad terms the vulnerability of a taxa or group of taxa to anthropogenic impacts, and abundance scores based on abundance data of each taxon usually obtained from by-catch.
The indicator scores in Morato et al. (2018) were based on the vulnerability criteria defined by FAO (2009): uniqueness or rareness, functional significance, fragility, life-history that makes recovery difficult, and structural complexity. The degree to which each group fit each of the five criteria were scored using a scale between 1 (low) and 5 (high) by a group of experts (Morato et al., 2018). As the five indicators are considered to be approximately orthogonal, an indicator score was computed for each group using the quadratic mean (Morato et al., 2018). We used the indicator scores of the seven VME indicator groups present in our study area (Table 2). Each of the 44 VME indicator taxon in our study were assigned to one of the seven VME indicator groups. Following Morato et al. (2018), sponges of the genera Asconema, Craniella, Geodia, Polymastia, Strypnhus, Tetilla, and Thenea were included in the “large sponges” group, while the remaining genera were considered as “generic sponges.”
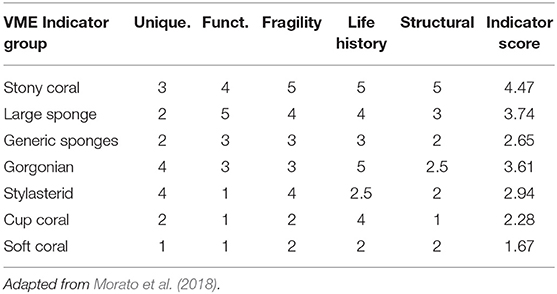
Table 2. Indicator scores for VME taxa groups, based on the degree to which each group fits the FAO VME criteria (FAO, 2009): uniqueness or rareness, functional significance, fragility, life-history that makes recovery difficult, and structural complexity.
The ICES VME index combines the indicator scores with an abundance score where by-catch weights are classified into a 1-5 scale based on current encounter threshold for live corals and live sponges established by NEAFC and the European Union (Morato et al., 2018). In an analogous manner, we scaled the predicted habitat suitability of each VME indicator taxon to a scale of 1-5 by computing the maxSSS threshold (Liu et al., 2016). Cells with values below this threshold received an abundance score of zero. Suitability values above the threshold were linearly scaled into a 1-5 scale, where 1 corresponds to the presence threshold and 5 corresponds to 1 (the maximum possible suitability value). The final SMD-based VME index was calculated by thresholding and scaling each of the predicted suitability for the 44 SDMs into a 1-5 scale, and multiplying by the corresponding VME indicator score. As opposed to Morato et al. (2018) we opted to average the VME index among the 44 indicator taxa, in order to highlight areas suitable for multiple VME indicator taxa.
3. Results
3.1. Model Performance
All models had average test AUC values above 0.85, with 30 models having AUC values above 0.9, which indicates good model performances and capacity to distinguish between observation and background points (Table 3). In addition, all models had relatively high SEDI values which indicate good model performance and a balance of omission and comission errors. In most cases (n = 33) the model with the highest AUC included all four feature classes (linear, quadratic, hinge, and product). This is the default behavior of MaxEnt, which selects the number of feature classes depending on the number of presence records. A total of 17 models had a regularization parameter (RM) of more than 1, which is the default in MaxEnt.
In general, models had a relatively low degree of overfitting, with an average OR10 (10% training omission rate) of 0.17. Only six models had OR10 values equal or above 0.25, which we took as indicator of high overfitting. The models with higher levels of overfitting were Anthothela grandiflora (OR10=0.50, Figure S6), Lycopodina sp. (OR10 = 0.30, Figure S7), Halipteris sp. (OR10 = 0.27, Figure S5) Radicipes sp. (OR10 = 0.26, Figure S6), Geodia phlegraei (OR10 = 0.25, Figure S2), and Isidella lophotensis (OR10 = 0.25, Figure S10). In addition to these, the models of Geodia atlantica (Figure S2), Asconema sp. (Figure S9), and Duva florida (Figure S9) produced relatively high values of AUC diff (the mean difference between training and testing AUC) also suggesting overfitting.
3.2. Importance of Environmental Variables
There was high variability in the explanatory power of the 16 predictor variables, as measured by their permutation importance, on the 44 MaxEnt models (Table 4). Minimum temperature was the most important predictor across all models, with an average permutation importance of 27.4% and explaining more than 10% of the variability in 40 of the 44 models. In individual models the importance of temperature reached up to 78.7% and it was particularly high for cold-water sponges (Caulophacus, Cladorrhiza, Chrondocladia Geodia phlegraei), but also for some cold-water corals (Lophelia pertusa, Madrepora oculata, Flabellum Gersemia), and gorgonians (Paragorgia arborea, Paramuricea, Primnoa resedaeformis, and Anthomastus). In addition, temperature difference had an average importance of 4.8%.
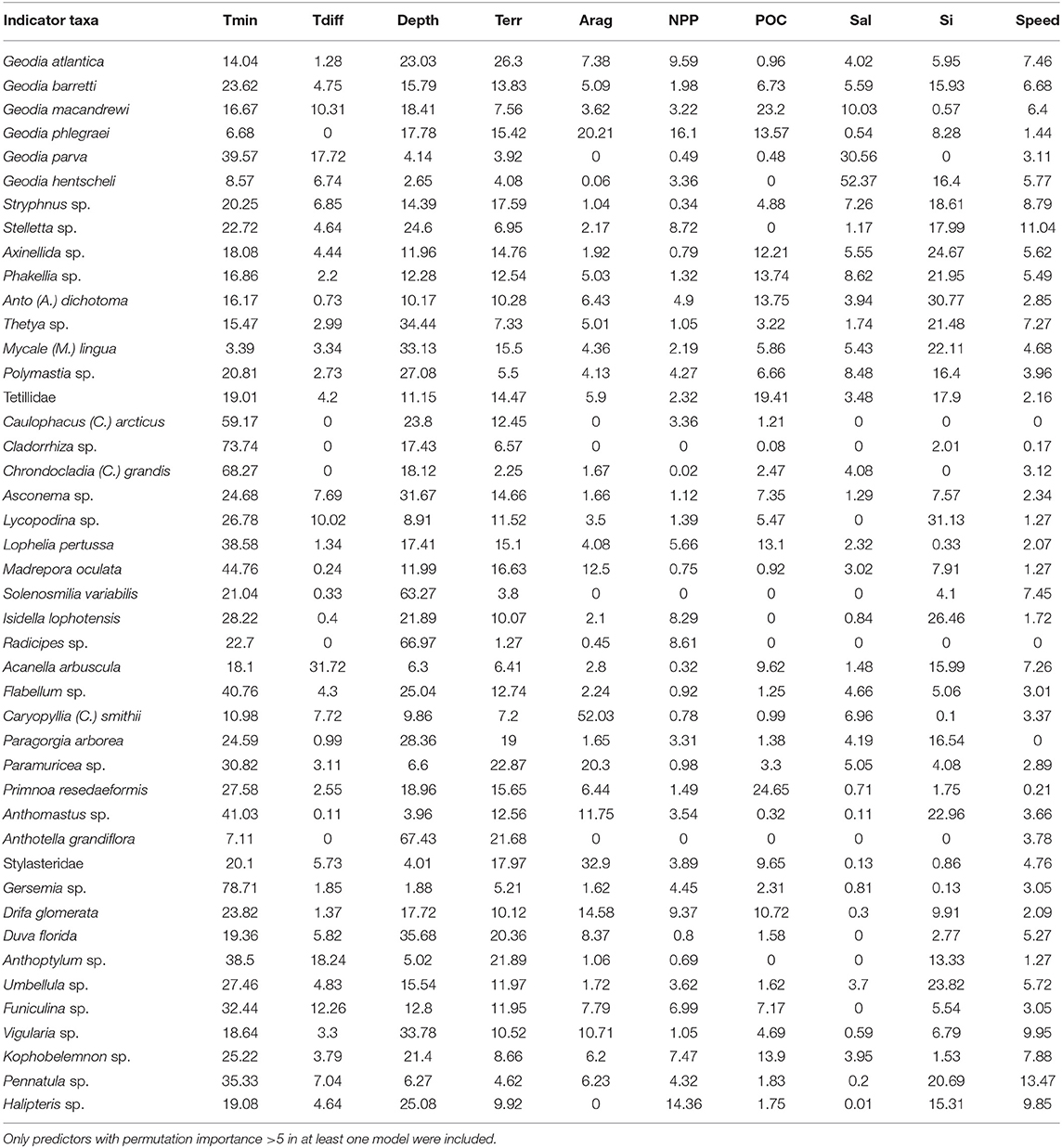
Table 4. Permutation importance of environmental predictors: Minimum temperature (Tmin), temperature difference (Tdiff), bottom depth (depth), combined importance of terrain parameters (Terr), aragonite saturation state (Arag), mean net primary productivity (NPP), particulate organic carbon (POC), salinity (Sal), silica concentration (Si), and near-bottom current speed (Speed).
Depth was the second most important predictor, with an average importance of 20.2% and reaching up to 67.4%. Variables describing the morphology of the seafloor (small and large scale BPI and roughness, slope, northness, eastness) did not have high average importance by themselves (0.13–3.6%), but their averaged combined contribution was relatively large (11.86%) and reached up to 26.3%.
The combined effect of variables related to seawater chemistry (salinity, aragonite saturation state and silica concentration) was also relatively large (average importance 21.9%). Aragonite saturation state had an average importance of 6.5%, but had higher importance on some taxa including Caryophyllia (52.0%), Geodia phlegraei (20.21%), Stylasterids (32.9%), Drifa glomerata (14.6%), and Madrepora oculata (12.5%). In general salinity had a low explanatory power, with an average importance of 4.4%. For two Geodia species, G. parva and G. hentschelli salinity explained a large proportion of the variance.
3.3. Predicted Distributions and Niche Similarity
Predicted distributions of individual taxa are shown in Figures S1–S10. Pairwise niche similarity, measured by the “I” similarity statistic, ranged between 0.03 and 0.95, indicating a wide range of similarities among the predicted distribution of the VME indicator taxa. The cluster dendrogram shows seven groups (1–7) of VME indicator taxa at a dissimilarity level of 0.4 (Figure 3). Three of the groups can be divided into two subgroups each based on their dissimilarity value and the similarity of the spatial patterns of the predicted distribution. These groups represents VME indicator taxa with similar habitat suitability as predicted by the models. The following groups were identified:
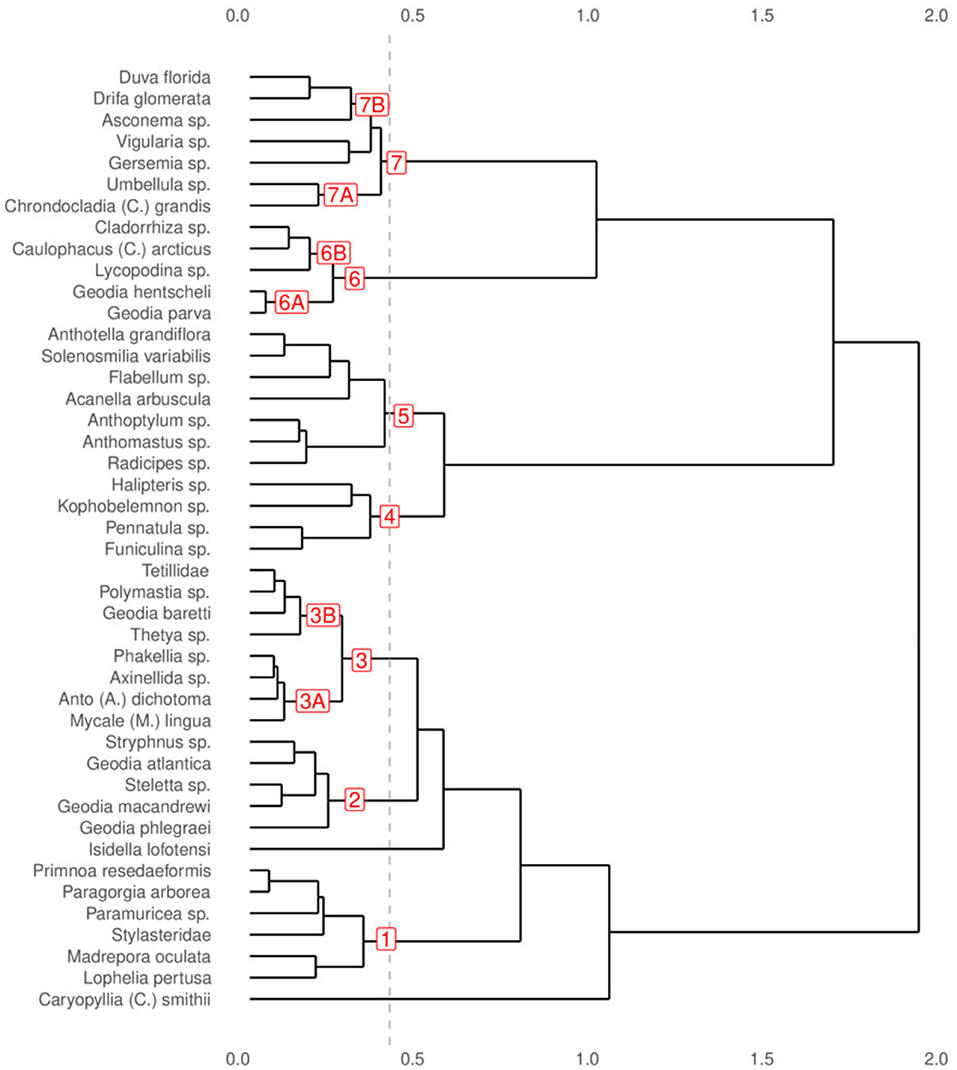
Figure 3. Dendrogram of the predicted distribution of 44 VME indicator taxa, based on a cluster analysis (Ward method) of the complement of the “I” similarity statistic computed among all predicted distributions. Red labels indicate the seven groups identified at the 0.4 dissimilarity value, and the six sub-groups identified by examining the distribution patterns of taxa in each group.
3.3.1. Group 1
The first group includes the reef-forming corals Lophelia pertusa and Madrepora oculata, the gorgonians Parmuricea sp., Primnoa resedaeformis, and Pargorgia arborea, as well as corals of the family Stylasteridae. These species are often found in close proximity (Buhl-Mortensen et al., 2015c). Depending on local conditions, L. pertusa and M. oculata may be the dominant species and form coral reefs or coral thickets, or they can be found as isolated colonies forming part of hard-bottom coral gardens together with the other species in this group. This group of VME taxa is predicted to be distributed in narrow areas on the southern and western Icelandic shelf, around the Faroe Islands, off southern Greenland, and broadly on the central Norwegian shelf (Figure 4A).
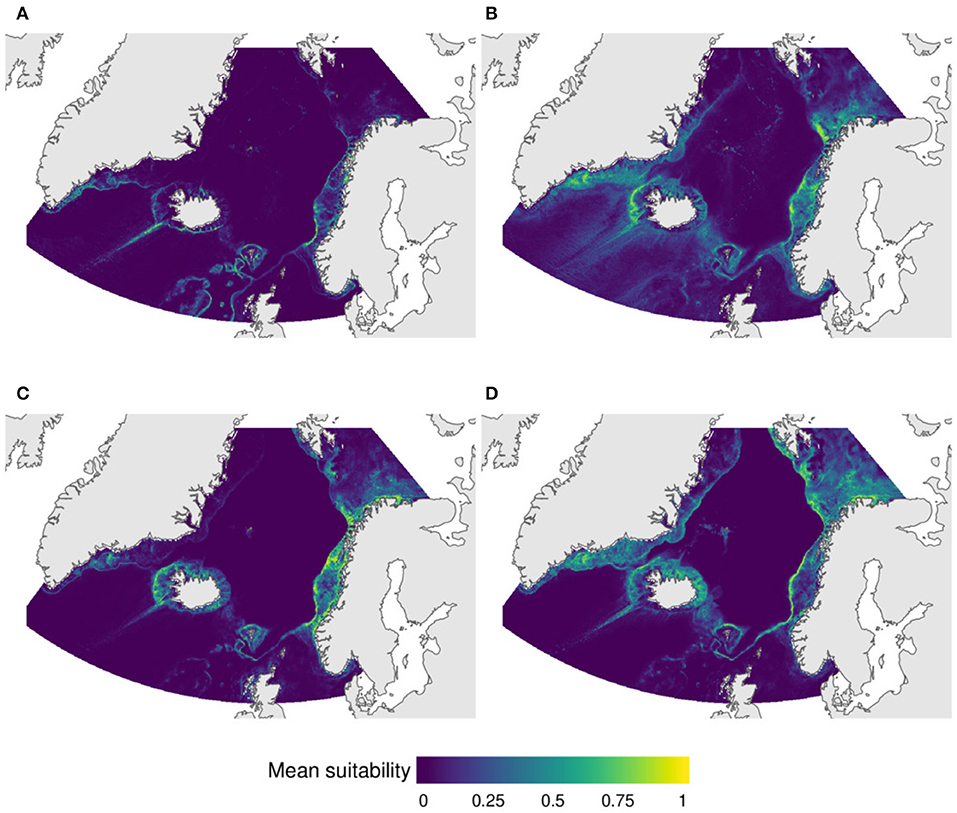
Figure 4. Predicted distribution of VMEs based on stacked species distribution model (SSMD) of (A) the reef-forming corals Lophelia pertusa and Madrepora oculata, the gorgonians Paragorgia arborea, Primnoa resedaeformis, and Paramuricea sp., and Stylasterid corals, (B) ssponges of the taxa Geodia atlantica, G. macandrewi, G. phlegraei, Stryphnus sp., and Stelletta sp., (C) sponges of the taxa Mycale sp., Axinellidae, Phakellia, and Antho (Antho) dichotoma, and (D) sponges of the taxa Thethya sp., Geodia baretti, Polymastia sp., and Tetillidae.
3.3.2. Group 2
The second group includes three Geodia species (G. atlantica, G. macandrewi, and G. phlegraei) considered characteristic of the boreal “ostur” community (Klitgaard and Tendal, 2004), as well as the sponges Stelletta sp. and Strypnhus sp. Klitgaard and Tendal (2004) included Stelletta normani and Strypnhus ponderosus as part of the boreal “ostur,” while Stelletta raphidiophora was considered associated to the cold “ostur” community. Although we modeled Stelletta sp. and Strypnhus sp. at the genus level, given the geographic distribution of our samples it is likely that the majority of our records correspond to the species associated boreal “ostur” (Figure S2). High suitability for this group was observed off western Iceland, the Denmark strait, and the southern Greenlandic shelf, off the Faroe Islands, and in broad areas of the central and northern Norway shelf (Figure 4B).
3.3.3. Group 3
The next group includes a number of sponge taxa usually associated to hard bottoms. Two subgroups can be recognized here. Subgroup 3A included Axinella sp., Phakellia sp., Antho (Antho) dichotoma, and Mycale (Mycale) lingua. Areas of high suitability for this group includes the central Norwegian shelf, the western and southern Icelandic shelf, and off the Faroe Islands (Figure 4C). Subgroup 3B included Thetya sp., Polymastia sp. and Tetillidae, and also Geodia baretti, which is one of the Geodia species usually considered as part of the boreal “ostur” community (Klitgaard and Tendal, 2004). This group was associated to the colder waters of the Barents Sea, the Greenlandic shelf and off north Iceland, and to the shelf break off Norway (Figure 4D).
3.3.4. Group 4
This group includes four indicator taxa of the shallow sea pen VME: Funiculina sp., Pennatula sp., Kophobelemnon sp., and Halipteris sp. Individual models for these taxa suggest that the four taxa have areas of high suitability south of the GISR, on the Skagerrak and in the North Sea, while only Funiculina sp. and Kophobelemnon sp. have relatively high suitability on the Norwegian shelf. The SSDM of these four taxa indicate some areas of high combined suitability on the southern Icelandic shelf and on the Skagerrak strait (Figure 5A).
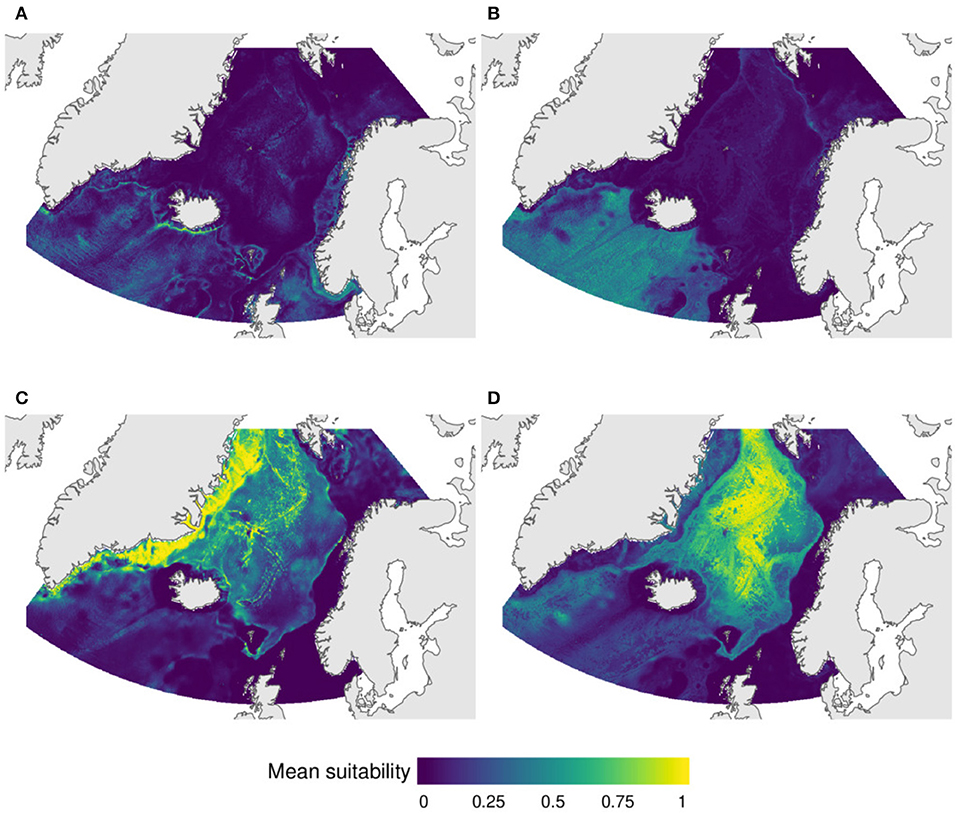
Figure 5. Predicted distribution of VMEs based on stacked species distribution model (SSMD) of (A) the sea pens Funiculina sp., Pennatula sp., Kophobelemnon sp., and Halipteris sp., (B) the gorgonians Anthotella grandiflora,/Radicipes/ sp. and Anthomastus sp., the deep-water sea pen Anthoptilum sp., the cup coral Flabellum sp., and the reef-forming coral Solenosmilia variabilis, (C) the sponges Geodia parva and G. hentschelli, and (D) the sponges Caulophacus (C.) arcticus, Chladorhiza sp., and Lycopodina sp.
3.3.5. Group 5
This group incorporates a mixture of taxa with predicted suitability mostly south of the GISR including the gorgonians Radicipes sp., Anthotella grandiflora, and Anthomastus sp., the deep-water sea pen Anthoptilum sp., the cup coral Flabellum sp., and the reef-forming coral Solenosmilia variabilis (Figure 5B).
3.3.6. Group 6
This group consists of two subgroups of sponge taxa associated to cold waters. Subgroup 6A included Geodia parva and G. hentschelli, two species characteristic of the cold “ostur” assemblage (Klitgaard and Tendal, 2004). This group showed high suitability on the Greenlandic shelf (Figure 5C). Subgroup 6B included three taxa of carnivorous sponges Caulophacus (C.) arcticus, Chladorhiza sp., and Lycopodina sp., and is predicted to be present mostly on the deep basins on the Norway and Greenland seas (Figure 5D).
3.3.7. Group 7
This group incorporates VME indicator species mostly associated to the continental slopes north of the GISR. Two subgroups can be identified in this group. Subgroup 7A included the deep-water sea pen Umbellula sp. and the carnivorous sponge Chrondocladia (C.) grandis, with predicted distribution mainly restricted to the deep continental slopes (Figure 6A). Subgroup 7B consisted of the VME cauliflower coral fields indicator species Duva florida, Drifa glomerata, and Gersemia sp., together with the sea pen Virgularia sp. and the carnivorous sponge Asconema sp. Its predicted distribution included the continental slope but also on broader areas of the shelves off Greenland, northern Iceland and the Faroe Islands, and on the Barents Sea (Figure 6B).
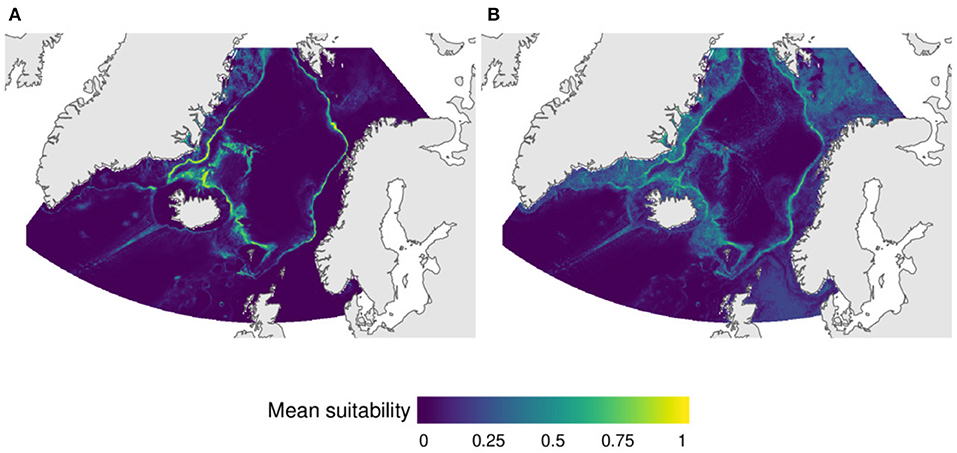
Figure 6. Predicted distribution of VMEs based on a stacked species distribution model (SSMD) of (A) the deep-water sea pen Umbellula sp. and the carnivorous sponge Chrondocladia (C.) grandis, and (B) the cauliflower corals Duva florida, Drifa glomerata, and Gersemia sp., the sea pen Virgularia sp. and the carnivorous sponge Asconema sp.
The two VME indicator taxa Isidella lofotensis and Caryophylla smithii had predicted distributions distinctly different from the others and were not part of any cluster. The gorgonian I. lofotensis is mainly restricted to Norwegian waters, but has been reported off northern Greenland (Mayer and Piepenburg, 1996; Buhl-Mortensen et al., 2015c), with a predicted distribution also including some areas around the Jan Mayen archipelago (Figure S10). The observed and predicted distributions of the cup coral C. (C.) smithii was restricted to some areas between the Shetland Islands and Norway, and within the North Sea.
3.4. VME Index
Values for the computed VME index ranged between 0 and 8.4 (Figure 7). Areas with high VME index values indicate locations where multiple VMEs are expected to be present, and/or where the VMEs present had high values in the indicator scores quantifying the vulnerability criteria defined by FAO (2009). The VME index suggest that even though some VME indicator taxa are predicted to have broad distributions, areas with high VME index values are more restricted. These areas include most of the Norwegian continental slope between 62°N and 71°N, coastal areas in the Barents Sea, the shelf break off the Faroe Islands and on the Faroe Bank, the southern and western Icelandic shelves, areas in the Reykjanes Ridge and the Kolbeinseyn Ridge, and some areas in the southern Greenlandic shelf and slope.
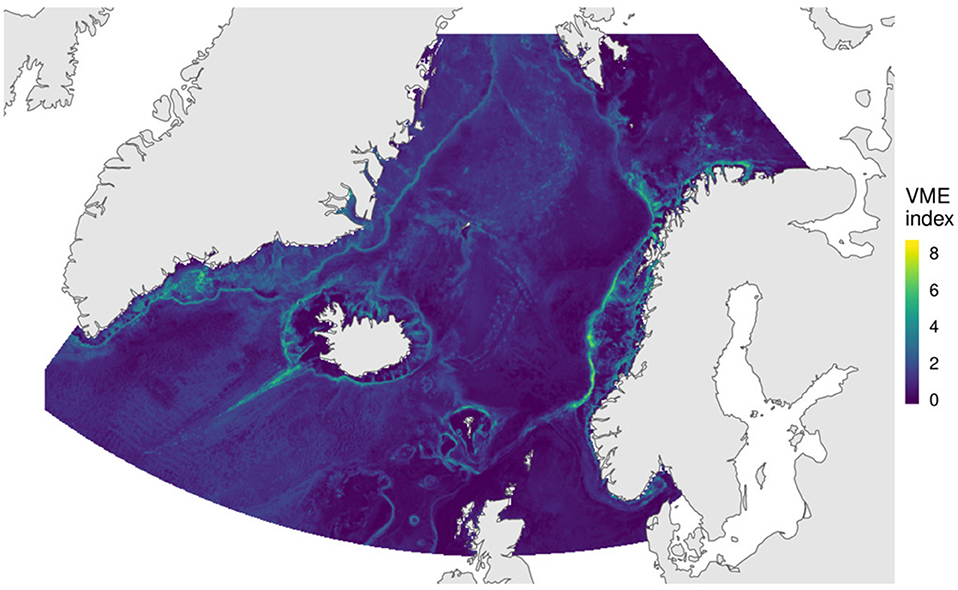
Figure 7. VME index combining indicator scores by Morato et al. (2018), and abundance scores from the species distribution models of 44 indicator taxa.
4. Discussion
Here we presented the first comprehensive broad-scale modeling effort for VME indicator taxa on the Nordic Seas, including Icelandic and Faroese waters. Models of VME indicator taxa have been produced at more local scales within this area, in particular off Norway (Gonzalez-Mirelis and Buhl-Mortensen, 2015; Sundahl, 2017). Previous efforts in broad-scale models for cold-water corals (e.g., Davies and Guinotte, 2011; Yesson et al., 2012) did not include our study area, and with the exception of Howell et al. (2016), who focused on Geodia sp., no broad scale models have been produced for VME indicator taxa for this area. The models presented in this study significantly expand the knowledge on the potential distribution of VME indicator taxa in Arctic and sub-Arctic waters, and provide a baseline for the evaluation of the presence of VMEs in the Nordic Seas.
4.1. Limitations of the Modeling Approach
SDMs are subjected to an array of sources of uncertainty (Vierod et al., 2014). Some degree of uncertainty is introduced by the observations of the taxa modeled. Positional uncertainty (Moudrý and Š́ımová, 2012; Naimi et al., 2014) and imperfect detection (Monk, 2014) are issues when using historical records, and data from fisheries by-catch and bottom trawl surveys. Sampling bias (Beck et al., 2014; Fourcade et al., 2014) is an important factor in our study, given that in some areas the number of observations is high (i.e., the Norwegian shelf, and to a lesser degree the Icelandic and Faroese shelves), while much lower in other locations (the Greenlandic shelf and the deep basins). Methods like the target group background approach used in this study can reduce the effects of sampling bias in the model prediction but cannot eliminate it completely. Additional sources of uncertainty arise from the environmental predictors. Oceanographic parameters are derived from databases like the World Ocean Atlas (Locarnini et al., 2013) or from physical ocean models (e.g., Logemann et al., 2013), often at relatively coarse resolutions. Estimates from both sources have their own uncertainty, but modeling approaches like MaxEnt do not incorporate this uncertainty in the predictions. Finally, the selection of the modeling approach itself can introduce biases in the prediction (Piechaud et al., 2015). In our study we utilized a single modeling framework, although some authors have suggested the use of ensemble models, averaging predictions from different models (Georgian et al., 2019). Given all the potential sources of the uncertainty, we echo Piechaud et al. (2015) and urge caution when using the output of these models for management purposes. Modeled distributions should be used not as evidence of the presence of VMEs, but rather as a data-driven approach to identify areas where the presence of these habitats is likely (Hourigan, 2014).
In this study we have not produced uncertainty estimates for the predicted distribution of VMEs. Before predicted VME distributions can be used for management applications, it is necessary to quantify their uncertainty and to develop methods to incorporate the uncertainty in management decisions (Guisan et al., 2013). Although the internal uncertainty of MaxEnt models is difficult to quantify, bootstrap methods have been used to quantify some aspects of the uncertainty of MaxEnt predictions (Anderson et al., 2016b). For example, if planning tools like Zonation (Lehtomäki and Moilanen, 2013) or Marxan (Watts et al., 2017) would be used to prioritize areas for protection, it would be possible to prioritize locations with high conservation value (i.e., with high VME suitability and low uncertainty, Anderson et al., 2016b). Locations with confirmed VME presence, for example from underwater video surveys, would have no uncertainty and receive the highest priority for conservation. Uncertainty maps could also inform which areas should be targeted by future surveys, by highlighting locations where VMEs are predicted to occur and where the predictions are uncertain because of the lack of samples. An analysis of this type should follow this study.
We need to perform independent validation of the models (Elith et al., 2006; Davies and Guinotte, 2011) which can confirm model predictions (e.g., Rooper et al., 2018) or can highlight limitations of the predicted distributions. One form of validation is to compare model predictions with new observations. For example, recent observations in the Schultz Massif Seamount indicated the presence of Geodia parva, G. hentscheli, Stelleta sp., Caulophacus articus (Roberts et al., 2018), in agreement with our predicted suitability for these taxa. A comparison between predicted distributions and independent observations can provide useful information about the performance of the models. In an illustrative example, Anderson et al. (2016a) validated models for four reef-forming corals in the South Pacific Ocean using data from photographic surveys collected independently from the data used to fit the model. They found that the observed frequency of corals was much lower than predicted and that the correlation between observed and predicted coral distribution was not particularly high. The poor performance of the models was attributed to the low precision of the global bathymetry data, and to the lack of data on geomorphology and substrate data at the scale appropriate to the taxa modeled (Anderson et al., 2016a). These factors may be also relevant for the models in our study. An inspection of high-resolution bathymetry derived from multibeam data available for the Norwegian shelf and some regions on the Icelandic shelf indicates that the GEBCO global bathymetry models are much less detailed and do not resolve small geomorphic features that may be important for the distribution of VMEs (Davies et al., 2009; Henry et al., 2010; Rengstorf et al., 2012). The lack of information describing substrates is also likely to affect the results of our models, as sediment composition is highly variable and is known to influence the distribution of epibenthic sessile organisms (Davies and Guinotte, 2011; Tracey et al., 2011). The effect of the lack of substrate data in our models can be illustrated by the fact that the cold-water coral model predicts high suitability in regions of the Skagerrak known to be dominated by soft sediments and where cold-water corals are usually not observed. The effect of the lack of sediment data is accentuated by the low resolution of the bathymetry model, because terrain variables derived from high-resolution bathymetry can play a better role serving as proxy variables for sediment composition (Dunn and Halpin, 2009). Given these factors, there is a need to produce SDMs at finer scales, incorporating high resolution bathymetry and sediment distribution data, if available.
When estimating the present distribution of VMEs and VME indicator taxa, depending on the goal of a study, it can be argued that the effect of historical fishing should be included (Ross et al., 2012). Some areas may have high predicted VME suitability, but if these areas are continuously being trawled, they may not have high concentrations of VME indicator species because of the cumulative effect of fishing-induced mortality. Penney and Guinotte (2013) suggested computing a “discounted suitability”, where the suitability of each cell is reduced proportionally to the swept-area ratio. This method assumes that VME indicator species do not survive the impact of a single trawling event, and therefore in cells that are fished more than once per year the suitability is reduced to zero. This assumption can be adjusted to incorporate differences in the vulnerability of each VME to bottom trawling. An analysis of historical fishing patterns may highlight relatively pristine areas with high suitability for VME indicator taxa. These areas should be targeted for exploration and conservation.
4.2. Environmental Factors Influencing the Distribution of VME Indicator Species
Temperature is an important factor determining the distribution of cold-water corals (Davies and Guinotte, 2011; Yesson et al., 2012; Buhl-Mortensen et al., 2015c) and sponges (Klitgaard and Tendal, 2004; Howell et al., 2016). Given the strong bottom temperature gradients in the study area, it is no surprise that this parameter explained a large proportion of the predicted distribution patterns of the VME indicator taxa. In our study, minimum temperature was the most important factor with a permutation importance higher than 10% in 42 of the 44 taxa modeled. It was particularly high for species associated with cold waters including the sponges Geodia parva, Caulophacus (C.) arcticus, Cladorrhiza sp., Chrondocladia (C.) grandis, and the Neptheidae coral Gersemia sp. Temperature was also an important predictor for the scleractinean corals Lophelia pertusa, Madrepora oculata, and for taxa with predominantly southern distributions like Flabellum sp., Anthomastus sp., and Anthoptylum sp.
Next to temperature, depth was the next most predominant factor predicting the distribution of the VME indicator taxa in this study. Depth had a permutation importance higher than 10% in 33 of the 44 models. Similar to temperature, our study area included a wide depth range (Buhl-Mortensen et al., 2015c). Depth is not considered a direct explanatory variable, as hydrostatic pressure does not limit the distribution of VME indicator species. Instead it acts as a surrogate for other environmental parameters that are usually correlated with depth (Thresher et al., 2014; Howell et al., 2016). In our study there was some correlation between bottom depth and aragonite saturation state, particulate organic carbon and silica concentration. In addition, the environmental data sets used in this study had relatively low spatial resolution. In these conditions depth can act as a proxy explaining some proportion of the spatial patterns controlled by more direct explanatory variables.
Although the permutation importance of individual variables describing the morphology (i.e., terrain variables) of the seafloor was relatively low, their combined contribution was considerable in most of the models. This is consistent with previous modeling efforts for cold-water corals and sponges in which terrain variables have been important predictors (e.g., Rengstorf et al., 2013; Gullage et al., 2017; Rowden et al., 2017). It is well-known that the topography of the seafloor has a strong influence on the distribution patterns of filter feeders like cold-water corals and sponges, which are often associated with complex and elevated topographic features where locally accelerated currents increase the provision of food particles (Thiem et al., 2006; Duineveld et al., 2007; Navas et al., 2014) and influence the transport of larvae (Piepenburg and Müller, 2004; Dullo et al., 2008). The permutation importance of near-bottom current speed as a predictor variable was higher than 5% in 17 of the 44 models. These include four of the six models of sponges of the genus Geodia, and in the models of Stryphnus sp. and Steletta sp, taxa considered indicators of soft bottom sponge aggregations. Current speed had also an importance >5% in five of the seven models of sea pens (Pennatulacea). On the other hand, the importance of current speed was below 5% for most other taxa including reef-forming scleractinean corals, gorgonians, and sponges associated to hard bottoms. This is likely the result of the relatively low resolution of the near-bottom current speed data, which helped to predict the distribution of species with relatively broad distributions associated to soft sediments, but could not resolve more localized effects to predict the distribution of species associated with more complex terrain features. In the later cases, and similar to previous models for VME indicator taxa, the terrain descriptor variables act as proxies of near-bottom current speed. When the output of high-resolution oceanographic models is used as a predictor of the distribution of scleractinean corals like Lophelia pertussa or Madrepora oculata, near-bottom current speed do explain much of the observed patterns (Mohn et al., 2014; Bargain et al., 2018). In addition to acting as proxies of near-bottom current speed, terrain variables can also explain some of the spatial patterns associated to substrate type, which is another factor that strongly influence the distribution of benthic megafauna including corals and sponges (Gass and Roberts, 2006; Greathead et al., 2014; Baker et al., 2019). For example, hard bottom habitats are associated with complex topographies (Dunn and Halpin, 2009) where sediments are less likely to accumulate.
As expected, silicate was an important variable explaining the distribution of several sponge taxa. The permutation importance of silicate was higher than 10% in 12 of the 20 models for sponges. It was particularly high in the models of sponges associated to hard bottoms, including Axinellida sp., Phakellia sp., Antho (A.) dichotoma, and Mycale (M.) lingua. Silicate is required by sponges that form siliceous spicules, and its concentration may be a factor limiting the spatial distribution of sponge habitats (Leys et al., 2004; Howell et al., 2016). Silicate was also an important predictor for the distribution of some corals and sea pens, i.e., Isidella lophotensis, Anthomastus sp., Umbellula sp., and Pennatula sp. It is likely that for these taxa the concentration of silicate is not a direct limiting factor but rather is acting as an indicator of water masses. In the case of corals and sea pens, aragonite saturation had a lower importance than expected with only seven of 24 models having a permutation importance above 10%.
Geodia parva and G. hentscheli are the only two species for which salinity was an important predictor (permutation importances of 30.6 and 52.8% respectively). Both species are considered indicators of the cold “ostur” assemblage, with their distribution restricted to the Greenland shelf and Denmark strait. Here it is likely that salinity is a proxy for water masses (Yesson et al., 2012) rather than being a direct physiological constraint, acting as an indicator of the waters on the Greenland shelf characterized by the low temperatures and low salinity due to the input of glacial meltwater. This is supported by the fact that for G. hentscheli the permutation importance of temperature is lower, and the permutation importance of salinity is higher than for G. parva, but the combined importance of both parameters is similar for both species (70.1 and 60.9%, respectively).
4.3. Spatial Distribution of Predicted Taxa and Taxa Groups
As expected, there was a good agreement between the observed locations of VME indicator species and the areas of predicted high suitability, in particular for taxa distributed along a narrow range of environmental variables like Lophelia pertusa and the other taxa in cluster group 1 (Figure S1), Umbellula sp., and for taxa with rather limited geographic distributions like Isidella sp. and Caryophila. For other taxa the prediction appears less precise, the models tended to predict broad areas of high suitability. Some examples are the models for Geodia phlegraei, Anthothela gradiflora, Anthomastus sp., Flabellum sp., and Lycopodina sp. This could be because the taxa modeled are generalist species with a broad distribution, or could be an artifact due to low sample sizes or the lack of environmental predictors that limit their distribution.
Our predicted distributions for Lophelia pertusa, Paragorgia arborea, and Primnoa resedaeformis are very similar to those obtained by Sundahl (2017) in Norwegian waters using mostly data from the MAREANO programme (Buhl-Mortensen et al., 2015a,b). This is reassuring, given that Sundahl (2017) produced models at a higher spatial resolution (176 m) and included environmental predictors that were not available for our models, including sediment type and the output of a high-resolution ocean model. In addition, our areas of predicted high suitability for Lophelia pertusa in the territorial waters of the United Kingdom and Ireland are similar to the areas identified by Ross and Howell (2013) as having medium and high probability of reef presence.
In general, the predicted distributions for sponges of the genus Geodia were comparable to those predicted by Howell et al. (2016) in our study area. In particular, the predicted presence of G. mandrewi were very similar to the areas of high suitability predicted by our model. Our predictions for G. parva and G. hentschelli were also similar, with the caveat that Howell et al. (2016) did not predicted distributions at depths <200 m, with includes most of the eastern Greenland shelf north of 66°N where our models predict high suitability. On the other hand, our predictions for G. phlegraei, G. atlantica, and G. baretti were more restricted than those in Howell et al. (2016), whose predicted areas with presence included the deep basins of the Norwegian Sea and off southern Iceland where our models predicted low suitability.
The cluster analysis of the “I” similarity statistic computed among the predicted distributions of VME indicator taxa produced 7 groups and 6 subgroups. These groups are not biotopes or communities in the strict sense, as they are not the result of direct observations of species living in close proximity, but rather are groups of taxa with similar predicted distributions. Predictions from the broad-scale models in our study are not expected to agree with the species composition and distribution of biotopes identified from high-resolution local predictions, but reveal similarities at large scales relevant for the management of large marine ecosystems. Nevertheless, in several instances the groups identified were analogous to known VMEs in the study area, and in some cases to biotopes identified on the Norwegian shelf by the MAREANO programme (Table 5).
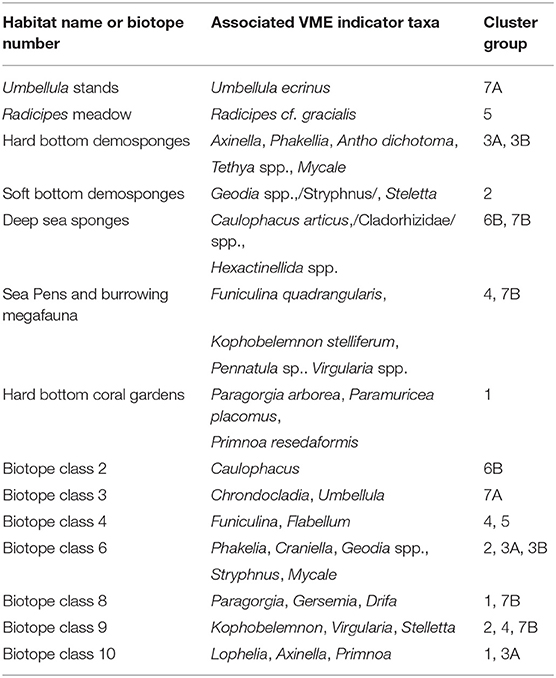
Table 5. Biotopes identified in the Norwegian shelf by Elvenes et al. (2014) and Gonzalez-Mirelis and Buhl-Mortensen (2015) with associated VME indicator taxa, and the cluster dendrogram groups in which those taxa were assigned in our study.
Group 1 included the gorgonians Paramuricea sp., Paragorgia arborea, and Primnoa resadaeformis, which are indicators of hard-bottom coral gardens (ICES, 2016) and were identified as an homogeneous community in the Norwegian shelf (Gonzalez-Mirelis and Buhl-Mortensen, 2015). Group 1 also included the scleractinean corals Lophelia pertusa and Madrepora oculata, which are the most important reef-forming corals in the study area. These two species are indicator taxa of cold-water coral reefs, but also of two types of hard-bottom coral gardens: colonial scleractineans on rocky outcrops, and non-reefal scleractinean aggregations (ICES, 2016). In our study we could not distinguish between L. pertusa and M. oculata records originating from different VMEs, and therefore we consider them indicators of a single VME termed reef-forming scleractineans. In addition, in the study area the taxa in this group are often found in close proximity (Buhl-Mortensen et al., 2015c), and therefore their predicted broad-scale distribution was similar. At local scales, it is likely that there are differences in the distribution patterns among the taxa in the groups identified in this study. For example, Elvenes et al. (2014) identified ten biotopes on the Norwegian shelf off the Lofoten and Vesterålen archipelagos (Elvenes et al., 2014), seven of which included VME indicator taxa. Lophelia and Primnoa were assigned to the same biotope (biotope class 10, Table 5), while Paragorgia was included in biotope class 8. These differences are expected when comparing predicted distributions at relatively large scales with biotopes identified at more local scales.
Five of the groups identified in our analysis were dominated by sponges and corresponded well to sponge VMEs within the study area. Our groups 2 and 6A include indicator species of soft-bottom sponge aggregations (ICES, 2016) and are analogous to the boreal and cold “ostur” assemblages described by Klitgaard and Tendal (2004), respectively. The stacked distribution models for groups 2 and 6a suggest that “ostur” habitats are widely distributed on the continental shelves and slopes of eastern Greenland, Denmark Strait, Iceland, the Faroe Islands, the Norwegian shelf, and the Bartents Sea. This agrees with previous studies by Klitgaard and Tendal (2004); Christiansen (2010); Howell et al. (2016). Geodia aggregations have been observed at depths between 150 and 1,700 m (Maldonado et al., 2015), which correspond well to the depth ranges of predicted high suitability for group 2 (boreal “ostur"). Areas of predicted high suitability for group 6a (cold “ostur”) includes areas in the Norwegian and Greenland seas at depths below 1,500 m. The sampling effort at these depths is very low and no Geodia aggregations have been previously reported. Group 3 included indicator taxa for hard-bottom sponge aggregations (ICES, 2016). The fact that these taxa form a distinct group from sponges associated to soft-bottoms supports the notion that deep-sea sponge aggregations form distinct habitats depending on their bottom type preferences as proposed by Gonzalez-Mirelis and Buhl-Mortensen (2015).
The list of indicator taxa for ICES/NAFO waters (ICES, 2016) also includes Caulophacus articus as an indicator for soft-sponge aggregations. In an analysis of community structure, Gonzalez-Mirelis and Buhl-Mortensen (2015) concluded that Hexactinellid and other sponges associated to deep, cold waters constitute a distinct habitat separated from soft-bottom and hard-bottom sponge aggregations. Our analysis placed C. articus, together with Cladorrhiza sp. and Lycopodina sp. into group 6B, which can be considered analogous to the deep sea sponges habitat defined by Gonzalez-Mirelis and Buhl-Mortensen (2015). This supports the need to define a distinct VME type for taxa in deep, cold waters, as suggested by Buhl-Mortensen et al. (2019). Caulophacus was associated to a distinct biotope (biotope class 2) by Elvenes et al. (2014), and our predicted distribution for Caulophacus sp. shows high suitability at depths below 1,500 m, which agrees with the predicted distribution of biotope 2 (Elvenes et al., 2014).
Group 4 included four sea pens considered indicators of the VME type “sea-pen fields” in the list of indicator taxa for ICES/NAFO waters (ICES, 2016): Funiculina sp., Pennatula sp., Kophobelemnon sp. and Haliperis sp., and it is analogous to the biotope “sea pen and burrowing megafauna communities” identified by Gonzalez-Mirelis and Buhl-Mortensen (2015) on the Norwegian shelf. The ICES/NAFO list also includes deep-sea sea pens Umbellula sp. and Anthoptilum sp., which our analysis did not included in group 4 given the differences in their predicted distributions. Similarly, Gonzalez-Mirelis and Buhl-Mortensen (2015) concluded that the sea pens Funiculina cuadrangularis, Kophobelemnon stelliferum, Pennatula sp., and Virgularia spp. formed an homogeneous community on the Norwegian shelf distinct from the Umbellula stands (e.g., areas with high densities of Umbellula sp). This supports the definition of two separate VME types which include shallow and deep-sea sea pen species (Buhl-Mortensen et al., 2019). Gonzalez-Mirelis and Buhl-Mortensen (2015) predicted the distribution of Umbellula sp. stands in two regions in the continental slope at depths below 500m roughly off the Lofoten and Vesterålen archipelagos, and in the Eggakanten north of 71°N. Our model for this taxa predicts very high suitability values in similar locations (Figure S8). In our analysis Umbellula spp. clustered together with the sponge Chrondocladia (C.) grandis, as both species have a distinct predicted distribution in the slopes north of the GISR. A biotope comprised by both species was also identified on the slopes of the Norwegian shelf (biotope class 3, Elvenes et al., 2014). Our models for both species and our stacked model for group 7a predicted high suitability along the continental slope between 800 and 1,500 m, which agrees well with the predicted distribution biotope class 3.
As opposed to most other groups, groups 5 is formed by taxa indicators of different VMEs characterized by a predicted distribution mostly in the deep basins south of the GISR. Among the taxa in this group are indicators of soft bottom coral gardens, including the gorgonians Radicipes sp., Acanella arbuscula, and the cup coral Flabellum, and indicators of hard bottom coral gardens including the gorgonians Anthomastus sp., Anthotella grandiflora. A. arbuscula has been reported to co-occur with Flabellum alabastrum (Buhl-Mortensen and Buhl-Mortensen, 2018). Our model for Radicipes sp. correctly predicted high suitability in the Bjørnøya slide area, which is the only area in Norwegian waters where Radicipes meadows have been observed (Buhl-Mortensen et al., 2015c; Gonzalez-Mirelis and Buhl-Mortensen, 2015, Figure S6). The group also includes Solenosmilia variabilis, a reef-forming scleractinean coral than in our study area was recorded south of the GISR, and the deep-sea sea pen Anthoptilum sp. This group includes taxa reported in the Mid-Atlantic ridge at a relatively wide depth range (800–2,400 m, Mortensen et al., 2008).
Group 7B includes mostly corals of the family Nephtheidae family that are indicator taxa of the VME subtype cauliflower coral fields (ICES, 2016; Buhl-Mortensen et al., 2019). These corals are found over a wide range of substrates including semiconsolidated mudstone (Buhl-Mortensen and Buhl-Mortensen, 2018), sometimes in relatively high densities as observed off the Westfjords in Iceland. On the Norwegian shelf, Elvenes et al. (2014) identified a biotope (biotope 8) that included Gersemia and Drifa, but also Paragorgia (Table 5).
Our VME Index provides a summary of the distribution of all 44 taxa, giving more weight to taxa that are considered to more closely fulfill the FAO criteria for VME identification (FAO, 2009). Areas with high VME Index include much of the shelf break and slope off Norway and the Barent Sea, Iceland, and the Faroe Islands, the shelf off southern Greenland, and the areas in the Reykjanes Ridge and the Kolbeinseyn Ridge. Several of these areas are being targeted by the MAREANO programme in Norway, and by the habitat mapping efforts by the Marine and Freshwater Research Institute (MFRI) in Iceland. Recent video observations carried out in the Faroe Islands are providing new information on the distribution of VME indicator species (Buhl-Mortensen et al., 2019). Nevertheless, there are vast areas where the number of observations is very low and should be the target of new research efforts. The continental shelf off southern Greenland is of particular interest because the predicted suitability of several VME indicator taxa is high in this region, and the area has not been subjected to intense fishing effort which increases the probability of finding pristine VMEs. Recent mapping efforts have been producing information off the west coast off Greenland (Yesson et al., 2016), but little is know off the eastern coast. Also there is a lack of data on the fauna on the deep basins of the Norwegian and Greenland sea.
Data Availability Statement
The datasets generated for this study are available on request to the corresponding author.
Author Contributions
LB-M, PB-M, JB, SÓ, SR, and PS conceived the study. LB-M secured funding. LB-M, PB-M, SÓ, PS, and SR compiled the database with records of VME indicator taxa. ØR provided data on temperature and salinity and performed spatial interpolations. JB compiled the remaining environmental predictors and carried out species distribution models and other analysis. The manuscript was written by JB, and was contributed to and edited by LB-M, PB-M, SÓ, SR, and ØR.
Funding
This article is a product of the Nordic Project On Vulnerable Marine Ecosystems And Anthropogenic Activities In Arctic And Sub-Arctic Waters (NovasArc, 2016-2018), a collaboration between the Institute of Marine Research (Norway), the Marine and Freshwater Research Institute (Iceland) and the Faroe Marine Research Institute (Faroe Islands). NovasArc was supported by the Marine Group (HAV) and the Working Group for Fisheries (AG-Fisk) of the Nordic Counsel of Ministers.
Conflict of Interest
The authors declare that the research was conducted in the absence of any commercial or financial relationships that could be construed as a potential conflict of interest.
Acknowledgments
L. Q. Jiang provided aragonite saturation data. Two reviewers and the journal editor provided useful comments that improved the quality of this article.
Supplementary Material
The Supplementary Material for this article can be found online at: https://www.frontiersin.org/articles/10.3389/fmars.2020.00131/full#supplementary-material
Footnotes
References
Allouche, O., Tsoar, A., and Kadmon, R. (2006). Assessing the accuracy of species distribution models: prevalence, kappa and the true skill statistic (tss). J. Appl. Ecol. 43, 1223–1232. doi: 10.1111/j.1365-2664.2006.01214.x
Anderson, O., Guinotte, J., Rowden, A., Clark, M., Mormede, S., Davies, A., et al. (2016a). Field validation of habitat suitability models for vulnerable marine ecosystems in the South Pacific Ocean: implications for the use of broad-scale models in fisheries management. Ocean Coast. Manage. 120, 110–126. doi: 10.1016/j.ocecoaman.2015.11.025
Anderson, O., Guinotte, J., Rowden, A., Tracey, D., Mackay, K., and Clark, M. (2016b). Habitat suitability models for predicting the occurrence of vulnerable marine ecosystems in the seas around New Zealand. Deep Sea Res. Part I Oceanogr. Res. Pap. 115, 265–292. doi: 10.1016/j.dsr.2016.07.006
Anonymous (2014). Meting av Fri?a?um og Stongdum ϕkjum (Evaluation of Protected and Closed Areas). Report to the Faroese Fisheries Ministry. Faroe Marine Research Institute, 41.
Aranda, S., and Lobo, J. (2011). How well does presence-only-based species distribution modelling predict assemblage diversity? A case study of the Tenerife flora. Ecography 34, 31–38. doi: 10.1111/j.1600-0587.2010.06134.x
Ardron, J. A., Clark, M. R., Penney, A. J., Hourigan, T. F., Rowden, A. A., Dunstan, P. K., et al. (2014). A systematic approach towards the identification and protection of vulnerable marine ecosystems. Mar. Policy 49, 146–154. doi: 10.1016/j.marpol.2013.11.017
Assis, J., Tyberghein, L., Bosch, S., Verbruggen, H., Serrão, E. A., and De Clerck, O. (2018). Bio-oracle v2. 0: extending marine data layers for bioclimatic modelling. Global Ecol. Biogeogr. 27, 277–284. doi: 10.1111/geb.12693
Baker, K. D., Snelgrove, P. V., Fifield, D. A., Edinger, E. N., Wareham-Hayes, V. E., Haedrich, R. L., et al. (2019). Small-scale patterns in the distribution and condition of bamboo coral, Keratoisis grayi, in submarine canyons on the grand banks, Newfoundland. Front. Mar. Sci. 6:374. doi: 10.3389/fmars.2019.00374
Baker, K. D., Wareham, V. E., Snelgrove, P. V., Haedrich, R. L., Fifield, D. A., Edinger, E. N., et al. (2012). Distributional patterns of deep-sea coral assemblages in three submarine canyons off Newfoundland, Canada. Mar. Ecol. Prog. Ser. 445, 235–249. doi: 10.3354/meps09448
Bargain, A., Foglini, F., Pairaud, I., Bonaldo, D., Carniel, S., Angele+tti, L., Taviani, M., Rochette, S., and Fabri, M. (2018). Predictive habitat modeling in two mediterranean canyons including hydrodynamic variables. Prog. Oceanogr. 169, 151–168. doi: 10.1016/j.pocean.2018.02.015
Beck, J., Böller, M., Erhardt, A., and Schwanghart, W. (2014). Spatial bias in the gbif database and its effect on modeling species' geographic distributions. Ecol. Inform. 19, 10–15. doi: 10.1016/j.ecoinf.2013.11.002
Behrenfeld, M. J., Boss, E., Siegel, D. A., and Shea, D. M. (2005). Carbon-based ocean productivity and phytoplankton physiology from space. Glob. Biogeochem. Cycles 19:1. doi: 10.1029/2004GB002299
Beisiegel, K., Darr, A., Gogina, M., and Zettler, M. L. (2017). Benefits and shortcomings of non-destructive benthic imagery for monitoring hard-bottom habitats. Mar. Pollut. Bull. 121, 5–15. doi: 10.1016/j.marpolbul.2017.04.009
Benn, A., Weaver, P., Billet, D., van den Hove, S., Murdock, A., Doneghan, G., and Le Bas, T. (2010). Human activities on the deep seafloor in the north east atlantic: an assessment of spatial extent. PLoS ONE 5:e12730. doi: 10.1371/journal.pone.0012730
Brix, S., and Svavarsson, J. (2010). Distribution and diversity of desmosomatid and nannoniscid isopods (crustacea) on the greenland–iceland–faeroe ridge. Polar Biol. 33, 515–530. doi: 10.1007/s00300-009-0729-8
Buhl-Mortensen, L., Aglen, A., Breen, M., Buhl-Mortensen, P., Ervik, A., Husa, V., et al. (2013). Impacts of Fisheries and Aquaculture on Sediments and Benthic Fauna: Suggestions for New Management Approaches. Fisken of havet 2/2013. Institute of Marine Research, 69.
Buhl-Mortensen, L., and Buhl-Mortensen, P. (2018). “Cold temperate coral habitats,” in Corals in a Changing World, eds C. Duque Beltran and E. Tello Camach (InTech), 9–27. doi: 10.5772/65203
Buhl-Mortensen, L., Buhl-Mortensen, P., Dolan, M., Dannheim, J., Bellec, V., and Holte, B. (2012). Habitat complexity and bottom fauna composition at different scales on the continental shelf and slope of Northern Norway. Hydrobiologia 685, 191–219. doi: 10.1007/s10750-011-0988-6.
Buhl-Mortensen, L., Buhl-Mortensen, P., Dolan, M., and Gonzalez-Mirelis, G. (2015a). Habitat mapping as a tool for conservation and sustainable use of marine resources: some perspectives from the mareano programme, Norway. J. Sea Res. 100, 6–61. doi: 10.1016/j.seares.2014.10.014
Buhl-Mortensen, L., Buhl-Mortensen, P., Dolan, M. F., and Holte, B. (2015b). The mareano programme–a full coverage mapping of the norwegian off-shore benthic environment and fauna. Mar. Biol. Res. 11, 4–17. doi: 10.1080/17451000.2014.952312
Buhl-Mortensen, L., Burgos, J., Steingrund, P., Buhl-Mortensen, P., Ólafsdóttir, S., and Ragnarsson, S. (2019). Vulnerable Marine Ecosystems (VME) Coral and Sponge VMEs in Arctic and Sub-Arctic Waters-Distribution and Threats. TemaNord 2019:519. Nordic Council of Ministers, 144.
Buhl-Mortensen, L., Ólafsdóttir, S., Buhl-Mortensen, P., Burgos, J., and Ragnarsson, S. (2015c). Distribution of nine cold-water coral species (scleractinia and gorgonacea) in the cold temperate North Atlantic: effects of bathymetry and hydrography. Hydrobiologia 759, 39–61. doi: 10.1007/s10750-014-2116-x
Buhl-Mortensen, L., Serigstad, B., Buhl-Mortensen, P., Olsen, M., Ostrowski, M., Błażewicz-Paszkowycz, M., et al. (2017). First observations of the structure and megafaunal community of a large Lophelia reef on the ghanaian shelf (the Gulf of Guinea). Deep Sea Res. II Top. Stud. Oceanogr. 137, 148–156. doi: 10.1016/j.dsr2.2016.06.007
Buhl-Mortensen, P., Buhl-Mortensen, L., and Purser, A. (2015d). “Trophic ecology and habitat provision in cold-water coral ecosystems,” in Marine Animal Forests: The Ecology of Benthic Biodiversity Hotspots, eds S. Rossi, L. Bramanti, A. Gori, and C. Orejas (Cham: Springer International Publishing), 919–944.
Calabrese, J. M., Certain, G., Kraan, C., and Dormann, C. F. (2014). Stacking species distribution models and adjusting bias by linking them to macroecological models. Glob. Ecol. Biogeogr. 23, 99–112. doi: 10.1111/geb.12102
Cárdenas, P., and Rapp, H. T. (2015). Demosponges from the northern mid-atlantic ridge shed more light on the diversity and biogeography of north atlantic deep-sea sponges. J. Mar. Biol. Assoc. U.K. 95, 1475–1516. doi: 10.1017/S0025315415000983
Christiansen, S. (2010). Background Document for Deep-Sea Sponge Aggregations. Biodiversity Series. OSPAR Comission, 47.
Clark, M., Althaus, F., Schlacher, T., Williams, A., Bowden, D., and Rowden, A. (2015). The impacts of deep-sea fisheries on benthic communities: a review. ICES J. Mar. Sci. J. Conseil 73(Suppl. 1), i51–i69. doi: 10.1093/icesjms/fsv123
Clark, M., Bowden, D., Baird, S., and Stewart, R. (2010). Effects of Fishing on the Benthic Biodiversity of Seamounts of the” Graveyard” Complex, Northern Chatham Rise. New Zealand Aquatic Environment and Biodiversity Report 46, 40.
Clark, M. R., and Dunn, M. R. (2012). Spatial management of deep-sea seamount fisheries: balancing sustainable exploitation and habitat conservation. Environ. Conserv. 39, 204–214. doi: 10.1017/S0376892912000021
Copley, J., Tyler, P., Sheader, M., Murton, B., and German, C. (1996). Megafauna from sublittoral to abyssal depths along the mid-atlantic ridge south of Iceland. Oceanol. Acta 19, 549–559.
Curd, A. (2010). Background Dcument for Seapen and Burrowing Megafauna Communities. Biodiversity Series. OSPAR Comission, 27.
D'Amen, M., Dubuis, A., Fernandes, R. F., Pottier, J., Pellissier, L., and Guisan, A. (2015). Using species richness and functional traits predictions to constrain assemblage predictions from stacked species distribution models. J. Biogeogr. 7, 1255–1266. doi: 10.1111/jbi.12485
D'Amen, M., Rahbek, C., Zimmermann, N. E., and Guisan, A. (2017). Spatial predictions at the community level: from current approaches to future frameworks. Biol. Rev. 92, 169–187. doi: 10.1111/brv.12222
Dauvin, J., Alizier, S., Weppe, A., and Gu?mundsson, G. (2012). Diversity and zoogeography of icelandic deep-sea ampeliscidae (crustacea: Amphipoda). Deep Sea Res. Part I Oceanogr. Res. Pap. 68, 12–23. doi: 10.1016/j.dsr.2012.04.013
Davies, A., Duineveld, G., Lavaleye, M., Bergman, M., Van Haren, H., and Roberts, J. (2009). Downwelling and deep-water bottom currents as food supply mechanisms to the cold-water coral Lophelia pertusa (scleractinia) at the mingulay reef complex. Limnol. Oceanogr. 54, 620–629. doi: 10.4319/lo.2009.54.2.0620
Davies, A. A. J. and Guinotte, J. M. J. (2011). Global habitat suitability for framework-forming cold-water corals. PLoS ONE 6:e18483. doi: 10.1371/journal.pone.0018483
Davies, J., Guillaumont, B., Tempera, F., Vertino, A., Beuck, L., Ólafsdóttir, S., et al. (2017). A new classification scheme of European cold-water coral habitats: implications for ecosystem-based management of the deep sea. Deep Sea Res. Part II Top. Stud. Oceanogr. 145, 102–109. doi: 10.1016/j.dsr2.2017.04.014
Davis, R. E. (1998). Preliminary results from directly measuring middepth circulation in the tropical and south pacific. J. Geophys. Res. Oceans 103, 24619–24639. doi: 10.1029/98JC01913
Davison, J. J., van Haren, H., Hosegood, P., Piechaud, N., and Howell, K. L. (2019). The distribution of deep-sea sponge aggregations (porifera) in relation to oceanographic processes in the faroe-shetland channel. Deep Sea Res. Part I Oceanogr. Res. Pap. 146, 55–61. doi: 10.1016/j.dsr.2019.03.005
Dormann, C. F., Elith, J., Bacher, S., Buchmann, C., Carl, G., Carré, G., et al. (2013). Collinearity: a review of methods to deal with it and a simulation study evaluating their performance. Ecography 36, 27–46. doi: 10.1111/j.1600-0587.2012.07348.x.
Duineveld, G. C., Lavaleye, M. S., Bergman, M. J., De Stigter, H., and Mienis, F. (2007). Trophic structure of a cold-water coral mound community (rockall bank, ne atlantic) in relation to the near-bottom particle supply and current regime. Bull. Mar. Sci. 81, 449–467.
Dullo, W.-C., Flögel, S., and Rüggeberg, A. (2008). Cold-water coral growth in relation to the hydrography of the celtic and nordic european continental margin. Mar. Ecol. Prog. Ser. 371, 165–176. doi: 10.3354/meps07623
Dunn, D., and Halpin, P. (2009). Rugosity-based regional modeling of hard-bottom habitat. Mar. Ecol. Prog. Ser. 377, 1–11. doi: 10.3354/meps07839
Durán Muñoz, P., Sayago-Gil, M., Murillo, F., Del Río, J., López-Abellán, L., Sacau, M., et al. (2012). Actions taken by fishing nations towards identification and protection of vulnerable marine ecosystems in the high seas: The spanish case (Atlantic Ocean). Mar. Policy 36, 536–543. doi: 10.1016/j.marpol.2011.09.005
Elith, J., and Graham, C. (2009). Do they? how do they? why do they differ? on finding reasons for differing performances of species distribution models. Ecography 32, 66–77. doi: 10.1111/j.1600-0587.2008.05505.x
Elith, J., Graham, C., Anderson, R., Dudik, M., Ferrier, S., Guisan, A., et al. (2006). Novel methods improve prediction of species' distributions from occurrence data. Ecography 29, 129–151. doi: 10.1111/j.2006.0906-7590.04596.x
Elith, J., Phillips, S., Hastie, T., Dudík, M., Chee, Y., and Yates, C. (2011). A statistical explanation of maxent for ecologists. Divers. Distrib. 17, 43–57. doi: 10.1111/j.1472-4642.2010.00725.x
Elvenes, S., Dolan, M. F., Buhl-Mortensen, P., and Bellec, V. K. (2014). An evaluation of compiled single-beam bathymetry data as a basis for regional sediment and biotope mapping. ICES J. Mar. Sci. J. Conseil 71, 867–881. doi: 10.1093/icesjms/fst154
Fabri, M., Pedel, L., Beuck, L., Galgani, F., Hebbeln, D., and Freiwald, A. (2014). Megafauna of vulnerable marine ecosystems in french mediterranean submarine canyons: spatial distribution and anthropogenic impacts. Deep Sea Res. Part II Top. Stud. Oceanogr. 104, 184–207. doi: 10.1016/j.dsr2.2013.06.016
FAO (2009). International Guidelines for the Management of Deep-Sea Fisheries in the High Seas. Rome: Food and Agriculture Organization of the United Nations, 73.
Ferrier, S., and Guisan, A. (2006). Spatial modelling of biodiversity at the community level. J. Applied Ecol. 43, 393–404. doi: 10.1111/j.1365-2664.2006.01149.x
Fisher, C. R., Hsing, P.-Y., Kaiser, C. L., Yoerger, D. R., Roberts, H. H., Shedd, W. W., et al. (2014). Footprint of deepwater horizon blowout impact to deep-water coral communities. Proc. Natl. Acad. Sci. U.S.A. 111, 11744–11749. doi: 10.1073/pnas.1403492111
Fosså, J., and Skjoldal, H. (2010). “Chapter 16: Conservation of cold-water coral reefs in norway,” in Handbook of Marine Fisheries Conservation and Management, eds R. Grafon, R. Hilborn, D. Squires, M. Tait, and M. Williams (Oxford: Oxford University Press), 215–230.
Fourcade, Y., Engler, J. O., Rödder, D., and Secondi, J. (2014). Mapping species distributions with maxent using a geographically biased sample of presence data: a performance assessment of methods for correcting sampling bias. PLoS ONE 9:e97122. doi: 10.1371/journal.pone.0097122
Fuller, S., Murillo, F., Wareham, V., and Kenchington, E. (2008). Vulnerable Marine Ecosystems Dominated by Deep-Water Corals and Sponges in the NAFO Convention Area. NAFO SCR Doc. 08/22 Serial No.N5524. Northwest Atlantic Fisheries Organization, 24.
Gass, S. E., and Roberts, J. M. (2006). The occurrence of the cold-water coral Lophelia pertusa (scleractinia) on oil and gas platforms in the North Sea: colony growth, recruitment and environmental controls on distribution. Mar. Pollut. Bull. 52, 549–559. doi: 10.1016/j.marpolbul.2005.10.002
Georgian, S. E., Anderson, O. F., and Rowden, A. A. (2019). Ensemble habitat suitability modeling of vulnerable marine ecosystem indicator taxa to inform deep-sea fisheries management in the South Pacific Ocean. Fish. Res. 211, 256–274. doi: 10.1016/j.fishres.2018.11.020
Gonzalez-Mirelis, G., and Buhl-Mortensen, P. (2015). Modelling benthic habitats and biotopes off the coast of norway to support spatial management. Ecol. Inform. 30, 284–292. doi: 10.1016/j.ecoinf.2015.06.005
Grassle, J. F. (2000). The ocean biogeographic information system (obis): an on-line, worldwide atlas for accessing, modeling and mapping marine biological data in a multidimensional geographic context. Oceanography 13, 5–7. doi: 10.5670/oceanog.2000.01
Greathead, C., González-Irusta, J., Clarke, J., Boulcott, P., Blackadder, L., Weetman, A., and Wright, P. (2014). Environmental requirements for three sea pen species: relevance to distribution and conservation. ICES J. Mar. Sci. 72, 576–586. doi: 10.1093/icesjms/fsu129
Guillera-Arroita, G., Lahoz-Monfort, J. J., Elith, J., Gordon, A., Kujala, H., Lentini, P. E., et al. (2015). Is my species distribution model fit for purpose? Matching data and models to applications. Glob. Ecol. Biogeogr. 24, 276–292. doi: 10.1111/geb.12268
Guinotte, J., Orr, J., Cairns, S., Freiwald, A., Morgan, L., and George, R. (2006). Will human-induced changes in seawater chemistry alter the distribution of deep-sea scleractinian corals? Front. Ecol. Environ. 4, 141–146. doi: 10.1890/1540-9295(2006)004[0141:WHCISC]2.0.CO;2
Guinotte, J. M., and Davies, A. J. (2014). Predicted deep-sea coral habitat suitability for the US West Coast. PLoS ONE 9:e93918. doi: 10.1371/journal.pone.0093918
Guisan, A., Tingley, R., Baumgartner, J. B., Naujokaitis-Lewis, I., Sutcliffe, P. R., Tulloch, A. I., et al. (2013). Predicting species distributions for conservation decisions. Ecol. Lett. 16, 1424–1435. doi: 10.1111/ele.12189
Gullage, L., Devillers, R., and Edinger, E. (2017). Predictive distribution modelling of cold-water corals in the Newfoundland and Labrador Region. Mar. Ecol. Prog. Ser. 582, 57–77. doi: 10.3354/meps12307
Henry, L.-A., Davies, A. J., and Roberts, J. M. (2010). Beta diversity of cold-water coral reef communities off Western Scotland. Coral Reefs 29, 427–436. doi: 10.1007/s00338-009-0577-6
Hestetun, J. T., Tompkins-Macdonald, G., and Rapp, H. T. (2017). A review of carnivorous sponges (porifera: Cladorhizidae) from the boreal North Atlantic and Arctic. Zool. J. Linnean Soc. 181, 1–69. doi: 10.1093/zoolinnean/zlw022
Hijmans, R. J., Phillips, S., Leathwick, J., and Elith, J. (2017). dismo: Species Distribution Modeling. R package version 1.1-4. Available online at: https://CRAN.R-project.org/package=dismo
Hourigan, T. (2014). “A Strategic Approach to Address Fisheries Impacts on Deep-Sea Coral Ecosystems,” in Interrelationships Between Corals and Fisheries, ed S. Bortone (CRS Press), 127–145.
Howell, K.-L., Piechaud, N., Downie, A.-L., and Kenny, A. (2016). The distribution of deep-sea sponge aggregations in the north atlantic and implications for their effective spatial management. Deep Sea Res. Part I Oceanogr. Res. Pap. 115, 309–320. doi: 10.1016/j.dsr.2016.07.005
Howell, K. L., Holt, R., Endrino, I. P., and Stewart, H. (2011). When the species is also a habitat: comparing the predictively modelled distributions of Lophelia pertusa and the reef habitat it forms. Biol. Conserv. 144, 2656–2665. doi: 10.1016/j.biocon.2011.07.025
ICES (2008). Report of the ICES-NAFO Joint Working Group on Deep-water Ecology (WGDEC). ICES CM 2008/ACOM:45. Technical Report. ICES 126.
ICES (2011). Report of the ICES/NAFO Joint Working Group on Deep-water Ecology (WGDEC). ICES CM 2011/ACOM:27, Copenhagen.
ICES (2016). Report of the Workshop on Vulnerable Marine Ecosystem Database (WKVME). ICES CM 2015/ACOM:62, Report. ICES 42. Peterborough.
Jiang, L.-Q., Feely, R. A., Carter, B. R., Greeley, D. J., Gledhill, D. K., and Arzayus, K. M. (2015). Climatological distribution of aragonite saturation state in the global oceans. Global Biogeochem. Cycles 29, 1656–1673. doi: 10.1002/2015GB005198
Jochumsen, K., Schnurr, S. M., and Quadfasel, D. (2016). Bottom temperature and salinity distribution and its variability around Iceland. Deep Sea Res. Part I Oceanogr. Res. Pap. 111, 79–90. doi: 10.1016/j.dsr.2016.02.009
Jørgensen, L. L., Ljubin, P., Skjoldal, H. R., Ingvaldsen, R. B., Anisimova, N., and Manushin, I. (2014). Distribution of benthic megafauna in the barents sea: baseline for an ecosystem approach to management. ICES J. Mar. Sci. 72, 595?–613. doi: 10.1093/icesjms/fsu106fsu106.
Jørgensen, L. L., Planque, B., Thangstad, T. H., and Certain, G. (2015). Vulnerability of megabenthic species to trawling in the Barents Sea. ICES J. Mar. Sci. 73, i84?–i97. doi: 10.1093/icesjms/fsv107
Kivlin, S. N., Muscarella, R., Hawkes, C. V., and Treseder, K. K. (2017). “The predictive power of ecological niche modeling for global arbuscular mycorrhizal fungal biogeography,” in Biogeography of Mycorrhizal Symbiosis eds E, Tedersoo (Springer), 143–158. doi: 10.1007/978-3-319-56363-3_7
Klitgaard, A., Tendal, O., and Westerberg, H. (1997). “Mass occurrences of large sponges (Porifera) in Faroe Island (NE Atlantic) Shelf and slope areas: characteristics, distribution and possible causes,” in The Responses of Marine Organisms to Their Enviroments eds L. Hawkins and S. Hutchinson (Southampton Ocenography Centre), 129–142.
Klitgaard, A. B., and Tendal, O. S. (2004). Distribution and species composition of mass occurrences of large-sized sponges in the Northeast Atlantic. Prog. Oceanogr. 61, 57–98. doi: 10.1016/j.pocean.2004.06.002
Kutti, T., Bannister, R., and Fosså, J. H. (2013). Community structure and ecological function of deep-water sponge grounds in the traenadypet MPA-northern Norwegian continental shelf. Contin. Shelf Res. 69, 21–30. doi: 10.1016/j.csr.2013.09.011
Lagasse, C. R., Knudby, A., Curtis, J., Finney, J. L., and Cox, S. P. (2015). Spatial analyses reveal conservation benefits for cold-water corals and sponges from small changes in a trawl fishery footprint. MEPS 528, 161–172. doi: 10.3354/meps11271
Lecours, V., Devillers, R., Simms, A. E., Lucieer, V. L., and Brown, C. J. (2017). Towards a framework for terrain attribute selection in environmental studies. Environ. Model. Softw. 89, 19–30. doi: 10.1016/j.envsoft.2016.11.027
Legendre, P., and Legendre, L. (1998). Numerical Ecology, 2nd Edition. Developments in Environmental Modelling 20. Amsterdam: Elsevier Science, 852.
Lehtomäki, J., and Moilanen, A. (2013). Methods and workflow for spatial conservation prioritization using zonation. Environ. Model. Softw. 47, 128–137. doi: 10.1016/j.envsoft.2013
Levin, L., and Le Bris, N. (2015). The deep ocean under climate change. Science 350, 766–768. doi: 10.1126/science.aad0126
Leys, S., Wilson, K., Holeton, C., Reiswig, H., Austin, W., and Tunnicliffe, V. (2004). Patterns of glass sponge (porifera, hexactinellida) distribution in coastal waters of British Columbia, Canada. Mar. Ecol. Prog. Ser. 283, 133–149. doi: 10.3354/meps283133
Liu, C., Newell, G., and White, M. (2016). On the selection of thresholds for predicting species occurrence with presence-only data. Ecol. Evol. 6, 337–348. doi: 10.1002/ece3.1878
Liu, C., P.M., B., Dawson, T., and Pearson, R. (2005). Selecting thresholds of occurrence in the prediction of species distributions. Ecography 28, 385–393. doi: 10.1111/j.0906-7590.2005.03957.x
Locarnini, R., Mishonov, A., Antonov, J., Boyer, T., Garcia, H., Zweng, M. M., et al. (2013). Temperature. NOAA Atlas NESDIS: 73, ed S. Levitus and A. Mishonov, 40.
Logemann, K., Ólafsson, J., Snorrason, Á., Valdimarsson, H., and Marteinsdóttir, G. (2013). The circulation of icelandic waters–a modelling study. Ocean Sci. Discuss. 10, 763–824. doi: 10.5194/os-9-931-2013
Lutz, M., Dunbar, R., and Caldeira, K. (2002). Regional variability in the vertical flux of particulate organic carbon in the ocean interior. Global Biogeochem. Cycles 16:3. doi: 10.1029/2000GB001383
Lutz, M. J., Caldeira, K., Dunbar, R. B., and Behrenfeld, M. J. (2007). Seasonal rhythms of net primary production and particulate organic carbon flux to depth describe the efficiency of biological pump in the global ocean. J. Geophys. Res. Oceans 112:C10. doi: 10.1029/2006JC003706
Maldonado, M., Aguilar, R., Bannister, R. J., Bell, J. J., Conway, K. W., Dayton, P. K., et al. (2015). “Sponge grounds as key marine habitats: a synthetic review of types, structure, functional roles, and conservation concerns,” in Marine Animal Forests: The Ecology of Benthic Biodiversity Hotspots (Cham: Springer International Publishing), 1–39.
Mayer, M., and Piepenburg, D. (1996). Epibenthic community patterns on the continental slope off east greenland at 75° N. Mar. Ecol. Prog. Ser. 143, 151–164. doi: 10.3354/meps143151
McKean, J., and Roering, J. (2004). Objective landslide detection and surface morphology mapping using high-resolution airborne laser altimetry. Geomorphology 57, 331–351. doi: 10.1016/S0169-555X(03)00164-8
Merow, C., Smith, M. J., and Silander, J. A. (2013). A practical guide to maxent for modeling species' distributions: what it does, and why inputs and settings matter. Ecography 36, 1058–1069. doi: 10.1111/j.1600-0587.2013.07872.x
Mohn, C., Rengstorf, A., White, M., Duineveld, G., Mienis, F., Soetaert, K., et al. (2014). Linking benthic hydrodynamics and cold-water coral occurrences: a high-resolution model study at three cold-water coral provinces in the ne atlantic. Prog. Oceanogr. 122, 92–104. doi: 10.1016/j.pocean.2013.12.003
Monk, J. (2014). How long should we ignore imperfect detection of species in the marine environment when modelling their distribution? Fish Fish. 15, 352–358. doi: 10.1111/faf.12039
Morato, T., Pham, C. K., Pinto, C., Golding, N., Ardron, J. A., Muñoz, P. D., et al. (2018). A multi criteria assessment method for identifying vulnerable marine ecosystems in the North-East Atlantic. Front. Mar. Sci. 5:460. doi: 10.3389/fmars.2018.00460
Mortensen, P., and Buhl-Mortensen, L. (2004). Distribution of deep-water gorgonian corals in relation to benthic habitat features in the northeast channel (Atlantic Canada). Mar. Biol. 144, 1223–1238. doi: 10.1007/s00227-003-1280-8
Mortensen, P., Buhl-Mortensen, L., Gebruk, A., and Krylova, E. (2008). Occurrence of deep-water corals on the mid-atlantic ridge based on mar-eco data. Deep Sea Res. Part II Top. Stud. Oceanogr. 55, 142–152. doi: 10.1016/j.dsr2.2007.09.018
Mortensen, P., Hovland, M., Brattegard, T., and Farestveit, R. (1995). Deep water bioherms of the scleractinian coral lophelia pertusa (l.) at 64° N on the norwegian shelf: structure and associated megafauna. Sarsia 80, 145–158. doi: 10.1080/00364827.1995.10413586
Mortensen, P., Hovland, M., Fosså, J., and Furevik, D. (2001). Distribution, abundance and size of Lophelia pertusa coral reefs in mid-norway in relation to seabed characteristics. J. Mar. Biol. Assoc. U.K. 81, 581–597. doi: 10.1017/S002531540100426X
Mortensen, P. B., and Buhl-Mortensen, L. (2005). “Deep-water corals and their habitats in the gully, a submarine canyon off Atlantic Canada,” in Cold-Water Corals and Ecosystems eds A. Freiwald and J. Murray Roberts (Springer), 247–277. doi: 10.1007/3-540-27673-4_12
Moudrý, V., and Šímová, P. (2012). Influence of positional accuracy, sample size and scale on modelling species distributions: a review. Int. J. Geogr. Inform. Sci. 26, 2083–2095. doi: 10.1080/13658816.2012.721553
Murillo, F., Wareham, V., Sacau, M., Román, E., and Durán-Muñoz, P. (2011). New data on deep-water corals and sponges from Spanish/EU and Canadian bottom trawl groundfish surveys in the NAFO Regulatory Area (Divs. 3LMNO): 2008-2010 period. Serial No. N6004 NAFO SCR Doc. 11/074. NAFO.
Murillo, F. J., Muñoz, P. D., Cristobo, J., Ríos, P., González, C., Kenchington, E., et al. (2012). Deep-sea sponge grounds of the flemish cap, flemish pass and the grand banks of newfoundland (Northwest Atlantic ocean): distribution and species composition. Mar. Biol. Res. 8, 842–854. doi: 10.1080/17451000.2012.682583
Muscarella, R., Galante, P. J., Soley-Guardia, M., Boria, R. A., Kass, J. M., Uriarte, M., et al. (2014). Enmeval: an R package for conducting spatially independent evaluations and estimating optimal model complexity for maxent ecological niche models. Methods Ecol. Evol. 5, 1198–1205. doi: 10.1111/2041-210X.12261
Naimi, B., Hamm, N. A., Groen, T. A., Skidmore, A. K., and Toxopeus, A. G. (2014). Where is positional uncertainty a problem for species distribution modelling? Ecography 37, 191–203. doi: 10.1111/j.1600-0587.2013.00205.x.
Navas, J. M., Miller, P. L., Henry, L.-A., Hennige, S. J., and Roberts, J. M. (2014). Ecohydrodynamics of cold-water coral reefs: a case study of the mingulay reef complex (Western Scotland). PLoS ONE 9:e98218. doi: 10.1371/journal.pone.0098218
NEAFC (2014). Recommendation 19:2014. Article 2(g) and Annex 5. North-East Atlantic Fisheries Comission, 24.
Nilsen, J. E. ?., H?t?n, H., Mork, K. A., and Valdimarsson, H. (2008). The NISE Dataset. Technical Report. The Faroese Fisheries Laboratory - Fiskiranns?knarstovan, 20.
Ólafsdóttir, S., and Burgos, J. (2012). “Fir?un kóralsvæ?a vi? Ísland og í nor?ur atlantshafi (cold water coral conservation in iceland and the North Atlantic),” in ?ættir úr vistfræ?i sjávar 2011 (Environmental Conditions in Icelandic Waters 2011), number 162 in Hafranns?knir nr. 162, 30–35.
Omarsdottir, S., Einarsdottir, E., Ögmundsdottir, H., Freysdottir, J., Olafsdottir, E., Molinski, T., et al. (2013). Biodiversity of benthic invertebrates and bioprospecting in icelandic waters. Phytochem. Rev. 12, 517–529. doi: 10.1007/s11101-012-9243-7
OSPAR (2010a). Descriptions of Habitats on the OSPAR List of Threatened and/or Declining Species and Habitats. OSPAR Agreeement 2008-07. OSPAR Comission.
OSPAR (2010b). OSPAR List of Threatened and/or Declining Species and Habitas. OSPAR Agreement 2008-6. OSPAR Comission.
Penney, A. J., and Guinotte, J. M. (2013). Evaluation of New Zealand's high-seas bottom trawl closures using predictive habitat models and quantitative risk assessment. PLoS ONE 8:e82273. doi: 10.1371/journal.pone.0082273
Phillips, S., Anderson, R., and Schapire, R. (2006). Maximum entropy modeling of species geographic distributions. Ecol. Model. 190, 231–259. doi: 10.1016/j.ecolmodel.2005.03.026
Phillips, S., Dudík, M., Elith, J., Graham, C., Lehmann, A., Leathwick, J., et al. (2009). Sample selection bias and presence-only distribution models: implications for background and pseudo-absence data. Ecol. Appl. 19, 181–197. doi: 10.1890/07-2153.1
Phillips, S. J., Anderson, R. P., Dudík, M., Schapire, R. E., and Blair, M. E. (2017). Opening the black box: an open-source release of maxent. Ecography 40, 1–7. doi: 10.1111/ecog.03049
Piechaud, N., Downie, A., Stewart, H. A., and Howell, K. L. (2015). The impact of modelling method selection on predicted extent and distribution of deep-sea benthic assemblages. Earth Environ. Sci. Trans. R. Soc. Edinburgh 105, 251–261. doi: 10.1017/S1755691015000122
Piepenburg, D., and Müller, B. (2004). Distribution of epibenthic communities on the great meteor seamount (North-East Atlantic) mirrors pelagic processes. Arch. Fish. Mar. Res. 51, 55–70.
R Core Team (2019). R: A Language and Environment for Statistical Computing. Vienna: R Foundation for Statistical Computing.
Radosavljevic, A., and Anderson, R. P. (2014). Making better maxent models of species distributions: complexity, overfitting and evaluation. J. Biogeogr. 41, 629–643. doi: 10.1111/jbi.12227
Ramirez-Llodra, E., Tyler, P., Baker, M., Bergstad, O., Clark, M., Escobar, E., et al. (2011). Man and the last great wilderness: human impact on the deep sea. PLoS ONE 6:e22588. doi: 10.1371/journal.pone.0022588
Rengstorf, A. M., Grehan, A., Yesson, C., and Brown, C. (2012). Towards high-resolution habitat suitability modeling of vulnerable marine ecosystems in the deep-sea: resolving terrain attribute dependencies. Mar. Geodesy 35, 343–361. doi: 10.1080/01490419.2012.699020
Rengstorf, A. M., Yesson, C., Brown, C., and Grehan, A. J. (2013). High-resolution habitat suitability modelling can improve conservation of vulnerable marine ecosystems in the deep sea. J. Biogeogr. 40, 1702–1714. doi: 10.1111/jbi.12123
Roberts, E., Mienis, F., Rapp, H., Hanz, U., Meyer, H., and Davies, A. (2018). Oceanographic setting and short-timescale environmental variability at an arctic seamount sponge ground. Deep Sea Res. Part I Oceanogr. Res. Pap. 138, 98–113. doi: 10.1016/j.dsr.2018.06.007
Roberts, J., Wheeler, A., Freiwald, A., and Cairns, S. (2009) Cold-Water Corals. The Biology Geology of Deep-Sea Coral Habitats. Cambridge: Cambridge University Press, 334.
Rooper, C. N., Wilborn, R., Goddard, P., Williams, K., Towler, R., and Hoff, G. R. (2018). Validation of deep-sea coral and sponge distribution models in the Aleutian Islands, Alaska. ICES J. Mar. Sci. 75, 199–209. doi: 10.1093/icesjms/fsx087
Ross, R. E., and Howell, K. L. (2013). Use of predictive habitat modelling to assess the distribution and extent of the current protection of ‘listed' deep-sea habitats. Divers. Distrib. 19, 433–445. doi: 10.1111/ddi.12010
Ross, S. W., Carlson, M. C., and Quattrini, A. M. (2012). The utility of museum records for documenting distributions of deep-sea corals off the Southeastern United States. Mar. Biol. Res. 8, 101–114.
Rowden, A., Stephenson, F., Clark, M., Anderson, O., Guinotte, J., Baird, S., et al. (2019). Examining the utility of a decision-support tool to develop spatial management options for the protection of vulnerable marine ecosystems on the high seas around New Zealand. Ocean Coast. Manage. 170, 1–16. doi: 10.1016/j.ocecoaman.2018.12.033
Rowden, A. A., Anderson, O. F., Georgian, S. E., Bowden, D. A., Clark, M. R., Pallentin, A., and Miller, A. (2017). High-resolution habitat suitability models for the conservation and management of vulnerable marine ecosystems on the louisville seamount chain, South Pacific Ocean. Front. Mar. Sci. 4:335. doi: 10.3389/fmars.2017.00335
Schlacher, T. A., Baco, A. R., Rowden, A. A., O'Hara, T. D., Clark, M. R., Kelley, C., et al. (2014). Seamount benthos in a cobalt-rich crust region of the central pacific: conservation challenges for future seabed mining. Divers. Distrib. 20, 491–502. doi: 10.1111/ddi.12142
Skagseth, Ø., and Mork, K. A. (2012). Heat content in the norwegian sea, 1995–2010. ICES J. Mar. Sci. 69, 826–832. doi: 10.1093/icesjms/fss026
Sundahl, H. (2017). Driving forces in the distributions of the three most common deep-water coral species in Norway: Lophelia pertusa, Paragorgia arborea, and Primnoa resedaeformis (Master?s Thesis). University of Bergen, Bergen, Norway.
Tendal, O. S., and Barthel, D. (1993). Chondrocladia gigantea (demospongiae)-the giant clubsponge of the northeast atlantic. Deep Sea Newslett. 20, 12–15.
Thiem, Ø., Ravagnan, E., Fosså, J., and Berntsen, J. (2006). Food supply mechanisms for cold-water corals along a continental shelf edge. J. Mar. Syst. 60, 207–219. doi: 10.1016/j.jmarsys.2005.12.004
Thresher, R., Althaus, F., Adkins, J., Gowlett-Holmes, K., Alderslade, P., Dowdney, J., et al. (2014). Strong depth-related zonation of megabenthos on a rocky continental margin (700–4000 m) off Southern Tasmania, Australia. PLoS ONE 9:e85872. doi: 10.1371/journal.pone.0085872
Tracey, D., Rowden, A., Mackay, K., and Compton, T. (2011). Habitat-forming cold-water corals show affinity for seamounts in the New Zealand region. Mar. Ecol. Prog. Ser. 430, 1–22. doi: 10.3354/meps09164
Vierod, A. D., Guinotte, J. M., and Davies, A. J. (2014). Predicting the distribution of vulnerable marine ecosystems in the deep sea using presence-background models. Deep Sea Res. Part II Top. Stud. Oceanogr. 99, 6–18. doi: 10.1016/j.dsr2.2013.06.010
Voet, G., Quadfasel, D., Mork, K. A., and Søiland, H. (2010). The mid-depth circulation of the nordic seas derived from profiling float observations. Tellus A Dyn. Meteorol. Oceanogr. 62, 516–529. doi: 10.1111/j.1600-0870.2010.00444.x
Warren, D. L., Glor, R. E., and Turelli, M. (2008). Environmental niche equivalency versus conservatism: quantitative approaches to niche evolution. Evolution 62, 2868–2883. doi: 10.1111/j.1558-5646.2008.00482.x
Warren, D. L., and Seifert, S. N. (2011). Ecological niche modeling in maxent: the importance of model complexity and the performance of model selection criteria. Ecol. Appl. 21, 335–342. doi: 10.1890/10-1171.1
Warren, D. L., Wright, A. N., Seifert, S. N., and Shaffer, H. B. (2014). Incorporating model complexity and spatial sampling bias into ecological niche models of climate change risks faced by 90 California vertebrate species of concern. Divers. Distrib. 20, 334–343. doi: 10.1111/ddi.12160
Watts, M. E., Stewart, R. R., Martin, T. G., Klein, C. J., Carwardine, J., and Possingham, H. P. (2017). “Systematic conservation planning with marxan,” in Learning Landscape Ecology eds S. Gergel and M. Turner (New York, NY: Springer), 211–227.
Weatherall, P., Marks, K. M., Jakobsson, M., Schmitt, T., Tani, S., Arndt, J. E., et al. (2015). A new digital bathymetric model of the world's oceans. Earth Space Sci. 2, 331–345. doi: 10.1002/2015EA000107
Weaver, P., Benn, A., Arana, P., Ardron, J., Bailey, D., Baker, K., et al. (2011). The impact of deep-sea fisheries and implementation of the UNGA Resolutions 61/105 and 64/72. Report of an international scientific Workshop, National Oceanography Centre, Southampton.
Westberry, T., Behrenfeld, M., Siegel, D., and Boss, E. (2008). Carbon-based primary productivity modeling with vertically resolved photoacclimation. Global Biogeochem. Cycles 22. doi: 10.1029/2007GB003078
Wheeler, A., Bett, B. J., Billett, D. S. M., Masson, D. G., and Mayor, D. (2005). “The impact of demersal trawling on Northeast Atlantic deepwater coral habitats: The case of the Darwin Mounds, United Kingdom,” in Benthic Habitats and the Effects of Fishing, eds P. Barnes and J. Thomas (Bethesda, MD: American Fisheries Society), 890.
Williams, A., Schlacher, T. A., Rowden, A. A., Althaus, F., Clark, M. R., Bowden, D. A., et al. (2010). Seamount megabenthic assemblages fail to recover from trawling impacts. Mar. Ecol. 31, 183–199. doi: 10.1111/j.1439-0485.2010.00385.x
Wilson, M. F. J., O'Connell, B., Brown, C., Guinan, J. C., and Grehan, A. J. (2007). Multiscale terrain analysis of multibeam bathymetry data for habitat mapping on the continental slope. Mar. Geodesy 30, 3–35. doi: 10.1080/01490410701295962
Wiltshire, K. H., Tanner, J. E., Althaus, F., Sorokin, S. J., and Williams, A. (2018). Predicting environmental suitability for key benthic species in an ecologically and economically important deep-sea environment. Deep Sea Res. Part II Top. Stud. Oceanogr. 157, 121–133. doi: 10.1016/j.dsr2.2018.06.011
Wunderlich, R. F., Lin, Y.-P., Anthony, J., and Petway, J. R. (2019). Two alternative evaluation metrics to replace the true skill statistic in the assessment of species distribution models. Nat. Conserv. 35:97. doi: 10.3897/natureconservation.35.33918
Yesson, C., Fisher, J., Gorham, T., Turner, C. J., Hammeken Arboe, N., Blicher, M. E., et al. (2016). The impact of trawling on the epibenthic megafauna of the west greenland shelf. ICES J. Mar. Sci. 74, 866–876. doi: 10.1093/icesjms/fsw206.
Keywords: vulnerable marine ecosystems, indicator taxa, cold-water corals, sponge aggregations, species distribution models, Nordic Seas
Citation: Burgos JM, Buhl-Mortensen L, Buhl-Mortensen P, Ólafsdóttir SH, Steingrund P, Ragnarsson SÁ and Skagseth Ø (2020) Predicting the Distribution of Indicator Taxa of Vulnerable Marine Ecosystems in the Arctic and Sub-arctic Waters of the Nordic Seas. Front. Mar. Sci. 7:131. doi: 10.3389/fmars.2020.00131
Received: 08 September 2019; Accepted: 18 February 2020;
Published: 10 March 2020.
Edited by:
Anthony Grehan, National University of Ireland Galway, IrelandReviewed by:
Michael Vecchione, National Oceanic and Atmospheric Administration (NOAA), United StatesIan David Tuck, National Institute of Water and Atmospheric Research (NIWA), New Zealand
Copyright © 2020 Burgos, Buhl-Mortensen, Buhl-Mortensen, Ólafsdóttir, Steingrund, Ragnarsson and Skagseth. This is an open-access article distributed under the terms of the Creative Commons Attribution License (CC BY). The use, distribution or reproduction in other forums is permitted, provided the original author(s) and the copyright owner(s) are credited and that the original publication in this journal is cited, in accordance with accepted academic practice. No use, distribution or reproduction is permitted which does not comply with these terms.
*Correspondence: Julian M. Burgos, anVsaWFuLmJ1cmdvc0BoYWZvZ3ZhdG4uaXM=