- 1Institute of Marine Science, The University of Auckland, Auckland, New Zealand
- 2School of Science, University of Waikato, Hamilton, New Zealand
- 3National Institute of Water and Atmospheric Research, Hamilton, New Zealand
- 4Cawthron Institute, Nelson, New Zealand
- 5Department of Marine Science, University of Otago, Dunedin, New Zealand
- 6Department of Biological Sciences, Faculty of Science, Marine Research (Ma-Re) Institute, University of Cape Town, Cape Town, South Africa
With globally accelerating rates of environmental disturbance, coastal marine ecosystems are increasingly prone to non-linear regime shifts that result in a loss of ecosystem function and services. A lack of early-detection methods, and an over reliance on limits-based approaches means that these tipping points manifest as surprises. Consequently, marine ecosystems are notoriously difficult to manage, and scientists, managers, and policy makers are paralyzed in a spiral of ecosystem degradation. This paralysis is caused by the inherent need to quantify the risk and uncertainty that surrounds every decision. While progress toward forecasting tipping points is ongoing and important, an interim approach is desperately needed to enable scientists to make recommendations that are credible and defensible in the face of deep uncertainty. We discuss how current tools for developing risk assessments and scenario planning, coupled with expert opinions, can be adapted to bridge gaps in quantitative data, enabling scientists and managers to prepare for many plausible futures. We argue that these tools are currently underutilized in a marine cumulative effects context but offer a way to inform decisions in the interim while predictive models and early warning signals remain imperfect. This approach will require redefining the way we think about managing for ecological surprise to include actions that not only limit drivers of tipping points but increase socio-ecological resilience to yield satisfactory outcomes under multiple possible futures that are inherently uncertain.
Introduction
Global change is having unprecedented impacts on marine ecosystems and human well-being (Vitousek et al., 1997; Costanza et al., 2014; Rocha et al., 2015). The cumulative effects of multiple drivers of change can cause non-linear shifts in ecosystem functions and services, known as tipping points or regime shifts (see Appendix for glossary). Tipping points can have catastrophic consequences for human well-being when ecosystem services associated with people’s livelihoods are lost (e.g., Newfoundland cod collapse, or degradation of water quality in Chesapeake Bay; Steele et al., 1992; Boesch et al., 2001; Rocha et al., 2015; Selkoe et al., 2015; Hicks et al., 2016). Coastal ecosystems are among the most heavily impacted and are particularly prone to tipping points due to the complex interaction of intrinsic and extrinsic drivers and processes. These interactions result in hysteresis, where feedbacks between intrinsic ecosystem components limit recovery even when extrinsic drivers are removed (e.g., eutrophication-induced bottom water anoxia results in nutrient release from the sediments that continues to fuel algal blooms after external nutrient loading is reduced) (Boesch et al., 2001; Nyström et al., 2012). Intensifying human pressures increase the number and strength of extrinsic drivers on marine ecosystems (IPBES, 2018) and are likely to raise the frequency of tipping points (Drijfhout et al., 2015; Rocha et al., 2015). These tipping points manifest as surprises because seemingly small drivers of change can cumulatively cause large effects. Further, a lack of proven ecological detection methods (de Young et al., 2008; Thrush et al., 2009; Dakos et al., 2015; Scheffer et al., 2015; Burthe et al., 2016) and an over-reliance on limits-based management (Charles, 1997; Thrush et al., 2016) has impeded the ability to make decisions that prevent tipping points.
Broad-scale limits-based management (e.g., limits on nutrient loading) is not fit-for-purpose, because stressor thresholds are context-sensitive (e.g., response to eutrophication depends on multiple site-specific and temporally varying biophysical factors; Anderson et al., 2015). Furthermore, there can be an implicit assumption in how the monitored variable (an ecosystem indicator) will track linearly or otherwise toward a threshold providing an opportunity for intervention. However, this assumption often does not consider that change can happen fast and without warning and a crossed threshold may be difficult to re-cross (Thrush and Dayton, 2010; but see Foley et al., 2015). Our understanding of marine ecosystem interactions from empirical data (experimental and observational) is often incomplete, context-, and scale-dependent (Thrush et al., 2009; Snelgrove et al., 2014). To date, analysis of marine time series data has been unsuccessful in identifying generalizable early warning signals to forecast tipping points, and theory of early warning from “critical slowing down” and “flickering” have rarely been demonstrated empirically (Dakos et al., 2015; Burthe et al., 2016; Hewitt and Thrush, 2019). While continued development of methods and data collection for forecasting tipping points is important, it is critical that scientists and decision-makers are not paralyzed by the deep uncertainty that surrounds this problem.
We must bridge the gap between limited empirical data at the correct spatial and temporal resolution, models and effective decision-making to maintain the biodiversity, ecosystem functions, and provision of services on which humanity relies (Knight et al., 2008; Carpenter et al., 2009; Figure 1). This requires consideration of the socio-ecological implications of a tipping point and the feedbacks that can lock a system into an alternative state (Scheffer et al., 2001; Folke et al., 2004; Nyström et al., 2012; Rocha et al., 2018). There are various modeling approaches that can bridge data gaps, combining qualitative and quantitative data through expert elicitation (Canavese et al., 2014). Integrating expert opinions with empirical data allows data collected at a local scale to inform and address problems at the ecosystem scale. We argue that models aimed at supporting decisions need to be linked to management action via an iterative framework that incorporates society (including culture), ecology, and deep uncertainty. The first step is to combine ecological realities, societal values, and possible actions to identify options that will increase ecosystem resilience.
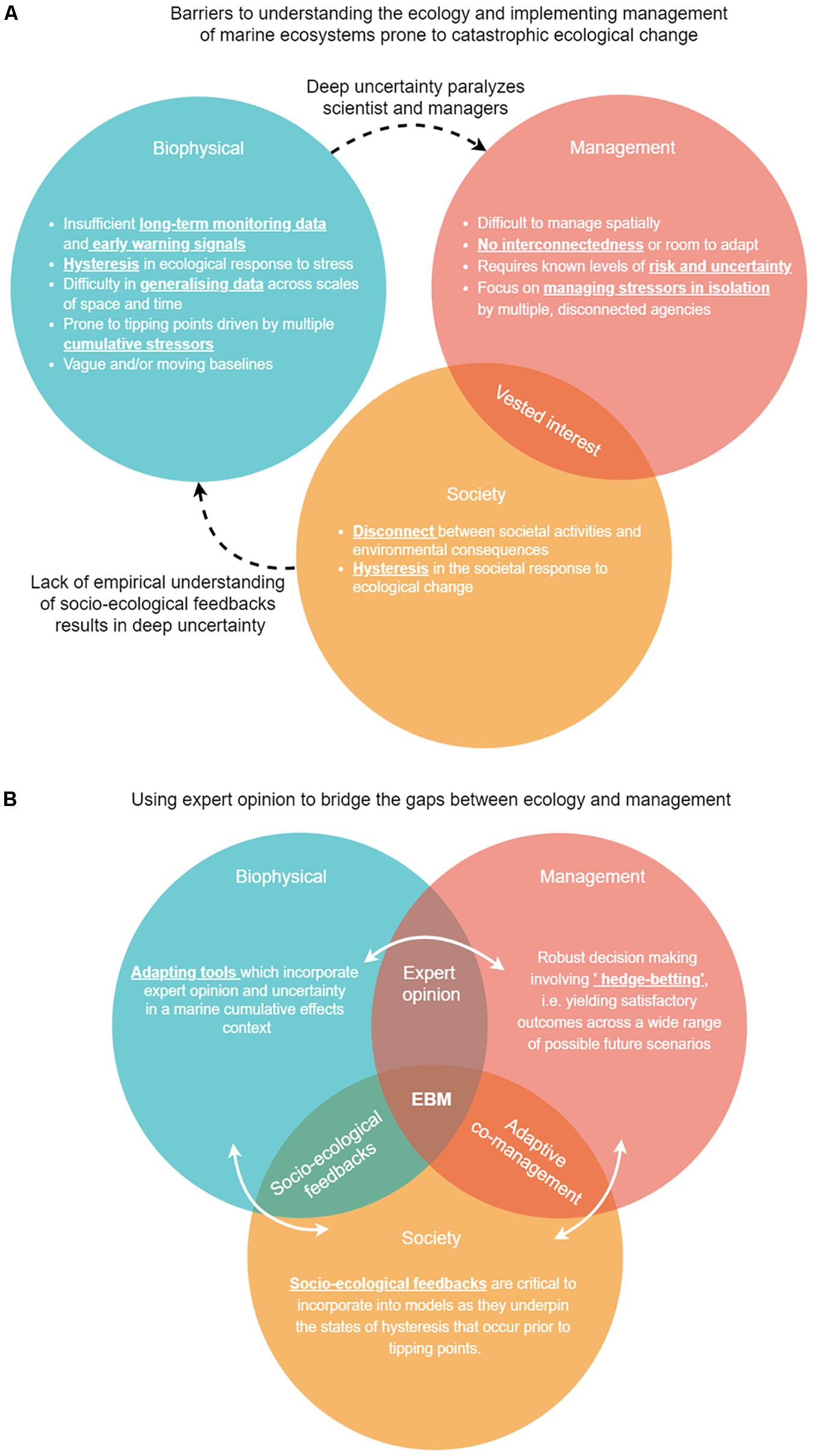
Figure 1. Graphical summary of (A) the current limitations to understanding and managing marine ecosystems in the context of surprise change arising from cumulative effects, and (B) the suggested solutions that use expert opinions to bridge the gaps between ecological knowledge, management, and society to consider multiple plausible futures in robust decision making.
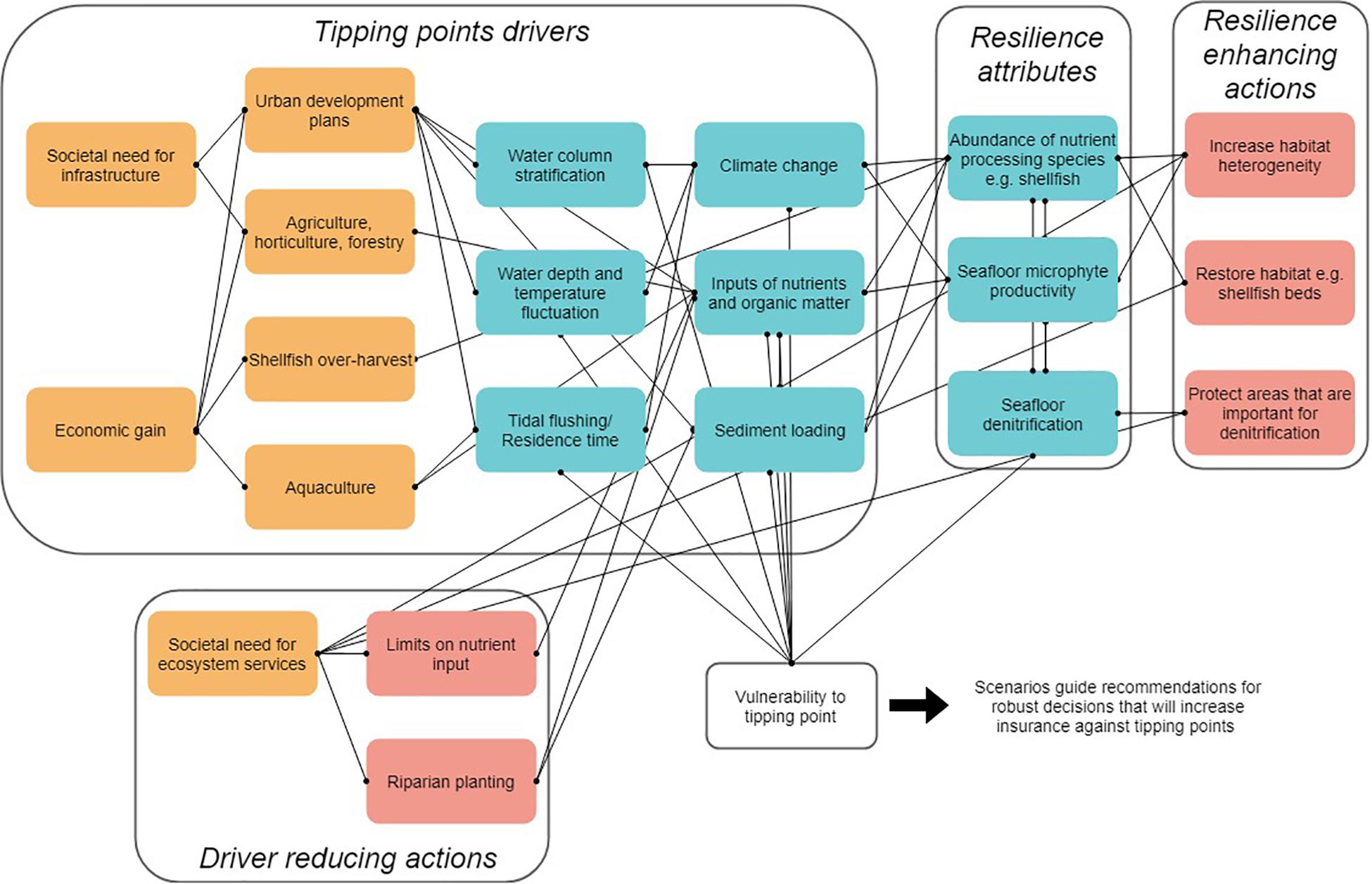
Figure 2. Coastal eutrophication – a simplified model linking biophysical ecosystem properties (blue), social drivers (orange), and management actions (pink). The hypothetical model shows how the different spheres in Figure 1 can be linked together to determine vulnerability of a coastal area to tipping points induced by nutrient enrichment.
Expert opinion models have been applied in marine spatial management, risk and impact assessment, and fisheries assessment, identifying the most likely outcomes through quantifying a probabilistic risk of human activities (e.g., Bayesian Networks and fuzzy set models; Silvert, 2000; Marcot et al., 2001; Wooldridge and Done, 2004; Cheung et al., 2005; Stelzenmüller et al., 2010, 2011; Landuyt et al., 2013; Ban et al., 2014; Jones and Cheung, 2018). In this context, uncertainty is partitioned into sectors (e.g., single species distribution or single-stressor impacts). However, in the context of tipping points where complex interactions introduce deep uncertainty, a more holistic approach is required. We need an approach that is not limited to cause-and-effect relationships, known probabilities, and quantifiable levels of uncertainty, but rather one that considers the possibility of different socio-ecological changes in the context of multiple plausible futures. Our key point is that models incorporating expert opinions are currently underutilized in a holistic marine cumulative effects’ context, but they offer a practical way forward to bridge gaps in quantitative data, enabling scientists and managers to consider and prepare for many possible futures (Figure 1). Here, we discuss the methodological and ideological considerations for using expert opinions in models of decision scenarios that can feed into a broader ecosystem-based management (EBM) framework with a key focus of building resilience; a framework that is now considered necessary to avoid further loss of societally valuable ecosystem services (IPBES, 2018).
Modeling With Expert Opinions in the Context of Cumulative Effects
In classical decision theory, it is assumed that probabilities can be assigned to the occurrence of events, where a model decision is portrayed as “clear-cut,” true or false. This theory has limited application in tipping points, as it requires specification of all potential future states and their probabilities of occurrence, which are often unknown when dealing with multiple drivers of change (Polasky et al., 2011). In this context, it is more appropriate to qualitatively assign variables to linguistic categories using expert opinions (e.g., “low,” “moderate,” “high”), accompanied by a qualitative level of confidence (e.g., a “low” increase in a stressor will be “most likely” to have a “low impact” on biodiversity) (Liu et al., 2015). This qualitative use of expert opinions allows progress to be made in identifying the likelihood of various outcomes even when uncertainty is deep (see below). There are several modeling approaches that integrate expert opinions with empirical data to investigate multiple ecosystem interactions: mental models (Moon et al., 2019), fuzzy set-theory models (Jarre et al., 2008; Zadeh, 2008), Bayesian networks (Uusitalo, 2007; Uusitalo et al., 2016), structural equation models (Hox and Bechger, 2009; Foley et al., 2015), agent-based models (Grimm et al., 2005), artificial neural networks (Lek and Guégan, 1999), or combinations of the above (Liu et al., 2015). In the context of tipping points, they must be designed acknowledging both socio-ecological feedbacks and importantly, deep uncertainty (Figure 1). Of course, expert opinion has caveats that need to be acknowledged and built into models, which include: (1) level of experience and confidence of experts; (2) representation of diverse world views; (3) shifting baselines in expert opinion over time; and (4) personal biases (Uusitalo, 2007; Kuhnert et al., 2010; Krueger et al., 2012).
Recognizing and Dealing With Uncertainty
Uncertainty can be classified into five levels along a spectrum from complete certainty to total ignorance (see Appendix; as described by Walker et al., 2013). The expert models we suggest can easily cope with intermediate uncertainty (levels 1–3) by exploring drivers and their interactions through sensitivity analysis and scenario planning (Mahmoud et al., 2009; Carpenter et al., 2015; Booth et al., 2016). While identifying all possible interactions may seem intractable, expert opinions offer a way of restricting combinations, and in the case of intermediate uncertainty, experts can often rank the likelihood of occurrence for several possible consequences (e.g., Teck et al., 2010). Sensitivity analysis identifies and quantifies which parts of a model contribute to uncertainty (e.g., Wooldridge and Done, 2004), pinpointing areas that need further expert elicitation and/or research (Saltelli et al., 2007). Scenario planning allows ecologists and managers to work coherently to iterate between stressor inputs and ecosystem outcomes (Bennett et al., 2003; Mahmoud et al., 2009; Carpenter et al., 2015; Booth et al., 2016). We can visualize this as dialing up or dialing back extrinsic drivers and observing the outcome on ecosystem function and the loss of intrinsic feedbacks and interactions within a network, advancing knowledge of early warning signals (Thrush and Dayton, 2010; Thrush et al., 2014; Foley et al., 2015); and providing insight into the combination and level of stressors that increase the vulnerability to tipping points.
Besides addressing intermediate uncertainty, decisions that manage for abrupt change need to consider deeper levels of uncertainty (levels 4–5; Walker et al., 2013). Deep uncertainty includes “black swan” events, which are events that lie outside the realm of regular expectations, carry extreme impacts, and are only identifiable on a retrospective basis (Taleb, 2007). Examples of this include the unexpected collapse of the Newfoundland cod fishery (and food web) and the multiple regime shifts in Chesapeake Bay, which were driven by feedbacks between human behavior, decisions, and multiple biophysical interactions (Steele et al., 1992; Milich, 1999; Hicks et al., 2016). In such contexts, experts need to collectively identify as many interacting factors as possible, and then qualitatively assign levels of vulnerability associated with these interactions (Box 1). Approaching uncertainty from an exploratory perspective rather than focusing on predicted outcomes helps stakeholders expand the range of futures they will consider; as there is no necessity for assignment of probability, one only need to accept the possibility that a scenario could occur (Marchau et al., 2019).
Box 1. Developing decision options for managing coastal ecosystems in the face of tipping points.
Five starting points:
1. Tipping points drivers – Use expert opinions to identify place-specific social and biophysical variables that make a particular area prone to a tipping point.
2. Resilience attributes – Identify social and biophysical variables that contribute to making the area of interest resilient to change.
3. Resilience enhancing actions – List actions that would enhance the resilience attributes.
4. Build place-specific models (e.g., Bayesian networks) that include tipping points drivers + resilience attributes + resilience enhancing actions + driver reducing actions (and their interactions) to explore vulnerability to a tipping point.
5. Use modeled scenarios to inform recommendations for robust actions that are likely to future proof the ecosystem to further cumulative changes (and tipping points). These modeled scenarios will provide information to participatory processes in a broader EBM framework where trade-offs on the recommended decisions (or lack of) can be explored.
Coastal eutrophication – an example:
Coastal eutrophication is a global driver of abrupt change in coastal areas (e.g., Chesapeake Bay, Baltic Sea; Zimmerman and Canuel, 2000; Boesch et al., 2001; Nyström et al., 2012; Tomczak et al., 2013; Carstensen et al., 2014). Often risk assessments and management decisions emphasize the cause and effect relationship between increases in the delivery of nutrients (the driver) and the (known) response of the ecosystem, without considering interactions between stressors (e.g., Wulff et al., 2007; Wulff et al., 2013; Plew et al., 2018). Despite knowledge of the non-linear dynamics of cumulative effects (Thrush and Dayton, 2010), ecosystems are still managed by this simple cause and effect framework, where management is solely focused on nutrients at the “source” and setting limits based on perceived thresholds. In some contexts, these limits may prevent eutrophication-induced tipping points, but in other contexts the coastal ecosystem flips into an alternative state (e.g., hypoxia, prevalent algal blooms, low biodiversity, and ecosystem service delivery), which can occur even when rational management decisions are made (e.g., lake ecosystems; Peterson et al., 2003).
Here, we use coastal eutrophication to show how the five-step program can be used to explore decisions that insure ecosystems against future cumulative effects and tipping points. In our hypothetical model structure, expert opinions would identify important drivers and actions (step 1–3) and define relationships between the nodes (step 4) (ideally direction, magnitude, and likelihood; Figure 2). Tipping points drivers are biophysical (blue) and social (orange) properties of a system that make it prone to tipping points. Many of the tipping points drivers are linked (directly or indirectly) to the resilience attributes and the driver reducing actions. The resilience attributes are the biophysical (blue) properties of the ecosystem that make it resilient to increased nutrient loading. The driver reducing actions are based on social need for ecosystem services (orange) and are actions (pink) that respond to this need by reducing the stressor (e.g., nutrient loading). Unfortunately, these driver reducing actions are not enough in isolation because other stressors (e.g., sediment loading, shellfish overharvesting) diminish the resilience attributes and exacerbate the tipping points drivers. Thus, models must also include resilience enhancing actions, which are management actions (pink) that improve resilience and insure against future cumulative effects (e.g., resulting from climate change). In this example, the driver reducing actions and resilience enhancing actions would be altered in different scenarios to assess the magnitude of the benefits of an action for reducing vulnerability to tipping points (step 5). The magnitude of benefit can be used to assess trade-offs between actions and their benefits, including costs (often difficult to monetize) associated with the gain or loss of various human values. This analysis should include the cost of inaction. Outcomes of scenarios can inform and provide the basis for various decision options (e.g., NEST model to explore decision options for restoration in the Baltic Sea; Wulff et al., 2013, 2014), but importantly these scenarios must inform resilience-enhancing decisions, as these are the decisions that will be important in the context of deep uncertainty and move beyond business as usual. Models focused on combining the social, biophysical, and management spheres (Figure 1 in the main text) are needed because of interactions between drivers and responses that make tipping points a multi-dimensional and multi-faceted problem.
Tipping points are deeply uncertain and must be managed on the basis that surprise can happen. However, decision-making in marine management still tends to focus on optimal policies, which aim to achieve the best possible outcome under a restricted set of criteria and assumptions (e.g., threshold assumptions). These criteria and assumptions are usually based on incomplete knowledge of the current state of a system (introducing deep uncertainty); therefore, the model that informed the policy is unlikely to reflect reality and the desired outcome is unlikely to be achieved. As with many fisheries, the Newfoundland cod fishery was managed to maximize yield assuming an equilibrium population state, but in reality, socio-ecological systems show an element of chaos and unpredictability in population and community fluctuations (Milich, 1999). Further, Chesapeake Bay nutrient management initially focused on point sources of pollution in isolation of other stressors (e.g., sediments and oyster overharvest) (Boesch et al., 2001; Hicks et al., 2016). Such limits-based management (e.g., on fishing intensity or nutrient input) is an example of an optimal policy that is typically designed to optimize exploitation within thresholds that protect some component(s) of the ecosystem. This assumes (1) thresholds remain the same under all conditions and (2) recovery of the managed ecosystem component does not involve hysteresis or is influenced by interacting stressors; assumptions that are often false (Crain et al., 2008; Darling and Côté, 2008). In addition to false assumptions, resource managers often fail to integrate ecological and economic models appropriately due to difficulty in assigning “values” to ecological functions and services, meaning that an optimal outcome is unlikely to capture the complexities of natural systems. Thus, policies that are considered or seen as optimal can result in regime shifts. When uncertainty is deep and “values” are subjective, approaches that seek robust and flexible policies (Marchau et al., 2019) are more appropriate than strategies defined under a narrow set of criteria and potentially incorrect assumptions.
Progress has been made to address decisions notwithstanding deep uncertainty, with research recommending: (1) policies that are robust across different scenarios (e.g., robust decision-making, info-gap decision theory) and (2) adaptive pathways where plans adapt as the system changes (Marchau et al., 2019). Robust policies are designed to yield “satisfactory” outcomes across a wide range of futures, and do not always maximize the immediate output or a perceived “value” like those of optimal policies (Rosenhead et al., 1972). While optimal policies put all our eggs in one basket, robust policies hedge our bets and seek a level of insurance against unknown future events. A robust policy or collection of decisions in the context of tipping points management may involve identifying not only drivers of change, but also actions that could change how an ecosystem responds to those drivers [i.e., resilience-enhancing actions; e.g., restoration of key habitats/species (Box 1), or fishing at levels where recruitment is likely to be successful under changing environmental conditions]. Tipping points are irreducibly uncertain, thus we cannot focus on predicting occurrence, but instead we should focus on identifying a desired future ecosystem state and developing robust plans to navigate toward that future (Walker et al., 2013; Le Heron et al., 2016). The incorporation of expert opinions into decision support models enables current quantitative and qualitative information to be used in an iterative process exploring future narratives, while eliminating need for probabilities, absolute numbers, and thresholds.
Linking Multiple Knowledge Systems to Decision Making
The effects of social feedbacks on ecosystem function are often ignored when modeling cumulative effects and abrupt change. Hysteresis (or lags in recovery) from intrinsic ecological feedbacks can be exacerbated in socio-ecological systems because of inertia in human reactions to ecological change (Sugiarto et al., 2015; Yletyinen et al., 2019), and mismatches between time scales of social and ecological change (e.g., Baltic Sea ecosystem response lags behind eutrophication management action; Nyström et al., 2012; Varjopuro et al., 2014). Managers are driven by the societal consequences of change in an ecosystem, yet the feedbacks among ecosystem components are often forgotten. Despite research on socio-ecological feedbacks and hysteresis (Carpenter et al., 2006; Yletyinen et al., 2019), most current management practices aimed at reducing environmental degradation of marine ecosystems have kept disciplines and knowledge systems separate (Folke, 2006). For example, Newfoundland fishery scientists deemed traditional ecological knowledge from the fishing community to be too inaccurate for assessing the state of the marine environment, a mistake that contributed to a socio-ecological collapse (Milich, 1999). Lessons from past tipping points tell us it is critical that decision scenarios are informed by the socio-ecological system as a whole, incorporating feedbacks and multiple knowledge systems from the various societal players (e.g., government, indigenous people, stakeholders, and community organizations). Socio-ecological interactions are embedded in a broader global socio-economic context which adds further complexity that needs to be considered (although out of the scope of this perspective see Österblom et al., 2017).
The social consequence of change will be context dependent. For a governing body with a mandate to protect the marine environment (e.g., UN Sustainable Development Goal #14 life below water), the priority should be to protect and maintain ecosystem function and services. Ensuring these mandates are prescribed is a critical part of EBM. Assessing “risk” involves both the probability of occurrence but also the severity/consequence of the event should it occur (Cormier et al., 2013; Selkoe et al., 2015). Since the occurrence of tipping points cannot be predicted, the assessment of “risk” should identify the societal and ecological harm it would cause. If the harm is high, but the probability of occurrence is deeply uncertain, then robust decisions that increase resilience are a way forward (Selkoe et al., 2015). Expert opinions from multiple fields and knowledge systems to build mind models (Folke, 2006; Moon et al., 2019) are a powerful way to identify the vulnerability of a marine area to tipping points and can inform participatory processes (an essential part of EBM) of resilience-enhancing actions that can reduce this vulnerability.
Models including expert opinions feed into an EBM framework allowing incorporation of multiple knowledge systems (Leslie and McLeod, 2007; Stephenson et al., 2018) so that interactive and cumulative impacts of multiple activities can be evaluated, even in the presence of uncertainty. This will require recognition and prioritization of trade-offs between different human values (Lester et al., 2013). In marine socio-ecological systems ecological functions such as nutrient cycling and primary production are commonly traded-off against more tangible ecosystem services such as food provisioning. However, such tradeoffs are unreasonable as the supply of these goods to society is underpinned by multiple, interacting processes, functions, and supporting services (Snelgrove et al., 2014). The loss of one ecosystem function may be accompanied by loss of multiple ecosystem services. Decision-making that incorporates the consequences of both ecological and social systems, as well as the intrinsic feedbacks are therefore essential for identifying trade-offs in possible future states when there is deep uncertainty. All this is critical if we are to move toward the EBM approaches required to maintain the function and services of marine ecosystems that contribute to humanity’s well-being (IPBES, 2018).
Given the burgeoning extrinsic forces pushing marine ecosystems into alternative states, we must find ways to inform effective decision making that not only focus on limiting drivers of change, but also building resilience. We argue that we must use the resources available today to provide practical recommendations for the management of marine ecosystems that are increasingly prone to tipping points. We already have the knowledge and tools, it is now a matter of applying these in the context of environmental surprise (associated with changes to intrinsic interaction networks). From an ideological perspective, this means redefining the way we think about managing socio-ecological systems for tipping points to include “surprise” and multiple possible futures that may be somewhat uncertain and may not have appropriately scaled data. This approach might be initially unpalatable to those who seek to make recommendations based on probabilities of occurrence and known levels of risk and uncertainty. However, the current expectation that we can assign a quantitative level of certainty to our actions and decisions is paralyzing us within a spiral of accelerating degradation to our marine environments. Holistic models driven by expert elicitation are currently underutilized in marine socio-ecological system management, but they offer an opportunity for generating practical and productive information to the wicked ecological tipping point problem (we offer five starting points in Box 1).
Data Availability Statement
No datasets were generated or analyzed for this study.
Author Contributions
RG-G, JH, RB, DC, and FS wrote the first draft of the manuscript. All authors contributed to the discussions which lead to the development of the ideas in the manuscript, to the review of the literature that is cited in the manuscript, and to the subsequent revisions, and read and approved the manuscript for publication.
Conflict of Interest
The authors declare that the research was conducted in the absence of any commercial or financial relationships that could be construed as a potential conflict of interest.
Funding
The New Zealand National Science Challenge Sustainable Seas, Tipping Points project funded a 3-day workshop in June 2017 that started the development of the ideas and recommendations in this manuscript. The Tipping Points project also funded RG-G, RB, FS, CP, CS, and SiT during the writing of the manuscript. RG-G was also supported by a New Zealand Rutherford Foundation Postdoctoral Fellowship from Government funding, administered by the Royal Society Te Apārangi, and the Walter and Andrée de Nottbeck Foundation.
Acknowledgments
We thank the two reviewers who provided constructive feedback that improved the manuscript.
References
Anderson, C. R., Moore, S. K., Tomlinson, M. C., Silke, J., and Cusack, C. K. (2015). “Chapter 17 - Living with harmful algal blooms in a changing world: strategies for modeling and mitigating their effects in coastal marine ecosystems,” in Coastal and Marine Hazards, Risks, and Disasters, eds J. F. Shroder, J. T. Ellis, and D. J. Sherman, (Boston, MA: Elsevier), 495–561. doi: 10.1016/b978-0-12-396483-0.00017-0
Ban, S. S., Pressey, R. L., and Graham, N. A. J. (2014). Assessing interactions of multiple stressors when data are limited: a Bayesian belief network applied to coral reefs. Glob. Environ. Change 27, 64–72. doi: 10.1016/j.gloenvcha.2014.04.018
Bennett, E., Carpenter, S., Peterson, G., Cumming, G., Zurek, M., and Pingali, P. (2003). Why global scenarios need ecology. Front. Ecol. Environ. 1, 322–329. doi: 10.2307/3868093
Boesch, D. F., Brinsfield, R. B., and Magnien, R. E. (2001). Chesapeake bay eutrophication: scientific understanding, ecosystem restoration, and challenges for agriculture. J. Environ. Qual. 30, 303–320. doi: 10.2134/jeq2001.302303x
Booth, E. G., Qiu, J., Carpenter, S. R., Schatz, J., Chen, X., Kucharik, C. J., et al. (2016). From qualitative to quantitative environmental scenarios: translating storylines into biophysical modeling inputs at the watershed scale. Environ. Modell. Softw. 85, 80–97. doi: 10.1016/j.envsoft.2016.08.008
Burthe, S. J., Henrys, P. A., Mackay, E. B., Spears, B. M., Campbell, R., Carvalho, L., et al. (2016). Do early warning indicators consistently predict nonlinear change in long-term ecological data? J. Appl. Ecol. 53, 666–676. doi: 10.1111/1365-2664.12519
Canavese, D., Ortega, N. R. S., and Queirós, M. (2014). The assessment of local sustainability using fuzzy logic: an expert opinion system to evaluate environmental sanitation in the Algarve region, Portugal. Ecol. Indicat. 36, 711–718. doi: 10.1016/j.ecolind.2013.09.030
Carpenter, S., Booth, E., Gillon, S., Kucharik, C., Loheide, S., Mase, A., et al. (2015). Plausible futures of a social-ecological system: Yahara watershed, Wisconsin, USA. Ecol. Soc. 20:10. doi: 10.5751/ES-07433-200210
Carpenter, S. R., Bennett, E. M., and Peterson, G. D. (2006). Scenarios for ecosystem services: an overview. Ecol. Soc. 11:29.
Carpenter, S. R., Mooney, H. A., Agard, J., Capistrano, D., DeFries, R. S., Díaz, S., et al. (2009). Science for managing ecosystem services: beyond the millennium ecosystem assessment. Proc. Natl. Acad. Sci. U.S.A. 106, 1305–1312. doi: 10.1073/pnas.0808772106%J
Carstensen, J., Conley, D. J., Bonsdorff, E., Gustafsson, B. G., Hietanen, S., Janas, U., et al. (2014). Hypoxia in the Baltic Sea: biogeochemical cycles, Benthic Fauna, and management. AMBIO 43, 26–36. doi: 10.1007/s13280-013-0474-7
Charles, A. T. (1997). Fisheries management in Atlantic Canada. Ocean Coast. Manag. 35, 101–119. doi: 10.1016/S0964-5691(97)00028-8
Cheung, W. W. L., Pitcher, T. J., and Pauly, D. (2005). A fuzzy logic expert system to estimate intrinsic extinction vulnerabilities of marine fishes to fishing. Biol. Conser. 124, 97–111. doi: 10.1016/j.biocon.2005.01.017
Cormier, R., Kannen, A., Elliott, M., Hall, P., and Davies, I. M. (2013). Marine and Coastal Ecosystem-Based Risk Management Handbook. ICES Cooperative Research Report No. 317. Denmark: ICES.
Costanza, R., de Groot, R., Braat, L., Kubiszewski, I., Fioramonti, L., Sutton, P., et al. (2017). Twenty years of ecosystem services: how far have we come and how far do we still need to go? Ecosyst. Ser. 28, 1–16. doi: 10.1016/j.ecoser.2017.09.008
Costanza, R., de Groot, R., Sutton, P., van der Ploeg, S., Anderson, S. J., Kubiszewski, I., et al. (2014). Changes in the global value of ecosystem services. Glob. Environ. Change 26, 152–158. doi: 10.1016/j.gloenvcha.2014.04.002
Crain, C. M., Kroeker, K., and Halpern, B. S. (2008). Interactive and cumulative effects of multiple human stressors in marine systems. Ecol. Lett. 11, 1304–1315. doi: 10.1111/j.1461-0248.2008.01253.x
Dakos, V., Carpenter, S. R., van Nes, E. H., and Scheffer, M. (2015). Resilience indicators: prospects and limitations for early warnings of regime shifts. Philos. Trans. R. Soc. B Biol. Sci. 370:1659. doi: 10.1098/rstb.2013.0263
Darling, E. S., and Côté, I. M. (2008). Quantifying the evidence for ecological synergies. Ecol. Lett. 11, 1278–1286. doi: 10.1111/j.1461-0248.2008.01243.x
de Young, B., Barange, M., Beaugrand, G., Harris, R., Perry, R. I., Scheffer, M., et al. (2008). Regime shifts in marine ecosystems: detection, prediction and management. Trends Ecol. Evol. 23, 402–409. doi: 10.1016/j.tree.2008.03.008
Douglas, E. J., Pilditch, C. A., Kraan, C., Schipper, L. A., Lohrer, A. M., and Thrush, S. F. (2017). Macrofaunal functional diversity provides resilience to nutrient enrichment in coastal sediments. Ecosystems 20, 1–13. doi: 10.1007/s10021-017-0113-4
Drijfhout, S., Bathiany, S., Beaulieu, C., Brovkin, V., Claussen, M., Huntingford, C., et al. (2015). Catalogue of abrupt shifts in intergovernmental panel on climate change climate models. Proc. Natl. Acad. Sci. U.S.A. 112, E5777–E5786. doi: 10.1073/pnas.1511451112
Foley, M. M., Martone, R. G., Fox, M. D., Kappel, C. V., Mease, L. A., Erickson, A. L., et al. (2015). Using ecological thresholds to inform resource management: current options and future possibilities. Front. Mar. Sci. 2:95. doi: 10.3389/fmars.2015.00095
Folke, C. (2006). Resilience: the emergence of a perspective for social–ecological systems analyses. Glob. Environ. Change 16, 253–267. doi: 10.1016/j.gloenvcha.2006.04.002
Folke, C., Carpenter, S. R., Walker, B. H., Scheffer, M., Elmqvist, T., Gunderson, L., et al. (2004). Regime shifts, resilience, and biodiversity in ecosystem management. Annu. Rev. Ecol. Evol.Syst. 35, 557–581. doi: 10.1146/annurev.ecolsys.35.021103.105711
Grimm, V., Revilla, E., Berger, U., Jeltsch, F., Mooij, W. M., Railsback, S. F., et al. (2005). Pattern-oriented modeling of agent-based complex systems: lessons from ecology. Science 310, 987–991. doi: 10.1126/science.1116681
Hewitt, J. E., and Thrush, S. F. (2019). Monitoring for tipping points in the marine environment. J. Environ. Manage. 234, 131–137. doi: 10.1016/j.jenvman.2018.12.092
Hicks, C. C., Crowder, L. B., Graham, N. A., Kittinger, J. N., and Cornu, E. L. (2016). Social drivers forewarn of marine regime shifts. Front. Ecol. Environ. 14, 252–260. doi: 10.1002/fee.1284
Hodgson, D., McDonald, J. L., and Hosken, D. J. (2015). What do you mean, ‘resilient’? Trends Ecol. Evol. 30, 503–506. doi: 10.1016/j.tree.2015.06.010
Hox, J. J., and Bechger, T. M. (2009). An introduction to structural equation modelling. Fam. Sci. Rev. 11, 354–373.
IPBES (2018). Summary for Policymakers of the Regional Assessment Report on Biodiversity and Ecosystem Services for Asia and the Pacific of the Intergovernmental Science-Policy Platform on Biodiversity and Ecosystem Services. Bonn: IPBES Secretariat.
Jarre, A., Paterson, B., Moloney, C. L., Miller, D. C. M., Field, J. G., and Starfield, A. M. (2008). Knowledge-based systems as decision support tools in an ecosystem approach to fisheries: comparing a fuzzy-logic and a rule-based approach. Progr. Oceanogr. 79, 390–400. doi: 10.1016/j.pocean.2008.10.010
Jones, M. C., and Cheung, W. W. L. (2018). Using fuzzy logic to determine the vulnerability of marine species to climate change. Glob. Change Biol. 24:e719–e731. doi: 10.1111/gcb.13869
Knight, A. T., Cowling, R. M., Rouget, M., Balmford, A., Lombard, A. T., and Campbell, B. M. (2008). Knowing but not doing: selecting priority conservation areas and the research–implementation gap. Conser. Biol. 22, 610–617. doi: 10.1111/j.1523-1739.2008.00914.x
Krueger, T., Page, T., Hubacek, K., Smith, L., and Hiscock, K. (2012). The role of expert opinion in environmental modelling. Environ. Modell. Softw. 36, 4–18. doi: 10.1016/j.envsoft.2012.01.011
Kuhnert, P. M., Martin, T. G., and Griffiths, S. P. (2010). A guide to eliciting and using expert knowledge in Bayesian ecological models. Ecol. Lett. 13, 900–914. doi: 10.1111/j.1461-0248.2010.01477.x
Landuyt, D., Broekx, S., D’Hondt, R., Engelen, G., Aertsens, J., and Goethals, P. L. M. (2013). A review of Bayesian belief networks in ecosystem service modelling. Environ. Modell. Softw. 46, 1–11. doi: 10.1016/j.envsoft.2013.03.011
Le Heron, R., Lewis, N., Fisher, K., Thrush, S., Lundquist, C., Hewitt, J., et al. (2016). Non-sectarian scenario experiments in socio-ecological knowledge building for multi-use marine environments: insights from New Zealand’s Marine Futures project. Mar. Policy 67, 10–21. doi: 10.1016/j.marpol.2016.01.022
Lek, S., and Guégan, J. F. (1999). Artificial neural networks as a tool in ecological modelling, an introduction. Ecol. Modell. 120, 65–73. doi: 10.1016/S0304-3800(99)00092-7
Leslie, H. M., and McLeod, K. L. (2007). Confronting the challenges of implementing marine ecosystem-based management. Front. Ecol. Environ. 5, 540–548. doi: 10.1890/060093
Lester, S. E., Costello, C., Halpern, B. S., Gaines, S. D., White, C., and Barth, J. A. (2013). Evaluating tradeoffs among ecosystem services to inform marine spatial planning. Mar. Policy 38, 80–89. doi: 10.1016/j.marpol.2012.05.022
Liu, K. F.-R., Kuo, J.-Y., Yeh, K., Chen, C.-W., Liang, H.-H., Sun, Y.-H., et al. (2015). Using fuzzy logic to generate conditional probabilities in Bayesian belief networks: a case study of ecological assessment. Int. J. Environ. Sci. Technol. 12, 871–884. doi: 10.1007/s13762-013-0459-x
Mac Nally, R., Albano, C., and Fleishman, E. (2014). A scrutiny of the evidence for pressure-induced state shifts in estuarine and nearshore ecosystems. Austral Ecol. 39, 898–906. doi: 10.1111/aec.12162
Mahmoud, M., Liu, Y., Hartmann, H., Stewart, S., Wagener, T., Semmens, D., et al. (2009). A formal framework for scenario development in support of environmental decision-making. Environ. Modell. Softw. 24, 798–808. doi: 10.1016/j.envsoft.2008.11.010
Marchau, V. A. W. J., Walker, W. E., Bloeman, P. J. T. M., and Popper, S. W. (eds) (2019). Decision Making Under Deep Uncertainty: From Theory to Practice. Switzerland. Berlin: Springer.
Marcot, B. G., Holthausen, R. S., Raphael, M. G., Rowland, M. M., and Wisdom, M. J. (2001). Using Bayesian belief networks to evaluate fish and wildlife population viability under land management alternatives from an environmental impact statement. Forest Ecol. Manag. 153, 29–42. doi: 10.1016/S0378-1127(01)00452-2
Milich, L. (1999). Resource mismanagement versus sustainable livelihoods: the collapse of the newfoundland cod fishery. Soc. Nat. Resour. 12, 625–642. doi: 10.1080/089419299279353
Moon, K., Guerrero, A. M., Adams, V. M., Biggs, D., Blackman, D. A., Craven, L., et al. (2019). Mental models for conservation research and practice. Conser. Lett. 2019:e12642. doi: 10.1111/conl.12642
Nyström, M., Norström, A. V., Blenckner, T., de la Torre-Castro, M., Eklöf, J. S., Folke, C., et al. (2012). Confronting feedbacks of degraded marine ecosystems. Ecosystems 15, 695–710. doi: 10.1007/s10021-012-9530-6
Österblom, H., Crona, B. I., Folke, C., Nyström, M., and Troell, M. J. E. (2017). Marine ecosystem science on an intertwined planet. Ecosystems 20, 54–61. doi: 10.1007/s10021-016-9998-6
Peterson, G. D., Carpenter, S. R., and Brock, W. A. (2003). Uncertainty and the management of multistate ecosystems: an apparently rational route to collapse. Ecology 84, 1403–1411. doi: 10.1890/0012-9658(2003)084
Plew, D., Dudley, B., Shankar, U., and Zeldis, J. (2018). Assessment of the Eutrophication Susceptibility of New Zealand Estuaries. New Zealand: National Institute of Water and Atmospheric Research.
Polasky, S., Carpenter, S. R., Folke, C., and Keeler, B. (2011). Decision-making under great uncertainty: environmental management in an era of global change. Trends Ecol. Evol. 26, 398–404. doi: 10.1016/j.tree.2011.04.007
Rocha, J., Yletyinen, J., Biggs, R., Blenckner, T., and Peterson, G. (2015). Marine regime shifts: drivers and impacts on ecosystems services. Philos. Trans. R. Soc. Lond. Ser. B Biol. Sci. 370:20130273. doi: 10.1098/rstb.2013.0273
Rocha, J. C., Peterson, G., Bodin, Ö., and Levin, S. (2018). Cascading regime shifts within and across scales. Science 362, 1379–1383. doi: 10.1126/science.aat7850
Rosenhead, J., Elton, M., and Gupta, S. K. (1972). Robustness and optimality as criteria for strategic decisions. Operat. Res. Q. 23, 413–431. doi: 10.2307/3007957
Saltelli, A., Ratto, M., Andres, T., Campolongo, F., Cariboni, J., Gatelli, D., et al. (2007). Global Sensitivity Analysis. The Primer. Hoboken, NJ: John Wiley & Sons, Ltd.
Scheffer, M., Carpenter, S., Foley, J. A., Folke, C., and Walker, B. (2001). Catastrophic shifts in ecosystems. Nature 413, 591–596. doi: 10.1038/35098000
Scheffer, M., Carpenter, S. R., Dakos, V., and Nes, E. H. V. (2015). Generic indicators of ecological resilience: inferring the chance of a critical transition. Annu. Rev. Ecol. Evol. Syst. 46, 145–167. doi: 10.1146/annurev-ecolsys-112414-054242
Selkoe, K. A., Blenckner, T., Caldwell, M. R., Crowder, L. B., Erickson, A. L., Essington, T. E., et al. (2015). Principles for managing marine ecosystems prone to tipping points. Ecosyst. Health Sustainabil. 1, 1–18. doi: 10.1890/EHS14-0024.1
Silvert, W. (2000). Fuzzy indices of environmental conditions. Ecol. Modell. 130, 111–119. doi: 10.1016/S0304-3800(00)00204-0
Snelgrove, P. V. R., Thrush, S. F., Wall, D. H., and Norkko, A. (2014). Real world biodiversity–ecosystem functioning: a seafloor perspective. Trends Ecol. Evol. 29, 398–405. doi: 10.1016/j.tree.2014.05.002
Steele, D. H., Andersen, R., and Green, J. M. (1992). The managed commercial annihilation of northern cod. Newfoundland Stud. 8, 34–68.
Stelzenmüller, V., Lee, J., Garnacho, E., and Rogers, S. I. (2010). Assessment of a Bayesian Belief Network-GIS framework as a practical tool to support marine planning. Mar. Pollut. Bull. 60, 1743–1754. doi: 10.1016/j.marpolbul.2010.06.024
Stelzenmüller, V., Schulze, T., Fock, H. O., and Berkenhagen, J. (2011). Integrated modelling tools to support risk-based decision-making in marine spatial management. Mar. Ecol. Progr. Ser. 441, 197–212. doi: 10.3354/meps09354
Stephenson, F., Mill, A. C., Scott, C. L., Stewart, G. B., Grainger, M. J., Polunin, N. V. C., et al. (2018). Socio-economic, technological and environmental drivers of spatio-temporal changes in fishing pressure. Mar. Policy 88, 189–203. doi: 10.1016/j.marpol.2017.11.029
Sugiarto, H. S., Chung, N. N., Lai, C. H., and Chew, L. Y. (2015). Socioecological regime shifts in the setting of complex social interactions. Phys. Rev. E 91:062804. doi: 10.1103/PhysRevE.91.062804
Taleb, N. N. (2007). The Black Swan: The Impact of the Highly Improbable. New York, NY: The Random House Publishing Group.
Teck, S. J., Halpern, B. S., Kappel, C. V., Micheli, F., Selkoe, K. A., Crain, C. M., et al. (2010). Using expert judgment to estimate marine ecosystem vulnerability in the California Current. Ecol. Appl. 20, 1402–1416. doi: 10.1890/09-1173.1
Thrush, S. F., and Dayton, P. K. (2010). What can ecology contribute to ecosystem-based management? Annu. Rev. Mar. Sci. 2, 419–441. doi: 10.1146/annurev-marine-120308-181129
Thrush, S. F., Hewitt, J. E., Dayton, P. K., Coco, G., Lohrer, A. M., Norkko, A., et al. (2009). Forecasting the limits of resilience: integrating empirical research with theory. Proc. R. Soc. B Biol. Sci. 276, 1–9. doi: 10.1098/rspb.2009.0661
Thrush, S. F., Hewitt, J. E., Parkes, S., Lohrer, A. M., Pilditch, C., Woodin, S. A., et al. (2014). Experimenting with ecosystem interaction networks in search of threshold potentials in real-world marine ecosystems. Ecology 95, 1451–1457. doi: 10.1890/13-1879.1
Thrush, S. F., Lewis, N., Le Heron, R., Fisher, K. T., Lundquist, C. J., and Hewitt, J. (2016). Addressing surprise and uncertain futures in marine science, marine governance, and society. Ecol. Soc. 21:44. doi: 10.5751/ES-08574-210244
Tomczak, M. T., Heymans, J. J., Yletyinen, J., Niiranen, S., Otto, S. A., and Blenckner, T. (2013). Ecological network indicators of ecosystem status and change in the baltic sea. PLoS One 8:e75439. doi: 10.1371/journal.pone.0075439
Uusitalo, L. (2007). Advantages and challenges of Bayesian networks in environmental modelling. Ecol. Modell. 203, 312–318. doi: 10.1016/j.ecolmodel.2006.11.033
Uusitalo, L., Korpinen, S., Andersen, J. H., Niiranen, S., Valanko, S., Heiskanen, A.-S., et al. (2016). Exploring methods for predicting multiple pressures on ecosystem recovery: a case study on marine eutrophication and fisheries. Cont. Shelf Res. 121, 48–60. doi: 10.1016/j.csr.2015.11.002
Varjopuro, R., Andrulewicz, E., Blenckner, T., Dolch, T., Heiskanen, A.-S., Pihlajamäki, M., et al. (2014). Coping with persistent environmental problems: systemic delays in reducing eutrophication of the Baltic Sea. Ecol. Soc. 19:48. doi: 10.5751/ES-06938-190448
Vitousek, P. M., Mooney, H. A., Lubchenco, J., and Melillo, J. M. (1997). Human domination of Earth’s ecosystems. Science 277, 494–499. doi: 10.1126/science.277.5325.494
Walker, W. E., Lempert, R. J., and Kwakkel, J. H. (2013). “Deep Uncertainty,” in Encyclopedia of Operations Research and Management Science, eds S. I. Gass, and M. C. Fu, (Boston: Springer), 395–402.
Wooldridge, S., and Done, T. J. C. R. (2004). Learning to predict large-scale coral bleaching from past events: a Bayesian approach using remotely sensed data, in-situ data, and environmental proxies. Coral Reefs 23, 96–108. doi: 10.1007/s00338-003-0361-y
Wulff, F., Humborg, C., Andersen, H. E., Blicher-Mathiesen, G., Czajkowski, M., Elofsson, K., et al. (2014). Reduction of baltic sea nutrient inputs and allocation of abatement costs within the baltic sea catchment. AMBIO 43, 11–25. doi: 10.1007/s13280-013-0484-5
Wulff, F., Oleg, P. S., Alexander, S., Humborg, C., and Mörth, C.-M. (2007). Management options and effects on a marine ecosystem: assessing the future of the baltic. AMBIO 36, 243–249. doi: 10.1579/0044-7447(2007)36
Wulff, F., Sokolov, A., and Savchuk, O. (2013). Nest – A Decision Support System for Management of the Baltic Sea: A User Manual. Baltic Nest Institute Technical Report No. 10. Stockholm: Baltic Nest Institute.
Yletyinen, J., Brown, P., Pech, R., Hodges, D., Hulme, P. E., Malcolm, T. F., et al. (2019). Understanding and managing social–ecological tipping points in primary industries. Bioscience 69, 335–347. doi: 10.1093/biosci/biz031
Zadeh, L. A. (2008). Is there a need for fuzzy logic? Inf. Sci. 178, 2751–2779. doi: 10.1016/j.ins.2008.02.012
Zimmerman, A. R., and Canuel, E. A. (2000). A geochemical record of eutrophication and anoxia in Chesapeake Bay sediments: anthropogenic influence on organic matter composition. Mar. Chem. 69, 117–137. doi: 10.1016/S0304-4203(99)00100-0
Appendix
We recognize that many definitions and interpretation of terminology exist. Thus, we include this list of terms that are used in this perspective piece to explicitly define our use of terminology.
Ecosystem function: The biological, geochemical, and physical changes in energy, matter, and processes that contribute to the self-maintenance of the ecosystem. Ecosystem functions underpin ecosystem services.
Ecosystem services: The benefits that humans derive from functioning ecosystems, which are underpinned by the ecological processes that contribute to human well-being (both directly or indirectly) (Costanza et al., 2017).
Extrinsic variable: A variable that is not part of how an ecosystem functions but can drive change in the intrinsic ecosystem processes. For example, the delivery of land-derived sediments and nutrients into the coastal waters can be considered extrinsic variables that drive change to an ecosystem’s intrinsic processes (Thrush et al., 2014).
Hysteresis: When ecosystems occur in different states under the same environmental conditions because of slow or altered recovery trajectories. Hysteresis can occur due to the feedbacks between internal ecosystem components which slow or change the trajectory of recovery back toward an original state, but they can also occur when one process lags behind another process, for example in the context of socio-ecological systems, the ecological effect can often lag the social change (Nyström et al., 2012; Sugiarto et al., 2015).
Intrinsic variable: A variable that is included in the network of processes that make an ecosystem function. For example, the micro-algae that grow on the seafloor are an intrinsic variable that deliver primary production and thus are integral to how the ecosystem functions (Thrush et al., 2014).
Resilience: We refer to resilience broadly (Hodgson et al., 2015) as the capacity of an ecosystem to maintain ecosystem function and services under stress or disturbance.
Resilience attributes: An attribute or variable that increases the resilience of the ecosystem [e.g., the presence of nutrient processing species on the seafloor increases resilience against eutrophication (Douglas et al., 2017)].
Scenario planning: a process of identifying future scenarios that are plausible but not always the most likely (Mahmoud et al., 2009).
Tipping point: A threshold after which the ecosystem enters another state of functionality, where recovery to the former state does not occur even when the pressure is released (Mac Nally et al., 2014). When we refer to tipping points, we are interested in tipping points/regime shifts that occur from cumulative effects of multiple drivers of change. These drivers of change can be small, but when they occur in combination with others, they can result in a surprise changes to an ecosystem state.
Uncertainty: The level of confidence we have in predicting an outcome. In the literature, there is a continuum of uncertainty that goes from complete certainty to total ignorance (Walker et al., 2013):
- Level 1: Confidence in the outcome of a decision and the probability of its occurrences.
- Level 2: Can assign a probability to a range of possible consequences.
- Level 3: Can rank the likelihood of occurrence of a range of possible consequences.
- Level 4: Unable to rank the likelihood of occurrence of multiple plausible outcomes.
- Level 5: The full range of possible trajectories or consequences is impossible to fully imagine or realize.
- Intermediate uncertainty: Levels 1–3 on the spectrum of uncertainty.
- Deep uncertainty: Levels 4 and 5 on the spectrum of uncertainty.
Keywords: regime shift, ecosystem function, tipping point, abrupt change, deep uncertainty
Citation: Gladstone-Gallagher RV, Hope JA, Bulmer RH, Clark DE, Stephenson F, Mangan S, Rullens V, Siwicka E, Thomas SF, Pilditch CA, Savage C and Thrush SF (2019) Old Tools, New Ways of Using Them: Harnessing Expert Opinions to Plan for Surprise in Marine Socio-Ecological Systems. Front. Mar. Sci. 6:696. doi: 10.3389/fmars.2019.00696
Received: 19 June 2019; Accepted: 29 October 2019;
Published: 14 November 2019.
Edited by:
Di Jin, Woods Hole Oceanographic Institution, United StatesReviewed by:
Geret Sean DePiper, National Oceanic and Atmospheric Administration (NOAA), United StatesMelina Kourantidou, Dalhousie University, Canada
Copyright © 2019 Gladstone-Gallagher, Hope, Bulmer, Clark, Stephenson, Mangan, Rullens, Siwicka, Thomas, Pilditch, Savage and Thrush. This is an open-access article distributed under the terms of the Creative Commons Attribution License (CC BY). The use, distribution or reproduction in other forums is permitted, provided the original author(s) and the copyright owner(s) are credited and that the original publication in this journal is cited, in accordance with accepted academic practice. No use, distribution or reproduction is permitted which does not comply with these terms.
*Correspondence: Rebecca V. Gladstone-Gallagher, rebecca.gladstone-gallagher@auckland.ac.nz