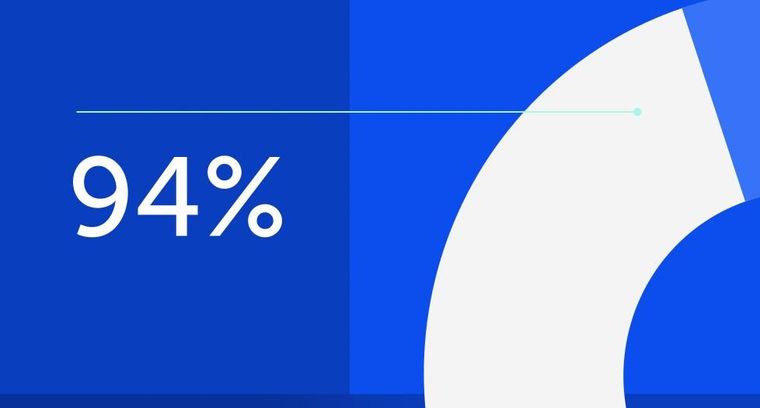
94% of researchers rate our articles as excellent or good
Learn more about the work of our research integrity team to safeguard the quality of each article we publish.
Find out more
ORIGINAL RESEARCH article
Front. Mar. Sci., 01 November 2019
Sec. Marine Molecular Biology and Ecology
Volume 6 - 2019 | https://doi.org/10.3389/fmars.2019.00659
Nowadays, most biodiversity assessments involving meiofauna are mainly carried out using very time-consuming, specimen-wise morphological identifications, which demands comprehensive taxonomic knowledge. Animals have to be examined for minor differences of setae compositions, mouthpart morphology or number of segments for various extremities. DNA-based methods such as metabarcoding as well as recently emerged rapid analyses using MALDI-TOF mass spectrometry to identify specimens based on a proteome fingerprint could vastly accelerate the process of specimen identification in biodiversity assessments. However, these techniques depend on reference libraries to connect collected data to morphologically described species. In this study the success rate of both approaches have been tested based on reference libraries constructed using part of the samples from a new study area to identify unknown samples. Using MALDI-TOF MS we found, that species which do not exist in an incomplete mass spectra reference library only have minor impact on the results, when employing a post hoc test for Random Forest classifications. This test reveals specimens that demand morphological re-examination for the final species assignment. Metabarcoding however strongly demands a rich reference library to provide correct MOTU assessments in congruence with morphological determination. Nevertheless, with a complete library and a suitable data transformation [herein log(x + 1)], the number of reads per MOTU reflects relative species abundances in metabarcoding inference. The results of this study facilitate specimen identification by using MALDI-TOF MS, which is incomparably cheap for specimen-by-specimen identification, but when it comes to sample-wise analyses, metabarcoding outperforms other techniques by far.
Assessing species’ diversity, distribution, and community structure is crucial to understand the relationship of species to the surrounding environment. Moreover, monitoring of communities is necessary to detect the influence of environmental changes on species compositions. Therefore, accurate species identification is necessary for biodiversity research.
Morphological identification in particular for the tiny meiofauna organisms is very challenging and time-consuming (e.g., Brannock et al., 2014; Morad et al., 2017; Rzeznik-Orignac et al., 2017). An exact determination often demands dissection of the smallest appendages (Huys et al., 1996) and a comprehensive taxonomic knowledge. Unfortunately, taxonomic identification using morphology only has shown to underestimate the true diversity compared to DNA-based methods (Tang et al., 2012), mainly because of cryptic diversity for many species (Knowlton, 1993).
Over the last years, several methods have been introduced to improve and accelerate species identification. The most commonly applied method is COI mtDNA barcoding, based on amplification of the mitochondrial cytochrome oxidase c subunit I fragment (Hebert et al., 2003a, b). Large data sets for many groups of animals have been published (e.g., Knebelsberger et al., 2014; Barco et al., 2016) and have been applied for rapid and reliable species identifications. However, this technique often demands taxa-specific optimization and several processing steps. Therefore, meiofauna barcoding surveys which often focus on identification of voucher specimens only (Vogt et al., 2014; Avó et al., 2017) may underestimate the true diversity (Tang et al., 2012) and consequently the importance of meiofauna.
Next generation sequencing approaches such as metabarcoding allow community analysis using batch samples (e.g., Taberlet et al., 2012; Leray and Knowlton, 2015; Fonseca V. et al., 2017) which not only reduces the expenses, but also decreases the effort of extraction, fragment amplification, purification, and sequencing from several 100 specimens to just processing whole samples in DNA-based biodiversity assessments. However, to reliably identify species from bulk samples, well-curated reference libraries are essential (e.g., Fonseca G et al., 2017) to provide connection between obtained OTUs to morphospecies.
Another promising method for species identification in biodiversity assessments originates from the field of microbiology. Matrix-Assisted Laser Desorption/Ionization Time-of-Flight mass spectrometry (MALDI-TOF MS) is commonly used to identify bacteria, viruses or fungi (Moura et al., 2008; La Scola et al., 2010; De Bruyne et al., 2011) based on a proteomic fingerprint. Also, pilot studies were carried out for several animal groups like fish (Mazzeo et al., 2008), dipterans (Feltens et al., 2010; Kaufmann et al., 2012) or copepods (Laakmann et al., 2013; Rossel and Martínez Arbizu, 2018a, 2019).
The method provides a fast and reliable workflow for specimen-by-specimen identification at low expenses. Studies on copepods and dipterans showed the ability to differentiate between cryptic species and the congruence of COI barcode and MALDI-TOF MS-based species delimitation (Müller et al., 2013; Bode et al., 2017). In case of whole specimen extraction from copepods, exuviae were retained and could be examined afterward for morphological identifications (Laakmann et al., 2013; Rossel and Martínez Arbizu, 2018a, b). In contrast to morphospecies assignments that were often used in ecological studies MALDI-TOF MS provides mass spectra that are comparable between studies.
Faunistic assessments are very important for understanding species relationships to the environment and DNA-based delimitation methods are widely used. Despite the fact that the accuracy of metabarcoding and MALDI-TOF MS strongly depend on reference libraries, DNA databases are remarkably poor in case of meiofauna organisms, even from well-studied areas like the North Sea (e.g., Vogt et al., 2014). Therefore, in this study, we aim at comparing the efficiency of metabarcoding and MALDI-TOF MS for community studies of previously un-sampled study sites by sampling a tidal flat and using some samples for building up DNA and mass spectra libraries and others for assessing biodiversity and community structures. Techniques will be analyzed in terms of identification success compared to morphological examination and expenses.
Samples were taken by hand with a syringe (Ø 3.1 cm, 5 cm depth) during low tide at a tidal flat (53°38′40.2′′ N 8°04′57.6′′ E) in front of the village Hooksiel in the littoral zone of the German North Sea coast on 19th April 2017. Twelve sandy sediment samples were fixed in absolute ethanol and stored overnight at −25°C.
Samples were sieved through a 40 μm sieve and density-gravity centrifuged according to McIntyre and Warwick (1984) employing Kaolin and Levasil® (Kurt Obermeier GmbH & Co. KG, Bad Berleburg, Germany). Until further processing, samples were stored at −25°C in absolute ethanol.
Adult specimens were sorted on ice from centrifuged samples and morphologically identified using different identification keys (Lang, 1948; Huys et al., 1996; Wells, 2007).
Five samples were used for Metabarcoding, five for MALDI-TOF MS measurements and two for the construction of 18S rRNA and mass spectra libraries (Figure 1).
Figure 1. Workflow of community identifications using metabarcoding and MALDI-TOF MS. DNA and MS reference libraries were constructed from specimens of two samples. The DNA library was used to assign species to Illumina MiSeq sequence reads from pooled samples. With incomplete library, this will however result in an unresolved community containing unidentified MOTUs. Based on the MS reference library a Random Forest model is constructed to classify all specimens from samples. The post hoc test is then used to validate or reject classifications. Rejected classifications are re-examined morphologically and subsequently assigned to the correct species. The resulting community consists of species based on MS and specimens identified morphologically.
Individual specimens were separated into 1.5 ml microcentrifuge tubes with 0.5 μl absolute ethanol. After complete evaporation of ethanol, 2 μl of a matrix solution containing α-Cyano-4-hydroxycinnamic acid (HCCA) as a saturated solution in 50% acetonitrile, 47.5% molecular grade water and 2.5% trifluoroacetic acid were added. After 5 min of incubation, the solution was applied to one spot for crystallization on a target plate.
Samples were measured using a Microflex LT/SH System (Bruker Daltonics), employing the flexControl 3.4 (Bruker Daltonics) software. Masses were measured from 2 to 20k Dalton. For peak evaluation, mass peak range from 2 to 10k Dalton was analyzed using a centroid peak detection algorithm, a signal to noise threshold of 2 and a minimum intensity threshold of 600, with a peak resolution higher than 400. Proteins/Oligonucleotide method was employed for fuzzy control with a maximal resolution 10 times above the threshold. For a sum spectrum, 240 satisfactory shots were summed up. One mass spectrum was measured for each specimen.
If retained after extraction of proteomic data, exuviae were stored in 70% ethanol at Senckenberg German Centre for Marine Biodiversity Research (DZMB, Wilhelmshaven, Germany).
18S rRNA (V1 and V2 hypervariable regions, ∼380 bp) was amplified using SSU-F04 forward primer (5′-GCTTGTCTCAAAGATTAAGCC-3′) and SSU-R22 reverse primer (5′-GCCTGCTGCCTTCCTTGGA-3′) (Blaxter et al., 1998) and were used as reference library for metabarcoding.
DNA was extracted from individual specimens using 20 μl InstaGene matrix (Bio-Rad Laboratories, Munich, Germany) in a vapo.protect Mastercycler pro S Cycler (Eppendorf, Hamburg, Germany) for 50 min at 56°C and 10 min at 96°C. The prosome body part was used for MALDI-TOF MS and urosome body part for extraction of DNA for simultaneous use of one specimen for proteomics and DNA sequencing. If retained after DNA extraction, exuviae were stored in 70% ethanol at Senckenberg German Centre for Marine Biodiversity Research.
Amplification of DNA fragments was done in a total reaction volume of 20 μl, containing 10 μl AccuStart II PCR ToughMix (QuantaBio, Beverly, MA, United States), 0.2 μl primers (20 pmol/μl), 2 to 5 μl DNA extract and the respective amount of molecular grade water. Amplifications were carried out in a vapo.protect Mastercycler pro S (Eppendorf, Hamburg, Germany) for both gene fragments.
Cycler settings for amplification of 18S fragment were: an initial denaturation step at 94°C for 3 minutes (min), denaturation at 94°C for 30 seconds (s), annealing at 57°C for 50 s and elongation at 72°C for 60 s. In total, 35 amplification cycles were carried out ending in a final elongation step for 2 min at 72°C.
Negative control samples were included in all amplification runs. From each PCR product, 2 μl were verified for size conformity by electrophoresis in a 1% agarose gel stained with GelREDTM using commercial DNA size standards.
PCR products were purified and sequenced at a contract sequencing facility (Macrogen Europe, Amsterdam, Netherlands). Trace files were assembled with SeqTrace (Stucky, 2012) and aligned in SeaView (Gouy et al., 2010). Sequences were checked for the amplification of the correct gene fragment by Blast search (Zhang et al., 2000; Morgulis et al., 2008).
18S rRNA gene fragments were amplified from seven harpacticoid species presented in the NGS library comprising Tachidius discipes Giesbrecht, 1881; Harpacticus flexus Brady & Robertson, 1873; Asellopsis intermedia (Scott, 1895); Laophonte sp.; Platychelipus littoralis Brady, 1880; Delavalia palustris Brady, 1868 and Ectinosomatidae sp. DNA amplification failed for the single specimen of D. palustris; hence, the 18S fragment was amplified using specimens of a former project (Table 1). Two of the 18S sequences from the selected specimens have shown the Q30 lower than optimal at both 5′ and 3′ end of the sequence immediately after and before the primer binding regions. To produce a reference library covering the full fragment size according to NGS libraries, the representative sequence of each species (with 100% similarity match) was retrieved from the Illumina reads.
Table 1. Specimens for which an 18S gene fragment was amplified with the according GenBank accession numbers.
Mass spectrometry data from all samples were processed together in R (version 3.2.3, R Core Team. (2018) using packages ‘MALDIquant’ (Gibb and Strimmer, 2012) and ‘MALDIquantForeign’ (Gibb, 2015). Protein mass spectra were trimmed to an identical range from 2,000 to 20,000 m/z and smoothed with the Savitzky-Golay method (Savitzky and Golay, 1964). The baseline was removed based on SNIP baseline estimation method (Ryan et al., 1988) and spectra were normalized using the TIC method implemented in MALDIquant. Noise estimation was carried out with a signal to noise ratio (SNR) of 7. Peaks were repeatedly binned using command ‘binpeaks’ with a tolerance of 0.002 in a strict approach to the number of peaks for the whole data set was reduced from 9344 to 652 peaks. The resulting intensity matrix was Hellinger transformed (Legendre and Gallagher, 2001) for further use in Random Forest (RF) (Breimann, 2001) analysis.
Based on the library (n = 115) an RF model was calculated using ‘randomForest’ R package (Liaw and Wiener, 2002) to classify specimens from the test samples (Figure 1). The model was generated using 2,000 trees with 35 analyzed characters at each tree split. To prevent overestimation of frequent species, ‘sampsize’ was set to 1.
RF classifications were tested using the post hoc test sensu Rossel and Martínez Arbizu (2018a) with a 1% quantile as the threshold for false positive classification. The function rf.post.hoc is available from the R package Rftools (Martínez Arbizu and Rossel, 2018). RF classifications rejected by the post hoc test were morphologically re-examined (Figure 1). For species with only one specimen in the reference library, a post hoc test could not be carried out. Hence, all specimens classified as this species were automatically regarded as false positive classifications and subsequently re-examined morphologically (Figure 1).
Five samples were prepared for metabarcoding containing selected specimens which were identified to the species level using a dissecting microscope. Specimens from one sample were pooled together and DNA was extracted from the pooled sample (Fontaneto et al., 2015) using E.Z.N.A. Mollusc DNA Kit (Omega Bio-tek).
A short fragment (∼380 bp) including the hypervariable regions V1 and V2 of the 18S gene (see Hadziavdic et al., 2014) was amplified using the primers SSUF04 and SSUR22 (Blaxter et al., 1998) in which the Nextera compatible Illumina adapter overhang sequences were added to the 5′ end of the locus-specific primers following the Illumina 16S Metagenomic Sequencing Library Preparation guide (15044223Rev.B), resulting in following composite primers:
5′-TCGTCGGCAGCGTCAGATGTGTATAAGAGACAG-SSUF04,
5′-GTCTCGTGGGCTCGGAGATGTGTATAAGAGACAG-SSUR22.
The first PCR was performed using the composite primers and Phusion High-Fidelity PCR Master Mix (Thermo Fisher) using 2 μl of template DNA and 0.5 μl of each primer (10 pmol) in a final 20 μl reaction volume with following protocol: initial denaturation for 2 min and 10 cycles of denaturation at 98°C for 15 s, annealing at 62°C for 30 s, extension at 72°C for 30 s followed by a final extension of 4 min.
Five μl of the first PCR products were purified using 2 μl of ExoSAP-IT PCR Product Cleanup Reagent (Thermo Fisher) following incubation of 5 min at 37°C and 1 min at 80°C.
A second PCR using Nextera XT V2 Indexed primers (dual indexing approach) was performed using 7 μl of the purified first PCR amplicons, 0.5 μl of each primer (10 pmol) in a final volume of 20 μl, and 10 amplification cycles of using the PCR protocol above. Incorporation of the full Illumina adapter to the amplicon was checked by comparing the length of the fragments of the 1st and 2nd PCR in a 1% Agarose Gel stained with 1% Gel Red. The amplicons from second PCR were purified from 1% agarose gel using Monarch DNA Gel Extraction Kit (NEB).
DNA concentration of each amplicon from second PCR was measured after extracting from agarose gel with Qubit Fluorometer using Qubit dsDNA High Sensitivity (HS) Assay (Thermo Fisher). For normalization, libraries were diluted to a concentration of 4 nM/μl and pooled together with libraries from another project at equal molarity. Five μl of the 4 nM pooled library was denatured into single stranded DNA using 5 μl of NaOH 0.2M and diluted to 20 pmol concentration using 990 μl of Hyb1 buffer (Illumina). To increase the diversity in the first sequencing runs, 10% volume of 20 pmol PhiX control was spiked into the library. The spiked Library was further diluted to 17 pmol prior sequencing on a MiSeq using V3 chemistry and 301 paired-end read frame.
The Illumina reads were processed using VSEARCH (Rognes et al., 2016) following the pipeline found at https://github.com/torognes/vsearch/wiki/VSEARCH-pipeline. Initially paired-end reads were used to create contigs for each sample with following settings: expected mean size 400 bp, allowed variation: ± 50 bp, minimum contig overlap: 50 bp, maximum allowed differences in the contig: 15 bp. Contigs were quality filtered with: maximum expected errors after contig creation: 0.5, maximum ambiguities in the contig: 0. Contigs were then de-replicated using two minimum counts to create a unique sequence. The initial OTU library was created by pooling all de-noised and de-replicated reads from all samples together and performing an initial clustering at 98% similarity. Then chimeras were detected and excluded. This final OTU library was used to create an OTU table by assigning the reads of the individual samples to OTUs at 97% similarity threshold. The generated OTUs have been clustered according to the reference library at the minimum identity of 97 and 100% length coverage.
The OTU table was analyzed with R package vegan (Oksanen et al., 2013). We tested four different transformations of the data in order to check which of them produces a higher similarity between community structure present in the sample (number of counts per specimen) and results of metabarcoding. The transformations applied were Chord (divide by total n per sample), Hellinger (square root of Chord), log(x + 1) and presence/absence. For each transformation, Spearman rank correlation between the similarity of samples based morphological identification and similarity based on metabarcoding results was tested with the Mantel test (function mantel in vegan). Community similarity was depicted using bar plots and non-metric multidimensional scaling (nMDS) (function metaMDS in vegan).
Expenses of different methods were calculated including kits, chemicals and prices for sequencing, excluding costs for pipette tips, microcentrifuge tubes, or instruments. For DNA barcoding, cost-effective chelex DNA extraction, plate purification and sequencing services were considered for calculations. Expenses of approaches using different volumes of matrix to adjust for specimen size and also matrix prices from different providers were analyzed for MALDI-TOF MS. Different indices kits for analysis of 24 or 96 samples simultaneously were compared for metabarcoding. Detailed information on the calculation of prices can be found in the supplementary (Supplementary Tables S1–S3).
The RF analysis was carried out based on a library containing 115 specimens of eight species (Table 2), including specimens for which DNA was extracted and a mass spectrum was measured simultaneously. However, test samples contained two further species, which were not part of the library (Enhydrosoma gariene Gurney, 1930, P. littoralis). Hence, the library was considered incomplete. Abundances in the test samples analyzed ranged from one specimen for Laophonte sp. and Ectinosomatidae sp. 38 to 19 for H. flexus (Table 2).
Table 2. Number of specimens identified for all samples morphologically (M) and classified by Random Forest (RF).
The incomplete library was used to calculate an RF model (n = 115, training error: 0.87%). This model was employed to classify specimens from the test samples (Table 2: RF). In total, of 133 specimens, five (3.76%) were misclassified by the RF model because the correct species was not part of the initial model. In the classification approach, one E. gariene and three P. littoralis specimens were misclassified as Laophonte spec., and a fourth P. littoralis specimen was classified as Microarthridion fallax Perkins, 1956. However, all of these classifications were rejected by the post hoc test (Tables 2, 3: FP) and could be morphologically re-examined to verify they belonged to species absent from the reference library (Table 2: FP – reviewed). Besides these, all specimens were classified correctly by Random Forest, including those species for which only one specimen was contained in the reference library (Laophonte sp., Ectinosomatidae sp. 38, and D. palustris). As described above, these were automatically recognized as false positives by the post hoc test and hence had to be re-examined mor.phologically. This confirmed the initial RF classification.
Table 3. Number of specimens present in the samples refers to metabarcoding and the resulting number of reads per sample.
The final reference library generated by sanger sequencing produced reference sequences ranging from 344 bp in Laophonte sp. and 369 bp in D. palustris. The pure pairwise genetic distances between the seven species of the library range between a minimum of 5% and a maximum of 14.1%. This validated our OTU clustering threshold of 97% similarity.
The MiSeq run had a cluster density of 1,024 K/mm2 generating 25.8 Million paired-end reads (92% cluster passing filter). From those, 69% (23.8 Million) passed the read filter and were de-multiplexed. The Hooksiel samples were multiplexed with a plankton metabarcoding project in the same run. A total of 5.6 million reads (23%) (between 3 and 5% or 1.2–0.71 million reads per sample) were assigned to this study. Samples used for metabarcoding are called AS, AT, AU, AV, and AW. Table 3 shows these samples as columns with the suffix ‘_r’ for reads from metabarcoding or ‘_s’ for specimens present in the sample. As DNA amplification from the only specimen of D. palustris failed, no reference for this species was available. Hence, metabarcoding failed to identify all species from the samples based on the incomplete reference library. However, the sequences of this species were assigned to a discrete MOTU, which could be further used for database searches or in general biodiversity assessments and comparisons. Adding genetic information for D. palustris from a previous project allowed further analyses of the data. With this, all seven species presented in the samples were detected by metabarcoding. However, H. flexus and Laophonte sp. Which were not included in the sample AU were recovered in the metabarcoding library by 14 reads each. Also P. littoralis has committed 3 and 1 reads from samples AT and AW, did not have any representative in the following reference libraries. Figure 2 shows the proportion of every species in the samples displaying the results of morphological identification (‘_s’) and metabarcoding number of reads (‘_r’) side by side to facilitate comparison. While Chord and Hellinger transformations show a certain similarity between the relative abundances of reads and specimens, the absolute values of relative abundances in a species by species comparison are still highly divergent. Remarkably, the relative abundance of A. intermedia (blue color in Figure 2) is constantly underestimated by the number of metabarcoding reads, while T. discipes (yellow) is overrepresented by the number of reads. The best agreement between morphological community structures and metabarcoding results derived from a log(x + 1) transformation of the data. The agreement in the relative proportions of number of specimens and number of reads is high across samples and species. The presence/absence transformation shows exact agreement of the samples AT and AW, and slight disagreement in samples AT, AU, and AW as explained above.
Figure 2. Comparison of the number of reads (‘_r’) to the number of morphologically identified specimens/species (‘_s’) for all samples with four different data transformations. Of the four transformations, log(x + 1) transformation and working with presence/absence data reflect the relative abundance and the presence of species best.
The Mantel test evidences significant spearman correlation among the similarity between samples of morphology assignment and metabarcoding (values shown in Figure 3) for Hellinger (r = 0.85, p = 0.042) and log(x + 1) (r = 0.9, p = 0.008) transformations, log(x + 1) over competing Hellinger transformation. However, Chord and presence/absence show no significant correlation in the Mantel test.
Figure 3. nMDS plots depicting reads per sample (‘_r’) and morphological species (‘_s’) for the four different data transformation. Again, presences/absence and log(x + 1) transformed data are most congruent comparing specimen and species abundances/presence.
The nMDS plots (Figure 3) show similarities of the samples based on community composition comparing morphological assessment and metabarcoding on the same ordination. The nMDS of the Chord transformed data appears visually as the worst of the three quantitative transformations (evidenced also by the non-significant mantel test). There is a raw agreement in the relative position of AU (green), AV (purple) and AS (blue), but AW (orange) and AT (red) show disagreement in the relative position between treatments. Interestingly, Hellinger transformation separates the treatments in ordination space, the samples from the metabarcoding (circles) showing less multivariate dispersal and located at the top-right quarter of the plot. The relative position of the samples to each other greatly agrees between treatments. The log(x + 1) nMDS plots shows the best agreement in the position of the samples in ordination space between the treatments. The couples of samples AV, AU appear close to each other and AT and AS show similar pattern. The nMDS with presence/absence data show complete agreement in samples AV and AS and lacks agreement in all others.
Based on MALDI-TOF mass spectra using RF and the post hoc test, all specimens were correctly identified. In contrast to the time demanding morphological identification, the post hoc test false positive assignment of the RF classification has greatly simplified and enhanced detection of the community composition and diversity assessments of the samples. Retaining the exuviae during the procession of specimens for MALDI-TOF MS has ensured the correct taxonomic assignment. Indeed an incomplete reference library, would lead to the misclassification of the specimens from the test samples according to the species available in the model. However, all of these classifications were recognized by the post-test and a subsequent morphological re-examination was carried out, identifying these specimens as new species. Therefore, it was shown that classification by RF using a post-test is also able to reveal species, which are new to the dataset. The results demonstrate the power of MALDI-TOF MS in combination with RF and the post hoc test for species identification based on an incomplete library as may be encountered when a new research area is accessed. According to the results, an incomplete library does not necessarily lead to misclassification of new species, which likely happens in unsupervised approaches like clustering algorithms (Collins and Cruickshank, 2013).
Therefore, even in new study areas, MALDI-TOF MS was found to be applicable by setting up a library from parts of the samples, accelerating biodiversity assessments by fast and reliable species identification compared to morphological assessments. Although one specimen per species was sufficient to create an RF model, which successfully classified all specimens of that species, using more specimens will certify the created RF model and implement a robust post hoc test to variability of the measured data.
Our results further align with low identification errors from other field studies of Bode et al. (2017) and Kaiser et al. (2018) on calanoid copepods or by Kaufmann et al. (2012) on biting midges (Diptera). However, most studies about metazoans using MALDI-TOF MS for species identification were pilot studies which did not provide biodiversity assessments (Kaufmann et al., 2012; Volta et al., 2012; Laakmann et al., 2013; Müller et al., 2013; Steinmann et al., 2013; Yssouf et al., 2013, 2014a,b; Dieme et al., 2014; Mathis et al., 2015; Hynek et al., 2018), making it difficult to compare results to existing literature. Nevertheless, all studies carried out so far provide high identification success making MALDI-TOF MS a promising tool for biodiversity assessments.
Our study showed the ability of metabarcoding to detect all species present in the samples including low abundant species as can be seen in Table 3. However, only a complete reference library can assure correct taxonomic assignment of the detected MOTUs to species. Because the public DNA depositories contain only partial records especially in case of meiofauna communities, enriching the reference libraries is crucial to assure factual diversity obtained by metabarcoding approach. Concerning the result of this study, the reads of D. palustris, the species missing from the reference library during the initial analyses, were clustered into a discrete MOTU, which further confirms the ability of metabarcoding using V1 and V2 hypervariable region of 18S in 97% pairwise similarity to discriminate the sequences into species. In congruence with previous metabarcoding studies (Guardiola et al., 2015; De Faria et al., 2018; Günther et al., 2018), we further suggest the successful use of 18S gene especially in case of metabarcoding of meiofauna communities in which amplification of COI barcode region has shown to be less successful for some organisms such as nematodes (Haenel et al., 2017). Laophonte sp. and D. palustris were presented with a single specimen in the libraries AS, AT, AW and AT, AW respectively and were recovered with the number of reads ranging from 34 to 1986, suggesting that, apart from the biomass which can effectively alter the number of reads (objective from A. intermedia, H. flexus, and T. discipes), other factors can also have influence on the absolute number of reads per MOTU.
As can be seen from Table 3 three species that were not present in the respective samples were recovered by metabarcoding with low number of reads. Sample AU had no H. flexus and Laophonte sp., however the species were recorded with 14 reads each. P. littoralis, which had no specimen in samples AT and AW, was recorded with 3 and 1 reads respectively. There are variety of reasons to explain the presence of these reads in the samples, the most probable explanations in our view being misassignment during de-multiplexing due to sequencing error in the index regions (Kircher et al., 2011) or tag jumping (Schnell et al., 2015). The wrong assignment of species to samples could be overcome by establishing a minimum number of reads to be achieved for a positive species assignment. The numbers of reads of wrong assignments are the lowest in the dataset (Table 3) ranging from 1 to 14 reads, which is less than the 34–1986 reads achieved when a single specimen was present (see above). However, we do not have an objective method for establishing a fixed threshold value for eliminating these reads. The threshold may change from study to study and even between sequencing runs of the same library. The wrong assignment of these species is the reason for the low performance of the presence/absence transformation, which otherwise would be very promising.
From the three quantitative transformations tested here, the log(x + 1) shows the best correlation between the similarity of samples based on morphological assignment and metabarcoding. Both the bar plot (Figure 2) and the multivariate nMDS plot (Figure 3) evidence greatest agreement with this transformation. Remarkably, the comparison of community structure between samples suggests the same ecological conclusion with both treatments. The samples AS and AW are converged while samples AU and AV are more divergent. Although there seems to be agreement that metabarcoding cannot estimate the absolute abundance of species in the samples (Elbrecht and Leese, 2015), our results show that the log(x + 1) transformation is accurate in estimating at least the relative abundance of the species within samples. The number of reads is most probably correlated to biomass, rather than abundance. The relatively low difference in biomass between the harpacticoid species analyzed here could be the reason for high quantitative agreements between abundances and number of reads after log(x + 1) transformation.
In this study, reliable results have been obtained from MALDI-TOF MS and metabarcoding for species identification in biodiversity assessments in an unexplored study area. Our results show that metabarcoding finds all of the species recorded morphologically if contained in a previously generated reference library. However, in studies lacking a rich reference library, metabarcoding can fail to connect the obtained sequences to morphospecies. Nevertheless, metabarcoding of bulk samples was found to reveal overseen diversity (Leray and Knowlton, 2015; Fonseca V. et al., 2017); for example, Platyhelminthes were excessively found by metabarcoding studies but less frequently by morphological analyses (Blaxter, 2016).
Regarding to the DNA barcoding, the delimitation thresholds defined by the user may under- or over-estimate the diversity (Carugati et al., 2015) because of varying inter- and intra-specific genetic distances (Bucklin et al., 2010). In addition, the effort, which has to be considered in case of DNA barcoding to sort out every individual, DNA extraction and PCRs, is considerably higher than both metabarcoding and MALDI-TOF.
MALDI-TOF MS and COI barcoding, however, can only analyze the submitted specimens; any diversity beyond this is disregarded. An overseen assignment due to uncovered very similar morphospecies can be re-checked for detection of minor morphological differences afterward. Therefore, assessed data is always related to an actual specimen and not only to a substitutional DNA sequence, fostering a better understanding.
Connectivity, population structures or phylogenetic relationships can only be analyzed employing DNA-based methods and more genetic markers amplified from available DNA extracts (Selkoe et al., 2016). Therefore, at the beginning of a study, it should be secured that the chosen method for species identification is the suitable one because MALDI-TOF MS can only be used to recognize species and different developmental stages (Laakmann et al., 2013; Bode et al., 2017) while metabarcoding assesses general biodiversity only.
Simultaneous use of individuals for barcoding and analysis in MALDI-TOF MS was shown several times (e.g., Laakmann et al., 2013; Bode et al., 2017; Rossel and Martínez Arbizu, 2018b; Rossel and Martínez Arbizu, 2019) even for small animals such as copepods, implying a possible combination of MALDI-TOF MS and DNA barcoding for biodiversity assessments and monitoring. Voucher specimens can be analyzed by MALDI-TOF MS and sequenced simultaneously to support identifications based on mass spectra by DNA without causing high costs by barcoding of all assessed specimens. Because MALDI-TOF MS provides a discrete species-specific signal, pitfalls for underestimating species diversity are avoided.
Unfortunately, obtaining DNA and mass spectra data from a single microscopic animal while retaining an intact voucher specimen for microscopic examination, has not been developed yet. Therefore, a subsequent comparison between morphological, DNA-based and MS-based methods is, yet, not possible for single specimens after measurements were carried out.
Based on our workflow and expenses, here we calculated the costs of single gene barcoding (e.g., COI barcoding), metabarcoding and MALDI-TOF MS (Figure 4) with hypothetical samples containing 96 specimens each. Although cost comparison strongly depends on the country where the study and analyses were carried out, the comparison can give a good impression of the difference in costs raised for the different applied methods. However, one has to be aware, that displayed costs will probably not reflect the actual costs in every country.
Figure 4. Comparison of expenses of the different species identification methods for hypothetical samples containing 96 specimens each. Set-ups for 24 and 96 samples were compared for metabarcoding. Besides the factory-delivered matrix for MALDI-TOF MS, cheaper competitive products are available, allowing identification of 9,216 specimens for less than 250€. In actual studies, metabarcoding would analyze 100s and 1000s of specimens within a single sample and would outperform the other techniques in terms of costs. Here, hypothetical samples were used to allow comparisons.
Metabarcoding is barely comparable to the other techniques considering the batch sample workflow. Using unsorted bulk samples, metabarcoding will outperform the other techniques in terms of costs and effort because especially in meiofauna biodiversity assessments, single samples can contain 1000s of specimens of different major taxa and species. However, the research questions and the desired output have to be considered, to justify possible high costs and effort caused by the method applied.
When comparing the two specimen-by-specimen methods, it is obvious that even the most expensive MALDI-TOF approach is still much cheaper than COI barcoding (Figure 4). Working plate-wise using 4 μl factory delivered matrix solution per specimen, one complete plate containing 95 specimens and one spot with bacterial standard (used for calibration) sums to only 40.15€ (0.42€ per specimen). In comparison to this, processing 96 specimens plate wise for COI barcoding including two directional sequencing costs 5.65€ per specimen. This adds up to more than 10-fold the price for MALDI-TOF MS. However, cheaper alternatives for MALDI-TOF MS are available. Competitive matrix can be purchased, reducing the costs per plate to 12.18€ while retaining the same purity of the solution. Less pure matrix can reduce the costs even more, to only 2.53€ per plate (0.03€ per specimen) and first tests showed no loss of signal quality, compared to the factory delivered matrix. This allows the identification of more than 9,000 specimens for only around 250€. Moreover, the volume of matrix should be adjusted to specimen size, thus it often has to be reduced to 3 or 2 μl, cutting the costs to around 187€ or 125€ respectively. Considering that barcoding of voucher specimens only was shown to underestimate true species diversity, MALDI-TOF MS provides a valuable alternative when assessing species diversities specimen-by-specimen because all specimens can be measured without causing high costs.
Metabarcoding is a promising tool to assess general diversity without emphasizing a certain animal group. Here we demonstrated that metabarcoding can detect species occurring in low biomass and abundances. However, providing several replicates from a sampling site is strongly recommended to produce a rich and complete reference library simultaneously, to be used for MOTU assignment for metabarcoding.
Our study shows that log(x + 1) transformation of the data produces multivariate community similarities that highly correlate with the morphological assignment. However, if a precise quantitative analysis of species and specimens is desired, MALDI-TOF MS is superior to metabarcoding as it results in more discrete quantitative results. Also, MALDI-TOF MS can overcome the COI barcoding as it is significantly cheaper and provides, with a good quality library, equally reliable species identifications within a considerably shorter time.
18S sequences are accessible via GenBank. Hellinger transformed data matrix containing all submitted MALDI-TOF MS spectra and respective metadata are available as a data set on dryad (doi: 10.5061/dryad.rxwdbrv46). Raw data can also be accessed via dryad (doi: 10.5061/dryad.rxwdbrv46).
SR, PM, and SK designed the study. SR and PM carried out the sampling. SR did the morphological identifications and setup of the reference libraries, and measured and analyzed the MALDI-TOF MS data. PM and SK carried out the metabarcoding analyses. SK and PM analyzed the metabarcoding data. All authors contributed to the writing of the manuscript.
SR was supported by a grant of Graduate School IBR from the Ministry for Science and Culture of Lower Saxony (IBR B7).
The authors declare that the research was conducted in the absence of any commercial or financial relationships that could be construed as a potential conflict of interest.
This is publication no. 8 of Senckenberg am Meer Proteome Laboratory and publication no. 65 of Senckenberg am Meer Metabarcoding and Molecular Laboratory.
The Supplementary Material for this article can be found online at: https://www.frontiersin.org/articles/10.3389/fmars.2019.00659/full#supplementary-material
Avó, A. P., Daniell, T. J., Neilson, R., Oliveira, S., Branco, J., and Adão, H. (2017). DNA barcoding and morphological identification of benthic nematodes assemblages of estuarine intertidal sediments: advances in molecular tools for biodiversity assessment. Front. Mar. Sci. 4:66.
Barco, A., Raupach, M. J., Laakmann, S., Neumann, H., and Knebelsberger, T. (2016). Identification of North Sea molluscs with DNA barcoding. Mol. Ecol. Resour. 16, 288–297. doi: 10.1111/1755-0998.12440
Blaxter, M. (2016). Imagining sisyphus happy: DNA barcoding and the unnamed majority. Phil. Trans. R. Soc. B 371:20150329. doi: 10.1098/rstb.2015.0329
Blaxter, M. L., De Ley, P., Garey, J. R., Liu, L. X., Scheldeman, P., Vierstraete, A., et al. (1998). A molecular evolutionary framework for the phylum Nematoda. Nature 392, 71–75. doi: 10.1038/32160
Bode, M., Laakmann, S., Kaiser, P., Hagen, W., Auel, H., and Cornils, A. (2017). Unravelling diversity of deep-sea copepods using integrated morphological and molecular techniques. J. Plankton Res. 39, 600–617. doi: 10.1093/plankt/fbx031
Brannock, P. M., Waits, D. S., Sharma, J., and Halanych, K. M. (2014). High-throughput sequencing characterizes intertidal meiofaunal communities in northern Gulf of Mexico (Dauphin Island and Mobile Bay, Alabama). Biol. Bull. 227, 161–174. doi: 10.1086/bblv227n2p161
Bucklin, A., Ortman, B. D., Jennings, R. M., Nigro, L. M., Sweetman, C. J., Copley, N. J., et al. (2010). A “Rosetta Stone” for metazoan zooplankton: DNA barcode analysis of species diversity of the Sargasso Sea (Northwest Atlantic Ocean). Deep Sea Res. Part II Top. Stud. Oceanogr. 57, 2234–2247. doi: 10.1016/j.dsr2.2010.09.025
Carugati, L., Corinaldesi, C., Dell’Anno, A., and Danovaro, R. (2015). Metagenetic tools for the census of marine meiofaunal biodiversity: an overview. Mar. Genom. 24, 11–20. doi: 10.1016/j.margen.2015.04.010
Collins, R. A., and Cruickshank, R. H. (2013). The seven deadly sins of DNA barcoding. Mol. Ecol. Resour. 13, 969–975. doi: 10.1111/1755-0998.12046
De Bruyne, K., Slabbinck, B., Waegeman, W., Vauterin, P., De Baets, B., and Vandamme, P. (2011). Bacterial species identification from MALDI-TOF mass spectra through data analysis and machine learning. Syst. Appl. Microbiol. 34, 20–29. doi: 10.1016/j.syapm.2010.11.003
De Faria, L. C., Di Domenico, M., Andrade, S. C., dos Santos, M. C., Fonseca, G., Zanol, J., et al. (2018). The use of metabarcoding for meiofauna ecological patterns assessment. Mar. Environ. Res. 140, 160–168. doi: 10.1016/j.marenvres.2018.06.013
Dieme, C., Yssouf, A., Vega-Rúa, A., Berenger, J.-M., Failloux, A.-B., Raoult, D., et al. (2014). Accurate identification of Culicidae at aquatic developmental stages by MALDI-TOF MS profiling. Parasit. Vectors 7:544. doi: 10.1186/s13071-014-0544-0
Elbrecht, V., and Leese, F. (2015). Can DNA-based ecosystem assessments quantify species abundance? Testing primer bias and biomass—sequence relationships with an innovative metabarcoding protocol. PLoS One 10:e0130324. doi: 10.1371/journal.pone.0130324
Feltens, R., Görner, R., Kalkhof, S., Gröger-Arndt, H., and von Bergen, M. (2010). Discrimination of different species from the genus Drosophila by intact protein profiling using matrix-assisted laser desorption ionization mass spectrometry. BMC Evol. Biol. 10:1. doi: 10.1186/1471-2148-10-95
Fonseca, G., Fontaneto, D., and Di Domenico, M. (2017). Addressing biodiversity shortfalls in meiofauna. J. Exp. Mar. Biol. Ecol. 502, 26–38. doi: 10.1016/j.jembe.2017.05.007
Fonseca, V., Sinniger, F., Gaspar, J., Quince, C., Creer, S., Power, D. M., et al. (2017). Revealing higher than expected meiofaunal diversity in Antarctic sediments: a metabarcoding approach. Sci. Rep. 7:6094. doi: 10.1038/s41598-017-06687-x
Fontaneto, D., Flot, J.-F., and Tang, C. Q. (2015). Guidelines for DNA taxonomy, with a focus on the meiofauna. Mar. Biodivers. 45, 433–451. doi: 10.1007/s12526-015-0319-7
Gibb, S. (2015). MALDIquantForeign: Import/Export routines for MALDIquant. A package for R. Available at: https://CRAN.R-project.org/package=MALDIquantForeign (accessed August 14, 2018).
Gibb, S., and Strimmer, K. (2012). MALDIquant: a versatile R package for the analysis of mass spectrometry data. Bioinformatics 28, 2270–2271. doi: 10.1093/bioinformatics/bts447
Gouy, M., Guindon, S., and Gascuel, O. (2010). SeaView version 4: a multiplatform graphical user interface for sequence alignment and phylogenetic tree building. Mol. Biol. Evol. 27, 221–224. doi: 10.1093/molbev/msp259
Guardiola, M., Uriz, M. J., Taberlet, P., Coissac, E., Wangensteen, O. S., and Turon, X. (2015). Deep-sea, deep-sequencing: metabarcoding extracellular DNA from sediments of marine canyons. PLoS One 10:e0139633. doi: 10.1371/journal.pone.0139633
Günther, B., Knebelsberger, T., Neumann, H., Laakmann, S., and Arbizu, P. M. (2018). Metabarcoding of marine environmental DNA based on mitochondrial and nuclear genes. Sci. Rep. 8:14822.
Hadziavdic, K., Lekang, K., Lanzen, A., Jonassen, I., Thompson, E. M., and Troedsson, C. (2014). Characterization of the 18S rRNA gene for designing universal eukaryote specific primers. PLoS One 9:e87624. doi: 10.1371/journal.pone.0087624
Haenel, Q., Holovachov, O., Jondelius, U., Sundberg, P., and Bourlat, S. J. (2017). NGS-based biodiversity and community structure analysis of meiofaunal eukaryotes in shell sand from Hållö island, Smögen, and soft mud from Gullmarn Fjord, Sweden. Biodivers. Data J. 2017:e12731. doi: 10.3897/bdj.5.e12731
Hebert, P. D. N., Cywinska, A., Ball, S. L., and deWaard, J. R. (2003a). Biological identifications through DNA barcodes. Proc. Biol. Sci. 270, 313–321. doi: 10.1098/rspb.2002.2218
Hebert, P. D. N., Ratnasingham, S., and deWaard, J. R. (2003b). Barcoding animal life: cytochrome c oxidase subunit 1 divergences among closely related species. Proc. Biol. Sci. 270(Suppl. 1), S96–S99.
Huys, R., Gee, J., Moore, C., and Hammond, R. (1996). Marine and Brackish Water Harpacticoid Copepods Part 1. Shrewsbury: Field Studies Council.
Hynek, R., Kuckova, S., Cejnar, P., Junková, P., Pvrikryl, I., and Rihová Ambrovzová, J. (2018). Identification of freshwater zooplankton species using protein profiling and principal component analysis. Limnol. Oceanogr. 16, 199–204. doi: 10.1002/lom3.10238
Kaiser, P., Bode, M., Cornils, A., Hagen, W., Arbizu, P. M., Auel, H., et al. (2018). High-resolution community analysis of deep-sea copepods using MALDI-TOF protein fingerprinting. Deep Sea Res. Part I Oceanogr. Res. Pap. 138, 122–130. doi: 10.1016/j.dsr.2018.06.005
Kaufmann, C., Steinmann, I. C., Hegglin, D., Schaffner, F., and Mathis, A. (2012). Spatio-temporal occurrence of Culicoides biting midges in the climatic regions of Switzerland, along with large scale species identification by MALDI-TOF mass spectrometry. Paras. Vectors 5:246. doi: 10.1186/1756-3305-5-246
Kircher, M., Sawyer, S., and Meyer, M. (2011). Double indexing overcomes inaccuracies in multiplex sequencing on the Illumina platform. Nucleic Acids Res. 40, e3. doi: 10.1093/nar/gkr771
Knebelsberger, T., Landi, M., Neumann, H., Kloppmann, M., Sell, A. F., Campbell, P. D., et al. (2014). A reliable DNA barcode reference library for the identification of the North European shelf fish fauna. Mol. Ecol. Resour. 14, 1060–1071. doi: 10.1111/1755-0998.12238
Knowlton, N. (1993). Sibling species in the sea. Ann. Rev. Ecol. Syst. 24, 189–216. doi: 10.1146/annurev.es.24.110193.001201
La Scola, B., Campocasso, A., N’Dong, R., Fournous, G., Barrassi, L., Flaudrops, C., et al. (2010). Tentative characterization of new environmental giant viruses by MALDI-TOF mass spectrometry. Intervirology 53, 344–353. doi: 10.1159/000312919
Laakmann, S., Gerdts, G., Erler, R., Knebelsberger, T., Martínez Arbizu, P., and Raupach, M. J. (2013). Comparison of molecular species identification for North Sea calanoid copepods (Crustacea) using proteome fingerprints and DNA sequences. Mol. Ecol. Resour. 13, 862–876. doi: 10.1111/1755-0998.12139
Legendre, P., and Gallagher, E. D. (2001). Ecologically meaningful transformations for ordination of species data. Oecologia 129, 271–280. doi: 10.1007/s004420100716
Leray, M., and Knowlton, N. (2015). DNA barcoding and metabarcoding of standardized samples reveal patterns of marine benthic diversity. Proc. Natl. Acad. Sci. U.S.A. 112, 2076–2081. doi: 10.1073/pnas.1424997112
Martínez Arbizu, P., and Rossel, S. (2018). RFtools: Miscellaneous Tools For Random Forest Models. Available at: https://github.com/pmartinezarbizu/RFtools (accessed October 1, 2017).
Mathis, A., Depaquit, J., Dvovrák, V., Tuten, H., Bañuls, A.-L., Halada, P., et al. (2015). Identification of phlebotomine sand flies using one MALDI-TOF MS reference database and two mass spectrometer systems. Paras. Vectors 8:266. doi: 10.1186/s13071-015-0878-2
Mazzeo, M. F., Giulio, B. D., Guerriero, G., Ciarcia, G., Malorni, A., Russo, G. L., et al. (2008). Fish authentication by MALDI-TOF mass spectrometry. J. Agric. Food Chem. 56, 11071–11076. doi: 10.1021/jf8021783
McIntyre, A., and Warwick, R. (1984). Meiofauna Techniques. Methods for the Study of Marine Benthos. Oxford: Blackwell, 217–244.
Morad, T. Y., Dubinsky, Z., and Iluz, D. (2017). Meiobenthos assemblages as bioindicators for coastal pollution assessment. Science 7, 409–423. doi: 10.4236/ojms.2017.73028
Morgulis, A., Coulouris, G., Raytselis, Y., Madden, T. L., Agarwala, R., and Schäffer, A. A. (2008). Database indexing for production MegaBLAST searches. Bioinformatics 24, 1757–1764. doi: 10.1093/bioinformatics/btn322
Moura, H., Woolfitt, A. R., Carvalho, M. G., Pavlopoulos, A., Teixeira, L. M., Satten, G. A., et al. (2008). MALDI-TOF mass spectrometry as a tool for differentiation of invasive and noninvasive Streptococcus pyogenes isolates. FEMS Immunol. Med. Microbiol. 53, 333–342. doi: 10.1111/j.1574-695X.2008.00428.x
Müller, P., Pflüger, V., Wittwer, M., Ziegler, D., Chandre, F., Simard, F., et al. (2013). Identification of cryptic Anopheles mosquito species by molecular protein profiling. PLoS One 8:e57486. doi: 10.1371/journal.pone.0057486
Oksanen, J., Blanchet, F. G., Kindt, R., Legendre, P., Minchin, P. R., O’hara, R., et al. (2013). Package “Vegan.” Community Ecology Package, Version 2.
Rognes, T., Flouri, T., Nichols, B., Quince, C., and Mahé, F. (2016). VSEARCH: a versatile open source tool for metagenomics. PeerJ 4, e2584. doi: 10.7717/peerj.2584
Rossel, S., and Martínez Arbizu, P. (2018a). Automatic specimen identification of Harpacticoids (Crustacea: Copepoda) using random forest and MALDI-TOF mass spectra, including a post hoc test for false positive discovery. Methods Ecol. Evol. 9, 1421–1434. doi: 10.1111/2041-210x.13000
Rossel, S., and Martínez Arbizu, P. (2018b). Effects of sample fixation on specimen identification in biodiversity assemblies based on proteomic data (MALDI-TOF). Front. Mar. Sci. 5:149.
Rossel, S., and Martínez Arbizu, P. (2019). Revealing higher than expected diversity of Harpacticoida (Crustacea: Copepoda) in the North Sea using MALDI-TOF MS and molecular barcoding. Sci. Rep. 9:9182. doi: 10.1038/s41598-019-45718-7
Ryan, C., Clayton, E., Griffin, W., Sie, S., and Cousens, D. (1988). SNIP, a statistics-sensitive background treatment for the quantitative analysis of PIXE spectra in geoscience applications. Nucl. Instrum. Methods Phys. Res. Section B 34, 396–402. doi: 10.1016/0168-583x(88)90063-8
Rzeznik-Orignac, J., Kalenitchenko, D., Mariette, J., Bodiou, J.-Y., Le Bris, N., and Derelle, E. (2017). Comparison of meiofaunal diversity by combined morphological and molecular approaches in a shallow Mediterranean sediment. Mar. Biol. 164, 40.
Savitzky, A., and Golay, M. J. (1964). Smoothing and differentiation of data by simplified least squares procedures. Anal. Chem. 36, 1627–1639. doi: 10.1021/ac60214a047
Schnell, I. B., Bohmann, K., and Gilbert, M. T. P. (2015). Tag jumps illuminated-reducing sequence-to-sample misidentifications in metabarcoding studies. Mol. Ecol. Resour. 15, 1289–1303. doi: 10.1111/1755-0998.12402
Selkoe, K. A., Aloia, C. C., Crandall, E. D., Iacchei, M., Liggins, L., Puritz, J. B., et al. (2016). A decade of seascape genetics: contributions to basic and applied marine connectivity. Mar. Ecol. Prog. Ser. 554, 1–19. doi: 10.3354/meps11792
Steinmann, I. C., Pflüger, V., Schaffner, F., Mathis, A., and Kaufmann, C. (2013). Evaluation of matrix-assisted laser desorption/ionization time of flight mass spectrometry for the identification of ceratopogonid and culicid larvae. Parasitology 140, 318–327. doi: 10.1017/S0031182012001618
Stucky, B. J. (2012). SeqTrace: a graphical tool for rapidly processing DNA sequencing chromatograms. J. Biomol. Tech. 23:90–93. doi: 10.7171/jbt.12-2303-004
Taberlet, P., Coissac, E., Pompanon, F., Brochmann, C., and Willerslev, E. (2012). Towards next-generation biodiversity assessment using DNA metabarcoding. Mol. Ecol. 21, 2045–2050. doi: 10.1111/j.1365-294X.2012.05470.x
Tang, C. Q., Leasi, F., Obertegger, U., Kieneke, A., Barraclough, T. G., and Fontaneto, D. (2012). The widely used small subunit 18S rDNA molecule greatly underestimates true diversity in biodiversity surveys of the meiofauna. Proc. Natl. Acad. Sci. U.S.A. 109, 16208–16212. doi: 10.1073/pnas.1209160109
Vogt, P., Miljutina, M., and Raupach, M. J. (2014). The application of DNA sequence data for the identification of benthic nematodes from the North Sea. Helgol. Mar. Res. 68, 549–558. doi: 10.1007/s10152-014-0411-6
Volta, P., Riccardi, N., Lauceri, R., and Tonolla, M. (2012). Discrimination of freshwater fish species by matrix-assisted laser desorption/ionization-time of flight mass spectrometry (MALDI-TOF MS): a pilot study. J. Limnol. 71:e17.
Wells, J. B. J. (2007). An Annotated Checklist and Keys to the Species of Copepoda Harpacticoida (Crustacea). Auckland: Magnolia Press.
Yssouf, A., Flaudrops, C., Drali, R., Kernif, T., Socolovschi, C., Berenger, J.-M., et al. (2013). Matrix-assisted laser desorption ionization-time of flight mass spectrometry for rapid identification of tick vectors. J. Clin. Microbiol. 51, 522–528. doi: 10.1128/JCM.02665-12
Yssouf, A., Parola, P., Lindström, A., Lilja, T., L’Ambert, G., Bondesson, U., et al. (2014a). Identification of European mosquito species by MALDI-TOF MS. Parasitol. Res. 113, 2375–2378. doi: 10.1007/s00436-014-3876-y
Yssouf, A., Socolovschi, C., Leulmi, H., Kernif, T., Bitam, I., Audoly, G., et al. (2014b). Identification of flea species using MALDI-TOF/MS. Comp. Immunol. Microbiol. Infect. Dis. 37, 153–157. doi: 10.1016/j.cimid.2014.05.002
Keywords: meiofauna, proteomic fingerprint, species identification, COI, barcoding, specimen identification, harpacticoida, copepoda
Citation: Rossel S, Khodami S and Martínez Arbizu P (2019) Comparison of Rapid Biodiversity Assessment of Meiobenthos Using MALDI-TOF MS and Metabarcoding. Front. Mar. Sci. 6:659. doi: 10.3389/fmars.2019.00659
Received: 06 May 2019; Accepted: 09 October 2019;
Published: 01 November 2019.
Edited by:
Andrew Stanley Mount, Clemson University, United StatesReviewed by:
Erik Michael Pilgrim, United States Environmental Protection Agency, United StatesCopyright © 2019 Rossel, Khodami and Martínez Arbizu. This is an open-access article distributed under the terms of the Creative Commons Attribution License (CC BY). The use, distribution or reproduction in other forums is permitted, provided the original author(s) and the copyright owner(s) are credited and that the original publication in this journal is cited, in accordance with accepted academic practice. No use, distribution or reproduction is permitted which does not comply with these terms.
*Correspondence: Sven Rossel, c3Zlbi5yb3NzZWxAc2VuY2tlbmJlcmcuZGU=
Disclaimer: All claims expressed in this article are solely those of the authors and do not necessarily represent those of their affiliated organizations, or those of the publisher, the editors and the reviewers. Any product that may be evaluated in this article or claim that may be made by its manufacturer is not guaranteed or endorsed by the publisher.
Research integrity at Frontiers
Learn more about the work of our research integrity team to safeguard the quality of each article we publish.