- 1Department of Economics and Management, University of Helsinki, Helsinki, Finland
- 2Centre for Ocean Life, National Institute of Aquatic Resources, Technical University of Denmark, Lyngby, Denmark
- 3Department of Sociology, Environmental and Business Economics, University of Southern Denmark, Esbjerg, Denmark
Game theory has been an effective tool to generate solutions for decision making in fisheries involving multiple countries and fleets. Here, we use a coupled bio-economic model based on a Baltic Sea dynamic multispecies food web model called BALMAR and, we compare non-cooperative (NC) and cooperative game (grand coalition: GC) solutions. Applications of game theory based on a food web model under climate change have not been studied before and the present study aims to fill this gap in the literature. The study focuses on the effects of climate variability on the biological, harvest and economic output of the game models by examining two different climate scenarios, a first scenario characterized by low temperature and high salinity and a second scenario by high temperature and low salinity. Our results showed that in the first scenario sprat spawning stock biomass (SSB) and harvest dropped dramatically both in the NC and the GC cases whereas, herring and cod SSBs and harvests were higher compared to a base scenario (BS) keeping temperature and salinity at mean historical levels. In the second scenario, the sprat SSB and the harvest was higher for both GC and NC cases while the cod and the herring SSBs and harvests were lower. The total GC payoffs clearly outperformed the NC payoffs across all scenarios. Likewise, the first and second scenario GC payoffs for countries were higher except for Poland. The findings suggested the climate vulnerability of Baltic Sea multi-species fisheries and these results would support future decision-making processes of Baltic Sea fisheries.
Introduction
Game theory has been an effective tool to generate solutions for decision making in many fields (e.g., policy making, military methodologies, environmental and natural resource economics and management) (Eatwell et al., 1989). In general, the nature of game theory is highly suited for management problems in fisheries, as the fishers want to increase their economic profits from their activity that generates positive and negative externalities for the resource users and non-users (Bailey et al., 2010). One of the important management problems around the world is open access use of the fisheries resources without cooperative agreement. Non-cooperation is quite common among fishing states. In particular, many conflicts arise concerning fishing rights on highly migratory and range shifting species (Pinsky et al., 2018), often as a response to climate change (Perry et al., 2005; Pinsky et al., 2013). Cooperative agreements provide resilience through time so that the states can react flexibly to the impact of unexpected shifts in, e.g., biology, climate, and economy (FAO, 2016). Therefore, there is a clear need to understand and predict the impacts of climate change. Otherwise, disputes over cooperative agreements can be inevitable (Miller et al., 2001; Sissener and Bjørndal, 2005). One instrument to provide flexibility for such conflicts is side payments that prevent the losses generated by the inequality raised by these shifts (induced mainly by climate change) (Miller and Munro, 2004). Previously, single-species game theoretic studies (e.g., Diekert et al., 2010; Bjørndal and Lindroos, 2012; Kulmala et al., 2013) and multispecies game theoretic models have been utilized for various fisheries management issues concerning climatic and other environmental variations in the literature (Nieminen et al., 2012, 2016).
Baltic Sea fisheries constitute prime examples of common pool fisheries managed by European Union Common Fisheries Policy (EU-CFP). The EU fishing nations in the Baltic Sea jointly agree on an annual total allowable catch (TAC) for each commercially important stock. The TACs are shared among participating nations considering the relative stability principle that determines harvest quotas based on historical catch records of the EU member states whereas, the nationwide TAC is shared among fishermen according to country specific rules (Nieminen et al., 2016). Denmark, Poland, and Sweden have been the dominant cod (Gadus morhua callarias) fishing nations in the Baltic Sea for the last two decades. These countries are also actively involved in fishing sprat (Sprattus sprattus) and herring (Clupea harengus membras) that are key prey for the Eastern Baltic cod. During the past decades the Baltic Sea has experienced pronounced changes in hydrographic conditions, notably a marked long-term increase in temperature, decrease in salinity and deep-water oxygen concentrations (Meier, 2006; Neumann, 2010), as well increased eutrophication causing widespread algae blooms (Mackenzie et al., 2007; Markus Meier et al., 2011; Neumann et al., 2012). These abiotic changes have led to large-scale ecosystem changes, i.e., regime shifts, occurring in the late 1980s (Möllmann et al., 2009; Casini et al., 2012; Blenckner et al., 2015) that particularly affected the recruitment of the commercially important species, cod, sprat, and herring (Cardinale et al., 2009; Margonski et al., 2010; Thøgersen et al., 2015).
In the literature there are few applications of game theory in different environmental variation problems. For example, management implications of sprat, herring, and cod in the Eastern Baltic Sea under changing climate scenarios were studied by Thøgersen et al. (2015). The authors represented the bioeconomic output of three management scenarios based on a multi-species multi-fleet bioeconomic model. They concluded that the management plan in practice for cod have negative impact on the cod abundance and on the economic gains of fishermen and, this negativity can be eradicated by a reduction in fishing mortality. In another study, Wang and Ewald (2010) highlighted the positive output for competing species survival in a prey-predator system under cooperative management with climate variation whereas, non-cooperation resulted in stock collapse. Brandt and Kronbak (2010) represented the changes in stability of fishery agreements under different scenarios. The authors investigated the stability of fishery agreements under climate uncertainty based on an age-structured bioeconomic model and concluded that climate change has negative impact on the payoffs by decreasing the likelihood of establishment of stable cooperative agreements. Nieminen et al. (2012) evaluated Baltic sprat, herring, and cod fisheries for changing salinity scenarios including a species interaction function into a bioeconomic model. They found that lower fishing mortality would result in higher economic input whereas, under a high salinity scenario, cod stock achieved better levels of recruitment. Nieminen et al. (2016) investigated a multispecies partition function game among three asymmetric nations bordering the Baltic Sea. They showed that the full cooperation among three nations can be stabilized if the dominant nation compensates the other nations. They also presented higher revenue under cooperation if the cod biomass declined under climate change.
In this study, we applied a novel multi-species game theory approach for Baltic Sea fisheries based on a food web model to investigate and compare non-cooperative (NC) and cooperative game (grand coalition: GC) solutions under different climate scenarios. Our study focus on the effects of climate variability on the biological, harvest, and economic output of the NC and GC approaches by examining two different climate scenarios representing favorable and unfavorable temperature and salinity conditions for the stock status of cod, sprat and herring compared to a base scenario (BS) with climate conditions maintained unchanged at their mean historical levels. To assess the sensitivity of the model we used varying economic parameters including cost, price and discount rate.
Materials and Methods
Biological Model: Setup and Validation
We established the bioeconomic models based on the previously developed Baltic Sea food-web model called BALMAR (Lindegren et al., 2009; Supplementary Figure S1). BALMAR represents the food web dynamics of sprat, herring and cod (the three ecologically and commercially most important species in the Central Baltic Sea) and accounts for their pair-wise species interactions, as well as climate and fishing impacts on their recruitment and survival. This statistical model is based on a theoretical approach for modeling long-term population dynamics (Ives et al., 2003) and is given by:
where X are spawning stock biomass (SSB) values of cod, sprat and herring derived from a multi-species fish stock assessment model (ICES, 1996) at time t and t − 1, respectively, and B is a 3 × 3 matrix of pair-wise species interaction parameters. The covariate vector U contains time-series of estimated mean annual fishing mortalities (F) and a number of selected environmental variables known to affect recruitment of cod, sprat, and herring, respectively (Köster et al., 2003, 2005; Nissling, 2004; Möllmann et al., 2005; Dickmann et al., 2007). These include time series of summer bottom (80–100 m) salinity, spring surface (0–10 m) temperature and the log(abundance) of the key zooplankton prey (Pseudocalanus acuspes) for herring (Möllmann et al., 2005). The data was provided by the ICES/HELCOM Working Group on Integrated Assessments of the Baltic Sea (ICES, 2008). The effect of the fishing and environmental variables on each species are represented by the diagonal parameters in the matrix (C). Regression parameters were found by maximum likelihood estimation using a Kalman filter (Harvey, 1989). E is the process error, V is the observation error of the covariance matrix of the normal random variable. Y is the true observed state. The fitted model parameters captured accurately the known mechanisms of species interactions (Supplementary Figure S1), including density-dependence, competition between sprat and herring and cod predation on both sprat and herring (Köster and Möllmann, 2000; Neuenfeldt and Köster, 2000; Möllmann et al., 2005). Furthermore, the model parameters also illustrate the negative effect of fishing and the positive effect of the environmental variables including temperature, salinity, and zooplankton on sprat, cod, and herring, respectively (MacKenzie and Köster, 2004; Köster et al., 2005; Möllmann et al., 2005).
A number of diagnostics were applied to assess whether the final food-web model (Supplementary Figure S1) gave a reasonable description of the food-web dynamics (Supplementary Figure S2). The assumption of normality of the error terms is supported by an analysis of the residuals (Supplementary Figure S3). A partial autocorrelation analysis of the residuals further indicates that the model errors were independent for all species and lags. Finally, a stability analysis of the final parameters of the community matrix, B reveal a dominant eigenvalue below one (λ1 = 0.93), indicating a stable food-web model dynamic. The predictive capabilities of the food-web model was validated by a sequential refitting procedure where the model was initially fitted to only the first 10 years of the data set and then refitted on a yearly basis, producing a prediction for each consecutive year. The predicted values and associated 95% prediction intervals were compared with the observed values to assess the predictive accuracy of the model. Additionally, the food-web dynamics was simulated using only the first-year values as initial conditions. This procedure is fundamentally different from a simple fit to the data, as the observed values from the second year onward are not used in forward predictions. Simulations were run 1,000 times with random process noise added at each time step. Mean values and a 95% confidence interval of the hindcast predictions were computed. To assess the relative contribution of environmental and species interactions in affecting the food-web dynamics, an additional hindcast simulation was performed using a simpler single-species model fitted only to fishing mortalities and biomasses of each individual species separately. Both the sequential refitting and the simulated dynamics demonstrated a distinct ability to “recreate the past” dynamics of cod, herring and sprat (Supplementary Figure S4). The hindcast simulations without accounting for environmental forcing and species interactions, however, did not at all explain nor recreate the past dynamics of the three species, especially in the case of cod and sprat (Supplementary Figure S1). Consequently, the food-web model including both species interactions and climate effects was used in the original publication by Lindegren et al. (2009) and in our bio-economic simulations.
Economic Model
To explore game theoretic scenarios, we investigated two strategic interactions between players, here represented by different fishing fleets (rather than individual vessels) as agents. The first is NC interactions where each fleet take its fishing decision by itself and the second is a fully cooperative (grand coalition: GC) interaction where all fleets cooperate by a binding agreement. Three fishing states, Denmark, Poland, and Sweden were considered where each state has its own pelagic fleet for sprat and herring, as well as a demersal fleet for cod. Hence, a total of six fishing fleets were considered for the models. In the NC games, the six fleets act independently and exploit the sprat, herring, and cod stocks whereas, in the GC game, the fleets act depending on a binding agreement. Additionally, the catch of these three dominant fishing nations amount to 70% of the total catch. Consequently, we focused on these three states as they also historically exploit the resource dominantly.
The economic parameters of the model were obtained from the literature. Following Nieminen et al. (2016), the species’ prices, pi,j, are constant over time and asymmetrical for the countries. Here, i is country and j is the species, discount rates for each country is constant over time, ri, were applied from Nieminen et al. (2016) and ci is the cost parameters (constant over time) for each species. In our model, use of dynamic prices and costs would be useful to evaluate our case study closer to the real-life case; however, such dynamic cost and price taking into account stock size are to our knowledge missing for all nations and species except for the Danish cod fishery (Röckmann et al., 2008). So that, we utilized the constant cost and price parameters over time. In our model, the costs are only depended on fishing mortalities (Table 1). All models were simulated in R Program (R Core Team, 2019).
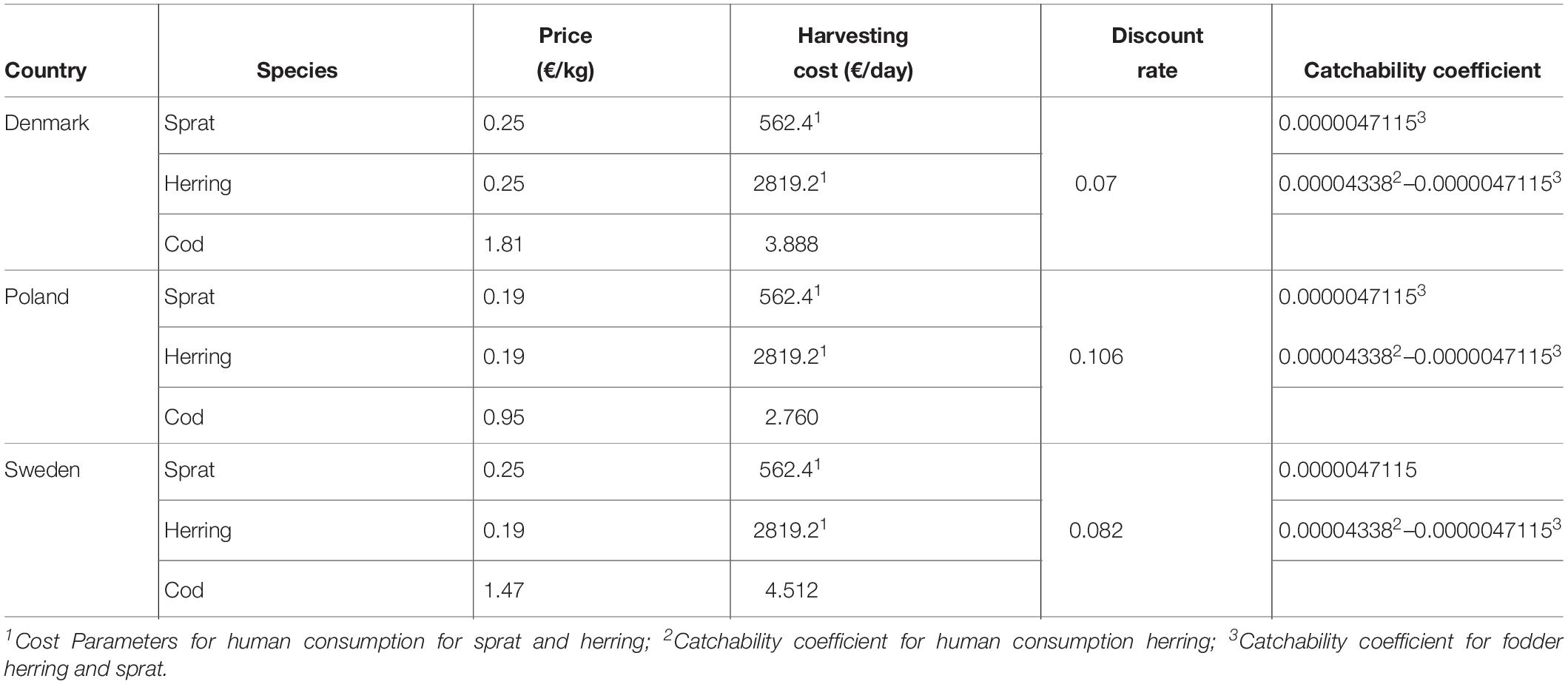
Table 1. The economic parameters used in the coupled bio-economic model in terms of market prices, fishing costs, and catchability coefficients for each country and target species.
Harvest costs were calculated depending on the following equational relationships. Ei,j is the effort in number of fishing days, fi,j is the fishing mortality per fleet per species and qj is the catchability parameter of the species.
Harvest per species and per fleet can be derived by
The cost function can be rewritten as
where ci is the cost parameter for species. Here, the costs are depended on fishing mortalities and cost parameters as well as effort.
In the NC case, each country maximizes its long-term profits independently. Term πi,j denotes the sum of discounted profits of each country i, from each species j across the years t. The countries maximize their economic benefits according to the formula below and, the profit maximization formula was subjected to the population dynamics explained in the biological part of the model above. We used a closed-loop Nash equilibrium where each player can observe the play of the others in the game.
When i denotes the fleets i = 1,3 and j denotes for species j = 1,3 and the objective function of the NC game for each country, i, is
The objective function of the GC is maximizing the joint discounted profit across countries and species as follows:
Climate Scenarios
For both the NC and GC cases, we forced the BALMAR food-web model with three climate scenarios reflecting time periods with different hydrographic conditions highly favorable or unfavorable for recruitment of cod, herring and sprat (Supplementary Figure S2), namely: (i) a BS keeping temperature and salinity at mean historical levels (1975–2010), (ii) a first scenario (S1) keeping temperature and salinity at the mean levels observed prior to the regime shift in the 1980s (1975–1980), a period with low temperatures and high salinities favorable for cod and herring, (iii) a second scenario (S2) keeping temperature and salinity at the mean levels observed after the regime shift (1990–1995), a period with high temperatures and low salinities favoring sprat recruitment. Here, we mainly aimed to see how NC or GC behavior was impacted by the changes in climate variables, temperature and salinity by comparing the pre- and post-regime shift scenario relative to the BS in terms of SSB, yield and revenue. In order to account for ecological uncertainty (arising from the food web model), we performed multiple (N = 100) stochastic simulations for each scenario by introducing multivariate random errors into the food web model for each realization (see Eq. 1). Furthermore, we performed a sensitivity analysis on the economic parameters by varying the discount rates, cost and price for all cases. We decided to leave out some of the results regarding sensitivity tests for the deterministic and stochastic simulations to reduce the length and complexity of the paper. However, we introduced Supplementary Text and figures that show these results (Supplementary Figures S6–S24).
Results
Biological and Harvest Outputs
In the first scenario, sprat SSB dropped dramatically both in the NC and the GC cases whereas, herring and cod stocks were higher compared to the BS (Figure 1). Furthermore, the herring SSB is the only one that was greater in the GC compared to the NC. In the second scenario, sprat SSB was higher for both GC and NC cases while cod and the herring were lower compared to the BS (Figure 1).
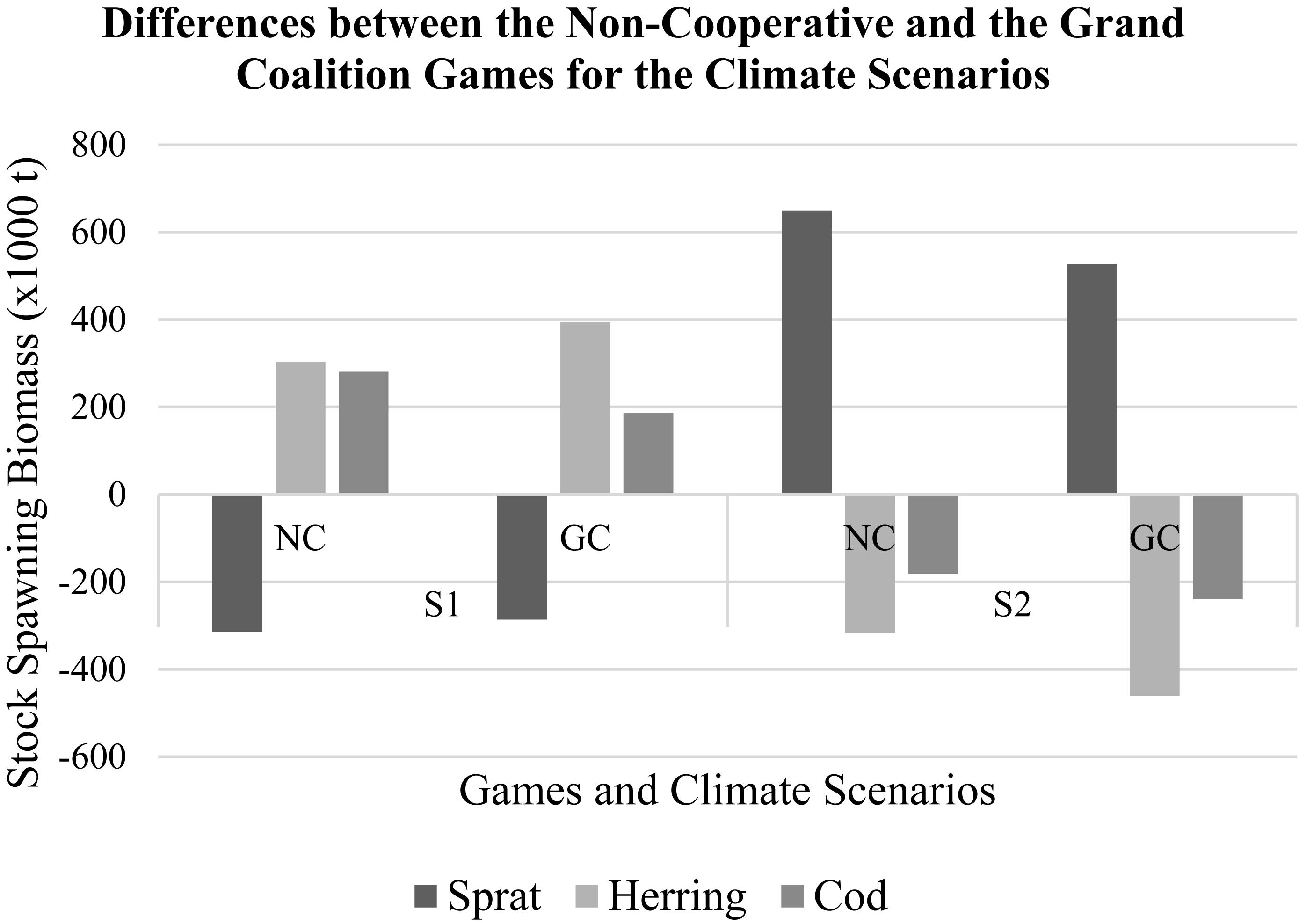
Figure 1. Relative changes in the stock spawning biomasses of the species in the non-cooperative (NC) and the grand coalition (GC) games under each climate scenario [i.e., low temperature/high salinity (S1); high temperature/low salinity (S2)], relative to the base scenario (temperature and salinity at mean observed levels).
In the first scenario, the NC and the GC harvest changes were highest for Poland, while the smallest changes in the NC and the GC were observed for Sweden. In the first scenario, the GC harvests of Denmark and Sweden were higher than their NC harvests, in contrast to Poland that got significantly higher harvest in the GC compared to the NC. In the second scenario, the GC harvest for Poland solely surpassed the NC harvest. Moreover, second scenario GC harvest changes of Denmark and Sweden stayed below the NC harvest (Figure 2).
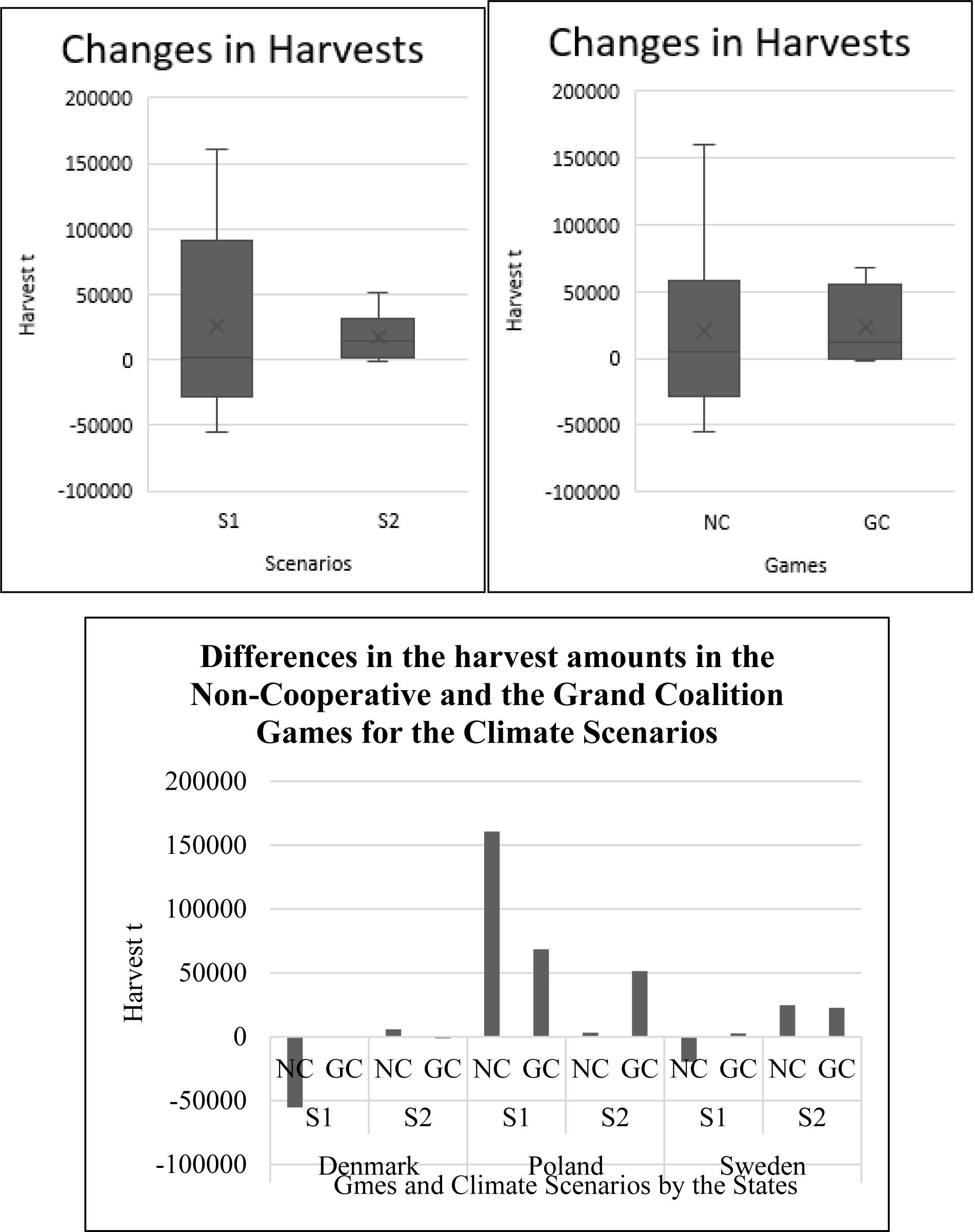
Figure 2. Changes in total harvest of the countries in the non-cooperative (NC) and the grand coalition (GC) games under climate scenarios [scenario 1 (S1): low temperature-high salinity; scenario 2 (S2): high temperature-low salinity].
In the first scenario, sprat harvests declined under the NC and the GC whereas herring and cod harvests increased. The NC sprat and herring harvest changes were less than their GC harvest changes while the GC cod harvest change was considerably higher than the NC cod harvest change. In the second scenario, in general, there were positive change in sprat harvest whereas, herring and cod harvests decreased markedly. Also, as in the first scenario, NC sprat and herring harvest changes were smaller compared to their GC harvest changes (Figure 3 and Supplementary Table S2).
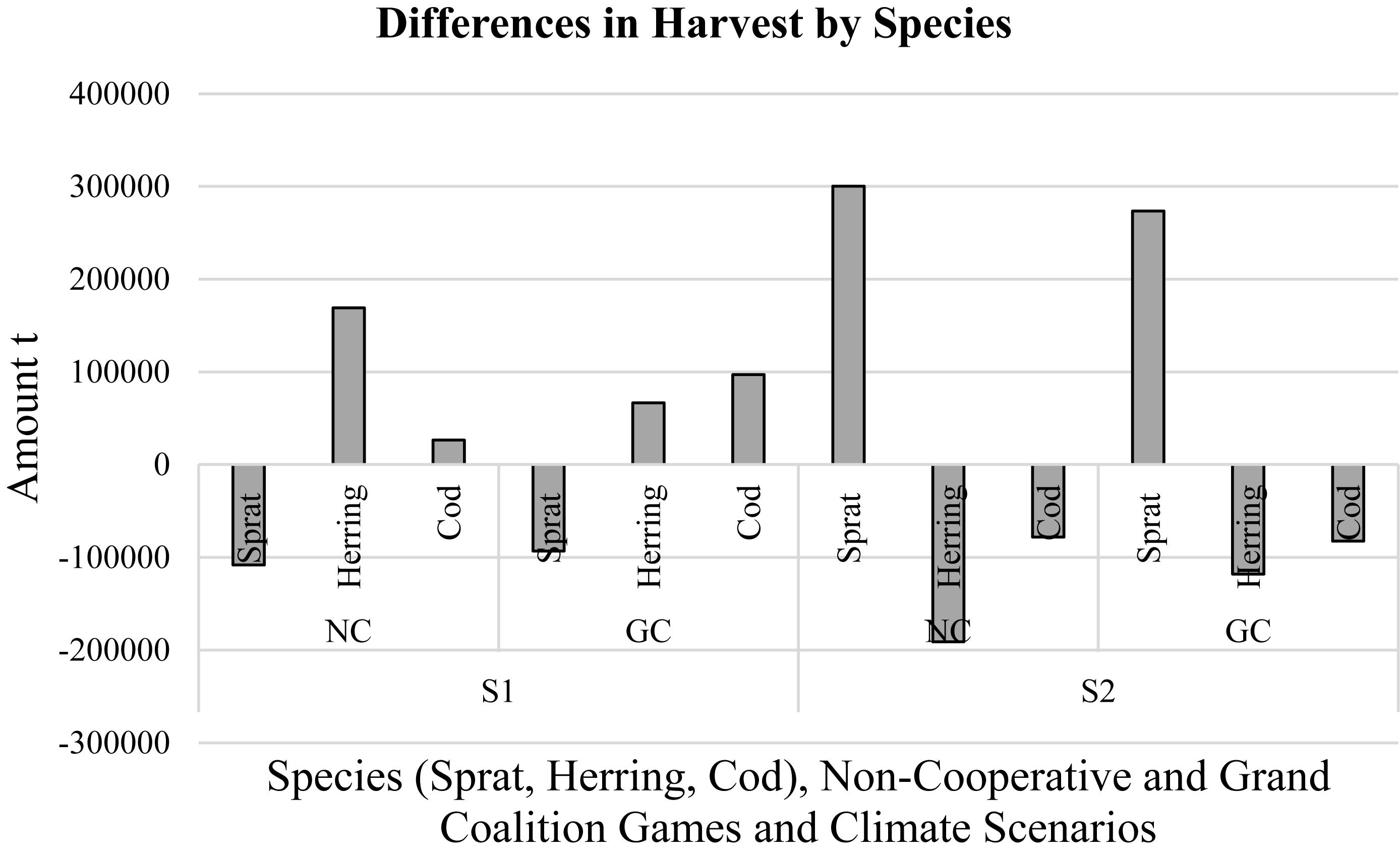
Figure 3. Changes in species’ harvests in the non-cooperative (NC) and the grand coalition (GC) games under climate scenarios [scenario 1 (S1): low temperature-high salinity; scenario 2 (S2): high temperature-low salinity].
Economic Output
The total payoffs of the GC clearly outperformed the NC case across all scenarios (Table 2). Likewise, the payoffs for each country separately were generally higher under GC, except for Poland that showed a slightly higher net present value under NC compare to GC for scenario 1 and 2 (Supplementary Table S1).
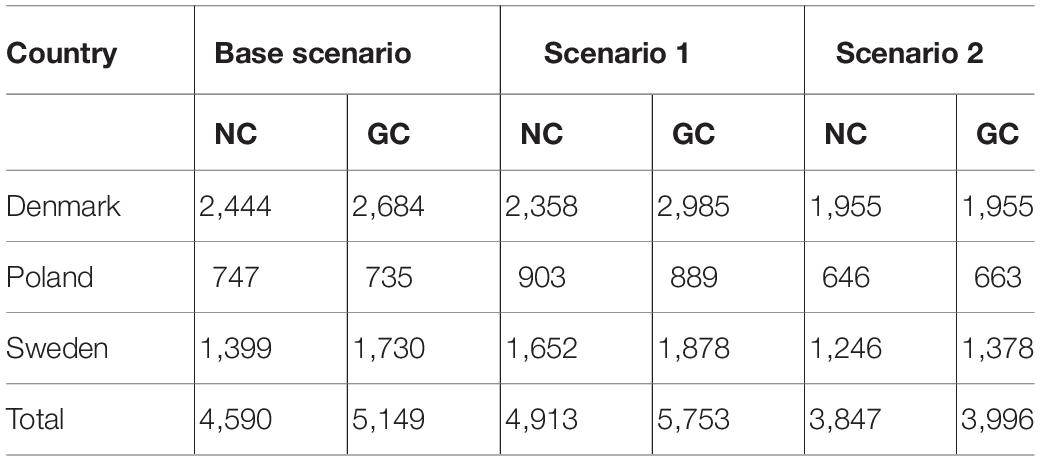
Table 2. Country level aggregated net present values (millions €) for the non-cooperative (NC) and the grand coalition (GC) games under the three climate scenarios considered.
If we compare payoffs between climate scenarios, scenario 1 yielded considerably higher total revenues compared to the BS for both NC and GC, while scenario 2 demonstrated considerably lower payoffs (Figure 4). For scenario 1, the gains were equally distributed between countries, while for scenario 2 Denmark show considerably lower payoffs compared to Poland and Sweden. In the first scenario, the GC solutions outperformed the NC case only for Denmark, whereas Poland and Sweden did not get higher economic benefits by joining the GC. In the second scenario, GC outperformed NC only for Poland while Denmark and Sweden demonstrated lower payoffs under GC compared to the base scenario (Supplementary Figure S5).
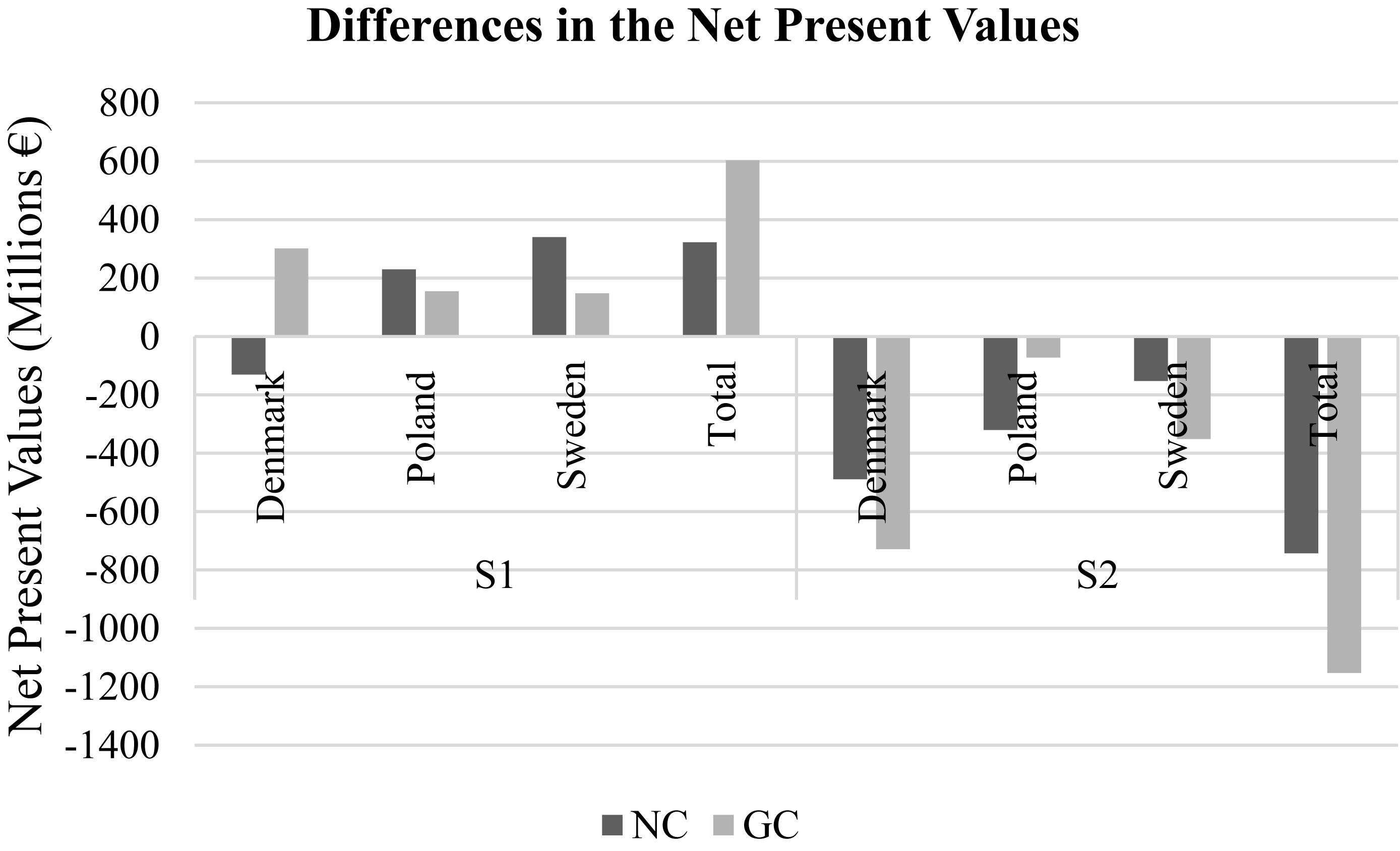
Figure 4. Differences from the base scenario – the aggregated NPVs in the non-cooperative (NC) and the grand coalition (GC) games under climate scenarios [scenario 1 (S1): low temperature-high salinity; scenario 2 (S2): high temperature-low salinity].
Finally, we also assessed the economic performance of NC and GC by sprat-herring and cod fleets separately. For the sprat-herring fleets, the Danish and the Swedish sprat-herring fleets were negatively impacted under the first scenario for both GC and NC whereas the Polish sprat-herring fleet showed almost equal payoffs compared to the BS under GC and NC (Figure 5). In the second scenario, all sprat-herring fleets show higher payoffs compared to the BS. For the Danish and the Swedish sprat-herring fleet NC payoffs were found higher than the GC payoffs in contrast to the Polish sprat-herring fleet. In general, the total payoffs of the GC exceed the total payoffs of the NC in the first scenario whereas, in the second scenario, the total NC payoff was higher than the GC. For the cod fleets, the NC and GC payoffs of the first scenario were higher compared to the BS and the second scenario. In the first scenario, the GC payoff of the Danish cod fleet surpassed the NC payoff whereas the NC payoffs of the Polish and the Swedish cod fleets were higher than the GC payoffs. Lastly, the first scenario total GC payoff was greater than the total NC payoff. In the second scenario, the GC payoffs were greater than the NC payoffs for all countries and the payoffs were significantly lower than the BS.
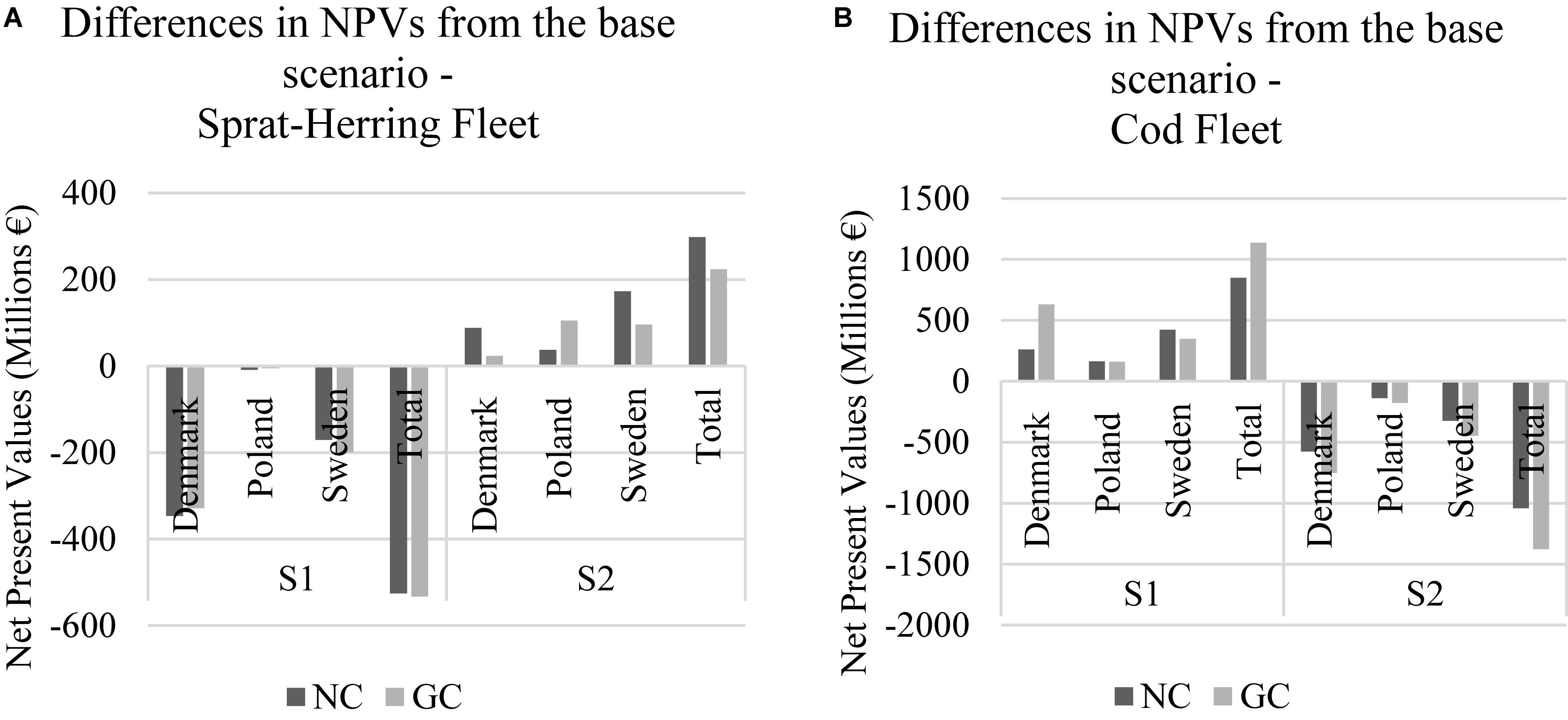
Figure 5. (A,B) Differences from the base scenario- the aggregated NPVs on fleet level in the non-cooperative (NC) and the grand coalition (GC) games under climate scenarios [scenario 1 (S1): low temperature-high salinity; scenario 2 (S2): high temperature-low salinity].
Discussion
In this study, we assessed the NC and GC payoffs for three asymmetric countries that optimize their rents from Baltic Sea cod, sprat and herring fisheries. In general, the GC payoffs were found to be much higher than the NC payoffs. Both in the GC and the NC games, Denmark is apparently the most profitable country, especially regarding the cod fishery. The GC benefits of Denmark is much higher than the benefits of Poland and Sweden and, so that, to provide equal share of the excess benefits in the GC, Denmark would pay compensation to Sweden and Poland as it has the highest profit among countries (Nieminen et al., 2016). Having said that, this would still be an issue of debate as the profits of Sweden is as similar as the profits of Denmark.
The payoffs of all the sprat-herring fleets were highest in the second scenario that is considered as favorable for sprat recruitment due to high temperatures but unfavorable for cod due to the low salinity levels (Lindegren et al., 2009). Hence, this scenario resulted in higher recruitment and survival of the sprat and herring stocks compared to cod and increased economic returns of the sprat-herring fleets. On the contrary, when the temperature is low and the salinity high, as in the first scenario, cod is benefited and the conditions where the GC payoffs are higher than the NC payoffs are better for Denmark and Sweden because of mainly, Danish and Swedish fishery is economically dependent on the cod fishery. These results contrast with previous findings (except for Poland) that showed weakened GC payoffs (Nieminen et al., 2016). Our results are in line with previous finding by Brandt and Kronbak (2010) that suggested lower cooperation or cooperative agreements with the negative impact on the resource rent. As mentioned in Nieminen et al. (2016), the impact of fluctuated cod, herring or sprat recruitment on fishery agreements would be better understood if other species, as well as environmental conditions are considered within the models.
Discount rate sensitivity of the NC model were found to be substantial. Especially, the economic returns of the cod fleets sharply increased with decreasing discount rates. In addition to weighting future payoffs higher, the lower discount rates also favor a more precautionary exploitation level allowing the stock to rebuild to a higher level. This in turn significantly increase long-term yields and reduce operating costs, as the same yield can be achieved with lower effort (Döring and Egelkraut, 2008; Lindegren et al., 2009). In contrast, a 100% increase in the discount rate yielded much lower cod and herring SSBs resulting in a considerably lower total net present value. The GC cases also followed the similar trend in discount rate sensitivity with relatively higher payoffs compared to the NC case (Supplementary Table S3). The sensitivity of the fish prices via 20% decrease or increase in prices resulted in very different economic output, especially for the NC case. Such volatility in payoffs due to changes in fish prices would not be wanted by the fishermen or industry. However, the GC results for the same price sensitivity intervals yielded much more positive output compared to the NC results. So that, the GC case reduced the price volatility compared to the NC case. Likewise, a 20% decrease or increase in costs also disproportionately decreased or increased the final economic output (Supplementary Table S4). But, the changes in the cost parameters did not affect the economic output as much as the prices. Interestingly, in the GC case, the countries, Denmark and Poland, did not get much benefit from the decrease in cost parameters as these countries in the GC were substantially lower compared to the NC case. Having said that, increase in the cost parameters resulted in relatively higher payoffs in the GC compared to the NC (Supplementary Table S5). To summarize, the discount rate, price and cost parameters’ sensitivity did have substantial impact in the economic shares of the NC and GC games. So that, this variation would likely to have additional increase or decreases in compensation amounts that the dominant fishing nation would likely to pay. So that, increase benefits with low discount or cost and high price would not only be good for the dominant fishing nation but also, good for the other fishing nations.
Policy Considerations
The recent reform of the EU-CFP states that “the CFP shall ensure that fishing and aquaculture activities contribute to long-term environmental, economic and social sustainability” (European Council [EC], 2013). Quota allocation schemes, such as TAC are commonly used around the world, including the Baltic Sea. The precautionary principle, that emphasizes the management of fish stocks within safe biological limits, has been the basis of TACs allocations. However, EU has previously been unsuccessful in meeting the precautionary approach, leading to overexploitation of fish stocks, partly driven by overcapacity and poor profitability of the fishing fleets. Consequently, costs and benefits of the fleets should be considered when determining the TAC. TAC allocation would be considered according to the relative stability principle to be accepted by the all member states. A sharing rule would be solution in sharing the resource benefits. In the current study, the GC did not result in positive returns for all the fishing states. For example, Poland (in all scenarios) and Denmark (in scenario 2) received no, little or negative economic outputs from the GC compared to the NC. In this case, the countries that receive positive economic return from the GC would compensate the countries that cannot yield positive economic return. As a solution, Nash Bargaining equal sharing rule could be useful to allocate the payoff increases (Nash, 1953; see e.g., Kaitala and Lindroos, 1998; Li, 1998). The allocation should be based on compensation schemes created collaboratively in which the dominant fishing state would be transferring surplus benefits to states with negative economic returns. Further cooperation among the Baltic Sea states would be inevitable, especially given the forecasted changes in fish stocks expected under climate change (Lindegren et al., 2010; MacKenzie et al., 2012; Bartolino et al., 2014; Blenckner et al., 2015). Costs of measures on the mitigation of the climate change would be provided by the surpass benefits. The area closure can also be substantial tool for the management of the stocks.
Conclusion
The cooperative management once again demonstrated to be fundamental in defining economically optimal use strategies for shared fish resources. In our case, considering the multi-species and multi-fleet nature of the fisheries, the effectiveness of the cooperative approach would be essential in the decision-making process. Furthermore, this effectiveness of the cooperation was not only limited with the existing climate conditions but also under changing climatic conditions that would be mitigated with the cooperative agreements. Finally, it is essential to increase the number of game theoretical studies focusing on the biological and economic externalities under changing environmental conditions.
Author Contributions
ST was the leading author with substantial support from principal investigators. MtL, LR-J, and MkL involved in software coding, editing, and revising the manuscript.
Funding
The MARMAED project has received funding from the European Union’s Horizon 2020 Research and Innovation Program under the Marie Skłodowska-Curie grant agreement no. 675997.
Disclaimer
The results of this publication “Game Theory Applications to the Baltic Sea Fisheries under Climate Change” reflects only the authors’ view and the Commission is not responsible for any use that may be made of the information it contains.
Conflict of Interest
The authors declare that the research was conducted in the absence of any commercial or financial relationships that could be construed as a potential conflict of interest.
Acknowledgments
The authors would like to thank Dr. Emmi Nieminen who provided fundamental insights in programing of the model.
Supplementary Material
The Supplementary Material for this article can be found online at: https://www.frontiersin.org/articles/10.3389/fmars.2019.00622/full#supplementary-material
References
Bailey, M., Sumaila, U. R., and Lindroos, M. (2010). Application of game theory to fisheries over three decades. Fish. Res. 102, 1–8. doi: 10.1016/j.fishres.2009.11.003
Bartolino, V., Margonski, P., Lindegren, M., Linderholm, H. W., Cardinale, M., Rayner, D., et al. (2014). Forecasting fish stock dynamics under climate change: Baltic herring (Clupea harengus) as a case study. Fish. Oceanogr. 23, 258–269. doi: 10.1111/fog.12060
Bjørndal, T., and Lindroos, M. (2012). Cooperative and non-cooperative management of the Northeast Atlantic cod fishery. J. Bioecon. 14, 41–60. doi: 10.1007/s10818-011-9106-0
Blenckner, T., Llope, M., Mollmann, C., Voss, R., Quaas, M. F., Casini, M., et al. (2015). Climate and fishing steer ecosystem regeneration to uncertain economic futures. Proc. R. Soc. B Biol. Sci. 282:20142809. doi: 10.1098/rspb.2014.2809
Brandt, U. S., and Kronbak, L. G. (2010). On the stability of fishery agreements under exogenous change: an example of agreements under climate change. Fish. Res. 101, 11–19. doi: 10.1016/j.fishres.2009.08.012
Cardinale, B. J., Hillebrand, H., Harpole, W. S., Gross, K., and Ptacnik, R. (2009). Separating the influence of resource “availability” from resource “imbalance” on productivity-diversity relationships. Ecol. Lett. 12, 475–487. doi: 10.1111/j.1461-0248.2009.01317.x
Casini, M., Blenckner, T., Mollmann, C., Gardmark, A., Lindegren, M., Llope, M., et al. (2012). Predator transitory spillover induces trophic cascades in ecological sinks. Proc. Natl. Acad. Sci. U.S.A. 109, 8185–8189. doi: 10.1073/pnas.1113286109
Dickmann, M., Möllmann, C., and Voss, R. (2007). Feeding ecology of Central Baltic sprat Sprattus sprattus larvae in relation to zooplankton dynamics: implications for survival. Mar. Ecol. Prog. Ser. 342, 277–289. doi: 10.3354/meps342277
Diekert, F. K., Hjermann, D., Nævdal, E., and Stenseth, N. C. (2010). Non-cooperative exploitation of multi-cohort fisheries-the role of gear selectivity in the North-East Arctic cod fishery. Resour. Energy Econ. 32, 78–92. doi: 10.1016/j.reseneeco.2009.09.002
Döring, R., and Egelkraut, T. M. (2008). Investing in natural capital as management strategy in fisheries: the case of the Baltic Sea cod fishery. Ecol. Econ. 64, 634–642. doi: 10.1016/j.ecolecon.2007.04.008
Eatwell, J., Milgate, M., and Newman, P., eds. (1989). Game Theory. The New Palgrave. New York: W.W. Norton and Compnay, Ltd.
European Council [EC] (2013). Regulation (EU) No 1380/2013 of the European Parliament and of the Council of 11 December 2013 on the Common Fisheries Policy, amending Council Regulations (EC) No 1954/2003 and (EC) No 1224/2009 and repealing Council Regulations (EC) No 2371/2002 and (EC) No 639/2004 and Council Decision 2004/585/EC.
FAO (2016). Food and Agriculture Organization of the United Nations. Rome: The state of world fisheries and aquaculture, 200.
Harvey, A. C. (1989). Forecasting, structural time series models and the Kalman filter. Cambridge: Cambridge University Press.
ICES (1996). Report of the Working Group on Multispecies Assessments of Baltic Fish. Copenhagen: International Council for the Exploration of the Sea.
ICES (2008). Report of the Working Group on Integrated Assessment of the Baltic Sea (WGIAB). CM 2008/BCC:04. Copenhagen: ICES, 145.
Ives, A. R., Dennis, B., Cottingham, K. L., and Carpenter, S. R. (2003). Estimating community stability and ecological interactions from time-series data. Ecol. Monogr. 73, 301–330. doi: 10.1890/0012-9615(2003)073[0301:ECSAEI]2.0.CO;2
Kaitala, V. and Lindroos, M. (1998). Sharing the benefits of cooperation in high seas fisheries: a characteristic function game approach. Nat. Resour. Model. 11, 275–299. doi: 10.1111/j.1939-7445.1998.tb00312.x
Köster, F. W., Hinrichsen, H., Dietrich, S., Maie, S. J., Brian, S. M., Jonna, T., et al. (2003). Recruitment of Baltic cod and sprat stocks: identification of critical life stages and incorporation of environmental variability into stock-recruitment relationships. Sci. Mar. 67, 129–154. doi: 10.3989/scimar.2003.67s1129
Köster, F. W., and Möllmann, C. (2000). Trophodynamic control by clupeid predators on recruitment success in Baltic cod? ICES J. Mar. Sci. 57, 310–323. doi: 10.1006/jmsc.1999.0528
Köster, F. W., Möllmann, C., Hans-Harald, H., Wieland, K., Jonna, T., Kraus, G., et al. (2005). Baltic cod recruitment - the impact of climate variability on key processes. ICES J. Mar. Sci. 62, 1408–1425. doi: 10.1016/j.icesjms.2005.05.004
Kulmala, S., Levontin, P., Lindroos, M., and Pintassilgo, P. (2013). Atlantic salmon fishery in the Baltic Sea – a case of trivial cooperation? Strateg. Behav. Environ. 3, 121–147.
Li, E. (1998). Cooperative high-seas straddling stock agreement as a characteristic function game. Mar. Resource Econ. 13, 247–258. doi: 10.1086/mre.13.4.42629240
Lindegren, M., Möllmann, C., Nielsen, A., Brander, K., MacKenzie, B. R., and Stenseth, N. C. (2010). Ecological forecasting under climate change: the case of Baltic cod. Proc. R. Soc. B Biol. Sci. 277, 2121–2130. doi: 10.1098/rspb.2010.0353
Lindegren, M., Möllmann, C., Nielsen, A., and Stenseth, N. C. (2009). Preventing the collapse of the Baltic cod stock through an ecosystem-based management approach. Proc. Natl. Acad. Sci. U.S.A. 106, 14722–14727. doi: 10.1073/pnas.0906620106
MacKenzie, B. R., and Köster, F. W. (2004). Fish production and climate: Sprat in the Baltic Sea. Ecology 85, 784–794. doi: 10.1890/02-0780
Mackenzie, B. R., Gislason, H., Möllmann, C., and Köster, F. W. (2007). Impact of 21st century climate change on the Baltic Sea fish community and fisheries. Glob. Chang. Biol. 13, 1348–1367. doi: 10.1111/j.1365-2486.2007.01369.x
MacKenzie, B. R., Meier, H. E. M., Lindegren, M., Neuenfeldt, S., Eero, M., Blenckner, T., et al. (2012). Impact of climate change on fish population dynamics in the baltic sea: a dynamical downscaling investigation. AMBIO 41, 626–636. doi: 10.1007/s13280-012-0325-y
Margonski, P., Hansson, S., Tomczak, M. T., and Grzebielec, R. (2010). Climate influence on Baltic cod, sprat, and herring stock-recruitment relationships. Prog. Oceanogr. 87, 277–288. doi: 10.1016/j.pocean.2010.08.003
Markus Meier, H. E., Eilola, K., and Almroth, E. (2011). Climate-related changes in marine ecosystems simulated with a 3-dimensional coupled physical-biogeochemical model of the Baltic sea. Clim. Res. 48, 31–55. doi: 10.3354/cr00968
Meier, H. E. M. (2006). Baltic Sea climate in the late twenty-first century: a dynamical downscaling approach using two global models and two emission scenarios. Clim. Dyn. 27, 39–68. doi: 10.1007/s00382-006-0124-x
Miller, K. A., and Munro, G. R. (2004). Climate and cooperation: a new perspective on the management of shared fish stocks. Mar. Resour. Econ. 19, 367–393. doi: 10.1086/mre.19.3.42629440
Miller, K. A., Munro, G. R., and Mcdorman, T. L. (2001). The 1999 Pacific Salmon agreement: a sustainable solution to the management game? Can. Am. Publ. Policy Occas. Pap. Num. 47:67.
Möllmann, C., Diekmann, R., Müller-karulis, B., Kornilovs, G., Plikshs, M., and Axe, P. (2009). Reorganization of a large marine ecosystem due to atmospheric and anthropogenic pressure: a discontinuous regime shift in the Central Baltic Sea. Glob. Chang. Biol. 15, 1377–1393. doi: 10.1111/j.1365-2486.2008.01814.x
Möllmann, C., Kornilovs, G., Fetter, M., and Köster, F. W. (2005). Climate, zooplankton, and pelagic fish growth in the central Baltic Sea. ICES J. Mar. Sci. 62, 1270–1280. doi: 10.1016/j.icesjms.2005.04.021
Neuenfeldt, S., and Köster, F. W. (2000). Trophodynamic control on recruitment success in Baltic cod: the influence of cannibalism. ICES J. Mar. Sci. 57, 300–309. doi: 10.1006/jmsc.2000.0647
Neumann, T. (2010). Climate-change effects on the Baltic Sea ecosystem: a model study. J. Mar. Syst. 81, 213–224. doi: 10.1016/j.jmarsys.2009.12.001
Neumann, T., Eilola, K., Gustafsson, B., Kuznetsov, I., Meier, H. E. M., and Savchuk, O. P. (2012). Extremes of temperature, oxygen and blooms in the Baltic Sea in a changing climate. AMBIO 41, 574–585. doi: 10.1007/s13280-012-0321-2
Nieminen, E., Grønbæk, L., and Lindroos, M. (2016). International agreements in the multispecies Baltic Sea fisheries. Environ. Resour. Econ. 65, 109–134. doi: 10.1007/s10640-015-9933-x
Nieminen, E., Lindroos, M., and Heikinheimo, O. (2012). Optimal bioeconomic multispecies fisheries management: a Baltic Sea case study. Mar. Resour. Econ. 27, 115–136. doi: 10.5950/0738-1360-27.2.115
Nissling, A. (2004). Effects of temperature on egg and larval survival of cod (Gadus morhua) and sprat (Sprattus sprattus) in the Baltic Sea - implications for stock development. Hydrobiologia 514, 115–123. doi: 10.1007/978-94-017-0920-0_11
Perry, A. J., Paula, J. L., Ellis, J. R., and John, D. R. (2005). Climate change and distribution shifts in marine fishes. Science 308, 1912–1915. doi: 10.1126/science.1111322
Pinsky, M. L., Reygondeau, G., Caddell, R., Palacios-Abrantes, J., Spijkers, J., and Cheung, W. W. L. (2018). Preparing ocean governance for species on the move. Science 360, 1189–1191. doi: 10.1126/science.aat2360
Pinsky, M. L., Worm, B., Fogarty, M. J., Sarmiento, J. L., and Levin, S. A. (2013). Marine taxa track local climate velocities. Science 341, 1239–1242. doi: 10.1126/science.1239352
R Core Team (2019). R: A language and environment for statistical computing. Vienna: R Foundation for Statistical Computing. Available at: http://www.R-project.org/
Röckmann, C., Tol, R. S. J., Schneider, U. A., and St.John, M. A. (2008). Rebuilding the Eastern Baltic cod stock under environmental change (Part II): taking into account the costs of a marine protected area. Nat. Resour. Model. 22, 1–25. doi: 10.1111/j.1939-7445.2008.00027.x
Sissener, E. H., and Bjørndal, T. (2005). Climate change and the migratory pattern for Norwegian spring-spawning herring - implications for management. Mar. Policy 29, 299–309. doi: 10.1016/j.marpol.2004.04.002
Thøgersen, T., Hoff, A., and Frost, H. S. (2015). Climate risk management fisheries management responses to climate change in the Baltic Sea. Clim. Risk Manag. 10, 51–62. doi: 10.1016/j.crm.2015.09.001
Keywords: Baltic Sea, fisheries, game theory, climate change, food web model
Citation: Tunca S, Lindegren M, Ravn-Jonsen L and Lindroos M (2019) Cooperative Fisheries Outperform Non-cooperative Ones in the Baltic Sea Under Different Climate Scenarios. Front. Mar. Sci. 6:622. doi: 10.3389/fmars.2019.00622
Received: 03 December 2018; Accepted: 20 September 2019;
Published: 11 October 2019.
Edited by:
Isaac C. Kaplan, Northwest Fisheries Science Center (NOAA), United StatesReviewed by:
Xiutang Yuan, National Marine Environmental Monitoring Center, ChinaPhilipp Neubauer, Independent Researcher, Wellington, New Zealand
Copyright © 2019 Tunca, Lindegren, Ravn-Jonsen and Lindroos. This is an open-access article distributed under the terms of the Creative Commons Attribution License (CC BY). The use, distribution or reproduction in other forums is permitted, provided the original author(s) and the copyright owner(s) are credited and that the original publication in this journal is cited, in accordance with accepted academic practice. No use, distribution or reproduction is permitted which does not comply with these terms.
*Correspondence: Sezgin Tunca, sezgin.tunca@gmail.com; sezgin.tunca@hotmail.com