- 1Glacier Bay Field Station, National Park Service, Juneau, AK, United States
- 2Southeast Alaska Pilots’ Association, Ketchikan, AK, United States
- 3Glacier Bay National Park and Preserve, National Park Service, Gustavus, AK, United States
- 4QEDA Consulting, LLC, Seattle, WA, United States
The recurrence of lethal ship-whale collisions (‘ship strikes’) has prompted management entities across the globe to seek effective ways for reducing collision risk. Here we describe ‘active whale avoidance’ defined as a mariner making operational decisions to reduce the chance of a collision with a sighted whale. We generated a conceptual model of active whale avoidance and, as a proof of concept, apply data to the model based on observations of humpback whales surfacing in the proximity of large cruise ships, and simulations run in a full-mission bridge simulator and commonly used pilotage software. Application of the model demonstrated that (1) the opportunities for detecting a surfacing whale are often limited and temporary, (2) the cumulative probability of detecting one of the available ‘cues’ of whale’s presence (and direction of travel) decreases with increased ship-to-whale distances, and (3) following detection time delays occur related to avoidance operations. These delays were attributed to the mariner evaluating competing risks (e.g., risk of whale collision vs. risk to human life, the ship, or other aspects of the marine environment), deciding upon an appropriate avoidance action, and achieving a new operational state by the ship once a maneuver is commanded. We thus identify several options for enhancing whale avoidance including training Lookouts to focus search efforts on a ‘Cone of Concern,’ defined here as the area forward of the ship where whales are at risk of collision based on the whale and ship’s transit/swimming speed and direction of travel. Standardizing protocols for rapid communication of relevant sighting information among bridge team members can also increase avoidance by sharing information on the whale that is of sufficient quality to be actionable. We also found that, for marine pilots in Alaska, a slight change in course tends to be preferable to slowing the ship in response to a single sighted whale, owing, in part, to the substantial distance required to achieve an effective speed reduction in a safe manner. However, planned, temporary speed reductions in known areas of whale aggregations, particularly in navigationally constrained areas, provide a greater range of options for avoidance, highlighting the value of real-time sharing of whale sighting data by mariners. Development and application of these concepts in modules in full mission ship simulators can be of significant value in training inexperienced mariners by replicating situations and effective avoidance maneuvers (reducing the need to ‘learn on the water’), helping regulators understand the feasibility of avoidance options, and, identifying priority research threads. We conclude that application of active whale avoidance techniques by large ships is a feasible yet underdeveloped tool for reducing collision risk globally, and highlight the value of local collaboration and integration of ideas across disciplines to finding solutions to mutually desired conservation outcomes.
Introduction
Lethal collisions between large ships and large whales (ship strikes) are a recurring and common threat to whale populations across the globe (Thomas et al., 2016). In some cases, such as with the critically endangered North Atlantic right whales (Fujiwara and Caswell, 2001), and an important sub-population of sperm whales in the Canary Islands (Fais et al., 2016), ship strikes have direct implications for population persistence and biodiversity. In other cases, such as with the population of blue whales in the eastern North Pacific, ship strikes do not appear to regulate population dynamics given the frequency of (known) ship strike mortalities (Monnahan et al., 2015), although the number of detected collisions may be an underestimate of the true number that occur (Rockwood et al., 2017). Regardless, management agencies and the general public value large cetaceans and seek effective ways to reduce ship strikes, even when population persistence is not at stake (Gende et al., 2018).
To date, most management efforts aimed at reducing ship strike risk have focused either on modifying shipping lanes, which can reduce the relative and absolute risk of strikes by reducing spatial and temporal overlap between ships and whales (Knowlton and Brown, 2007; Vanderlaan et al., 2008; van der Hoop et al., 2015), and/or reducing ship speed, which may reduce the probability of a collision (Conn and Silber, 2013) or the likelihood of mortality should a collision occur (Vanderlaan and Taggart, 2007). Yet each of these approaches has limitations. Modifying shipping lanes will only be as effective as the spatial persistence of whale aggregations, can require considerable regulatory effort, or may be impractical in narrow straits or for ships arriving into ports of call (Webb and Gende, 2015; Monnahan et al., 2019). Speed restrictions can generate resistance from the shipping industry owing to economic implications of the additional at-sea time that results from lower speeds, particularly when applied over large areas, which may be one reason voluntary reductions in speeds tend to have low compliance (McKenna et al., 2012). Regardless, whales can be notably unresponsive to approaching ships (Nowacek et al., 2004; McKenna et al., 2015), and thus any action that facilitates the avoidance of whales by mariner training and active avoidance techniques (lowering the reliance on whales to avoid ships) are important to develop.
Here we describe active whale avoidance by mariners aboard large ships which serves as a complementary, but comparatively underexplored, means to reduce whale strike risk. Active whale avoidance is defined here as a mariner making operational decisions, such as a course change or speed reduction, with the goal of reducing the chance of a collision with a sighted whale. Active avoidance differs from more ‘passive’ regulatory approaches in that the risk- reducing action is primarily initiated by the mariner upon sighting of a whale surfacing forward of the ship as opposed, for example, to a ship entering a mandatory speed reduction area which requires a change in operational state independent of whether a whale is present in the area and/or at risk of collision.
Active whale avoidance has been developed and successfully practiced for decades by marine pilots in Alaska (and possibly elsewhere) and is not new in the maritime community. However, a more formal exploration will help clarify (1) the development and application of these techniques by other mariners, (2) the regulatory language that makes implicit or explicit assumptions about a ship’s ability to avoid whales, and (3) important research questions with regard to the efficacy and effectiveness of different maneuvers under varying operational and environmental conditions. For example, the U.S. Code of Federal Regulations (50 CFR §224.103) states that it is illegal to approach [North Atlantic] right whales closer than 500 yards (457 m) with some exceptions for vessels ‘restricted in her ability to maneuver.’ In Alaska, federal regulation dictates that all vessels must operate at a ‘slow, safe speed when near a humpback whale’ (50 CFR §223.214) which assumes that the ship can take proper and effective action to avoid collision when near a humpback whale or that ship operators have advance knowledge of where whales are located. 36 CFR §13.1170 stipulates that a vessel in Glacier Bay inadvertently positioned within 1/4 nautical mile of a whale must “immediately slow the vessel to ten knots or less without shifting into reverse”, and “direct or maintain the vessel on as steady a course as possible away from the whale until at least 1/4 nautical mile of separation is established” – requirements that were largely established pertaining to smaller craft and may be unattainable by large ships.
Understanding the opportunities for, and feasibility of, active whale avoidance also serves to benefit mariners by clarifying conditions and actions that may facilitate effective whale avoidance. For example, large ship operators undergo years of training, including frequent maneuver testing in full-mission bridge simulators, which are often focused on collision avoidance with objects including reefs, shoals, and other vessels. Yet we know of no simulator modules for whale avoidance, which would provide opportunities for mariners to learn from others and test new ideas for maneuvering, particularly if they incorporated state-of-the-science information pertaining to whale behavior.
Finally, clarifying research needs and models derived from active whale avoidance will help scientists prioritize and/or refine existing efforts that will have tangible conservation outcomes and assist mariners in applications of these concepts. For example, a suite of efforts currently exist to facilitate mariners sharing information on whale sightings yet it’s unclear how well these sightings equate to changes in maritime operations and, ultimately, whether certain factors, such as the way the information is transmitted or when its received by the operator, equates to a reduction in ship strikes.
Our goal is to present a conceptual model of active whale avoidance derived by coupling perspectives from biologists, focused on the science of whale behavior, with the expertise of ship operators. To that end, our research team included Alaska marine pilots with over 90 years of combined experience developing and practicing active whale avoidance while piloting large ships. As proof of concept, we collected and applied data to our conceptual model focused on avoidance of humpback whales by large cruise ships transiting waters in Alaska. Data informing our conceptual model originated from (1) a study that has placed observers aboard large cruise ships in Alaska since 2006 focused on quantifying surfacing behavior of humpback whales around the ships and the ability of mariners to detect them; and (2) data collected during trial simulations in a full-mission bridge (ship) simulator to identify and quantify the practices that occur on the ship’s bridge during active whale avoidance. Large ship maneuvering capabilities were further explored using SEAiq, a navigation software commonly used by marine pilots to navigate and assess maneuvering possibilities1. Although our work is focused on a specific type of ship (large cruise ships) and single species of whale (humpback), variations of the components of our conceptual model can be applied to whale avoidance by other types of ships and other types of whales.
We emphasize that our goal is to generate a conceptual foundation upon which specific processes, such as the relationship between whale surfacing distance and appropriate maneuver response, can be subject to more rigorous testing and replication. To that end, our findings (at this stage) are not intended to prescribe what mariners should (or shouldn’t) do when in the vicinity of surfacing whales. Instead, we draw some more general but important inferences from our conceptual model and related data including the role of ship operations (e.g., speed and heading variables) in active whale avoidance. Ultimately we hope these ideas will help advance the development and application of active whale avoidance techniques on a global scale.
Materials and Methods
Our goal for this paper was to present (1) a conceptual model of active whale avoidance, and (2) provide a proof of concept by utilizing empirical data of humpback whale surfacing behavior collected from the bow of cruise ships and from simulations of large cruise ship operations in a full-mission bridge simulator and via commonly used pilotage software. The conceptual model, generated to help deconstruct this complex and highly variable process into components that could be informed by data, was developed during a series of meetings conducted since 2013 between a team of State of Alaska marine pilots from the Southeast Alaska Pilots’ Association (SEAPA), and scientists from Glacier Bay National Park, where ship strike reduction efforts have been implemented and refined since the early 1980s. The conceptual model is presented first (Figure 1) by describing each of the constituent processes, and factors that influence them. Components include availability and detection processes, reflecting how often and how long whales are available to be detected, and the ability of mariners to detect them once available; and command and maneuver processes, reflecting the procedures that occur on the bridge once a whale is detected, and the ability of a ship to achieve a new operational state commanded by the mariner that reduces collision risk. These components are based upon existing literature (e.g., availability and detection processes) and the collective experience of marine pilots (command and maneuver). To that end, the ‘results’ of the conceptual model include narrative describing how and why certain factors are important, particularly as it relates to ship operations and maneuvering, including events that transpire on the ship’s bridge when a whale surfaces and is detected forward of the ship. For our proof of concept, data collection procedures are organized according to the different components of the conceptual model. While more details on the field-based methods can be found elsewhere (see Gende et al., 2011; Harris et al., 2012; Williams et al., 2016) they are described briefly below.
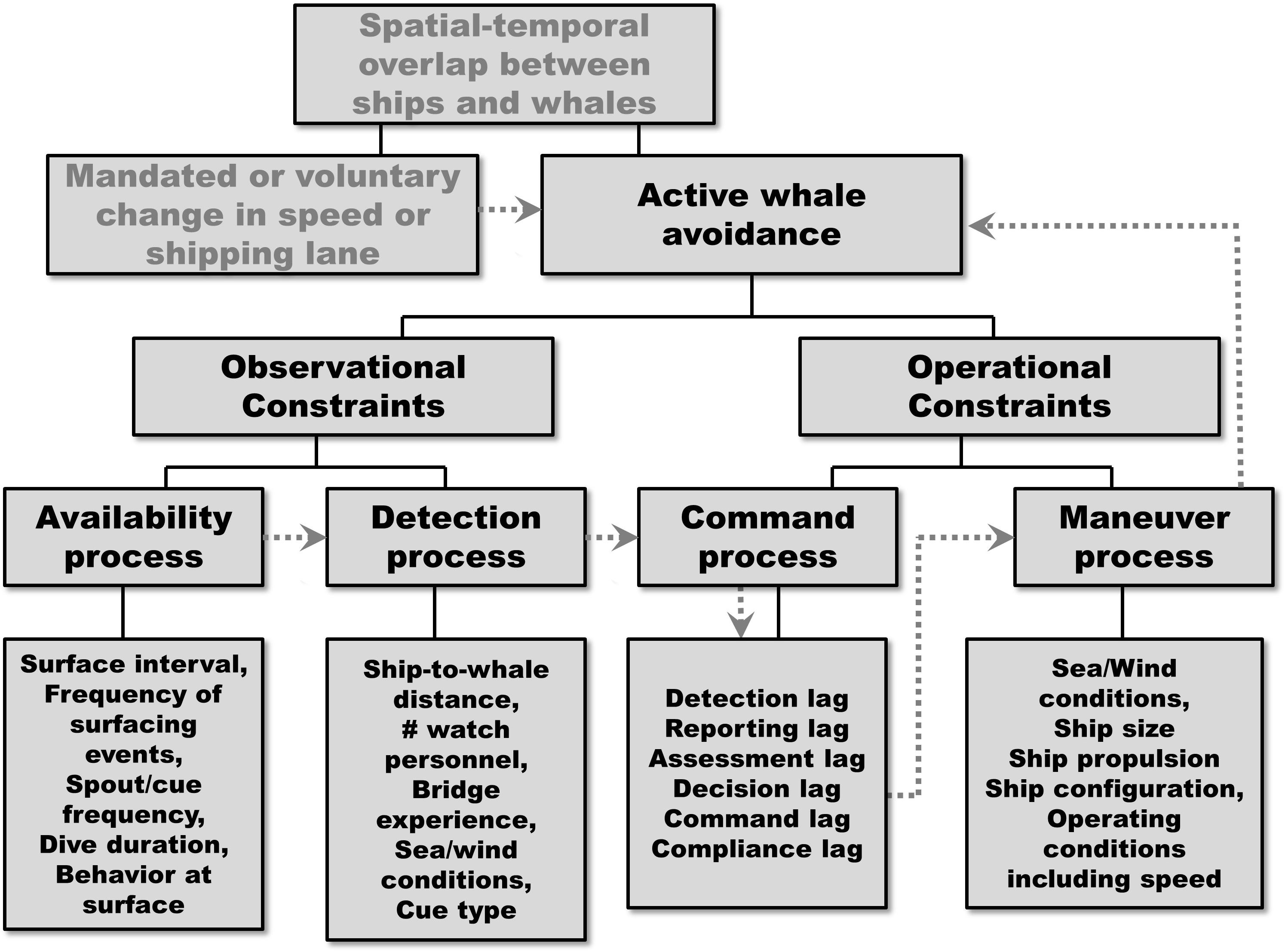
Figure 1. Conceptual model depicting some components related to active whale avoidance by mariners aboard large ships. The first two rows reflect opportunity: active whale avoidance can be practiced anywhere in the world where there is overlap between large ships and large whales, including within management areas that already require (or encourage voluntary) speed reductions or within shipping lanes that have been altered to minimize ship-whale encounters. Lowermost boxes represent a sampling of important factors that may influence their related processes either singly or in concert with other factors. The arrows among the lower boxes reflect the general chronology of whale avoidance.
Availability of Whales for Detection
In the context of active whale avoidance, whales need to be available for detection in order to be avoided. Thus the availability is dictated by the type, frequency, and duration of the opportunities for perception by the mariner. In Alaska, humpback whales (and other whale species) regularly embark on a repeated cycle of a foraging dive punctuated by a surface interval. For clarification we define a surface interval as the time the whale first comes to the surface following a dive to the time it embarks on another dive. Therefore the surface interval encapsulates one to many surfacing events defined as when the whale breaks the surface of the water to respire. Surfacing events are separated by brief submergences (e.g., Dolphin, 1987; Stelle et al., 2008; Godwin et al., 2016; Garcia-Cegarra et al., 2019). During each surfacing event (surfacing) the whale may provide multiple ‘cues’ that can be perceived by the mariner to infer the whale’s distance from the ship and direction of travel (Hiby and Ward, 1986). Cues include spouts/blows/breaths and presentation of the head, dorsal fin, back, or tail (flukes) breaking the surface. Cues are available for only a second or two, occur in rapid succession, and often overlap in time (such as when the water vapor from a spout lingers long enough to be visible when the whale’s flukes break the water’s surface). In contrast, the surfacing events are separated by submergences that may last 20–40 s or more, during which time the ship will move up to several hundred meters closer to the whale (depending upon speed). The change in ship-to-whale distances between cues (within a surfacing event) will thus be inconsequential (meters) whereas the change in distances between surfacing events will be sufficiently large to affect the probability of detection (see below).
To understand the nature by which humpback whales become available to be detected by mariners, we utilized data collected as part of an ongoing study that has placed an observer aboard large cruise ships in Alaska since 2006 (Figure 2A) to estimate (1) the frequency and duration of surfacing events throughout a surfacing interval, and (2) the probability that one or more surfacing events will be detected. We briefly summarize the relevant methods of whale detection here, but reference previously published work (Gende et al., 2011; Harris et al., 2012; Williams et al., 2016) containing more details on data collection and processing protocols.
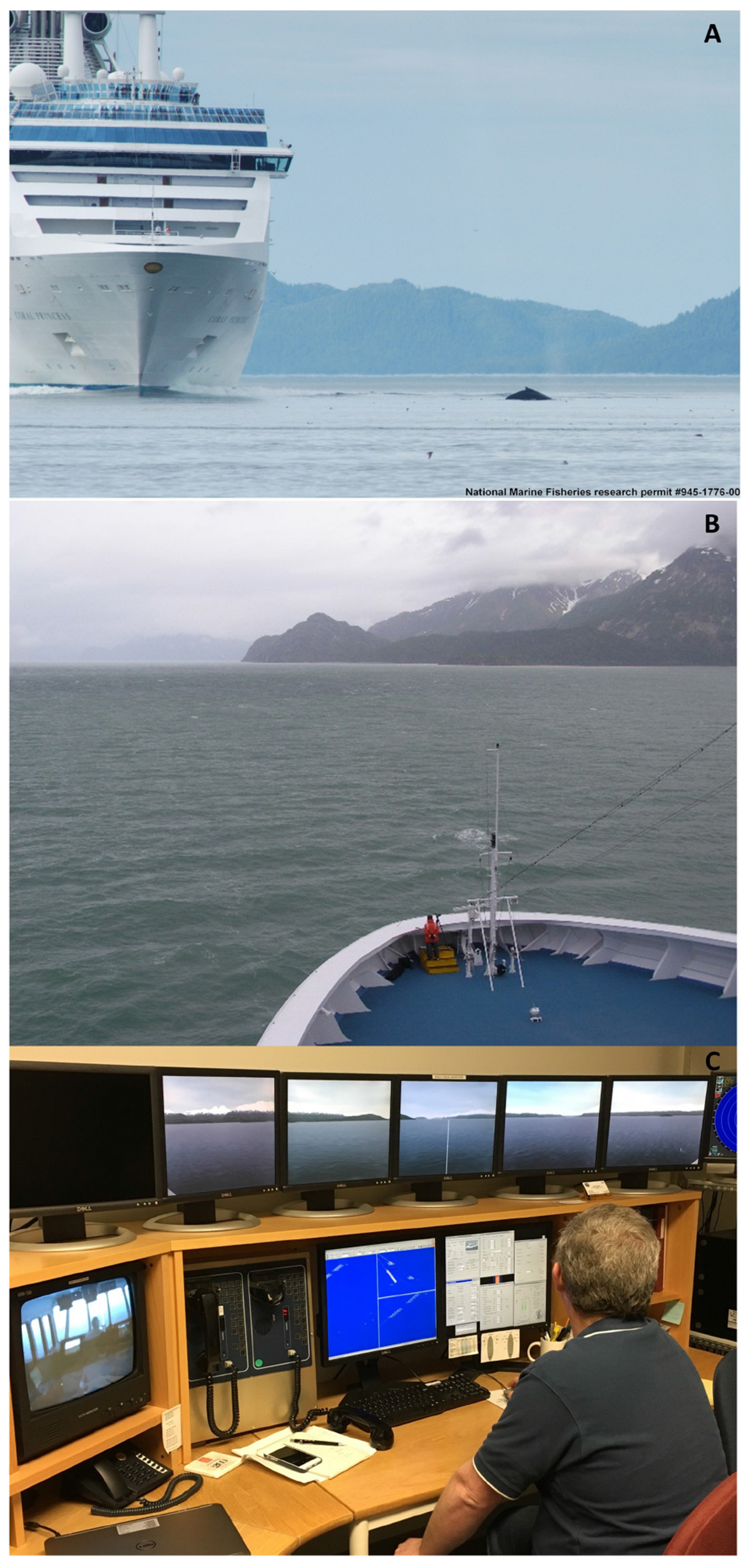
Figure 2. (A) A humpback whale surfaces in front of a large cruise ship, Glacier Bay, Alaska. (B) An observer standing at the bow of a large cruise ship in Alaska quantifying the frequency, proximity, and behavior of humpback whales that surface forward of the ship. The Observer program has occurred since 2006 and included more than 750 cruises. (C) The command center at the AVTEC Full Mission Bridge simulator in Seward, Alaska, during simulations whereby marine pilots simulated whale encounters and active whale avoidance. Closed-circuit video of 2 pilots in the bridge room can be seen in lower left of the photo enacting a whale avoidance maneuver.
Surfacing Behavior of Humpback Whales Near Cruise Ships
During the summers (May–September) of 2016 and 2017 a marine mammal observer embarked on N = 67 large cruise ship cruises (mean length = 268 m; Gende et al., 2018) while the ship transited the waters in Glacier Bay National Park, Alaska. The observer was transported out to the cruise ships just after it entered the park (only 1 or 2 ships entered per day) and boarded the ship via an NPS transport vessel. Regardless of weather, the observer proceeded to the bow (the forward-most point of the ship; Figure 2B) and conducted continuous naked-eye scans of the water in a 180-degree arc from directly forward to directly abeam, on both sides of the ship. Scans were assisted using Swarovski 10 × 42 binoculars and tripod-mounted laser rangefinder binoculars (Leica Viper II; accuracy + 1 m at 1 km; Leica, Charlottesville, VA, United States) to search for whales.
When the observer detected a humpback whale, the ship’s position was recorded using a Global Positioning System (Garmin 76Cx GPS, Olathe, KS, United States), and the distance between the observer and the whale was measured using tripod-mounted laser rangefinder binoculars, or estimated if the observer could not ‘ping’ the whale with the rangefinder. Based on training and testing throughout the study, estimated distances were deemed unbiased, and typically within 10% of the true distances (Williams et al., 2016). The relative bearing of the surfacing event was recorded using a tripod-mounted protractor along with group size, cue type (spout, fluke up, etc.), direction of travel, and sighting conditions (see Williams et al., 2016 for complete list). All data were recorded using a voice-activated recorder and transcribed following each cruise. Data were then summarized using (1) only whales with a group size of 1 (i.e., singletons) to ensure that surfacing events were not mixed in multi-whale groups (singletons constituted 91% of all groups detected in 2016 and 2017), and (2) only from a single surfacing interval per whale to insure independence. Owing to the speed of the ships (typically 14–20 kts; Webb and Gende, 2015), and foraging dives often lasting several minutes or more, only one surfacing interval was typically recorded (>90% of all sightings) before the whale passed abeam. To avoid using surface intervals that were ongoing when the whale passed abeam, the total number of surfacing events per surfacing interval was summarized across all of the surface intervals where whale flukes were displayed as the terminal cue (indicating a deep dive). In contrast, the length of submergences between surfacing events were summarized using all surface intervals, regardless of the nature of the terminal cue. Both of these parameters aid in understanding how many surfacing events are available for mariners to detect and the time elapsed between available events.
Probability of Detecting a Humpback Whale During a Surfacing Interval
Detection functions of humpback whales surfacing near cruise ships have been published previously by Williams et al. (2016) who used distance sampling applied to sighting data collected since 2008. Importantly, unlike some studies focused on estimating abundance of whales where detection functions were derived using line transects, Williams et al. (2016) derived detection functions tailored to the question of whale avoidance by using a series of instantaneous samples as point transects, with the ship-to-whale distances analyzed as radial measures from the bow. Accordingly, the proper interpretation of these detection functions is the instantaneous detection probability of a whale that becomes temporarily available at a specific ship-to-whale radial distance across the 180-degree arc forward of the ship.
In the context of active whale avoidance, the relevant inference is the probability the mariner detects at least one of the available surfacing events in a surfacing interval because whales often engage in multiple surfacing events (per surfacing interval) and mariners generally need only to detect one of the events to begin evaluating whether a whale avoidance maneuver is necessary and feasible. We thus utilized the Williams et al. (2016) estimates to calculate the cumulative probability of detecting one of the events in a series of surfacing events, i.e., the first or second surfacing event in a 2-surfacing interval, the first or second or third surfacing event in a 3-surfacing interval, and so on.
In this regard, the surfacing events are analogous to a series of Bernoulli trials with one of two outcomes (detected, non-detect) each of which are mutually exclusive and complementary. However, it is important to recognize two conditions when estimating cumulative probability of detection. First, once a whale is detected, it doesn’t matter (for detection) how many subsequent trials (surfacings) occur because it only takes one detected surfacing for the mariner to (1) know a whale is present and forward of the ship, and (2) begin to evaluate whether an avoidance maneuver may be necessary, effective, and safe (recognizing that the first detection may be of variable quality and that subsequent surfacings may need to occur to clarify relevant information such as the whale’s direction of travel). We assumed that once the mariner has detected the whale the detection probability for any subsequent surfacing events = 1 owing to the highly concentrated search efforts that ensue in the small area where the whale is likely to resurface.
Thus, if we characterize the two possible outcomes of a surfacing event as D = Detect and N = Non-detect, assume 100% detection probability for any subsequent surfacing event following detection, and that the initial surfacing is the key parameter of interest, the five possible outcomes for detecting at least one surfacing event in the series of (for example) five surfacing events simplifies from:
DDDDD, NDDDD, NNDDD, NNNDD, NNNND
to:
D, ND, NND, NNND, NNNND
Second, and perhaps more importantly, each trial (surfacing) occurs at different distances influencing the distance-specific instantaneous (radial) probability of detection. For example, if a ship is approaching a whale at 19 knots (9.77 m/s) and the time between surfacing events (duration of submergence) is 20 s, the second surfacing event can occur at a ship-to-whale distance of nearly 200 m less than the first surfacing event, the third surfacing event nearly 400 m closer than the first, etc.
To account for these conditions, we utilized the Williams et al. (2016) instantaneous detection probability estimates for the initial surfacing event, and estimated the cumulative probability of detection across the series of N surfacing events by adding the probability of detecting the second surfacing event after the first event went undetected (because if the first was detected, the second is assumed to be detected), and so on. By extension, the cumulative probability of detecting the second surfacing event will always be greater than the instantaneous probability of detecting the event at that distance because it represents the sum of two probabilities. To illustrate, for a 5-surfacing event interval, the cumulative probability of detection was calculated as:
The individual, radial distance-specific detection probabilities were defined using the hazard rate function:
where the scale parameter = e6.73157 and shape parameter = e0.747 is based on excellent sighting conditions (see Table 3 in Williams et al., 2016). R script (R Core Development Team) written for calculating the cumulative detection probabilities across any distance is provided in Supplementary Material.
To illustrate the cumulative chance that a mariner detects a whale that initially surfaces at different distances, we then plotted the cumulative probability of detecting at least one of the surfacing events for a whale engaged in an average surfacing interval of 3 surfacing events each separated by 20 s submergences (from our data below) initially surfacing at distances of 4000, 3000, 2000, or 1000 m from a ship. Note that because the speed of the ship is relevant to the changes in ship-to-whale distances among surfacings, we modeled these probabilities based on a ship traveling 19 knots.
Surfacing, Detection and Avoidance: An Example of a Ship Strike Scenario
The combined variation from ship operations (course, speed, etc.), whale behavior (swim speed, dive duration, surfacing frequency, direction of travel, etc.), and initial whale surfacing location (distance and relative bearing from the ship) produces an extremely large number of scenarios in which a ship strike can occur (final ship-to-whale distance and bearing = 0m). These scenarios range from virtually no opportunities for avoidance, such as when a whale initially surfaces from a dive just a few meters from the bulbous bow, to scenarios where mariners have an opportunity to avoid the whale, such as when it initially surfaces at a distance sufficient to allow the mariner to complete the command and maneuver processes and potentially avoid the whale.
To understand the interplay between ship operational state and whale avoidance, we chose a scenario where the mariner has the opportunity to invoke an avoidance maneuver. For our chosen scenario, we started at the point of collision, i.e., the ship and whale are in the same place and same time (horizontal distance = 0 m, time to collision = 0 s) and worked backward in time based on defined parameters of the whale’s behavior (constant course traveling adjacent from, and directly perpendicular toward, the ship’s path; constant swim speed = 1.23 m/s; Barendse et al., 2010; Kavanagh et al., 2017) and ship’s operational state (constant course; constant speed of either 10 knots – 5.14 m/s – or 19 knots – 9.77 m/s). Thus if the collision occurred at 0 s, at 100 s prior to collision the whale will be 123 m from the point of collision and the ship will be 514 m (slow ship) or 977 m (fast ship) from the point of collision.
Whales, however, may be at the same horizontal location of the ship but owing to their dive behavior may pass safely below the ship (vertical distance > 8m which is the average large cruise ship draft from our study). To account for the vertical movements of whales (surfacing events and dive intervals), we further modeled the whale to surface 3 times during its surfacing intervals (data from this study) with 20 s submergences (this study), followed by a foraging dive of 5.4 min (324 s; a typical dive length for foraging humpback whales in Alaska; Dolphin, 1987). For simplicity, we assumed linear travel even though the whale was diving. Using these parameters we then graphed the ship-to-whale distances and time to collision through two whale surfacing intervals and a foraging dive for mariners approaching a whale that will ultimately be struck on a fast (19 knots) and slow ship (10 knots). To illustrate the trade-off between detection probability and available time for ship personnel to decide on, and achieve, an avoidance maneuver, the cumulative probability of detection for each of the surfacing events were also plotted.
Where Whales Are at Risk: A Mariner’s ‘Cone of Concern’
Our estimates of the cumulative probability of detection represent the probability of detecting at least one of the surfacing events for a whale initially surfacing at different distances within the entire 180-degree arc forward of the ship from beam-to-beam (Williams et al., 2016). However, throughout development of our conceptual model, marine pilots in Alaska noted that when assessing risk in active whale avoidance they often focus search on a narrower area forward of the ship where a whale strike is more probable, which they define as the ‘Cone of Concern.’ This is because the relative bearing of the whale influences risk; a whale initially surfacing directly forward of the ship (relative bearing: 000°) at 3000 m is at a higher risk of a collision than a whale that surfaces an order of magnitude closer (300 m), but directly abeam (relative bearing: 090°) because the closer whale is unable to swim fast enough into the ship’s path to be struck.
We formalize this idea using simple vector analysis and a trigonometric representation of a whale crossing a ship’s path at a 90-degree angle. We contrasted ships traveling at 10 knots (5.14 m/s) and 19 knots (9.77 m/s) with whales swimming at an average speed of 1.23 m/s (2.4 knots) and at fast swimming speeds of 2.46 m/s (4.8 knots) to explore how these parameters influence the size of the Cone of Concern.
Decision-Making During Active Whale Avoidance: Full-Mission Bridge Simulation
A ship’s bridge represents a classic example of a socio-technical work environment because operational tasks, such as changing course or speed, must be achieved by a team requiring joint efforts of ‘human and technological interlocutors’ (Hontvedt, 2015). To that end, full-mission ship simulators are appropriate for understanding the decision-making process by coupling the human element with technology. To better understand the elements of decision-making and time lags related to active whale avoidance, we conducted familiarization and feasibility exercises during 2 days in 2016 using the Kongsberg full-mission bridge simulator (Figure 2C) at the Alaska Vocational Technical Center (AVTEC) in Seward, Alaska2. The full-mission simulator at AVTEC is regularly used for training Alaska’s marine pilots in the maneuvering of large ships as part of (re)certification and continuing education, and mirrors the platforms used by marine pilots at other training centers around the United States.
Seven simulations were conducted whereby a team of two pilots, one serving as the pilot, the other as the helmsman, operated the bridge of a ship, which had operational parameters similar to that of the M/S Diamond Princess, a 115,875 gross tonnage, 288 m cruise ship that is representative of the large cruise ships calling in Alaska during the summer. Also on the bridge was an observer who recorded the time of events including (1) the start of simulation, (2) the first detected surfacing event of a simulated humpback whale spout (the first actual surfacing event – detected or not – was known only to the simulator operator and scenario coordinator who were located in a different room; Figure 2C), (3) the communications that occurred between the pilot and helmsman, (4) when a command was initiated and (5) the end of the simulation, once the ship had passed the whale. Following each simulation, a de-brief discussion was held to review the events and clarify the reasoning related to the decision-making process. During the de-brief, the elapsed time between first detection and the time of the ordered command was quantified, and the common elements related to the decision-making process were identified.
Our simulations were limited in number as was our bridge team size, which would normally include a dedicated Lookout and one or more deck officers. Thus, we did not draw inferences on detection probability from the simulator. Additional limitations existed due to the lack of fidelity of the simulated whale/cues which are the subject of further refinement and improvement. Nevertheless, the descriptive data on time-to-command and archive of commonalities that influenced decision-making were appropriate as full-mission simulations are regularly used to describe processes that occur on the ship’s bridge, and can serve as realistic proxies for evaluating risk and commanding new operational states (Hontvedt, 2015).
Ship Maneuverability During Active Whale Avoidance
Once a decision is made on an appropriate avoidance maneuver (maintaining existing operations may also be an active decision; see section Discussion), the rapidity by which the new operational state is achieved can vary dramatically among ship types (e.g., bulk carriers vs. tankers vs. passenger vessels) and within similar-type ships based on technical features such as hull shape and maneuvering systems (e.g., Yasukawa et al., 2018; Zaky et al., 2018). Further, variation can occur based upon environmental conditions (e.g., Yasukawa et al., 2012; Rameesha and Krishnankutty, 2019) and/or the existing operational state of the ship (wave height, wind, ship’s existing speed, acceleration/deceleration, whether or not the ship is already engaged in a turn, etc.; Chen et al., 2017). Consequently, determining how quickly a ship can achieve an avoidance maneuver is well beyond the scope of this paper (although our simulations were insightful for which factors should be prioritized for further development).
We utilized the navigation software SEAiq, a commonly used platform by pilots across the U.S. for understanding ship maneuverability, and to focus on a simple and achievable question: for a typical large cruise ship traveling at 10 vs. 19 knots, how far in advance must a turn be initiated to achieve a CPA of at least 100 m with a stationary whale while remaining within defined safety parameters? We used a stationary whale because it simplified the vectors and isolated the focus on the maneuvering capacity of the ship. The 100 m CPA was also for simplicity purposes and should be viewed simply as a means to estimate maneuverability, not as a recommended CPA for mariners. We did not introduce confounding factors, instead simplifying the simulation to reflect ‘best case scenarios’ including unlimited visibility, calm water, no wind or current, deep water maneuvering, no other vessel traffic or whales, and the ship was initially traveling in straight line. Our defined safety parameters were guided by our working history of the ship’s safety parameters and a generalized Pilot Card describing the ship’s sensitivity to heading changes (e.g., maximum rate-of-turn; ROT) at varying speeds, as well as limitations in stopping distances. The turn, based on non-emergency safety parameters, conservatively did not exceed a 10-degree rate-of-turn and did not factor in progressively higher rates of turn.
We note that we did not use SEAiq to estimate how much time (and the total distance) it would take for the ship to slow down (e.g., from 19 to 10 knots) because during the full mission bridge simulations, pilots were found to avoid slowing speed in response to a single sighted whale, reflecting their normal practice. During de-briefings it was noted that while a moderate change in heading can be achieved in a relatively short time period (following whale detection), it takes much longer to achieve a moderate change in speed, reducing the effectiveness of speed reduction as a reactive response for whale avoidance, particularly avoidance of a single observed animal. Moreover, pilots never practice ‘crash stops,’ i.e., a rapid stopping of the ship to avoid a collision with a whale owing to the deleterious impacts it could have on the infrastructure of the ship. Instead, to get a general idea regarding how long it takes for a large cruise ship to reduce speed, and the distance covered during that non-emergency transitional state, we reproduce data from Nash (2009) and re-visit the role of speed reduction as a pre-emptive avoidance maneuver in the Section “Discussion.”
Finally, in typical ship operations, while only one person has ultimate ‘command’ authority while on the bridge, the person directing the movement of the vessel may vary depending upon time and duties, and may be the pilot, captain or deck officer. For simplicity, hereafter, we refer to this person collectively as the Person Directing the Movement of the Vessel (PDMV).
Results
Conceptual Model of Active Whale Avoidance by Large Ships
In its simplest terms, the process of active whale avoidance can be described as occurring in five sequential events (1) a whale surfaces somewhere forward of the ship where a collision with the vessel is possible; (2) bridge personnel tasked with ship navigational decisions detect the whale; (3) the PDMV evaluates the situation and decides that an avoidance maneuver is necessary, feasible, and safe; (3) the PDMV decides upon and commands a new operational state such as a change in course, speed or both; and (5) the ship obtains a new operational state resulting in a lower risk of a collision. Our conceptual model (Figure 1) includes Observational (whale surfacing behavior, detection) and Operational processes (commands and maneuvering) that are structured sequentially. Each of these components are described in more detail.
Availability and Detection Process
The first step in this process is dictated by whale behavior because whales need to be available for detection at the surface in order to be avoided. The availability and detection processes have been well studied owing to its relevance for abundance estimation (via distance sampling), and we refer to these studies for describing factors that influence cue frequency and behavior (Hiby and Ward, 1986; Zerbini et al., 2006). Gray, blue, and humpback whales (among many others) regularly embark on a cycle of surface intervals, consisting of several shallow submergences between respiration/surfacing events, punctuated by longer deep dives (e.g., Dolphin, 1987; Godwin et al., 2016; Garcia-Cegarra et al., 2019). Consequently, whales are infrequently but regularly available to be detected. In general the most frequent cue available during a surfacing event takes the form of the appearance of the whale’s body in concert with a vertical spout/blow, which is composed primarily of water vapor, air, and lung mucosa, that may extend to several meters above the water and persist for several seconds.
Command Process
Our conceptual model lists a series of steps that we have termed the Command and Maneuver Processes. The Command Process consists of Detection, Reporting, Assessment, Decision, Command, and Compliance actions best described as time lags because any time that elapses after a whale is detected reduces the ship-to-whale distance (as the ship moves toward the whale in the scenarios modeled) and decreases the options for an avoidance maneuver to occur. The Maneuver Process represents the time it takes for the ship, once commands have been executed, to achieve the desired new operational state. We describe these steps in more detail below.
The Detection Lag represents the time between when a bridge team member detects an object in the water, confirms its identity, and formulates their report to the PDMV. Based on anecdotal observations from marine pilots aboard large cruise ships in Alaska, this lag was estimated to vary from 1–2 s, as when a whale spout is immediately recognizable, or as much 5–10 s if the nature of the perceived object is not readily apparent (e.g., a whale lying motionless on the surface or a floating log?). What’s more, many Lookouts (personnel assigned to view the waters forward of the ship) are trained to simply make a report of an “object in the water,” if they cannot readily identify what it is, and then continue to observe the object to develop clarifying information.
The Reporting Lag represents the time it takes for the person making the observation to vocalize the observation which, from our experience, may vary from 2 to 10 or more seconds depending upon: (1) the volume or quality of the initial sighting information (which may require dialogue with the PDMV); (2) the observer’s ability to articulate the relative bearing, distance, direction of travel, or other relevant information; (3) existing bridge communications; and (4) language or cultural communication issues. For example, the Lookout may spot a whale spout and report, “whale two points to starboard” with no additional information on distance, direction of travel, or speed. At that point, the PDMV will look in the indicated direction and engage the Lookout for information needed to make an assessment which may result in an additional 10–20 s depending upon the length of the submergence between surfacing events or other ongoing action by the PDMV (e.g., communicating on the radio with other traffic, establishing and monitoring navigational parameters, etc.). In the meantime, the initial cue is often no longer available. The elapsed time associated with Detection and Reporting Lags will be minimized if the PDMV makes the observation him/herself (with a high degree of certainty) and immediately articulates the observation to the bridge team. In these instances the total time elapsed may be as short as 5 s, but more frequently it will be closer to 15 s.
The Assessment Lag represents time needed for the PDMV to verify the information and subsequently assess if a collision is possible. In the determination of collision risk, mariners are trained not to make assumptions on the basis of “scanty” information (see US Coast Guard Rule 7 Risk of Collision, International Rules of the Road3), highlighting the need for quality information before taking action. If, for example, direction and travel speed of the whale are not available, the process may cycle back to the Detection Lag, awaiting another surfacing event upon which to formulate an avoidance decision. Consequently, a simple report of a whale at a relative bearing and distance may not provide sufficient information upon which to base an avoidance action, even for a whale sighted directly ahead. Consequently, the Assessment Lag, as with the other lags, may be relatively quick (3–5 s) for the “obvious” situations or it may take longer if inconsistent or incomplete information is reported.
The Decision Lag represents the time needed for the PDMV to consider the available safe avoidance options based on competing risks. The decision by marine pilots (serving in the capacity of PDMV) is founded on the principle of do-no-harm, firstly to people, secondly to the ship, and thirdly to the environment. In practice, this results in a rapid and dynamic calculation of the trade-offs in the risk of whale collision with the risks of harm to people, the ship, or the environment (or some combination such as a collision with another ship, a shoal, or even another whale). Consequently, critical factors in the Decision Lag are based on the situational awareness of the PDMV to the proximity to these hazards and the operational state of the ship; i.e., what is in the realm of possibility based on its speed, sea state, etc. Based on opportunistic assessments, Decision lags can vary from a few to 20 s, based on complexity and competing risks.
The Command and Compliance Lag is the time needed for the PDMV to articulate the avoidance decision into a specific command and for the bridge team to comply. For example, the PDMV may command the Helmsman to initiate a new heading. For some shipping entities, the bridge procedures require ‘closed loop communication’ whereby the command cannot be executed until the initial order is first acknowledged (by the Quartermaster for course changes, or by the deck officer for speed changes), and then confirmed by the PDMV. This lag is generally 5–7 s in situations where all involved understand and are in agreement, but can be longer (upwards of 60 s) if the command is misunderstood, not heard, not acknowledged, or there is disagreement on the appropriate avoidance action.
Maneuver Process
The Maneuver Process is the time it takes for the ship, once commands have been ordered, to achieve the desired operational state. The maneuver process is also best considered in the context of a time lag because the new commanded operational state does not occur instantaneously. The Maneuver Process can vary dramatically among ships although approximate generalizations are appropriate for estimation and/or simulation scenarios. Similar to other large ships, safe maneuvering of large cruise ships encapsulate a range of turning and slowing options based on the interaction between ship type, existing operational state, and environmental conditions. Our experience, based on informal sampling of whale avoidance maneuvers during the past several summers in best-case scenarios, has been that the maneuver process can vary from 25 to 180 s depending upon operating conditions and the type of maneuver ordered.
Proof of Concept: Large Cruise Ships Avoiding Humpback Whales
Availability
The data collected by observers stationed at the bow of cruise ships transiting waters in Alaska demonstrate that humpback whales surfacing around the ships often provide a small but variable number of opportunities for detection. For all surfacing bouts that ended with a fluke-up dive, whales embarked on an average of 2.8 surfacing events per interval (N = 156 unique intervals; range of surfacing events per interval: 1–15; Figure 3A). We again clarify that this average is based on the number of surfacing events per surfacing interval, not the number of cues per surfacing event. Based on the empirical cumulative density function, about 40% of all surfacing intervals included more than three events (Figure 3A). As we only used surface intervals that terminated in a fluke-up dive, the data on surfacing frequency was not ‘right censored’ in that we had confidence that the surface interval did not include unrecorded events that occurred after the fluke-up dive. However, there is a possibility that Observers may have missed a surfacing event (or two) prior to detection (‘left censored’ data) resulting in the true number of events likely being larger.
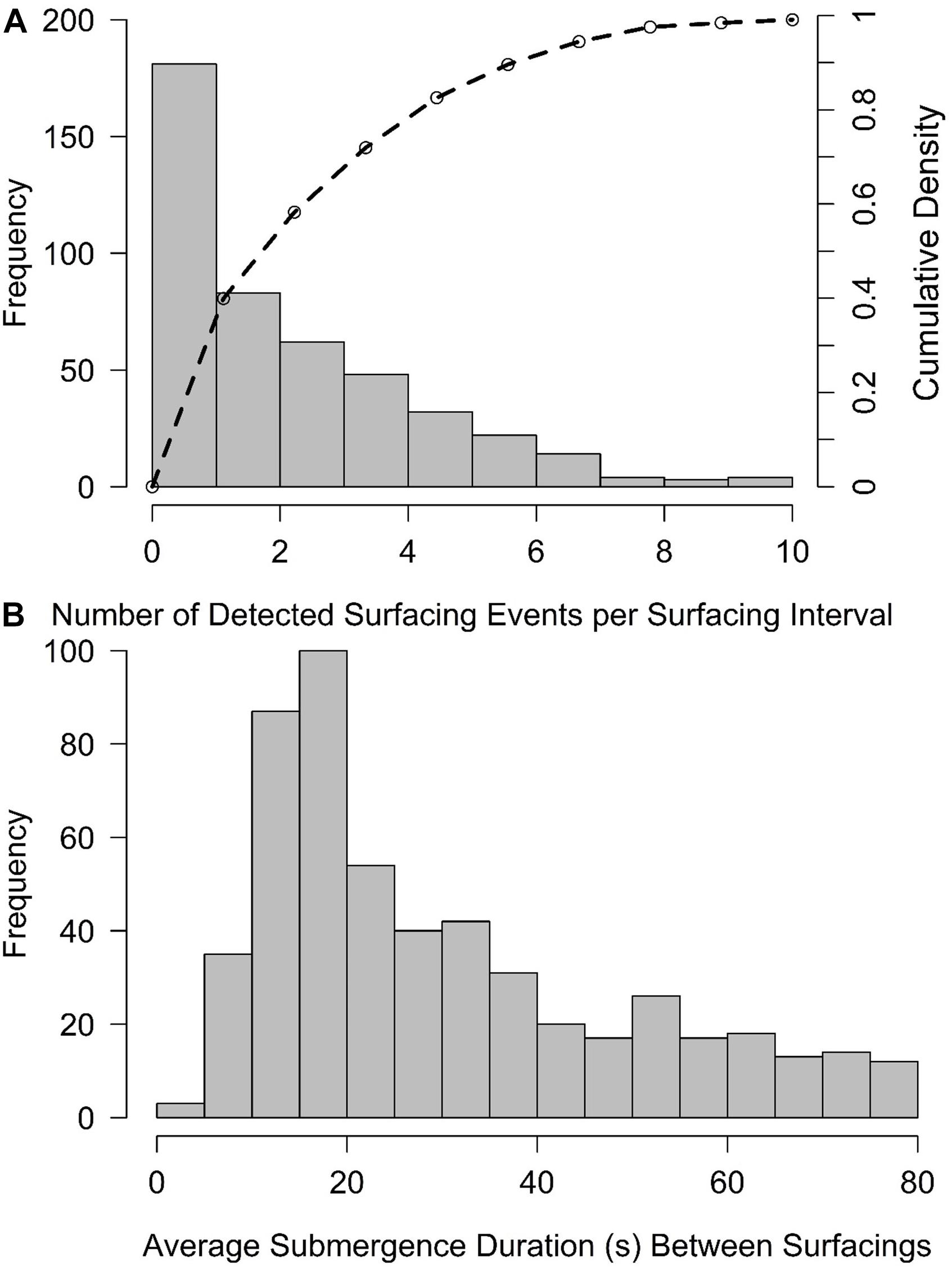
Figure 3. Quantitative descriptions of whale surfacing behavior that help describe the ‘availability’ of humpback whales to be detected by ship personnel engaged in active whale avoidance in Alaska. (A) The average number of detected surfacing events per surfacing interval of humpback whales surfacing near large cruise ships, 2016–2017, with a curve representing the empirical cumulative density function. (B) Histogram of the average elapsed time between available surfacing events (submergence duration) during surfacing intervals of humpback whales surfacing near cruise ships, 2016–2017.
The time elapsed between surfacing events was also variable, although the length of most submergences were centered in groups of 10–15 and 15–20 s (Figure 3B). We feel confident that, once a surfacing event was observed, detection probability of subsequent events was very high as observers (and bridge teams) focus on small area where whales are likely to resurface to gain as much quality information as necessary to evaluate collision risk. Together, the data suggest that mariners engaged in active (humpback) whale avoidance in Alaska generally have about three opportunities for detecting the whale during its surface interval, with an average of around 20 s between events.
Cumulative Probability of Detection
For a surfacing interval that included three surfacing events, the cumulative probability of detecting at least one of the events was lower at larger distances, and increased (non-linearly) with decreasing ship-to-whale distances (Figure 4). For example, based on detection functions for whales surfacing across the 180-degree arc in front of the ship, mariners have a nearly 60% chance of detecting at least one of the three surfacing events for a whale that initially surfaces from a dive 2000 m from the ship, but a less than 15% chance of detection for a whale that initially surfaced 4000 m from the ship (Figure 4). The doubling of the distance resulted in four-fold lowering probability of detection because at larger distances the cumulative increase in detection probability was more linear in nature (e.g., for a surfacing interval that begins at 4000 m) but more exponential in nature for intervals that began at mid distances (e.g., 2000 m). Whales that surface close to the ship (<1000 m) have near certainty of being detected (Figure 4).
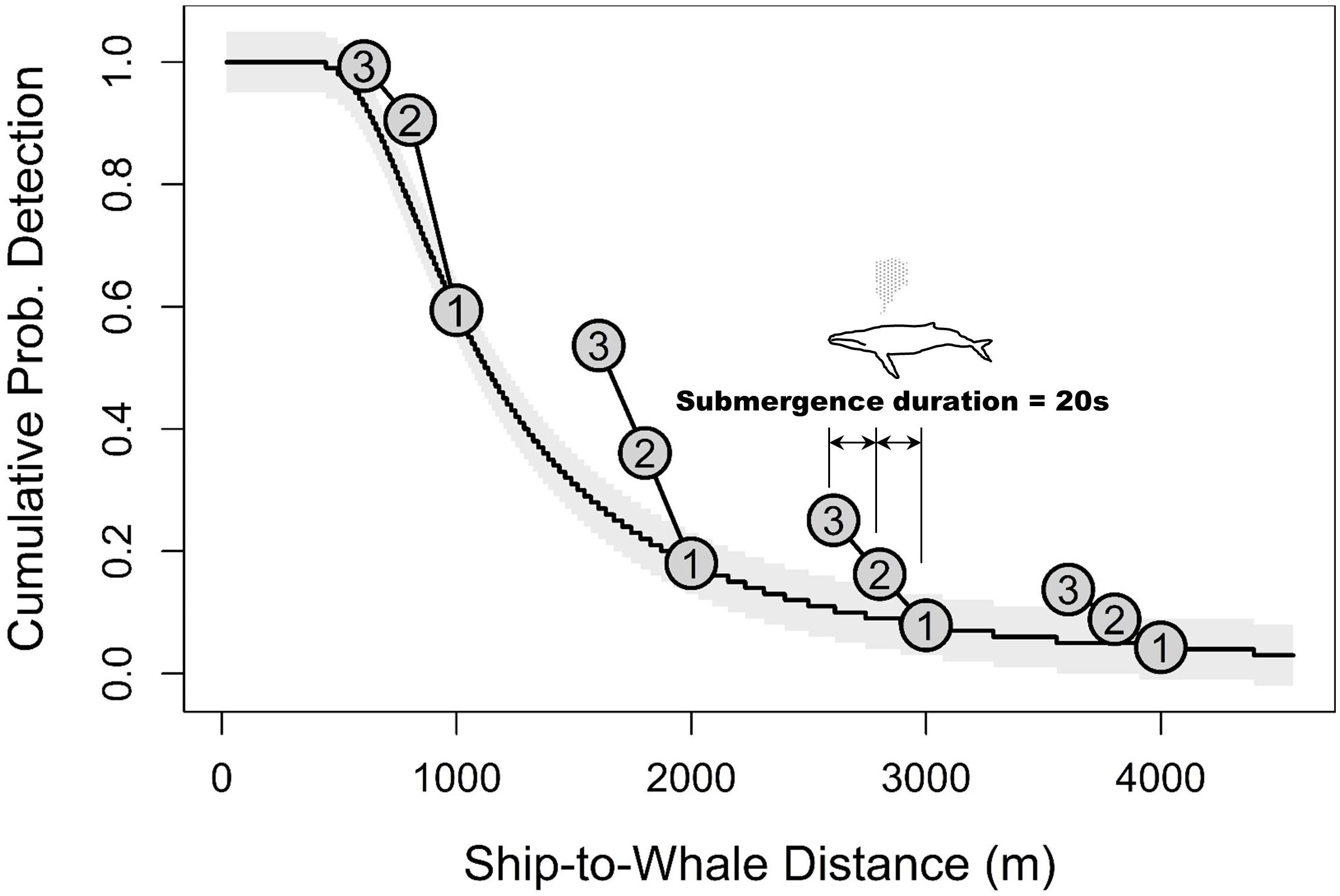
Figure 4. The cumulative probability of detecting at least one of the three surfacing events for 3-event surfacing intervals that began with an initial surfacing event at 4000, 3000, 2000, or 1000 m from a ship. Circles represent the surfacing event number. The detection function used for calculating cumulative probabilities were from Williams et al. (2016) based on excellent sighting conditions.
Surfacing, Detection, and Avoidance: An Example of a Ship Strike Scenario
In our chosen hypothetical ship-strike scenario (ultimate CPA = 0 m) involving ships traveling at different speeds (19 or 10 knots), the whale was struck (PoC; ship-to-whale distance = 0 m, time to collision = 0 s; Figures 5A,B) when it surfaced to take its third respiration during its second surfacing interval (red shaded area). Working backward in time (and space) from the Point of Collision, at 40 s prior to collision the whale surfaced about 211 m from the slower ship and about 394 m from the faster ship. At both those distances, the cumulative probability of detection was near certain (>0.99). Working further backward in time, the whale embarked on a 324 s dive at 364 s prior to collision which placed it over 3500 m from the faster ship but just over 1900 m from the slower ship. At this point, which represents the last chance to detect the whale before it dives, the cumulative probability of having detected at least one of the 3 surfacing events during the first surfacing interval (green shaded area, includes fast ship-to whale distances of 3978, 3781, and 3584 m; slow ship-to-whale distances = 2135, 2029, 1924 m) was approximately 60% for the 10-knot ship but less than 15% for the 19-knot ship (red lines; Figures 5A,B). Owing to the near 4-fold greater (cumulative) probability of detecting at least one of the surfacing events during the first (earlier) surfacing interval, the PDMV aboard the slower ship could have an additional 324 s (post detection and during the whale’s dive) to decide upon and implement an avoidance maneuver.
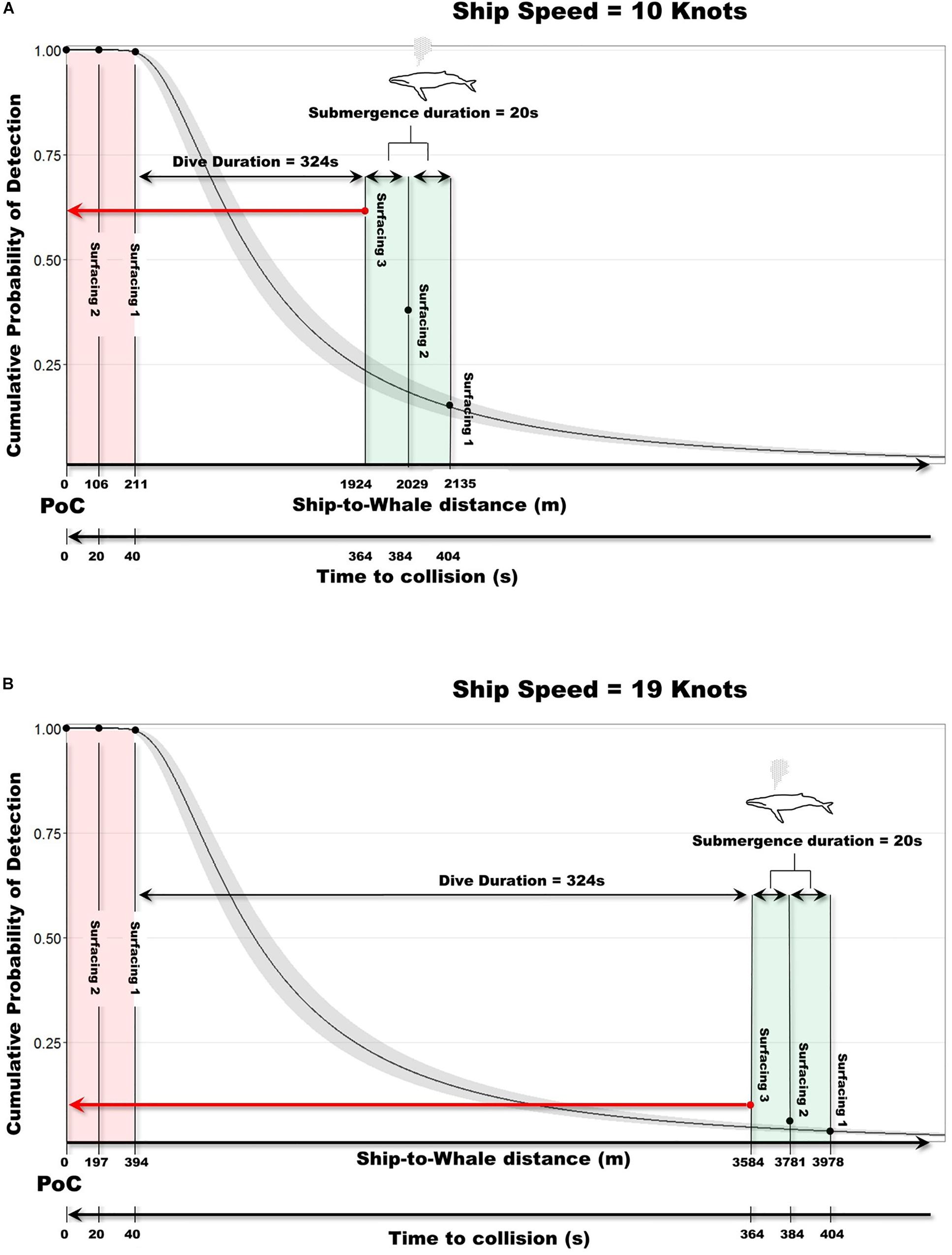
Figure 5. The cumulative probability of detecting the first, second, or third surfacing event of a 3-event surfacing interval of a humpback whale for mariners aboard a 10 knot ship (A) and a 19 knot ship (B) relative to the ship-to-whale distance and corresponding time to collision. In each scenario, the whale behavior is held constant and modeled as traveling at 1.23 m/s (2.3 knots) perpendicular to but toward the path of a ship and, following the initial surfacing interval (green shaded area) and a 5.4 min foraging dive, is struck when it surfaces a third time during the second surfacing interval (red shaded area) at the same location at same time as the ship. Surfacing events are indicated by dots and surfacing intervals by shaded areas. From the first surfacing event (Surfacing 1, green shaded area), the time to collision is held constant at 404 s for each scenario which results in an initial ship-to-whale distance of 2135 m from the slow ship (A) and 3978 m from the faster ship (B). Curved line with gray 95% CI from Williams et al. (2016). A ship strike occurs in both scenarios unless the whale or ship deviates course or speed. Distances are approximately to scale.
Note that, based on our estimates of the command and maneuver lags (see above), both the slow and fast ship would have limited (if any) opportunities to avoid the whale if it went undetected during the first surfacing interval (green shaded areas) because 40 s to collision (when the whale surfaced from its dive) exceeded the aggregate time to implement these processes.
Where Whales Are at Risk: A Mariner’s ‘Cone of Concern’
Figure 6 depicts the results of simple vector analysis demonstrating how a whale’s swimming speed and a ship’s transit speed influences the width of the Cone of Concern. Figures 6A,B depicts ships traveling at 10 knots (5.14 m/s) and 19 knots (9.77 m/s) on a collision course (toward PoC) with a humpback whale swimming at a typical speed (1.23 m/s; 6A) or at a fast swimming speed (2.47 m/s; 6B) perpendicular to, and toward, the ship’s path. For both scenarios, the opposite angle in the right triangle (defined by the ratio of the whale’s swimming speed and the ship’s travel speed) is maximized because the whale is in a ‘crossing’ situation; i.e., it is headed directly toward the ship’s path resulting in the shortest time for potential collision. For the Fast Ship/Typical Whale (6A) scenario, the Cone of Concern would be approximately 14 degrees (7.2 degrees on either side of the ship) and encapsulate a search area of nearly 0.8 km2. For the Slow Ship/Fast Whale scenario, the Cone of Concern has a nearly equal search surface area (0.84 km2) even though the search area is much wider (∼51.2 degrees).
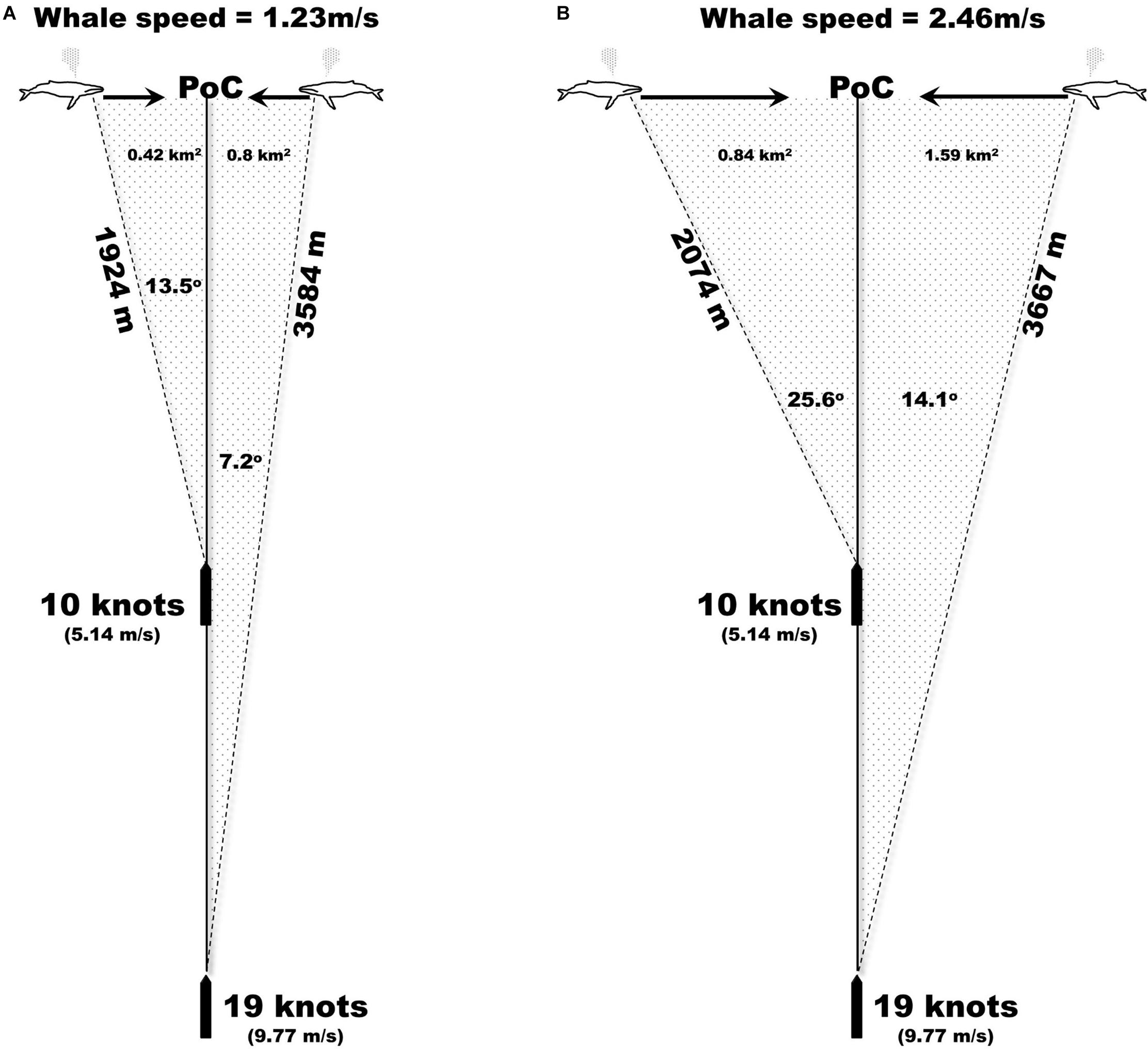
Figure 6. Two scenarios encapsulating different ship transit and whale swimming speeds that define the ‘Cone of Concern’ (shaded area), i.e., the area searched by marine pilots to more efficiently detect surfacing whales that have the potential to be struck by the ship (at the bulbous bow). Figures depict the Cone for a 19-knot ship and 10-knot ship relative to an average swimming speed of a humpback whales (1.23 m/s; A) and a fast swimming whale (2.46 m/s; B) on a crossing pattern and on a collision course with the ship (PoC, Point of Collision; CPA = 0 m). Cones range from 14.4° (7.2° on either side of ship for fast ship and slow whale) to 51.2° (25.6° on either side of ship for a slow ship and fast whales). Distances are approximately to scale to a 284 m ship.
Command Process
As part of the simulations conducted in the full-mission ship bridge simulator at AVTEC, the average elapsed time, resulting from the aggregate of the Command time lags, i.e., from detection of the simulated whale spout to initial compliance with an ordered avoidance action, was 23 s. This compared favorably with the informal observations conducted by several pilots during opportunistic whale avoidance efforts while navigating large cruise ships in 2016 and 2017. During the debriefing meetings, we found that, following initial detection, uncertainty in the whale’s direction of travel and swim speed were common factors that contributed to the delay in a command; the PDMV needed sufficient confidence in the information (making it ‘actionable’), particularly on whether the whale was swimming toward or away from the ship’s heading. Consequently, the PDMV regularly communicated with the Lookout and mate and, absent good information, waited for a subsequent surfacing event before deciding on an appropriate avoidance action.
During post-simulation de-briefs several common themes were discovered. First, marine pilots rarely command a speed reduction in response to a single sighted whale owing to their familiarity with the time it takes to achieve the new speed (Nash, 2009, reproduced in part in Table 1) and that a course change alone is most often more effective and efficient than a potential speed reduction. Second, and perhaps more importantly, the pilot’s maneuvering decisions were ubiquitously based on the evaluation of competing risks. For instance, once the pilots confirmed that a whale was within the Cone of Concern, a primary consideration was how a change in course would influence other risks, such as the risk of collision with other navigation hazards including, but not limited to, other vessels, reefs, or shoals. Likewise, in all simulations where the pilot decided that a course change was needed to reduce collision risk with a whale, an evaluation occurred whereby the efficacy of the course change was considered relative to the time needed to safely ‘build up’ to the required rate-of-turn. The rate-of-turn required to avoid the whale was then considered relative to that particular ship’s safe rate-of-turn guidelines and heel angles to mitigate the risk of deleterious impacts to the vessel and its passengers.
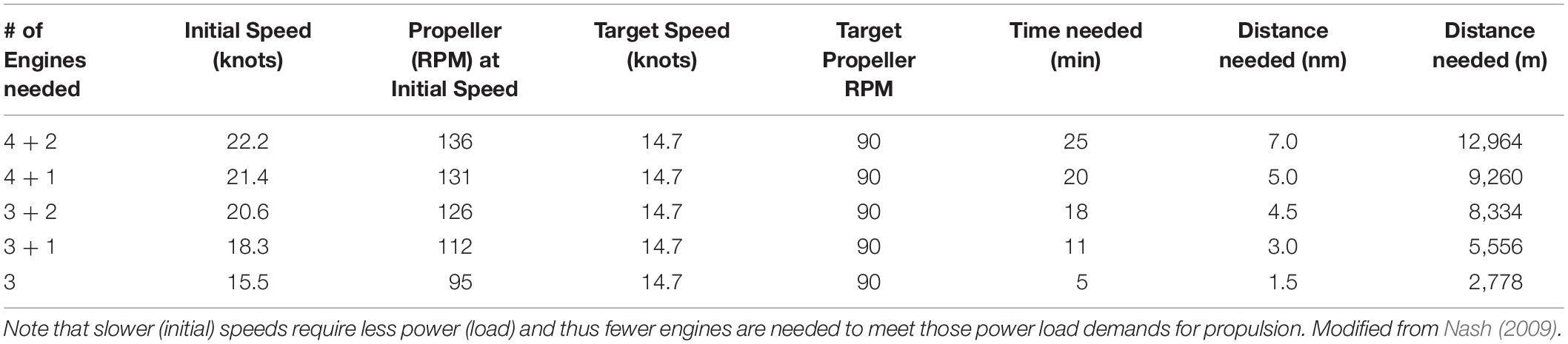
Table 1. Approximate time (min) and distance (nautical miles) needed for slowing a large cruise ship with multiple engine configurations from various initial speeds to an (arbitrary) target speed of 14.7 knots using a safe slowing speed reduction of 2 RPM per minute.
Maneuverability
Using SEAiq and defined safety parameters, we found that mariners aboard a ship traveling 10 knots (5.14 m/s) would require action not less than approximately 741 m from the whale to achieve a ‘near-miss CPA of 100 m’ (Figure 7A). In contrast, a CPA of 100 m aboard the 19 knot ship occurred only after it initiated a turn at least 1121 m from the confirmed sighting (Figure 7B; both scenarios occurred under optimal conditions). In both cases, the Command Lag was modeled as constant (based on results from the simulator), occurring in approximately 25 s, during which time the ship traveled 241 and 130 m closer to the whale for the 19 and 10 knot ship respectively. The Maneuver Lag was achieved over the course of approximately 90 s for the fast ship when it traveled 880 m, and approximately 119 s traveling just over 600 m for the 10 knot ship. Again, this was an abstract, best-case scenario, limiting the Reporting, Assessment and Decision Lags to minimums.
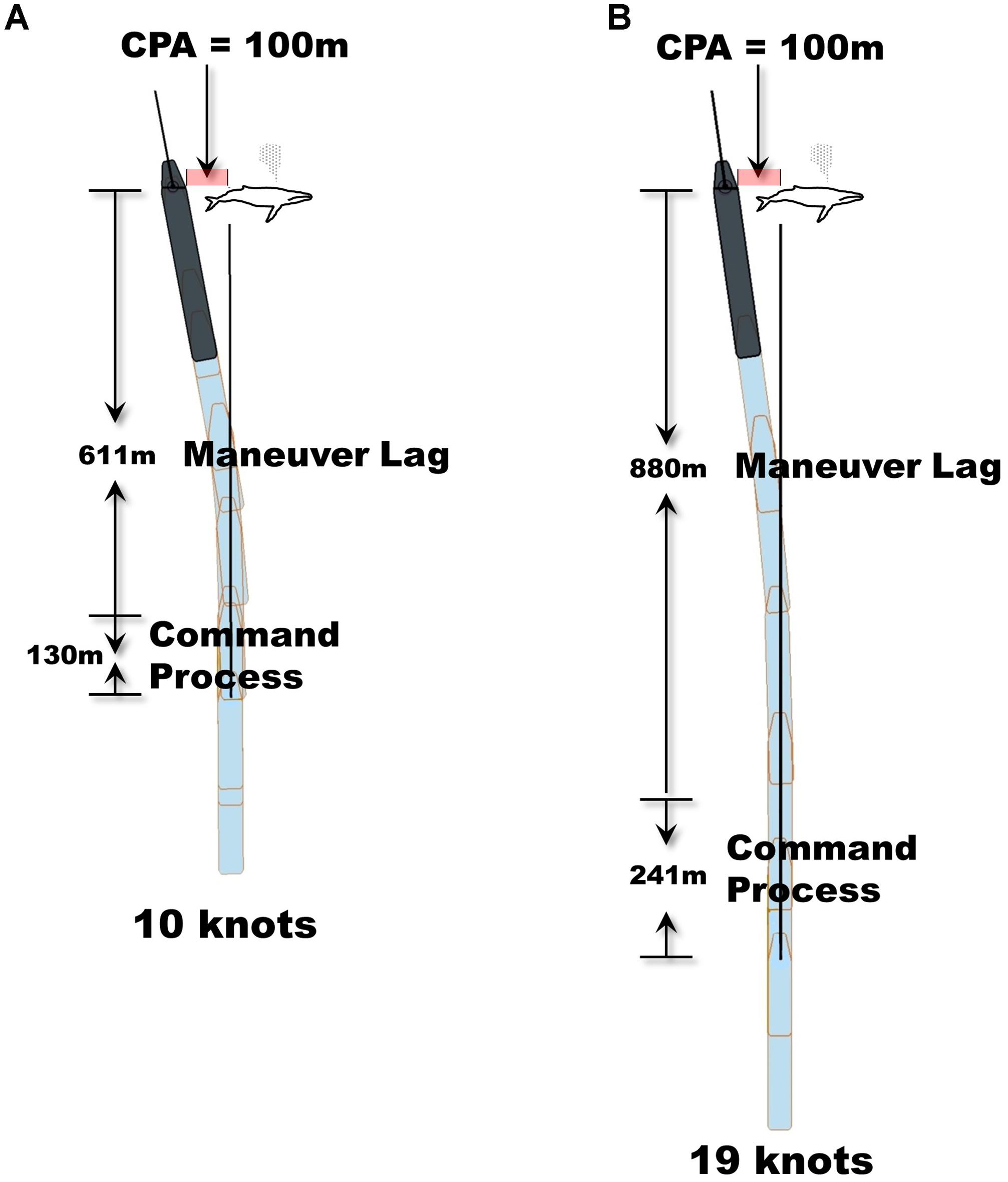
Figure 7. Example of the maneuvering capability of a large cruise ship traveling at 10 knots (A) and 19 knots (B) to achieve a Closest Point of Approach (CPA) of 100 m based on defined environmental conditions and safe turning constraints and assumptions (maximum rate of turn = 10 degrees per minute; see text). These scenarios depict situations that, should a mariner want to achieve a ‘near miss’ of a surfacing humpback whale, the ship would have to begin its turn at 741 and 1121 m away, owing to the constant time lags (the same regardless of ship speed) related to the Command process and maneuvering capability of a large ship. This distance represents a minimum owing to uncertainty in the location, swim speed, and direction of travel for a sighted whale.
Discussion
We coupled whale-surfacing data collected using a ship-based observer with data from simulations in a full-mission ship bridge simulator, opportunistic data collected by marine pilots aboard large cruise ships, and simulations using typical pilotage software to generate the first holistic model of active whale avoidance by large ships. While our goal was to provide a general introduction of the constituent processes such that development and more rigorous testing can build on our efforts, our results provided some insight into the opportunities and constraints for increasing the effectiveness of active whale avoidance and some priority avenues of research, which we discuss below.
Availability, Detection, and a Mariner’s Cone of Concern
Based on data from hundreds of surfacing events of humpback whales by observers, we demonstrate that mariners aboard large ships in Alaska typically have about three opportunities, each separated by about 20 s, to detect the whale and make a decision about whether an avoidance maneuver is necessary, possible, and safe. While these estimates were largely consistent with other studies of humpback whales in Alaska (Dolphin, 1987), we highlight that data on surfacing frequency was not corrected for any negative biases owing to the observer’s chance of missing the initial (or several) surfacing events, particularly at large ship-to-whale distances or limited to the mariner’s Cone-of-Concern. While we initially sought to minimize the chance for this distance bias by using only information from whales surfacing close to the ship, ultimately we decided against subsetting the data (1) because surfacing intervals that began close to the ship were often still continuing when the ship passed abeam (when observers terminated their observations of that whale) which would also underestimate the number of surfacing events per interval, and (2) to avoid biasing the inferences if, in fact, whales alter their surfacing behavior as a function of distance.
Recognizing these biases, however, helps identify possible ways in which the effectiveness of whale avoidance can be increased. For example, a key finding of our conceptual model is that processes that occur on the ship’s bridge such as reporting a whale sighting, assessment of the risk, and compliance to commands, couple with maneuvering constraints to produce a variable, yet important time lag between detection and achieving a new operational state (that reduces collision risk). This aggregate lag contributes to the inverse relationship between time available to make an avoidance maneuver and the range of maneuver options available. Any activity or operation that increases the chance of detection when a whale is first available to be detected thus increases the options for avoiding the whale and the odds of successful avoidance. We identified three factors that may help PDMVs detect a whale and obtain sufficient information to actively avoid it.
First, marine pilots in Alaska, based on decades of experience encountering and avoiding (primarily humpback) whales surfacing near large cruise ships, have developed a searching pattern ‘Cone of Concern’ based on familiarity with approximate travel speeds of humpback whales relative to the ships’ transit speeds. In doing so, pilots and other bridge personnel narrow their search efforts (by over 80% based on our simple vectors and geometry; Figure 6) by delineating the ‘population’ of surfacing whales at risk of collision vs. those that are not. This practice could easily be standardized by integrating the concept into transit planning and/or regular communications with the bridge team to focus on parameters of, and need to search within, the Cone of Concern.
Second, assigning a designated Lookout tasked solely with searching for whales in the Cone of Concern could also enhance detection probability and thus opportunities for whale avoidance. While we did not test whether different configurations of personnel (pilot, pilot + designated observer, etc.) produced different detection functions, experiments aboard large fast ferries have demonstrated that a dedicated whale ‘spotter’ vastly improved detection probability and the distance at which whales were detected (Weinrich et al., 2009). Research based on line transect theory and distance sampling also demonstrate that detection probability increases when additional observers are utilized (Schmidt et al., 2017) including with whales (Zerbini et al., 2006). While we recognize that transiting at night or in heavy seas may reduce or eliminate detection, we also highlight that technology continues to reduce barriers to detection in some of these conditions (Zitterbart et al., 2013) and application of the Cone of Concern may help inform development of the technology to maximize effectiveness.
The third potential way to facilitate the effectiveness of active whale avoidance is by reducing the time identified in the Command process. Pilots in Alaska are regularly conveyed unnecessary or incomplete information by members of the bridge team following a whale sighting. If the information is incomplete, the PDMV may have to wait for another surfacing event before having information of sufficient quality to be ‘actionable.’ This may equate to the ship traveling several hundred meters closer to the whale (based on average submergence data and typical transit speeds in Alaska) before the PDMV can confirm the whale’s location and direction of travel. Training bridge personnel with regards to what information is desirable and protocols for communicating that information (e.g., ‘whale approximately 2000 m three points to starboard, moving away from the ship’) can make a significant difference in time available for PDMV to assess the situation and implement the maneuver without further increasing the risk of harm to the people, the ship, or other components of the marine environment. A simple suggestion of utilizing the same training used for reporting of a man-overboard to continuously point to the person (whale) promotes the effectiveness of the PDMV’s detection and decision process significantly.
Ship Speed
Throughout our effort we consistently contrasted scenarios involving fast (19 knots) and slow ships (10 knots) to explore how speed may influence the constituent processes in active avoidance of a single whale surfacing near the ship. While our objectives were not to rigorously test the role of ship speed in these processes, nor were they to identify an optimal speed that balances whale avoidance vs. transit efficiency (should one exist), we highlight some insights based on our results that warrant discussion and further development.
First, when simulations of whale encounters were conducted in the full-mission ship simulator, pilots never attempted to slow the ship in response to the sighted whale, instead preferring slight changes in course. In the de-briefs that followed, pilots communicated that, while change in speed may influence the dynamics of a whale – ship encounter, the distance necessary to slow the ship to speeds necessary for effective avoidance based on speed change alone (mariner body of knowledge relative to vessel avoidance actions) tended to exceed the sighted distance to the whale owing to potential unsafe results of rapid speed changes. Given the absence of this response, we did not produce simulations that contrasted the efficacy of slowing the ship vs. slight course changes, instead reproducing some recommendations from Nash (2009) simply to provide context with regards to the magnitude of space/distance needed to slow (and recognizing that the target speeds listed in Nash were arbitrary relative to whale avoidance). However we feel a brief description as to why rapid changes in speed are not regularly practiced is necessary owing to its prominent relevance in ship strike dynamics and context for understanding the estimates in Table 1.
For large cruise ships (and likely other large ships) power management plays a major role in operational decision-making (e.g., Ancona et al., 2018), not just in the context of managing fuel costs and optimal fuel efficiency (and resulting levels of air pollution; Khan et al., 2012), but also for safety reasons. For large cruise ships, power needs are met using multiple engines that are variably configured for two different power loads including the propulsion load, which is typically about 80% of total load while transiting, and the hotel load, which is the electrical energy needed to power the ship’s lights, heating/air conditioning, galley, etc. Rapid changes in power use can negatively affect emissions, damage the generators (engines) and, in a worst-case scenario, cause a ‘blackout’ (total loss of power). To help guard against these negative outcomes, large cruise ships typically have some form of power management system, such as a ‘Load Control Program’ that limits dramatic fluctuations in power use. Given that propulsion is the primary power requirement, and that propulsion is a function of the propeller’s rotations per minute (RPM), as a general rule, when a large cruise ship is in Load Control the propeller RPMs are generally not reduced by more than 2–3 RPMs per minute. Consequently, gradual changes in speed represent best load management practices and the gradual change may not meet the more immediate change in operational state necessary for avoiding a whale.
Pre-emptive (planned) reductions in speed are, however, regularly used by pilots in Alaska as a strike risk reduction strategy. Pre-emptive speed reductions are those initiated in anticipation of, rather than in response to, a whale aggregation, and are utilized in two general scenarios. The first is when mariners are informed of a whale aggregation recently detected along the ship’s route and communicated to the ship personnel. The second general scenario is when the ship is approaching a narrow navigational area that also historically has supported whale aggregations. For example, with the cooperation of cruise ship Masters, pilots regularly slow cruise ships to 14 knots in Snow Passage, Alaska, because avoidance options are limited and the area is often characterized by small to large whale aggregations. Pilots have found that these pre-emptive speed reductions tend to produce less resistance from other bridge personnel when (1) they can be accounted for in transit planning and (2) they do not adversely affect port arrival times.
We thus encourage continued development of software applications4 ,5 in which mariners participate in a sighting network that helps inform others vessels that whales have been detected in their area. The type of information conveyed, its timeliness, and receiving platform is, however, critical for its utility. Receiving information via a mobile application (often with sporadic cell coverage) is a more cumbersome means than, for example, a ship’s Electronic Chart Display and Information System (ECDIS) which could overlay historical (e.g., weekly) and recent (e.g., <2 h) whale sightings to assist with transit planning. Recently, the programmer for Whale Alert and the developer for SEAiq coordinated to provide the ability to import weekly whale sighting information automatically for display on the electronic chart.
Our results also demonstrate how, in some scenarios, slower ships may have increased opportunities for whale avoidance acting through both the Maneuver and Detection processes. Faster ships, by definition, travel further distances compared to slower ships during set time periods, such as when whales are submerged between surfacing events (averaged 20 s in our study), on deep dives (324 s modeled based on literature), or during time lags related to decision-making and communications on the bridge following detection. For example, if the Command processes takes the same amount of time on fast and slow ships, and total time elapsed following detection to the point the ship begins to change course is approximately 115 s (Figure 7), we demonstrate that the faster ship achieving a ‘near miss’ of 100 m from a whale would need to detect the whale over 1100 m from the Point of Collision as opposed to just over 700 m for a slow ship, simply because the faster ship moves further over the same time period given the conservative safe maneuvering limitations imposed on the initial test scenarios. An alternative way of interpreting those results is that, had the slow ship and fast ship begun the Command and Maneuver process at the same distance from the whale (as opposed to the same time), the slower ship could have achieved a greater CPA because it would have had a longer time period to continue its turn.
Ship speed can also influence whale avoidance by influencing detection probability. To be clear our results do not indicate that mariners on slower ships are able to detect whales any better compared to mariners on faster ships – there is no logical reason why detection probability would differ for a surfacing whale at a set distance (e.g., 2000 m from the ship) for mariners aboard a fast or slow moving ship. However, if we held the time to a collision constant, as in the scenario in Figure 5, then, by definition, the faster ship will be further from the point of collision than a slower ship at the same time to collision. Thus, a surfacing event critical for detection would occur closer to the slower ship influencing the cumulative probability of detection, providing more time for a maneuver.
Future Research and Training
The conceptual model of active whale avoidance was derived primarily from the collective experience of pilots in Southeast Alaska who have ‘learned by doing,’ which has required significant time on the water. We thus submit a number of ideas for priority research and training development to hasten the adoption, applicability, and effectiveness of active whale avoidance.
First, ship personnel need sufficient time to make a decision related to an avoidance maneuver and achieve a new operational state, assuming one is commanded, that reduces collision risk. For example, marine pilots in Alaska are often challenged by predicting where a whale is likely to surface following its dive because, if they waited for the whale to resurface before initiating a maneuver, the options for avoidance would be significantly reduced. During simulation de-briefs pilots communicated that they will, at times, choose to ‘turn behind’ a whale if they ascertain it’s swimming direction based on a general rule of thumb that, informed by years of encounters, humpback whales are more likely to continue their general direction of travel than they are to turn 180° following a dive. However, pilots are less likely to enact the same maneuver if humpback whales are foraging along a tidal rip, which they’ve found tends to produce more unpredictable movements. Thus, a priority avenue of research could be to explore the ‘linearity’ of whale movements, loosely defined as the degree to which whales travel in a straight line vs. turning (see also Williams et al., 2002; Barendse et al., 2010), and how it may be influenced by environmental conditions or other factors.
Refining estimates of detection probability, particularly as it applies to the area with in the Cone of Concern also represents an important research thread. The instantaneous detection estimates of the radial ship-to-whale distances we utilized (from Williams et al., 2016) were derived based on detecting whales across the 180-degree arc (beam-to-beam) forward of the ship. We assume that the probability of detection will be much higher at a given distance, or much father at a given detection probability, if similar detection functions were derived based on search effort solely in the Cone of Concern. We acknowledge that some of the ‘gains’ in detection from focused search in the Cone would be offset somewhat if mariners tasked with sighting whales are also tasked with other duties (e.g., monitoring radar or responding to radio communications). However, updating estimates of detection probability based on a Lookout’s focused search within the Cone of Concern would provide more reliable estimates and produce a more realistic range of feasible options of avoidance maneuvers.
Another productive avenue of research is a more rigorous examination of competing risks related to whale avoidance. During our simulations, once pilots confirmed that a whale was forward of the ship at some risk of collision, a primary consideration was how to achieve a new (avoidance) heading while not increasing other risks, such as collision with other vessels, reefs, or shoals. For obvious reasons, ship operators will rarely increase the risk of deleterious impacts to passengers or damage to the electrical system that accompanies dramatic and unsafe operations [e.g., a ‘crash stop’ (Wirz, 2012) or rapid turn], unless those maneuvers are offset by reduction in risk to more consequential events such as a grounding (for example). The risk of negative impacts from dramatic changes in course or speed to avoid a whale will thus always be weighed against the potential benefits of whale avoidance. In all simulations where a course change was needed, the pilot evaluated the efficacy of the course change by considering the needed time to incrementally ‘build up’ to the desired rate-of-turn to minimize impacts to passengers, the ship, and the environment, thereby avoiding excess ‘heel.’ Larger heel angles aboard cruise ships increase the chance that furniture will begin to slide and passengers will be injured from falls/by falling objects, and swim pools will spill, etc. As previously discussed, the electrical or propulsions systems can be negatively impacted in extreme instances of abrupt speed changes. We note that parameters identified as “safe” often represent general guidance and can be modified depending upon the PDMV’s experience and the situation (e.g., commencing an initial rate-of-turn, within defined parameters, and then after the ship has stabilized at that rate-of-turn, incrementally increasing, and stabilizing at greater rates-of-turn, while maintaining the ship within safe heel angles).
The development of a whale avoidance module in a full-mission ship simulator can also advance whale avoidance by training mariners, through repetition and experimentation, who have less experience with conditions where whale avoidance may be effective, avoidance techniques, and range of maneuvers that may be possible. Training modules can also lead to improved transit planning by scripting exercises within a specific operating area where whales are likely to be encountered while also accounting for local environmental conditions (e.g., wind, current, sea state), traffic situations, and other navigation hazards commonly experienced (e.g., ice).
Finally training can assist in communicating the value of whale avoidance to other members of the bridge team, such as the ship captain/mates. Pilots in Southeast Alaska have found that, upon boarding the ship, communicating with the bridge team that whales may be encountered, emphasizing the importance of whale avoidance, and discussion of avoidance techniques has increased situational awareness of whales while in transit (similar to communicating local knowledge of navigational hazards) and, importantly, often reduced resistance to implementing proactive avoidance maneuvers or temporary reductions in ship speed. A recent study in the St. Lawrence Estuary demonstrated the value that marine pilots can have in implementing strike-risk reduction efforts, in part, through elevating its importance for the larger bridge team (Chion et al., 2018).
An important caveat is that the development of training modules not generate a ‘recipe’ for proper maneuvers. The range of variation in avoidance maneuvers is large, based on whale swim speed, direction of travel, ship speed, and operational constraints, as are the competing risks of an avoidance maneuver. In a whale avoidance situation, mariners are often faced with making rapid decisions to prevent making an undesirable situation (e.g., risk of collision with a whale) become an even more harmful event (to the whale, the passengers, the ship itself, the environment, or all four). Marine pilots in Alaska, when asked what they do to avoid a whale, answer ubiquitously: “it depends.” In “mariner-speak” avoidance actions are based upon all factors appropriate to the prevailing circumstances and conditions, and with due regard for good seamanship. And good seamanship is a direct function of good training. To that end, we follow the reasoning of De Terssac (1992), as cited in Chauvin et al. (2009) who stated that, to achieve an overarching objective (which in this case is a reduction in collision risk) the best approach is to define “…a space of operation within which formal rules no longer specify the solution to be implemented, but list a range of permissible solutions among which the operator will have to choose the one that seems most relevant in the context.” Within that range of potential solutions may be a decision to maintain course or speed either because the value of the information available is insufficient or the risk of a worse outcome exceeds the risk of the ship strike (e.g., the altered course resulting in coming too close to shore, increasing the risk of grounding and catastrophic oil spill).
Based on our findings and observations, we conclude that active whale avoidance is feasible and, in most cases, can be practiced without creating an increase in competing risks. What’s more the practice can complement existing efforts that increase situational awareness of whales (e.g., Whale Alert) even in areas where other risk-reduction measures, such as operating at slower speeds, are in place. Most importantly, continuing collaboration between professional mariners, scientists, and natural resource managers is vital to reaching mutually beneficial reductions in whale strikes.
Data Availability Statement
The datasets generated for this study are available on request to the corresponding author.
Author Contributions
SG and LV conceived the idea and initiated the collaborative efforts between the Southeast Alaska Pilots’ Association and the National Park Service. SG led the writing of the manuscript and construction of the figures including the data used for detection and availability. SG, LV, CG, RP, and AH participated in the simulator activities at AVTEC. JB and LV collaborated on generating maneuvering graphics. SG, LV, CG, and JB contributed to the writing of the manuscript and idea development.
Funding
This effort represents an inter-institutional collaboration between the U.S. National Park Service and the Southeast Marine Pilots’ Association. Support from both institutions has taken the form of in-kind support for meetings, time, and travel. Both institutions jointly shared expenses for rental of full mission bridge simulator at AVTEC.
Conflict of Interest
AH was employed by QEDA Consulting, LLC.
The remaining authors declare that the research was conducted in the absence of any commercial or financial relationships that could be construed as a potential conflict of interest.
Acknowledgments
Foremost, we wish to acknowledge the input and participation in simulator efforts from Barry Olver. We also wish to thank Kirby Day of the Holland America Group, for his organizational efforts of the Southeast Alaska Marine Safety Task Force where some of these ideas were initially discussed. Glacier Bay National Park and the Southeast Alaska Pilots’ Association provided support and funding for simulator efforts and the formation of these ideas. We also thank the efforts of Mike Angove, programmer at AVTEC, for his help and expertise in running successful simulations.
Supplementary Material
The Supplementary Material for this article can be found online at: https://www.frontiersin.org/articles/10.3389/fmars.2019.00592/full#supplementary-material
Footnotes
- ^ http://seaiq.com/
- ^ https://www.kongsberg.com/digital/products/maritime-simulation/k-sim-navigation/
- ^ https://www.navcen.uscg.gov/pdf/navRules/CG_NRHB_20151231.pdf
- ^ www.WhaleAlert.org
- ^ www.repcet.com
References
Ancona, M. A., Baldi, F., Bianchi, M., Branchini, L., Melino, F., Peretto, A., et al. (2018). Efficiency improvement on a cruise ship: load allocation optimization. Energy Cons. Manag. 164, 42–58. doi: 10.1016/j.enconman.2018.02.080
Barendse, J., Best, P. B., Thornton, M., Pomilla, C., Carvalho, I., and Rosenbaum, H. C. (2010). Migration redefined? Seasonality, movements and group composition of humpback whales Megaptera novaeangliae off the west coast of South Africa. Afr. J. Mar. Sci. 32, 1–22. doi: 10.2989/18142321003714203
Chauvin, C., Clostermann, J., and Hoc, J. (2009). Impact of training programs on decision–making and situation awareness of trainee watch officers. Saf. Sci. 47, 1222–1231. doi: 10.1016/j.ssci.2009.03.008
Chen, J., Zou, Z. J., Chen, X., Xia, L., and Zou, L. (2017). CFD-based simulation of the flow around a ship in turning motion at low speed. J. Mar. Sci. Technol. 22, 784–796. doi: 10.1007/s00773-017-0449-7
Chion, C., Turgeon, S., Cantin, G., Michaud, R., MeÂnard, N., Lesage, V., et al. (2018). A voluntary conservation agreement reduces the risks of lethal collisions between ships and whales in the St. Lawrence Estuary (Quebec, Canada): from co-construction to monitoring compliance and assessing effectiveness. PLoS One 13:e0202560. doi: 10.1371/journal.pone.0202560
Conn, P. B., and Silber, G. K. (2013). Vessel speed restrictions reduce risk of collision-related mortality for North Atlantic right whales. Ecosphere 4, 1–16. doi: 10.1890/ES13-00004.1
Dolphin, W. F. (1987). Ventilation and dive patterns of humpback whales, Megaptera novaeangliae, on their Alaskan feeding grounds. Can. J. Zool. 65, 83–90. doi: 10.1242/jeb.023366
Fais, A., Lewis, T. P., Zitterbart, D. P., Alvarez, O., Tejedor, A., Aguilar, et al. (2016). Abundance and distribution of sperm whales in the Canary Islands: can sperm whales in the archipelago sustain the current level of ship-strike mortalities? PLoS One 11:e0150660. doi: 10.1371/journal.pone.0150660
Fujiwara, M., and Caswell, H. (2001). Demography of the endangered North Atlantic right whale. Nature 414, 537–541. doi: 10.1038/35107054
Garcia-Cegarra, A. M., Villagra, D., Gallardo, D. I., and Pacheco, A. S. (2019). Statistical dependence for detecting whale-watching effects on humpback whales. J. Wildl. Manag. 83, 467–477. doi: 10.1002/jwmg.21602
Gende, S. M., Hendrix, A. N., Harris, K. R., Eichenlaub, B., Nielsen, J., and Pyare, S. (2011). A Bayesian approach for understanding the role of ship speed in whale – ship encounters. Ecol. Appl. 21, 2232–2240. doi: 10.1890/10-1965.1
Gende, S. M., Hendrix, A. N., and Schmidt, J. (2018). Somewhere between acceptable and sustainable: when do impacts to resources become too large in protected areas? Biol. Conserv. 223, 138–146. doi: 10.1016/j.biocon.2018.04.038
Godwin, E. M., Noad, M. J., Kniest, E., and Dunlop, R. A. (2016). Comparing multiple sampling platforms for measuring the behavior of humpback whales (Megaptera novaeangliae). Mar. Mamm. Sci. 32, 268–286. doi: 10.1111/mms.12262
Harris, K., Gende, S. M., Logsdon, M. G., and Klinger, T. (2012). Spatial pattern analysis of cruise ship-humpback whale interactions in and near Glacier Bay National Park, Alaska. Environ. Manag. 49, 44–54. doi: 10.1007/s00267-011-9754-9
Hiby, A. R., and Ward, A. J. (1986). Analysis of cue-counting and blow rate estimation experiments carried out during 1984/85 IDCR Minke whale assessment cruise. Rep. Int. Whaling Commiss. 36, 473–475.
Hontvedt, M. (2015). Professional vision in simulated environments – Examining professional maritime pilots’ performance of work tasks in a full-mission ship simulator. Learn. Cult. Soc. Int. 7, 71–84. doi: 10.1016/j.lcsi.2015.07.003
Kavanagh, A. S., Noad, M. J., Blomberg, S. P., Goldizen, A. W., Kniest, E., Cato, D. H., et al. (2017). Factors driving the variability in diving and movement behavior of migrating humpback whales (Magaptera novaeangliae): implications for anthropogenic disturbance studies. Mar. Mamm. Sci. 33, 413–439. doi: 10.1111/mms.12375
Khan, M. Y., Agrawal, H., Ranganathan, S., Welch, W. A., Miller, J. W., and Cocker, D. R. (2012). Greenhouse gas and criteria emission benefits through reduction of vessel speed at sea. Environ. Sci. Technol. 46, 12600–12607. doi: 10.1021/es302371f
Knowlton, A. R., and Brown, M. W. (2007). “Running the gauntlet: right whales and vessel strikes,” in The Urban Whale: North Atlantic Right Whales at the Crossroads, eds S. D. Kraus and R. M. Rolland (Cambridge, MA: Harvard University Press), 409–435.
McKenna, M. F., Calambokidis, J., Oleson, E. M., Laist, D. W., and Goldbogen, J. A. (2015). Simultaneous tracking of blue whales and large ships demonstrates limited behavioral responses for avoiding collision. Endanger. Spec. Res. 27, 219–232. doi: 10.3354/esr00666
McKenna, M. F., Katz, S. L., Condit, C., and Walbridge, S. (2012). Response of commercial ships to a voluntary speed reduction measure: are voluntary strategies adequate for mitigating ship-strike risk? Coast. Manag. 40, 634–650. doi: 10.1080/08920753.2012.727749
Monnahan, C. C., Acevedo, J., Hendrix, A. N., Gende, S., Aguayo-Lobo, A., and Martinez, F. (2019). Population trends for humpback whales (Megaptera novaeangliae) foraging in the Francisco Coloane Coastal-Marine Protected Area, Magellan Strait, Chile. Mar. Mamm. Sci. doi: 10.1111/mms.12582
Monnahan, C. C., Branch, T. A., and Punt, A. E. (2015). Do ship strikes threaten the recovery of endangered eastern North Pacific blue whales? Mar. Mamm. Sci. 31, 279–297. doi: 10.1111/mms.12157
Nowacek, D., Johnson, M., and Tyack, P. (2004). North Atlantic right whales (Eubalaena glacialis) ignore ships but respond to alerting stimuli. Proc. R. Soc. Lond. B Biol. Sci. 271, 227–231. doi: 10.1098/rspb.2003.2570
Rameesha, T. V., and Krishnankutty, P. (2019). Numerical study on the manoeuvring of a container ship in regular waves. Ships Offshore Struc. 14, 141–152. doi: 10.1080/17445302.2018.1482706
Rockwood, R. C., Calambokidis, J., and Jahncke, J. (2017). High mortality of blue, humpback and fin whales from modeling of vessel collisions on the U.S. west coast suggests population impacts and insufficient protection. PLoS One 13:e0183052. doi: 10.1371/journal.pone.0183052
Schmidt, J. H., Rattenbury, K. L., Robison, H. L., Gorn, T. S., and Shults, B. S. (2017). Using non-invasive mark-resight and sign occupancy surveys to monitor low-density brown bear populations across large landscapes. Biol. Cons. 207, 47–54. doi: 10.1016/j.biocon.2017.01.005
Stelle, L. L., Megill, W. M., and Kinzel, M. R. (2008). Activity budget and diving behavior of gray whales (Eschrichtius robustus) in feeding grounds off coastal British Columbia. Mar. Mamm. Sci. 24, 462–478. doi: 10.1111/j.1748-7692.2008.00205.x
Thomas, P., Reeves, R., and Brownell, R. Jr. (2016). Status of the world’s baleen whales. Mar. Mamm. Sci. 32, 682–734.
van der Hoop, J. M., Vanderlaan, A. S. M., Cole, T. V. N., Henry, A. G., Hall, L., Mase-Guthrie, B., et al. (2015). Vessel strikes to large whales before and after the 2008 ship strike rule. Cons. Lett. 8, 24–32. doi: 10.1111/conl.12105
Vanderlaan, A. S. M., and Taggart, C. T. (2007). Vessel collisions with whales: the probability of lethal injury based on vessel speed. Mar. Mamm. Sci. 23, 144–156. doi: 10.1111/j.1748-7692.2006.00098.x
Vanderlaan, A. S. M., Taggart, C. T., Serdynska, A. R., Kenney, R. D., and Brown, M. W. (2008). Reducing the risk of lethal encounters: vessels and right whales in the Bay of Fundy and on the Scotian shelf. Endanger. Spec. Res. 4, 283–297. doi: 10.3354/esr00083
Webb, K. R., and Gende, S. M. (2015). Activity patterns and speeds of large cruise ships in Southeast Alaska. Coast. Manag. 43, 67–83. doi: 10.1080/08920753.2014.989148
Weinrich, M., Pekarcik, C., and Tackaberry, J. (2009). The effectiveness of dedicated observers in reducing risks of marine mammal collisions with ferries: a test of the technique. Mar. Mamm. Sci. 26, 460–470. doi: 10.1111/j.1748-7692.2009.00343.x
Williams, R., Trites, A. W., and Bain, D. E. (2002). Behavioural responses of killer whales (Orcinus orca) to whale-watching boats: opportunistic observations and experimental approaches. J. Zool. Lond. 256, 255–270. doi: 10.1017/s0952836902000298
Williams, S. H., Gende, S. M., Lukacs, P. M., and Webb, K. (2016). Factors affecting whale detection from large ships in Alaska with implications for whale avoidance. Endanger. Spec. Res. 30, 209–223. doi: 10.3354/esr00736
Wirz, D. (2012). Optimisation of the crash-stop manoeuvre of vessels employing slow speed two-stroke engines and fixed pitch propellers. J. Mar. Eng. Technol. 11, 35–43.
Yasukawa, H., Hirata, N., and Yamazaki, Y. (2018). Effect of bilge keels on maneuverability of a fine ship. J. Mar. Sci. Technol. 23, 302–318. doi: 10.1007/s00773-017-0474-6
Yasukawa, H., Hirono, T., Nakayama, Y., and Koh, K. K. (2012). Course stability and yaw motion of a ship in steady wind. J. Mar. Sci. Technol. 17, 291–304. doi: 10.1007/s00773-012-0168-z
Zaky, M., Sano, M., and Yasukawa, H. (2018). Improvement of maneuverability in a VLCC by a high lift rudder. Ocean Eng. 165, 438–449. doi: 10.1016/j.oceaneng.2018.07.030
Zerbini, A. N., Waite, J. M., Laake, J. L., and Wade, P. R. (2006). Abundance, trends and distribution of baleen whales off Western Alaska and the central Aleutian Islands. Deep-Sea Res. Pt. 1 53, 1772–1790. doi: 10.1016/j.dsr.2006.08.009
Keywords: vessel strike, active whale avoidance, ship operations, speed, detection probability
Citation: Gende SM, Vose L, Baken J, Gabriele CM, Preston R and Hendrix AN (2019) Active Whale Avoidance by Large Ships: Components and Constraints of a Complementary Approach to Reducing Ship Strike Risk. Front. Mar. Sci. 6:592. doi: 10.3389/fmars.2019.00592
Received: 31 March 2019; Accepted: 05 September 2019;
Published: 30 September 2019.
Edited by:
Jessica Redfern, Southwest Fisheries Science Center (NOAA), United StatesReviewed by:
Mason Weinrich, Center for Coastal Studies, United StatesPaul Conn, Alaska Fisheries Science Center (NOAA), United States
Copyright © 2019 Gende, Vose, Baken, Gabriele, Preston and Hendrix. This is an open-access article distributed under the terms of the Creative Commons Attribution License (CC BY). The use, distribution or reproduction in other forums is permitted, provided the original author(s) and the copyright owner(s) are credited and that the original publication in this journal is cited, in accordance with accepted academic practice. No use, distribution or reproduction is permitted which does not comply with these terms.
*Correspondence: Scott M. Gende, Scott_Gende@nps.gov