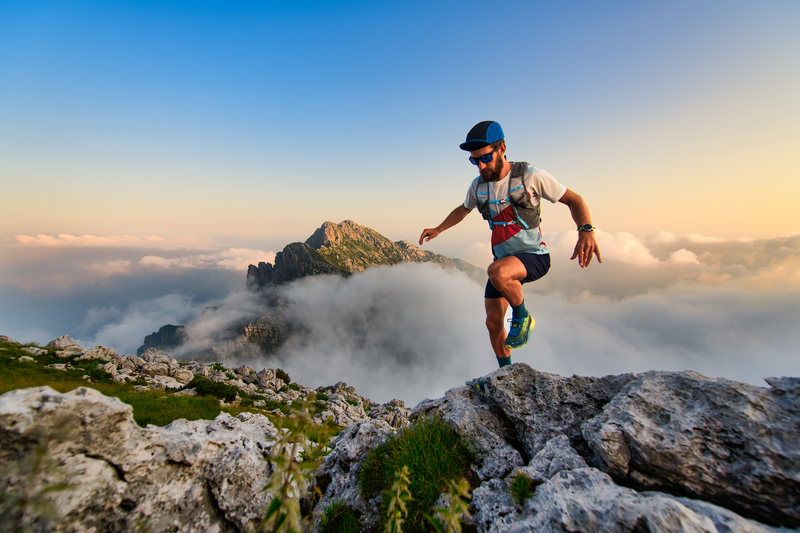
94% of researchers rate our articles as excellent or good
Learn more about the work of our research integrity team to safeguard the quality of each article we publish.
Find out more
ORIGINAL RESEARCH article
Front. Mar. Sci. , 02 August 2019
Sec. Marine Ecosystem Ecology
Volume 6 - 2019 | https://doi.org/10.3389/fmars.2019.00492
The responses of food webs to simultaneous changes in several environmental drivers are still poorly understood. As a contribution to filling this knowledge gap, we investigated the major pathways through which two interlinked environmental drivers, eutrophication and climate, affect the biomass and community composition of fish and benthic macrofauna. For this aim, we conducted a systematic sensitivity analysis using two models simulating the dynamics of benthic and pelagic food webs in the Baltic Sea. We varied environmental forcing representing primary productivity, oxygen conditions and water temperature in all possible combinations, over a range representative of expected changes during the 21st century. Both models indicated that increased primary productivity leads to biomass increase in all parts of the system, however, counteracted by expanding hypoxia. Effects of temperature were complex, but generally small compared to the other drivers. Similarities across models give confidence in the main results, but we also found differences due to different representations of the food web in the two models. While both models predicted a shift in benthic community composition toward an increased abundance of Limecola (Macoma) balthica with increasing productivity, the effects on deposit-feeding and predatory benthic groups depended on the presence of fish predators in the model. The model results indicate that nutrient loads are a stronger driver of change for ecosystem functions in the Baltic Sea than climate change, but it is important to consider the combined effects of these drivers for proper management of the marine environment.
Marine ecosystems are threatened by multiple anthropogenic pressures (Halpern et al., 2008). Eutrophication and associated hypoxia have deteriorated coastal seas and continue to pose a major threat to marine ecosystems globally (Cloern, 2001; Gray et al., 2002; Breitburg et al., 2018). Climate change affects the life-histories and distributions of marine species, and is recognized as a major driver of food-web reorganizations (Poloczanska et al., 2013; Cloern et al., 2016). Unraveling the effects of these and other interacting drivers on the marine ecosystem is imperative for proper ecosystem management to ensure the future functioning of marine ecosystems and provision of ecosystem services (Atkins et al., 2011; Cloern et al., 2016).
Most studies so far have looked at the effects of isolated drivers (Richardson et al., 2012; Pascual et al., 2017), although Crain et al. (2008) show that synergistic and antagonistic interactions among drivers are common. It is logistically difficult to test the effects of multiple drivers across a large gradient of levels in empirical studies (Boyd et al., 2018). Process-based ecological models are powerful tools to conduct such tests (Christensen and Walters, 2004; Coll et al., 2015; Seidl, 2017). Additionally, such models can be used to study the relative importance of direct (e.g., physiological) and indirect (e.g., food-web mediated) effects on ecosystem components (Blackford, 2002; Fulton et al., 2004; Condie et al., 2012).
Here, we use two different process-based ecological models to systematically analyze the responses of benthic and pelagic food webs to multiple combinations of drivers across a large range of levels. In general, marine ecological modeling is associated with large uncertainties, especially when implemented on large temporal and spatial scales. Comparing and synthesizing the results of multiple models is one way to address that uncertainty (Fulton, 2010; Uusitalo et al., 2016). The models complement each other in terms of spatial and temporal scales and level of abstraction. The Ecopath with Ecosim (EwE) model focuses on the ecosystem scale and describes food web interactions in both benthic and pelagic compartments of the central Baltic Sea (Bauer et al., 2018). The Benthic Macrofauna (BM) model focuses on local macrobenthos in the area (at 40 m depth) and uses a physiologically more detailed, mechanistic description of the system (Ehrnsten et al., 2019).
There are a number of previous modeling studies investigating the potential future responses of marine ecosystems to various drivers with the help of future scenario simulations, including those using the EwE approach (Evans et al., 2013; Niiranen et al., 2013; Österblom et al., 2013) and a previous version of the BM model (Timmermann et al., 2012). Scenarios are valuable to gain an insight into possible ranges of future states of the ecosystem (Cheung et al., 2009; Brochier et al., 2013). However, as they typically vary several drivers (e.g., temperature, oxygen, and salinity) at the same time, they only provide a limited mechanistic understanding of the pathways by which such drivers and their interactions affect ecosystems. In their recent review of studies on climate change impacts on ecosystem services, Runting et al. (2017) pointed out that a systematic analysis of both cumulative and relative effects of multiple drivers is needed, but often overlooked. Such an analysis enables a better interpretation of the results of scenario studies, by identifying which specific drivers, or their interactions, drive scenario results. In addition, an estimation of the uncertainty associated with studies solely looking at the effects of single drivers is made possible.
Both models are parameterized to be representative of the Baltic Sea, a coastal sea that due to its location and hydrography is particularly exposed to multiple anthropogenic pressures, such as nutrient loading and climate change (Karlson et al., 2002; Belkin, 2009; Carstensen et al., 2014). Since the 1950s, increased nutrient loading has led to increased primary production (Gustafsson et al., 2012), providing food for zooplankton and planktivorous fish in the pelagic food web (Eero et al., 2016). It has also increased sedimentation of organic matter which acts as a food source for benthic communities (Cederwall and Elmgren, 1980). The degradation of organic matter consumes oxygen, and has led to spreading hypoxic and anoxic areas in Baltic Sea (Conley et al., 2009; Carstensen et al., 2014). Hypoxia diminishes the diversity and functionality of benthic communities (Villnäs et al., 2013; Gammal et al., 2017). The effects of decreasing oxygen concentrations on growth and survival differ among marine groups, with demersal fish and crustaceans generally showing high sensitivity while bivalves can survive extended periods of complete anoxia by closing their valves (Gray et al., 2002). In the Baltic Sea, the common deposit-feeding amphipods Monoporeia affinis and Pontoporeia femorata are highly sensitive to hypoxia while the predatory isopod Saduria entomon is among the most tolerant (Timmermann et al., 2012). Hypoxia also has negative impacts on reproductive conditions for demersally spawning fish, such as cod (MacKenzie et al., 2000; Margonski et al., 2010).
At the same time, the Baltic Sea has been one of the fastest warming marine ecosystems in the world during the last decades (Belkin, 2009), and water temperatures are projected to increase further during the 21st century (Meier et al., 2011, 2012a). Temperature directly affects most physiological rates across the whole food web, with varying effects of warming on different species and their interactions (Pörtner and Farrell, 2008; Brander, 2010). It increases growth rates of herring larvae (Hakala et al., 2003) and has a positive effect on herring and sprat productivity (Margonski et al., 2010; Bartolino et al., 2014). On the other hand, there are indications that several common marcobenthic species in the Baltic Sea have declined due to rising temperatures, including the glacial relicts M. affinis and S. entomon (Segerstråle, 1962; Rousi et al., 2013). Beukema et al. (2009) found negative effects of warming on the growth, reproduction and survival of the Baltic clam Limecola (Macoma) balthica in the Wadden Sea, suggesting that warming might affect also Baltic populations in the near future. Increasing temperature also increases the sensitivity of benthic groups to hypoxia (Vaquer-Sunyer and Duarte, 2011). Furthermore, rising temperatures increase the microbial degradation of organic matter, consuming oxygen, and reducing food availability for macrobenthos.
The aim of this study is to identify the most important environmental drivers of selected benthic and pelagic ecosystem components, potential non-linear responses in the ecosystem, and to explore possible interactive effects between drivers. We focus on the effects of two major external pressures of the marine ecosystem, nutrient loading and climate, on the biomass and community composition of macrobenthos and pelagic and demersal fish (Figure 1). More specifically, we hypothesize that: (1) increasing productivity increases biomass of planktivourous fish and detritivorous benthos, (2) hypoxia counteracts the effects of productivity and alters community composition with strongest negative effects on sensitive groups like deposit-feeders and demersally spawning and feeding fish, and (3) increasing temperature has interactive effects with productivity and oxygen conditions, improving growth conditions for small pelagic fish (herring and sprat) while diminishing those of BM.
Figure 1. Overview of interacting environmental drivers and marine ecosystem components considered in this study.
We use two ecological models to study the effects of changes in the environmental drivers: (1) productivity, (2) oxygen conditions, and (3) water temperature on the biomass of BM, demersal and pelagic fish in the Baltic Sea (Figure 1). These environmental drivers are represented in the two models as forcing affecting different variables (Table 1). Below the models are described in general terms with a focus on the implementation of forcing affecting ecosystem components in each model. References to full model descriptions including mathematical formulations are given in the respective sections.
Table 1. Studied environmental drivers, corresponding forcing, and functional groups directly affected by the forcing in the two models.
The Baltic Sea is a semi-enclosed brackish-water basin with a short geological history, and, consequently, a low diversity of macroscopic species and simple food-webs characterized by a few dominating species (Snoeijs-Leijonmalm, 2017). The sea encompasses positive north to south gradients in environmental conditions, such as salinity and temperature, and extent of anthropogenic pressures, such as nutrient loads, as well as in species and functional diversity. Here, we focus on the Baltic Proper basin including offshore pelagic food-webs and benthic food-webs in the deep, aphotic (non-sunlit) zone. Soft sediments inhabited by the facultative suspension/deposit-feeding Baltic clam Limecola balthica dominate the sea (Gogina and Zettler, 2010; Gogina et al., 2016). Other common deposit-feeders include the amphipods M. affinis and P. femorata and the non-native polychaetes Marenzelleria spp. Key predators in the benthic system include invertebrates, such as the isopod S. entomon, and demersal fish, such as European flounder (Platichthys flesus) and cod (Gadus morhua), the latter linking the benthic and pelagic systems. Key fish species in the pelagic system are the planktivorous sprat (Sprattus sprattus) and herring (Clupea harengus) and their main predator, cod.
The EwE modeling approach and software1 is used to describe biomass flows among ecosystem components (Ecopath) and the system’s dynamic response to external forcing such as changing fishing effort or environmental conditions (Ecosim). The biomass dynamics of a functional group is dependent on the biomass production of the group (assimilated consumption minus respiratory losses), predation on the group, fishing and other sources of mortality, as well as egg production (juveniles) or the number of juveniles maturing into adults (adults) for the groups represented by multiple life stages (“stanzas”). The theory behind EwE, its implementation as a software and its limitations have been extensively described by Pauly et al. (2000), Christensen and Walters (2004), and Plagányi (2007). EwE has been used numerous times to investigate environmental and physical effects on ecosystem state, especially on fish production (e.g., Coll et al., 2009; Morato et al., 2009; Libralato et al., 2015).
The EwE model of the offshore central Baltic Sea (ICES subdivisions 25–29, excl. the Gulf of Riga) used here represents the ecosystem as 21 living functional groups and species and one detritus group. Living groups encompass all trophic levels from phytoplankton to gray seals (Halichoerus grypus), including the four commercially most important fish species (cod, flounder, herring, and sprat). The latter are each represented as two stanzas (adult and juveniles). Macrobenthos groups in EwE are S. entomon, two bivalve groups (L. balthica and Mytilus spp.), and “other macrobenthos,” consisting of oligochaetes, polychaetes (e.g., Bylgides sarsi), and amphipods. The model is parameterized to represent the ecosystem state in the early 2000s. It has been used previously to explore alternative fisheries management strategies (Bauer et al., 2019) and spatial effects of nutrient load management in the Baltic Sea (Bauer et al., 2018). The model parameterization used here is the same as in Bauer et al. (2018), except for small modifications described in Bauer et al. (2019). Fishing mortalities were kept constant at their values in Ecopath (representative of 2004) for all analyses (adult/juvenile cod: 0.84/0.05, flounder: 0.59/0.02, herring: 0.13/0.05, sprat: 0.4/0.14).
The effects of environmental drivers in EwE are implemented in three ways: primary production forcing, forcing affecting consumption and egg production forcing (Christensen et al., 2008). All three types of forcing are implemented as a time series of multipliers applied on certain model parameters (Table 1). The first value of each time series has to be 1, that is, all parameters are initially at their baseline values. Primary production forcing values are applied as a multiplier of the r parameter (maximum production to biomass ratio, P/B) in the equation relating primary producer biomass to production via a saturating relationship, of which r is the asymptote (Christensen et al., 2008). Forcing affecting consumption rate is here applied as a multiplier on the “effective search rate” of predators, which is equivalent to the attack rate parameter commonly used in functional response formulations (Holling, 1959). Egg production forcing is applied as a multiplier on the number of eggs produced by default (Walters et al., 2010). In previous studies on another central Baltic Sea EwE model, forcing time series were derived from time series of environmental drivers (Tomczak et al., 2012; Niiranen et al., 2013) and we assume similar relationships between forcing and environmental drivers here. Consumption rates of cod and several benthic groups are related to the extent of oxic areas (OxAr) (Sarvala, 1971; Eero et al., 2015; Köster et al., 2017). Cod egg production is related to cod reproductive volume (CodRV, the volume of water with salinity > 11 psu and oxygen concentration > 2 mL L-1), and herring and sprat egg production to sea surface temperature in August (TSurf) (MacKenzie et al., 2000; Margonski et al., 2010). Forcing functions affecting several groups (OxAr, TSurf) have the same effect on all groups’ consumption or egg production rates. Representing environmental forcing as linear multipliers on certain rates is a major difference to the more mechanistic BM. In the latter, forcing is applied as time series of absolute values of a given environmental variable (e.g., temperature in °C, see below) affecting ecological rates described by different mechanistic relationships.
The BM model simulates the flows of carbon through functional groups of macrofauna and their food banks in the sediment. The model is based on the physiological macrofauna model of Timmermann et al. (2012), but uses some novel formulations and parameterizations, including a dynamic feedback between benthic fauna and sediment organic carbon and a new functional response formulation for predation on multiple prey (Ehrnsten et al., 2019). The carbon biomass dynamics of a functional group is described by the physiological processes of ingestion, assimilation, egestion and respiration as well as the population-level processes of predation, other mortality and recruitment, similarly to the benthic biology module in the European Regional Seas Ecosystem Model (Ebenhöh et al., 1995; Butenschön et al., 2016; Lessin et al., 2019).
Here, we use the implementation of the model representing a 40 m deep soft-sediment area in the western Baltic Proper described in Ehrnsten et al. (2019). It includes three functional groups of macrofauna and two sediment food banks (freshly deposited and older organic carbon). The freshly deposited carbon is consumed by the surface deposit-feeder functional group (hereafter referred to as “deposit-feeders”) dominated by the amphipods M. affinis and P. femorata. The second functional group only comprises the key species L. balthica, which is capable of suspension-feeding when plankton concentrations are high. This behavior is modeled as a switch to feeding on suspended matter when bottom water chlorophyll-a concentrations are > 2 mg m-3 (see Timmermann et al., 2012). However, in the present implementation the chlorophyll-a threshold is rarely reached, and L. balthica mostly consumes organic carbon from the older sediment food bank. Invertebrate predator/scavengers (hereafter “predators”) are a taxonomically diverse group including the priapulid Halicryptus spinulosus, the isopod S. entomon and the polychaetes B. sarsi and Hediste diversicolor. Predators feed on both other macrofauna groups with a strong preference for deposit-feeders.
Forcing to the BM model consists of daily time-series of bottom water temperature (TBot), concentration of oxygen (O2Conc), concentrations of suspended plankton and detritus, as well as organic matter sedimentation rate (Sed). These are provided by the physical-biogeochemical BALTSEM model (Gustafsson et al., 2012; Savchuk et al., 2012). Sedimentation is accumulated in the food banks, which are consumed by the fauna and degraded at temperature-dependent rates. Temperature also affects the production of macrofauna through ingestion, basal respiration, and mortality rates. These direct temperature effects are modeled according to a standard, exponentially increasing Q10-formulation. Low oxygen concentrations cause mortality of the fauna via a temperature-dependent negative exponential function, with different sensitivities toward hypoxia for the three groups (deposit-feeders > L. balthica > predators) (Timmermann et al., 2012).
Mean values for January 1st 2000–2005 simulated by Ehrnsten et al. (2019) were used as initial conditions for the state variables. Otherwise all model settings and parameter values were applied as in Ehrnsten et al. (2019).
To test our hypotheses on the effects of changes in productivity, oxygen conditions, and temperature compared to recent conditions on a number of ecosystem components, both models were run under systematically varied forcing (Table 1). Subsequently, we calculated biomass change of key functional groups compared to the baseline run. In EwE the baseline meant a simulation with all forcing set to the value of 1, i.e., all affected parameters were kept at their default values. In BM, baseline forcing were the daily values of all forcing time series simulated by BALTSEM averaged over the years 2000-2005 (Figure 2). In both models, productivity- and temperature-related forcing were varied in the range of 0.5–2 times the baseline. This range corresponds roughly to changes that can be realistically expected to occur in the region in the 21st century (Meier et al., 2011, 2012a; Saraiva et al., 2018), except for a decrease in temperature which is included in the test for completeness. Cod reproductive volume (CodRV) was tested in the range of 0–10 times the baseline, approximately corresponding to a range of no reproductive volume to 1500 km3 (the latter representing a tenfold increase in CodRV, which could only happen under an extremely optimistic scenario without climate change). OxAr were tested in the range of 0.75–1.5, approximately corresponding to half to the whole of the Baltic Proper seafloor being oxic. In BM, bottom water oxygen concentrations (O2Conc) of 0–1 times the baseline was tested, as the baseline corresponds to approximately fully oxygen saturated conditions, so only decreases are possible. We show biomass changes under selected forcing combinations, all combinations are shown under Supplementary Figure S2 (EwE) and Supplementary Figure S3 (BM). The sensitivity of EwE model results to important parameter settings are shown in Supplementary Figure S1.
Figure 2. Forcing time-series used in the BM model, showing the tested levels of sedimentation (A), oxygen concentration (B), and bottom temperature (C) over 1 year. Level 1 denotes the baseline.
In EwE simulations were run for 400 yearly time steps under constant forcing. That is, we applied a time series of 400 steps, the first one being 1, the next 399 being the tested value of the forcing. In BM forcing was repeated as an identical seasonal cycle every year for 100 years, using daily time steps. The range of tested values of the forcing time-series in BM are shown in Figure 2. The tested time periods in both models were chosen to be long enough for the dynamics to surpass the transition period and reach an equilibrium state, defined as the lack of interannual increasing or decreasing trends in biomass of the studied functional groups.
The P/B forcing, representing phytoplankton productivity, was the most influential factor affecting community biomass (Figure 3). Cod and sprat showed non-linear positive responses, adult flounder, L. balthica and “other macrobenthos” showed linear positive responses and S. entomon a saturating positive response. Herring biomass did not respond strongly to P/B or to any other forcing (Figure 3C and Supplementary Figure S1.2).
Figure 3. Change in biomass of cod (A), flounder (B), herring (C), sprat (D), Limecola balthica (E), “other macrobenthos” (F), and Saduria entomon (G) as a function of relative changes in P/B and OxAr, where 1 denotes baseline forcing. Biomass change is relative to baseline equilibrium value. Error bars show the variability in biomass change due to varying TSurf and CodRV. Horizontal line indicates no change. Results from simulations with CodRV = 0, resulting in 0 cod biomass, are excluded from the figure. Forcing abbreviations are explained in Table 1.
P/B and extent of OxAr had indirect effects on several groups due to trophic relationships. OxAr directly increased consumption rates of cod and some other benthic groups on their prey (Table 1). Thus, OxAr had a positive effect on cod (Figure 3A) and L. balthica (Figure 3E), due to their increased consumption of benthic food. In contrast, OxAr had a weak negative effect on sprat, probably as an indirect effect of increased cod biomass under higher OxAr, as sprat is a main non-benthic prey of cod (Figure 3D and Supplementary Figure S1.1). Even though OxAr also increased consumption rates of the “other macrobenthos” group, OxAr ≥ 1 resulted in lower biomasses than OxAr = 0.75 (Figure 3F), presumably due to higher cod biomass causing higher predation mortality under higher OxAr values. For the same reason, the highest tested level (1.5) of OxAr, resulted in lower S. entomon biomasses than at OxAr < 1.5, although only in combination with high (> 1) P/B (Figure 3G).
Although surface temperature (TSurf) and cod reproductive volume (CodRV) only directly affected fish groups, they also impacted some benthic groups (Figure 4) due to a chain of indirect effects. Temperature had a positive effect on juvenile sprat (Figure 5D) which feeds on zooplankton, thereby freeing phytoplankton from predation pressure, and increased phytoplankton increased L. balthica biomass (Figures 3E, 4A). Increasing CodRV had a negative effect on S. entomon (Figure 4B) due to increased predation especially by juvenile cod (Figure 5C).
Figure 4. Change in biomass of L. balthica as a function of relative changes in TSurf (A) and S. entomon as a function of relative changes in CodRV (B) at four different levels of P/B, where 1 denotes baseline forcing. Biomass change is relative to baseline equilibrium value. Error bars show the variability in biomass change due to varying OxAr and CodRV (A) and OxAr and TSurf (B). Horizontal line indicates no change. Results from simulations with CodRV = 0, resulting in 0 cod biomass, are excluded from the figure. Forcing abbreviations are explained in Table 1.
Figure 5. Change in biomass of adult (A) and juvenile (C) cod and adult (B), and juvenile (D) sprat as a function of their respective egg production forcing (Table 1) at four different levels of P/B, where 1 denotes baseline forcing. Biomass change is relative to baseline equilibrium value. Error bars show the variability in biomass change due to varying forcing factors other than P/B and egg production. Horizontal line indicates no change. Forcing abbreviations are explained in Table 1.
We found that the effects of egg production forcing were modified based on productivity (Figure 5). Except for P/B = 0.5, where adult cod biomass was mostly insensitive to changes in CodRV, adult cod biomass increased up to a point with increasing CodRV but saturated (at P/B = 1) and even decreased (at P/B > 1) when CodRV increased above ∼1.75, hinting at a negative effect of a large number of juveniles produced on the adult population, especially at high primary productivity. The relationships between TSurf, P/B and sprat were very similar: at P/B = 0.5 increasing TSurf had no effect, at P/B ∼1 it had a positive effect on both adult and juvenile sprat and at P/B > 1 it had a positive effect on juvenile, but not on adult sprat. Thus, competition between juveniles and adults of both species was higher at high P/B. That seems counterintuitive, as at high P/B there is more primary production to support higher trophic levels. However, the production of juveniles is, without any change in egg forcing functions, already relatively high at high P/B because of the high adult fish biomass implying a large number of mature fish. A further increase when applying an increase in egg production forcing thus means a disproportionately large (compared to the increased P/B) increase in juvenile fish and their predation pressure on groups that also serve as prey for the adults. High juvenile sprat biomass had a stronger negative effect on adult sprat biomass than it was the case for cod, due to a very high diet overlap between juveniles and adults of sprat.
Organic matter sedimentation (Sed) as an indicator of productivity and food availability was the most influential forcing affecting benthic community biomass. It had a mostly linear positive effect on L. balthica and predator biomasses (Figures 6, 7). Temperature (TBot) counteracted the positive effects of sedimentation on these two groups: increased bottom water temperature decreased the slope of the biomass response to sedimentation (Figure 6). For L. balthica, this was due to both to increased degradation rate of the food source counteracting the potential increase in ingestion rate, and to increased metabolic losses with increasing temperature. Oxygen concentrations (O2Conc) showed synergistic effects with sedimentation, i.e., increasing oxygen concentrations increased the positive response to sedimentation for the two groups (Figure 7).
Figure 6. Change in biomass of deposit-feeders (A), L. balthica (B), and predators (C) as a function of relative changes in Sed and TBot, where 1 denotes baseline forcing. Biomass change is relative to baseline equilibrium value. Error bars show the variability in biomass change due to varying O2Conc. Horizontal line indicates no change. Forcing abbreviations are explained in Table 1.
Figure 7. Change in biomass of deposit-feeders (A), L. balthica (B), and predators (C) as a function of relative changes in Sed and O2Conc, where 1 denotes baseline forcing. Biomass change is relative to baseline equilibrium value. Error bars show the variability in biomass change due to varying TBot. Horizontal line indicates no change. Forcing abbreviations are explained in Table 1.
The effects of O2Conc were sigmoidal and saturating for all groups: oxygen levels below a certain threshold led to extinction, with a strong increase in biomass above the threshold (Figure 8). The form of the response was group-specific with L. balthica being least sensitive to hypoxia. Reduced O2Conc affected predators both directly through mortality and indirectly through reduction in prey biomass, leading to a higher sensitivity than would be predicted from hypoxia mortality rates alone.
Figure 8. Change in biomass of deposit-feeders (A), L. balthica (B), and predators (C) as a function of relative changes in O2Conc and TBot, where 1 denotes baseline forcing. Biomass change is relative to baseline equilibrium value. Error bars show the variability in biomass change due to varying Sed. Horizontal line indicates no change. Forcing abbreviations are explained in Table 1.
The effects of forcing on deposit-feeders were complex with interacting effects of all three forcing types. Low sedimentation rate, indicating low food availability, in combination with high temperature and/or low oxygen concentration led to severely reduced biomass or even extinction. This means that the ability to survive in increased temperatures and/or reduced oxygen concentrations was greatly reduced by food shortage. However, at forcing combinations outside of this “lethal space,” i.e., when assuming a higher sedimentation rate and/or more suitable abiotic conditions, the biomass response to increasing sedimentation was slightly negative (Figures 6, 7). The effects of temperature were slightly positive except at hypoxia or very low sedimentation rate (Figures 6, 8).
The effects of sedimentation and temperature on deposit-feeders was opposite to those on L. balthica and predators. This pattern was due to food web interactions that became an important driver of deposit-feeder biomass responses under non-lethal environmental conditions, as can be seen from the relationship between benthic predators and their prey (Figure 9). At severe hypoxia there was no correlation between predators and prey. In normoxic conditions, there was a positive relationship between L. balthica and predators, but a negative relationship between deposit-feeders and predators. This indicates that L. balthica biomass drives predator biomass, but there is little reciprocal effect as L. balthica biomasses are generally one order of magnitude larger than predator biomasses (bottom-up control). For deposit-feeders, the negative relationship indicates top-down control, i.e., that predation is a significant driver of deposit-feeder biomass.
Figure 9. Relationship between biomass of L. balthica and predators (A) and surface deposit-feeders and predators (B) at hypoxia (O2Conc < 0.1, blue) and normoxia (baseline O2Conc, yellow). Biomass values are in mg C m-2. Forcing abbreviations are explained in Table 1.
We have used two distinct food web models to study the effects of environmental drivers on marine ecosystem components in the Baltic Sea. Both models showed the same main responses to environmental drivers. Primary productivity as a basis of the food web was the strongest driver of community biomass, having a positive effect on most groups as hypothesized. In EwE, all groups but herring showed a clear positive response to increases in phytoplankton production. In BM, two out of three groups (L. balthica and predators) showed a positive response to increased sedimentation. The other two groups (herring in EwE and deposit-feeders in BM) showed weak responses to productivity. The effects of oxygen conditions, measured as extent of oxic areas (EwE), cod reproductive volume (EwE) or oxygen concentration (BM) forcing, were mostly non-linear. A strong negative effect of low oxygen concentrations on all benthic groups was evident in BM, but biomasses saturated quickly as concentrations increased. Similarly, cod reproductive volume had a strong effect on cod biomass at low values, but the biomass response saturated quickly. Temperature effects were more variable than initially hypothesized (e.g., contrasting effects between adult and juvenile sprat and between L. balthica and deposit-feeders). Nevertheless, they were generally small compared to the other two environmental drivers within the tested range, representative of anticipated change in the 21st century.
The models showed several interactive effects of the studied drivers, both synergistic and antagonistic. In many cases these effects were indirect and modified by food web interactions. For example, the result that the relationship between sedimentation and deposit-feeders was slightly negative in BM seems counterintuitive, but can be explained by indirect effects through the food web. Sedimentation had a positive effect on the food availability for L. balthica, which represents the majority of benthic biomass. Increased biomass of L. balthica was one of the main drivers of predator biomass, which in turn asserted an increased predation pressure on deposit-feeders. This is a phenomenon known as “apparent competition” when two prey biomasses are negatively related due to a shared predator (Holt, 1977; Holt and Lawton, 1994). Thus, the indirect effect of increased sedimentation via intensifying apparent competition between L. balthica and deposit-feeders had a stronger effect on deposit-feeder biomass than the direct effect via increased food availability. Tight predator-prey coupling between S. entomon and the deposit-feeder M. affinis has been recorded in the northern Baltic Sea where L. balthica was absent (Sparrevik and Leonardsson, 1999; Englund et al., 2008; Englund and Leonardsson, 2008). In an area with few amphipods present, S. entomon predated actively L. balthica (Ejdung and Bonsdorff, 1992), but to our knowledge there are no studies that included both prey types that could have revealed apparent competition in the lab or field.
Our study shows that the choice of system boundaries are important to consider when estimating impacts of environmental drivers on ecosystems. In EwE, the benthic predator S. entomon did not show a similar strong response to increased environmental productivity as in BM. This is at least partly due to a stabilizing effect of predation by cod. Cod strongly benefited from increased primary productivity through increased availability of one of its main prey, sprat. Thus, under high productivity cod caused high predation mortality on S. entomon, counteracting the positive effect of increased prey availability for S. entomon. In BM, predation on benthic predators is included as a closure term, and is therefore less dynamic than in EwE that explicitly models the dynamics of the top predator (cod) feeding on both predatory benthos and pelagic prey. Thus, EwE showed an apparent competition effect between sprat and S. entomon, mediated by cod predation, that was missing from BM. Real world marine ecosystems are extremely complex, and models are by definition a simplification of that immense complexity. There is no “best model” for any given system, as the choice of elements to be included in any model depends on the question to be addressed, Data Availability and planned uses of the model (Fulton et al., 2003; Fulton, 2010). There are a number of modeling approaches describing marine ecosystems and populations that differ in their representations of main processes, but they can all be used the generate projections of how the system reacts to external pressures. As such projections are sensitive to model formulation, as shown by this as well as other studies (e.g., Fulton et al., 2003; Gårdmark et al., 2013), they should be viewed as alternative hypotheses and as a potential basis for empirical studies.
Besides model scale, another difference between the models is the implementation of forcing. In EwE, it is assumed that the effects of environmental drivers on the affected parameters, such as consumption or egg production rates, are linear (Christensen et al., 2008). Additionally, due to the complexity and large number of groups included in our EwE model, each environmental forcing only affected a selected number of groups to keep model dynamics more tractable. In BM, environmental variables were used as forcing in a more realistic manner. For example, temperature effects were only included in EwE on herring and sprat egg production, while in BM all state variables were affected by temperature through more mechanistic Q10-relationships for several process rates. We note that there are recent approaches to incorporate more mechanistic forcing in EwE models as well (de Mutsert et al., 2012; Libralato et al., 2015). Despite these very different approaches, both models showed relatively small effects of temperature on the studied food-webs. Overall temperature responses were largely dampened by compensatory effects in different parts of the system, e.g., in BM the increased potential ingestion rates of L. balthica and deposit-feeders were counteracted by faster degradation of the food sources under increasing temperatures. It has been argued that estuarine areas with strong seasonality and low diversity like the Baltic Sea should harbor organisms with large tolerance ranges to environmental drivers such as temperature (Jernelöv and Rosenberg, 1976). Nevertheless, some of the dominant species of the deep zone included in our models (M. affinis, Mysis relicta, and S. entomon) are glacial relicts that are believed to be cold-adapted (Segerstråle, 1962). The reason for a lack of a larger temperature response from these groups may partly be that some important temperature effects are not captured by either model, e.g., on species distributions, phenology or body sizes (Sommer et al., 2012; Poloczanska et al., 2013; Birchenough et al., 2015).
There are additional relevant environmental drivers with potential effects on both the benthic and pelagic food web in the Baltic Sea that were not considered here. Salinity in this study was only represented as a component of cod reproductive volume, as in an earlier EwE model of the Baltic other salinity effects had little impact on model dynamics (Niiranen et al., 2013). However, salinity is considered a major determinant of present and future species distributions in the Baltic Sea (Gogina and Zettler, 2010; Ojaveer et al., 2010; Jokinen et al., 2015; Vuorinen et al., 2015). Oxygen conditions on the seafloor do not only affect the benthic communities through permanent low oxygen concentrations, but also through occasional extreme hypoxic events. These occur with increasing frequency under elevated eutrophication (Conley et al., 2011). From our two models, only the BM is suitable to study the effects of short extreme events, due to its daily timescale. Here, we focused on effects of permanent changes in environmental drivers, such as elevated water temperatures over the whole year, which could be studied by both models.
In general, both models consider a fixed number of functional groups in a non-spatial framework representing the central Baltic ecosystem. Thus, potential effects of migration, both small-scale movement of species to avoid adverse conditions (e.g., local hypoxia), and invasion of completely new species, are beyond the scope of this study. Small-scale movement of species could be addressed using spatial models like in Bauer et al. (2018). The impacts of specific novel species or potential invaders could be addressed in a straightforward way in both modeling frameworks by including them as additional functional groups. In this case, the biomass responses of current functional groups presented in this study would serve as a baseline. Changes in those responses compared to the baseline due to the inclusion of invasive groups are a quantitative measure of how much a novel ecological configuration affects the system’s sensitivity to environmental pressures (Radeloff et al., 2015). It should also be noted that the results may not be directly generalizable to shallow coastal systems or other basins of the Baltic Sea, as environmental conditions and food-web structures in those habitats are quite different from the ones considered here. For example, suspension-feeding had a minor role in the current study, but may be important for pelagic-benthic organic matter fluxes and benthic dynamics in other areas (Kautsky and Evans, 1987; Lessin et al., 2019).
It is uncertain how primary production in the Baltic Sea is going to develop during the next century. Climate change alone would increase primary production due to increased water temperatures and nutrient runoff from land (Meier et al., 2011, 2012b; Saraiva et al., 2018). Our simulations indicate that this would increase biomass and alter community composition in both benthic and pelagic parts of the system. Regarding the fish community, EwE predicts that further increases in primary productivity favor sprat and cod equally. However, when coupled with hypoxia, positive effects may only be seen in sprat. Negative effects of hypoxia on cod have been previously proposed based on empirical data (Eero et al., 2015; Casini et al., 2016), as well as positive effects of increased productivity on sprat production (Chassot et al., 2007; Eero et al., 2016). The model indicated that positive effects of increased productivity could be limited by density-dependence within the cod and especially sprat population (Casini et al., 2011).
Regarding the macrobenthos, the simulated increases in community biomass with increased organic matter supply are supported by studies from coastal OxAr of the Baltic Sea, but the balance with biomass losses due to simultaneously spreading hypoxia are not well quantified on the ecosystem scale (Cederwall and Elmgren, 1980, 1990; Karlson et al., 2002; Timmermann et al., 2012). Both models predict a change in community composition toward a stronger dominance of L. balthica with increased productivity. An uneven community composition could decrease the functional diversity contributing to efficient nutrient recycling as well as decrease the resilience of the benthic community to further environmental perturbations (Villnäs et al., 2013). Changes in macrofaunal and fish community composition could also have large effects on the magnitude of fish predation on benthos and therefore on the biomass flows from the benthic to the pelagic food web, with important consequences on marine ecosystem functioning (Griffiths et al., 2017).
On the other hand, continued efforts to reduce nutrient loads could lead to decreased primary production (Saraiva et al., 2019). This could mean less production of certain commercial fish, as was the case during the first half of the 20th century, when low nutrient availability limited the growth of the cod population (Eero et al., 2011). Additionally, it may have negative consequences for benthivorous birds (Laursen and Møller, 2014).
Our models assume a simple relationship between productivity and food availability for the studied groups, as the measures of productivity used here only represent the production of edible plankton, such as diatoms. In the last decades, increases in primary production have mostly been due to increases in cyanobacteria and late summer phytoplankton species in the pelagic, which can be of poor nutritional quality or even toxic (Raateoja et al., 2005; Suikkanen et al., 2007; Kahru et al., 2016), and in filamentous algae in coastal areas that reduce habitat quality for commercial fish and benthic infauna (Norkko and Bonsdorff, 1996; Troell et al., 2005). Additionally, higher water temperatures may increase respiratory losses during pelagic heterotrophic processing of organic matter, decreasing sedimentation (Griffiths et al., 2017; Tamelander et al., 2017). Thus, it is possible that both fish and BM community biomasses would decrease as response to lower food and/or habitat availability despite continued increases in primary production.
In conclusion, this study demonstrates the insights that can be gained through a combined use of complementary modeling approaches. Both models generally agree that nutrient loads are a stronger driver of pelagic and benthic community composition than warming in the study system. However, the effects on individual functional groups were sensitive to the number of groups and trophic interactions included in the model. Ecosystem models like EwE consider a larger number of such interactions and also include socio-ecological components. Thus, EwE is more capable to generate broad predictions on the state of ecosystem services under environmental change. However, flexible parameterization and more physiologically explicit forcing make BM more readily validated against experimental or field studies. In both models the strengths of predator-prey interactions were allowed to vary in response to the change in biomasses of the interacting groups. As we observed extreme biomass changes across the simulations ranging from almost extinction to a tenfold increase, we consider this an important added value compared to statistical models where no or only limited change is possible in interaction strengths (e.g., Lindegren et al., 2014; Blenckner et al., 2015). The models also showed multiple interactive effects of environmental drivers on different ecosystem components, adding to the evidence that multiple stressors should be considered in combination in empirical studies and environmental management.
The datasets generated for this study are available on request to the corresponding author.
BB, EE, and BG conceived and designed the study. EE and BB performed the model simulations, analyzed the results, and wrote the manuscript with considerable support from BG.
This study received funding from the EU, the Academy of Finland, and the Swedish Research Council Formas through BONUS (Art 185) as part of the BONUS BALTICAPP project, and from the Swedish Agency for Marine and Water Management through their grant 1.11 – Measure for Marine and Water Environment.
The authors declare that the research was conducted in the absence of any commercial or financial relationships that could be construed as a potential conflict of interest.
We thank Prof. Alf Norkko for the fruitful discussions and comments related to this study.
The Supplementary Material for this article can be found online at: https://www.frontiersin.org/articles/10.3389/fmars.2019.00492/full#supplementary-material
Atkins, J. P., Burdon, D., Elliott, M., and Gregory, A. J. (2011). Management of the marine environment: Integrating ecosystem services and societal benefits with the DPSIR framework in a systems approach. Mar. Pollut. Bull. 62, 215–226. doi: 10.1016/j.marpolbul.2010.12.012
Bartolino, V., Margonski, P., Lindegren, M., Linderholm, H. W., Cardinale, M., Rayner, D., et al. (2014). Forecasting fish stock dynamics under climate change: baltic herring (Clupea harengus) as a case study. Fish. Oceanogr. 23, 258–269. doi: 10.1111/fog.12060
Bauer, B., Horbowy, J., Rahikainen, M., Kulatska, N., Müller-Karulis, B., Tomczak, M. T., et al. (2019). Model uncertainty and simulated multispecies fisheries management advice in the Baltic Sea. PLoS One 14:22. doi: 10.1371/journal.pone.0211320
Bauer, B., Meier, H. E. M., Casini, M., Hoff, A., Margoński, P., Orio, A., et al. (2018). Reducing eutrophication increases spatial extent of communities supporting commercial fisheries: a model case study. ICES J. Mar. Sci. 75, 1306–1317. doi: 10.1093/icesjms/fsy003
Belkin, I. M. (2009). Rapid warming of large marine ecosystems. Prog. Oceanogr. 81, 207–213. doi: 10.1016/j.pocean.2009.04.011
Beukema, J. J., Dekker, R., and Jansen, J. M. (2009). Some like it cold: populations of the tellinid bivalve macoma balthica (L.) suffer in various ways from a warming climate. Mar. Ecol. Prog. Ser. 384, 135–145. doi: 10.3354/meps07952
Birchenough, S. N. R., Reiss, H., Degraer, S., Mieszkowska, N., Borja,Á., Buhl-Mortensen, L., et al. (2015). Climate change and marine benthos: a review of existing research and future directions in the North Atlantic. WIRES Clim. Chang. 6, 203–223. doi: 10.1002/wcc.330
Blackford, J. C. (2002). The influence of microphytobenthos on the Northern Adriatic ecosystem: a modelling study. Estuar. Coast. Shelf Sci. 55, 109–123. doi: 10.1006/ecss.2001.0890
Blenckner, T., Llope, M., Möllmann, C., Voss, R., Quaas, M. F., Casini, M., et al. (2015). Climate and fishing steer ecosystem regeneration to uncertain economic futures. Proc. R Soc. B Biol. Sci. 282:20142809. doi: 10.1098/rspb.2014.2809
Boyd, P. W., Collins, S., Dupont, S., Fabricius, K., Gattuso, J. P., Havenhand, J., et al. (2018). Experimental strategies to assess the biological ramifications of multiple drivers of global ocean change—a review. Glob. Chang. Biol. 24, 2239–2261. doi: 10.1111/gcb.14102
Brander, K. (2010). Impacts of climate change on fisheries. J. Mar. Syst. 79, 389–402. doi: 10.1016/j.jmarsys.2008.12.015
Breitburg, D., Levin, L. A., Oschlies, A., Grégoire, M., Chavez, F. P., Conley, D. J., et al. (2018). Declining oxygen in the global ocean and coastal waters. Science 359:eaam7240. doi: 10.1126/science.aam7240
Brochier, T., Echevin, V., Tam, J., Chaigneau, A., Goubanova, K., and Bertrand, A. (2013). Climate change scenarios experiments predict a future reduction in small pelagic fish recruitment in the humboldt current system. Glob. Chang. Biol. 19, 1841–1853. doi: 10.1111/gcb.12184
Butenschön, M., Clark, J., Aldridge, J. N., Icarus Allen, J., Artioli, Y., Blackford, J., et al. (2016). ERSEM 15.06: a generic model for marine biogeochemistry and the ecosystem dynamics of the lower trophic levels. Geosci. Model Dev. 9, 1293–1339. doi: 10.5194/gmd-9-1293-2016
Carstensen, J., Andersen, J. H., Gustafsson, B. G., and Conley, D. J. (2014). Deoxygenation of the Baltic Sea during the last century. Proc. Natl. Acad. Sci. U.S.A. 111, 5628–5633. doi: 10.1073/pnas.1323156111
Casini, M., Käll, F., Hansson, M., Plikshs, M., Baranova, T., Karlsson, O., et al. (2016). Hypoxic areas, density-dependence and food limitation drive the body condition of a heavily exploited marine fish predator. R Soc. Open Sci. 3:160416. doi: 10.1098/rsos.160416
Casini, M., Kornilovs, G., Cardinale, M., Möllmann, C., Grygiel, W., Jonsson, P., et al. (2011). Spatial and temporal density dependence regulates the condition of central Baltic Sea clupeids: compelling evidence using an extensive international acoustic survey. Popul. Ecol. 53, 511–523. doi: 10.1007/s10144-011-0269-2
Cederwall, H., and Elmgren, R. (1980). Biomass increase of benthic macrofauna demonstrates eutrophication of the Baltic Sea. Ophelia Suppl. 1, 287–304.
Cederwall, H., and Elmgren, R. (1990). Biological effects of eutrophication in the Baltic Sea, particularly the coastal zone. Ambio 19, 109–112.
Chassot, E., Mélin, F., Le Pape, O., and Gascuel, D. (2007). Bottom-up control regulates fisheries production at the scale of eco-regions in european seas. Mar. Ecol. Prog. Ser. 343, 45–55. doi: 10.3354/meps06919
Cheung, W. W. L., Lam, V. W. Y., Sarmiento, J. L., Kearney, K., Watson, R., and Pauly, D. (2009). Projecting global marine biodiversity impacts under climate change scenarios. Fish 10, 235–251. doi: 10.1111/j.1467-2979.2008.00315.x
Christensen, V., and Walters, C. J. (2004). Ecopath with Ecosim: methods, capabilities and limitations. Ecol. Model. 172, 109–139. doi: 10.1016/j.ecolmodel.2003.09.003
Christensen, V., Walters, C. J., Pauly, D., and Forrest, R. (2008). Ecopath with Ecosim Version 6. User Guide. Vancouver: University of British Columbia.
Cloern, J. E. (2001). Our evolving conceptual model of the coastal eutrophication problem. Mar. Ecol. Prog. Ser. 210, 223–253. doi: 10.3354/meps210223
Cloern, J. E., Abreu, P. C., Carstensen, J., Chauvaud, L., Elmgren, R., Grall, J., et al. (2016). Human activities and climate variability drive fast-paced change across the world’s estuarine–coastal ecosystems. Glob. Chang. Biol. 22, 513–529. doi: 10.1111/gcb.13059
Coll, M., Akoglu, E., Arreguín-Sánchez, F., Fulton, E. A., Gascuel, D., Heymans, J. J., et al. (2015). Modelling dynamic ecosystems: venturing beyond boundaries with the Ecopath approach. Rev. Fish Biol. Fish. 25, 413–424. doi: 10.1007/s11160-015-9386-x
Coll, M., Santojanni, A., Palomera, I., and Arneri, E. (2009). Food-web changes in the Adriatic Sea over the last three decades. Mar. Ecol. Prog. Ser. 381, 17–37. doi: 10.3354/meps07944
Condie, S. A., Hayes, D., Fulton, E. A., and Savina, M. (2012). Modelling ecological change over half a century in a subtropical estuary: impacts of climate change, land-use, urbanization and freshwater extraction. Mar. Ecol. Prog. Ser. 457, 43–66. doi: 10.3354/meps09718
Conley, D. J., Björck, S., Bonsdorff, E., Carstensen, J., Destouni, G., Gustafsson, B. G., et al. (2009). Hypoxia-related processes in the Baltic Sea. Environ. Sci. Technol. 43, 3412–3420. doi: 10.1021/es802762a
Conley, D. J., Carstensen, J., Aigars, J., Axe, P., Bonsdorff, E., Eremina, T., et al. (2011). Hypoxia is increasing in the coastal zone of the Baltic Sea. Environ. Sci. Technol. 45, 6777–6783. doi: 10.1021/es201212r
Crain, C. M., Kroeker, K., and Halpern, B. S. (2008). Interactive and cumulative effects of multiple human stressors in marine systems. Ecol. Lett. 11, 1304–1315. doi: 10.1111/j.1461-0248.2008.01253.x
de Mutsert, K., Cowan, J. H., and Walters, C. J. (2012). Using Ecopath with Ecosim to explore nekton community response to freshwater diversion into a louisiana estuary. Mar. Coast. Fish. 4, 104–116. doi: 10.1080/19425120.2012.672366
Ebenhöh, W., Kohlmeier, C., and Radford, P. J. (1995). The benthic biological submodel in the european regional seas ecosystem model. Neth. J. Sea Res. 33, 423–452. doi: 10.1016/0077-7579(95)90056-X
Eero, M., Andersson, H. C., Almroth-Rosell, E., and MacKenzie, B. R. (2016). Has eutrophication promoted forage fish production in the Baltic Sea? Ambio 45, 649–660. doi: 10.1007/s13280-016-0788-3
Eero, M., Hjelm, J., Behrens, J., Buchmann, K., Cardinale, M., Casini, M., et al. (2015). Eastern Baltic cod in distress: biological changes and challenges for stock assessment. ICES J. Mar. Sci. 72, 2180–2186. doi: 10.1093/icesjms/fsv109
Eero, M., MacKenzie, B. R., Köster, F. W., and Gislason, H. (2011). Multi-decadal responses of a cod (Gadus morhua) population to human-induced trophic changes, fishing, and climate. Ecol. Appl. 21, 214–226. doi: 10.1890/09-1879.1
Ehrnsten, E., Norkko, A., Timmermann, K., and Gustafsson, B. G. (2019). Benthic-pelagic coupling in coastal seas – modelling macrofaunal biomass and carbon processing in response to organic matter supply. J. Mar. Syst. 196, 36–47. doi: 10.1016/j.jmarsys.2019.04.003
Ejdung, G., and Bonsdorff, E. (1992). Predation on the bivalve Macoma balthica by the isopod saduria entomon: laboratory and field experiments. Mar. Ecol. Prog. Ser. 88, 207–214. doi: 10.3354/meps088207
Englund, G., and Leonardsson, K. (2008). Scaling up the functional response for spatially heterogeneous systems. Ecol. Lett. 11, 440–449. doi: 10.1111/j.1461-0248.2008.01159.x
Englund, G., Rydberg, C., and Leonardsson, K. (2008). Long-term variation of link strength in a simple benthic food web. J. Anim. Ecol. 77, 883–890. doi: 10.1111/j.1365-2656.2008.01404.x
Evans, L. S., Hicks, C. C., Fidelman, P., Tobin, R. C., and Perry, A. L. (2013). Future scenarios as a research tool: investigating climate change impacts, adaptation options and outcomes for the great barrier reef, australia. Hum. Ecol. 41, 841–857. doi: 10.1007/s10745-013-9601-00
Fulton, E. A. (2010). Approaches to end-to-end ecosystem models. J. Mar. Syst. 81, 171–183. doi: 10.1016/j.jmarsys.2009.12.012
Fulton, E. A., Parslow, J. S., Smith, A. D. M., and Johnson, C. R. (2004). Biogeochemical marine ecosystem models II: the effect of physiological detail on model performance. Ecol. Model. 173, 371–406. doi: 10.1016/j.ecolmodel.2003.09.024
Fulton, E. A., Smith, A. D. M., and Johnson, C. R. (2003). Mortality and predation in ecosystem models: is it important how these are expressed? Ecol. Model. 169, 157–178. doi: 10.1016/S0304-3800(03)00268-0
Gammal, J., Norkko, J., Pilditch, C. A., and Norkko, A. (2017). Coastal hypoxia and the importance of benthic macrofauna communities for ecosystem functioning. Estuaries Coasts 40, 457–468. doi: 10.1007/s12237-016-0152-7
Gårdmark, A., Lindegren, M., Neuenfeldt, S., Blenckner, T., Heikinheimo, O., Müller-Karulis, B., et al. (2013). Biological ensemble modeling to evaluate potential futures of living marine resources. Ecol. Appl. 23, 742–754. doi: 10.1890/12-0267.1
Gogina, M., Nygård, H., Blomqvist, M., Daunys, D., Josefson, A. B., Kotta, J., et al. (2016). The Baltic Sea scale inventory of benthic faunal communities. ICES J. Mar. Sci. 73, 1196–1213. doi: 10.1093/icesjms/fsv265
Gogina, M., and Zettler, M. L. (2010). Diversity and distribution of benthic macrofauna in the Baltic Sea. J. Sea Res. 64, 313–321. doi: 10.1016/j.seares.2010.04.005
Gray, J. S., Wu, R. S., and Or, Y. Y. (2002). Effects of hypoxia and organic enrichment on the coastal marine environment. Mar. Ecol. Prog. Ser. 238, 249–279. doi: 10.3354/meps238249
Griffiths, J. R., Kadin, M., Nascimento, F. J. A., Tamelander, T., Törnroos, A., Bonaglia, S., et al. (2017). The importance of benthic-pelagic coupling for marine ecosystem functioning in a changing world. Glob. Chang. Biol. 23, 2179–2196. doi: 10.1111/gcb.13642
Gustafsson, B. G., Schenk, F., Blenckner, T., Eilola, K., Meier, H. E. M., Müller-Karulis, B., et al. (2012). Reconstructing the development of Baltic Sea eutrophication 1850-2006. Ambio 41, 534–548. doi: 10.1007/s13280-012-0318-x
Hakala, T., Viitasalo, M., Rita, H., Aro, E., Flinkman, J., and Vuorinen, I. (2003). Temporal and spatial variation in the growth rates of Baltic herring (clupea harengus membras L.) larvae during summer. Mar. Biol. 142, 25–33. doi: 10.1007/s00227-002-0933-3
Halpern, B. S., Walbridge, S., Selkoe, K. A., Kappel, C. V., Micheli, F., D’Agrosa, C., et al. (2008). A global map of human impact on marine ecosystems. Science 319, 948–952. doi: 10.1126/science.1149345
Holling, C. S. (1959). Some characteristics of simple types of predation and parasitism. Can. Entomol. 91, 385–398. doi: 10.4039/Ent91385-7
Holt, R. D. (1977). Predation, apparent competition, and the structure of prey communities. Theor. Popul. Biol. 12, 197–229. doi: 10.1016/0040-5809(77)90042-9
Holt, R. D., and Lawton, J. H. (1994). The ecological consequences of shared natural enemies. Annu. Rev. Ecol. Syst. 25, 495–520. doi: 10.1146/annurev.es.25.110194.002431
Jernelöv, A., and Rosenberg, R. (1976). Stress tolerance of ecosystems. Environ. Conserv. 3, 43–46. doi: 10.1017/s0376892900017732
Jokinen, H., Wennhage, H., Lappalainen, A., Ådjers, K., Rask, M., and Norkko, A. (2015). Decline of flounder (Platichthys flesus (L.) at the margin of the species’ distribution range. J. Sea Res. 105, 1–9. doi: 10.1016/j.seares.2015.08.001
Kahru, M., Elmgren, R., and Savchuk, O. P. (2016). Changing seasonality of the Baltic Sea. Biogeosciences 13, 1009–1018. doi: 10.5194/bg-13-1009-16
Karlson, K., Rosenberg, R., and Bonsdorff, E. (2002). Temporal and spatial large-scale effects of eutrophication and oxygen deficiency on benthic fauna in scandinavian and baltic waters: a review. Oceanogr. Mar. Biol. 40, 427–489. doi: 10.1201/9780203180594.ch8
Kautsky, N., and Evans, S. (1987). Role of biodepostion by Mytilus edulis in the circulation of matter and nutrients in a Baltic coastal ecosystem. Mar. Ecol. Prog. Ser. 38, 201–212. doi: 10.3354/meps038201
Köster, F. W., Huwer, B., Hinrichsen, H. H., Neumann, V., Makarchouk, A., Eero, M., et al. (2017). Eastern Baltic cod recruitment revisited - dynamics and impacting factors. ICES J. Mar. Sci. 74, 3–19. doi: 10.1093/icesjms/fsw172
Laursen, K., and Møller, A. P. (2014). Long-term changes in nutrients and mussel stocks are related to numbers of breeding eiders Somateria mollissima at a large baltic colony. PLoS One 9:e95851. doi: 10.1371/journal.pone.0095851
Lessin, G., Bruggeman, J., McNeill, C. L., and Widdicombe, S. (2019). Time scales of benthic macrofaunal response to pelagic production differ between major feeding groups. Front. Mar. Sci. 6:15. doi: 10.3389/fmars.2019.00015
Libralato,A., and Pranovi, F. (2015).Modeling species invasions using thermal and trophic niche dynamics under climate change. Front. Mar. Sci. 2, 1–14. doi: 10.3389/fmars.2015.00029
Lindegren, M., Andersen, K. H., Casini, M., Neuenfeldt, S., Lindegren, M., Andersen, K. H., et al. (2014). A metacommunity perspective on source — sink dynamics and management: the Baltic Sea as a case study. Ecol. Appl. 24, 1820–1832. doi: 10.1890/13-0566.1
MacKenzie, B. R., Hinrichsen, H. H., Plikshs, M., Wieland, K., and Zezera, A. S. (2000). Quantifying environmental heterogeneity: habitat size necessary for successful development of cod Gadus morhua eggs in the Baltic Sea. Mar. Ecol. Prog. Ser. 193, 143–156. doi: 10.3354/meps193143
Margonski, P., Hansson, S., Tomczak, M. T., and Grzebielec, R. (2010). Climate influence on Baltic cod, sprat, and herring stock-recruitment relationships. Prog. Oceanogr. 87, 277–288. doi: 10.1016/j.pocean.2010.08.003
Meier, H. E. M., Eilola, K., and Almroth, E. (2011). Climate-related changes in marine ecosystems simulated with a 3-dimensional coupled physical-biogeochemical model of the Baltic Sea. Clim. Res. 48, 31–55. doi: 10.3354/cr00968
Meier, H. E. M., Hordoir, R., Andersson, H. C., Dieterich, C., Eilola, K., Gustafsson, B. G., et al. (2012a). Modeling the combined impact of changing climate and changing nutrient loads on the Baltic Sea environment in an ensemble of transient simulations for 1961–2099. Clim. Dyn. 39, 2421–2441. doi: 10.1007/s00382-012-1339-7
Meier, H. E. M., Müller-Karulis, B., Andersson, H. C., Dieterich, C., Eilola, K., Gustafsson, B. G., et al. (2012b). Impact of climate change on ecological quality indicators and biogeochemical fluxes in the baltic sea: a multi-model ensemble study. Ambio 41, 558–573. doi: 10.1007/s13280-012-0320-3
Morato, T., Bulman, C., and Pitcher, T. J. (2009). Deep-sea research II modelled effects of primary and secondary production enhancement by seamounts on local fish stocks. Deep Sea Res. Part 2 Top. Stud. Oceanogr. 56, 2713–2719. doi: 10.1016/j.dsr2.2008.12.029
Niiranen, S., Yletyinen, J., Tomczak, M. T., Blenckner, T., Hjerne, O., MacKenzie, B. R., et al. (2013). Combined effects of global climate change and regional ecosystem drivers on an exploited marine food web. Glob. Chang. Biol. 19, 3327–3342. doi: 10.1111/gcb.12309
Norkko, A., and Bonsdorff, E. (1996). Population responses of coastal zoobenthos to stress induced by drifting algal mats. Mar. Ecol. Prog. Ser. 140, 141–151. doi: 10.3354/meps140141
Ojaveer, H., Jaanus, A., Mackenzie, B. R., Martin, G., Olenin, S., Telesh, I., et al. (2010). Status of biodiversity in the Baltic Sea. PLoS One 5:e12467. doi: 10.1371/journal.pone.0012467
Österblom, H., Merrie, A., Metian, M., Boonstra, W. J., Blenckner, T., Watson, J. R., et al. (2013). Modeling social–ecological scenarios in marine systems. Bioscience 63, 735–744. doi: 10.1525/bio.2013.63.9.9
Pascual, U., Palomo, I., Adams, W. M., Chan, K. M. A., Daw, T. M., Garmendia, E., et al. (2017). Off-stage ecosystem service burdens: a blind spot for global sustainability. Environ. Res. Lett. 12:75001.
Pauly, D., Christensen, V., and Walters, C. (2000). Ecopath, Ecosim, and Ecospace as tools for evaluating ecosystem impact of fisheries. ICES J. Mar. Sci. 57, 697–706. doi: 10.1006/jmsc.2000.0726
Plagányi, ÉE. (2007). Models for an Ecosystem Approach to Fisheries. Rome: Food and Agriculture Organisation.
Poloczanska, E. S., Brown, C. J., Sydeman, W. J., Kiessling, W., Schoeman, D. S., Moore, P. J., et al. (2013). Global imprint of climate change on marine life. Nat. Clim. Chang. 3, 919–925. doi: 10.1038/nclimate1958
Raateoja, M., Seppälä, J., Kuosa, H., and Myrberg, K. (2005). Recent changes in trophic state of the Baltic Sea along SW coast of finland. Ambio 34:188. doi: 10.1639/0044-74472005034
Radeloff, V. C., Williams, J. W., Bateman, B. L., Burke, K. D., Carter, S. K., Childress, E. S., et al. (2015). The rise of novelty in ecosystems. Ecol. Appl. 25, 2051–2068. doi: 10.1890/14-1781.1
Richardson, A. J., Brown, C. J., Brander, K., Bruno, J. F., Buckley, L., Burrows, M. T., et al. (2012). Climate change and marine life. Biol. Lett. 8, 907–909. doi: 10.1098/rsbl.2012.0530
Rousi, H., Laine, A. O., Peltonen, H., Kangas, P., Andersin, A.-B., Rissanen, J., et al. (2013). Long-term changes in coastal zoobenthos in the northern Baltic Sea: the role of abiotic environmental factors. ICES J. Mar. Sci. 70, 440–451. doi: 10.1093/icesjms/fss197
Runting, R. K., Bryan, B. A., Dee, L. E., Maseyk, F. J. F., Mandle, L., Hamel, P., et al. (2017). Incorporating climate change into ecosystem service assessments and decisions: a review. Glob. Chang. Biol. 23, 28–41. doi: 10.1111/gcb.13457
Saraiva, S., Markus Meier, H. E., Andersson, H., Höglund, A., Dieterich, C., Gröger, M., et al. (2019). Baltic Sea ecosystem response to various nutrient load scenarios in present and future climates. Clim. Dyn. 52, 3369–3387. doi: 10.1007/s00382-018-4330-0
Saraiva, S., Meier, H. E. M., Andersson, H., Höglund, A., Dieterich, C., Gröger, M., et al. (2018). Uncertainties in projections of the baltic sea ecosystem driven by an ensemble of global climate models. Front. Earth Sci. 6:244. doi: 10.3389/feart.2018.00244
Sarvala, J. (1971). Ecology of Harmothoe sarsi (Malmgren) (Polychaeta, Polynoidae) in the northern Baltic area. Ann. Zool. Fenn. 8, 231–309.
Savchuk, O. P., Gustafsson, B. G., and Müller-Karulis, B. (2012). BALTSEM - a Marine Model for Desicion Support within the Baltic Sea Region. Sweden, SE: Stockholm University, BNI Techincal report No 7.
Segerstråle, S. G. (1962). The immigration and prehistory of the glacial relicts of Eurasia and North America. A survey and discussion of modern views. Int. Rev. Der Gesamten Hydrobiol. Hydrogr. 47, 1–25. doi: 10.1002/iroh.19620470102
Seidl, R. (2017). To model or not to model, that is no longer the question for ecologists. Ecosystems 20, 222–228. doi: 10.1007/s10021-016-0068-x
Snoeijs-Leijonmalm, P. (2017). “Patterns of biodiversity,” in Biological Oceanography of the Baltic Sea, eds P. Snoeijs-Leijonmalm, H. Schubert, and T. Radziejewska (Dordrecht: Springer), doi: 10.1007/978-94-007-0668-2-4
Sommer, U., Adrian, R., Bauer, B., and Winder, M. (2012). The response of temperate aquatic ecosystems to global warming: novel insights from a multidisciplinary project. Mar. Biol. 159, 2367–2377. doi: 10.1007/s00227-012-2085-4
Sparrevik, E., and Leonardsson, K. (1999). Direct and indirect effects of predation by Saduria entomon (Isopoda) on the size-structure of Monoporeia affinis (Amphipoda). Oecologia 120, 77–86. doi: 10.1007/s004420050835
Suikkanen, S., Laamanen, M., and Huttunen, M. (2007). Long-term changes in summer phytoplankton communities of the open northern Baltic Sea. Estuar. Coast. Shelf Sci. 71, 580–592. doi: 10.1016/j.ecss.2006.09.004
Tamelander, T., Spilling, K., and Winder, M. (2017). Organic matter export to the seafloor in the Baltic Sea: drivers of change and future projections. Ambio 46, 842–851. doi: 10.1007/s13280-017-0930-x
Timmermann, K., Norkko, J., Janas, U., Norkko, A., Gustafsson, B. G., and Bonsdorff, E. (2012). Modelling macrofaunal biomass in relation to hypoxia and nutrient loading. J. Mar. Syst. 10, 60–69. doi: 10.1016/j.jmarsys.2012.06.001
Tomczak, M. T., Niiranen, S., Hjerne, O., and Blenckner, T. (2012). Ecosystem flow dynamics in the Baltic proper-using a multi-trophic dataset as a basis for food-web modelling. Ecol. Model. 230, 123–147. doi: 10.1016/j.ecolmodel.2011.12.014
Troell, M., Pihl, L., Rönnbäck, P., Wennhage, H., Söderqvist, T., and Kautsky, N. (2005). Regime shifts and ecosystem services in Swedish coastal soft bottom habitats: when resilience is undesirable. Ecol. Soc. 10:30. doi: 10.5751/ES-01374-100130
Uusitalo, L., Korpinen, S., Andersen, J. H., Niiranen, S., Valanko, S., Heiskanen, A. S., et al. (2016). Exploring methods for predicting multiple pressures on ecosystem recovery: a case study on marine eutrophication and fisheries. Cont. Shelf Res. 121, 48–60. doi: 10.1016/j.csr.2015.11.002
Vaquer-Sunyer, R., and Duarte, C. M. (2011). Temperature effects on oxygen thresholds for hypoxia in marine benthic organisms. Glob. Chang. Biol. 17, 1788–1797. doi: 10.1111/j.1365-2486.2010.02343.x
Villnäs, A., Norkko, J., Hietanen, S., Josefson, A. B., Lukkari, K., and Norkko, A. (2013). The role of recurrent disturbances for ecosystem multifunctionality. Ecology 94, 2275–2287. doi: 10.1890/12-1716.1
Vuorinen, I., Hänninen, J., Rajasilta, M., Laine, P., Eklund, J., Montesino-Pouzols, F., et al. (2015). Scenario simulations of future salinity and ecological consequences in the Baltic Sea and adjacent North Sea areas-implications for environmental monitoring. Ecol. Indic. 50, 196–205. doi: 10.1016/j.ecolind.2014.10.019
Keywords: interacting stressors, benthic fauna, fish, Baltic Sea, sensitivity analysis, Ecopath with Ecosim, numerical model
Citation: Ehrnsten E, Bauer B and Gustafsson BG (2019) Combined Effects of Environmental Drivers on Marine Trophic Groups – A Systematic Model Comparison. Front. Mar. Sci. 6:492. doi: 10.3389/fmars.2019.00492
Received: 17 May 2019; Accepted: 22 July 2019;
Published: 02 August 2019.
Edited by:
Cosimo Solidoro, Istituto Nazionale di Oceanografia e di Geofisica Sperimentale (OGS), ItalyReviewed by:
Anna Törnroos, Åbo Akademi University, FinlandCopyright © 2019 Ehrnsten, Bauer and Gustafsson. This is an open-access article distributed under the terms of the Creative Commons Attribution License (CC BY). The use, distribution or reproduction in other forums is permitted, provided the original author(s) and the copyright owner(s) are credited and that the original publication in this journal is cited, in accordance with accepted academic practice. No use, distribution or reproduction is permitted which does not comply with these terms.
*Correspondence: Eva Ehrnsten, ZXZhLmVocm5zdGVuQHN1LnNl
†These authors have contributed equally to this work
Disclaimer: All claims expressed in this article are solely those of the authors and do not necessarily represent those of their affiliated organizations, or those of the publisher, the editors and the reviewers. Any product that may be evaluated in this article or claim that may be made by its manufacturer is not guaranteed or endorsed by the publisher.
Research integrity at Frontiers
Learn more about the work of our research integrity team to safeguard the quality of each article we publish.