- 1US Integrated Ocean Observing System Program, National Oceanic and Atmospheric Administration/National Ocean Service, Silver Spring, MD, United States
- 2PO.DAAC, NASA Jet Propulsion Laboratory, Pasadena, CA, United States
- 3Institute of Marine Research, Bergen, Norway
- 4Centre for the Study of Marine Systems, Patagonian National Research Centre, National Scientific and Technical Research Council, Puerto Madryn, Argentina
- 5Joint Institute for the Study of the Atmosphere and Ocean, University of Washington, Seattle, WA, United States
- 6National Centers for Environmental Information, National Oceanic and Atmospheric Administration, Silver Spring, MD, United States
- 7GODAE Ocean Services, Melbourne, VIC, Australia
- 8Institute of Marine Research, Norwegian Marine Data Centre, Bergen, Norway
- 9University Corporation for Atmospheric Research/Unidata, Boulder, CO, United States
The data management landscape associated with the Global Ocean Observing System is distributed, complex, and only loosely coordinated. Yet interoperability across this distributed landscape is essential to enable data to be reused, preserved, and integrated and to minimize costs in the process. A building block for a distributed system in which component systems can exchange and understand information is standardization of data formats, distribution protocols, and metadata. By reviewing several data management use cases we attempt to characterize the current state of ocean data interoperability and make suggestions for continued evolution of the interoperability standards underpinning the data system. We reaffirm the technical data standard recommendations from previous OceanObs conferences and suggest incremental improvements to them that can help the GOOS data system address the significant challenges that remain in order to develop a truly multidisciplinary data system.
Introduction
Ocean observing programs of varying geographic or disciplinary scope have been coordinating globally for decades in an effort to develop an efficient, sustainable, and complete Global Ocean Observing System (GOOS) of systems. Many efforts focus on national or regional priorities that are often limited in geographical scope. Others are globally focused but constrained by sampling methodologies such as through the use of profiling floats [e.g., Argo (Riser et al., 2016)] or by sampling geometry such as time series measurement at one location [e.g., OceanSITES (Send et al., 2010)]. These programs are independently governed and funded and can serve different stakeholders, though they often have commonalities. Addressing the needs of the individual stakeholders influences how the observing programs design the information systems that manage and distribute the observations. This individuality of the stakeholders leads to individuality of the information systems, which contributes to a lack of interoperability across systems.
The Framework for Ocean Observation (FOO) (Lindstrom et al., 2012) defines a set of key processes to guide the ocean observing community toward the establishment of an integrated, sustained ocean observing system with fit-for-purpose data/information streams for societal and scientific benefit. It provides a set of overarching principles and conceptual structures useful in guiding the coherent development of ocean observing systems and coordinating their supporting data infrastructures in a manner that mitigates the aforementioned structural issues. The FOO is based on observing system success stories and best practices, a collaboration-focused governance structure, and the concept of Essential Ocean Variables (EOVs). Global progress is measured through maturity/readiness levels which are central organizing principles for the establishment of coherent requirements for observing system elements consistent with systems engineering approaches. The FOO also highlights the critical role of data management and data interoperability standards in addressing the enormous challenge of open access to harmonized, integrated data across a very diverse ocean observing “system of systems,” comprised of multi-scale, multi-platform/sensor observations supporting various applications and science domains. Given the associated highly heterogeneous data landscapes and data management infrastructures, “the desire to ‘measure once and use many times’ requires that standards be developed and adopted by observing components.” Data interoperability across the data lifecycle and information value chain, from raw observational data through modeled synthesis products, is seen as a foundational element of ocean observing systems that are efficient and fit for purpose. Efficient, fit for purpose, observing systems provide useful inputs to science-based, data-driven decision support processes of societal importance, relevant to ecosystem management, food security, maritime safety, energy, climate monitoring, and other emerging areas of the Blue economy. The FOO additionally recognizes the role of international entities such as the WMO-IOC Joint Technical Commission for Oceanography and Marine Meteorology (JCOMM), and the International Oceanographic Data and Information Exchange (IODE) in coordinating such data management efforts for physical, geological, chemical, and biological observing system elements of the GOOS, leveraging also work done by Earth science data standards authorities. Finally, the Framework identifies the importance of education, outreach, and capacity building, including in the area of data interoperability standards and best practices for oceanographic data management.
While the Framework acknowledges the importance of data interoperability and provides a structure for global collaboration toward better interoperability, it does not describe specific steps, tools, or actions to be taken. In this paper we focus on data interoperability across the global ocean observing community. We first describe this global community and define interoperability between community members. We then examine several use cases that demonstrate various levels of interoperability in an effort to distill best practices that can be widely adopted. The use cases also help demonstrate limitations of our current understanding of data interoperability that we suggest can be opportunities for future work or investment. Finally, we close with listing recommendations for evolving the information management systems supporting the GOOS.
Significance of Data Interoperability for Ocean Observing
Defining the GOOS Data System
The GOOS1 is a global system for sustained, interdisciplinary observations of the ocean comprising the oceanographic component of the Global Earth Observing System of Systems (GEOSS)2. It is a coordinated but highly decentralized system and organizational network overseeing the planning and implementation of observations for the world’s oceans, aimed ultimately at delivering data, related services, and information products in support of research and applications. By necessity and design, it leverages heavily from regional and national programs and infrastructures but also from community partnerships that are related to specific observing system elements under the umbrella of JCOMM. Data is managed in a federated manner and accessible via regional observing system nodes, JCOMM partnership program data assembly centers (DACs), space agency satellite data centers, integrated DACs and designated IODE/International Council for Science (ICSU) World Data Centers (WDC) (Figure 1). Data offerings are diverse in type, coverage, and extent. They include multi-variate observational data from in situ and remote sensing platforms that are sustained, and often available in near real-time, and data from research field campaigns that are more ephemeral in nature. Data from numerical models are also an important data type that differ from observational in some important ways (e.g., model data are less diverse in structure but typically much higher volume). The ability to integrate across such a distributed and complex, multi-agency data management landscape, in support of GOOS, hinges critically on the widespread adoption of data interoperability standards.
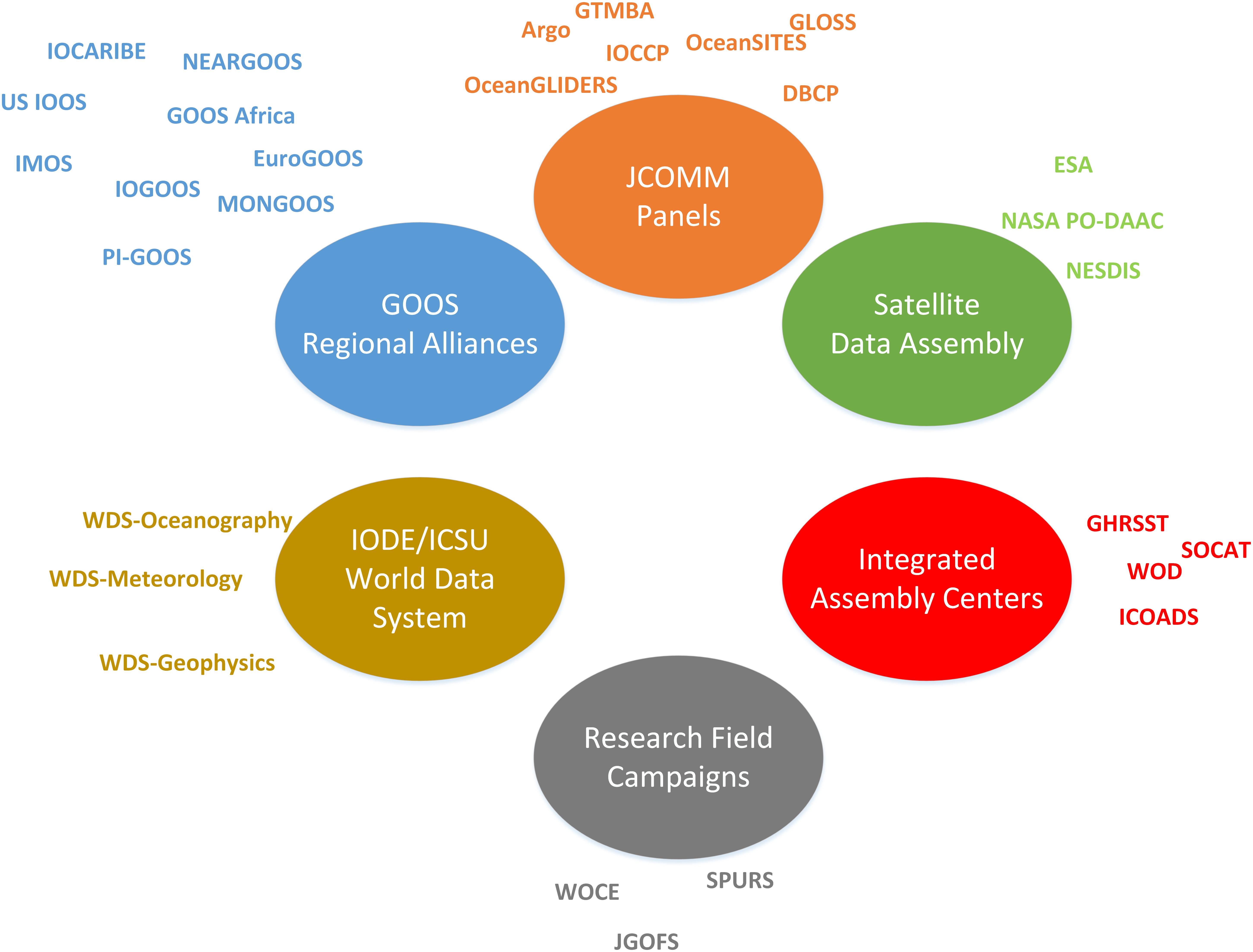
Figure 1. The GOOS Data System comprised of many efforts that are funded and coordinated nationally and internationally. To ensure the successful information sharing between the component systems is enhanced through (i) data interoperability standards and protocols for measurements, data exchange and data management, (ii) ensure effective and efficient linkage among elements (measurements, data management and communications, data analysis), (iii) formulate rules of engagement for establishing the operational elements of the global ocean.
Through national efforts such as the U.S. Integrated Ocean Observing System, or through GOOS Regional Alliances like EuroGOOS, system are being built that integrate data from across numerous observing system elements in an attempt to provide integrated products to regional or local customers. These efforts frequently result in more customer focused relationships and products. However, integrating across different platforms taxes the data interoperability standards which are often not general enough to span use cases across platforms and across disciplines. Maintaining a strong standards foundation while still providing flexible enough tools to tailor products to local customer needs is an ongoing challenge for the global ocean observations community.
Why Is Interoperability Important?
While we are unaware of any single effort to design and construct a monolithic GOOS Data System, in this paper we use this term to collectively and generically refer to the independent but often coordinated efforts across all of the organizations in Figure 1. It is a distributed data system with responsibilities for different stages of the data management lifecycle spread across myriad organizations. We make a few assumptions about the principles that each of the component efforts share. If these assumptions are true, they serve as design principles for the GOOS Data System. Enhancing data interoperability then becomes a means to achieve these design principles. There are efforts that will not ascribe to these principles and for those efforts, we must exclude them from consideration in the GOOS Data System for pragmatic reasons. However, we believe that the trend toward embracing these principles will increase as policy and technology evolve.
Data Reuse
Data reuse is one of the Guiding Principles for the FOOs (see section “Web Service Based Data Exchange: Data Access Services,” Lindstrom et al., 2012). The measure once/use many times philosophy underpins the entire FOO and is increasingly driving policy at federal levels. For example, the United States Government has committed to an Open Data policy3 predicated on the notion that open accessible data will spur innovation and lead to efficiencies. Examining the global policy landscape for data sharing is beyond the scope of this paper but we note that increased data sharing may be motivated by recognition that reusing data has potential economic benefits and encourage open data sharing across the GOOS Data System.
Data Preservation
A further, often unappreciated benefit stemming from Earth science data standards adoption is that long-term preservation of archive quality data with associated metadata and provenance information is facilitated. This ensures protection not only of significant public investments in costly data collection but also preservation of observations that are unique in time and space and thus irreplaceable. Understanding and alleviating underlying challenges and constraints to widespread adoption of applicable data standards, whether technical, capacity or resource related, will be central to ensuring sustained contributions to and effective usage of a vibrant and integrated ocean observing system data commons.
Data Integration
Many data management efforts are organized around an observing platform such as research vessels, profiling floats (Argo4) or particular satellites (e.g., AVHRR Pathfinder5). This organization is sensible because in the early stages of the data lifecycle, there are efficiencies to be gained by managing all data from a single platform in a single place. However, for scientific or operational applications, stakeholders are often more interested in obtaining data organized around a sampling method (e.g., all ocean profile data in World Ocean Database6) or by EOV (e.g., Sea Surface Temperature data from the Group for High Resolution Sea Surface Temperature, GHRSST7). Creating these integrated data sets is made easier if all of the source data sets are available in interoperable standards compliant formats. It is worth noting that Argo, World Ocean Database (WOD), and GHRSST have all adopted common conventions for representing data and metadata in file formats that enhance system wide interoperability.
Minimizing Lifecycle Costs
The importance of data interoperability for the development of an operationally sustainable GOOS lies in the significant cost saving and scalability that automated data discovery, access and processing pipelines provide. Data conforming to established Earth science data interoperability standards have the necessary structural, syntactic and semantic characteristics rendering them searchable, more easily integrated within software systems and amongst themselves, and generally more usable. Standards compliance increases the likelihood that custom, unmaintainable and invariably costly to implement one-off solutions for handling of data are averted.
Software development is an essential and expensive part of the data management lifecycle. Widespread adoption of data standards encourages the development of generic rather than single purpose software tools. Generic tools, especially when developed using Open Source Software principles and methods, can attract more developers because they can focus their time as a team working on a common tool rather than individually on their particular application. This often results in higher quality, better documented, and better tested software which has implications for the efficiency of the global community. Open Source software policies should accompany Open Data Sharing Policies in the GOOS governance framework. This recommendation should not be adopted without consideration of long term implications. For example, the legal framework for software licensing is complex and potentially conflicts with some institutional policies. Further, open source software may not have dedicated technical support. In sum, the authors believe that Open Source Software is a net positive for the community but acknowledge that some caution is warranted.
FAIR Principles
These considerations are effectively embodied by the FAIR guiding principles for the improved data management, stewardship and accessibility of science that have recently been advanced (Wilkinson et al., 2016) and that are gaining increased traction. FAIR emphasizes: (1) Findable data, with machine-readable metadata essential for automated discovery and utilization of data and metadata by software services. (2) Access to data and persistent metadata records using open/free, standards-based protocols that support authentication. (3) Interoperable such that data are machine interpretable and can be automatically combined with other data, leveraging standard vocabularies and ontologies for knowledge representation accessible via semantic Web technologies. (4) Reusable data: well-characterized, rich community metadata enabling traceable, reproducible, and easily integrated data in support of research and applications into the future. FAIR provides a high-level conceptual framework useful to the design of contemporary information systems in support of ocean observation, the more detailed technical underpinnings of which are based on widely applied Earth science data interoperability standards that we now describe. While the focus of this paper is on data interoperability, it is difficult to decouple Interoperability from the other elements of FAIRness when describing the GOOS Data System current and future states (Tanhua et al., 2019).
Defining Interoperability for Ocean Data Stakeholders
Interoperability in a general sense can be defined as the “degree to which two or more systems, products or components can exchange information and use the information that has been exchanged” (ISO/IEC/IEEE, 2017). It is the ability by which coupled software systems can communicate and exchange data via common formats and protocols and also meaningfully interpret and reproducibly act on exchanged data. This definition prompts an important recognition: interoperability is a characteristic of a relationship between two or more systems. It is not a characteristic of a single data file or data set. The two (or more) systems in this relationships are both stakeholders in the exchange of information. Generically, these stakeholders can be classified as Data Producers/Providers or Data Consumers.
Data Producers/Providers are responsible for generating data, typically through observation or simulation and make it available to a consumer. The scope of these activities can be local to an individual Principal Investigator conducting a lone experiment, or globally coordinated efforts like the World Ocean Circulation Experiment of the 1990s or the Argo program of today. These stakeholders have common objectives, to plan and design their experimental or sampling scheme so that the data addresses the scientific or operational objective. As noted above, we also assume that these Producers/Providers subscribe to the principles listed above. They wish to see their data maximally reused, preserved for the future, combined with other data into integrated products, and they want to minimize costs in the process. If Data Producers/Providers do not subscribe to these common principles, then the significant overhead of adopting and using global data interoperability standards is a resource drain that is often un- or under-funded. It is a requirement for national and international governance groups and funding agencies to encourage adherence to these common goals and to resource the work adequately if the GOOS Data System is to become more interoperable. However, it is also critical for the scientific Information Management community to help bridge the gap between data producers and the relevant metadata and data standards by providing tools that can improve conformance of their data to well-established data interoperability standards. This will facilitate integration of data in a range of software applications and Web services including data distribution, processing, modeling and visualization capabilities, thus enabling more widespread usage of data.
Data Consumers require data to solve problems or make decisions. They could be interested in obtaining data for scientific study, for assimilation into a numerical model, or to create a web based visualization. A scientist likely requires all available data and extensive metadata to ensure that they understand as much as possible about how the data was generated. They are interested in ingesting the data into their analysis tool of choice and may be willing to wait to download enormous file collections. A web developer on the other hand may only require a small subset of a data set but they need it on demand with minimal latency. For the scientist, downloading a large collection of files from an FTP server may be an acceptable access method while the web developer requires a web based Application Programming Interface (API) with flexible query methods and extremely fast response. By observing and documenting different types of Provider – Consumer relationships that are successful, we can generalize strategies that enhance the likelihood that future relationships are successful – i.e., interoperable. The following sections describe principles or design patterns that have underscored successful interoperable Provider – Consumer relationships and offer background for the Use Cases that follow.
Common Elements of Data Interoperability
Typical Provider-Consumer exchanges occur when a file subset or a file are transferred from one system to another. The ability of the consumer system to understand and use the information received has both syntactic and semantic elements. Syntactic elements describe the Consumers ability to decode the electronic data file and accurately access the objects within the file. Semantic elements describe the Consumer’s ability to understand the data objects. Simple semantics include understanding parameter names and units while more complex semantics allow for translating between colloquial names for species types and standardized registries of species names like the World Register of Marine Species (WoRMS). Interoperability standards for Earth science data are comprised of three core elements (Figure 2):
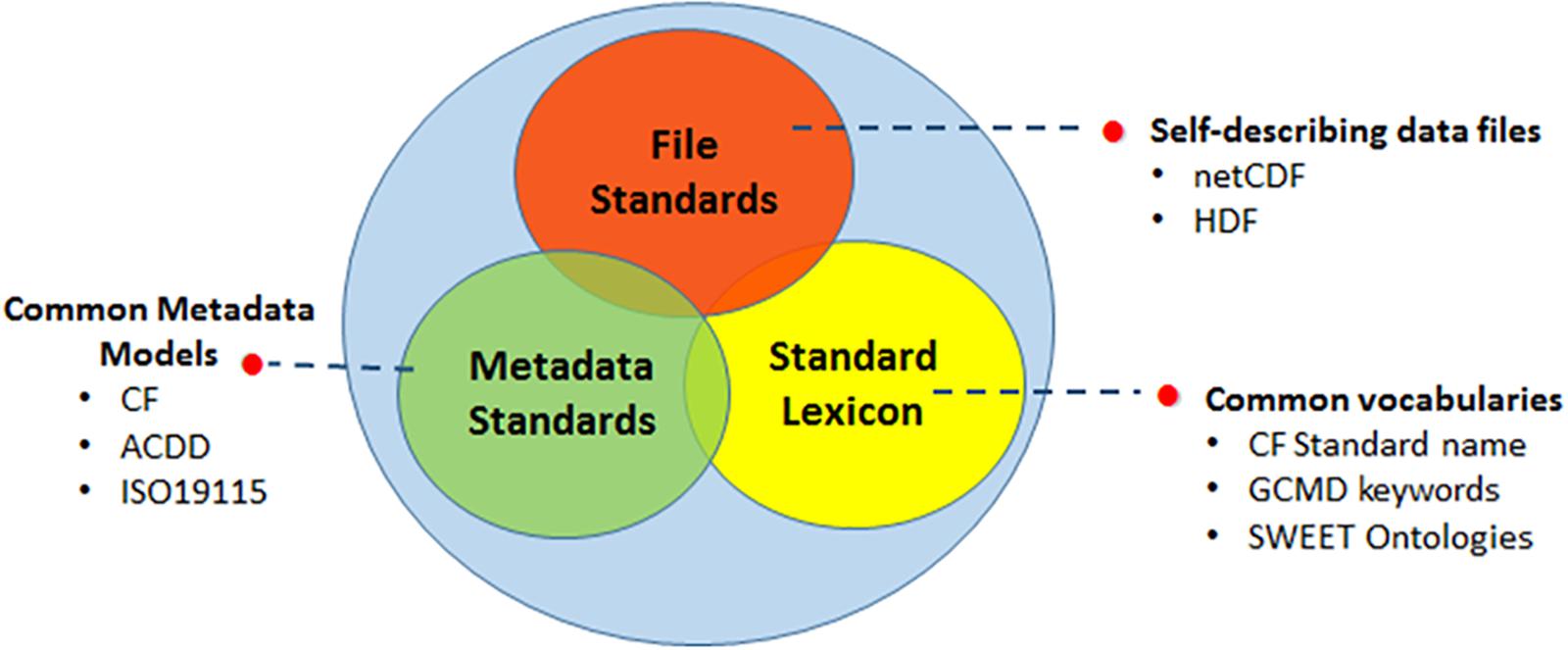
Figure 2. The three main categories of data interoperability standards: file standards, metadata standards and standard lexicon, allow integration of data in a range of software applications and Web services including data distribution, processing, modeling and visualization capabilities, thus enabling more widespread usage of data.
• File standards, based on self-describing scientific data file formats;
• Common data and metadata models;
• Controlled vocabularies and ontologies that define terms, concepts and their relationships for a given science domain.
Much of the emphasis on the data file stems historically from its ubiquitous usage as the storage and exchange medium for science instrument data, including from ocean observing systems. Widely used scientific data file formats such as HDF8 and netCDF9 provide compact, binary formats optimized for efficient storage and access of large, complex datasets. They include features such as internal compression, and support hierarchical structuring of data within files. Significantly, from a data interoperability and data preservation perspective, they are, or can be made to be, self-describing. A self-describing file includes metadata that describes both the data and data structures comprising a file. Further, self-describing files minimize or eliminate the need for external sources of information (e.g., quality control code tables) allowing the file stand alone and be understood by a consumer. Common data models (CDMs) are important enablers of interoperability because they allow generic software to predict the structure of these self-describing files and access the encoded data as the author intended. Interoperability has been further facilitated by the parallel community development of metadata models supporting a broad range of geospatial Earth science data that have been built around or are compatible with the CDM. These include the Climate Forecast (CF) conventions10, the Attribute Conventions for Data Discovery (ACDD)11, and the ISO 1911512 standard metadata schema for geographic information and services. These conventions provide a standard set of attributes and technical framework for the encoding of metadata and data in self-describing data files. Such common data and metadata models have in turn promoted the development of an ecosystem of broadly used, open source software libraries, APIs, web service standards and Web server technologies. Hankin et al. (2010) describe the important role that the Climate and Forecast Conventions and the netCDF file format fill in our global data management and dissemination framework. In the decade since OceanObs ‘09, CF/netCDF has been further cemented as the de facto standard for file based storage and exchange of in situ, remotely sensed, and model generated data. Further progress since OceanObs ‘09 is nicely summarized in Tanhua et al. (2019).
The third ingredient for data to be interoperable relates to the semantic interpretability of the metadata that qualify or describe the geophysical data values themselves via the use of controlled vocabularies. Of particular importance is the application of a standard term for the observable parameter and its associated units, but also the use of standard vocabularies for metadata attributes relating to the sampling platform, sensor, and other categorical keyword descriptors. Application of standard terms is vital from a data interoperability perspective because it ensures valid interpretation of values by human users and enables correct aggregation and computation on integrated sets of data to be performed. Examples of actively maintained and widely used vocabularies include the CF standard names13 and the UDUnits library14 and the NASA Global Change Master Directory (GCMD) keywords. There are also specific science domain ontologies that are being developed by particular expert communities that once integrated provide a refined set of terms applicable to broader types of ocean science data. Vocabulary servers, such as those maintained by the British Oceanographic Data Center (BODC)15 and the Marine Metadata Interoperability project (MMI)16, aggregate and systematically organize such curated lists of standardized terms covering a broad spectrum of oceanographic disciplines that are machine query able and can help resolve metadata definitions or reconcile ambiguity in terms being applied.
In describing the key elements underlying Earth science data interoperability standards appropriate to the ocean observing domain, it is important to understand that there a couple of levels at which these are applicable and between which there are some differences: the dataset and granule levels. A dataset or collection is an aggregation comprised of data files or granules of a common type. While the definition of granule can be somewhat arbitrary, it is simply a convenient building block for the complete data set. For example, the Aquarius L3 Sea Surface Salinity V5.0 dataset17 is a collection of the entire series of data files (“granules”) over the course of the Aquarius/SAC-D satellite mission spanning 2011-Aug-25 to 2015-Jun-07. The individual granules in this dataset represent 7-day averaged snapshots covering the entire globe. Similarly for in situ data, there is an analogous collection of over 2000 profiles (granules) from an underwater glider that are aggregated together into a complete dataset that represents a glider deployment between November 11 and 15, 201518. Metadata characterizing the complete dataset is different in composition and detail to that of the constituent data files comprising the collection, although both will ideally conform to the same ISO19115 geospatial metadata standard framework that enables interoperability. The use of files as a basic element of designing an information system is based on their use in common data management systems today, and because their ubiquity makes it easier to describe some of the conceptual elements of interoperability. However, this is not meant to preclude the use of other Information Technology staples such as Relational Database Management Systems, sometimes referred to as geodatabases. Geodatabases can be equally well-suited to interoperable data systems.
Web Service Based Data Exchange: Data Access Services
Data exchange between the nodes of the distributed system shown in Figure 1 requires APIs that are designed for Web protocols. Downloading collections of files published as hyperlinks on web pages or on FTP sites cannot scale to address the types of exchanges needed for distributed global science and operational needs. Machine to machine APIs, or Web Services, enable querying, subsetting, and other advantages over bulk download of files.
Mature, well-supported software to enable web based APIs for data and metadata access exists for use by the entire GOOS Data System. Hankin et al. (2010) describe the utility of the OPeNDAP protocol for serving data. OPeNDAP19 has become ubiquitous in the earth science community and several software packages implement this protocol. For example, THREDDS20, Hyrax19, and ERDDAP21 are three data servers that ingest data files in various formats and publish data to the web using the OPeNDAP protocol.
The THREDDS Data Server, developed by Unidata, is an implementation of the OPeNDAP protocol which enables aggregations of WMO GriB files and netCDF files to be aggregated and served as a single data resource. The APIs implemented by THREDDS allow subsetting in space and time. THREDDS is particularly well-suited to gridded data collections and is currently being enhanced to serve unstructured and staggered grids common in modern ocean models (see section “Operational and Research Modeling”).
Another example is the ERDDAP data platform that was developed by NOAA’s Southwest Fisheries Environmental Research Division22. As an open source data platform, one of the functions ERDDAP performs is to serve data to users through web-based services. Similar to the THREDDS Data Server, ERDDAP supports the OPeNDAP protocol, allowing for remote accessing of data. To the data consumer, ERDDAP provides a uniform, RESTful service for accessing data that allows for machine-to-machine exchange. Many interesting tools have been built to take advantage of the services that ERDDAP provides23 and ERDDAP is a key element of the TPOS 2020 strategy (see section “TPOS 2020”).
Both THREDDS and ERDDAP are developed as open source software projects that encourage outside developers to contribute feature enhancements and bug fixes.
Web Service Based Metadata Exchange: Data Discovery Services
While the focus of this article is on interoperability, the need to support data discovery is also an important driver for developing and adhering to interoperability standards. Well-structured and well-populated granule and dataset metadata makes discovery possible. Tools exist that extract information from data files to create discovery metadata records that can be indexed into queryable metadata catalogs.
Support for common metadata standards at both file and dataset levels, allow geospatial metadata services to make data discoverable through efficiently queryable metadata repositories. Furthermore, support for common metadata standards across repositories facilitates integration and unified search horizontally, agency-wide or even across inter-agency repositories. NASA’s Common Metadata Repository (CMR)24 and NOAA’s OneStop25 system are examples of distributed metadata systems that integrate information on holdings across distributed data archives conforming to the aforementioned enterprise architecture and interoperability standards that enables unified search and access to science data enterprise-wide. Taking this approach to an even broader level are schema.org-based approaches like the new Google Dataset Search26 utility. While schema.org limits the amount of metadata to a relatively narrow set of searchable fields, that simplification makes it easier for many distributed groups to ensure their holdings are available to the big commercial search engines and other interoperable data systems. It also makes it possible for data access services that support schema.org markup, to be discoverable from Google searches.
Support for common metadata standards in turn enables integration of distinct agency metadata repository systems for discovery and access to data by users centrally with much greater efficiency across agency, national, or other jurisdictions. An example of cross-jurisdictional discovery is the Committee on Earth Observation Systems (CEOS) Common Data Assets (CDA) infrastructure, which facilitates federated search of interagency data holdings, including, NASA, NOAA, ESA, and other space agencies. This general architecture and approach, fundamentally enabled by data interoperability across systems that stems from the harmonized use of International Organization for Standardization (ISO) geospatial metadata standards, serves as a scalable implementation model for GEOSS, of which GOOS is a part. Improved integration of distributed ocean observing systems should leverage such existing development models, data system architectures and their associated data interoperability standard frameworks. Currently, these appear to implemented more at the regional and national level by responsible entities such as IOOS27 for the US, the Integrated Marine Observing System (IMOS)28 for Australia and the European Marine Observation and Data Network (EMODnet)29 for Europe, or for particular observing system elements, such as the JCOMMOPS30 asset monitoring system.
Use Cases
Long Term Preservation at US National Centers for Environmental Information
The FOO places high importance on data preservation, so those data can be reused in the future. Long term preservation is the mandate of archive centers such as the US National Centers for Environmental Information (NCEI). In this use case, NCEI is the Data Consumer, receives data from the Data Producers and assuming the responsibility for data stewardship. This transfer of stewardship responsibility places a heavy burden on the archive centers, because they must ensure future users, who will undoubtedly use different tools than today’s users, can find, access, and understand the data. The archives cannot rely on personal connections to Data Producers, who won’t be around decades into the future to explain their methods. Instead, the archives must ensure that the necessary information is captured today and encoded, preferably in machine readable data systems, so it is usable for solving tomorrow’s problems.
Long term preservation requires managing data through information technology evolutions. Over the next 50 years the storage system will evolve from spinning disks to some yet to be envisioned technology. Similarly the software libraries enabling the use of scientific data formats will evolve. For example, the netCDF libraries in use today are primarily version 3.0 and 4.0. As hardware architectures evolve it is reasonable to assume that netCDF 3.0 will no longer be supported at some point and the archive center will need to undertake a mass migration of millions of data sets encoded in netCDF 3.0 to netCDF 5, 6, or 7, or some other representation suitable for cloud-based processing, for example. The scale of a task like that underscores the need for the archive data holdings to be as interoperable as possible to allow for more automated data migration. For highly heterogeneous holdings, data migrations involving substantial individual analysis and human interventions will be prohibitively expensive.
To address these concerns, and to facilitate the broader adoption of these Earth science data interoperability standards components within the oceans community, NOAA/NCEI developed the netCDF templates31. These templates, along with documentation and examples, serve as a practical roadmap for the implementation of existing CF and ACDD standards to the range of spatial feature types characteristic of ocean and other environmental data: point, profile, trajectory, time series, and combinations of these discrete geometry types. These templates are being leveraged by other agency data centers such as NASA/PODAAC to ensure that oceanographic field campaign datasets submitted are archive quality and interoperable, such that they can be readily assimilated and disseminated via standards-aware tools/services and consumed by remote software applications. Regional data management efforts such as IOOS and IMOS have also adopted these templates as the de facto standard for data formats, supporting both current dissemination and long term preservation strategies. Global adoption of the NCEI templates would greatly enhance GOOS Data System interoperability.
TPOS 2020
The tropical Pacific Ocean has hosted some of most innovative ocean data and information management initiatives over the last 30 years (McPhaden et al., 1998). Smith and Hankin (2014) examined user requirements for the 2014 Tropical Pacific Observing System (TPOS) 2020 review and Smith (2018) undertook a similar task for the review of the Tropical Atlantic Observing System. One common finding was that neither observing system had significant gaps or issues at a technical level that were peculiar to that region; globally implemented systems such as those overseen by WMO and IOC data and information systems and including those under JCOMM oversight (Pinardi et al., 2019), were the best route for improvement, innovation and enhancements. Routine ocean and climate productions systems (data assembly, analyses, forecasts) and associated downstream users drove real-time data and information system requirements, but in both cases, research remained an important pathway for impact.
There are no systems or components that are TPOS specific. Rather TPOS information is managed and delivered by the information systems that support the platforms comprising the tropical Pacific observing system: Argo, tropical moorings, the Voluntary Observing Ship Program (VOS), the Ship-of-Opportunity Program (SOOP) (Goni et al., 2010), and surface drifters (Elipot et al., 2016). Independently, these data are collected, subjected to automated Quality Control and submitted to the Global Telecommunication System. For moorings, the infrastructure developed for TAO/TRITON (and for PIRATA in the Atlantic) continue to be supported, for delayed-mode QC and for reprocessing, among other things. Standards have been developed so that tropical observations are intercomparable across the basins, but interoperability across platforms is more problematic. Knowledge of the climate of these basins is needed for this process. The arrays are at different levels of maturity, and involve multi-national efforts, so basin-centric coordination is needed. As well, the various components that comprise the TPOS are at different levels of maturity in terms of meeting the FAIR guidelines. Data systems, such as the aforementioned Argo and tropical mooring systems, are rather mature and therefore have higher levels of conformity to those guidelines. Interoperability among the various networks, however, is an issue that needs improvement and that the JCOMM community is working to improve (Pinardi et al., 2019). The most successful data systems, such as Argo, tend to be those systems that are also widely used by the community for which they were built. The Argo community uses the Argo data system, and therefore has provided feedback on the completeness of the data and metadata, and on the utility of the data system. This feedback, and the enhancements it provides, benefits the global community of users as well, and therefore improves overall interoperability – both of the data and the data system.
Remote sensing data are generally global in coverage and are provided without any distinction between basins. For more experimental data streams, e.g., research vessel measurements of pCO2, BioGeoChemical-Argo, there is a transition from PI-based to regional and then global data systems.
Opportunities for improved efficiency, robustness and effectiveness were identified in both cases. “We want it now” was a common theme among users which impacts consideration of timeliness, efficiency, and simplicity. Systems that deliver services through multiple channels, and with different offerings in terms of integration and quality, were seen as a priority (for example, ERDDAP Pinardi et al. (2019), Tanhua et al. (2019), and Harscoat and Pouliquen for AtlantOS, personal communication). A significant finding was that complexity was a barrier to stakeholder engagement, either as a provider or as a user.
That complexity arose as a barrier to engagement should not be surprising. The tropical Pacific Ocean is home to a variety of observing elements, particularly when compared to the design of the original mooring array. As other systems, such as unmanned autonomous surface vehicles, become more common, the complexity of integrating data streams from these heterogeneous platforms will increase. Though TPOS 2020 recommends the use of CF compliant netCDF formatted data files, as previously noted, in some cases this can provide a barrier to stakeholder engagement. Typically, the barrier is in the creation of data files that conform to a standard, such as CF, by a data producer that doesn’t typically use those types of files, either in their own work or within their community. However, there is clearly a big advantage to having metadata attached to the data file, as it is in netCDF. In order to successfully engage all data providers, it is advantageous to allow providers to work in the data formats they are comfortable with, while still providing the data to the global community via data standards, conventions and web services. This is possible with brokering tools such as ERDDAP (see section “Recommendation for Addressing Some Gaps”). It is important to note, however, that such tools are less able to broker metadata between communities, such as physical oceanography and ecology communities. This is a role in which linked semantic data concepts, as discussed later in this paper, are very relevant.
National and institutional data policy also remains an issue despite successive OceanObs conferences highlighting the value of a data sharing paradigm being adopted across all systems. In the tropical Pacific it is mainly an institutional/research issue, while for developing countries in both basins it has both technical capability and historical roots. Because of this, it will be important for TPOS 2020 to embrace a distributed data landscape.
Smith (2018) noted that the FAIR Principles do provide a basis for defining a set of essential characteristics for data and information system. Such principles, and the best practice efforts, might provide a more effective pathway for improved harmonization and performance; maturity levels are useful for individual technical elements but are very uneven across the data system. Finally, both papers highlight the need for improved knowledge and use of systems architecture. Improvements over the next decade will be difficult without this.
Fisheries Data From Trawl Surveys
Surveys for fish, plankton and zooplankton have a long history, and some time series are more than 100 years old. Examples include the Norwegian beach seine survey from 1921, the Sir Alister Hardy Foundation for Ocean Science (SAHFOS) continuous plankton recorder survey from 1931, the Norwegian spring spawning herring series and the northeast arctic cod series, both from 1900. The series serve as input to fisheries stock assessments (Gulland, 1988; Beamish et al., 2009), and are important for studies addressing fish and nekton responses to climate change, ecological regime shifts etc. (Cury et al., 2008). The series are typically tracking regional populations of a species (fish stocks), and is typically regional in extent.
Several data centers are hosting the data from these surveys, including international bodies like the International Council for Exploration of the Sea and national institutions like NOAA Fisheries, CSIRO Australia, and Institute of Marine Research, Norway. The data sets typically consist both of sample data at a station or transect level, and integrated time series that tracks the abundance of a fish stock used as input to assessment or other models. Discoverability metadata is implemented to a varying degree, for example at the International Council for the Exploration of the Sea (ICES) data center.
Data Access solutions for fisheries data can vary significantly. The sample data from the joint Norwegian-Russian winter survey underpinning the advice for the North East Arctic Cod are only partially available due to a strict Russian data policy. The SAHFOS data is freely available, but only upon request. The data from most European trawl surveys are typically available through the ICES data center, and the data is accessible for automated downloads via web services. There are also standard vocabularies available, and fields like platform or vessel, species and gear types are in some cases available through web services, e.g., from the ICES data center.
Since most of these surveys are regional, the emphasis has been to ensure that data time series are consistent rather than trying to harmonize between the various interoperability standards. There is, however, a push from data managers to employ metadata standards to facilitate better discoverability and interoperability. Physical oceanography has been leading this field and advanced much further than the biological component in this respect. However, when moving forward with the biological data, employing existing standards that were developed for data from other disciplines may pose some challenges and impart additional costs. This can be in terms of costs in developing mechanisms to host the information, adding additional labor costs during data collection, or costs for make historical data compliant to the new standard. This may be less of a problem if the sensors can supply this information directly, but any manual labor is costly.
The other challenge is that it may create a false conception of interoperability in cases where a given metadata standard is used to accommodate data types that it was not designed for. An example may be trawl survey data, where the information from each trawl station is available (the primary sampling unit), but where no standard exists for the other key parameters, like survey design, survey area, stratification, data filtering parameters etc. Without this information and in the absence of suitable accompanying documentation, the data cannot be correctly used even if the metadata on the individual trawl station, from a data center point of view, conforms to the FAIR principles.
What would be the best way forward to obtain more complete interoperability for such complex datasets? Trawl surveys have one great strength: the data is tightly linked to management decision. This allows us to map out, machine to machine, if necessary, the pipeline from raw data to the data product and ensure that the information crucial to derive the desired data product, being indices of abundance for fisheries assessments or biodiversity indices for ecosystem state studies, are in place. At IMR the process of making open source software for the processing, e.g., the StoX program (Johnsen et al., 2019), relied on this approach, and it offered an approach to prioritize what was critically important for the process. It does not necessarily mean that other metadata fields are not important, but it offered a method to prioritize what was needed to obtain interoperability.
The next step would be to review the process and define a best practice guide for coding trawl survey data that is based on the actual processing pipeline. Rather than adopting a standard that was fit for another field and data type, we argue that this process would be more efficient when moving trawl surveys toward true interoperability. It may turn out that there are large overlaps with existing standards, but that should not be the prior assumption.
Cross-Disciplinary Research Cruises
The Ministry of Science, Technology and Productive Innovation (MINCyT Argentina) established a national initiative to promote improved scientific understanding of the Argentine Sea as a scientific basis for defining a national policy of biodiversity conservation. The initiative, known as Pampa Azul, was officially launched in 2014 to link interdisciplinary oceanographic cruise datasets and develop repositories capable of disseminating Marine data (SNDM32) and biodiversity data (SNDB33) from National Data Systems. These systems were created to integrate historical, current, and future information consistent with national policy and international programs. For oceanographic data, SNDM uses the Ocean Data Portal34 with BODC vocabulary servers and for biodiversity data SNDB uses the Darwin Core standard.
In this policy and technical framework of stakeholders, the principal investigators began by planning cross-disciplinary oceanographic cruises. The implementation of interoperability concepts from the beginning had different levels of maturity in each discipline. In the case of physical oceanography, the maturity is high because principal investigators are familiarized with interoperability concepts and have history of participation in global projects (e.g., SAMOC, ATLANTOS, IPCC). Chemical oceanography adopted the BODC vocabulary (I1 and I2) for laboratory analysis and the IODE developed training courses to highlight best practices, but there is no data currently being uploaded to the systems (low maturity). Fisheries biology data has some mature elements, but is not currently widely accessible and is administered by the National Fisheries Agency (acronym INIDEP). They apply acoustic methods, perform validations of abundance and biomass data, and have developed protocols defining processes from the acquisition to the analysis of importance commercial species.
In this attempt to integrate all data types into a single system, it is important that new cross disciplinary oceanographic cruises, recognize the interoperability challenge and plan in advance, where possible, to comply with FAIR principles.
The steering committee of the Pampa Azul adopted the IMBER recommendations which elaborated protocols for cross-disciplinary cruises and explained, in detail, the processes for each stage of a cruise [see IMBER Cookbook (Pollard et al., 2011)]. The IMBER recommendations were the starting point for the design of the data acquisition system which was shared between the members of the cruise. The acquisition system managed the various types of data collected: (1) continuous shipboard data; (2) observation data collected by principal investigators; (3) the analysis of samples in the laboratory; and (4) the derived products. Ideally metadata describing transect design, data collection activities, the instruments to be used and the different surveys at each station should be integrated in a single platform. However, this can be a challenge because often the surveys are conducted from different vessels and equipment across vessels can vary. Therefore, the presence of the data manager, starting in the early stages of planning project, is helpful to coordinate and simplify the collection of data and metadata.
One of the first post-cruise responsibilities is to submit the report to the co-participants. It is at this stage where a non-integrated system reveals its limitations. Due the heterogeneity of the observational data, as well as the laboratory analyzed data, unless the metadata has clearly documented the processes, modeling groups that use the data may struggle in understanding it. Often, this disruption of the data life cycle can be traced back to the origin of the data. If, at that time, the data was not documented properly using standards, there is little hope for improvement as the data moves toward access and archival.
At the system level, the SNDM and SNDB work as two separate worlds and due to these drawbacks we propose improving the interoperability with the use of Linked Data (LD) (Janowicz et al., 2014).
Linked Data as a paradigm describes how to break up data silos35 and support the publication, retrieval, reuse, and interlinkage of data on the Web. Together with other Semantic Web (SW) technologies, Linked Data shows promise to address many challenges that have affected semantic interoperability between repositories and services within and across domains that are highly heterogeneous in nature (Berners-Lee et al., 2001).
We develop an oceanographic linked dataset following the life cycle proposed by Villazón-Terrazas et al. (2011) with information provided by national cruises. For this we use the controlled vocabularies NERC36, ISO19115 standard to represent metadata records in conjunction with the geospatial standard for the SW GeoSPARQL37 and the reuse of the ontology design pattern (ODP) for oceanographic cruises (Krisnadhi et al., 2014). Publishing the vocabularies and metadata in standard RDF XML and exposing SPARQL endpoints renders them five-star Linked Data repositories.
In addition to enabling FAIR principles for these datasets, the benefits of this approach include: greater interoperability between the metadata created by cross-disciplinary projects; improved data discovery and newly developed tools can be used to explore the data. Here we have shown that the linked data provide a framework for better discovery and access to data, and it is possible to provide the highest standard of linked oceanographic data, and some of the benefits of the approach. Particularly when the results of the research have multiple stakeholders or are used by non-experts for manage and conservation purposes.
The interaction of biological, physical, geological, and chemical data in a single platform leads to the loss of information in the generalization of some parameters. From the technical point of view, each discipline has international formats and, as in the case of biological ones, they have extensions that avoid the loss of complementary information. With the use of linked data it is possible to keep the distributed system and solve the problem of the combination of different disciplines and sources of information.
There is no doubt, from a technical perspective, that solutions exist. However, often the problem lies in the fact that policies are required for actual implementation of those solutions. For example the permanent position of data manager in government agencies facilitate curation of data. For collecting new data, it is necessary to promote traceability throughout the whole data life cycle and ensure the availability of essential and observational metadata collected during cruise surveys.
As a final recommendation, international coordination entities should encourage national agencies to implement FAIR and ISO standards in their data systems as a requirement for project funding.
Operational and Research Modeling
Operational and research numerical modeling efforts form a critical source of information to complement ocean observations. Numerical models are consumers of observational data and they are providers of data sets generated through simulation. They require observational data for model validation and verification and they increasingly ingest observational through data assimilation methods during the model integration. As such, they provide a use case for testing the efficacy of data interoperability standards for both ingesting observations and publishing model results.
Operational modeling centers typically access observational data through private networks governed by the World Meteorological Organization (WMO) standards, like the Global Telecommunications System (GTS) (Blanc et al., 2010; Hankin et al., 2010). While these networks are built upon different standards than are described here they serve their intended purpose for a very specific, and important, group of stakeholders. The broader research community does not have easy access to the GTS and therefore requires an alternative access pathway. Wilkin et al. (2017) describe a framework that would advance coastal modeling in the US and advocate for an open access portal that serves quality controlled near real time observations for ingestion into ocean models. Ideally these portals also include deep archives of long time series observations in addition to near real time data. These comprehensive access portals are a challenge to design because they involve integrating data from many individual data sources with their own data stewardship lifecycles. Nevertheless, providing a reliable source of quality observations to both operational and research modelers will accelerate the transition of modeling advances from the research community to the operational community. Ensuring both communities are served should be a requirement for the GOOS Data System. The JCOMM Open Access to GTS project is taking steps to address this inefficiency by simplifying the process of getting data onto and off of the GTS for the research community (Pinardi et al., 2019). NOAA’s Observing System Monitoring Center (OSMC)38 provides access to near real-time ocean observations through the ERDDAP data platform. The interoperable web services that ERDDAP provides allows consumers of varied technical levels to access and use the real time data stream from the GTS through the software clients with which they are most familiar. Capabilities like these become ever important as the WMO community continues to require complicated, binary, table-based data formats for distribution on the GTS.
Signell and Snowden (2014), describe a framework for model data dissemination built on CF/netCDF/THREDDS that provides standards compliant data through a THREDDS Data Server. The THREDDS data service provides access to data through, among others, the OPeNDAP protocol which has been a popular tool for providing access to model data on a regular horizontal grid (Hankin et al., 2010). Recent developments in standardizing the encoding of unstructured grids (UGRID)39 and staggered grids (SGRID)40 have led to proposed improvements in the CF conventions. These improvements are at the Concept phase of maturity but are prompting debate within the standards governance community and development of software libraries to further test the concepts. Further development of software tools, along with adoption of these standards by the community modeling developers, is necessary to advance UGRID and SGRID to the Mature phase.
Standards for model data, and their inclusion in web service tools is critical due to the high volumes of data models provide. Increasingly it is infeasible to download and entire simulation for scientific or operational application. Therefore it is essential to support development of robust flexible server side subsetting tools if data consumers are to fully exploit the high volumes of information created by the modeling community. A promising area of development is in server side processing, especially when combined with cloud computing architectures (Vance et al., 2019).
Limitations of Current Systems
Challenges to Data Interoperability Adoption
There are several constraints, conceptual and practical, to the broader adoption of the kinds of established data interoperability standards described to projects involved in ocean observation, which in turn impacts the usability and accessibility of the data, and the advancement of GOOS more generally.
Importance of Standards Not Understood
First, the importance of data standards often are not fully understood, and the broader value of publicly sponsored data collection efforts beyond the specific science purpose for which they may have been collected and as part of an important data commons may not be adequately appreciated. This plus the implementation of data management practices that ensure the preservation and usability of data assets long-term must motivate and be promoted amongst stakeholders at all levels and further reinforced both by engineering requirements and governing program policies.
Data Standards Are Hard to Understand and Use
A second significant constraint is the lack of understanding of the applicable technical data interoperability standards that we have previously described (e.g., TPOS 2020) and which are integral to ocean observing data management best practices. For non-experts, just understanding Earth science/geospatial data standards such as CF, ACDD, Darwin Core, ISO19115, and then how to practically apply them in the context of one’s own particular datasets is a significant effort. Therefore, implementation of these standards is non-trivial. Our experience in working with oceanographic field campaigns and other in situ data producers is that even with resources such as the NCEI netCDF templates, implementing the necessary custom software routines to correctly undertake the necessary conversions and validate outputs for individual datasets is often involved and an iterative process. Even with the availability of compliance checker utilities online41, the process often requires multiple consultations with experts at data archives to resolve issues. The effort and resources necessary to do this is typically underestimated, and often included in project data management plans as an afterthought. Increasing the focus on data management and stewardship to ensure adequate technical skills and funding, at the proposal stage is a strong recommendation of this article.
Recommendation for Addressing Some Gaps
Addressing these issues will require a multifaceted approach, including further outreach, education, resources and practical tools, promoting improved data interoperability best practices. As has been emphasized, tackling interoperability issues as early on in the data lifecycle and as close as possible to the time of production is important. An area that can be improved upon and can have significant impact is greater engagement and partnership with in situ instrument and platform manufacturers to facilitate production of standards compliant data file outputs natively at source as an option in their processing software. Manufacturers are responsive to market demands, and so should be receptive to user, project and program sponsor requests in this space given the necessary awareness. There are examples where this has been achieved and produced the desired outcomes. Invariably, however, it is a process to secure the necessary buy-in to affect change if the business case and incentive for doing so is unclear.
Tools facilitate file creation and translation
While well-structured, complete, self-describing data files that comply with accepted metadata content models are a building block of a more interoperable data system, the complexity of these files is a barrier to adoption for many data providers. Tools are needed to enable conversion from commonly used formats like Comma Separated Value into netCDF and to augment the converted files with rich metadata complying with modern data content standards. ROSETTA42 and ERDDAP are two examples of software that facilitate file creation.
ROSETTA is a Web-based data format transformation service developed by Unidata and available Open Source43. It provides an easy, wizard-based interface44 and service for data providers to interactively transform their ASCII output instrument data into Climate and Forecast (CF) compliant netCDF files. ROSETTA also provides a RESTful web service interface (API) for bulk data conversion45. In addition to CF, ROSETTA supports also ACDD, the NCEI templates, a metadata standard profile developed for the biologging community, and is readily extensible to support metadata profiles for other science domains. ROSETTA provides full support for all spatial feature types associated with the range of discrete sampling geometries characteristic of in situ data consistent with the CF standard and NCEI templates. ROSETTA is built upon the netCDF-Java library, which is an implementation of the CDM, and which underlies widely used data access technologies such as THREDDS. It also employs commonly used web-based technologies such as Spring and JavaScript for the Web-front end.
ERDDAP takes a slightly different approach to creating standards compliant netCDF files. In addition to functioning as a data access service to publish data on the web, ERDDAP acts as a data broker that can convert between dozens of scientific file formats, including CF compliant netCDF files. For example, ERDDAP can ingest a collection of CSV files and serve the same information to users as a CF/netCDF file. The dataset can be augmented with additional metadata through a markup language that is part of the server configuration file. ERDDAP also provides automatic generation of ISO 19115 metadata, and can create BagIt46 archive packages which can automate submission to national archive data centers.
Limitations of Existing Technical Standards
Earth science data interoperability standards that govern the production of archive-quality data files support a broad range of oceanographic data types and are integral to ocean observing system data management infrastructures. However, as has been illustrated in some of the use cases above, there are some limitations to current standards such as CF/ACDD and the associated oceanographic NCEI netCDF template implementations that constrain their more universal application.
One issue is that these standards focus primarily on geospatial characteristics of data and their metadata representation. There is a need to extend standards specifications so as to additionally support richer sets of metadata that may be specific to certain science domains and to package such augmented metadata in a non-ad hoc, machine-readable manner within self-describing science data files (nc, HDF). Such metadata attributes would document more fully for example critical information on aspects of instrument deployment, sampling and other protocols necessary to properly and reproducibly interpret the associated file data. This is important because dataset level metadata used to catalog collections of data files invariably do not capture this necessary information with sufficient granularity for in situ datasets that will differ in descriptive content between files. This is also likely to be particularly important for certain classes of data, such as biological datasets. The ability to package richer metadata in a machine-readable manner will inevitably have broader applicability and facilitate improved, more granular data search. It can also enable the integration of the existing SensorML metadata framework to better describe instrument characteristics with associated data and then potentially expose that information via Open Geospatial Consortium (OGC) Sensor Web Enablement47 (SWE) standards. There are already examples of satellite missions such as SMAP utilizing Group structures in HDF5 data files to organize hierarchically related sets of metadata attributes and encode these consistent with ISO standards. An analogous approach has also been implemented to support community metadata specifications for animal telemetry data involving 130 attributes organized thematically within 10 categorical Groups and dispositioned as required/recommended/optional. The biodiversity community uses the Darwin Core metadata standard to encode several categories of attributes related to the discrete sampling events, their geospatial characteristics, taxonomic composition and associated quantitative metrics for which the aforementioned framework will also be applicable for. A further example comes from the marine bio-acoustics community in which the international MESOPP48 (Mesopelagic Southern Ocean Prey and Predators) project has delivered acoustic backscatter and related modeled data products as netCDF files with CF metadata supplemented with ICES metadata conventions for active acoustic systems49.
A further interoperability challenge relates to frequently observed partial overlap of certain categories of attributes used by different science domain metadata frameworks. Several schemas share certain categories of attributes (e.g., Geospatial) that may conflict. There are also examples where even closely related communities (Biodiversity and Ecological sciences) may be using different metadata models (Darwin Core and EML) to represent even the exact same types of data. There is also considerable overlap in geospatial attributes across domain metadata conventions and Earth science such as CF/ACDD. This highlights the importance of establishing mappings across these schemas to facilitate semantic reconciliation of attributes between datasets produced by different communities, and to be able to do so in an automated service-based manner so as to enable also improved granule level search.
Improved support for provenance information is another important area for extensions to existing Earth Science data standards. This is becoming increasingly important as greater integration of data occurs and there is a proliferation of derived products. Currently CF foresees only a single History attribute to capture provenance and processing information, invariably implemented in ad hoc ways that are generally also undocumented. PROV50 is a W3C standard for the representation of provenance information that could be leveraged here, and implemented using groups in a manner consistent with the aforementioned suggested approach to support richer metadata more generally. Tracking of provenance in the evolution of metadata standards themselves and their inter-relationships is another area that can be improved upon to facilitate improved granule level search across data series where a blend of metadata profiles and versions may have been employed over time.
There is considerable interest in the inclusion of measurement uncertainty and data quality information in data files. CF standards provide a framework for quality flagging of observational data at a very granular level within variables of self-describing data files. Furthermore, certain communities (e.g., GHRSST) have worked to develop a standardized approach to the representation of uncertainty in geophysical data. However, there is a need to extend these approaches to also represent error in geolocation variables given that positional determination (and in some cases estimation) may be a particular issue for in situ datasets and may take the form of a qualitative category code or quantitative error estimate. The Argo profiling float data format standard makes extensive use of category codes, including beyond geolocation variables.
The trawl survey use case discussed in the previous section highlights the broader issue of how to better support complex datasets in a manner that better captures inter-relationships between recorded data elements when Earth Science data interoperability standards and typical system architectures focus on simple collections of discrete data files. Even with support for hierarchical structured data in the nc4/HDF5 data models, the ability to comprehensively represent complex event-based datasets such as cruise data in a single data file is an unrealistic expectation. Instead there may be more promise in developing a framework in which time course and functional relationships between data files are represented in a standard manner so as to better define a given dataset or file collection.
Summary and Recommendations
The use cases in the previous section lead to several key considerations for charting the course ahead for the next 10 years of ocean data management, specifically in enhancing interoperability across components of the GOOS Data System. These issues impact stewardship, discoverability and access to ocean data. In general, we recommend adherence to the FAIR data principles, though the details of what precisely that entails may differ between communities, and specific definitions of what FAIR compliance means within a community are still under discussion (Tanhua et al., 2019). The coming decade should do much to provide more specific implementation details for FAIR compliance.
Hankin et al. (2010) asserted that the combination of the netCDF file format, the Climate and Forecast Conventions, and the OPeNDAP network protocol formed the basis of a data management strategy for GOOS. Although at that time, the strength of this combination was gridded data. In the last 10 years the importance of netCDF/CF/OPeNDAP has grown and the capabilities now extend to in situ data types (points, profiles, time series etc.). The standards and the tools have evolved methodically and deliberately to address important data management problems. We conclude that a netCDF/CF/OPeNDAP remain the building blocks for ocean data management and that by focusing on incremental enhancements to mature technologies will be a sustainable path for the global community. The NCEI templates are the gold standard examples for many of the common data types encountered in GOOS and should be the starting point for any data management effort.
This collection of standards is complex and incomplete. The complexity requires strategies to simplify adoption through education and tool development. Communities of practice help spread knowledge and focus development efforts on tools of general utility, especially when combined with Open Source development practices. The incompleteness requires evolving the family of standards and finding ways to cross-reference and link to data that is managed according to other discipline specific but mature technologies. The issues surrounding multidisciplinary observations in general (see section “Cross-disciplinary Research Cruises”) and trawl surveys in particular (see section “Fisheries Data from Trawl Surveys”), shed light on the differences between community data standards across science disciplines and suggest that pragmatic ways to bridge those standards is more likely to garner adoption than attempting to develop one standard for all data types. Further, the balance between global interoperability and local project priorities is a factor that should be considered in the funding and policy framework nationally and internationally. Linked Open Data strategies show promise in bridging discipline specific communities but those capabilities are nascent and at a relatively low maturity level.
Standards provide a framework to record and share essential metadata, but without concerted effort to populate the files with metadata, interoperability is still lacking. Strategies to incorporate metadata into the data stream automatically and without human intervention will be critical to automating work flows and dealing with growing volumes of data. Engaging the sensor and platform manufacturers and applying market pressure is an essential step.
Finally, the information management and data science skills needed to develop a truly global and interoperable system require an influx of talent from outside traditional marine disciplines. Incorporating data science and system engineering perspectives into the global policy framework can help identify areas for collaboration in the future. Balancing an operational perspective based on mature technologies (e.g., CF/netCDF/OPeNDAP) against the need for research into new technologies (e.g., the semantic web and Linked Open Data) to bridge communities will be essential.
Author Contributions
DS, VT, NH, MZ, KO’B, and KC helped to conceive the manuscript, coordinate author contributions, wrote text, and edited and contributed figures. NS, HS, KB, ML, and SA contributed manuscript ideas and text.
Funding
This work was partially funded by the Joint Institute for the Study of the Atmosphere and Ocean (JISAO) under the NOAA Cooperative Agreement NA15OAR4320063, Contribution No. 2018-0178.
Conflict of Interest Statement
The authors declare that the research was conducted in the absence of any commercial or financial relationships that could be construed as a potential conflict of interest.
Acknowledgments
We acknowledge the contributions of specific colleagues, institutions, or agencies who aided the efforts of the authors.
Footnotes
- ^ http://www.goosocean.org/
- ^ https://www.earthobservations.org/geoss.php
- ^ https://www.data.gov/developers/open-data-executive-order/
- ^ http://www.argodatamgt.org/
- ^ https://podaac.jpl.nasa.gov/datasetlist?ids=&values=&search=Pathfinder
- ^ https://www.nodc.noaa.gov/OC5/WOD/pr_wod.html
- ^ https://www.ghrsst.org/
- ^ https://www.hdfgroup.org/solutions/hdf5/
- ^ https://doi.org/10.5065/D6H70CW6
- ^ The CF Conventions are a set of guidelines for creating netCDF files with good interoperability characteristics ( http://cfconventions.org/).
- ^ http://wiki.esipfed.org/index.php/Attribute_Convention_for_Data_Discovery
- ^ https://www.iso.org/standard/53798.html
- ^ http://cfconventions.org/Data/cf-standard-names/60/build/cf-standard-name-table.html
- ^ https://doi.org/10.5065/D6KD1WN0
- ^ https://www.bodc.ac.uk/resources/products/web_services/vocab/
- ^ https://mmisw.org/ont/#/
- ^ http://dx.doi.org/10.5067/AQR50-3S7CS
- ^ https://data.ioos.us/dataset/cp_387-20151014t011988915
- ^ https://www.opendap.org/
- ^ https://doi.org/10.5065/D6N014KG
- ^ https://upwell.pfeg.noaa.gov/erddap/information.html
- ^ https://upwell.pfeg.noaa.gov/erddap/information.html
- ^ https://github.com/IrishMarineInstitute/awesome-erddap
- ^ https://earthdata.nasa.gov/cmr
- ^ https://data.noaa.gov/onestop
- ^ https://toolbox.google.com/datasetsearch
- ^ https://ioos.noaa.gov/
- ^ http://imos.org.au/
- ^ http://www.emodnet.eu/
- ^ https://www.jcommops.org/
- ^ https://www.nodc.noaa.gov/data/formats/netcdf/v2.0/
- ^ http://www.datosdelmar.mincyt.gob.ar/
- ^ https://datos.sndb.mincyt.gob.ar/ala-hub/search
- ^ http://www.oceandataportal.org/
- ^ An information silo, or a group of such silos, is an insular management system in which one information system or subsystem is incapable of reciprocal operation with others that are, or should be, related.
- ^ https://www.bodc.ac.uk/resources/vocabularies/vocabulary_search/P01/
- ^ http://www.opengeospatial.org/standards/geosparql
- ^ http://www.osmc.noaa.gov
- ^ http://ugrid-conventions.github.io/ugrid-conventions/
- ^ http://sgrid.github.io/sgrid/
- ^ https://compliance.ioos.us/
- ^ https://doi.org/10.5065/D6N878N2
- ^ https://github.com/Unidata/rosetta
- ^ https://youtu.be/2IrSDTUfeNU
- ^ https://youtu.be/_4jIDvrqiZo
- ^ https://tools.ietf.org/html/draft-kunze-bagit-17
- ^ http://www.opengeospatial.org/ogc/markets-technologies/swe
- ^ http://www.mesopp.eu/
- ^ http://www.ices.dk/sites/pub/Publication%20Reports/ICES%20Survey%20Protocols%20(SISP)/SISP-4%20A%20metadata%20convention%20for%20processed%20acoustic%20data%20from%20active%20acoustic%20systems.pdf
- ^ https://www.w3.org/TR/prov-overview/
References
Beamish, R. J., and Rothschild, B. J. American Institute of Fishery Research, Biologists. (2009). The Future of Fisheries Science in North America. Dordrecht: Springer.
Berners-Lee, T. I. M., Hendler, J., and Lassila, O. R. A. (2001). The semantic web. Sci. Am. 284, 34–43.
Blanc, F., Baralle, V., Blower, J. D., Bronner, E., Cornillon, P., deLaBeaujardiere, J., et al. (2010). “Evolution in Data and Product Management for Serving Operational Oceanography, a GODAE Feedback,” in Proceedings of OceanObs’09: Sustained Ocean Observations and Information for Society, (Paris: ESA), 40–46.
Cury, P. M., Shin, Y.-J., Planque, B., Durant, J. M., Fromentin, J.-M., Kramer-Schadt, S., et al. (2008). Ecosystem oceanography for global change in fisheries. Trends Ecol. Evol. 23, 338–346. doi: 10.1016/j.tree.2008.02.005
Elipot, S., Lumpkin, R., Perez, R. C., Lilly, J. M., Early, J. J., and Sykulski, A. M. (2016). A global surface drifter data set at hourly resolution. J. Geophys. Res. Oceans 121, 2937–2966. doi: 10.1002/2016JC011716
Goni, G., Roemmich, D., Molinari, R., Meyers, G., Sun, C., Boyer, T., et al. (2010). “The ship of opportunity program,” in Proceedings of OceanObs’09: Sustained Ocean Observations and Information for Society, Vol. 2, eds J. Hall, D. E. Harrison, and D. Stammer (Venice: ESA Publication WPP-306). doi: 10.5270/OceanObs09.cwp.35
J. A. Gulland (ed.) (1988). Fish Population Dynamics: Implications for Management. New York, NY: John Wiley and Sons.
Hankin, S. C., Blower, J. D., Carval, T., Casey, K. S., Donlon, C., Lauret, O., et al. (2010). “NetCDF-CF-OPeNDAP: Standards for Ocean Data Interoperability and Object Lessons for Community Data Standards Processes,” in Proceedings of OceanObs’09, (Paris: European Space Agency), 450–458.
Janowicz, K., Hitzler, P., Adams, B., Kolas, D., and Charles Vardeman, I. (2014). Five stars of Linked Data vocabulary use. Semant. Web 5, 173–176.
Johnsen, E., Totland, A., Skålevik, Å., Holmin, A. J., Dingsør, G. E., Fuglebakk, E., et al. (2019). StoX – an open source software for marine survey analyses. Methods Ecol. Evol. (in press). doi: 10.1111/2041-210x.13250
Krisnadhi, A., Arko, R., Carbotte, S., Chandler, C., Cheatham, M., Finin, T., et al. (2014). An Ontology Pattern for Oceanographic Cruises: Towards an Oceanographer’s Dream of Integrated Knowledge Discovery. Available at: https://corescholar.libraries.wright.edu/cse/167/
Lindstrom, E., Gunn, J., Fischer, A., McCurdy, A., and Glover, L. K. (2012). A Framework for Ocean Observing. Available at: http://www.oceanobs09.net/foo/FOO_Report.pdf
McPhaden, M. J., Busalacchi, A. J., Cheney, R., Donguy, J.-R., Gage, K. S., Halpern, D., et al. (1998). The tropical ocean-global atmosphere observing system: a decade of progress. J. Geophys. Res. Oceans 103, 14169–14240. doi: 10.1029/97JC02906
Pinardi, N., Stander, J., Legler, D., O’Brien, K., Boyer, T., Cuff, T., et al. (2019). The joint IOC (of UNESCO) and WMO collaborative effort for met-ocean services. Front. Mar. Sci. 6:410. doi: 10.3389/fmars.2019.00410
Pollard, R., Moncoiffé, G., and O’Brien, T. D. (2011). The IMBER Data Management Cookbook - A Project Guide to good Data practices. Plouzane: Institut Universitaire European de la Mer (IUEM).
Riser, S. C., Freeland, H. J., Roemmich, D., Wijffels, S., Troisi, A., Belbéoch, M., et al. (2016). Fifteen years of ocean observations with the global Argo array. Nat. Clim. Chang. 6, 145–153.
Send, U., Weller, R. A., Wallace, D., Chavez, F., Lampitt, R. L., Dickey, T., et al. (2010). “OceanSITES,” in Proceedings of OceanObs’09: Sustained Ocean Observations and Information for Society, Vol. 2, eds J. Hall, D. E. Harrison, and D. Stammer (Venice: ESA Publication WPP-306). doi: 10.5270/OceanObs09.cwp.79
Signell, R., and Snowden, D. (2014). Advances in a Distributed Approach for Ocean Model Data Interoperability. J. Mar. Sci. Eng. 2, 194–208. doi: 10.3390/jmse2010194
Smith, N. (2018). Data Flow and Information Products. Available at: http://www.clivar.org/sites/default/files/3.1_Data%20Flow%20and%20Information%20Products_Neville%20Smith_0.pdf
Smith, N., and Hankin, S. (2014). “White Paper #13 – Data and information delivery: communication, assembly and uptake,” in Proceedings of the Tropical Pacific Observing System 2020 Workshop, (La Jolla CA: Ocean Observations Panel for Climate).
Tanhua, T., Pouliquen, S., Hausman, J., O’Brien, K., Bricher, P., Bruin, T. D., et al. (2019). Ocean FAIR data services. Front. Mar. Sci. (in press). doi: 10.3389/fmars.2019.00440
Vance, T. C., Wengren, M., Burger, E., Hernandez, D., Kearns, T., Medina-Lopez, E., et al. (2019). From the oceans to the cloud: opportunities and challenges for data, models, computation and workflows. Front. Mar. Sci. 6:211. doi: 10.3389/fmars.2019.00211
Villazón-Terrazas, B., Vilches-Blázquez, L. M., Corcho, O., and Gómez-Pérez, A. (2011). “Methodological Guidelines for Publishing Government Linked Data,” in Linking Government Data, ed. D. Wood (New York, NY: Springer), 27–49. doi: 10.1007/978-1-4614-1767-5_2
Wilkin, J., Rosenfeld, L., Allen, A., Baltes, R., Baptista, A., He, R., et al. (2017). Advancing coastal ocean modelling, analysis, and prediction for the US integrated ocean observing system. J. Operat. Oceanogr. 10, 1–12. doi: 10.1080/1755876X.2017.1322026
Keywords: interoperability, data management, data lifecycle, data preservation, standards, metadata
Citation: Snowden D, Tsontos VM, Handegard NO, Zarate M, O’ Brien K, Casey KS, Smith N, Sagen H, Bailey K, Lewis MN and Arms SC (2019) Data Interoperability Between Elements of the Global Ocean Observing System. Front. Mar. Sci. 6:442. doi: 10.3389/fmars.2019.00442
Received: 15 November 2018; Accepted: 05 July 2019;
Published: 26 July 2019.
Edited by:
Justin Manley, Just Innovation, Inc., United StatesReviewed by:
Jan Robert Van Smirren, Ocean Sierra LLC, United StatesJay S. Pearlman, Institute of Electrical and Electronics Engineers (France), France
Copyright © 2019 Snowden, Tsontos, Handegard, Zarate, O’ Brien, Casey, Smith, Sagen, Bailey, Lewis and Arms. This is an open-access article distributed under the terms of the Creative Commons Attribution License (CC BY). The use, distribution or reproduction in other forums is permitted, provided the original author(s) and the copyright owner(s) are credited and that the original publication in this journal is cited, in accordance with accepted academic practice. No use, distribution or reproduction is permitted which does not comply with these terms.
*Correspondence: Derrick Snowden, ZGVycmljay5zbm93ZGVuQG5vYWEuZ292