- 1Scripps Institution of Oceanography, University of California, San Diego, La Jolla, CA, United States
- 2Laboratoire d'Océanographie Physique et Spatiale, Univ. Brest, CNRS, IRD, Ifremer, IUEM, Brest, France
- 3LMD/IPSL, École Normale Supérieure, PSL Research University, Paris, France
- 4COAPS, Florida State University, Tallahassee, FL, United States
- 5GEOMAR Helmholtz Centre for Ocean Research Kiel, Kiel, Germany
- 6Christian-Albrechts-Universität zu Kiel, Faculty of Mathematics and Natural Sciences, Kiel, Germany
- 7Woods Hole Oceanographic Institution, Woods Hole, MA, United States
- 8College of Earth, Ocean, and Atmospheric Sciences, Oregon State University, Corvallis, OR, United States
- 9Los Alamos National Laboratory (DOE), Department of Earth, Environmental and Planetary Sciences, Brown University, Providence, RI, United States
- 10National Oceanography Centre, Southampton, United Kingdom
- 11Department of Geological Sciences, Jackson School of Geosciences, The University of Texas, Austin, TX, United States
- 12Department of Earth Observation Projects, European Space Agency, Paris, France
- 13Jet Propulsion Laboratory, California Institute of Technology, Pasadena, CA, United States
- 14Centre for Geography and Environmental Science, College of Life and Environmental Sciences, University of Exeter, Exeter, United Kingdom
- 15Centre for Polar Observation and Modelling, Earth Sciences, University College London, London, United Kingdom
- 16CLS, Toulouse, France
- 17Institute for Marine and Atmospheric Research, Utrecht University, Utrecht, Netherlands
Ocean surface winds, currents, and waves play a crucial role in exchanges of momentum, energy, heat, freshwater, gases, and other tracers between the ocean, atmosphere, and ice. Despite surface waves being strongly coupled to the upper ocean circulation and the overlying atmosphere, efforts to improve ocean, atmospheric, and wave observations and models have evolved somewhat independently. From an observational point of view, community efforts to bridge this gap have led to proposals for satellite Doppler oceanography mission concepts, which could provide unprecedented measurements of absolute surface velocity and directional wave spectrum at global scales. This paper reviews the present state of observations of surface winds, currents, and waves, and it outlines observational gaps that limit our current understanding of coupled processes that happen at the air-sea-ice interface. A significant challenge for the coming decade of wind, current, and wave observations will come in combining and interpreting measurements from (a) wave-buoys and high-frequency radars in coastal regions, (b) surface drifters and wave-enabled drifters in the open-ocean, marginal ice zones, and wave-current interaction “hot-spots,” and (c) simultaneous measurements of absolute surface currents, ocean surface wind vector, and directional wave spectrum from Doppler satellite sensors.
1. Introduction
The Earth's climate is regulated by the energetic balance between ocean, atmosphere, ice, and land. This balance is driven by processes that couple the component systems in a multitude of complex interactions that happen at the boundaries. In particular, the marine atmospheric boundary layer provides a conduit for the ocean and the atmosphere to constantly exchange information in the form of fluxes of energy, momentum, heat, freshwater, gases, and other tracers (Figure 1). These fluxes are strongly modulated by interactions between surface winds, currents, and waves; thus, improved understanding and representation of air-sea interactions demand a combined cross-boundary approach that can only be achieved through integrated observations and modeling of ocean winds, surface currents, and ocean surface waves.
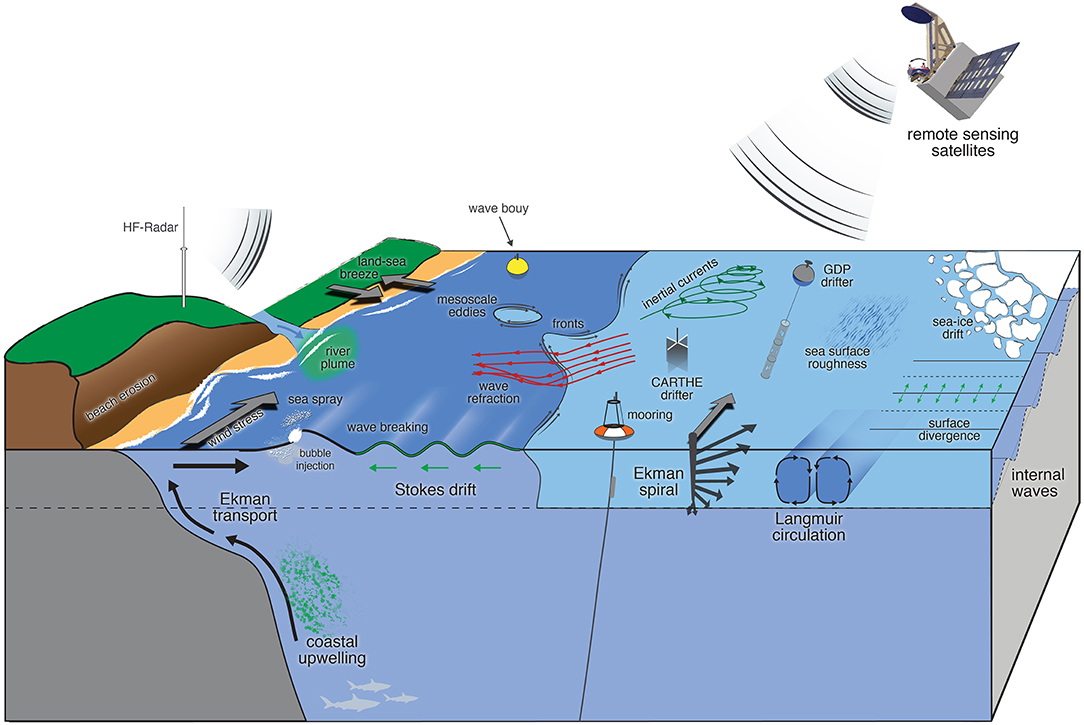
Figure 1. Schematic representation of upper-ocean processes that are coupled through the interaction between surface winds, currents, and waves. Processes that are driven by these interactions range from regional to global scales and happen in coastal areas (e.g., coastal upwelling and land-sea breeze), open ocean (e.g., inertial currents and mesoscale eddies), and marginal ice-zones (e.g., sea ice drift). Multiple components of the observing system including in situ (e.g., surface drifters, wave buoys, and moorings) and remote sensing (e.g., HF-radar and satellites) platforms are also illustrated.
Surface winds, currents, and waves interact over a broad range of spatial and temporal scales, ranging from centimeters to global scales and from seconds to decades (Figure 2). At present, there are fundamental gaps in the observations of these variables. For example, high-resolution satellite observations of ocean color and sea surface temperature reveal an abundance of ocean fronts, vortices, and filaments at scales below 10 km, but measurements of ocean surface dynamics at these scales are rare (McWilliams, 2016). Recent findings based on airborne measurements (Romero et al., 2017), numerical models (Ardhuin et al., 2017a), and satellite altimeter data (Quilfen et al., 2018) have shown that the variability of significant wave height at scales shorter than 100 km is dominated by wave-current interactions. Yet, the observational evidence from altimetry that supports that idea is limited to wavelengths longer than 50 km, due to signal-to-noise limitations of present satellite altimeters and tracking techniques that are not specifically optimized to estimate significant wave heights. Another notable observational gap lies in coastal, shelf, and marginal ice zones (MIZs), regions that control important exchanges between land, ocean, atmosphere, and cryosphere and are particularly relevant for society. Over one-fourth of the world's population lives in coastal areas (Nicholls and Cazenave, 2010; Wong et al., 2014) and could be impacted by processes resulting from wind-current-wave interactions, such as beach erosion, extreme sea level events, and dispersion of pollutants or pathogens. Unraveling these interactions to guide adaptation and mitigation strategies and increase resilience to natural hazards and environmental change calls for high spatial resolution and synoptic observations of total ocean surface current vectors, winds, and waves that will enable the development of improved model parameterizations, improved model representations of air-sea interactions, and improved forecasts and predictions.
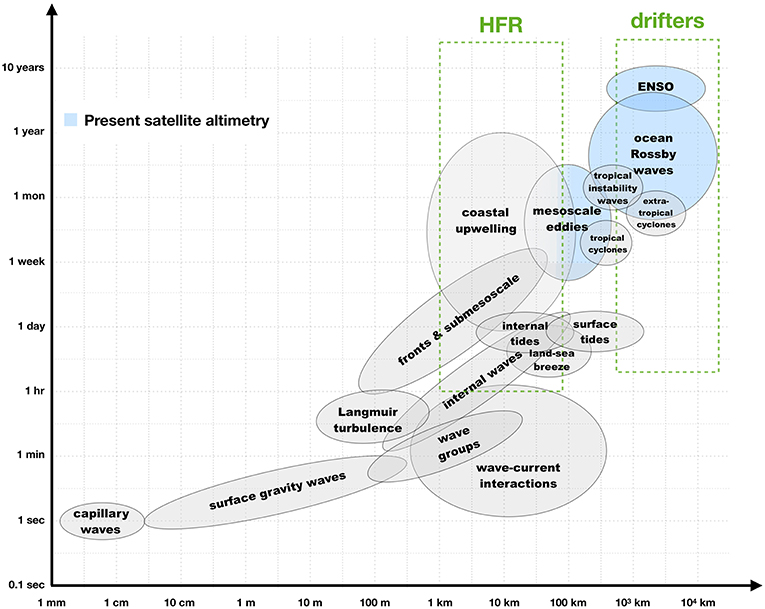
Figure 2. Spatial and temporal scales of multiple ocean and atmosphere process [courtesy of Dudley Chelton, adapted from Chelton (2001)]. Processes that can be observed by the present constellation of altimeters are shaded in blue. The square green boxes delimit the approximate range of scales that can be captured by high-frequency radars (HFR) and drifters from the Global Drifter Program.
Community efforts to fill the observational gaps for combined wind, current, and wave measurements have led to several recent proposals for new Doppler oceanography satellite concepts, such as the Sea surface KInematics Multiscale monitoring satellite mission, SKIM the Winds and Currents Mission, WaCM; and the SEASTAR mission. These missions propose to deliver a variety of simultaneous measurements of absolute surface velocity vector, Stokes drift, directional wave spectrum, and ocean surface wind vector. But although SKIM, WaCM, and SEASTAR share the common goal of measuring coupled air-sea variables simultaneously, each mission is intrinsically different, driven by different objectives, and targeting specific processes at different scales as enabled by the capabilities of their different technological solutions. Thus, the focus for WaCM lies in global monitoring of surface currents at scales comparable to scatterometer winds (~30 km) and temporal scales of one to several days, seeking to better observe wind-current interactions and their impact on global surface fluxes. In turn, SKIM's objectives include the exploration of global mesoscale surface currents and their impact on heat, carbon and freshwater budgets from the equator (where they are not observed today), to high latitudes including the emerging Arctic (which is poorly sampled by altimeters). SKIM also promises to explore intense currents and associated extreme waves by measuring the total current vector together with the directional spectrum of the wave field, at medium-resolution and covering 99% of the world ocean, on average once every 4 days. Finally, at the high spatial resolution end of the spectrum, SEASTAR focuses on ocean submesoscale dynamics and complex processes in coastal, shelf and polar seas. SEASTAR would provide a two-dimensional synoptic imaging capability for total surface current vectors and wind vectors at ~1 km resolution supported by coincident directional wave spectra. The key scientific drivers for SEASTAR are to deliver high-accuracy observations of the two-dimensional surface flow field and atmospheric forcing to understand processes linked to frontogenesis and upper ocean mixing that determine the vertical structure of the upper ocean. This includes observing the generation of strong vertical velocities and the fast and efficient transfer of heat, gases and energy from the air-sea interface into the ocean interior, with the ultimate aim of developing improved parameterizations of these processes for operational monitoring and Earth system models used for predicting future climate.
In this context, a significant challenge for the next decade will be to combine and interpret measurements of wind, currents, and waves from existing in situ and remote sensing observational platforms with new measurements from future Doppler oceanography satellites, in a modeling framework that constantly evolves toward finer spatial and temporal resolutions and increasingly complex coupled systems. In this paper, we review the present status of wind, current, and wave observations as well as existing platforms and their respective limitations, with an emphasis on remote sensing techniques (section 2). Then, we discuss the scientific community requirements for observations of these variables in the context of physical processes that happen at the ocean-atmosphere interface (section 3). Lastly, we explore the opportunities for better observations of surface winds, currents, and waves, as proposed by possible future Doppler oceanography from space missions (section 4). A summary and recommendations are presented in section 5.
2. Present State and Limitations of Wind, Current, and Wave Observations
During the past few decades, the oceanographic community has been trying to overcome the issue of sparse and heterogeneous measurements by adapting existing technology, applying novel data analysis techniques and processing tools, and combining observations from multiple sensors, with efforts to achieve higher resolution in space and time. For example, high-resolution imagery from synthetic aperture radars (SAR) and optical sensors onboard of satellites have been successfully used to study wind-current-wave interactions in specific regions (e.g., Rascle et al., 2016, 2017; Kudryavtsev et al., 2017), but these results have not yet led to the routine production of data. Significant scientific progress has been enabled by products, such as the Ocean Surface Current Analysis Real-time (OSCAR, Bonjean and Lagerloef, 2002), GlobCurrent (Rio et al., 2014), and the Cross-Calibrated Multi-Platform ocean surface wind velocity product (CCMP, Atlas et al., 2011); however, observational gaps in measurements of winds, currents, and waves still remain. Many components of the current observing system for surface winds (e.g., surface buoys and satellites), currents (e.g., HF-radar, surface drifters, and moorings), and waves (e.g., wave buoys) are illustrated in Figure 1. Below we discuss applications and limitations of each specific component.
2.1. Surface Winds
2.1.1. In situ Measurements
Measurements of surface winds over the ocean from weather ships and later from buoys began after World War II, motivated by the development of the aviation industry. Meteorological measurements from surface buoys remain an important source of near-real-time wind data for weather and navigational applications, and they are increasingly important for developing and validating estimates of winds from satellite and land-based remote sensing (Bourassa et al., 2019). Buoys are important for remote sensing because they provide an absolute calibration reference for satellite wind retrievals (Wentz et al., 2017). The buoys most commonly used for validating satellite wind retrievals are the tropical moored buoy arrays (TAO/TRITON in the Pacific, the PIRATA array in the Atlantic, and the RAMA array in the Indian Ocean), the network of buoys maintained by the US National Data Buoy Center (NDBC), the handful of the National Oceanic and Atmospheric Administration (NOAA) Ocean Reference Station buoys, and the coastal buoys maintained by the Canadian Department of Fisheries and Oceans (Wentz et al., 2017).
2.1.2. Scatterometers and Radiometers
As the wind blows over the surface of the ocean, short waves with scales of centimeters are formed, giving rise to what we refer as sea surface roughness. Remote sensing of ocean surface winds relies on the relationship between the wind speed and direction and the sea surface roughness, which modulates reflective and emissive properties of the ocean surface at those scales. Over the past two decades, the two most common sensors used to measure surface winds from space are microwave radiometers and scatterometers. Below we present a short description of these two technologies. For a detailed review of remotely sensed winds including instrument specifications, the reader is referred to Bourassa et al. (2019).
Microwave radiometers are passive sensors that estimate the wind speed based on the spectrum of the microwave radiation emitted by the sea surface, which, among other things, is a function of the sea surface roughness. Present oceanography satellites with onboard radiometers (e.g., the Advanced Microwave Scanning Radiometers, AMRS-2; and the Global Precipitation Measurement, GPM) are capable of estimating the wind speed with spatial resolution of about 30 km and accuracy of up to 1 m s−1; however, the quality of the wind speed measurements from this type of sensor is significantly degraded by the presence of rain (Meissner and Wentz, 2009). Another drawback of conventional microwave radiometers is that it is limited to measuring the surface wind only as a scalar quantity. Polarimetric microwave radiometers, such as WindSat, can be used to address this issue and retrieve the surface ocean vector wind, although the directional signal can be noisy for low wind speeds (< 7 m s−1) leading to uncertainties in the wind direction that can be >30° (Meissner and Wentz, 2005).
Scatterometers are active sensors that measure the fraction of energy from the radar pulse reflected back to the satellite, also known as backscatter. The backscatter is a function of the sea surface roughness, which is, in turn, a function of the wind speed and direction. The intensity of the backscatter for a given incidence angle determines the wind speed, while the wind direction is estimated by taking advantage of the fact that the measured backscatter is a function of the relative angle between the wind direction and the azimuth angle. The present constellation of scatterometers maps the surface wind field globally, with typical spatial resolution of 25 km and has been successfully used in weather forecasting applications (e.g., Atlas et al., 2001; Chelton et al., 2006), long-term climate studies (e.g., Halpern, 2002), and air-sea interactions (e.g., Xie et al., 1998; Chelton and Xie, 2010). The main limitations of scatterometers are contamination by rain (depending on the frequency of the transmitted signal), lack of data near the coast, and poor temporal sampling. Additionally, because of the way that backscatter depends on azimuth angle, possible wind directions can differ by 180°, which degrade the quality of the data. In rain-free conditions, wind directions (so-called ambiguities) are correctly identified more than 90% of the time; however, in or near rain events errors are more likely to occur. These problems can be reduced with antenna designs that obtain three or more looks at each location measured on the ocean surface (such the fan-beam design employed by NSCAT, ASCAT, and SCAT on board the China-France Oceanography SATellite, CFOSAT; and the rotating pencil-beam design used in QuickSCAT and RapidScat). Further improvements in the estimation of wind direction can be achieved by using Doppler directional information. Finer resolution would provide observations closer to the coast and better capture smaller-scale variability and derivative fields. Sufficiently small resolution (around 5 km) would allow scatterometers to see between rain features in hurricanes, and provide much greater utility in rain events. Temporal sampling could be improved with a mid-earth orbit or a synergetic constellation.
2.1.3. Synthetic Aperture Radars
Synthetic aperture radar (SAR) satellites are the only space system able to observe the ocean sea surface at day and night regardless of cloud coverage, with resolution of tens of meters and spatial coverage of hundreds of kilometers. Launched in 1978, SEASAT was the first satellite carrying a SAR (L-Band) together with a scatterometer (Ku-Band). Although originally designed for wave measurements, early comparisons demonstrated a strong correlation between the SEASAT SAR image intensity and SEASAT scatterometer wind speed (e.g., Weissman et al., 1979; Beal, 1980). Despite the short lifetime of SEASAT, the first analysis revealed some of the most interesting potential for SAR, such as its ability to monitor the ocean surface at high resolution under hurricanes (Fu and Holt, 1982) and the signature of the secondary atmospheric circulation in the marine atmospheric boundary layer (Brown, 1980, 1986). Gerling (1986) directly compared SAR wind speed and direction with scatterometer measurements, opening perspectives for high-resolution wind measurements from space.
Like existing scatterometers, SAR systems only measure the ocean surface backscattering in co-polarization (VV or HH). Taking advantage of accurate calibration with respect to SEASAT, algorithms were designed to provide a quantitative estimate of the wind speed and direction. Most of them rely on the so-called “scatterometry approach,” as described in section 2.1.2. However, in contrast to scatterometers, SAR systems do not have multiple (e.g., ASCAT) or rotating (e.g., QuikSCAT) antennae but only a single antenna pointing across track. This limits how well the inverse problem can be constrained, as only a single measurement is available to infer both wind speed and direction, in contrast to the three or more measurements that can be combined in the inversion scheme for scatterometers.
Various techniques exist to retrieve the wind direction and wind speed from the SAR image intensity, such as image processing techniques (e.g., Koch, 2004) that use ancillary data (e.g., wind direction from buoys, scatterometers or numerical weather prediction models). Recent missions, such as Radarsat-2 and Envisat allowed retrieval techniques to be refined to consider weak wind speeds and better calibrated data (Zhang et al., 2011; Mouche and Chapron, 2015). When Applied to C-band SAR, the scatterometry approach currently results in ocean wind vector measurements with root mean squared errors of <2 m s−1 for wind speed and <20° for wind direction.
The launch of Envisat and Radarsat-2 in the mid 2000s, opened a new area for SAR by providing the first evidence of a geophysical signature in the Doppler signal from a spaceborne SAR (Chapron et al., 2004, 2005). The relationship between wind waves and the Doppler from SAR allowed for inversion schemes that take advantage of the strong modulation of the Doppler with respect to wind direction in order to retrieve the surface wind vector (Mouche et al., 2012). The present generation of C-band SARs (e.g., Sentinel-1) have both co- and cross-polarization acquisition, which have recently been combined to retrieve ocean wind measurements in extreme conditions. These provide reliable wind measurements for maximum wind speeds of up to 60 m s−1 (Mouche et al., 2017). These results have attracted interest from outside of the SAR community. In particular, the high-sensitivity of the cross-polarization signal inspired future mission concepts (Fois et al., 2015), and EUMETSAT (European Organization for the Exploitation of Meteorological Satellites) together with ESA (European Space Agency) now plan to add a cross-polarized channel to the next generation of operational scatterometer missions (i.e., the next Polar System Second Generation) dedicated to the ocean surface wind measurement at medium resolution (Stoffelen et al., 2017). Other mission concepts (e.g., Ardhuin et al., 2018; Rodriguez, 2018; Gommenginger, 2019) also suggest relying on Doppler and radar backscatter measurements at multiple angles and targeting combined wind, waves, and current measurements.
Radarsat-2 and Envisat also allowed a new stage in the data acquisition by providing routine acquisitions over specific areas, yielding practical applications, such as the high-resolution wind Atlas for Europe (Hasager et al., 2015), and scientific applications, such as the study of the marine atmospheric boundary layer rolls in hurricanes (Foster, 2005). However, the very high resolution of SAR makes the analysis of the signal challenging. Many geophysical phenomena other than wind can impact the scales of wind-waves. These phenomena include rain (Atlas, 1994; Alpers et al., 2016), oceanic fronts (Kudryavtsev et al., 2014b), internal waves (Fu and Holt, 1982), and waves-current interactions (Kudryavtsev et al., 2014b). In addition, SAR is often used in coastal areas where strong interactions with topography and bathymetry can occur and sometimes dominate the wind-induced signal. This also lends support for a new generation of algorithms relying on multiple radar quantities to jointly invert for several geophysical parameters rather than deriving each parameter through an independent strategy.
2.1.4. Global Navigation Satellite System-Reflectometry
Global Navigation Satellite System-Reflectometry (GNSS-R) is an innovative Earth observation technique that exploits signals of opportunity from Global Navigation Satellite System (GNSS) constellations after reflection on the Earth surface. In brief, navigation signals from GNSS transmitters, such as those of the Global Positioning System (GPS) or Galileo are forward scattered off the Earth's surface in the bistatic specular direction. Dedicated GNSS-R receivers on land, on airborne platforms, or on separate spaceborne platforms detect and cross-correlate the reflected signals with direct signals from the same GNSS transmitter to provide geophysical information about the reflecting surface. GNSS-R can provide geophysical information about numerous surface properties and has multiple applications in Earth observation, including remote sensing of ocean roughness, soil moisture, snow depth, and sea ice extent (e.g., Cardellach et al., 2011; Zavorotny et al., 2014).
The exploitation of GNSS signals for ocean wind and sea state monitoring is one of the earliest and most mature applications of GNSS-R (e.g., Hall and Cordey, 1988; Garrison et al., 1998; Clarizia et al., 2009; Foti et al., 2015; Ruf et al., 2016). One key advantage of GNSS-R is the passive nature of the receiving hardware, which enables the design of low mass, low-power, low-cost instruments that can be flown on constellations of small satellites (e.g., Unwin et al., 2013) or as payloads of opportunity on other platforms/missions. This potential for low-cost implementation provides the option to build a comparably affordable Earth observation system characterized by sensors on multiple satellites to achieve very high spatio-temporal sampling of surface geophysical parameters. This offers significant benefits when trying to observe fast-varying processes, such as surface winds, sea state and tropical cyclones. In addition, by operating in the L-band microwave frequency range, GNSS-R is much less affected by heavy precipitation than other spaceborne measurement techniques, such as scatterometry, which operates at higher microwave frequencies (e.g., Quilfen et al., 1998).
Significant progress has been made over the past 5 years to quantify the capabilities of spaceborne GNSS-R to measure ocean winds and sea state, thanks to two GNSS-R missions: the UK TechDemoSat-1 mission launched in July 2014 (Foti et al., 2015) and the NASA Cyclone Global Navigation Satellite System (CYGNSS) launched in December 2016 (Ruf et al., 2016). In both cases, reported retrieval performances for GNSS-R wind speeds are better than 2 m s−1 root mean squared error (RMSE) for winds from 3 to 20 m s−1. In addition, GNSS-R observations from TechDemoSat-1 obtained in tropical cyclones indicate that spaceborne GNSS-R can depict fine-scale structures near the eye wall of hurricanes (Foti et al., 2017), thereby opening promising new opportunities as well as new challenges regarding the exploitation of GNSS-R to improve our understanding of hurricanes.
2.2. Surface Currents
2.2.1. Satellite Altimetry
Over the last 25 years, the most exploited system for the monitoring of ocean surface currents for ice-free global scale has been altimetry. This is due to the fact that the flow in the ocean interior (away from the boundary layers) and away from the equator is to leading order in geostrophic balance, which means that the ocean surface velocity field can be readily obtained from the gradients of the ocean dynamic topography (the sea level relative to the geoid). In ice-free conditions, altimetry provides global, accurate, and repeated measurements of the Sea Surface Height (SSH), which is the sea level above a reference ellipsoid and is made of two components: the geoid and the absolute dynamic topography. To cope with the lack of an accurate geoid at the spatial resolution of the altimeter measurements (a few kilometers along-track), altimeter measurements are time-averaged over a long time period (typically 20 years for the latest solutions). The resulting mean sea surface height (Andersen et al., 2016; Pujol et al., 2018) is removed from the instantaneous altimeter measurements to obtain measurements of the Sea Level Anomalies (SLA). Along-track SLA from multiple altimeter missions are combined to calculate gridded maps. The effective resolution of the SLA grid depends both on the number of satellites in the altimeter constellation and on the prescribed mapping scales. Analyzing the spatial coherence between the Copernicus Marine Environment Monitoring Service (CMEMS) altimeter maps and independent datasets, Ballarotta et al. (2019) found that multi-mission altimeter maps based on three satellites (available 70% of the time over the period 1993–2017) resolve mesoscale structures ranging from 100 km wavelength at high latitude to 800 km wavelength in the equatorial band over 4 weeks timescales.
A key reference surface needed to reconstruct the ocean dynamic topography from the sea level anomalies is the ocean Mean Dynamic Topography (MDT). The MDT is now known to centimeter accuracy at 100 km resolution through combined use of state-of-the-art mean sea surface (MSS) and GOCE (Gravity field and Ocean Circulation Experiment) data, at least in open ocean regions and away from coastal and ice-covered areas (Andersen et al., 2016). The use of additional information from in-situ oceanographic measurements (drifting buoy velocities and hydrographic profiles) allows the MDT to be refined to resolve scales down to 30–50 km (Maximenko et al., 2009; Rio et al., 2014; Rio and Santoleri, 2018). Effective resolution depends on the in-situ data density and is therefore not homogeneous (e.g., there are fewer in situ data at high latitudes and in coastal areas). Further developments are needed to increase the resolution of the MDT, in particular in the context of the upcoming SWOT mission, the primary objective of which is to characterize the ocean mesoscale and sub-mesoscale circulation with scales larger than 15 km. We refer the reader to Morrow et al. (2019) for a detailed description of the SWOT mission.
The first baroclinic Rossby radius in the ocean, which defines the expected spatial scales of geostrophic structures, ranges from 200 km at the equator to 10–20 km at high latitudes (Chelton et al., 1998; Nurser and Bacon, 2014). The mapping capability of the present altimeter constellation, coupled with the resolution and accuracy of the available MDT products, is not sufficient to resolve the full geostrophic flow at mid latitudes, and this is even worse at high latitudes. In addition, geostrophic currents are only one component of the total surface current in the ocean; other components include the Ekman currents, which are set up by a stationary wind field; tidal currents; and a number of other ageostrophic (i.e., not geostrophic) currents. In addition, the geostrophic approximation is not valid at the equator. At high latitudes, another limitation comes from the very coarse sampling of the ice-covered ocean where leads allow only a sparse view of the dynamic topography (Armitage et al., 2017), which particularly excludes the mesoscale, and the MIZs. The altimeter observing system, therefore, suffers from two major limitations in monitoring ocean surface currents: only the geostrophic component of the currents can be derived, and in some areas, only for a limited range of spatial scales.
In order to obtain more realistic ocean surface currents, corrections may be made to the altimeter-derived geostrophic currents. In ice-free oceans (Dotto et al., 2018), Ekman currents can be estimated, given knowledge of the wind field (Rio and Hernandez, 2003), and added to the geostrophic currents. Various global ocean surface current products are now available based on such an approach: the OSCAR product (Bonjean and Lagerloef, 2002), the Geostrophic and Ekman Current Observatory (GEKCO) product (Sudre et al., 2013), and the GlobCurrent product (Rio et al., 2014). Figure 3 shows an example of the surface velocity field for December, 31st 2017 from the GlobCurrent product, which includes both altimetry-based geostrophic velocity and wind-derived Ekman currents. Alternatively, the spatial and temporal resolution of the altimeter-derived ocean surface currents may be enhanced by exploiting the synergy between altimetry and other satellite observations. A number of methods have been tested, including Maximum Cross Correlation (e.g., Bowen et al., 2002; Warren et al., 2016), the effective Surface Quasi Geostrophy framework (e.g., Isern-Fontanet et al., 2006; González-Haro et al., 2016), and inversion of the SST conservation equation (e.g., Vigan et al., 2000; Rio et al., 2016; Rio and Santoleri, 2018), as illustrated in Figure 4.
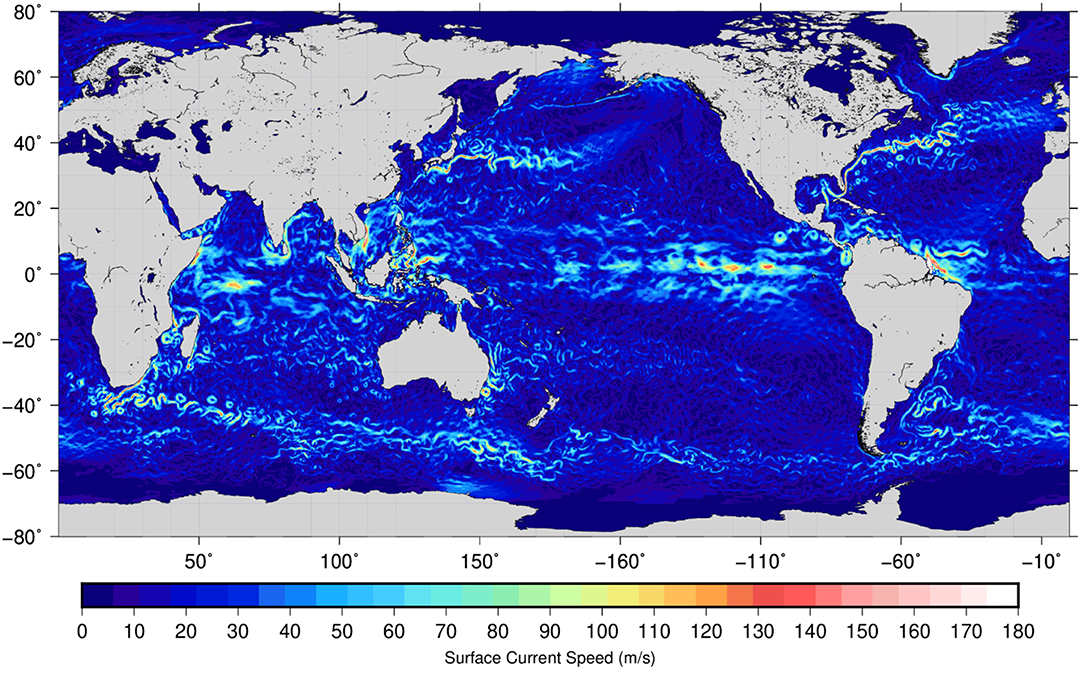
Figure 3. Map of combined geostrophic and Ekman surface currents on December, 31st 2017 from the GlobCurrent project (Rio et al., 2014).
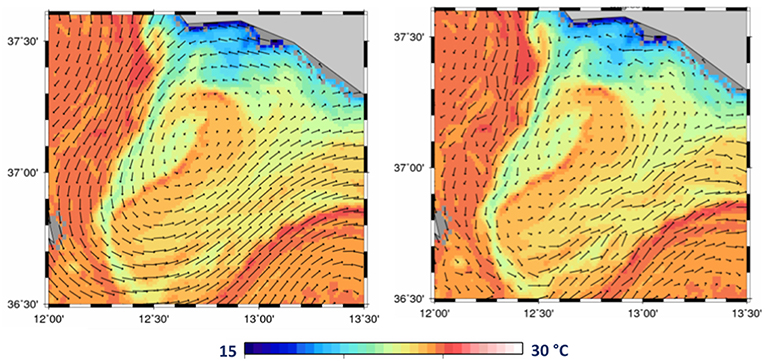
Figure 4. Sea surface temperature (SST) in the Sicily channel (Mediterranean Sea) on July, 23rd 2016 from Sentinel-3 and ocean surface currents derived (left) from the Sentinel-3 altimeter data and (right) from the combination of the Sentinel-3 altimeter and SST information using the method described in Rio and Santoleri (2018).
2.2.2. Surface Drifters
Surface drifters are semi-Lagrangian drifting buoys that approximately follow the current at the ocean surface and can be used in climate and oceanographic research. For over four decades, satellite-tracked surface drifters have been used to map near-surface currents in the global oceans (Lee and Centurioni, 2018) as part of the Global Drifter Program (GDP). Currently, an array of over 1,400 surface drifters is maintained through GDP, with the goal to keep an average drifter spacing of 5 degrees in the entire globe. However, sustaining the number of drifters in regions of predominantly divergent flows, such as the equatorial region, is difficult since the divergence of the surface flow results in a continuous drifter loss toward the subtropics.
Surface drifters from the GDP consist of surface drifting buoys that have an attached holey-sock drogue (sea anchor) centered at a depth of 15 m and are tracked mostly using the Argos positioning system (http://www.argos-system.org), but recently also using GPS (Elipot et al., 2016). Motions due to slip caused by windage, surface gravity wave rectification, and Stokes drift are major challenges for interpreting currents from surface drifters (Lumpkin et al., 2017). Even though the use of a drogue and careful design of the surface buoy can greatly reduce slip to 0.1% of the wind speed for 10-m winds of up to 10 m s−1, the resulting velocity estimated from the drifter is still a combination of the direct wind-driven surface current, plus the slip, plus the integrated shear between the surface and the end of the drogue. Several methods for correcting for slip bias in both drogued and undrogued drifters have been proposed (e.g., Pazan and Niiler, 2001; Poulain et al., 2009) and have been recently updated by Laurindo et al. (2017). On average, GDP drifter position fixes are received every ~1.2 h and can be used to estimate near-surface velocities by finite differencing consecutive fixes. The standard product distributed by GDP objectively interpolates velocities to regular 6-h intervals and has been used to map large-scale ocean currents (Lumpkin and Johnson, 2013), study pathways of marine debris (Maximenko et al., 2012), and improve satellite-based products (Rio et al., 2014). Taking advantage of improvements in the temporal sampling of the drifters since 2005, the GDP has recently developed an alternative drifter velocity product that distributes surface velocities at 1-h intervals. These higher-frequency velocities have the potential to be used to investigate inertial, tidal, and super-inertial motions (Elipot et al., 2016; Lumpkin et al., 2017).
The coarse and scattered distribution of drifters from the GDP limits their application to relatively large-scale processes. The development of low-cost, disposable, and biodegradable drifters (e.g., the CARTHE drifter) has allowed for large deployments of an unprecedented number of drifters (O(10)3) capable of monitoring for the first time rapidly-evolving submesoscale (<10 km) motions as well as clustering and dispersion of floating particles. At these scales, surface convergences and divergences lead to abrupt changes in the concentration of floating materials, resulting in strong gradients that can have profound implications for oil spills, larval dispersion, and pathways of plastic debris (D'Asaro et al., 2018). While surface drift measurements from a few experiments, such as the Grand Lagrangian Deployment (GLAD) and the Lagrangian Submesoscale Experiment (LASER) have shed some light onto submesocale dynamics, a systematic means of monitoring the surface of the ocean at these scales is needed in order to bridge the gap between mesoscale and submesoscale processes and to improve model predictions in response to environmental disasters.
2.2.3. High Frequency Radar
Shore-based high-frequency radars (HFR), which provide measurements of surface currents, are important components of coastal observing systems. HFRs transmit radio signals (3–45 MHz) and make use of Bragg resonant reflection from wind-driven surface gravity waves, in combination with the dispersion relationship, to derive surface currents from the Doppler shift in the returned signal (Crombie, 1955; Barrick et al., 1977). Operational networks of HFRs provide near real-time measurements of surface current fields with 0.5–6 km horizontal and 1-h temporal resolution for distances extending to 300 km offshore. Data from these systems support both scientific and operational efforts, including oil spill response, water quality and pollution tracking studies, fisheries research, maritime domain awareness, search and rescue, and adaptive sampling (Terrill et al., 2006; Harlan et al., 2010).
HFR derived surface currents have been used in a wide variety of scientific studies (see Paduan and Washburn, 2013) to map tidal currents, eddies, wind and buoyancy-driven currents, and for model validation and data assimilation. Kim et al. (2011) used 2 years of data from the US West Coast HFR network to capture various scales of oceanic variability, including the existence of poleward propagating wave-like signals along the US coastline presumably associated with coastal-trapped waves. Wavenumber (k) spectra of measured currents show a k−2 decay at scales smaller than 100 km, consistent with theoretical submesoscale spectra (McWilliams, 1985). HFR spatial resolution is generally higher than satellite altimeters, providing unique insight into submesoscale variability in the coastal zone. Marine ecological studies have used HFR systems to map harmful algal blooms (Anderson et al., 2006) and larval transport pathways (e.g., Gawarkiewicz et al., 2007), tying the biological response to the physical environment.
HFR is susceptible to external Radio Frequency Interference (RFI), which has been mitigated in recent years by international adoption by the radio community of set aside bands for oceanographic applications. While HFR for oceanography can span 3–45 MHz, at lower frequencies (typically below 8 MHz), HFR can be impacted by interference from diurnal variations in the ionosphere, which result in higher noise levels as a result of long-range propagation conditions. Within embayments, such as San Francisco Bay, HFRs have been shown to be effective when operated at the higher frequency bands, due to the availability of short period Bragg waves. The radar systems require ongoing maintenance and recalibration of antenna patterns due to seasonal changes in surrounding vegetation and other effects (Cook et al., 2008). HFR has also been used to measure components of the surface wave field due to the second order backscatter effects in the Doppler spectrum. However, this technique has not been shown to provide the same level of fidelity as in-situ measurements or imaging style radars that operate at X-band. An in-depth review on HFR can be found in Roarty et al. (2019).
2.2.4. Moorings
One direct approach to measuring ocean currents is to install current meters or current profilers on a mooring line that runs between an anchor on the seafloor and a flotation buoy. If the flotation buoy is on the surface, it is a “surface mooring,” and, if the buoy is beneath the surface, it is a “subsurface mooring.” Early current meters measured current speed by measuring the revolutions of a propeller or rotor (e.g., Weller and Davis, 1980), but almost all modern “in situ” ocean velocity measurements use acoustic techniques relying on measurement of acoustic travel times or Doppler shifts. Acoustic Doppler current profilers (ADCPs) allow measurement of velocity profiles and are now one of the most commonly used instruments for measuring ocean currents in situ. A great advantage of moored velocity measurements is that they can provide very good temporal resolution, with a typical temporal resolution of 1 h for a 1-year record.
The near-surface environment is challenging because of the action of surface waves and biofouling. The surface waves cause physical heaving and strong, oscillatory wave-driven flow past the instruments, which can cause: (1) mechanical damage to the mooring and instruments, (2) flow-distortion errors (e.g., from flow separation near the buoy or instrument), (3) sampling errors (e.g., from aliasing of the wave orbital velocity), and (4) difficulties in interpretation because the instruments heave up and down in a surface-following reference frame (which is a mix of Eulerian and Lagrangian reference frames and consequently causes partial contamination of the mean velocity by the Stokes drift (Pollard, 1973). Although there are many oceanographic surface moorings, most of these moorings do not measure near-surface ocean currents. There are only a handful of moored records of open-ocean currents taken in the upper 10 m of the ocean. The records that do exist should be used with caution because of the challenges listed above.
2.2.5. Sea Ice Drift
Finally, a special case of surface currents is the drift of sea ice. Different methods probe different parts of the spatiotemporal spectrum. Buoys drifting with the sea ice (Rampal et al., 2011; Gimbert et al., 2012) provide a very high sampling rate but offer a very local sampling of the sea ice cover. On the other hand, image correlation techniques from passive microwave sensors (Tschudi et al., 2016) or SAR (Kwok et al., 1998) offer a pan-Arctic view of the deformation features of the sea ice but are limited to coarser length scales of deformation, typically larger than 10 km for passive microwave and 1 km for SAR imagery and to daily to monthly timescales (for more recent reviews see Sumata et al., 2015; Muckenhuber and Sandven, 2017). Doppler shift analysis techniques (Chapron et al., 2005) provide near instantaneous (sub-hourly) surface displacements but offer sparse spatial sampling that limits measurements to one component of the ice drift (Kræmer et al., 2018). Finally, recent results (Oikkonen et al., 2017) using correlation of ship-based radar images offer a sub-kilometric view of sea ice kinematics at timescales down to tens of seconds but are inherently limited in space and time to icebreaker routes. In this context the new rotating multibeam Doppler SAR technology on board the proposed SKIM ESA explorer mission will complement existing techniques and in particular will expand on the existing delay-Doppler products by resolving the second component of the sea ice drift vector at a near instantaneous frequency and kilometric resolution with a daily coverage over most of the Arctic (Ardhuin et al., 2018).
2.3. Surface Waves
2.3.1. Wave Buoys and Wave-Enabled Drifting Buoys
The majority of historical wave measurements have been collected from moored sensors near coastlines with limited spatiotemporal information about the wave field offshore. In general, high-seas wave observations are sparsely collected from ship observations or from satellites, which have long duration repeat intervals. Moored buoys use heave-pitch-roll sensors, accelerometers, or displacement sensors to measure orthogonal components of some combination of the surface wave kinematics, and they invert these data for the first five directional moments at each frequency (Longuet-Higgins et al., 1963), which can be used to obtain an estimate of the wave directional spectrum using statistical methods (e.g., Lygre and Krogstad, 1986). To eliminate the cost and effort of maintaining moored buoys, a growing number of small-form-factor, easily deployable surface drifters (Veeramony et al., 2014; Centurioni et al., 2017) with high fidelity wave measurements have been developed for remote and under-sampled regions of the global ocean.
Drifting wave buoys use GPS signals from a single GPS receiver to measure horizontal and vertical velocities (De Vries et al., 2003). The three-axis GPS velocity data are used to obtain wave displacement spectra in a manner similar to the more traditional buoy technology referred to above. Wave measurements from these cost-effective and compact counterparts to the moored wave buoys have been shown to compare well with traditional accelerometer methods (Colbert, 2010; Herbers et al., 2012). Applications of these drifting buoys include wave attenuation in ice (Doble and Bidlot, 2013; Doble et al., 2017; Sutherland and Dumont, 2018), targeted sampling under storm tracks, wave-current interactions (Zippel and Thomson, 2017; Veras Guimarães et al., 2018), and wave observations on high seas where mooring buoys are technically challenging and costly. For detailed characteristics of in situ wave measurements, we refer the reader to Ardhuin et al. (2019).
2.3.2. Satellite Remote Sensing
In contrast to the point measurements provided by buoys, remote sensing satellites provide a unique global view of the ocean that is capable of sampling the most extreme conditions, for which no buoy record is available. Currently, the most robust satellite-based measurement of the sea state is the significant wave height (Hs) derived from satellite altimeter waveforms as a byproduct of the SSH processing. Since measurements of Hs are not the primary goal of present altimeters, their sensors are not optimized for measuring the sea state, and the first step one typically goes through when using standard altimetric products is to smooth out the noise by averaging Hs values along-track over a distance of the order of 50 km. In addition to being relevant to the wave community, altimeter measurements of Hs are also an important parameter for estimating and correcting the sea state bias in the SSH measurements (Fu and Glazman, 1991). Because of their global sampling, altimeters are uniquely capable of measuring the most extreme sea states: the highest Hs value ever recorded in a 1-Hz product is 20.1 m (Hanafin et al., 2012). At the other extreme, altimeters have difficulty resolving wave heights below 1 m (e.g., Sepulveda et al., 2015). Altimeters also provide a back-scatter power that, when well-calibrated, can be used to estimate the mean square slope of the sea surface (Jackson et al., 1992; Nouguier et al., 2016).
More information on the sea state, in particular, the direction, wavelength, and energy of swells can be obtained from high-resolution imagery of the ocean. The most common form of wave measurement from imagery uses the specially designed “wave mode” of SARs on ESA satellites ERS-1/2, Envisat, and Sentinel 1 (Hasselmann et al., 2013). This wave mode is particularly well-suited for the routine tracking of swell fields across the oceans (Collard et al., 2009). Unfortunately, it is unable to detect the part of the wave spectrum associated with shorter wind waves, due to the blurring of the SAR image by the wave orbital velocities; the orbital velocities can still be estimated by statistical methods, albeit with limited accuracy (Li et al., 2011). This “cut-off” between the resolved and blurred part of the spectrum is strongest in the azimuth (along-track) direction and is a function of the sea state. Waves traveling in the azimuth direction with wavelengths shorter than 100 m can only be measured in quiet conditions or ice-covered oceans (Ardhuin et al., 2017b). In fact, SARs are the only satellite systems that have been proven to measure wave heights in ice-covered regions. Other types of radars (e.g., wave scatterometers) do not use SAR processing and provide 1D spectra along the line of sight of a rotating beam that can be combined to produce a 2D spectrum (Jackson et al., 1992; Caudal et al., 2014). The first space-borne wave scatterometer, the China-France Ocean Satellite mission (CFOSAT), was recently launched on October, 2018 (Hauser et al., 2017).
Other optical imagery approaches, even if they cannot offer a full global monitoring due to particular observation (cloud cover and sun position), are unique in their resolving capability with, for example all coastal areas covered by Landsat and Sentinel 2A and 2B satellites. Figure 5 shows an example of a Sentinel 2 image and the wave analysis from it compared to wave data from NDBC buoy 46086. The omnidirectional spectrum (panel c), shows overall good agreement with the measurements from the wave-buoy.
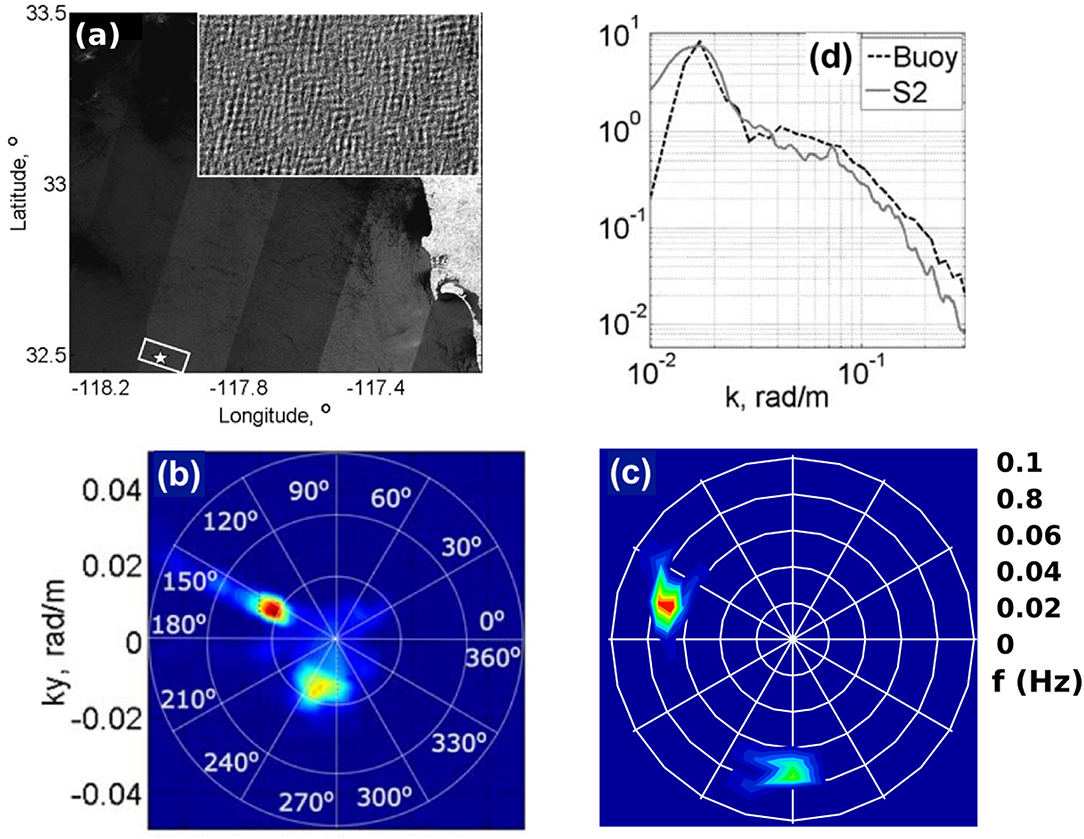
Figure 5. (a) A Sentinel-2 image off the California coast taken on 29, April 2016. The inset delimits the region over which the spectral analysis (shown in the other panels) is performed and the star marks the location of the NDBC buoy 46086. (b) The two dimensional unambiguous image spectrum, over the area show in the inset on (a), from Sentinel-2 using the time separation of different detector acquisitions. Blue colors indicate low wave energy density, whereas warm colors indicate high wave energy density (c). The directional spectrum from the NDBC buoy 46086 estimated using the maximum entropy method of Lygre and Krogstad (1986). (d) The direction-integrated surface wave spectrum from the Sentinel-2 (solid) corresponds well to the buoy data (dashed) for wavelengths from 62 to 420 m, namely k between 0.015 and 0.1 rad/m or frequency from 0.06 to 0.15 Hz. This figure is adapted from Kudryavtsev et al. (2017).
3. Science Topics: Community Needs for Integrated Observations of Surface Currents, Winds, and Waves
3.1. Open Ocean Circulation and Budgets
3.1.1. Equatorial Dynamics
Climate variability in the tropical oceans is dominated by air-sea interactions associated with thermodynamic and dynamic feedback mechanisms. Surface wind is a crucial parameter for the turbulent heat flux, which has implications, for example, for establishing the meridional climate mode in the Atlantic. At the same time, surface winds dynamically drive tropical upwelling along the eastern boundary and at the equator. The zonal winds along the equator are an integral element of the Bjerknes feedback responsible for the development of the Pacific El Niño or the Atlantic Niño (Bjerknes, 1969). Besides the wind, ocean surface velocity is an essential parameter defining tropical ocean dynamics and air-sea interactions including processes, such as equatorial waves, tropical instabilities, as well as heat and freshwater advection and entrainment contributing to the mixed layer budgets (Foltz et al., 2018). Surface velocity divergence and associated upwelling is responsible for changes in the mixed-layer depth that is additionally forced by air-sea buoyancy fluxes or mixing and entrainment at the base of the mixed layer. The mixed-layer heat budget represents a central element for understanding the mechanisms governing tropical SST variability and the causes of the still severe biases in tropical regions in climate models (Zuidema et al., 2016). Within the seasonal cycle, zonal advection is, besides diapycnal mixing, the main cooling agent in the central equatorial Atlantic, and a dominant term in the mixed-layer salinity budget (Foltz and McPhaden, 2008). Eddy advection mostly by tropical instability waves counteracts the cooling by diapycnal mixing in the eastern equatorial cold tongue region (Weisberg and Weingartner, 1988; Hummels et al., 2014).
Up to now, velocity data used to estimate tropical mixed-layer heat and freshwater budgets are based on spatially distributed surface drifters and surface displacements by Argo floats, as well as on velocity observations at moored buoys. Surface drift data allow climatological mean heat advection to be estimated and, in combination with total advection derived from temperature changes along Lagrangian surface drifter paths, eddy heat advection (Swenson and Hansen, 1999). However, mean seasonal budgets have substantial error estimates (Hummels et al., 2014), indicating the inadequacy of combined drifter and float data for addressing interannual variability or long-term changes of advective terms within the heat and freshwater budgets. Moreover, the mixed-layer depth in tropical upwelling regions is often <10 m. Under such conditions, surface drifters, equipped with drogues centered at 15 m depth, measure velocity in the shear zone below the mixed layer and thus do not represent mixed-layer advection. Argo floats drifting at the surface instead measure the velocity in the upper meter of the ocean, which becomes complicated for mixed-layer budget calculations due to the existence of diurnal shear (Smyth et al., 2013; Wenegrat and McPhaden, 2015). Moored velocity observations performed at the tropical buoy array at a depth of 10 m deliver high-resolution time series. However, the spacing between the buoys (typically more than 10 degrees in longitude and a few degrees in latitude) do not resolve the near-equatorial current bands or the mesoscale variability including tropical instability waves. Surface currents from merged products, such as OSCAR, described in section 2.2.1, are often used in addition to directly-measured velocities from drifters, floats, and moorings. While OSCAR velocities are generally a well-proven data product, they largely fail to represent intraseasonal meridional velocity fluctuations near the equator and misrepresent seasonal and longer-term equatorial zonal velocity variability (Schlundt et al., 2014).
Continuous high-resolution measurements of absolute surface velocity would represent a significant step forward by improving mixed-layer heat and freshwater budgets and by refining our understanding of the general circulation of the tropical ocean. At the same time they would pave the way for new process studies, for example by enabling study of the role of tropical instability waves on the heat budget (Jochum et al., 2004) or the imprint of equatorial deep jets or high baroclinic mode waves on the sea surface and their impact on SST (Brandt et al., 2011), none of which are currently possible due to limited and sparse data coverage.
3.1.2. Atmospheric-Ocean Carbon Exchange and Transport
The oceans act as a sink of atmospheric carbon dioxide (CO2), and they are the largest long-term natural sink of CO2 (Sabine et al., 2004), annually absorbing more than 25% of anthropogenic emissions (Le Quéré et al., 2017). Quantifying this absorption is critical for quantifying global carbon budgets (e.g., as quantified by Le Quéré et al., 2017). Once dissolved in seawater, CO2 is partitioned into different carbonate species, and these are transported throughout the ocean. This long-term absorption of carbon is slowly lowering the pH of the water, impacting the marine environment. Consequently the synoptic and long-term monitoring of the atmosphere-ocean exchange of carbon and the subsequent transport of carbon within the ocean interior and across continental shelves is highly relevant to society. We are currently able to observe the total atmosphere-ocean exchange of CO2 (e.g., Watson et al., 2009; Woolf et al., 2016), and synoptic scale observations of this exchange require both satellite observations (e.g., sea state, temperature, wind) and in situ observations (e.g., gas concentrations). Existing synoptic scale observations of surface transport predominantly rely upon satellite altimetry or exploit spatially and temporally sparse in situ measurements (e.g., Painter et al., 2016).
However, atmosphere-ocean gas exchange is primarily driven by surface turbulence, such as wind-wave-current interactions, but most gas exchange relationships are parameterized solely in terms of wind speed (e.g., Wanninkhof, 2014). Similarly, the exchange of waters between the shelf seas and the open ocean (at both the surface and at depth) is highly dependent upon surface currents flowing onto the shelf, which include ageostrophic components not well-captured by altimetry.
A lack of suitable synoptic-scale measurements of surface currents, winds, waves, and their interactions hampers our understanding how these processes combine and control atmosphere-ocean exchange and across-shelf exchange. Doppler oceanography from space has the potential to address this gap in observations. For example, satellite sensors which are able to directly observe wind-wave-current interactions hold the potential to provide direct observations of energy dissipation and turbulence at the surface. This would enable the development and evaluation of new physically based atmosphere-ocean gas exchange parameterizations.
3.1.3. Inertial Currents
“Inertial currents” or “inertial oscillations” occur when the Coriolis force causes water that is moving only by virtue of its own inertia to rotate anticyclonically (clockwise in the Northern Hemisphere and counterclockwise in the Southern Hemisphere) at the local Coriolis (or “inertial”) frequency. Whenever there is a short-lived wind event, such as a storm, the inevitable result is a mixed-layer inertial current, because the ocean freely resonates at the inertial frequency. In addition, the ocean can also be forced to resonate at the inertial frequency if the wind vector rotates at this frequency (e.g., D'Asaro, 1985).
Frequency spectra of oceanic velocity records almost always exhibit a prominent spectral peak near the local inertial frequency, and these near-inertial oscillations are typically the dominant velocity signal in the open ocean at periods less than a few days (e.g., Fu and Glazman, 1991). Inertial oscillations are an important source of vertical shear in the ocean and can thus drive vertical mixing (e.g., Alford, 2010). There are several unresolved research questions related to upper-ocean inertial currents, including ones related to the energy input from the wind to inertial motions, the interaction of inertial oscillations with mesoscale motions (Alford et al., 2016), and the amount of inertial energy that penetrates below the mixed layer via near-inertial waves (e.g., MacKinnon et al., 2013). Because near-inertial oscillations tend to be the largest contribution to velocity variability at periods less than a few days, they are also important for operational applications.
These high-frequency inertial currents pose a sampling challenge for the limited temporal sampling for the WaCM, SKIM, or SEASTAR missions (on the order of a day for WaCM), but there are three factors that should make this challenge more manageable. First, while the inertial oscillations are more prominent than other high-frequency motions, they still have less variance than lower frequency motions, such as mesoscale eddies, which limits the potential contamination of low frequencies. Second, it may be possible to remove inertial currents that are not well-resolved in time using simple dynamical models, which have shown skill in simulating mixed-layer inertial currents given estimates of the local wind stress (e.g., D'Asaro, 1985; Plueddemann and Farrar, 2006), and continuing improvements in ocean general circulation models and the forcing fields should allow even more realistic simulations (e.g., Simmons and Alford, 2012; MacKinnon et al., 2017). Finally, ongoing work from numerical simulations suggests that one could use physical properties of inertial oscillations to better separate low and high frequencies. Since inertial oscillations ring for several inertial periods, their amplitude and phase could be estimated even if they are not resolved in time, for instance, with daily observations of velocity.
3.1.4. Lagrangian Pathways of Plastic Debris and Other Floating Material
The issue of marine debris, most prominently plastic, has received significant attention in the last decade. There are at least a few trillion pieces of plastic afloat on the surface ocean, weighing at least 100,000 metric tons (Van Sebille et al., 2015). This plastic enters the ocean from coastlines and rivers (Jambeck et al., 2015; Lebreton et al., 2017) and is then transported by currents, waves, and winds. Due to biofouling, most of the plastic will beach or sink on time scales of weeks to years, but the fraction that stays afloat will eventually move into one of the infamous garbage patches in the centers of the subtropical gyres (e.g., Law et al., 2010, 2014; Law, 2017), where it can linger for many decades.
The transport and pathways that this floating material takes are very sensitive to the ocean currents, on scales from meters to kilometers (LaCasce, 2008; D'Asaro et al., 2018). Furthermore, it has recently been shown that Stokes drift can have a profound impact on the basin-scale pathways of floating material. Using a finding of invasive kelp on the shores of the Antarctic Peninsula, that was genetically identical to kelp found on the Kerguelen Islands, Fraser et al. (2018) were able to explain the southward transport of floating kelp against the dominant Ekman transport only when they included Stokes drift in their model simulation; without this Stokes drift, no Lagrangian particles were able to travel from Kerguelen to Antarctica.
It has long been known that surface drifting buoys travel differently when they are drogued vs. when they are undrogued (Lumpkin and Pazos, 2007). The tsunami following the Fukushima disaster also highlighted the importance of windage in cross-basin transport (and particularly speed) of debris. In order to be able to compute the dispersion of floating debris, biological material and human-made objects in search and rescue, it is critical to have as accurate flow, waves and winds fields as possible (Van Sebille et al., 2017). Ideally, these should come from novel remote sensing techniques capable of measuring surface winds, total surface currents, and waves globally.
3.2. Coastal, Shelf, and Marginal Ice Zone Processes
3.2.1. Continental Shelf Flows
At time scales longer than diurnal, currents on continental shelves and shelf slopes tend to flow nearly along-isobath (Lentz and Fewings, 2012) and often transport water for long distances along shelves. An example is the current system that transports water from east of Greenland, around the Labrador Sea to the Gulf of Maine and Middle Atlantic Bight, before turning offshore at Cape Hatteras (Chapman and Beardsley, 1989; Fratantoni and Pickart, 2007). The quasi-continuous shelf flow and shelfbreak jet system is an important conduit of cold low salinity water from high to mid latitudes in the western North Atlantic (Lentz, 2010) and transports anomalies in both heat and salt (Shearman and Lentz, 2010; Feng et al., 2016). Similar shelf current systems exist in other ocean basins. In the southwestern Atlantic, for example, there is a continuous along-shelf flow that transports high-nutrient waters from the Drake Passage to the Brazil/Malvinas Confluence (Matano et al., 2010). We do not have long-term observations or monitoring of the intraseasonal to interannual variations of these important continental shelf and shelf break current systems except in a few locations with moored current meter arrays (e.g., https://oceanobservatories.org/array/coastal-endurance/ and https://oceanobservatories.org/array/coastal-pioneer/). Satellite altimeters presently give limited information on flows on continental shelves, especially shoreward of the shelf break, except in regions with tide gauges (Feng et al., 2016; Risien and Strub, 2016). The along-shelf velocities along both eastern and western ocean boundaries are 10s of cm s−1 and could be monitored with Doppler surface current measurements. Simultaneous observations of winds would permit better understanding of the forcing of these shelf flows, which are driven by a combination of wind and along-shelf pressure and density gradients (Pringle, 2018). A better understanding of the dynamics of continental shelf, shelf break, and slope flows would lead to better capability for ocean monitoring and prediction, such as monitoring across-shelf exchange of carbon, heat, nutrients, and marine debris and improving seasonal forecasts of shelf water conditions in the downstream direction. Such capability would improve our understanding of the connections between shelf and deep ocean waters, allowing us to better anticipate the impacts of large spatial and temporal scale phenomena, such as our changing climate, on coastal regions.
Though continental shelf flows are constrained by the Earth's rotation to flow mostly along-isobath on long time scales, continental shelves do exchange water with the adjacent open ocean, with consequences for marine productivity (Brink, 2016b). Shelf eddies (Brink, 2016a, 2017; Brink and Seo, 2016), for example, play important roles in cross-shelf transport of heat, freshwater, and biogeochemical tracers. Deep-water mesoscale eddies and warm- and cold-core rings impacting the shelf slope can draw filaments of shelf water offshore or inject offshore water onto the shelf (Gawarkiewicz et al., 1990; Zhang and Gawarkiewicz, 2015; Cherian, 2016; Cherian and Brink, 2016, 2018). In other regions, such as the Brazil/Malvinas Confluence, exchange between the shelf and the deep ocean is not only controlled by eddies but also by narrow and well-defined coastal currents (Piola et al., 2005; Matano et al., 2010). The spatial scales of the shelf eddies and the filaments are 10–50 km, and the velocity scale is 10s of cm s−1. Measurements of the velocity structure of these eddies, filaments, and narrow coastal currents, and simultaneously the wind fields that affect transport in the surface boundary layer, would enable better understanding of ocean productivity and shelf-ocean exchange of carbon, pollutants, and other substances.
Wind-driven cross-shelf transport is an important mechanism for nearshore-midshelf and shelf-ocean exchange. In broad, shallow shelf seas, cross-shelf transport of water can bring open ocean low nutrient surface waters onto the shelf, and help to force the offshore transport lower in the water column of carbon rich water from the shelf to the deep ocean, sometimes called the “Ekman drain” (Painter et al., 2016). In eastern boundary upwelling systems (Chavez and Messié, 2009) where the mean wind forcing is substantial, upwelling brings nutrient rich, but low pH, low oxygen water to the surface, which can be detrimental to marine ecosystems (Grantham et al., 2004; Chan et al., 2008; Connolly et al., 2010; Siedlecki et al., 2015; Adams et al., 2016). Weakening, or relaxation, of upwelling-favorable winds in these systems can enable transport of carbon off the shelf (Karp-Boss et al., 2004; Hales et al., 2006) and lead to coastally trapped warm oceanic poleward flows with cross-coast scales of 10s of km or less and velocities of 10s of cm s−1 (Washburn et al., 2011; Suanda et al., 2016). Coastal and shelf seas are considered important for the surface absorption of atmospheric CO2, but the strength and state of this sink remain uncertain (Hales et al., 2005; Chen et al., 2013; Laruelle et al., 2014) and may diminish in the future due to changes in climate, environmental conditions and management (Regnier et al., 2013). On shallow inner shelves where the surface and bottom boundary layers overlap, the dynamics controlling the flow are different from on the middle and outer shelf (Fewings and Lentz, 2010; Lentz and Fewings, 2012). On broad shelves characteristic of passive continental margins, the inner shelf may extend 10s of km offshore, depending on the wind forcing and density stratification. In this region, cross-shelf winds and surface gravity waves can both drive cross-shelf flows, in contrast to the middle and outer shelf where the along-shelf wind is the primary driver for cross-shelf transport (Fewings et al., 2008; Lentz et al., 2008; Kirincich et al., 2009; Horwitz and Lentz, 2014, 2016). Due to the asymmetry in fetch for onshore and offshore winds in coastal areas, low-frequency flows associated with surface gravity waves, apparently due to Stokes-Coriolis forcing, can confound observations of wind-driven surface flows (Fewings et al., 2008; Kirincich et al., 2010; Ohlmann et al., 2012). Surface currents within ~10 km of the coast can vary on alongshore scales <10 km and be poorly correlated with or even opposite in direction to currents farther offshore (Fewings et al., 2015). These alongshore flows are important for modifying local water temperatures on both weather-band and seasonal time scales (Austin, 1999; Fewings and Lentz, 2011; Connolly and Lentz, 2014). Simultaneous measurements of the surface currents, surface waves, and local wind stress along coastlines worldwide would enable monitoring of ongoing changes in ecologically and economically important boundary current systems (García-Reyes and Largier, 2010) and better process-based understanding, modeling, and prediction of cross-shore transport of nutrients and pollutants, transport of phytoplankton and larvae (Cowen and Sponaugle, 2009; Drake et al., 2013; Simons et al., 2013; Criales et al., 2015), and coastal fisheries productivity (Kaplan et al., 2016; Siedlecki et al., 2016).
River plume outflows and the resulting fresh coastal currents are heavily influenced by local wind forcing (Garcia Berdeal et al., 2002). During upwelling-favorable winds, the plume waters are transported offshore and the wind causes dilution of the plume by mixing with ambient ocean water (Fong and Geyer, 2001; Lentz, 2004; Hickey et al., 2005). Conversely, in light wind conditions or under downwelling-favorable winds, the plume becomes trapped against the coast and can propagate rapidly (>1 m s−1) alongshore for 10s to hundreds of km, spreading nutrients, larvae, and pollutants (Münchow and Garvine, 1993; Rennie et al., 1999; Fong and Geyer, 2002; Lentz et al., 2003; Hickey et al., 2005; Lentz and Largier, 2006). Individual SAR images can show these plumes in great detail (Donato and Marmorino, 2002) but do not provide information about the time evolution of the plumes. In the Pacific Northwest in the U.S., the $100M shellfish industry (Dumbauld et al., 2011) is affected when Columbia River plume waters enter nearby estuaries, modifying the water chemistry in areas where oyster aquaculture takes place (Banas et al., 2004). In the southwestern Atlantic, the freshwater discharge from the Rio de la Plata extends hundred of kilometers, influencing the most densely populated regions of Argentina, Uruguay and Brazil and affecting some of the richest marine ecosystems of the South Atlantic (Piola et al., 2005). The strong fronts associated with river plumes affect the transport and fate of pollutants, including oil spills (Roth et al., 2017). The plume currents are 10s of cm s−1 to 1 m s−1, strong enough to be detected by satellite Doppler scatterometer. Numerical models and observations suggest surface waves can also contribute to the mixing and dilution of the plume waters (Gerbi et al., 2013; Thomson et al., 2014). High-resolution satellite measurements of the velocity variations in these plumes together with the local wind and wave forcing would enable tests of new models for the evolution of the river plumes (Chen and Chen, 2017; Hetland, 2017) and the effects of the plumes on pollutant transport (Kuitenbrouwer et al., 2018). Fresh coastal currents in fjords and inland seas, such as Puget Sound or the inland sea in Chilean Patagonia, also transport harmful algal blooms that affect aquaculture operations. Better understanding and prediction of the behavior of river plumes and coastal currents from simultaneous satellite measurements of winds, surface currents, and surface waves would benefit management of shellfish aquaculture, oil spills, and harmful algal blooms.
3.2.2. Orographic Wind Intensification and Small-Scale Coastal Flows
Orographic wind intensification near coastal capes, reduced wind stress in the lee of capes, and wind jets through mountain gaps all generate ocean currents in response to the spatially varying wind field (Pringle and Dever, 2009; Perlin et al., 2011; Ràfols et al., 2017). Both along-shore variations in along-shore winds and smaller scale cross-shelf variations in winds affect the shelf flows and spatial structure of upwelling over the shelf. The wind and current features can have scales <25 km (Winant et al., 1988; Perlin et al., 2011; Rahn et al., 2013; Fewings et al., 2015). The long-term variability of these flows is not well-characterized by existing measurements, and knowledge of the spatial variability is limited to locations with aircraft studies and HF radar (Kim et al., 2011; Ràfols et al., 2017). In the lee of capes in eastern boundary upwelling systems, “upwelling shadows” create regions of low wind speeds and warm sea-surface temperature, accompanied by near coastal flows opposite to the direction of the prevailing regional wind (Graham and Largier, 1997; Roughan, 2005a,b; Piñones et al., 2007; Ryan et al., 2008, 2014; Woodson et al., 2009; Walter et al., 2016, 2018). When the regional wind weakens or “relaxes” periodically (Fewings et al., 2016; Fewings, 2017), the local diurnal wind patterns and ocean stratification change (Aristizábal et al., 2017). Therefore, the regional wind relaxations not only cause regional sea-surface temperature variability offshore (Flynn et al., 2017) but also lead to changes in the coastal cross-shore flows on diurnal and semidiurnal time scales, affecting the internal temperature variability (Aristizábal et al., 2016), which is associated with nutrient supply to kelp forests in marine protected areas (McPhee-Shaw et al., 2007; Fram et al., 2008). More comprehensive measurements of the wind and current variability associated with coastal capes would enable better understanding and process-based modeling of upwelling of nutrients and retention of larvae and phytoplankton, including harmful algal blooms, in the lee of capes, and nutrient supply to marine protected areas, all processes that affect fisheries productivity.
Surface wave variability in coastal areas on scales of 25 km and smaller can be created locally by spatial variations in winds, currents, or bottom topography. High winds in hydraulic expansion fans near capes and coastline bends (Winant et al., 1988; Rogerson, 1999; Edwards et al., 2002; Monteiro et al., 2016) are the source of much of the wave energy in those regions (Villas Bôas et al., 2017). When these high winds weaken during wind relaxation events, coastally-trapped wind reversals can result (Nuss et al., 2000). The trapped reversal events are difficult to capture in existing numerical weather prediction (NWP) models due to the small cross-coast scale of the wind reversals (~10–20 km), but the reversals are associated with thickening of the marine boundary layer and tend to cause fog formation (Dorman et al., 2017) and reduced wave heights in regions that frequently experience large wind waves (Villas Bôas et al., 2017). Better observations of these topographically controlled wind intensification, relaxation, and reversal events and the associated wave heights from high-resolution satellite data would enable improvements in modeling and forecasting marine navigational hazards. Numerical models of surface waves show substantial along-coast variability in wave heights near shore due to refraction over canyons and other bathymetric features on continental shelves (García-Medina et al., 2013). Temporal and spatial variability in wave heights also occurs due to coastal boundary jets formed when mountains block passing fronts (Ellenson and Özkan-Haller, 2018). Fully coupled models for wave and current prediction are underway to aid safety and planning for marine shipping and navigation, especially near river mouths (Akan et al., 2017); simultaneous satellite measurements of winds, waves, and surface currents would enable testing and improving these models.
3.2.3. Island Wakes and Flows Around Submarine Banks
Island wakes in the ocean are important sources of upwelling of nutrients to support biological productivity. The oceanic wakes are driven by both wind variability due to the small-scale wind divergence and curl generated by the island (Caldeira et al., 2005) and by topographic effects in the ocean (Xie et al., 2001). The currents in these wakes have spatial scales of km to 10s of km and velocities of 0.2–1.5 m s−1 (Teinturier et al., 2010). Though HF radar surface current measurements have been made around some islands, such as Hawaii and Puerto Rico (https://hfradar.ioos.us/), for many geographically isolated islands the shoreline geometry does not permit overlapping coverage from two or more radars, which is needed to derive both components of the horizontal current. In addition, little information is available about the wind field within ~25 km of many islands due to the small-scale variations in the wind, the difficulty of maintaining in-situ buoy measurements, and the land mask of existing satellite measurements. Simultaneous, high-resolution measurements of winds and currents from satellites would enable better understanding and modeling of the dynamics that control these upwelling island wakes, including their dependence on ocean stratification, and whether the fisheries productivity near these islands is vulnerable to future changes in ocean stratification. Such understanding could be particularly variable in the assessment of dynamical processes that supply nutrients in the Southern Ocean, where areas near islands such as South Georgia and Kergulean are the main regions of carbon drawdown (Schlitzer, 2002).
Submarine banks are often locations of valuable fisheries, such as at Georges Bank off the northeastern U.S. (Miller et al., 1998). The partial barrier to flow on and off a bank created by the tidally-rectified flow and tidal mixing front around the bank (Houghton and Ho, 2001) provides an important retention mechanism for the plankton that support high fish production (Lough and Manning, 2001; Wishner et al., 2006). The spatial scales of the currents associated with the front are ~10–25 km (Loder and Wright, 1985; Loder et al., 1992). However, the water velocity variability on subseasonal time scales, and the interannual variability, are not well-known. Numerical modeling suggests wind forcing is also important for providing a mechanism for nutrients to be supplied to the bank across the tidal mixing front (Chen, 2003). On longer time scales, off the northwest U.S., pressure gradients associated with Heceta Bank off Oregon strongly influence the along-shore currents and local upwelling and retention patterns (Kirincich and Barth, 2009). Simultaneous measurements of winds and currents over submarine banks would enable better understanding of the physical forcing of these economically valuable ecosystems by enabling tests of dynamical models of such flows (Brink and Cherian, 2013; Dong et al., 2015) and supporting fisheries management.
3.2.4. Diurnal Variability of Surface Winds
The Earth's 24-h rotation period drives diurnal variability in atmospheric and upper ocean temperatures, winds, air-sea fluxes, and upper ocean mixing (e.g., Gille et al., 2003, 2005; Dai and Trenberth, 2004; Gentemann et al., 2009). Diurnal wind variability is most prominent along coastlines, where the land-sea breeze circulation is driven by differential daytime warming of the land and ocean (Simpson, 1994), but the signatures of diurnal winds are detectable throughout the tropics (Dai and Deser, 1999). The diurnal cycle in the upper ocean is mainly forced by solar heating, yet diurnal and higher-frequency winds play an important role in regulating vertical mixing (e.g., Giglio et al., 2017), and air-sea fluxes of heat and gases. High-frequency wind variability also impacts cross-shore exchanges (e.g., Hendrickson and MacMahan, 2009) and larvae transport (e.g., Fujimura et al., 2014).
If only one component of the Earth system experienced diurnal variability (surface air-temperature, for example), then the diurnal oscillation might be expected to cancel itself out, so that only the daily-average value would ultimately influence long-term processes. In reality, since multiple variables undergo diurnal cycles, they interact non-linearly and thus can produce a net rectified effect, working together to determine upper-ocean mixing, planetary boundary layer structure, sea surface temperature and surface air temperature (e.g., Lee and Liu, 2005).
New high-resolution wind observations, coordinated with currents, waves, and other variables, have the potential to provide the information needed to evaluate diurnal interactions of winds, temperature, and other processes. Most Earth-observing satellites have been launched on sun synchronous orbits, with measurements at two fixed times each day (e.g., 6 am and 6 pm local time). Sun-synchronous measurements are effectively at the Nyquist frequency of the diurnal cycle, providing insufficient information to resolve the details of the diurnal cycle. A greater understanding of coupled diurnal processes could be gained through a multi-satellite approach or by using a carefully selected non-sun-synchronous orbit.
3.2.5. Processes in Marginal Ice Zones and Polar Regions
In ice-covered regions the interface between atmosphere and ocean differs from its open ocean counterpart in many ways. The surface topography appears as frozen on time and length scales spanned normally by the surface wave spectra. Furthermore, the ice cover acts as an additional insulating layer both thermodynamically, due to the low conductivity of ice and snow, and mechanically, due to the rigidity of ice floes. The seasonal evolution of the sea ice drives a buoyancy forcing at the ocean surface via modulations of energy (wind forcing and heat fluxes) and salinity (brine rejection and freezing). In addition, the complex surface topography and two-phase nature of the sea ice, with alternating open ocean (leads in the pack ice or of open ocean in the MIZ) and ice features (floes), modifies turbulent fluxes of momentum, heat, freshwater, humidity, gas, and other tracers.
Sea ice in the Arctic is predicted to transition from a multi-year consolidated to a first-year seasonal fragmented ice (Aksenov et al., 2017) akin to the MIZ defined by low concentration conditions in the 15–80% range (Strong and Rigor, 2013) that are currently observed on a narrow band on the Arctic sea ice edges and more commonly throughout most of the Antarctic. This rapid transition is accompanied by a general decline in sea ice extent, concentration, thickness, age, and roughness of the ice cover (Stroeve and Notz, 2018) as well as a mechanical weakening and acceleration of the surface ice drift (Rampal et al., 2011). The increase in open water and related changes will offer new challenges and opportunities for observing and interpreting winds, waves, and currents, and their interactions. We summarize these issues below within the context of this paper.
In the MIZ, Heorton et al. (2014) found that the sharp change in surface roughness from the open ocean to the pack ice results in the formation of jets parallel to the sea ice edge over a band of ~100 km in the atmosphere and ~10 km in the ocean that modify accordingly the sea ice motion. Also in the MIZ, (Horvat et al., 2016) described in a model the interaction between floe size distribution, ocean eddies and sea ice at the origin of ocean-mixed-layer instabilities and energetic eddies at the sea ice edge that have been observed in SAR imagery (Ardhuin et al., 2017b). The MIZ can also be defined as the region over which the effects of the waves from the open ocean persist over the ice pack (Dumont et al., 2011) and to date the wave ice interactions are a key missing ingredient of sea ice-ocean coupled models (Squire, 2018). The mechanisms by which waves are dissipated in this transition region have been recently reviewed in (Boutin et al., 2018) and were shown to contribute significantly to the turbulent momentum fluxes between atmosphere and ocean (Stopa et al., 2018).
The state of the sea ice is controlled by an interplay of dynamics and thermodynamics on all spatiotemporal scales represented by a myriad of processes, such as ice growth and melt, mechanical strength of the ice, ridging, sea ice wave interactions, fast ice or leads formation (Notz and Marotzke, 2012). An observational gap remains at short time and length scales to resolve those faster processes. Marcq and Weiss (2012) found that while leads constitute <5% of the surface of the ice-covered sea they contribute to almost half of the turbulent losses. Frazil ice formation in leads and polynyas in winter (Heorton et al., 2014) and lateral melt and fragmentation of ice floes in summer (Tsamados et al., 2015), are modulated by high-frequency winds, waves and sea ice motion. Tides modify the fracture patterns over sea ice (Hutchings et al., 2005) and via a complex interplay with the sea ice and sea-floor bathymetry (i.e., at continental shelves slopes) can significantly enhance vertical turbulent fluxes (Rippeth et al., 2015). Sea ice has also been shown to act as an important controlling factor for ocean-ice shelves and marine-terminating glaciers interactions (Carr et al., 2014) via its mechanical buttressing effect but also in modulating the exchanges of heat and the degree of upwelling and impacting the amount of warm waters that can reach the continental shelves and melt ice shelves from below (Cowton et al., 2018).
The interaction between winds, ice drift, and surface ocean currents is also important in the pack ice. Over synoptic and slower time scales, the wind and ocean forcings, together with the internal forces in the sea ice (Steele et al., 1997; Feltham, 2008) control the sea ice motion and ultimately the total and regional ice volume contained in the polar oceans via redistribution of ice and export mostly out of the Fram Strait in the Arctic (Hibler et al., 2006; Ricker et al., 2018) or via Ekman transport to the warmer Southern latitudes in the Antarctic (Holland and Kwok, 2012). With the advent of polar oceanography from altimeters in ice-covered regions (Peacock and Laxon, 2004; Kwok and Morison, 2011), important new questions can now be addressed regarding the freshwater fluxes (Armitage et al., 2016), surface currents and Eddy kinetic energy (Armitage et al., 2017), as well as the spinning up or down of polar gyres (Giles et al., 2012; Dotto et al., 2018). To improve further upon the resolution probed by conventional altimetry requires the joint measurements of surface winds, ice drift and ocean currents at sub-synoptic and Eddy resolving length scales that SKIM, WaCM, and SEASTAR can achieve.
3.3. Wave-Current-Wind Interactions
3.3.1. Langmuir Turbulence
Langmuir turbulence, a physical process resulting from the interactions between the ocean surface waves and the wind-driven upper ocean sheared currents, transferring energy from the wave field to turbulence by the straining of vortices caused by the Stokes drift (Teixeira and Belcher, 2002; Ardhuin and Jenkins, 2006; Suzuki and Fox-Kemper, 2016). Langmuir turbulence is one of the most prominent wave-dependent processes that requires parameterizations in a global climate model (Belcher et al., 2012; Cavaleri et al., 2012; D'Asaro, 2014). Enhanced vertical turbulent mixing in the wavy ocean surface boundary layer (OSBL) as compared to a wall boundary layer are commonly seen in both the observations (e.g., D'Asaro, 2001, 2014; Kukulka et al., 2009) and Large Eddy Simulations (LES, e.g., McWilliams et al., 1997; Harcourt and D'Asaro, 2008; Grant and Belcher, 2009). Some of the ideas to parameterize the effects of Langmuir turbulence on vertical mixing include enhanced vertical eddy diffusivity and viscosity in the OSBL (McWilliams and Sullivan, 2000), enhanced entrainment at the base of the OSBL (e.g., McWilliams et al., 2014) and a down-Stokes drift-gradient momentum flux (Harcourt, 2013, 2015).
Parameterizing some of the effects of Langmuir turbulence in a global climate model has shown promising results, improving the simulated mixed layer depth and subsurface temperature in the extratropical regions, especially in the Southern Ocean (e.g., Li et al., 2016; Li and Fox-Kemper, 2017), although not all Langmuir turbulence parameterizations lead to climate model improvements (Fan and Griffies, 2014). Yet challenges remain in Langmuir turbulence parameterizations for global climate models. For example, due to the limited direct observations, the developments of Langmuir turbulence parameterizations have heavily relied upon LESs, which usually represent a quasi-equilibrium state and only focus on limited regimes in parameter space. The effects of Langmuir turbulence under transitioning conditions over a wide range of scenarios remain unexplored. In addition, the extent of the agreement between proposed Langmuir turbulence parameterizations remains unclear. Global-scale high-resolution measurements of ocean currents, waves, and winds will be invaluable for constraining the parameter space to be explored and for validating the parameterization schemes of Langmuir turbulence. Another challenge is the high computational cost of running a full wave model along with a climate model in order to provide the necessary wave information for Langmuir turbulence parameterizations. A wave climatology dataset has been shown to be useful for parameterizing the Langmuir turbulence-enhanced vertical mixing (Li and Fox-Kemper, 2017). Datasets from global-scale high-resolution wave measurements will be highly valuable for this purpose and potentially helpful for parameterizing other effects of Langmuir turbulence without a full-wave model.
Additionally, there are other known impacts of waves on upper-ocean turbulence and macroturbulence, such as wave breaking and bubble injection (Liang et al., 2013; Deike et al., 2016), and wave-driven submesoscale frontogenesis (McWilliams and Fox-Kemper, 2013; Suzuki et al., 2016). There are alternative theories and experiments of spontaneous turbulence driven by non-breaking waves and in the absence of prior turbulence (Babanin, 2006) that differ conceptually from Langmuir turbulence, but it is unclear to what extent these theories represent distinct phenomena as opposed to being alternative explanations of the same effects, because some theoretical framings include both aspects. Detailed laboratory experiments and high-resolution measurements of co-located currents, waves, and winds would be an ideal resource for evaluating these new and alternative theories and their impacts on global-scale questions, such as air-sea exchange and interactions and their impact on climate change.
3.3.2. Ocean Fronts
Observations, models, and theory indicate that in all regions and seasons, the ocean surface is filled with permanent, recurring, and transient fronts: strong horizontal gradients in buoyancy on an O(100 m–10 km) scale with magnitudes of 10−5–10−8 s−2 (Small et al., 2008; McWilliams, 2017). Frontal regions have strong and atypical air-sea interactions (e.g., D'Asaro, 2001). Coupled models (Small et al., 2008) and observations (Frenger et al., 2013; Villas Bôas et al., 2015) indicate that SST contrasts at the front can localize responses in the atmospheric boundary layer above, which affects winds, clouds, uplift, turbulence, precipitation, turbulent heat fluxes, and wind shear profiles. This coupling is qualitatively different from coupling that occurs at larger scales, as oceanic variability tends to drive atmospheric variability, rather than vice versa. In addition, oceanic fronts have a different response to forcing than regions without fronts. In non-frontal regions, winds and cooling tend to deepen the boundary layer, while warming tends to shoal it. In frontal regions, observations and theory indicate that winds and cooling interact with the fronts–in particular, downfront winds tend to enhance fronts and trigger frontal instabilities (symmetric and baroclinic) and turbulence, while upfront winds tend to shoal the boundary layer (Thomas and Lee, 2005; D'Asaro et al., 2011; Thomas et al., 2013, 2016). Fronts refract and scatter waves and can lead to large gradients in surface roughness and wave forcing (Ardhuin et al., 2017a; Romero et al., 2017).
The origin of fronts is sometimes from localized atmospheric mixing (e.g., Price, 1981; D'Asaro et al., 2007; Mrvaljevic et al., 2013), sometimes from topographic features (e.g., Srinivasan et al., 2017) and river mouths (Luo et al., 2016), and sometimes through the straining by mesoscale features (Shakespeare and Taylor, 2013). Fronts and filaments can be enhanced by wave-induced vertical velocities by a mechanism similar to that driving Langmuir turbulence (McWilliams and Fox-Kemper, 2013; Suzuki et al., 2016) and through boundary layer mixing (Gula et al., 2014; McWilliams, 2017). The arrest and frontolysis that controls the width, strength, and lifetime of these features is an area of active research (Sullivan and McWilliams, 2018; Tozuka et al., 2018) and plays an important role in parameterizations that depend on frontal width (e.g., Fox-Kemper et al., 2011). The instabilities that form at fronts extend into the features that populate the macroturbulence of the submesoscale (Haine and Marshall, 1998; Haney et al., 2015) and form the basis of most submesoscale parameterizations (e.g., Fox-Kemper et al., 2011; Bachman et al., 2017). Significant surface convergence frequently occurs along the nose of the front, which is important for transport of buoyant debris and oil (D'Asaro et al., 2018) as well as for the strengthening of the front (Suzuki et al., 2016; McWilliams, 2017). Frontal strength–and related submesoscale variability–have strong seasonality because of the connections between fronts and air-sea interaction (Mensa et al., 2013; Qiu et al., 2014; Brannigan et al., 2015; Callies et al., 2015) and seasonality of boundary forcing, such as rivers (Luo et al., 2016).
What is presently not well-constrained observationally are the typical interactions at fronts between the fronts, winds, waves, and small-scale features. While there are many studies using in situ instruments to study these interactions (e.g., D'Asaro et al., 2007, 2011; Mrvaljevic et al., 2013; Thomas et al., 2013, 2016; Callies et al., 2015), and a few point source seasonal studies (e.g., Thompson et al., 2016), a global-scale survey of simultaneous fronts, winds, and waves does not exist. Without such a survey, many of the inferences from models and theory remain largely speculative. Note that the interactions between mesoscale strain, fronts, and turbulence induced by winds spans roughly five orders of magnitude in horizontal scales from 100 km to 1 m (Figure 2), which is orders of magnitude larger than the largest simulations presently possible. A global simulation resolving these processes remains over a century away (Fox-Kemper et al., 2014).
3.3.3. Surface Wave Response to Currents and Winds
Ocean waves respond differently to winds and currents, as illustrated in Figure 6. Away from coasts and sea ice, and at scales larger than 100 km, fields of wave heights are similar to low-pass filtered winds with a wavenumber spectrum of Hs that is steeper than the wind kinetic energy spectrum. At smaller scales, the variability of Hs is expected to be mostly due to refraction over current gradients (Lavrenov, 2013; Ardhuin et al., 2017a), and the wavenumber spectrum of Hs generally follows the shape of the current kinetic energy spectrum. Hence, sharp current fronts result in sharp wave heights and might enhance wave breaking (Phillips, 1984; Romero et al., 2017). When the average wave height is around 4 m, standard products from altimetry typically give three regimes as illustrated in Figure 6C: slopes on the order of k−1 for wavelengths longer than 100 km, probably associated with scales in the wind field; a k−3 slope for scales between 50 and 100 km, which follows the shape and level of the current kinetic energy spectrum; and a much flatter region at scales below a threshold on the order of 50 km, which we interpret as nearly white tracker noise. The effective resolution is even coarser for lower sea states, so that the nominal resolution of 25 km is generally not achieved, even in the along-track dimension of satellite data. This along-track resolution can be strongly improved with re-tracking (Ardhuin et al., 2017a) or filtering (Quilfen et al., 2018), and Delay-Doppler altimetry can produce less noisy estimates of Hs.
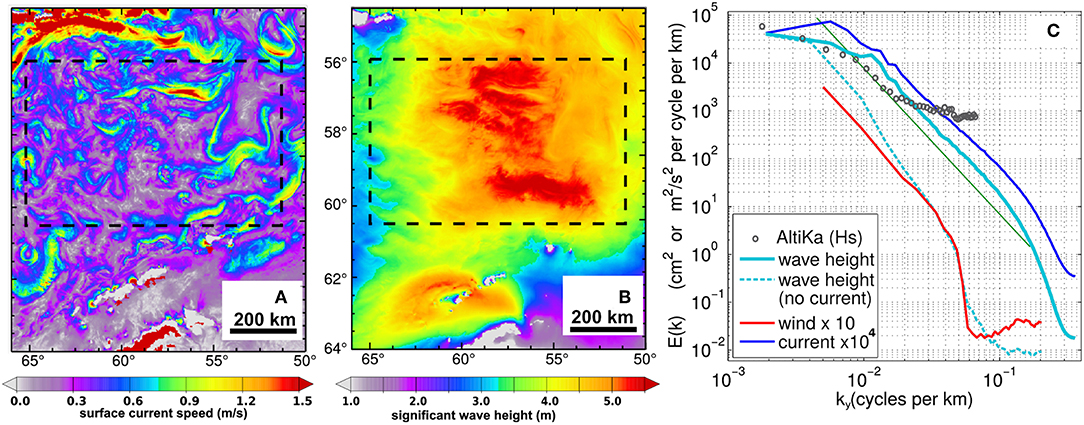
Figure 6. (A,B) Show snapshots of modeled surface currents and waves in Drake Passage, respectively. (C) Shows corresponding spectra of modeled winds (red), currents (dark blue), and significant wave heights (light blue), as well as the spectrum of significant wave height observed from the AltiKa altimeter (circles). This figure is adapted from Ardhuin et al. (2017a). The green solid thin line has a k−3 slope.
Even perfect satellite measurements of the wave field would not, at least in the near future, provide the 3-h revisit time required to resolve the temporal variability associated with storms and tidal cycles, which is now only available at discrete point locations with moored buoys. Hence, any progress toward faster revisit times, possibly by measuring across a wide swath and not just along a track, could take us closer to resolving the variability of sea states. Given accurate forcing fields, including surface vector winds, surface currents, and sea ice properties, sea states can be predicted fairly accurately once the wave generation and dissipation processes are well-documented and parameterized (e.g., Ardhuin et al., 2010). Observing the spatial patterns of wave heights and other sea state parameters is key to arriving at this understanding and improving the parameterization of source terms in wave models.
Indeed, significant wave height is only one parameter, and a full description of the sea state requires a two-dimensional (2D) spectrum for which few measurements are available. The 2D wave spectrum can be integrated to yield moments, such as the mean square slope and surface Stokes drift that are expected to impact wind stress and surface drift velocities, and different mean periods and directions that are needed to know the wave-induced energy flux, forces on structures or wave-induced coastal sea level variations.
3.3.4. Sea State Dependent Air-Sea Fluxes
Ocean waves define the random moving multi-scale interface between the ocean and the atmosphere, key subsystems governing the dynamics of climate. A precise description of the physical processes, forcings, interactions, and feedbacks occurring at this interface is essential for determining air-sea fluxes of momentum, sensible and latent heat, CO2 and other trace gases, in addition to aerosols, which all together govern the coupling between the two subsystems. While there is an agreement among the oceanographic community that wave motions and dissipative breaking processes are intimately involved in all these fluxes (Cavaleri et al., 2012), surface wave physics has yet to be consistently represented in most air-sea interaction parameterizations.
Beyond atmospheric stability, measurements systematically indicate that surface wind stress can be significantly impacted by the sea state directionality, degree of development, interaction with upper ocean currents (e.g., Vandemark et al., 1997; Grachev et al., 2003; Hristov et al., 2003; Edson et al., 2013), and also by unsteady winds and the presence of swell (e.g., Hwang et al., 2011). Without considering all the aforementioned sources of inhomogeneous conditions, wind stress is already reported to be significantly enhanced with respect to a flat surface for winds up to 25 m s−1. This has been well-captured by conceptual models (e.g., Janssen, 2004, and references therein). Several studies demonstrate that incorporating wave-dependent surface flux parameterizations leads to significant effects in the atmospheric state (e.g., Cavaleri et al., 2012; Shimura et al., 2017; Pineau-Guillou et al., 2018). Studies of the impact of small-scale breaking distribution and modulation have shown wave breaking to be significant for momentum fluxes (e.g., Melville and Rapp, 1985; Kudryavtsev et al., 2014a; Kudryavtsev and Chapron, 2016). However, relating the variations of the sea surface drag coefficient to the degree of development of the sea state, significant wave steepness, phase velocity of dominant waves or wave age (e.g., Kitaigorodsky, 1970; Donelan et al., 1993) is still an open question.
In particular, measurements of surface stress are too scarce and often exhibit significant, and sometimes unexpected, scatter around the predicted equilibrium value for a given wind, which suggests that this variability could be due to a sensitivity to external parameters (Garratt, 1977; Edson et al., 2013). Under low wind conditions, the presence of swell is considered a major source of variability. For example, Soloviev and Kudryavtsev (2010) reported swell-induced wind-flow undulations, exponentially attenuated with heights up to half the peak wavelength. These results are in line with theoretical predictions (Makin, 2008; Kudryavtsev et al., 2014a). However, more accurate measurements of the complete wind-wave-current system are still needed to help understand the complex interplay between processes controlling air-sea interactions, possibly including physical-biological effects near upper-ocean fronts, such as biological surfactants and sea surface temperature influencing short-scale wave growth. Examples of recent experiments include ship observations in the frontally active Brazil-Malvinas confluence region (Hackerott et al., 2018), or airborne observations covering varying fetch and current conditions (Romero and Melville, 2010; Romero et al., 2017). These measurements are needed to proceed further in an improved description of the statistical properties of the turbulence and impacts on the profile of the atmospheric flow.
The observational challenge to be faced is first to improve the variety and the precision of the wind-wave-current state variables (e.g., better estimate atmospheric turbulence statistics, which requires an improvement in temporal sampling). Moreover, there is a need to design a coordinated array of surveys (e.g., a swarm of drones) to optimally document the variety of physical conditions in the wind-wave-current system and to explore how its heterogeneity can affect the resulting large-scale wind stress and surface flow.
3.3.5. Wind Modulation by Surface Currents
Winds drive ocean currents, but the ocean can also couple to the atmosphere through a surface current feedback (Dewar and Flierl, 1987; Pacanowski, 1987) or a thermal feedback (Chelton et al., 2004; Small et al., 2008; Chelton and Xie, 2010). The current feedback is due to momentum transfer between the ocean and atmosphere which occurs in the moving frame provided by the moving ocean, so that the surface stress, τ, is given by
where ρ is the air density, CD is the drag coefficient, Ua is the air velocity, and Uo is the ocean velocity. The signature of surface currents on stress was first shown at large scales by Kelly et al. (2001, 2005), who showed that scatterometer neutral winds, which are proportional to τ, were modulated by equatorial currents at the TAO buoy array, and by Cornillon and Park (2001), who showed the same effect over mesoscale eddies. It was soon realized (Hughes and Wilson, 2008; Scott and Xu, 2009) that the net effect of the stress modulation resulted in energy flowing from the ocean mesoscale circulation into the atmosphere, damping the eddy kinetic energy (EKE). A detailed study of the interplay between surface currents and wind induced vertical pumping for mesoscale eddies was conducted by Chelton et al. (2011) and by Gaube et al. (2015), who observed patterns of upwelling for cyclonic and anticyclonic eddies, and estimated the eddy decay resulting from Ekman pumping and associated energy release into the atmosphere. Subsequently, in a study using coupled models in the California Current System (CCS), (Renault et al., 2016c) showed that the wind-stress curl could be approximately related to the surface current relative vorticity via a linear relationship
where ζ is the current relative vorticity, and sw(Ua) is a (negative) coupling coefficient that depends on the wind speed. This relationship was also characterized for large mesoscales using coupled models for the Gulf Stream region (Renault et al., 2016b), the Agulhas Retroreflection region (Renault et al., 2017b), and globally, using satellite data (Renault et al., 2017a). This coupling is expected to depend on the scales of averaging, and a first look at the changes was obtained using a coupled model study for submesoscales in the CCS (Renault et al., 2018). The validity and scale dependence of this relationship is and important and open question. Recent airborne results using a Doppler scatterometer (Rodriguez and Wineteer, 2018; Rodriguez et al., 2018) document the validity of the relationship at very high (km-scale) resolution, as shown in Figure 7. Understanding the validity of this relationship globally at high resolution is an important goal for future winds and currents ocean observations. Among important applications of improved understanding of this coupling is the impact of wind and current interactions for ocean productivity (Gaube et al., 2014; Renault et al., 2016a).
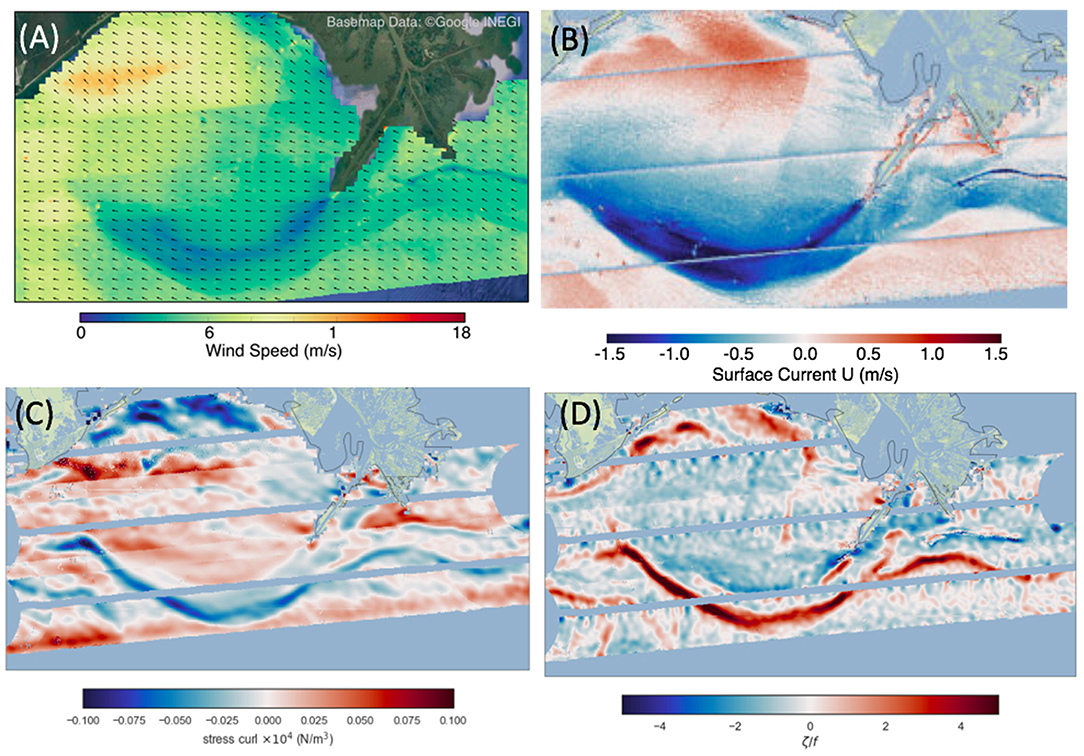
Figure 7. (A) Neutral wind speed (color) and direction (arrows) from NASA DopplerScatt data over the Mississippi River plume and Barataria Bay, USA; (B) eastward surface current component for the same region; (C) wind stress curl computed from neutral winds; (D) surface current relative vorticity divided by the Coriolis parameter f computed from DopplerScatt surface currents. Note the negative correlation between (C,D), as expected from Equation (2).
The other source of coupling between currents and winds is due to the influence of heat carried by surface currents to alter the marine boundary layer, leading to increases (decreases) of wind speed as winds travel from cold to hot (hot to cold) ocean regions. A linear relationship between sea-surface temperature (SST) gradients and wind speed, wind stress, and wind-stress curl has been documented by multiple studies (O'Neill et al., 2003, 2005, 2010, 2012; Chelton et al., 2004, 2007; Liu et al., 2007; Small et al., 2008; Chelton and Xie, 2010; O'Neill, 2012). Coupling also has an impact of Ekman upwelling for ocean eddies, although the effect is much smaller than the coupling caused by current modulation (Gaube et al., 2015). However, this coupling has been shown to have an impact on winds, clouds, rain, and turbulent heat fluxes for the lower atmosphere (Frenger et al., 2013; Villas Bôas et al., 2015), with the potential to reach higher in the atmosphere for western boundary currents (Minobe et al., 2008, 2010). Since the magnitude of the coupling depends on the SST gradient, it is expected that the coupling will appear more pronounced when observed at higher resolutions for sharper SST fronts than have been observed to date, and this is also an important issue to be settled by future ocean observations.
3.4. Modeling and Data Assimilation
Fundamentally, data assimilation seeks to extract and combine the maximum amount of information contained in observations and numerical models to obtain a more complete and synthetic view of the system considered. Stammer et al. (2016) note that the generic term “data assimilation” encompasses two distinct approaches. Numerical weather prediction (NWP), or ocean prediction, is focused on near-real-time (NRT) prediction and it is done operationally, by sequentially updating the model state to make the best possible forecasts. Error in the model physics and dynamics and sparse data are major obstacles to skillful analyses and forecasts. The second approach is state (and parameter) estimation, which is usually focused on hindcasts, also called reanalysis, which test models by requiring them to match the time evolution seen in the observations. The purpose of state estimation is to reconstruct the past, evaluate prediction skill as well as identify model errors and reduce them.
Model errors are often largest in boundary regions where important physics at small scales are unresolved and must be parameterized. The most important boundary layer is the interface between the ocean and atmosphere. Reducing model error in this boundary layer requires representing the coupling physics and dynamics as accurately as possible, but these physics must be learned from theory, modeling, and observations together. Overlapping observations of wind, current, and surface waves will enable process studies to develop and refine our understanding of the surface layers, using models in the data analysis to enforce known physics. Identifying model errors is a challenging problem and requires comprehensive observations that can both supply enough information to specify the model state and check its evolution over time. The first step is to adjust the physics to maximize the consistency of the model with the observations over a time range that is comparable or longer than the timescale of the dynamics under investigation, but models can fit the observations for the wrong reasons if the dataset is insufficient to determine all the model parameters within errors that are small enough. A second step of cross-validation against observations not used in the assimilation (called “independent” or “withheld”) may guard against this (Cornuelle et al., 2000; Verdy and Mazloff, 2017), although there is some debate on the functionality of this method for heavily under-sampled systems.
Many of the open questions for the physics of the boundary layer come from the turbulent flows above and below the air-sea interface, where waves are a key component. Knowledge of the sea state should improve model estimates of momentum, heat, mass, and gas fluxes (Cavaleri et al., 2012; Shimura et al., 2017). Moreover, propagation of observation information between the atmosphere and ocean model components will be improved by the inclusion of a wave model component. Weather forecasts beyond a week or two are increasingly thought to depend on accurate air-sea fluxes, so accurate modeling of the air-sea boundary layers should enhance sub-seasonal to seasonal predictions (Belcher et al., 2015). Including a wave model component to a coupled ocean-atmosphere model could reduce both data and model error. Several observational platforms (e.g., altimeters) cannot completely remove ocean wave signals and thus having a wave component in the assimilation system may help to unbias the observations (e.g., Peral et al., 2015). Ocean-atmosphere assimilation systems may also benefit from fully incorporating wave models due to the coupling of these components. There will be instances where observations of waves will constrain estimates of the atmosphere and ocean states, although they cannot replace direct measurements of winds and currents.
4. Discovery: Taking Doppler Oceanography to Space
Following early airborne and space-borne demonstrations of line-of-sight surface current retrieval using interferometric radars (Goldstein et al., 1989; Romeiser, 2013) and the systematic interpretation of surface velocity from the Doppler centroid of a single SAR system (Chapron et al., 2005) into wave and current contributions, it is now well-understood that all-phase related measurements measure the same velocity. This velocity is usually a weighted-mean surface velocity, where the weight is related to the local backscatter, with the possible addition of the intrinsic scatterer velocities (Romeiser et al., 2014; Nouguier et al., 2018; Rodriguez, 2018). As a result, the measured velocity combines currents and waves, and has a sensitivity to the near-surface current shear, which varies with the choice of radar wavelength and incidence angle. A possible proxy for wave-related motions can be derived from the surface wind vector (Mouche et al., 2008; Martin et al., 2016), although this is less accurate for the near-nadir incidence angles (Ardhuin et al., 2018; Nouguier et al., 2018). This understanding has been supported by recent platform-based and airborne measurements (Rodriguez, 2018; Yurovsky et al., 2018). Many efforts have been devoted to the development of satellite systems able to measure both components of the vector currents rather than a single component in the cross-track direction.
Three satellite mission concepts that could measure surface currents in the coming decade are now at various stages of development, although none of them are so far confirmed. The SKIM mission (Ardhuin et al., 2018), pre-selected along with the Far-Infrared Outgoing Radiation (FORUM) in response to the ESA Earth Explorer 9 call, has a potential launch scheduled for 2025. Detailed design studies will lead to the final selection (either SKIM or FORUM) by September 2019. The SEASTAR mission was one of 21 mission concepts proposed in 2018 to the ESA Earth Explorer 10 call for mission ideas (Gommenginger et al., 2018) and, while not selected by ESA for EE10, continues to be promoted for implementation through other avenues and opportunities within ESA, Europe and beyond. The WaCM (WaCM, Bourassa et al., 2016; Rodriguez et al., 2018), listed in the 2017 US Decadal Survey, would address one of the seven priority areas highlighted by the U.S. National Academy of Sciences, of which three are expected to be implemented as explorer missions by NASA.
These three missions concepts all have different objectives and use different designs, leading to different products, performance expectations and sampling. SEASTAR is based on the principle of SAR Along-Track Interferometry (ATI), which was already demonstrated from space on the space shuttle and with Tandem-X, but with the difference that SEASTAR features two pairs of radar beams “squinted”, respectively 45° fore and aft of the satellite, enabling measurements of the surface motion in two orthogonal directions from which, with the help of a third dual-polarized beam in the broadside direction, both current vector and wind vector can be derived. In its present inception, SEASTAR provides current vector and wind vector products at 1 km resolution over a single continuous swath of 170 km, and a random noise performance for current vectors better than 10 cm s−1 and 10° at 1 km resolution. Details about SEASTAR can be found in the OceanObs'19 mini-review by Gommenginger (2019) associated with this paper.
SKIM and WaCM are rotating pencil-beam sensors that provide a diversity of look directions and thus provide the two current vector components. For WaCM and SKIM instruments, the velocity is estimated by measuring the phase between pulse pairs, which, as in the ATI case, is proportional to the Doppler centroid (DC) of scatterers within the real-aperture radar footprint. The pulse-pair and ATI methods would essentially measure the same velocity (Romeiser, 2013; Rodriguez et al., 2018), but with differing noise levels. The variability of surface velocities in the real-aperture footprint is greater, since footprints are on the order of kilometers in the azimuth direction, while the azimuth footprint size is on the order of 100 m, due to wave motion. The greater Doppler variability in the real-aperture techniques results in greater noise (all other things being equal) relative to the ATI technique. Details about SKIM and WaCM can be found in the OceanObs'19 mini-reviews by Ardhuin (2019) and by Rodriguez (2019) associated with the present review.
Whereas SEASTAR seeks to achieve a resolution of 1 km or finer, WaCM and SKIM are planned to estimate currents at 25 km or greater scales, once data are averaged to yield an appropriate noise level. One drawback of the SEASTAR approach is the high power and data downlink requirements, which under the programmatic constraints of the ESA Earth Explorer 10 call, led to limiting data acquisitions to coastal, shelf and polar seas and a few open ocean sites of special interest, with a revisit time between 1 and 30 day at 45°N depending on orbital mission phase. In contrast, WaCM achieves near global coverage of both surface currents and winds in <1 day, while SKIM achieves global coverage of surface currents and waves in about 3 days at mid-latitudes. The largest difference between SKIM and WaCM is the incidence angles used for observations. SKIM is derived from the SWIM instrument flown on the China-France Ocean SATellite (CFOSAT, Hauser et al., 2017), with only a small plate rotating, carrying horns near the focal point of a fixed reflector. The SKIM technology allows incidence angles up to 12°, yielding a 6 km footprint for individual measurements over a 330 km wide swath for an orbit altitude of 850 km. Because of antenna and spin parameters, the SKIM coverage has gaps which must be filled using optimal interpolation. The interpolated data allow global coverage with a revisit time of 3 days at 45°N, at the expense of an additional mapping error. The SKIM incidence angles allow the observation of not only Doppler, but also backscatter tilt modulation, from which surface wave spectra can be estimated, as in SWIM. WaCM uses a fan-beam antenna with an incidence angle of 56°, which achieves a 2–3 km azimuth resolution and better range resolution, allowing continuous gap-less coverage over a wide 1,700–1,800 km swath (depending on the orbit) resulting measurements twice a day at 45°N. The WaCM backscatter noise level is sufficient for wind and current retrievals at resolutions better than 5 km, but the currents must be further averaged to about 25 km to achieve noise levels appropriate for surface current mapping. The engineering design for WaCM has not been finalized, and the current performance of WaCM may vary from about 6 cm s−1 (30 cm s−1), for the low-power option, to 1.6 cm s−1 (8 cm s−1), for the high-power option, at spatial sampling of 25 km (5 km). These sampling characteristics are important for defining the effective space-time resolution and the spatial scales that will be aliased in time.
Overall, the three proposed Doppler oceanography missions share common scientific interests but also show good levels of complementarities in terms of products, capabilities and sampling. Detailed descriptions of the performance and sampling advantages of the SEASTAR, SKIM and WaCM concepts can be found in the respective OceanObs mini-reviews by Ardhuin (2019), Gommenginger (2019), and Rodriguez (2019). It is worth remembering that, despite the high relevance and broad general interests in these issues, the exciting opportunities afforded by recent technological advances, and the high level of apparent effort expended on each concept, none of these concepts is presently approved to proceed with implementation.
5. Summary and Recommendations
Ocean surface winds, currents, and waves are essential climate variables playing a crucial role in exchanges of momentum, energy, heat, freshwater, gases, and other tracers between the ocean, atmosphere, and ice. This paper reviewed the present state of observations of these variables and outlined observational gaps that limit our current understanding of coupled processes that happen at the air-sea-ice interface as summarized below.
The mapping capability of the present constellation of satellite altimeters is limited to resolving wavelengths larger than 100 km. Even though higher resolution (10s of kilometers) might be achieved with SWOT, the applicability of altimeters is restricted to geostrophic flows. Total ocean surface current measurements (geostrophic and ageostrophic) at mesoscales (30–300 km) are needed to constrain heat and freshwater budgets in equatorial regions as well as the pathways of floating material and cross-shelf transport of tracers. An accuracy of 10 cm/s at 30 km spatial grid every 10 days would allow a considerable reduction in air-sea flux residuals and surface transport pathways. In addition, the momentum transfer between the atmosphere and the ocean via surface stress depends on the difference between the total surface current and surface wind vector; thus joint measurements of these variables are essential for assessing the impacts of currents in modulating the wind stress and for quantifying the energy input from the wind.
The dynamics governing surface currents and air-sea interactions dramatically change at scales smaller than 10 km (submesoscale). Apart from HFR stations, which are only available in some areas and restricted to regions inshore of 300 km, there is currently no means of systematically monitoring ocean currents at these scales. High-resolution measurements of surface currents (1–10 km) are necessary to understand processes linked to frontogenesis, cross-shelf flows associated with upwelling/downwelling, and river plume outflows. Additionally, the variability of surface waves at these scales is largely explained by wave-current interactions and dominated by the variability of the surface current field. Joint high-resolution wind, current, and wave observations are needed in order to assess the impact of wave-current interactions on extreme sea states and marine and coastal hazards.
In coastal regions, high-resolution (scales under 25 km) and high-frequency (more frequent than 4 times per day) measurements of winds, currents, and waves are necessary to resolve the details of the land-sea breeze, which impacts the sea surface temperature, stratification, and upper-ocean mixing. Furthermore, at these scales, winds associated with orographic features modulate the surface current and surface wave fields, with implications for upwelling of nutrients, transport of larvae, recreation, and navigation. A main conclusion of the inaugural Mooers Coastal Ocean and Atmosphere Prediction Workshop was that simultaneous, global satellite-based measurements of winds and currents have great potential to improve forecasting for the coastal ocean (Samelson, 2019).
Surface gravity waves are a primary source of turbulence in the upper ocean. Yet, ocean models represent unresolved processes that control vertical mixing through parameterization schemes that often do not explicitly take into account the effects of surface waves. Global observations of the 2D wave spectrum are key for constraining the parameter space in schemes of Langmuir turbulence. Another fundamental problem in the ocean-atmosphere boundary layer is the modulation of the surface wind stress by surface waves. Sea state-dependent parameterizations of air-sea fluxes lead to significant differences in the atmospheric state. Measurements of directional wave spectra along with surface currents and winds are essential information to improve empirical relationships for the drag coefficient and improve bulk formulae. It is also worth noting that wave-induced Stokes drift velocities generally exceed Ekman currents at the sea surface and are important for constraining Lagrangian pathways, impacting the transport of tracers, plastic, oil, and debris.
From a modeling perspective, a priority of the coming decade must be to better integrate ocean, wave, and atmospheric models to enable accurate observational constraint propagation between components in a forecasting or reanalysis system. Components of this system will still need to be parameterized, but these parameterizations can be improved by including estimates of the sea state.
In marginal ice zones (MIZs), measurements of sea-ice drift, surface currents, and surface waves are needed to address questions regarding freshwater fluxes and interactions between eddies and floes. Further, the directional spectrum of surface waves in polar regions is necessary to address wave-ice interactions, more specifically, wave dissipation by sea-ice.
The most fundamental idea that summarizes this review lies in the concept that surface winds, currents, and waves are coupled variables and hence require integrated observations and modeling. Future Doppler oceanography satellite concepts discussed here (i.e., SKIM, WaCM, and SEASTAR) have the potential to help fill in some of the identified observational gaps and to deliver systematic and global joint observations of surface winds, currents, and waves. The first step toward this direction was taken with the recent launch of CFOSAT, which will provide simultaneous measurements of surface winds and waves in the upcoming months. We believe that much can be learned from additional air-sea flux observational campaigns carried out in different sea state conditions in support of upcoming satellite missions. Understanding the physics of processes that mediate air-sea exchanges will lay the groundwork for incorporating their effects into model parameterizations, fostering the development of coupled wave-ocean-atmosphere-ice models. Integrated observations of these variables will facilitate the validation of such models. In a climate change scenario, better knowledge of the air-sea interactions and upper-ocean dynamics will be important for adaptation and mitigation in response to extreme events and environmental disasters.
Author Contributions
AV led the conceptualization, writing, and editing of the paper. FA, AA, MB, PB, BC, BDC, JF, MF, BF-K, SG, CG, PH, MH, QL, MM, SM, AM, MR, ER, JS, AS, ET, MT, CU, and EvS have contributed equally to writing sections and revising the manuscript. All authors have approved the submitted version.
Funding
AV was funded by NASA Earth and Space Science Fellowship award number 80NSSC17K0326. MB was funded by NOAA (FundRef number 100007298) through the NGI (grant number 18-NGI3-42). SG was funded by NASA grants NNX16AH67G, NNX14A078G, NNX17AH53G, and 80NSSC19K0059. MT acknowledges support from the Natural Environment Research Council (grant number NE/R000654/1). MT, MR, JS, and EvS were partially funded by the SKIM Mission Science Study (SKIM-SciSoc) project ESA RFP 3-15456/18/NL/CT/gp. AA was supported by DGA grant No D0456JE075 and the French Brittany Regional Council. MF was supported by NASA Ocean Vector Winds Science Team Grant 80NSSC18K1611 and Jet Propulsion Laboratory/CalTech subcontract 1531731. FA, BC, and AM were supported by ESA under the Sea State CCI project, with additional support from CNES and ANR grants for ISblue (ANR-17-EURE-0015) and LabexMER (ANR-10-LABX-19). MZ was funded by NASA (grant number NNX16AH67G).
Conflict of Interest Statement
The authors declare that the research was conducted in the absence of any commercial or financial relationships that could be construed as a potential conflict of interest.
Acknowledgments
The authors thank the two reviewers for their comments and suggestions. AV, AA, FA, BF-K, and QL acknowledge the Kavli Institute for Theoretical Physics for partially supporting this research through the National Science Foundation Grant No. NSF PHY17-48958. AV thank Guilherme Castelão (Scripps Institution of Oceanography) for fruitful discussion, suggestions, and edits. MF thank Jessica Benthuysen (Australian Institute of Marine Science); Ted Strub, H. Tuba Özkan-Haller, Ricardo Matano, and Roger Samelson (Oregon State University); Libe Washburn (University of California, Santa Barbara); Clive Dorman (University of California, San Diego); Jamie Pringle (University of New Hampshire); Ata Suanda (University of Otago, New Zealand); Parker MacCready (U. Washington); Helga Huntley (U. Delaware); and Steve Morey (U. Miami) for helpful comments and suggestions.
Abbreviations
2D, two-dimensional; ADCP, Acoustic Doppler current profiler; AMRS-2, Advanced Microwave Scanning Radiometers; ASCAT, Advanced Scatterometer; ATI, Along-Track Interferometry; CCMP, Cross-Calibrated Multi-Platform ocean surface wind velocity product; CCS, California Current System; CFOSAT, China-France Oceanography SATellite; CMEMS, Copernicus Marine Environment Monitoring Service; CO2, carbon dioxide; CYGNSS, NASA Cyclone Global Navigation Satellite System; DC, Doppler centroid; EKE, eddy kinetic energy; ERS-1/2, European Remote Sensing-1/2; ESA, European Space Agency; EUMETSAT, European Organization for the Exploitation of Meteorological Satellites; GDP, Global Drifter Program; GEKCO, Geostrophic and Ekman Current Observatory; GLAD, Grand Lagrangian Deployment; GNSS-R, Global Navigation Satellite System-Reflectometry; GNSS, Global Navigation Satellite System; GOCE, Gravity field and Ocean Circulation Experiment; GPM, Global Precipitation Measurement; GPS, Global Positioning System; HFR, high-frequency radars; LASER, Lagrangian Submesoscale Experiment; LES, Large Eddy Simulations; MDT, Mean Dynamic Topography; MIZ, Marginal Ice Zone; MSS, mean sea surface; NDBC, US National Data Buoy Center; NOAA, National Oceanic and Atmospheric Administration; NRT, Near Real Time; NSCAT, NASA Scatterometer; NWP, Numerical weather prediction; OSBL, ocean surface boundary layer; OSCAR, Ocean Surface Current Analysis Real-time; PIRATA, Prediction and Research Moored Array in the Tropical Atlantic; QuickSCAT, Quick Scatterometer; RAMA, Research Moored Array for African-Asian-Australian Monsoon Analysis and Prediction; RFI, Radio Frequency Interference; RMSE, Root Mean Square Error; RMS, Root Mean Square; RapidScat, International Space Station Rapid Scatterometer; SAR, Synthetic aperture radar; SEASAT, first satellite carrying a SAR; SKIM, Sea surface KInematics Multiscale monitoring satellite mission; SLA, Sea Level Anomalies; SSH, Sea Surface Height; SST, Sea Surface Temperature; SWOT, Surface Water and Ocean Topography; TAO, Tropical Atmosphere Ocean project; TRITON, Triangle Trans-Ocean Buoy Network; WaCM, Winds and Currents Mission.
References
Adams, K., Barth, J., and Shearman, R. (2016). Intraseasonal cross-shelf variability of hypoxia along the Newport, Oregon, hydrographic line. J. Phys. Oceanogr. 46, 2219–2238. doi: 10.1175/JPO-D-15-0119.1
Akan, Ç., Moghimi, S., Özkan-Haller, H. T., Osborne, J., and Kurapov, A. (2017). On the dynamics of the Mouth of the Columbia River: results from a three-dimensional fully coupled wave-current interaction model. J. Geophys. Res. Oceans 122, 5218–5236. doi: 10.1002/2016JC012307
Aksenov, Y., Popova, E. E., Yool, A., Nurser, A. G., Williams, T. D., Bertino, L., et al. (2017). On the future navigability of Arctic sea routes: high-resolution projections of the Arctic Ocean and sea ice. Mar. Policy 75, 300–317. doi: 10.1016/j.marpol.2015.12.027
Alford, M. H. (2010). Sustained, full-water-column observations of internal waves and mixing near Mendocino Escarpment. J. Phys. Oceanogr. 40, 2643–2660. doi: 10.1175/2010JPO4502.1
Alford, M. H., MacKinnon, J. A., Simmons, H. L., and Nash, J. D. (2016). Near-inertial internal gravity waves in the ocean. Annu. Rev. Mar. Sci. 8, 95–123. doi: 10.1146/annurev-marine-010814-015746
Alpers, W., Zhang, B., Mouche, A., Zeng, K., and Chan, P. W. (2016). Rain footprints on C-band synthetic aperture radar images of the ocean–revisited. Rem. Sens. Environ. 187, 169–185. doi: 10.1016/j.rse.2016.10.015
Andersen, O. B., Stenseng, L., Piccioni, G., and Knudsen, P. (2016). “The DTU15 MSS (mean sea surface) and DTU15LAT (lowest astronomical tide) reference surface,” in ESA Living Planet Symposium 2016 (Prague).
Anderson, C. R., Brzezinski, M. A., Washburn, L., and Kudela, R. (2006). Circulation and environmental conditions during a toxigenic Pseudo-nitzschia australis bloom in the Santa Barbara Channel, California. Mar. Ecol. Progr. Series 327, 119–133. doi: 10.3354/meps327119
Ardhuin, F. (2019). SKIM, a candidate satellite mission exploring global ocean currents and waves. Front. Mar. Sci. 6:209. doi: 10.3389/fmars.2019.00209
Ardhuin, F., Aksenov, Y., Benetazzo, A., Bertino, L., Brandt, P., Caubet, E., et al. (2018). Measuring currents, ice drift, and waves from space: the Sea surface KInematics Multiscale monitoring (SKIM) concept. Ocean Sci. 14, 337–354. doi: 10.5194/os-14-337-2018
Ardhuin, F., Gille, S. T., Menemenlis, D., Rocha, C. B., Rascle, N., Chapron, B., et al. (2017a). Small-scale open ocean currents have large effects on wind wave heights. J. Geophys. Res. Oceans 122, 4500–4517. doi: 10.1002/2016JC012413
Ardhuin, F., and Jenkins, A. D. (2006). On the interaction of surface waves and upper ocean turbulence. J. Phys. Oceanogr. 36, 551–557. doi: 10.1175/JPO2862.1
Ardhuin, F., Rogers, E., Babanin, A. V., Filipot, J.-F., Magne, R., Roland, A., et al. (2010). Semiempirical dissipation source functions for ocean waves. Part I: definition, calibration, and validation. J. Phys. Oceanogr. 40, 1917–1941. doi: 10.1175/2010JPO4324.1
Ardhuin, F., Stopa, J., Chapron, B., Collard, F., Smith, M., Thomson, J., et al. (2017b). Measuring ocean waves in sea ice using SAR imagery: a quasi-deterministic approach evaluated with Sentinel-1 and in situ data. Rem. Sens. Environ. 189, 211–222. doi: 10.1016/j.rse.2016.11.024
Ardhuin, F., Stopa, J. E., Chapron, B., Collard, F., Husson, R., Jensen, R. E., et al. (2019). Observing sea states. Front. Mar. Sci. 6:124. doi: 10.3389/fmars.2019.00124
Aristizábal, M. F., Fewings, M. R., and Washburn, L. (2016). Contrasting spatial patterns in the diurnal and semidiurnal temperature variability in the Santa Barbara Channel, California. J. Geophys. Res. Oceans 121, 427–440. doi: 10.1002/2015JC011239
Aristizábal, M. F., Fewings, M. R., and Washburn, L. (2017). Effects of the relaxation of upwelling-favorable winds on the diurnal and semidiurnal water temperature fluctuations in the Santa Barbara Channel, California. J. Geophys. Res. Oceans 122, 7958–7977. doi: 10.1002/2017JC013199
Armitage, T. W., Bacon, S., Ridout, A. L., Petty, A. A., Wolbach, S., and Tsamados, M. (2017). Arctic Ocean surface geostrophic circulation 2003–2014. Cryosphere 11, 1767–1780. doi: 10.5194/tc-11-1767-2017
Armitage, T. W., Bacon, S., Ridout, A. L., Thomas, S. F., Aksenov, Y., and Wingham, D. J. (2016). Arctic sea surface height variability and change from satellite radar altimetry and GRACE, 2003–2014. J. Geophys. Res. Oceans 121, 4303–4322. doi: 10.1002/2015JC011579
Atlas, D. (1994). Origin of storm footprints on the sea seen by synthetic aperture radar. Science 266, 1364–1366. doi: 10.1126/science.266.5189.1364
Atlas, R., Hoffman, R., Leidner, S., Sienkiewicz, J., Yu, T.-W., Bloom, S., et al. (2001). The effects of marine winds from scatterometer data on weather analysis and forecasting. Bull. Am. Meteorol. Soc. 82, 1965–1990. doi: 10.1175/1520-0477(2001)082<1965:TEOMWF>2.3.CO;2
Atlas, R., Hoffman, R. N., Ardizzone, J., Leidner, S. M., Jusem, J. C., Smith, D. K., et al. (2011). A cross-calibrated, multiplatform ocean surface wind velocity product for meteorological and oceanographic applications. Bull. Am. Meteorol. Soc. 92, 157–174. doi: 10.1175/2010BAMS2946.1
Austin, J. (1999). The role of the alongshore wind stress in the heat budget of the North Carolina inner shelf. J. Geophys. Res. 104, 18187–18204. doi: 10.1029/1998JC900122
Babanin, A. V. (2006). On a wave-induced turbulence and a wave-mixed upper ocean layer. Geophys. Res. Lett. 33:L20605. doi: 10.1029/2006GL027308
Bachman, S. D., Fox-Kemper, B., Taylor, J. R., and Thomas, L. (2017). Parameterization of frontal symmetric instabilities. I: theory for resolved fronts. Ocean Modell. 109, 72–95. doi: 10.1016/j.ocemod.2016.12.003
Ballarotta, M., Ubelmann, C., Pujol, M.-I., Taburet, G., Legeais, J.-F., Faugere, Y., et al. (2019). On the resolution of ocean altimetry maps. Ocean Sci. 156. doi: 10.5194/os-2018-156
Banas, N. S., Hickey, B. M., MacCready, P., and Newton, J. A. (2004). Dynamics of Willapa Bay, Washington: a highly unsteady, partially mixed estuary. J. Phys. Oceanogr. 34, 2413–2427. doi: 10.1175/JPO2637.1
Barrick, D. E., Evans, M., and Weber, B. (1977). Ocean surface currents mapped by radar. Science 198, 138–144. doi: 10.1126/science.198.4313.138
Beal, R. C. (1980). The Seasat SAR Wind and Ocean Wave Monitoring Capabilities: A Case Study for Pass 1339M. APL Tech. Rep. S1R 79U-019. Laurel, MD: Johns Hopkins University.
Belcher, S. E., Grant, A. L., Hanley, K. E., Fox-Kemper, B., Van Roekel, L., Sullivan, P. P., et al. (2012). A global perspective on Langmuir turbulence in the ocean surface boundary layer. Geophys. Res. Lett. 39:L18605. doi: 10.1029/2012GL052932
Belcher, S. E., Hewitt, H. T., Beljaars, A., Brun, E., Fox-Kemper, B., Lemieux, J.-F., et al. (2015). “Ocean–waves–sea ice–atmosphere interactions,” in Seamless Prediction of the Earth System: From Minutes to Months, eds G. Brunet, S. Jones, and P. M. Ruti (Geneva: World Meteorological Organization, 2015).
Bjerknes, J. (1969). Atmospheric teleconnections from the equatorial pacific. Monthly Weather Rev. 97, 163–172. doi: 10.1175/1520-0493(1969)097<0163:ATFTEP>2.3.CO;2
Bonjean, F., and Lagerloef, G. S. (2002). Diagnostic model and analysis of the surface currents in the tropical Pacific Ocean. J. Phys. Oceanogr. 32, 2938–2954. doi: 10.1175/1520-0485(2002)032<2938:DMAAOT>2.0.CO;2
Bourassa, M., Meissner, T., Cerovecki, I., Chang, P., Dong, X., De Chiara, G., et al. (2019). Remotely sensed winds and wind stresses for marine forecasting and ocean modeling. Front. Mar. Sci. 9:443. doi: 10.3389/fmars.2019.00443
Bourassa, M. A., Rodriguez, E., and Chelton, D. (2016). “Winds and currents mission: ability to observe mesoscale AIR/SEA coupling,” in Geoscience and Remote Sensing Symposium (IGARSS), 2016 IEEE International (Beijing: IEEE), 7392–7395.
Boutin, G., Ardhuin, F., Dumont, D., Sévigny, C., Girard-Ardhuin, F., and Accensi, M. (2018). Floe size effect on wave-ice interactions: possible effects, implementation in wave model, and evaluation. J. Geophys. Res. Oceans 123, 4779–4805. doi: 10.1029/2017JC013622
Bowen, M. M., Emery, W. J., Wilkin, J. L., Tildesley, P. C., Barton, I. J., and Knewtson, R. (2002). Extracting multiyear surface currents from sequential thermal imagery using the maximum cross-correlation technique. J. Atmos. Ocean. Technol. 19, 1665–1676. doi: 10.1175/1520-0426(2002)019<1665:EMSCFS>2.0.CO;2
Brandt, P., Funk, A., Hormann, V., Dengler, M., Greatbatch, R. J., and Toole, J. M. (2011). Interannual atmospheric variability forced by the deep equatorial Atlantic Ocean. Nature 473:497. doi: 10.1038/nature10013
Brannigan, L., Marshall, D. P., Naveira Garabato, A., and Nurser, A. G. (2015). The seasonal cycle of submesoscale flows. Ocean Modell. 92, 69–84. doi: 10.1016/j.ocemod.2015.05.002
Brink, K. H. (2016a). Continental shelf baroclinic instability. Part I: Relaxation from upwelling or downwelling. J. Phys. Oceanogr. 46, 551–568. doi: 10.1175/JPO-D-15-0047.1
Brink, K. H. (2016b). Cross-shelf exchange. Annu. Rev. Mar. Sci. 8, 59–78. doi: 10.1146/annurev-marine-010814-015717
Brink, K. H. (2017). Surface cooling, winds, and eddies over the continental shelf. J. Phys. Oceanogr. 47, 879–894. doi: 10.1175/JPO-D-16-0196.1
Brink, K. H., and Cherian, D. A. (2013). Instability of an idealized tidal mixing front: symmetric instabilities and frictional effects. J. Mar. Res. 71, 425–450. doi: 10.1357/002224013812587582
Brink, K. H., and Seo, H. (2016). Continental shelf baroclinic instability. Part II: oscillating wind forcing. J. Phys. Oceanogr. 46, 569–582. doi: 10.1175/JPO-D-15-0048.1
Brown, R. A. (1980). Longitudinal instabilities and secondary flows in the planetary boundary layer: a review. Rev. Geophys. 18, 683–697. doi: 10.1029/RG018i003p00683
Brown, R. A. (1986). On a satellite scatterometer as an anemometer. J. Geophys. Res. 91, 2221–2232. doi: 10.1029/JC091iC02p02221
Caldeira, R. M. A., Marchesiello, P., Nezlin, N. P., DiGiacomo, P. M., and McWilliams, J. C. (2005). Island wakes in the Southern California Bight. J. Geophys. Res. 110:90. doi: 10.1029/2004JC002675
Callies, J., Ferrari, R., Klymak, J. M., and Gula, J. (2015). Seasonality in submesoscale turbulence. Nat. Commun. 6:6862. doi: 10.1038/ncomms7862
Cardellach, E., Fabra, F., Nogués-Correig, O., Oliveras, S., Ribó, S., and Rius, A. (2011). GNSS-R ground-based and airborne campaigns for ocean, land, ice, and snow techniques: application to the GOLD-RTR data sets. Radio Sci. 46, 1–16. doi: 10.1029/2011RS004683
Carr, J. R., Stokes, C., and Vieli, A. (2014). Recent retreat of major outlet glaciers on Novaya Zemlya, Russian Arctic, influenced by fjord geometry and sea-ice conditions. J. Glaciol. 60, 155–170. doi: 10.3189/2014JoG13J122
Caudal, G., Hauser, D., Valentin, R., and Le Gac, C. (2014). KuROS: a new airborne Ku-band Doppler radar for observation of surfaces. J. Atmos. Ocean. Technol. 31, 2223–2245. doi: 10.1175/JTECH-D-14-00013.1
Cavaleri, L., Fox-Kemper, B., and Hemer, M. (2012). Wind waves in the coupled climate system. Bull. Am. Meteorol. Soc. 93, 1651–1661. doi: 10.1175/BAMS-D-11-00170.1
Centurioni, L., Braasch, L., Di Lauro, E., Contestabile, P., De Leo, F., Casotti, R., et al. (2017). A new strategic wave measurement station off Naples port main breakwater. Coast. Eng. Proc. 1:36. doi: 10.9753/icce.v35.waves.36
Chan, F., Barth, J. A., Lubchenco, J., Kirincich, A., Weeks, H., Peterson, W. T., et al. (2008). Emergence of anoxia in the California Current large marine ecosystem. Science 319:920. doi: 10.1126/science.1149016
Chapman, D. C., and Beardsley, R. C. (1989). On the origin of shelf water in the Middle Atlantic Bight. J. Phys. Oceanogr. 19, 384–391. doi: 10.1175/1520-0485(1989)019<0384:OTOOSW>2.0.CO;2
Chapron, B., Collard, F., and Ardhuin, F. (2005). Direct measurements of ocean surface velocity from space: interpretation and validation. J. Geophys. Res. Oceans 110:C07008. doi: 10.1029/2004JC002809
Chapron, B., Collard, F., and Kerbaol, V. (2004). “Satellite synthetic aperture radar sea surface doppler measurements,” in 2nd Workshop on Coastal and Marine Applications of Synthetic Aperture Radar (Svalbard), 133–141.
Chavez, F. P., and Messié, M. (2009). A comparison of eastern boundary upwelling ecosystems. Progr. Oceanogr. 83, 80–96. doi: 10.1016/j.pocean.2009.07.032
Chelton, D. (2001). Report of the High-Resolution Ocean Topography Science Working Group Meeting. Oregon State University, College of Oceanic and Atmospheric Sciences.
Chelton, D. B., Deszoeke, R. A., Schlax, M. G., El Naggar, K., and Siwertz, N. (1998). Geographical variability of the first baroclinic Rossby radius of deformation. J. Phys. Oceanogr. 28, 433–460. doi: 10.1175/1520-0485(1998)028<0433:GVOTFB>2.0.CO;2
Chelton, D. B., Freilich, M. H., Sienkiewicz, J. M., and Von Ahn, J. M. (2006). On the use of QuikSCAT scatterometer measurements of surface winds for marine weather prediction. Monthly Weather Rev. 134, 2055–2071. doi: 10.1175/MWR3179.1
Chelton, D. B., Gaube, P., Schlax, M. G., Early, J. J., and Samelson, R. M. (2011). The influence of nonlinear mesoscale eddies on near-surface oceanic chlorophyll. Science 334, 328–332. doi: 10.1126/science.1208897
Chelton, D. B., Schlax, M. G., Freilich, M. H., and Milliff, R. F. (2004). Satellite measurements reveal persistent small-scale features in ocean winds. Science 303, 978–983. doi: 10.1126/science.1091901
Chelton, D. B., Schlax, M. G., and Samelson, R. M. (2007). Summertime coupling between sea surface temperature and wind stress in the California current system. J. Phys. Oceanogr. 37, 495–517. doi: 10.1175/JPO3025.1
Chelton, D. B., and Xie, S.-P. (2010). Coupled ocean-atmosphere interaction at oceanic mesoscales. Oceanography 23, 52–69. doi: 10.5670/oceanog.2010.05
Chen, C. (2003). Wind-induced, cross-frontal exchange on Georges Bank: a mechanism for early summer on-bank biological particle transport. J. Geophys. Res. 108:589. doi: 10.1029/2002JC001358
Chen, C.-T. A., Huang, T.-H., Chen, Y.-C., Bai, Y., He, X., and Kang, Y. (2013). Air–sea exchanges of CO2 in the world's coastal seas. Biogeosciences 10, 6509–6544. doi: 10.5194/bg-10-6509-2013
Chen, S.-Y., and Chen, S.-N. (2017). Generation of upwelling circulation under downwelling-favorable wind within bottom-attached, buoyant coastal currents. J. Phys. Oceanogr. 47, 2499–2519. doi: 10.1175/JPO-D-16-0271.1
Cherian, D. A. (2016). When an eddy encounters shelf-slope topography (PhD thesis), Cambridge, MA: Massachusetts Institute of Technology.
Cherian, D. A., and Brink, K. H. (2016). Offshore transport of shelf water by deep-ocean eddies. J. Phys. Oceanogr. 46, 3599–3621. doi: 10.1175/JPO-D-16-0085.1
Cherian, D. A., and Brink, K. H. (2018). Shelf flows forced by deep-ocean anticyclonic eddies at the shelf break. J. Phys. Oceanogr. 48, 1117–1138. doi: 10.1175/JPO-D-17-0237.1
Clarizia, M., Gommenginger, C., Gleason, S., Srokosz, M., Galdi, C., and Di Bisceglie, M. (2009). Analysis of GNSS-R delay-Doppler maps from the UK-DMC satellite over the ocean. Geophys. Res. Lett. 36:L02608. doi: 10.1029/2008GL036292
Colbert, D. B. (2010). Field Evaluation of Ocean Wave Measurement With GPS Buoys. Technical report. Monterey, CA: Department of Oceanography, Naval Postgraduate School.
Collard, F., Ardhuin, F., and Chapron, B. (2009). Monitoring and analysis of ocean swell fields from space: new methods for routine observations. J. Geophys. Res. Oceans 114:C07023. doi: 10.1029/2008JC005215
Connolly, T. P., Hickey, B. M., Geier, S. L., and Cochlan, W. P. (2010). Processes influencing seasonal hypoxia in the northern California current system. J. Geophys. Res. 115:C03021. doi: 10.1029/2009JC005283
Connolly, T. P., and Lentz, S. J. (2014). Interannual variability of wintertime temperature on the inner continental shelf of the Middle Atlantic Bight. J. Geophys. Res. Oceans 119, 6269–6285. doi: 10.1002/2014JC010153
Cook, T., Hazard, L., Otero, M., and Zelenke, B. (2008). Deployment and Maintenance of a High-Frequency Radar for Ocean Surface Current Mapping: Best Practices. San Diego, CA: Coastal Observing Research and Development Center. Available online at: http://cordc.ucsd.edu/projects/mapping/documents/SCCOOS-BestPractices.pdf
Cornillon, P., and Park, K.-A. (2001). Warm core ring velocities inferred from NSCAT. Geophys. Res. Lett. 28, 575–578. doi: 10.1029/2000GL011487
Cornuelle, B., Chereskin, T., Niiler, P., Morris, M., and Musgrave, D. (2000). Observations and modeling of a California undercurrent eddy. J. Geophys. Res. Oceans 105, 1227–1243. doi: 10.1029/1999JC900284
Cowen, R. K., and Sponaugle, S. (2009). Larval dispersal and marine population connectivity. Annu. Rev. Mar. Sci. 1, 443–466. doi: 10.1146/annurev.marine.010908.163757
Cowton, T., Sole, A., Nienow, P., Slater, D., and Christoffersen, P. (2018). Linear response of east Greenland's tidewater glaciers to ocean/atmosphere warming. Proc. Natl. Acad. Sci. U.S.A. 115, 7907–7912. doi: 10.1073/pnas.1801769115
Criales, M. M., Cherubin, L. M., and Browder, J. A. (2015). Modeling larval transport and settlement of pink shrimp in South Florida: dynamics of behavior and tides. Mar. Coast. Fish. 7, 148–176. doi: 10.1080/19425120.2014.1001541
Crombie, D. D. (1955). Doppler spectrum of sea echo at 13.56 Mc./s. Nature 175:681. doi: 10.1038/175681a0
Dai, A., and Deser, C. (1999). Diurnal and semidiurnal variations in global surface wind and divergence fields. J. Geophys. Res. Atmos. 104, 31109–31125. doi: 10.1029/1999JD900927
Dai, A., and Trenberth, K. E. (2004). The diurnal cycle and its depiction in the community climate system model. J. Clim. 17, 930–951. doi: 10.1175/1520-0442(2004)017<0930:TDCAID>2.0.CO;2
D'Asaro, E. A. (1985). The energy flux from the wind to near-inertial motions in the surface mixed layer. J. Phys. Oceanogr. 15, 1043–1059. doi: 10.1175/1520-0485(1985)015<1043:TEFFTW>2.0.CO;2
D'Asaro, E. A. (2001). Turbulent vertical kinetic energy in the ocean mixed layer. J. Phys. Oceanogr. 31, 3530–3537. doi: 10.1175/1520-0485(2002)031<3530:TVKEIT>2.0.CO;2
D'Asaro, E. A. (2014). Turbulence in the upper-ocean mixed layer. Annu. Rev. Mar. Sci. 6, 101–115. doi: 10.1146/annurev-marine-010213-135138
D'Asaro, E. A., Lee, C., Rainville, L., Thomas, L., and Harcourt, R. (2011). Enhanced turbulence and energy dissipation at ocean fronts. Science 332:1201515. doi: 10.1126/science.1201515
D'Asaro, E. A., Sanford, T. B., Niiler, P. P., and Terrill, E. J. (2007). Cold wake of hurricane Frances. Geophys. Res. Lett. 34:L15609. doi: 10.1029/2007GL030160
D'Asaro, E. A., Shcherbina, A. Y., Klymak, J. M., Molemaker, J., Novelli, G., Guigand, C. M., et al. (2018). Ocean convergence and the dispersion of flotsam. Proc. Natl. Acad. Sci. U.S.A. 115:201718453. doi: 10.1073/pnas.1718453115
De Vries, J., Waldron, J., and Cunningham, V. (2003). Field tests of the new Datawell DWR-G GPA wave buoy. Sea Technol. 44, 50–55. Available online at: http://datawell.nl/Portals/0/Documents/Publications/datawell_publication_dwr-g_seatechnology-dec2003_2003-12-01.pdf
Deike, L., Melville, W. K., and Popinet, S. (2016). Air entrainment and bubble statistics in breaking waves. J. Fluid Mech. 801, 91–129. doi: 10.1017/jfm.2016.372
Dewar, W., and Flierl, G. (1987). Some effects of the wind on rings. J. Phys. Oceanogr. 17, 1653–1667. doi: 10.1175/1520-0485(1987)017<1653:SEOTWO>2.0.CO;2
Doble, M. J., and Bidlot, J.-R. (2013). Wave buoy measurements at the Antarctic sea ice edge compared with an enhanced ECMWF WAM: progress towards global waves-in-ice modelling. Ocean Modell. 70, 166–173. doi: 10.1016/j.ocemod.2013.05.012
Doble, M. J., Wilkinson, J. P., Valcic, L., Robst, J., Tait, A., Preston, M., et al. (2017). Robust wavebuoys for the marginal ice zone: experiences from a large persistent array in the Beaufort Sea. Elem. Sci. Anth. 5:47. doi: 10.1525/elementa.233
Donato, T. F., and Marmorino, G. O. (2002). The surface morphology of a coastal gravity current. Cont. Shelf Res. 22, 141–146. doi: 10.1016/S0278-4343(01)00066-8
Donelan, M. A., Dobson, F. W., Smith, S. D., and Anderson, R. J. (1993). On the dependence of sea surface roughness on wave development. J. Phys. Oceanogr. 23, 2143–2149. doi: 10.1175/1520-0485(1993)023<2143:OTDOSS>2.0.CO;2
Dong, C., Chen, D., and Ou, H.-W. (2015). Numerical study on tidally induced cross-frontal mean circulation. Atmos. Ocean 53, 363–375. doi: 10.1080/07055900.2015.1056082
Dorman, C. E., Mejia, J., Koračin, D., and McEvoy, D. (2017). “Worldwide marine fog occurrence and climatology,” in Marine Fog: Challenges and Advancements in Observations, Modeling, and Forecasting, eds D. Koračin, and C. E. Dorman (Cham: Springer International Publishing), 7–152.
Dotto, T. S., Naveira Garabato, A. C., Bacon, S., Tsamados, M., Holland, P. R., Hooley, J., et al. (2018). Variability of the Ross Gyre, Southern Ocean: drivers and responses revealed by satellite altimetry. Geophys. Res. Lett. 45, 6195–6204. doi: 10.1029/2018GL078607
Drake, P. T., Edwards, C. A., Morgan, S. G., and Dever, E. P. (2013). Influence of larval behavior on transport and population connectivity in a realistic simulation of the California Current System. J. Mar. Res. 71, 317–350. doi: 10.1357/002224013808877099
Dumbauld, B. R., Kauffman, B. E., Trimble, A. C., and Ruesink, J. L. (2011). The Willapa Bay oyster reserves in Washington State: fishery collapse, creating a sustainable replacement, and the potential for habitat conservation and restoration. J. Shellfish Res. 30, 71–83. doi: 10.2983/035.030.0111
Dumont, D., Kohout, A., and Bertino, L. (2011). A wave-based model for the marginal ice zone including a floe breaking parameterization. J. Geophys. Res. Oceans 116:C04001. doi: 10.1029/2010JC006682
Edson, J. B., Jampana, V., Weller, R. A., Bigorre, S. P., Plueddemann, A. J., Fairall, C. W., et al. (2013). On the exchange of momentum over the open ocean. J. Phys. Oceanogr. 43, 1589–1610. doi: 10.1175/JPO-D-12-0173.1
Edwards, K. A., Rogers, D. P., and Dorman, C. E. (2002). Adjustment of the marine atmospheric boundary layer to the large-scale bend in the California coast. J. Geophys. Res. 107:3213. doi: 10.1029/2001JC000807
Elipot, S., Lumpkin, R., Perez, R. C., Lilly, J. M., Early, J. J., and Sykulski, A. M. (2016). A global surface drifter data set at hourly resolution. J. Geophys. Res. Oceans 121, 2937–2966. doi: 10.1002/2016JC011716
Ellenson, A., and Özkan-Haller, H. T. (2018). Predicting large ocean wave events characterized by bimodal energy spectra in the presence of a low-level southerly wind feature. Weather Forecast. e0035.1. doi: 10.1175/WAF-D-17-0035.1
Fan, Y., and Griffies, S. M. (2014). Impacts of parameterized Langmuir turbulence and nonbreaking wave mixing in global climate simulations. J. Clim. 27, 4752–4775. doi: 10.1175/JCLI-D-13-00583.1
Feltham, D. L. (2008). Sea ice rheology. Annu. Rev. Fluid Mech. 40, 91–112. doi: 10.1146/annurev.fluid.40.111406.102151
Feng, H., Vandemark, D., and Wilkin, J. (2016). Gulf of Maine salinity variation and its correlation with upstream Scotian shelf currents at seasonal and interannual time scales. J. Geophys. Res. Oceans 121, 8585–8607. doi: 10.1002/2016JC012337
Fewings, M. R. (2017). Large-scale structure in wind forcing over the California current system in summer. Monthly Weather Rev. 145, 4227–4247. doi: 10.1175/MWR-D-17-0106.1
Fewings, M. R., and Lentz, S. J. (2010). Momentum balances on the inner continental shelf at Martha's Vineyard Coastal Observatory. J. Geophys. Res. 115:C12023. doi: 10.1029/2009JC005578
Fewings, M. R., and Lentz, S. J. (2011). Summertime cooling of the shallow continental shelf. J. Geophys. Res. 116:C07015. doi: 10.1029/2010JC006744
Fewings, M. R., Lentz, S. J., and Fredericks, J. (2008). Observations of cross-shelf flow driven by cross-shelf winds over the inner continental shelf. J. Phys. Oceanogr. 38, 2358–2378. doi: 10.1175/2008JPO3990.1
Fewings, M. R., Washburn, L., Dorman, C. E., Gotschalk, C., and Lombardo, K. (2016). Synoptic forcing of wind relaxations at Pt. Conception, California. J. Geophys. Res. Oceans 121, 5711–5730. doi: 10.1002/2016JC011699
Fewings, M. R., Washburn, L., and Ohlmann, J. C. (2015). Coastal water circulation patterns around the Northern Channel Islands and Point Conception, California. Progr. Oceanogr. 138, 283–304. doi: 10.1016/j.pocean.2015.10.001
Flynn, K. R., Fewings, M. R., Gotschalk, C., and Lombardo, K. (2017). Large-scale anomalies in sea-surface temperature and air-sea fluxes during wind relaxation events off the United States West Coast in summer. J. Geophys. Res. Oceans 122, 2574–2594. doi: 10.1002/2016JC012613
Fois, F., Hoogeboom, P., Le Chevalier, F., Stoffelen, A., and Mouche, A. (2015). DopSCAT: a mission concept for simultaneous measurements of marine winds and surface currents. J. Geophys. Res. Oceans 120, 7857–7879. doi: 10.1002/2015JC011011
Foltz, G. R., and McPhaden, M. J. (2008). Impact of Saharan dust on tropical North Atlantic SST. J. Clim. 21, 5048–5060. doi: 10.1175/2008JCLI2232.1
Foltz, G. R., Schmid, C., and Lumpkin, R. (2018). An enhanced PIRATA dataset for tropical Atlantic Ocean–atmosphere research. J. Clim. 31, 1499–1524. doi: 10.1175/JCLI-D-16-0816.1
Fong, D. A., and Geyer, R. W. (2001). Response of a river plume during an upwelling favorable wind event. J. Geophys. Res. Oceans 106, 1067–1084. doi: 10.1029/2000JC900134
Fong, D. A., and Geyer, W. R. (2002). The alongshore transport of freshwater in a surface-trapped river plume. J. Phys. Oceanogr. 32, 957–972. doi: 10.1175/1520-0485(2002)032<0957:TATOFI>2.0.CO;2
Foster, R. C. (2005). Why rolls are prevalent in the hurricane boundary layer. J. Atmos. Sci. 62, 2647–2661. doi: 10.1175/JAS3475.1
Foti, G., Gommenginger, C., Jales, P., Unwin, M., Shaw, A., Robertson, C., et al. (2015). Spaceborne GNSS reflectometry for ocean winds: first results from the UK TechDemoSat-1 mission. Geophys. Res. Lett. 42, 5435–5441. doi: 10.1002/2015GL064204
Foti, G., Gommenginger, C., and Srokosz, M. (2017). First spaceborne GNSS-reflectometry observations of hurricanes from the UK TechDemoSat-1 mission. Geophys. Res. Lett. 44, 12358–12366. doi: 10.1002/2017GL076166
Fox-Kemper, B., Bachman, S., Pearson, B., and Reckinger, S. (2014). Principles and advances in subgrid modelling for eddy-rich simulations. Clivar Exchanges 65, 42–46. Available online at: http://bit.ly/1qSMTzA
Fox-Kemper, B., Danabasoglu, G., Ferrari, R., Griffies, S., Hallberg, R., Holland, M., et al. (2011). Parameterization of mixed layer eddies. III: implementation and impact in global ocean climate simulations. Ocean Modell. 39, 61–78. doi: 10.1016/j.ocemod.2010.09.002
Fram, J., Stewart, H., Brzezinski, M., Gaylord, B., Reed, D., Williams, S., et al. (2008). Physical pathways and utilization of nitrate supply to the giant kelp, Macrocystis pyrifera. Limnol. Oceanogr. 53, 1589–1603. doi: 10.4319/lo.2008.53.4.1589
Fraser, C. I., Morrison, A. K., Hogg, A. M., Macaya, E. C., van Sebille, E., Ryan, P. G., et al. (2018). Antarctica's ecological isolation will be broken by storm-driven dispersal and warming. Nat. Clim. Change 8:704. doi: 10.1038/s41558-018-0209-7
Fratantoni, P. S., and Pickart, R. S. (2007). The western North Atlantic shelfbreak current system in summer. J. Phys. Oceanogr. 37, 2509–2533. doi: 10.1175/JPO3123.1
Frenger, I., Gruber, N., Knutti, R., and Münnich, M. (2013). Imprint of southern ocean eddies on winds, clouds and rainfall. Nat. Geosci. 6:608. doi: 10.1038/ngeo1863
Fu, L.-L., and Glazman, R. (1991). The effect of the degree of wave development on the sea state bias in radar altimetry measurement. J. Geophys. Res. Oceans 96, 829–834. doi: 10.1029/90JC02319
Fu, L. L., and Holt, B. (1982). Seasat Views Oceans and Seas With Synthetic Aperture Radar. Tech. Rep. JPL Publ. 81–102. Pasadena, CA: Jet Propulsion Laboratory, California Institute of Technology.
Fujimura, A. G., Reniers, A. J., Paris, C. B., Shanks, A. L., MacMahan, J. H., and Morgan, S. G. (2014). Numerical simulations of larval transport into a rip-channeled surf zone. Limnol. Oceanogr. 59, 1434–1447. doi: 10.4319/lo.2014.59.4.1434
Garcia Berdeal, I., Hickey, B. M., and Kawase, M. (2002). Influence of wind stress and ambient flow on a high discharge river plume. J. Geophys. Res. Oceans 107, 13–11. doi: 10.1029/2001JC000932
García-Medina, G., Özkan-Haller, H. T., Ruggiero, P., and Oskamp, J. (2013). An inner-shelf wave forecasting system for the US Pacific Northwest. Weather Forecast. 28, 681–703. doi: 10.1175/WAF-D-12-00055.1
García-Reyes, M., and Largier, J. (2010). Observations of increased wind-driven coastal upwelling off central California. J. Geophys. Res. Oceans 115:C04011. doi: 10.1029/2009JC005576
Garratt, J. (1977). Review of drag coefficients over oceans and continents. Monthly Weather Rev. 105, 915–929. doi: 10.1175/1520-0493(1977)105<0915:RODCOO>2.0.CO;2
Garrison, J. L., Katzberg, S. J., and Hill, M. I. (1998). Effect of sea roughness on bistatically scattered range coded signals from the global positioning system. Geophys. Res. Lett. 25, 2257–2260. doi: 10.1029/98GL51615
Gaube, P., Chelton, D. B., Samelson, R. M., Schlax, M. G., and O'Neill, L. W. (2015). Satellite observations of mesoscale eddy-induced Ekman pumping. J. Phys. Oceanogr. 45, 104–132. doi: 10.1175/JPO-D-14-0032.1
Gaube, P., McGillicuddy, D. J., Chelton, D. B., Behrenfeld, M. J., and Strutton, P. G. (2014). Regional variations in the influence of mesoscale eddies on near-surface chlorophyll. J. Geophys. Res. Oceans 119, 8195–8220. doi: 10.1002/2014JC010111
Gawarkiewicz, G., McCarthy, R. K., Barton, K., Masse, A. K., and Church, T. M. (1990). A Gulf Stream-derived pycnocline intrusion on the Middle Atlantic Bight shelf. J. Geophys. Res. Oceans 95, 22305–22313. doi: 10.1029/JC095iC12p22305
Gawarkiewicz, G., Monismith, S., and Largier, J. (2007). Observing larval transport processes affecting population connectivity: progress and challenges. Oceanography 20, 40–53. doi: 10.5670/oceanog.2007.28
Gentemann, C. L., Minnett, P. J., and Ward, B. (2009). Profiles of ocean surface heating (POSH): a new model of upper ocean diurnal warming. J. Geophys. Res. Oceans 114:C07017. doi: 10.1029/2008JC004825
Gerbi, G. P., Chant, R. J., and Wilkin, J. L. (2013). Breaking surface wave effects on river plume dynamics during upwelling-favorable winds. J. Phys. Oceanogr. 43, 1959–1980. doi: 10.1175/JPO-D-12-0185.1
Gerling, T. W. (1986). Structure of the surface wind field from the Seasat SAR. J. Geophys. Res. 91, 2308–2320. doi: 10.1029/JC091iC02p02308
Giglio, D., Gille, S. T., Subramanian, A. C., and Nguyen, S. (2017). The role of wind gusts in upper ocean diurnal variability. J. Geophys. Res. Oceans 122, 7751–7764. doi: 10.1002/2017JC012794
Giles, K. A., Laxon, S. W., Ridout, A. L., Wingham, D. J., and Bacon, S. (2012). Western Arctic Ocean freshwater storage increased by wind-driven spin-up of the Beaufort Gyre. Nat. Geosci. 5:194. doi: 10.1038/ngeo1379
Gille, S. T., Llewellyn Smith, S. G., and Lee, S. M. (2003). Measuring the sea breeze from QuikSCAT scatterometry. Geophys. Res. Lett. 30:1114. doi: 10.1029/2002GL016230
Gille, S. T., Llewellyn Smith, S. G., and Statom, N. M. (2005). Global observations of the land breeze. Geophys. Res. Lett. 32:L05605. doi: 10.1029/2004GL022139
Gimbert, F., Marsan, D., Weiss, J., Jourdain, N. C., and Barnier, B. (2012). Sea ice inertial oscillations in the Arctic Basin. Cryosphere 6, 1187–1201. doi: 10.5194/tc-6-1187-2012
Goldstein, R., Zebker, H., and Barnett, T. (1989). Remote sensing of ocean currents. Science 246, 1282–1285. doi: 10.1126/science.246.4935.1282
Gommenginger, C. (2019). SEASTAR: a mission to study ocean submesoscale dynamics and small- scale atmosphere-ocean processes in coastal, shelf and polar seas. Front. Mar. Sci. 601. doi: 10.13140/RG.2.2.20415.61601
Gommenginger, C., Chapron, B., Martin, A., Marquez, J., Brownsword, C., and Buck, C. (2018). “SEASTAR: a new mission for high-resolution imaging of ocean surface current and wind vectors from space,” in EUSAR 2018; 12th European Conference on Synthetic Aperture Radar (Aachen: VDE), 1–4.
González-Haro, C., Autret, E., Isern-Fontanet, J., Tandeo, P., and Garello, R. (2016). “Ocean surface current reconstruction: on the transfer function between infrared SST and along-track altimeter observations,” in OCEANS 2016 MTS/IEEE Monterey (Monterey, CA: IEEE), 1–5.
Grachev, A., Fairall, C., Hare, J., Edson, J., and Miller, S. (2003). Wind stress vector over ocean waves. J. Phys. Oceanogr. 33, 2408–2429. doi: 10.1175/1520-0485(2003)033<2408:WSVOOW>2.0.CO;2
Graham, W. M., and Largier, J. L. (1997). Upwelling shadows as nearshore retention sites: the example of northern Monterey Bay. Cont. Shelf Res. 17, 509–532. doi: 10.1016/S0278-4343(96)00045-3
Grant, A. L., and Belcher, S. E. (2009). Characteristics of Langmuir turbulence in the ocean mixed layer. J. Phys. Oceanogr. 39, 1871–1887. doi: 10.1175/2009JPO4119.1
Grantham, B. A., Chan, F., Nielsen, K. J., Fox, D. S., Barth, J. A., Huyer, A., et al. (2004). Upwelling-driven nearshore hypoxia signals ecosystem and oceanographic changes in the northeast Pacific. Nature 429, 749–754. doi: 10.1038/nature02605
Gula, J., Molemaker, M. J., and McWilliams, J. C. (2014). Submesoscale cold filaments in the Gulf Stream. J. Phys. Oceanogr. 44, 2617–2643. doi: 10.1175/JPO-D-14-0029.1
Hackerott, J. A., Pezzi, L. P., Paskyabi, M. B., Oliveira, A. P., Reuder, J., de Souza, R. B., et al. (2018). The role of roughness and stability on the momentum flux in the marine atmospheric surface layer: a study on the southwestern atlantic ocean. J. Geophys. Res. Atmos. 123, 3914–3932. doi: 10.1002/2017JD027994
Haine, T. W., and Marshall, J. (1998). Gravitational, symmetric, and baroclinic instability of the ocean mixed layer. J. Phys. Oceanogr. 28, 634–658. doi: 10.1175/1520-0485(1998)028<0634:GSABIO>2.0.CO;2
Hales, B., Karp-Boss, L., Perlin, A., and Wheeler, P. A. (2006). Oxygen production and carbon sequestration in an upwelling coastal margin. Glob. Biogeochem. Cycles 20:GB3001. doi: 10.1029/2005GB002517
Hales, B., Takahashi, T., and Bandstra, L. (2005). Atmospheric CO2 uptake by a coastal upwelling system. Glob. Biogeochem. Cycles 19:GB1009. doi: 10.1029/2004GB002295
Hall, C., and Cordey, R. (1988). “Multistatic scatterometry,” in International Geoscience and Remote Sensing Symposium, 1988. IGARSS'88. Remote Sensing: Moving Toward the 21st Century, Vol. 1 (Edinburgh: IEEE), 561–562.
Halpern, D. (2002). Offshore Ekman transport and Ekman pumping off Peru during the 1997–1998 El Nino. Geophys. Res. Lett. 29, 19-1–19-4. doi: 10.1029/2001GL014097
Hanafin, J. A., Quilfen, Y., Ardhuin, F., Sienkiewicz, J., and Queffeulou, P. (2012). Phenomenal sea states and swell from a North Atlantic Storm in February 2011: a comprehensive. Geophys. Res. Lett 36:L06607. doi: 10.1175/BAMS-D-11-00128.1
Haney, S., Fox-Kemper, B., Julien, K., and Webb, A. (2015). Symmetric and geostrophic instabilities in the wave-forced ocean mixed layer. J. Phys. Oceanogr. 45, 3033–3056. doi: 10.1175/JPO-D-15-0044.1
Harcourt, R. R. (2013). A second-moment closure model of Langmuir turbulence. J. Phys. Oceanogr. 43, 673–697. doi: 10.1175/JPO-D-12-0105.1
Harcourt, R. R. (2015). An improved second-moment closure model of Langmuir turbulence. J. Phys. Oceanogr. 45, 84–103. doi: 10.1175/JPO-D-14-0046.1
Harcourt, R. R., and D'Asaro, E. A. (2008). Large-eddy simulation of Langmuir turbulence in pure wind seas. J. Phys. Oceanogr. 38, 1542–1562. doi: 10.1175/2007JPO3842.1
Harlan, J., Terrill, E., Hazard, L., Keen, C., Barrick, D., Whelan, C., et al. (2010). The integrated ocean observing system high-frequency radar network: status and local, regional, and national applications. Mar. Technol. Soc. J. 44, 122–132. doi: 10.4031/MTSJ.44.6.6
Hasager, C. B., Mouche, A., Badger, M., Bingol, F., Karagali, I., Driesenaar, T., et al. (2015). Offshore wind climatology based on synergetic use of Envisat ASAR, ASCAT and QuikSCAT. Rem. Sens. Environ. 156, 247–263. doi: 10.1016/j.rse.2014.09.030
Hasselmann, K. F., Chapron, B., Aouf, L., Ardhuin, F., Collard, F., Engen, G., et al. (2013). The ERS SAR wave mode: a breakthrough in global ocean wave observations. ESA Commun. 1326, 167–197.
Hauser, D., Tison, C., Amiot, T., Delaye, L., Corcoral, N., and Castillan, P. (2017). SWIM: the first spaceborne wave scatterometer. IEEE Trans. Geosci. Rem. Sens. 55, 3000–3014. doi: 10.1109/TGRS.2017.2658672
Hendrickson, J., and MacMahan, J. (2009). Diurnal sea breeze effects on inner-shelf cross-shore exchange. Cont. Shelf Res. 29, 2195–2206. doi: 10.1016/j.csr.2009.08.011
Heorton, H. D., Feltham, D. L., and Hunt, J. C. (2014). The response of the sea ice edge to atmospheric and oceanic jet formation. J. Phys. Oceanogr. 44, 2292–2316. doi: 10.1175/JPO-D-13-0184.1
Herbers, T., Jessen, P., Janssen, T., Colbert, D., and MacMahan, J. (2012). Observing ocean surface waves with GPS-tracked buoys. J. Atmos. Ocean. Technol. 29, 944–959. doi: 10.1175/JTECH-D-11-00128.1
Hetland, R. D. (2017). Suppression of baroclinic instabilities in buoyancy-driven flow over sloping bathymetry. J. Phys. Oceanogr. 47, 49–68. doi: 10.1175/JPO-D-15-0240.1
Hibler, W., Hutchings, J., and Ip, C. (2006). Sea-ice arching and multiple flow states of Arctic pack ice. Ann. Glaciol. 44, 339–344. doi: 10.3189/172756406781811448
Hickey, B., Geier, S., Kachel, N., and MacFadyen, A. (2005). A bi-directional river plume: the Columbia in summer. Cont. Shelf Res. 25, 1631–1656. doi: 10.1016/j.csr.2005.04.010
Holland, P. R., and Kwok, R. (2012). Wind-driven trends in Antarctic sea-ice drift. Nat. Geosci. 5:872. doi: 10.1038/ngeo1627
Horvat, C., Tziperman, E., and Campin, J.-M. (2016). Interaction of sea ice floe size, ocean eddies, and sea ice melting. Geophys. Res. Lett. 43, 8083–8090. doi: 10.1002/2016GL069742
Horwitz, R., and Lentz, S. J. (2014). Inner-shelf response to cross-shelf wind stress: the importance of the cross-shelf density gradient in an idealized numerical model and field observations. J. Phys. Oceanogr. 44, 86–103. doi: 10.1175/JPO-D-13-075.1
Horwitz, R. M., and Lentz, S. J. (2016). The effect of wind direction on cross-shelf transport on an initially stratified inner shelf. J. Mar. Res. 74, 201–227. doi: 10.1357/002224016820870648
Houghton, R. W., and Ho, C. (2001). Diapycnal flow through the Georges Bank tidal front: a dye tracer study. Geophys. Res. Lett. 28, 33–36. doi: 10.1029/2000GL012298
Hristov, T., Miller, S., and Friehe, C. (2003). Dynamical coupling of wind and ocean waves through wave-induced air flow. Nature 422:55. doi: 10.1038/nature01382
Hughes, C. W., and Wilson, C. (2008). Wind work on the geostrophic ocean circulation: an observational study of the effect of small scales in the wind stress. J. Geophys. Res. Oceans 113:C02016. doi: 10.1029/2007JC004371
Hummels, R., Dengler, M., Brandt, P., and Schlundt, M. (2014). Diapycnal heat flux and mixed layer heat budget within the Atlantic Cold Tongue. Clim. Dyn. 43, 3179–3199. doi: 10.1007/s00382-014-2339-6
Hutchings, J. K., Heil, P., and Hibler III, W. D. (2005). Modeling linear kinematic features in sea ice. Monthly Weather Rev. 133, 3481–3497. doi: 10.1175/MWR3045.1
Hwang, P. A., García-Nava, H., and Ocampo-Torres, F. J. (2011). Observations of wind wave development in mixed seas and unsteady wind forcing. J. Phys. Oceanogr. 41, 2343–2362. doi: 10.1175/JPO-D-11-044.1
Isern-Fontanet, J., Chapron, B., Lapeyre, G., and Klein, P. (2006). Potential use of microwave sea surface temperatures for the estimation of ocean currents. Geophys. Res. Lett. 33:L24608. doi: 10.1029/2006GL027801
Jackson, F., Walton, W., Hines, D., Walter, B., and Peng, C. (1992). Sea surface mean square slope from K u-band backscatter data. J. Geophys. Res. Oceans 97, 11411–11427. doi: 10.1029/92JC00766
Jambeck, J. R., Geyer, R., Wilcox, C., Siegler, T. R., Perryman, M., Andrady, A., et al. (2015). Plastic waste inputs from land into the ocean. Science 347, 768–771. doi: 10.1126/science.1260352
Jochum, M., Malanotte-Rizzoli, P., and Busalacchi, A. (2004). Tropical instability waves in the Atlantic Ocean. Ocean Modell. 7, 145–163. doi: 10.1016/S1463-5003(03)00042-8
Kaplan, I. C., Williams, G. D., Bond, N. A., Hermann, A. J., and Siedlecki, S. A. (2016). Cloudy with a chance of sardines: forecasting sardine distributions using regional climate models. Fish. Oceanogr. 25, 15–27. doi: 10.1111/fog.12131
Karp-Boss, L., Wheeler, P. A., Hales, B., and Covert, P. (2004). Distributions and variability of particulate organic matter in a coastal upwelling system. J. Geophys. Res. 109:C09010. doi: 10.1029/2003JC002184
Kelly, K. A., Dickinson, S., and Johnson, G. C. (2005). Comparisons of scatterometer and TAO winds reveal time-varying surface currents for the tropical Pacific Ocean. J. Atmos. Ocean. Technol. 22, 735–745. doi: 10.1175/JTECH1738.1
Kelly, K. A., Dickinson, S., McPhaden, M. J., and Johnson, G. C. (2001). Ocean currents evident in satellite wind data. Geophys. Res. Lett. 28, 2469–2472. doi: 10.1029/2000GL012610
Kim, S. Y., Terrill, E. J., Cornuelle, B. D., Jones, B., Washburn, L., Moline, M. A., et al. (2011). Mapping the US West Coast surface circulation:a multiyear analysis of high-frequency radar observations. J. Geophys. Res. Oceans 116:C03011. doi: 10.1029/2010JC006669
Kirincich, A., Lentz, S., and Barth, J. (2009). Wave-driven inner-shelf motions on the Oregon coast. J. Phys. Oceanogr. 39, 2942–2956. doi: 10.1175/2009JPO4041.1
Kirincich, A., Lentz, S. J., and Gerbi, G. P. (2010). Calculating Reynolds stresses from ADCP measurements in the presence of surface gravity waves using the cospectra-fit method. J. Atmos. Ocean. Technol. 27, 889–890. doi: 10.1175/2009JTECHO682.1
Kirincich, A. R., and Barth, J. A. (2009). Alongshelf variability of inner-shelf circulation along the central Oregon coast during summer. J. Phys. Oceanogr. 39, 1380–1398. doi: 10.1175/2008JPO3760.1
Kitaigorodsky, S. A. (1970). The Physics of Air-Sea Interaction. Jerusalem: Israel Program for Scientific Translations.
Koch, W. (2004). Directional analysis of SAR images aiming at wind direction. IEEE Trans. Geosci. Rem. Sens. 42, 702–710. doi: 10.1109/TGRS.2003.818811
Kræmer, T., Johnsen, H., Brekke, C., and Engen, G. (2018). Comparing SAR-based short time-lag cross correlation and Doppler-derived sea ice drift velocities. IEEE Trans. Geosci. Rem. Sens. 56, 1898–1908. doi: 10.1109/TGRS.2017.2769222
Kudryavtsev, V., and Chapron, B. (2016). On growth rate of wind waves: impact of short-scale breaking modulations. J. Phys. Oceanogr. 46, 349–360. doi: 10.1175/JPO-D-14-0216.1
Kudryavtsev, V., Chapron, B., and Makin, V. (2014a). Impact of wind waves on the air-sea fluxes: a coupled model. J. Geophys. Res. Oceans 46, 1022–1037. doi: 10.1002/2013JC009412
Kudryavtsev, V., Kozlov, I., Chapron, B., and Johannessen, J. A. (2014b). Quad-polarization SAR features of ocean currents. J. Geophys. Res. Oceans 119, 6046–6065. doi: 10.1002/2014JC010173
Kudryavtsev, V., Yurovskaya, M., Chapron, B., Collard, F., and Donlon, C. (2017). Sun glitter imagery of ocean surface waves. Part 1: directional spectrum retrieval and validation. J. Geophys. Res. Oceans 122, 1369–1383. doi: 10.1002/2016JC012425
Kuitenbrouwer, D., Reniers, A., Jamie, M., and Roth, M. K. (2018). Coastal protection by a small scale river plume against oil spills in the Northern Gulf of Mexico. Cont. Shelf Res. 163, 1–11. doi: 10.1016/j.csr.2018.05.002
Kukulka, T., Plueddemann, A. J., Trowbridge, J. H., and Sullivan, P. P. (2009). Significance of Langmuir circulation in upper ocean mixing: Comparison of observations and simulations. Geophys. Res. Lett. 36:L10603. doi: 10.1029/2009GL037620
Kwok, R., and Morison, J. (2011). Dynamic topography of the ice-covered Arctic Ocean from ICESat. Geophys. Res. Lett. 38:L02501. doi: 10.1029/2010GL046063
Kwok, R., Schweiger, A., Rothrock, D., Pang, S., and Kottmeier, C. (1998). Sea ice motion from satellite passive microwave imagery assessed with ERS SAR and buoy motions. J. Geophys. Res. Oceans 103, 8191–8214. doi: 10.1029/97JC03334
LaCasce, J. (2008). Statistics from Lagrangian observations. Progr. Oceanogr. 77, 1–29. doi: 10.1016/j.pocean.2008.02.002
Laruelle, G. G., Lauerwald, R., Pfeil, B., and Regnier, P. (2014). Regionalized global budget of the CO2 exchange at the air-water interface in continental shelf seas. Glob. Biogeochem. Cycles 28, 1199–1214. doi: 10.1002/2014GB004832
Laurindo, L. C., Mariano, A. J., and Lumpkin, R. (2017). An improved near-surface velocity climatology for the global ocean from drifter observations. Deep Sea Res. 124, 73–92. doi: 10.1016/j.dsr.2017.04.009
Lavrenov, I. (2013). Wind-Waves in Oceans: Dynamics and Numerical Simulations. Berlin: Springer Science & Business Media.
Law, K. L. (2017). Plastics in the marine environment. Annu. Rev. Mar. Sci. 9, 205–229. doi: 10.1146/annurev-marine-010816-060409
Law, K. L., Morét-Ferguson, S., Maximenko, N. A., Proskurowski, G., Peacock, E. E., Hafner, J., et al. (2010). Plastic accumulation in the North Atlantic subtropical gyre. Science 329, 1185–1188. doi: 10.1126/science.1192321
Law, K. L., Morét-Ferguson, S. E., Goodwin, D. S., Zettler, E. R., DeForce, E., Kukulka, T., et al. (2014). Distribution of surface plastic debris in the eastern Pacific Ocean from an 11-year data set. Environ. Sci. Technol. 48, 4732–4738. doi: 10.1021/es4053076
Le Quéré, C., Andrew, R. M., Friedlingstein, P., Sitch, S., Pongratz, J., Manning, A. C., et al. (2017). Global carbon budget 2017. Earth Syst. Sci. Data 10, 405–448. doi: 10.5194/essd-10-405-2018
Lebreton, L. C., Van der Zwet, J., Damsteeg, J.-W., Slat, B., Andrady, A., and Reisser, J. (2017). River plastic emissions to the world's oceans. Nat. Commun. 8:15611. doi: 10.1038/ncomms15611
Lee, D.-K., and Centurioni, L. (2018). Water following characteristics of Global Drifter Program drifters with and without subsurface float. Deep Sea Res. 137, 20–29. doi: 10.1016/j.dsr.2018.05.003
Lee, T., and Liu, W. T. (2005). Effects of high-frequency wind sampling on simulated mixed layer depth and upper ocean temperature. J. Geophys. Res. Oceans 110:C05002. doi: 10.1029/2004JC002746
Lentz, S. J. (2004). The response of buoyant coastal plumes to upwelling-favorable winds. J. Phys. Oceanogr. 34, 2458–2469. doi: 10.1175/JPO2647.1
Lentz, S. J. (2010). The mean along-isobath heat and salt balances over the Middle Atlantic Bight continental shelf. J. Phys. Oceanogr. 40, 934–948. doi: 10.1175/2009JPO4214.1
Lentz, S. J., Elgar, S., and Guza, R. T. (2003). Observations of the flow field near the nose of a buoyant coastal current. J. Phys. Oceanogr. 33, 933–943. doi: 10.1175/1520-0485(2003)33<933:OOTFFN>2.0.CO;2
Lentz, S. J., and Fewings, M. R. (2012). The wind- and wave-driven inner-shelf circulation. Annu. Rev. Mar. Sci. 4, 317–343. doi: 10.1146/annurev-marine-120709-142745
Lentz, S. J., Fewings, M. R., Howd, P., Fredericks, J., and Hathaway, K. (2008). Observations and a model of undertow over the inner continental shelf. J. Phys. Oceanogr. 38, 2341–2357. doi: 10.1175/2008JPO3986.1
Lentz, S. J., and Largier, J. (2006). The influence of wind forcing on the Chesapeake Bay buoyant coastal current. J. Phys. Oceanogr. 36, 1305–1316. doi: 10.1175/JPO2909.1
Li, Q., and Fox-Kemper, B. (2017). Assessing the effects of Langmuir turbulence on the entrainment buoyancy flux in the ocean surface boundary layer. J. Phys. Oceanogr. 47, 2863–2886. doi: 10.1175/JPO-D-17-0085.1
Li, Q., Webb, A., Fox-Kemper, B., Craig, A., Danabasoglu, G., Large, W. G., et al. (2016). Langmuir mixing effects on global climate: WAVEWATCH III in CESM. Ocean Modell. 103, 145–160. doi: 10.1016/j.ocemod.2015.07.020
Li, X.-M., Lehner, S., and Bruns, T. (2011). Ocean wave integral parameter measurements using Envisat ASAR wave mode data. IEEE Trans. Geosci. Rem. Sens. 49, 155–174. doi: 10.1109/TGRS.2010.2052364
Liang, J.-H., Deutsch, C., McWilliams, J. C., Baschek, B., Sullivan, P. P., and Chiba, D. (2013). Parameterizing bubble-mediated air-sea gas exchange and its effect on ocean ventilation. Glob. Biogeochem. Cycles 27, 894–905. doi: 10.1002/gbc.20080
Liu, T. W., Xie, X., and Niiler, P. P. (2007). Ocean–atmosphere interaction over Agulhas Extension meanders. J. Clim. 20, 5784–5797. doi: 10.1175/2007JCLI1732.1
Loder, J. W., Brickman, D., and Horne, E. P. W. (1992). Detailed structure of currents and hydrography on the northern side of Georges Bank. J. Geophys. Res. 97:14331. doi: 10.1029/92JC01342
Loder, J. W., and Wright, D. G. (1985). Tidal rectification and frontal circulation on the sides of Georges Bank. J. Mar. Res. 43, 581–604. doi: 10.1357/002224085788440367
Longuet-Higgins, M. S., Cartwright, D. E., and Smith, N. D. (1963). “Observations of the directional spectrum of sea waves using the motions of a floating buoy,” in Ocean Wave Spectra, Proceedings of a Conference, Easton, Maryland (Upper Saddle River, NJ: National Academy of Sciences, Prentice-Hall), 111–136.
Lough, R. G., and Manning, J. P. (2001). Tidal-front entrainment and retention of fish larvae on the southern flank of Georges Bank. Deep-Sea Res. 48, 631–644. doi: 10.1016/S0967-0645(00)00130-2
Lumpkin, R., and Johnson, G. C. (2013). Global ocean surface velocities from drifters: mean, variance, El Niño–southern oscillation response, and seasonal cycle. J. Geophys. Res. Oceans 118, 2992–3006. doi: 10.1002/jgrc.20210
Lumpkin, R., Özgökmen, T., and Centurioni, L. (2017). Advances in the application of surface drifters. Annu. Rev. Mar. Sci. 9, 59–81. doi: 10.1146/annurev-marine-010816-060641
Lumpkin, R., and Pazos, M. (2007). “Measuring surface currents with surface velocity program drifters: the instrument, its data, and some recent results,” in Lagrangian Analysis and Prediction of Coastal and Ocean Dynamics, eds A. Griffa, A. D. Kirwan, A. J. Mariano, T. Özgökmen, and H. T. Rossby (Cambridge, UK: Cambridge University Press), 39–67.
Luo, H., Bracco, A., Cardona, Y., and McWilliams, J. C. (2016). Submesoscale circulation in the northern Gulf of Mexico: surface processes and the impact of the freshwater river input. Ocean Modell. 101, 68–82. doi: 10.1016/j.ocemod.2016.03.003
Lygre, A., and Krogstad, H. E. (1986). Maximum entropy estimation of the directional distribution in ocean wave spectra. J. Phys. Oceanogr. 16, 2052–2060. doi: 10.1175/1520-0485(1986)016<2052:MEEOTD>2.0.CO;2
MacKinnon, J., St Laurent, L., and Naveira Garabato, A. C. (2013). Diapycnal mixing processes in the ocean interior. Int. Geophys. 103, 159–183. doi: 10.1016/B978-0-12-391851-2.00007-6
MacKinnon, J. A., Zhao, Z., Whalen, C. B., Waterhouse, A. F., Trossman, D. S., Sun, O. M., et al. (2017). Climate process team on internal wave–driven ocean mixing. Bull. Am. Meteorol. Soc. 98, 2429–2454. doi: 10.1175/BAMS-D-16-0030.1
Makin, V. K. (2008). On the possible impact of a following-swell on the atmospheric boundary layer. Bound. Layer Meteorol. 129, 469–478. doi: 10.1007/s10546-008-9320-z
Marcq, S., and Weiss, J. (2012). Influence of sea ice lead-width distribution on turbulent heat transfer between the ocean and the atmosphere. Cryosphere 6, 143–156. doi: 10.5194/tc-6-143-2012
Martin, A. C., Gommenginger, C., Marquez, J., Doody, S., Navarro, V., and Buck, C. (2016). Wind-wave-induced velocity in ATI SAR ocean surface currents: first experimental evidence from an airborne campaign. J. Geophys. Res. Oceans 121, 1640–1653. doi: 10.1002/2015JC011459
Matano, R. P., Palma, E. D., and Piola, A. R. (2010). The influence of the Brazil and Malvinas Currents on the Southwestern Atlantic shelf circulation. Ocean Sci. 6, 983–995. doi: 10.5194/os-6-983-2010
Maximenko, N., Hafner, J., and Niiler, P. (2012). Pathways of marine debris derived from trajectories of Lagrangian drifters. Mar. Pollut. Bull. 65, 51–62. doi: 10.1016/j.marpolbul.2011.04.016
Maximenko, N., Niiler, P., Centurioni, L., Rio, M.-H., Melnichenko, O., Chambers, D., et al. (2009). Mean dynamic topography of the ocean derived from satellite and drifting buoy data using three different techniques. J. Atmos. Ocean. Technol. 26, 1910–1919. doi: 10.1175/2009JTECHO672.1
McPhee-Shaw, E., Siegel, D., Washburn, L., Brzezinski, M., Jones, J., Leydecker, A., et al. (2007). Mechanisms for nutrient delivery to the inner shelf: observations from the Santa Barbara Channel. Limnol. Oceanogr. 52, 1748–1766. doi: 10.4319/lo.2007.52.5.1748
McWilliams, J. C. (1985). Submesoscale, coherent vortices in the ocean. Rev. Geophys. 23, 165–182. doi: 10.1029/RG023i002p00165
McWilliams, J. C. (2016). Submesoscale currents in the ocean. Proc. R. Soc. A 472:20160117. doi: 10.1098/rspa.2016.0117
McWilliams, J. C. (2017). Submesoscale surface fronts and filaments: secondary circulation, buoyancy flux, and frontogenesis. J. Fluid Mech. 823, 391–432. doi: 10.1017/jfm.2017.294
McWilliams, J. C., and Fox-Kemper, B. (2013). Oceanic wave-balanced surface fronts and filaments. J. Fluid Mech. 730, 464–490. doi: 10.1017/jfm.2013.348
McWilliams, J. C., Huckle, E., Liang, J., and Sullivan, P. P. (2014). Langmuir turbulence in swell. J. Phys. Oceanogr. 44, 870–890. doi: 10.1175/JPO-D-13-0122.1
McWilliams, J. C., and Sullivan, P. P. (2000). Vertical mixing by Langmuir circulations. Spill Sci. Technol. Bull. 6, 225–237. doi: 10.1016/S1353-2561(01)00041-X
McWilliams, J. C., Sullivan, P. P., and Moeng, C.-H. (1997). Langmuir turbulence in the ocean. J. Fluid Mech. 334, 1–30. doi: 10.1017/S0022112096004375
Meissner, T., and Wentz, F. (2005). “Ocean retrievals for WindSat: radiative transfer model, algorithm, validation,” in OCEANS, 2005. Proceedings of MTS/IEEE (Seoul: IEEE), 130–133.
Meissner, T., and Wentz, F. J. (2009). Wind-vector retrievals under rain with passive satellite microwave radiometers. IEEE Trans. Geosci. Rem. Sens. 47, 3065–3083. doi: 10.1109/TGRS.2009.2027012
Melville, W. K., and Rapp, R. J. (1985). Momentum flux in breaking waves. Nature 317, 514–516. doi: 10.1038/317514a0
Mensa, J. A., Garraffo, Z., Griffa, A., Özgökmen, T. M., Haza, A., and Veneziani, M. (2013). Seasonality of the submesoscale dynamics in the Gulf Stream region. Ocean Dyn. 63, 923–941. doi: 10.1007/s10236-013-0633-1
Miller, C. B., Lynch, D. R., Carlotti, F., Gentleman, W., and Lewis, C. V. W. (1998). Coupling of an individual-based population dynamic model of Calanus finmarchicus to a circulation model for the Georges Bank region. Fish. Oceanogr. 7, 219–234. doi: 10.1046/j.1365-2419.1998.00072.x
Minobe, S., Kuwano-Yoshida, A., Komori, N., Xie, S.-P., and Small, R. (2008). Influence of the Gulf Stream on the troposphere. Nature 452, 206–209. doi: 10.1038/nature06690
Minobe, S., Miyashita, M., Kuwano-Yoshida, A., Tokinaga, H., and Xie, S.-P. (2010). Atmospheric response to the Gulf Stream: seasonal variations. J. Clim. 23, 3699–3719. doi: 10.1175/2010JCLI3359.1
Monteiro, I. T., Santos, A. J., Belo-Pereira, M., and Oliveira, P. B. (2016). Adjustment of the summertime marine atmospheric boundary layer to the western Iberia coastal morphology. J. Geophys. Res. Atmos. 121, 3875–3893. doi: 10.1002/2016JD025055
Morrow, R., Fu, L.-L., Ardhuin, F., Benkiran, M., Chapron, B., Cosme, E., et al. (2019). Global observations of fine-scale ocean surface topography with the Surface Water and Ocean Topography (SWOT) mission. Front. Mar. Sci. 6:232. doi: 10.3389/fmars.2019.00232
Mouche, A., and Chapron, B. (2015). Global C-band Envisat, RADARSAT-2 and Sentinel-1 SAR measurements in co-polarization and cross-polarization. J. Geophys. Res. Oceans 120, 7195–7207. doi: 10.1002/2015JC011149
Mouche, A., Chapron, B., Reul, N., and Collard, F. (2008). Predicted Doppler shifts induced by ocean surface wave displacements using asymptotic electromagnetic wave scattering theories. Waves Random Complex Media 18, 185–196. doi: 10.1080/17455030701564644
Mouche, A., Chapron, B., Zhang, B., and Husson, R. (2017). Combined co- and cross-polarized SAR measurements under extreme wind conditions. IEEE Trans. Geosci. Rem. Sens. 55, 6476–6755. doi: 10.1109/TGRS.2017.2732508
Mouche, A., Collard, F., Chapron, B., Dagestad, K.-F., Guitton, G., Johannessen, J. A., et al. (2012). On the use of Doppler shift for sea surface wind retrieval from SAR. IEEE Trans. Geosci. Rem. Sens. 50, 2901–2909. doi: 10.1109/TGRS.2011.2174998
Mrvaljevic, R. K., Black, P. G., Centurioni, L. R., Chang, Y.-T., D'Asaro, E. A., Jayne, S. R., et al. (2013). Observations of the cold wake of Typhoon Fanapi (2010). Geophys. Res. Lett. 40, 316–321. doi: 10.1029/2012GL054282
Muckenhuber, S., and Sandven, S. (2017). Open-source sea ice drift algorithm for Sentinel-1 SAR imagery using a combination of feature tracking and pattern matching. Cryosphere 11, 1835–1850. doi: 10.5194/tc-11-1835-2017
Münchow, A., and Garvine, R. W. (1993). Buoyancy and wind forcing of a coastal current. J. Mar. Res. 51, 293–322. doi: 10.1357/0022240933223747
Nicholls, R. J., and Cazenave, A. (2010). Sea-level rise and its impact on coastal zones. Science 328, 1517–1520. doi: 10.1126/science.1185782
Notz, D., and Marotzke, J. (2012). Observations reveal external driver for Arctic sea-ice retreat. Geophys. Res. Lett. 39:L08502. doi: 10.1029/2012GL051094
Nouguier, F., Chapron, B., Collard, F., Mouche, A., Rascle, N., Ardhuin, F., et al. (2018). Sea surface kinematics from near-nadir radar measurements. IEEE Trans. Geosci. Rem. Sens. 56, 6169–6179. doi: 10.1109/TGRS.2018.2833200
Nouguier, F., Mouche, A., Rascle, N., Chapron, B., and Vandemark, D. C. (2016). Analysis of dual-frequency ocean backscatter measurements at Ku-and Ka-bands using near-nadir incidence GPM radar data. IEEE Geosci. Rem. Sens. Lett. 13, 1310–1314. doi: 10.1109/LGRS.2016.2583198
Nurser, A., and Bacon, S. (2014). The Rossby radius in the Arctic Ocean. Ocean Sci. 10, 967–975. doi: 10.5194/os-10-967-2014
Nuss, W. A., Bane, J. M., Thompson, W. T., Holt, T., Dorman, C. E., Ralph, F. M., et al. (2000). Coastally trapped wind reversals: progress toward understanding. Bull. Am. Meteorol. Soc. 81, 719–743. doi: 10.1175/1520-0477(2000)081<0719:CTWRPT>2.3.CO;2
Ohlmann, J. C., Fewings, M. R., and Melton, C. (2012). Lagrangian observations of inner-shelf motions in Southern California: can surface waves decelerate shoreward-moving drifters just outside the surf zone? J. Phys. Oceanogr. 42, 1313–1326. doi: 10.1175/JPO-D-11-0142.1
Oikkonen, A., Haapala, J., Lensu, M., Karvonen, J., and Itkin, P. (2017). Small-scale sea ice deformation during N-ICE2015: from compact pack ice to marginal ice zone. J. Geophys. Res. Oceans 122, 5105–5120. doi: 10.1002/2016JC012387
O'Neill, L., Chelton, D., and Esbensen, S. (2012). Covariability of surface wind and stress responses to sea surface temperature fronts. J. Clim. 25, 5916–5942. doi: 10.1175/JCLI-D-11-00230.1
O'Neill, L. W. (2012). Wind speed and stability effects on coupling between surface wind stress and SST observed from buoys and satellite. J. Clim. 25, 1544–1569. doi: 10.1175/JCLI-D-11-00121.1
O'Neill, L. W., Chelton, D. B., and Esbensen, S. K. (2003). Observations of SST-induced perturbations of the wind stress field over the Southern Ocean on seasonal timescales. J. Clim. 16, 2340–2354. doi: 10.1175/2780.1
O'Neill, L. W., Chelton, D. B., and Esbensen, S. K. (2010). The effects of SST-induced surface wind speed and direction gradients on midlatitude surface vorticity and divergence. J. Clim. 23, 255–281. doi: 10.1175/2009JCLI2613.1
O'Neill, L. W., Chelton, D. B., Esbensen, S. K., and Wentz, F. J. (2005). High-resolution satellite measurements of the atmospheric boundary layer response to SST variations along the Agulhas Return Current. J. Clim. 18, 2706–2723. doi: 10.1175/JCLI3415.1
Pacanowski, R. (1987). Effect of equatorial currents on surface stress. J. Phys. Oceanogr. 17, 833–838. doi: 10.1175/1520-0485(1987)017<0833:EOECOS>2.0.CO;2
Paduan, J. D., and Washburn, L. (2013). High-frequency radar observations of ocean surface currents. Annu. Rev. Mar. Sci. 5, 115–136. doi: 10.1146/annurev-marine-121211-172315
Painter, S. C., Hartman, S. E., Kivimäe, C., Salt, L. A., Clargo, N. M., Bozec, Y., et al. (2016). Carbon exchange between a shelf sea and the ocean: the Hebrides Shelf, west of Scotland. J. Geophys. Res. Oceans 121, 4522–4544. doi: 10.1002/2015JC011599
Pazan, S. E., and Niiler, P. P. (2001). Recovery of near-surface velocity from undrogued drifters. J. Atmos. Ocean. Technol. 18, 476–489. doi: 10.1175/1520-0426(2001)018<0476:RONSVF>2.0.CO;2
Peacock, N. R., and Laxon, S. W. (2004). Sea surface height determination in the Arctic Ocean from ERS altimetry. J. Geophys. Res. Oceans 109:C07001. doi: 10.1029/2001JC001026
Peral, E., Rodríguez, E., and Esteban-Fernández, D. (2015). Impact of surface waves on SWOT's projected ocean accuracy. Rem. Sens. 7, 14509–14529. doi: 10.3390/rs71114509
Perlin, N., Skyllingstad, E. D., and Samelson, R. M. (2011). Coastal atmospheric circulation around an idealized cape during wind-driven upwelling studied from a coupled ocean–atmosphere model. Monthly Weather Rev. 139, 809–829. doi: 10.1175/2010MWR3372.1
Phillips, O. (1984). On the response of short ocean wave components at a fixed wavenumber to ocean current variations. J. Phys. Oceanogr. 14, 1425–1433. doi: 10.1175/1520-0485(1984)014<1425:OTROSO>2.0.CO;2
Pineau-Guillou, L., Ardhuin, F., Bouin, M.-N., Redelsperger, J.-L., Chapron, B., Bidlot, J.-R., et al. (2018). Strong winds in a coupled wave–atmosphere model during a North Atlantic storm event: evaluation against observations. Q. J. Royal Meteorol. Soc. 144, 317–332. doi: 10.1002/qj.3205
Piñones, A., Castilla, J. C., Guiñez, R., and Largier, J. L. (2007). Nearshore surface temperatures in Antofagasta Bay (Chile) and adjacent upwelling centers. Cien. Mar. 33, 37–48. doi: 10.7773/cm.v33i1.1226
Piola, A. R., Matano, R. P., Palma, E. D., Möller, O. O. Jr., and Campos, E. J. (2005). The influence of the Plata river discharge on the western South Atlantic shelf. Geophys. Res. Lett. 32:L01603. doi: 10.1029/2004GL021638
Plueddemann, A., and Farrar, J. (2006). Observations and models of the energy flux from the wind to mixed-layer inertial currents. Deep Sea Res. 53, 5–30. doi: 10.1016/j.dsr2.2005.10.017
Pollard, R. (1973). Interpretation of near-surface current meter observations. Deep Sea Res. Oceanogr. Abstr. 20, 261–268. doi: 10.1016/0011-7471(73)90015-6
Poulain, P.-M., Gerin, R., Mauri, E., and Pennel, R. (2009). Wind effects on drogued and undrogued drifters in the eastern Mediterranean. J. Atmos. Ocean. Technol. 26, 1144–1156. doi: 10.1175/2008JTECHO618.1
Price, J. F. (1981). Upper ocean response to a hurricane. J. Phys. Oceanogr. 11, 153–175. doi: 10.1575/1912/10271
Pringle, J. M. (2018). Remote forcing of shelf flows by density gradients and the origin of the annual mean flow on the Mid-Atlantic Bight. J. Geophys. Res. Oceans 123, 4464–4482. doi: 10.1029/2017JC013721
Pringle, J. M., and Dever, E. P. (2009). Dynamics of wind-driven upwelling and relaxation between Monterey Bay and Point Arena: local-, regional-, and gyre-scale controls. J. Geophys. Res. Oceans 114. doi: 10.1029/2008JC005016
Pujol, M.-I., Schaeffer, P., Faugère, Y., Raynal, M., Dibarboure, G., and Picot, N. (2018). Gauging the improvement of recent mean sea surface models: a new approach for identifying and quantifying their errors. J. Geophys. Res. Oceans 123, 5889–5911. doi: 10.1029/2017JC013503
Qiu, B., Chen, S., Klein, P., Sasaki, H., and Sasai, Y. (2014). Seasonal mesoscale and submesoscale eddy variability along the North Pacific subtropical countercurrent. J. Phys. Oceanogr. 44, 3079–3098. doi: 10.1175/JPO-D-14-0071.1
Quilfen, Y., Chapron, B., Elfouhaily, T., Katsaros, K., and Tournadre, J. (1998). Observation of tropical cyclones by high-resolution scatterometry. J. Geophys. Res. Oceans 103, 7767–7786. doi: 10.1029/97JC01911
Quilfen, Y., Yurovskaya, M., Chapron, B., and Ardhuin, F. (2018). Storm waves focusing and steepening in the Agulhas current: Satellite observations and modeling. Rem. Sens. Environ. 216, 561–571. doi: 10.1016/j.rse.2018.07.020
Ràfols, L., Grifoll, M., Jordà, G., Espino, M., Sairouní, A., and Bravo, M. (2017). Shelf circulation induced by an orographic wind jet. J. Geophys. Res. Oceans 122, 8225–8245. doi: 10.1002/2017JC012773
Rahn, D. A., Parish, T. R., and Leon, D. (2013). Airborne measurements of coastal jet transition around Point Conception, California. Monthly Weather Rev. 141, 3827–3839. doi: 10.1175/MWR-D-13-00030.1
Rampal, P., Weiss, J., Dubois, C., and Campin, J.-M. (2011). IPCC climate models do not capture Arctic sea ice drift acceleration: consequences in terms of projected sea ice thinning and decline. J. Geophys. Res. Oceans 116:C00D07. doi: 10.1029/2011JC007110
Rascle, N., Molemaker, J., Marié, L., Nouguier, F., Chapron, B., Lund, B., et al. (2017). Intense deformation field at oceanic front inferred from directional sea surface roughness observations. Geophys. Res. Lett. 44, 5599–5608. doi: 10.1002/2017GL073473
Rascle, N., Nouguier, F., Chapron, B., Mouche, A., and Ponte, A. (2016). Surface roughness changes by finescale current gradients: properties at multiple azimuth view angles. J. Phys. Oceanogr. 46, 3681–3694. doi: 10.1175/JPO-D-15-0141.1
Regnier, P., Friedlingstein, P., Ciais, P., Mackenzie, F. T., Gruber, N., Janssens, I. A., et al. (2013). Anthropogenic perturbation of the carbon fluxes from land to ocean. Nat. Geosci. 6:597. doi: 10.1038/ngeo1830
Renault, L., Deutsch, C., McWilliams, J. C., Frenzel, H., Liang, J.-H., and Colas, F. (2016a). Partial decoupling of primary productivity from upwelling in the California current system. Nat. Geosci. 9, 505–508. doi: 10.1038/ngeo2722
Renault, L., McWilliams, J. C., and Gula, J. (2018). Dampening of submesoscale currents by air-sea stress coupling in the Californian upwelling system. Sci. Rep. 8:13388. doi: 10.1038/s41598-018-31602-3
Renault, L., McWilliams, J. C., and Masson, S. (2017a). Satellite observations of imprint of oceanic current on wind stress by air-sea coupling. Sci. Rep. 7:17747. doi: 10.1038/s41598-017-17939-1
Renault, L., McWilliams, J. C., and Penven, P. (2017b). Modulation of the Agulhas current retroflection and leakage by oceanic current interaction with the atmosphere in coupled simulations. J. Phys. Oceanogr. e0168.1. doi: 10.1175/JPO-D-16-0168.1
Renault, L., Molemaker, J., Gula, J., Masson, S., and McWilliams, J. (2016b). Control and stabilization of the Gulf stream by oceanic current interaction with the atmosphere. J. Phys. Oceanogr. 6:e0115.1. doi: 10.1175/JPO-D-16-0115.1
Renault, L., Molemaker, J., McWilliams, J., Shchepetkin, A., Lemarie, F., Chelton, D., et al. (2016c). Modulation of wind work by oceanic current interaction with the atmosphere. J. Phys. Oceanogr. 46, 1685–1704. doi: 10.1175/JPO-D-15-0232.1
Rennie, S. E., Largier, J. L., and Lentz, S. J. (1999). Observations of a pulsed buoyancy current downstream of Chesapeake Bay. J. Geophys. Res. 104, 18227–18240. doi: 10.1029/1999JC900153
Ricker, R., Girard-Ardhuin, F., Krumpen, T., and Lique, C. (2018). Satellite-derived sea ice export and its impact on Arctic ice mass balance. Cryosphere 12, 3017–3032. doi: 10.5194/tc-12-3017-2018
Rio, M.-H., and Hernandez, F. (2003). High-frequency response of wind-driven currents measured by drifting buoys and altimetry over the world ocean. J. Geophys. Res. Oceans 108:NO. C8, 3283. doi: 10.1029/2002JC001655
Rio, M.-H., Mulet, S., and Picot, N. (2014). Beyond GOCE for the ocean circulation estimate: synergetic use of altimetry, gravimetry, and in situ data provides new insight into geostrophic and Ekman currents. Geophys. Res. Lett. 41, 8918–8925. doi: 10.1002/2014GL061773
Rio, M.-H., and Santoleri, R. (2018). Improved global surface currents from the merging of altimetry and sea surface temperature data. Rem. Sens. Environ. 216, 770–785. doi: 10.1016/j.rse.2018.06.003
Rio, M.-H., Santoleri, R., Bourdalle-Badie, R., Griffa, A., Piterbarg, L., and Taburet, G. (2016). Improving the altimeter-derived surface currents using high-resolution sea surface temperature data: a feasability study based on model outputs. J. Atmos. Ocean. Technol. 33, 2769–2784. doi: 10.1175/JTECH-D-16-0017.1
Rippeth, T. P., Lincoln, B. J., Lenn, Y.-D., Green, J. M., Sundfjord, A., and Bacon, S. (2015). Tide-mediated warming of Arctic halocline by Atlantic heat fluxes over rough topography. Nat. Geosci. 8:191. doi: 10.1038/ngeo2350
Risien, C. M., and Strub, P. T. (2016). Blended sea level anomaly fields with enhanced coastal coverage along the U.S. West Coast. Sci. Data 3:160013. doi: 10.1038/sdata.2016.13
Roarty, H., Cook, T., Hazard, L., George, D., Harlan, J., Cosoli, S., et al. (2019). The global high frequency radar network. Front. Mar. Sci. 6:164. doi: 10.3389/fmars.2019.00164
Rodriguez, E. (2018). On the optimal design of doppler scatterometers. Rem. Sens. 10:1765. doi: 10.3390/rs10111765
Rodriguez, E. (2019). The winds and currents mission concept. Front. Mar. Sci. 438. doi: 10.3389/fmars.2019.00438
Rodriguez, E., Wineteer, A., Perkovic-Martin, D., Gal, T., Stiles, B., Niamsuwan, N., et al. (2018). Estimating ocean vector winds and currents using a Ka-band pencil-beam Doppler scatterometer. Rem. Sens. 10:576. doi: 10.3390/rs10040576
Rodriguez, E., and Wineteer, A. G. (2018). “Observations of submesoscale surface currents and winds with a Doppler scatterometer,” in AGU Fall Meeting Abstracts (Washington, DC).
Rogerson, A. (1999). Transcritical flows in the coastal marine atmospheric boundary layer. J. Atmos. Sci. 56, 2761–2779. doi: 10.1175/1520-0469(1999)056<2761:TFITCM>2.0.CO;2
Romeiser, R. (2013). The future of SAR-based oceanography: high-resolution current measurements by along-track interferometry. Oceanography 26, 92–99. doi: 10.5670/oceanog.2013.37
Romeiser, R., Runge, H., Suchandt, S., Kahle, R., Rossi, C., and Bell, P. S. (2014). Quality assessment of surface current fields from TerraSAR-X and TanDEM-X along-track interferometry and Doppler centroid analysis. IEEE Trans. Geosci. Rem. Sens. 52, 2759–2772. doi: 10.1109/TGRS.2013.2265659
Romero, L., Lenain, L., and Melville, W. K. (2017). Observations of surface wave–current interaction. J. Phys. Oceanogr. 47, 615–632. doi: 10.1175/JPO-D-16-0108.1
Romero, L., and Melville, W. K. (2010). Airborne observations of fetch-limited waves in the gulf of tehuantepec. J. Phys. Oceanogr. 40, 441–465. doi: 10.1175/2009JPO4127.1
Roth, M. K., MacMahan, J., Reniers, A., Özgökmen, T. M., Woodall, K., and Haus, B. (2017). Observations of inner shelf cross-shore surface material transport adjacent to a coastal inlet in the northern Gulf of Mexico. Cont. Shelf Res. 137, 142–153. doi: 10.1016/j.csr.2016.12.017
Roughan, M. (2005a). Observations of divergence and upwelling around Point Loma, California. J. Geophys. Res. 110:1899. doi: 10.1029/2004JC002662
Roughan, M. (2005b). Subsurface recirculation and larval retention in the lee of a small headland: a variation on the upwelling shadow theme. J. Geophys. Res. 110:161. doi: 10.1029/2005JC002898
Ruf, C. S., Atlas, R., Chang, P. S., Clarizia, M. P., Garrison, J. L., Gleason, S., et al. (2016). New ocean winds satellite mission to probe hurricanes and tropical convection. Bull. Am. Meteorol. Soc. 97, 385–395. doi: 10.1175/BAMS-D-14-00218.1
Ryan, J., Davis, C., Tufillaro, N., Kudela, R., and Gao, B.-C. (2014). Application of the hyperspectral imager for the coastal ocean to phytoplankton ecology studies in Monterey Bay, CA, USA. Rem. Sens. 6, 1007–1025. doi: 10.3390/rs6021007
Ryan, J. P., Gower, J. F. R., King, S. A., Bissett, W. P., Fischer, A. M., Kudela, R. M., et al. (2008). A coastal ocean extreme bloom incubator. Geophys. Res. Lett. 35:L12602. doi: 10.1029/2008GL034081
Sabine, C. L., Feely, R. A., Gruber, N., Key, R. M., Lee, K., Bullister, J. L., et al. (2004). The oceanic sink for anthropogenic CO2. Science 305, 367–371. doi: 10.1126/science.1097403
Samelson, R. M. (2019). Challenges and opportunities in coastal prediction. EOS 100. doi: 10.1029/2019EO113841
Schlitzer, R. (2002). Carbon export fluxes in the Southern Ocean: results from inverse modeling and comparison with satellite-based estimates. Deep Sea Res. 49, 1623–1644. doi: 10.1016/S0967-0645(02)00004-8
Schlundt, M., Brandt, P., Dengler, M., Hummels, R., Fischer, T., Bumke, K., et al. (2014). Mixed layer heat and salinity budgets during the onset of the 2011 Atlantic cold tongue. J. Geophys. Res. Oceans 119, 7882–7910. doi: 10.1002/2014JC010021
Scott, R. B., and Xu, Y. (2009). An update on the wind power input to the surface geostrophic flow of the World Ocean. Deep Sea Res. 56, 295–304. doi: 10.1016/j.dsr.2008.09.010
Sepulveda, H. H., Queffeulou, P., and Ardhuin, F. (2015). Assessment of SARAL/AltiKa wave height measurements relative to buoy, Jason-2, and Cryosat-2 data. Mar. Geod. 38, 449–465. doi: 10.1080/01490419.2014.1000470
Shakespeare, C. J., and Taylor, J. (2013). A generalized mathematical model of geostrophic adjustment and frontogenesis: uniform potential vorticity. J. Fluid Mech. 736, 366–413. doi: 10.1017/jfm.2013.526
Shearman, K. R., and Lentz, S. J. (2010). Long-term sea surface temperature variability along the U.S. East Coast. J. Phys. Oceanogr. 40, 1004–1017. doi: 10.1175/2009JPO4300.1
Shimura, T., Mori, N., Takemi, T., and Mizuta, R. (2017). Long-term impacts of ocean wave-dependent roughness on global climate systems. J. Geophys. Res. Oceans 122, 1995–2011. doi: 10.1002/2016JC012621
Siedlecki, S. A., Banas, N. S., Davis, K. A., Giddings, S., Hickey, B. M., MacCready, P., et al. (2015). Seasonal and interannual oxygen variability on the Washington and Oregon continental shelves. J. Geophys. Res. Oceans 120, 608–633. doi: 10.1002/2014JC010254
Siedlecki, S. A., Kaplan, I. C., Hermann, A. J., Nguyen, T. T., Bond, N. A., Newton, J. A., et al. (2016). Experiments with seasonal forecasts of ocean conditions for the northern region of the California Current upwelling system. Sci. Rep. 6:27203. doi: 10.1038/srep27203
Simmons, H. L., and Alford, M. H. (2012). Simulating the long-range swell of internal waves generated by ocean storms. Oceanography 25, 30–41. doi: 10.5670/oceanog.2012.39
Simons, R. D., Siegel, D. A., and Brown, K. S. (2013). Model sensitivity and robustness in the estimation of larval transport: a study of particle tracking parameters. J. Mar. Syst. 119–120, 19–29. doi: 10.1016/j.jmarsys.2013.03.004
Small, R., Xie, S., O'Neill, L., Seo, H., Song, Q., Cornillon, P., et al. (2008). Air–sea interaction over ocean fronts and eddies. Dyn. Atmos. Oceans 45, 274–319. doi: 10.1016/j.dynatmoce.2008.01.001
Smyth, W., Moum, J., Li, L., and Thorpe, S. (2013). Diurnal shear instability, the descent of the surface shear layer, and the deep cycle of equatorial turbulence. J. Phys. Oceanogr. 43, 2432–2455. doi: 10.1175/JPO-D-13-089.1
Soloviev, Y. P., and Kudryavtsev, V. (2010). Wind-speed undulations over swell: field experiment and interpretation. Bound. Layer Meteorol. 136, 341–363. doi: 10.1007/s10546-010-9506-z
Squire, V. A. (2018). A fresh look at how ocean waves and sea ice interact. Phil. Trans. R. Soc. A 376:20170342. doi: 10.1098/rsta.2017.0342
Srinivasan, K., McWilliams, J. C., Renault, L., Hristova, H. G., Molemaker, J., and Kessler, W. S. (2017). Topographic and mixed layer submesoscale currents in the near-surface southwestern tropical Pacific. J. Phys. Oceanogr. 47, 1221–1242. doi: 10.1175/JPO-D-16-0216.1
Stammer, D., Balmaseda, M., Heimbach, P., Köhl, A., and Weaver, A. (2016). Ocean data assimilation in support of climate applications: status and perspectives. Annu. Rev. Mar. Sci. 8, 491–518. doi: 10.1146/annurev-marine-122414-034113
Steele, M., Zhang, J., Rothrock, D., and Stern, H. (1997). The force balance of sea ice in a numerical model of the Arctic Ocean. J. Geophys. Res. Oceans 102, 21061–21079. doi: 10.1029/97JC01454
Stoffelen, A., Aaboe, S., Calvet, J.-C., Cotton, J., De Chiara, G., Saldana, J., et al. (2017). Scientific developments and the EPS-SG scatterometer. IEEE J. Select. Top. Appl. Earth Observ. Rem. Sens. 10, 2086–2097. doi: 10.1109/JSTARS.2017.2696424
Stopa, J., Ardhuin, F., Thomson, J., Smith, M. M., Kohout, A., Doble, M., et al. (2018). Wave attenuation through an Arctic marginal ice zone on 12 October 2015. 1. Measurement of wave spectra and ice features from sentinel 1A. J. Geophys. Res. Oceans 123, 3619–3634. doi: 10.1029/2018JC013791
Stroeve, J., and Notz, D. (2018). Changing state of Arctic sea ice across all seasons. Environ. Res. Lett. 13:103001. doi: 10.1088/1748-9326/aade56
Strong, C., and Rigor, I. G. (2013). Arctic marginal ice zone trending wider in summer and narrower in winter. Geophys. Res. Lett. 40, 4864–4868. doi: 10.1002/grl.50928
Suanda, S. H., Kumar, N., Miller, A. J., Di Lorenzo, E., Haas, K., Cai, D., et al. (2016). Wind relaxation and a coastal buoyant plume north of Pt. Conception, CA: observations, simulations, and scalings. J. Geophys. Res. Oceans 121, 7455–7475. doi: 10.1002/2016JC011919
Sudre, J., Maes, C., and Garçon, V. (2013). On the global estimates of geostrophic and Ekman surface currents. Limnol. Oceanogr. Fluids Environ. 3, 1–20. doi: 10.1215/21573689-2071927
Sullivan, P. P., and McWilliams, J. C. (2018). Frontogenesis and frontal arrest of a dense filament in the oceanic surface boundary layer. J. Fluid Mech. 837, 341–380. doi: 10.1017/jfm.2017.833
Sumata, H., Kwok, R., Gerdes, R., Kauker, F., and Karcher, M. (2015). Uncertainty of Arctic summer ice drift assessed by high-resolution SAR data. J. Geophys. Res. Oceans 120, 5285–5301. doi: 10.1002/2015JC010810
Sutherland, P., and Dumont, D. (2018). Marginal ice zone thickness and extent due to wave radiation stress. J. Phys. Oceanogr. 48, 1885–1901. doi: 10.1175/JPO-D-17-0167.1
Suzuki, N., and Fox-Kemper, B. (2016). Understanding stokes forces in the wave-averaged equations. J. Geophys. Res. 121, 3579–3596. doi: 10.1002/2015JC011566
Suzuki, N., Fox-Kemper, B., Hamlington, P. E., and Van Roekel, L. P. (2016). Surface waves affect frontogenesis. J. Geophys. Res. Oceans 121, 3597–3624. doi: 10.1002/2015JC011563
Swenson, M. S., and Hansen, D. V. (1999). Tropical Pacific Ocean mixed layer heat budget: the Pacific cold tongue. J. Phys. Oceanogr. 29, 69–81. doi: 10.1175/1520-0485(1999)029<0069:TPOMLH>2.0.CO;2
Teinturier, S., Stegner, A., Didelle, H., and Viboud, S. (2010). Small-scale instabilities of an island wake flow in a rotating shallow-water layer. Dyn. Atmos. Oceans 49, 1–24. doi: 10.1016/j.dynatmoce.2008.10.006
Teixeira, M., and Belcher, S. (2002). On the distortion of turbulence by a progressive surface wave. J. Fluid Mech. 458, 229–267. doi: 10.1017/S0022112002007838
Terrill, E., Otero, M., Hazard, L., Conlee, D., Harlan, J., Kohut, J., et al. (2006). “Data management and real-time distribution in the HF-radar national network,” in OCEANS 2006 (Boston, MA: IEEE), 1–6.
Thomas, L. N., and Lee, C. M. (2005). Intensification of ocean fronts by down-front winds. J. Phys. Oceanogr. 35, 1086–1102. doi: 10.1175/JPO2737.1
Thomas, L. N., Taylor, J. R., D'Asaro, E. A., Lee, C. M., Klymak, J. M., and Shcherbina, A. (2016). Symmetric instability, inertial oscillations, and turbulence at the Gulf Stream front. J. Phys. Oceanogr. 46, 197–217. doi: 10.1175/JPO-D-15-0008.1
Thomas, L. N., Taylor, J. R., Ferrari, R., and Joyce, T. M. (2013). Symmetric instability in the Gulf Stream. Deep Sea Res. 91, 96–110. doi: 10.1016/j.dsr2.2013.02.025
Thompson, A. F., Lazar, A., Buckingham, C., Naveira Garabato, A. C., Damerell, G. M., and Heywood, K. J. (2016). Open-ocean submesoscale motions: a full seasonal cycle of mixed layer instabilities from gliders. J. Phys. Oceanogr. 46, 1285–1307. doi: 10.1175/JPO-D-15-0170.1
Thomson, J., R, H. A., Zippel, S., Rusch, C., and Geyer, W. (2014). Wave breaking turbulence at the offshore front of the Columbia River Plume. Geophys. Res. Lett. 41, 8987–8993. doi: 10.1002/2014GL062274
Tozuka, T., Ohishi, S., and Cronin, M. F. (2018). A metric for surface heat flux effect on horizontal sea surface temperature gradients. Clim. Dyn. 51, 547–561. doi: 10.1007/s00382-017-3940-2
Tsamados, M., Feltham, D., Petty, A., Schroeder, D., and Flocco, D. (2015). Processes controlling surface, bottom and lateral melt of Arctic sea ice in a state of the art sea ice model. Phil. Trans. R. Soc. A 373:20140167. doi: 10.1098/rsta.2014.0167
Tschudi, M., Fowler, C., Maslanik, J., Stewart, J., and Meier, W. (2016). Polar Pathfinder Daily 25 km EASE-Grid Sea Ice Motion Vectors, Version 3. Boulder, CO: National Snow and Ice Data Center Distributed Active Archive Center.
Unwin, M., Gao, S., De Vos Van Steenwijk, R., Jales, P., Maqsood, M., Gommenginger, C., et al. (2013). Development of low-cost spaceborne multi-frequency GNSS receiver for navigation and GNSS remote sensing. Int. J. Space Sci. Eng. 1, 20–50. doi: 10.1504/IJSPACESE.2013.051757
Van Sebille, E., Griffies, S. M., Abernathey, R., Adams, T. P., Berloff, P., Biastoch, A., et al. (2017). Lagrangian ocean analysis: fundamentals and practices. Ocean Modell. 121, 49–75. doi: 10.1016/j.ocemod.2017.11.008
Van Sebille, E., Wilcox, C., Lebreton, L., Maximenko, N., Hardesty, B. D., Van Franeker, J. A., et al. (2015). A global inventory of small floating plastic debris. Environ. Res. Lett. 10:124006. doi: 10.1088/1748-9326/10/12/124006
Vandemark, D., Edson, J. B., and Chapron, B. (1997). Altimeter estimation of sea surface wind stress for light to moderate winds. J. Atmos. Ocean. Technol. 14, 716–722. doi: 10.1175/1520-0426(1997)014<0716:AEOSSW>2.0.CO;2
Veeramony, J., Orzech, M. D., Edwards, K. L., Gilligan, M., Choi, J., Terrill, E., et al. (2014). Navy nearshore ocean prediction systems. Oceanography 27, 80–91. doi: 10.5670/oceanog.2014.70
Veras Guimarães, P., Ardhuin, F., Sutherland, P., Accensi, M., Hamon, M., Pérignon, Y., et al. (2018). A surface kinematics buoy (skib) for wave-current interactions studies. Ocean Sci. Discuss. 2018, 1–20. doi: 10.5194/os-2018-45
Verdy, A., and Mazloff, M. (2017). A data assimilating model for estimating Southern Ocean biogeochemistry. J. Geophys. Res. Oceans 122, 6968–6988. doi: 10.1002/2016JC012650
Vigan, X., Provost, C., and Podesta, G. (2000). Sea surface velocities from sea surface temperature image sequences: 2. Application to the Brazil-Malvinas Confluence area. J. Geophys. Res. Oceans 105, 19515–19534. doi: 10.1029/2000JC900028
Villas Bôas, A., Sato, O., Chaigneau, A., and Castelão, G. (2015). The signature of mesoscale eddies on the air-sea turbulent heat fluxes in the South Atlantic Ocean. Geophys. Res. Lett. 42, 1856–1862. doi: 10.1002/2015GL063105
Villas Bôas, A. B., Gille, S. T., Mazloff, M. R., and Cornuelle, B. D. (2017). Characterization of the deep water surface wave variability in the California Current region. J. Geophys. Res. C Oceans 122, 8753–8769. doi: 10.1002/2017JC013280
Walter, R. K., Armenta, K. J., Shearer, B., Robbins, I., and Steinbeck, J. (2018). Coastal upwelling seasonality and variability of temperature and chlorophyll in a small coastal embayment. Cont. Shelf Res. 154, 9–18. doi: 10.1016/j.csr.2018.01.002
Walter, R. K., Stastna, M., Woodson, C. B., and Monismith, S. G. (2016). Observations of nonlinear internal waves at a persistent coastal upwelling front. Cont. Shelf Res. 117, 100–117. doi: 10.1016/j.csr.2016.02.007
Wanninkhof, R. (2014). Relationship between wind speed and gas exchange over the ocean revisited. Limnol. Oceanogr. Methods 12, 351–362. doi: 10.4319/lom.2014.12.351
Warren, M., Quartly, G. D., Shutler, J., Miller, P. I., and Yoshikawa, Y. (2016). Estimation of ocean surface currents from maximum cross correlation applied to GOCI geostationary satellite remote sensing data over the Tsushima (Korea) Straits. J. Geophys. Res. Oceans 121, 6993–7009. doi: 10.1002/2016JC011814
Washburn, L., Fewings, M., Melton, C., and Gotschalk, C. (2011). The propagating response of coastal circulation due to wind relaxations along the central California coast. J. Geophys. Res. 116:C12028. doi: 10.1029/2011JC007502
Watson, A. J., Schuster, U., Bakker, D. C., Bates, N. R., Corbière, A., Gonzáez-Dávila, M., et al. (2009). Tracking the variable North Atlantic sink for atmospheric CO2. Science 326, 1391–1393. doi: 10.1126/science.1177394
Weisberg, R. H., and Weingartner, T. J. (1988). Instability waves in the equatorial Atlantic Ocean. J. Phys. Oceanogr. 18, 1641–1657. doi: 10.1175/1520-0485(1988)018<1641:IWITEA>2.0.CO;2
Weissman, D. E., King, D., and Thompson, T. W. (1979). Relationship between hurricanes surface winds and L-band radar backscatter from the sea surface. J. Appl. Meteorol. 18, 1023–1034. doi: 10.1175/1520-0450(1979)018<1023:RBHSWA>2.0.CO;2
Weller, R. A., and Davis, R. E. (1980). A vector measuring current meter. Deep Sea Res. 27, 565–581. doi: 10.1016/0198-0149(80)90041-2
Wenegrat, J. O., and McPhaden, M. J. (2015). Dynamics of the surface layer diurnal cycle in the equatorial atlantic ocean (0°, 23° w). J. Geophys. Res. Oceans 120, 563–581. doi: 10.1002/2014JC010504
Wentz, F. J., Ricciardulli, L., Rodriguez, E., Stiles, B. W., Bourassa, M. A., Long, D. G., et al. (2017). Evaluating and extending the ocean wind climate data record. IEEE J. Select. Top. Appl. Earth Observ. Rem. Sens. 10, 2165–2185. doi: 10.1109/JSTARS.2016.2643641
Winant, C. D., Dorman, C. E., Friehe, C. A., and Beardsley, R. C. (1988). The marine layer off Northern California: an example of supercritical channel flow. J. Atmos. Sci. 45, 3588–3605. doi: 10.1175/1520-0469(1988)045<3588:TMLONC>2.0.CO;2
Wishner, K. F., Outram, D. M., and Ullman, D. S. (2006). Zooplankton distributions and transport across the northeastern tidal front of Georges Bank. Deep Sea Res. 53, 2570–2596. doi: 10.1016/j.dsr2.2006.06.003
Wong, P. P., Losada, I. J., Gattuso, J. P., Hinkel, J., Khattabi, A., McInnes, K. L., et al. (2014). “Coastal systems and low-lying areas,” in Climate Change 2014: Impacts, Adaptation, and Vulnerability. Part A: Global and Sectoral Aspects. Contribution of Working Group II to the Fifth Assessment Report of the Intergovernmental Panel on Climate Change, Chapter 5 (Cambridge; New York, NY: Cambridge University Press), 361–409.
Woodson, C. B., Washburn, L., Barth, J. A., Hoover, D. J., Kirincich, A. R., McManus, M. A., et al. (2009). Northern Monterey Bay upwelling shadow front: observations of a coastally and surface-trapped buoyant plume. J. Geophys. Res. Oceans 114. doi: 10.1029/2009JC005623
Woolf, D. K., Land, P. E., Shutler, J. D., Goddijn-Murphy, L. M., and Donlon, C. J. (2016). On the calculation of air-sea fluxes of CO2 in the presence of temperature and salinity gradients. J. Geophys. Res. 121, 1229–1248. doi: 10.1002/2015JC011427
Xie, S.-P., Ishiwatari, M., Hashizume, H., and Takeuchi, K. (1998). Coupled ocean-atmospheric waves on the equatorial front. Geophys. Res. Lett. 25, 3863–3866. doi: 10.1029/1998GL900014
Xie, S. P., Liu, W. T., Liu, Q., and Nonaka, M. (2001). Far-reaching effects of the Hawaiian Islands on the Pacific Ocean-atmosphere system. Science 292, 2057–2060. doi: 10.1126/science.1059781
Yurovsky, Y., Kudryavtsev, V., Grodsky, S., and Chapron, B. (2018). Low-frequency sea surface radar Doppler echo. Rem. Sens. 10:870. doi: 10.3390/rs10060870
Zavorotny, V. U., Gleason, S., Cardellach, E., and Camps, A. (2014). Tutorial on remote sensing using GNSS bistatic radar of opportunity. IEEE Geosci. Rem. Sens. Mag. 2, 8–45. doi: 10.1109/MGRS.2014.2374220
Zhang, B., Perrie, W., and He, Y. (2011). Wind speed retrieval from RADARSAT-2 quad-polarization images using a new polarization ratio model. J. Geophys. Res. Oceans 116:C08008. doi: 10.1029/2010JC006522
Zhang, W. G., and Gawarkiewicz, G. G. (2015). Dynamics of the direct intrusion of Gulf Stream ring water onto the Mid-Atlantic Bight shelf. Geophys. Res. Lett. 42, 7687–7695. doi: 10.1002/2015GL065530
Zippel, S., and Thomson, J. (2017). Surface wave breaking over sheared currents: observations from the mouth of the Columbia River. J. Geophys. Res. Oceans 122, 3311–3328. doi: 10.1002/2016JC012498
Zuidema, P., Chang, P., Medeiros, B., Kirtman, B. P., Mechoso, R., Schneider, E. K., et al. (2016). Challenges and prospects for reducing coupled climate model SST biases in the eastern tropical Atlantic and Pacific oceans: the US CLIVAR Eastern Tropical Oceans Synthesis Working Group. Bull. Am. Meteorol. Soc. 97, 2305–2328. doi: 10.1175/BAMS-D-15-00274.1
Keywords: air-sea interactions, Doppler oceanography from space, surface waves, absolute surface velocity, ocean surface winds
Citation: Villas Bôas AB, Ardhuin F, Ayet A, Bourassa MA, Brandt P, Chapron B, Cornuelle BD, Farrar JT, Fewings MR, Fox-Kemper B, Gille ST, Gommenginger C, Heimbach P, Hell MC, Li Q, Mazloff MR, Merrifield ST, Mouche A, Rio MH, Rodriguez E, Shutler JD, Subramanian AC, Terrill EJ, Tsamados M, Ubelmann C and van Sebille E (2019) Integrated Observations of Global Surface Winds, Currents, and Waves: Requirements and Challenges for the Next Decade. Front. Mar. Sci. 6:425. doi: 10.3389/fmars.2019.00425
Received: 01 November 2018; Accepted: 05 July 2019;
Published: 24 July 2019.
Edited by:
Amos Tiereyangn Kabo-Bah, University of Energy and Natural Resources, GhanaReviewed by:
Jeffrey Carpenter, Institute of Coastal Research, Helmholtz-Zentrum Geesthacht, GermanyMariona Claret Cortes, University of Washington, United States
Copyright © 2019 Villas Bôas, Ardhuin, Ayet, Bourassa, Brandt, Chapron, Cornuelle, Farrar, Fewings, Fox-Kemper, Gille, Gommenginger, Heimbach, Hell, Li, Mazloff, Merrifield, Mouche, Rio, Rodriguez, Shutler, Subramanian, Terrill, Tsamados, Ubelmann and van Sebille. This is an open-access article distributed under the terms of the Creative Commons Attribution License (CC BY). The use, distribution or reproduction in other forums is permitted, provided the original author(s) and the copyright owner(s) are credited and that the original publication in this journal is cited, in accordance with accepted academic practice. No use, distribution or reproduction is permitted which does not comply with these terms.
*Correspondence: Ana B. Villas Bôas, YXZpbGxhc2JvYXMmI3gwMDA0MDt1Y3NkLmVkdQ==