- 1Euro-Mediterranean Center on Climate Change (CMCC) Foundation, Bologna, Italy
- 2Centre for Maritime Research and Experimentation (CMRE), La Spezia, Italy
- 3GHER, University of Liège, Liège, Belgium
- 4European Centre for Medium-Range Weather Forecasts (ECMWF), Reading, United Kingdom
- 5Météo-France, Toulouse, France
- 6Nansen Environmental and Remote Sensing Center (NERSC), Bergen, Norway
- 7ACE CRC, CLEX, IMAS, University of Tasmania (UTAS), Hobart, TAS, Australia
- 8Mercator Ocean, Ramonville-Saint-Agne, France
- 9Massachusetts Institute of Technology (MIT), Cambridge, MA, United States
- 10Department of Meteorology, University of Reading, Reading, United Kingdom
- 11National Centre for Earth Observation (NCEO), Reading, United Kingdom
- 12LEGOS, Institut de Recherche pour le Développement (IRD), Toulouse, France
- 13Met Office, Exeter, United Kingdom
- 14Department of Meteorology and Geophysics, University of Vienna, Vienna, Austria
- 15Commonwealth Scientific and Industrial Research Organisation (CSIRO), Hobart, TAS, Australia
- 16Department of Atmospheric and Oceanic Science, University of Maryland, College Park, College Park, MD, United States
- 17Environmental Numerical Research Section, Environment and Climate Change Canada, Dorval, QC, Canada
- 18Institute of Marine Sciences, National Research Council of Italy, Rome, Italy
Ocean reanalyses combine ocean models, atmospheric forcing fluxes, and observations using data assimilation to give a four-dimensional description of the ocean. Metrics assessing their reliability have improved over time, allowing reanalyses to become an important tool in climate services that provide a more complete picture of the changing ocean to end users. Besides climate monitoring and research, ocean reanalyses are used to initialize sub-seasonal to multi-annual predictions, to support observational network monitoring, and to evaluate climate model simulations. These applications demand robust uncertainty estimates and fit-for-purpose assessments, achievable through sustained advances in data assimilation and coordinated inter-comparison activities. Ocean reanalyses face specific challenges: (i) dealing with intermittent or discontinued observing networks, (ii) reproducing inter-annual variability and trends of integrated diagnostics for climate monitoring, (iii) accounting for drift and bias due, e.g., to air-sea flux or ocean mixing errors, and (iv) optimizing initialization and improving performances during periods and in regions with sparse data. Other challenges such as multi-scale data assimilation to reconcile mesoscale and large-scale variability and flow-dependent error characterization for rapidly evolving processes, are amplified in long-term reanalyses. The demand to extend reanalyses backward in time requires tackling all these challenges, especially in the emerging context of earth system reanalyses and coupled data assimilation. This mini-review aims at documenting recent advances from the ocean reanalysis community, discussing unsolved challenges that require sustained activities for maximizing the utility of ocean observations, supporting data rescue and advancing specific research and development requirements for reanalyses.
Introduction: The Usefulness of Reanalyses
Ocean and sea-ice reanalyses (ORAs, or ocean syntheses) are reconstructions of the ocean and sea-ice states using an ocean model integration constrained by atmospheric surface forcing, and ocean observations via a data assimilation method (e.g., Storto and Masina, 2016). Over the last decade, performance of ocean reanalyses has improved thanks to (a) increased model resolution (e.g., Zuo et al., 2018); (b) improved physics (e.g., Breivik et al., 2015); (c) better forcing from new atmospheric reanalyses (e.g., Dee et al., 2011); (d) more and improved quality-controlled observation data sets (e.g., Legeais et al., 2018); and (e) advances in data assimilation methods (e.g., Sakov et al., 2012; Storto et al., 2018b). This is exemplified by several works showing the increase of verification skill scores between successive versions of reanalyses (e.g., Lellouche et al., 2013, 2018; Storto et al., 2016; Zuo et al., 2017). Balmaseda et al. (2015) gave a detailed overview of the recent development of ocean reanalyses, with focus on the uncertainty level of climate signals. Ocean reanalyses have thus become an established tool for a variety of climate services and science-driven studies.
For instance, on-line tools examining multiple ocean reanalyses (e.g., the CREATE1 initiative, Potter et al., 2018) enable immediate evaluation of model simulations such as the Coupled Model Inter-comparison Project (CMIP5). Visualization tools for near real-time reanalyses permit the monitoring of climate-key diagnostics, such as heat and salinity budgets in the Tropics (Xue et al., 2017)2.
Reanalyses are superior to objective analyses since, by construction, they provide increased and multi-variate dynamical consistency (e.g., Forget, 2010; Forget et al., 2015a; Evans et al., 2017; Fukumori et al., 2018) as compared to objective analyses (i.e., statistical processing of observations without model constraints, e.g., Johnson G.C. et al., 2018). One major advantage of reanalyses is their potential usefulness as a tool for studying important climate diagnostics that are only partly or indirectly observed. Examples include deep ocean variability (Balmaseda et al., 2013b), ocean transports (Duan et al., 2016), Atlantic Meridional Overturning Circulation (AMOC, e.g., Jackson et al., 2018), sea-ice thickness and drift (Massonnet et al., 2013), deep convection, and many more.
Dynamical ocean reanalysis have shown better capabilities than their statistical counterparts to assess observables where short timescale variability, or a changing or irregular observing network have large influences. Toyoda et al. (2017) showed that an ensemble of ocean reanalysis could outperform monthly mean statistical gridded products when validating monthly means of (shallow, especially summer time) mixed layer depths derived directly from original profile data, likely due to the reanalysis ability to more accurately simulate the short temporal timescale fluctuations the profiles measured (Figure 1, top panel). Similarly, Storto et al. (2017) showed the enhanced capabilities of ocean reanalyses over gridded statistical products to capture the inter-annual variability of extra-tropical steric sea level changes, particularly in the southern ocean, where a sparse observational network combined with small temporal and spatial scale eddy activity would have a heavy influence (Figure 1, bottom panel).
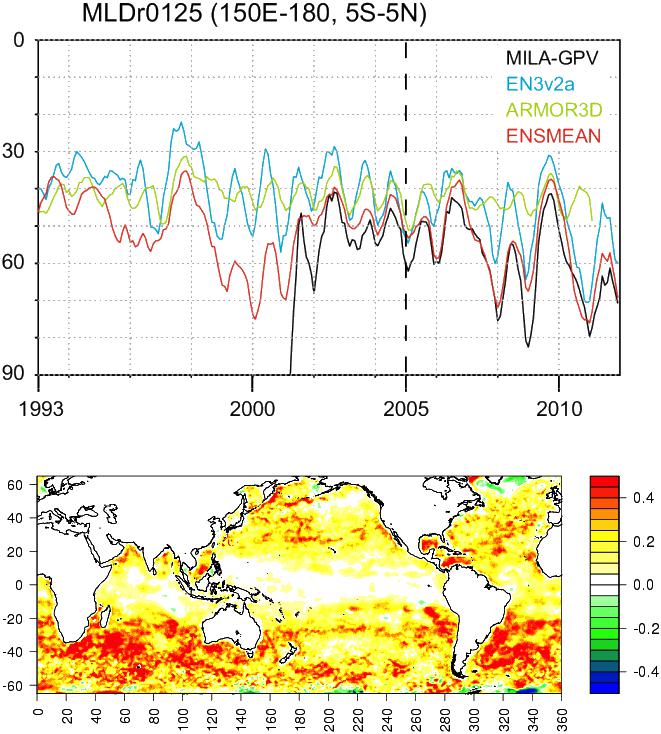
Figure 1. Examples of reanalyses outperforming objective analyses. Top panel, timeseries of mixed layer depth (in meters, using 0.125 Kg m– 3 density criterion) from profile data (MILA-GPV), the ensemble of reanalyses (REAENS) and two statistical objective analyses (EN3v2a, ARMOR3D) in the western Pacific warm pool region. Source: Toyoda et al. (2017). Bottom panel, map of the difference of steric sea level correlation (with respect to independent verifying data) between reanalysis and objective analysis ensemble means. Source: Storto et al. (2017). Image reproduced with permission of the rights holder.
Appropriateness of using reanalyses for ocean transport investigations is particularly relevant to understand large to regional scale climate changes (Zuo et al., 2011; Vidar et al., 2016). Transports are mostly unobserved, unavailable from objective analyses and poorly reproduced by free-model running simulations. Reanalyses have been shown to reproduce the AMOC variability seen in the RAPID data at 26°N (Jackson et al., 2016, 2018; Masina et al., 2017), although these data are not assimilated. Reanalyses extending back prior to the deployment of the RAPID array may indicate longer term variability, although the changing observational coverage from distinct periods may affect results. Heat and volume transports through the Indonesian Throughflow, which are not continuously observed and are relevant to ENSO monitoring, are another excellent example where ocean reanalyses successfully reproduce anomalous events (Mayer et al., 2018). Monitoring multi-annual heat, mass and freshwater transports at key Arctic ocean gateways by means of reanalyses has also successfully emerged in several recent studies (Bricaud et al., 2018; Garric et al., 2018; Uotila et al., 2018).
Reanalyses are also consistent integrators of the ocean dynamics, and there exist several studies that indicate the superiority of reanalyses with respect to model simulations and observation-only products, e.g., improving Mean Kinetic Energy at depth (1000 m) at low- and mid-latitudes (Storto et al., 2016); improving the near-surface Eddy Kinetic Energy (Masina et al., 2017), improving the statistics of the eddy population (Cipollone et al., 2017), and improving the dynamics of poorly observed regions (Koenig et al., 2017a).
Reanalyses that enforce strict budget closures such as ECCO (Estimating the Circulation and Climate of the Ocean) can be very informative for balance studies even though sometimes they may also underfit data (Forget and Ponte, 2015; Forget et al., 2015a). Reanalyses also avoid spurious high-frequency variability in global energy budgets, which is typically found in objective analyses (Trenberth et al., 2016).
In the cryosphere, sea-ice volume from the PIOMAS reanalysis fits well with independent validating data (Schweiger et al., 2011) although the PIOMAS data assimilation only ingests sea-ice concentration and sea surface temperature data. Melia et al. (2015) used the PIOMAS reanalysis to bias-correct climate model scenario predictions using both mean and interannual variability from the reanalysis, demonstrating a new use for such products.
One long-term application of ocean reanalyses has been for the initialization of ocean and sea ice components of seasonal (e.g., at Met Office, ECMWF, NCAR, GFDL, CMCC, Meteo-France, etc.; e.g., Johnson S.J. et al., 2018), and more recently decadal, retrospective forecasts (e.g., Bellucci et al., 2011). The importance of the role of the ocean (e.g., Alessandri et al., 2010; Doblas-Reyes et al., 2013) and sea ice (e.g., Guemas et al., 2016) in initialization is indeed widely accepted. Despite the growing community interest, ocean reanalyses, and the ocean component of coupled reforecasts, are underutilized, largely due to the existing terrestrial emphasis of most of the derived products of these systems. Efforts are being made, for instance by the Copernicus Climate Change Service (C3S3) and Copernicus Marine Environment Monitoring Service (CMEMS4), to release the ocean reanalysis component in a more timely manner, and to incorporate their information into climate monitoring reports (i.e., the Ocean State Report, von Schuckmann et al., 2018a, b, or the State of the Climate, Hartfield et al., 2018). A particular benefit of having the reanalysis of these systems readily available, is that this now leads to the ability to access error growth in the forecast components, leading to much better assessment of model forecast error and ultimately to bias correction and model improvement (e.g., Mulholland et al., 2016).
Global reanalyses also provide lateral boundary conditions for regional high-resolution simulations/reanalyses, which are found to significantly improve the local circulation compared to the use of, e.g., climatology (Barth et al., 2008). Additionally, reanalyses are extensively used to monitor the impact of past and present observing networks, thus helping the optimal maintenance and the future design of the global ocean observation system (e.g., Fujii et al., 2019; Gasparin et al., 2019).
A relatively new application of reanalyses is the investigation of climate variability over centennial timescales by extending back throughout the 20th century and even further. Such historical ocean reanalyses (Giese et al., 2016; de Boisséson et al., 2017; Yang et al., 2017) can particularly provide initial conditions for more extensive decadal predictions. However, caution must be used with “20th century” ocean reanalyses because of the spurious variability resulting from the inhomogeneous observations, especially for ocean transport diagnostics (Yang et al., 2017). Historical ocean reanalyses rely on historical atmospheric reanalyses also extending back to the 19th century (e.g., 20CR, Compo et al., 2011), making the emerging field of historical coupled reanalyses (Laloyaux et al., 2018) doubly attractive to make use of the sparse observations.
Assessing the Uncertainty of Ocean Reanalyses
The quality of ocean reanalysis relies upon the accuracy of ocean models, forcing products, and observational data accuracy, namely both data and model errors contribute to the reanalysis uncertainty, as in any other data assimilation context. Model and forcing errors are reduced through assimilation of satellite and in situ data, and the availability of accurate observations, with reliable uncertainty estimates associated, is therefore crucial.
Unfortunately, the first satellite missions sensing the ocean surface (sea surface temperature and sea-ice concentration) are available only after 1979; high-precision altimetry missions sampling the sea surface height only began in 1992; in situ surface observations only increased when the Global Drifter Program became efficient, after the 1990s, and subsurface profile data from the Argo program achieved near-global coverage only around 2007 (Roemmich et al., 2019). This implies that ocean reanalyses are increasingly less reliable going further back in time. So, it is crucial to carefully assess their performance, particularly in light of the problems noted in the section “Introduction.”
Estimating ocean reanalysis skill and uncertainty can be performed in several ways. First, validating the reanalyses against independent (non-assimilated) datasets, typically available from special campaigns at regional scale (e.g., Koenig et al., 2017b, for the Arctic Ocean), delayed time reprocessed data (e.g., drifter-derived currents or cross-calibrated tide-gauge measurements) or by using multi-source data to evaluate complex frontal dynamics (Artana et al., 2018) and adopting popular skill score metrics (bias, root mean square error, etc.).
Second, probabilistic reanalyses based on ensemble data assimilation possibly maintained through perturbed forcing and physics, provide probability distribution functions of the ocean state. These also require that the dispersion of the limited-size ensemble also requires validation based on objective methods (Desroziers et al., 2005; Candille et al., 2015; Yan et al., 2015; Rodwell et al., 2016; Storto et al., 2018a).
Third, inter-comparison activities that assess reanalyses through evaluation of inter-system consistency provide valuable information. The spread of different reanalyses inform on the relative accuracy of the reanalyses for different ocean parameters. This approach has been performed by the CLIVAR/GSOP and GODAE communities, in particular during the recent ORA-IP project (Balmaseda et al., 2015; and the Climate Dynamics Special Issue5), followed by regional reanalysis inter-comparison assessments (e.g., the POLAR ORA-IP, Uotila et al., 2018).
An example is provided in Figure 2, which compiles findings from the ORA-IP project, showing the ensemble mean and spread of the reanalyses and reporting the Signal-to-Noise Ratio (SNR, see the figure caption for details) for several inter-compared parameters. The figure highlights how the net surface heat flux, the halosteric (hence salinity content) sea level, the thermosteric sea level below 700 m (hence heat content), and the sea-ice volume are much less constrained than sea surface temperature, 0–700 m thermosteric sea level and sea-ice concentration as indicated by the SNR values. CMEMS also provides multi-reanalysis assessments (e.g., Storto et al., 2018a) and a reanalysis ensemble mean product to end users, following a first attempt by Masina et al. (2017).
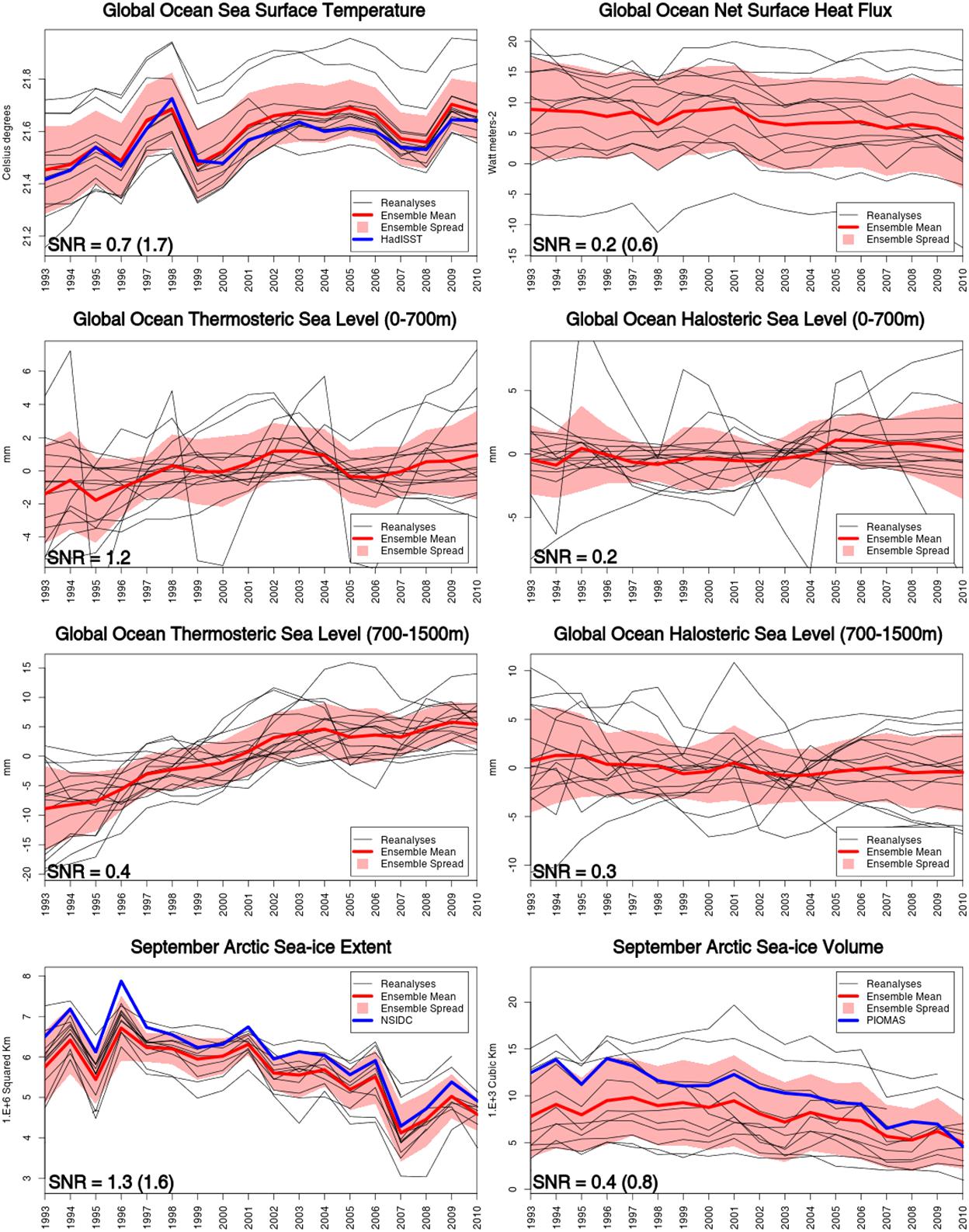
Figure 2. Timeseries of global ocean sea surface temperature, net surface heat flux, thermo, and halo steric sea level (0–700 and 700–1500 m), September Arctic sea-ice extent and volume, from the ORA-IP inter-comparison project. Each plot shows the ensemble mean and standard deviation and the individual ocean reanalyses. Reference datasets are shown in blue for selected parameters. Each plot reports also the Signal-to-noise ratio (SNR), defined here for simplicity as the ratio between the temporal standard deviation of the ensemble mean divided by the temporal mean of the ensemble standard deviation. Numbers in parentheses refer to the SNR ratio computed from anomalies rather than full field data, except for thermo and halo steric sea level data that are already anomalies by construction. Adapted from Chevallier et al. (2017), Storto et al. (2017), and Valdivieso et al. (2017). Image reproduced with permission of the rights holder.
Inter-comparison exercises also provide detailed information on the deficiency of the current ocean observing network. For instance, Mignac et al. (2018) showed that the AMOC in the South Atlantic among several ocean reanalyses strongly disagree with each other, due to the discrepancies in the flow strength in the upper and deep western boundary currents. However, the interior gyre circulations showed much greater consistency between products. This shows the effectiveness of data assimilation in the basin interior but also highlights the difficulty of constraining the western boundary currents with the present observations of Argo and altimetry, and illustrates the requirement for a sustained coastal observing system (De Mey-Frémaux et al., 2019).
Challenges
A number of specific challenges concern ocean reanalyses that are not necessarily shared with operational systems (Masina and Storto, 2017; Moore et al., 2019), because one of the fundamental goals of reanalyses is the reproduction of low-frequency (e.g., inter-annual) variability and trends. This is conceptually linked with the fact that most reanalyses implement sequential data assimilation tools that correct the state of the ocean at high frequencies (typically daily), thus introducing an intrinsic jump of spatial and temporal scales (Tardif et al., 2015).
Additionally, discontinuous observing networks often lead to inhomogeneous reanalysis time-series. The sampling of in situ profiles is always intrinsically irregular in space and time, but this is particularly so during periods of expansion of observing networks such as XBTs (1970s) and Argo floats (2000s). This is still true today with the inhomogeneous vertical sampling that can lead to large and unrealistic drift in unobserved layers below 2000 m depth (Lellouche et al., 2018).
At the surface new observation types are set to provide more complete global coverage, for instance Surface Water and Ocean Topography (SWOT) altimetry will provide sea level measurements reaching the sub-mesoscale, extended HF-radar systems can provide high-resolution surface current observations in the coastal areas, and a strengthened constellation of ocean-sensing satellites (e.g., from the Copernicus program) should increase dramatically in the next decades. Reanalyses will need to adapt their capabilities (i.e., resolution, data assimilation schemes) to the new observation sampling and types in order to still provide consistent climatic metrics.
Accounting for drift and bias due, e.g., to air-sea flux errors, inadequate model resolution and parameterizations is another non-trivial problem (Zuo et al., 2017). Model bias correction through observation-based (Balmaseda et al., 2007b; Storto et al., 2016), analysis increments-based (Dee, 2005; Canter et al., 2016), ensemble (Zuo et al., 2018), or adaptive (Lea et al., 2008) bias estimates, assimilation of additional climatological observations (Lellouche et al., 2018), on-line correction of atmospheric forcing and mixing terms (Stammer et al., 2004; Forget and Ponte, 2015; Forget et al., 2015a), assimilating anomalies instead of full-field observations (Weber et al., 2015; Counillon et al., 2016), are all bias mitigation methods which have been used in the last decade.
In data-sparse periods, the initialization of ocean reanalyses remains challenging, due to our intrinsic lack of knowledge about the ocean state at earlier times, and is acute in ocean reanalyses before the 1980s (see, e.g., de Boisséson et al., 2017). Inclusion of stochastic initialization (Zuo et al., 2018), point-wise linear regression from objective analyses (Lellouche et al., 2018), or adjoint methods resolving the entire reanalysis period at once (Wunsch and Heimbach, 2007; Forget et al., 2015a) are all possible solution methods that generally require additional computational costs. Other problems such as spurious vertical velocity fields caused by dynamical imbalance after data assimilation, are particularly detrimental to downstream applications such as biogeochemical reanalyses, where these imbalances propagate onto unrealistic distributions of nutrients (Forget et al., 2015b; Park et al., 2018), revealing consistency problems otherwise neglected.
In the context of high-resolution ocean reanalyses that approach eddying scales, additional challenging topics, derived by the increase of ocean model resolution, include among others (i) the coupling with tides and waves; (ii) dealing with mesoscale eddies and sharp gradients in properties; (iii) two-way nested analysis schemes (e.g., Barth et al., 2007); (iv) discontinuities at choke points or in key coastal areas; (v) downscaling of atmospheric forcing, and (vi) accuracy of bathymetry. All these aspects call for a close collaboration between the ocean modeling and the reanalysis communities. Furthermore the increasing demand for temporal and spatial resolution by end-users, for instance for ocean downscaling applications, translate into a big data challenge for high-resolution reanalyses, making the dissemination of full-resolution datasets prohibitively expensive with the current data infrastructure paradigms.
Observational Requirements
The need for sustained, long-term global observations to support climate monitoring and forecast activities has long been recognized (Karl et al., 2010), as well as the complementary need for reliable and sophisticated pre-processing procedures (bias-correction, redundancy checks, quality control, etc.). The impact of different observing networks on the quality of ocean reanalyses and forecast systems has been assessed in several studies. Observational networks like Argo and altimetry satellite data are crucial to correctly resolve the vertical structure of the water column and the mesoscale ocean circulation, respectively (e.g., Oke et al., 2015; Shao et al., 2015; Zhang et al., 2017). Their availability therefore has a direct impact in the quality of ocean reanalyses (Balmaseda et al., 2007a, 2013). Tropical observing systems, like the TAO/TRITON, RAMA and PIRATA arrays, provide important information for the accuracy of ocean forecast systems (see Oke et al., 2015 and references therein). The recent degradation in the TAO/TRITON arrays had a significant detrimental impact on forecast skill in the tropical Pacific (Fujii et al., 2015), showing that it is critical to quickly address such degradations.
Although the number of oceanic observations has increased in recent decades, high-quality observations remain scarce in places, especially around and underneath the sea ice (Pellichero et al., 2017). While remotely sensed ice concentration has had good spatial coverage since 1979, the amount of in situ measurements of sea ice thickness (SIT) is very limited (Worby et al., 2008), and long-term and large-scale data sets do yet not exist. Nevertheless, improved SIT estimates are crucial to improve Arctic seasonal forecasts (Blockley and Peterson, 2018) and to reduce climate-model uncertainties (Lisæter et al., 2007; Mathiot et al., 2012; Melia et al., 2015). To improve model confidence in predicting polar sea ice conditions, satellite missions aiming at retrieving information on SIT (such as CryoSat2 and SMOS, and their combination, see Ricker et al., 2017) have been found to improve the performance of reanalyses in polar regions (Allard et al., 2018; Mu et al., 2018; Xie et al., 2018).
For global estimates of key climate parameters such as ocean heat content, the depths below 2000 m are currently unobserved, leading to obvious deficiencies of reanalyses in the representation of deep water characteristics and variability (Storto et al., 2017). Repeated observational transects such as GO-SHIP (Talley et al., 2016), as a follow-up to the WOCE sections (Macdonald, 1998), are fundamental for multi-decadal climate monitoring, but may be inadequate to constrain the deep ocean. For example, when numerous upper ocean observations are available, the use of unreliable vertical covariances in data assimilation may induce the wrong response at depth. There is hope that the developing deep Argo program (Zilberman, 2017) will gradually fill the gaps between the upper and deep ocean observing networks.
For both coupled and uncoupled reanalyses, observations of air-sea fluxes, at the moment available from a small number of dedicated ocean stations (e.g., Curry et al., 2004), appear crucial for understanding the coupled data assimilation paradigm and improving air-sea flux formulations. On top of all these observational requirements, independent research-quality validation datasets (e.g., research quality tide-gauge data, Caldwell et al., 2015) must be sustained and made available in standard format (e.g., ADCP data from vessel-mounted or fixed location instruments to validate currents from reanalyses) to ensure reliable estimation of reanalysis uncertainty. The same applies to the development of independent remote sensing capabilities, both from existing and new satellites, such as the use of Gravity Recovery and Climate Experiment (GRACE) data to verify steric sea level rise in ocean reanalyses (Storto et al., 2017), or the use of IceSat sea ice freeboard measurements to validate sea ice thickness in reanalyses (Chevallier et al., 2017; Uotila et al., 2018).
Finally, as important as the data availability itself and data rescue projects such as GODAR6, improvements to quality-control and bias adjustment procedures for reanalysis applications need to be maintained and supported. Efforts are on-going, testified by the recent release of the first interim version of the IQuOD7 temperature profile dataset (Domingues and Palmer, 2015)8.
A Future Outlook
Recent years have seen the pioneering production of Earth system reanalyses that simultaneously produce a dynamically consistent reconstruction of the atmosphere, ocean, land and sea-ice, although with varying complexity in the data assimilation coupling (e.g., Penny et al., 2017). These products are preliminary but provide promising results (e.g., Feng et al., 2018) and will likely be developed much further in the coming decade. Earth system reanalyses can potentially improve the representation of budgets (Mayer et al., 2017), although propagation and amplification of coupled biases and errors may occur (Zhang et al., 2013). The role of (uncoupled) ocean reanalyses is expected to focus on the added value of eddying resolution (tackling eddy energetics, including tides at global scale, and improving the representation and the impact of coastal and shallow water processes on the global ocean), as well as consolidating its role as a validation tool for assessing the ocean model component of Earth system simulations. Emerging multi-scale data assimilation schemes (e.g., Miyoshi and Kondo, 2013) may further reconcile the mesoscale and large-scale circulation variability, whose simultaneous correction is generally lacking in the current state-of-the-art reanalyses. The increase in resolution also poses the challenge of data infrastructures capable of handling these large datasets; cloud computing services, new format standards, extraction services, etc., should all be investigated to facilitate access to high-resolution reanalysis datasets.
Flow-dependent error characterization for both rapidly evolving processes (marine weather) and low frequency processes (climate modes, changes in observational sampling, etc.) will likely become an important component of both long- and short-term ocean reanalyses, inheriting the positive experience from atmospheric reanalyses (Poli et al., 2013). In particular, the importance of flow-dependent ensemble based data assimilation methods, compared to prescribed error implementations, has recently been demonstrated at global scales by Penny et al. (2015) and Storto et al. (2018b), and regionally for sub-polar gyre variability (Counillon et al., 2016), and also for the assimilation of sea ice (Sakov et al., 2012; Barth et al., 2015; Kimmritz et al., 2018).
Changes in the observing network expected over the next decade will offer reanalyses the possibility to integrate a larger number of heterogeneous observing networks. Deep Argo, surface salinity, high resolution altimetry, gravimetry, coastal observing networks, together with conventional profile data, may all be ingested to improve the representation of heat, mass and freshwater budgets in uncoupled and coupled reanalyses.
Finally data rescue programs also increase the appetite for the backward extension of ocean reanalyses, but need to be complemented with realistic error estimates and sophisticated bias- and drift- aware data assimilation methods that currently lack sufficient robustness. Enhancing the ocean observing network will also enable better bias estimates to be developed and used. This appears particularly relevant in light of new projects aiming at producing millennial timescale reanalyses from multi-proxy data (e.g., PAGES2k Consortium, 2017).
Author Contributions
AS coordinated the individual contributions and compiled them into the manuscript. All co-authors contributed to the text by proposing the text, points of discussion, and references, and provided the corrections to a preliminary version of the manuscript.
Funding
We are grateful to the “Evaluation of Ocean Synthesis (EOS)” COST Action ES1402, which provided support for visiting research periods and networking opportunities contributing to some of the cited ocean reanalysis inter-comparison works. CLIVAR through the Global Synthesis and Observations Panel (GSOP) has also provided support for the reanalysis inter-comparison and assessment activities. CMD was funded by the Australian Research Council (FT130101532 and DP160103130); the Scientific Committee on Oceanic Research (SCOR) Working Group 148, funded by national SCOR committees and a grant to SCOR from the U.S. National Science Foundation (Grant OCE-1546580); and the Intergovernmental Oceanographic Commission of UNESCO/International Oceanographic Data and Information Exchange (IOC/IODE) IQuOD Steering Group.
Conflict of Interest Statement
The authors declare that the research was conducted in the absence of any commercial or financial relationships that could be construed as a potential conflict of interest.
Acknowledgments
We thank the two reviewers for their valuable help in improving the quality of the manuscript.
Footnotes
- ^ Available at: https://esgf.nccs.nasa.gov/search/create-ip/
- ^ Real-Time Ocean Reanalysis Inter-comparison Project (RT ORA-IP), available at: https://www.cpc.ncep.noaa.gov/products/GODAS/multiora93_body.html
- ^ See product catalog at: https://climate.copernicus.eu/; https://cds.climate.copernicus.eu
- ^ See product catalog at: http://marine.copernicus.eu/
- ^ Climate Dynamics Special Issue on “Ocean estimation from an ensemble of global ocean reanalysis,” Volume 49, Issue 3, August 2017, available at: https://link.springer.com/journal/382/49/3/page/1
- ^ Global Oceanographic Data Archaeology and Rescue (GODAR), Information available at: https://www.nodc.noaa.gov/about/international_godar.html
- ^ Data available at: https://data.nodc.noaa.gov/cgi-bin/iso?id=gov.noaa.nodc:IQuODv0.1
- ^ www.iquod.org
References
Alessandri, A., Borrelli, A., Masina, S., Cherchi, A., Gualdi, S., Navarra, A., et al. (2010). The INGV-CMCC seasonal prediction system: improved ocean initial conditions. Mon. Wea. Rev. 138, 2930–2952. doi: 10.1175/2010MWR3178.1
Allard, R. A., Farrell, S. L., Hebert, D. A., Johnston, W. F., Li, L., Kurtz, N. T., et al. (2018). Utilizing CryoSat-2 sea ice thickness to initialize a coupled ice-ocean modeling system. Adv. Space Res. 62, 1265–1280. doi: 10.1016/j.asr.2017.12.030
Artana, C., Lellouche, J.-M., Park, Y.-H., Garric, G., Koenig, Z., Sennéchael, N., et al. (2018). Fronts of the Malvinas current system: surface and subsurface expressions revealed by satellite altimetry, Argo floats, and Mercator operational model outputs. J. Geophys. Res. Oceans 123, 5261–5285. doi: 10.1029/2018JC013887
Balmaseda, M. A., Dee, D., Vidard, A., and Anderson, D. L. (2007b). A multivariate treatment of bias for sequential data assimilation: application to the tropical oceans. Q .J. R. Meteorol. Soc. 133, 167–179. doi: 10.1002/qj.12
Balmaseda, M., Anderson, D., and Vidard, A. (2007a). Impact of Argo on analyses of the global ocean. Geophys. Res. Lett. 34:L16605. doi: 10.1029/2007GL030452
Balmaseda, M. A., Hernandez, A., Storto, M. D., Palmer, O., Alves, L., and Shi, G. C. (2015). The ocean reanalyses intercomparison project (ORA-IP). J. Operat. Oceanogr. 8(Suppl. 1), s80–s97. doi: 10.1080/1755876X.2015.1022329
Balmaseda, M. A., Trenberth, K. E., and Källén, E. (2013b). Distinctive climate signals in reanalysis of global ocean heat content. Geophys. Res. Lett. 40, 1754–1759. doi: 10.1002/grl.50382
Balmaseda, M. A., Mogensen, K., and Weaver, A. T. (2013). Evaluation of the ECMWF ocean reanalysis system ORAS4. Q. J. R. Meteorol. Soc. 139, 1132–1161. doi: 10.1002/qj.2063
Barth, A., Alvera-Azcárate, A., Beckers, J.-M., Rixen, M., and Vandenbulcke, L. (2007). Multigrid state vector for data assimilation in a two-way nested model of the Ligurian Sea. J. f Mar. Syst. 65, 41–59. doi: 10.1016/j.jmarsys.2005.07.006
Barth, A., Alvera-Azcárate, A., and Weisberg, R. H. (2008). Benefit of nesting a regional model into a large-scale ocean model instead of climatology. Application to the West Florida Shelf. Cont. Shelf Res. 28, 561–573. doi: 10.1016/j.csr.2007.11.004
Barth, A., Canter, M., Van Schaeybroeck, B., Vannitsem, S., Massonnet, F., Zunz, V., et al. (2015). Assimilation of sea surface temperature, sea ice concentration and sea ice drift in a model of the Southern Ocean. Ocean Model. 93, 22–39. doi: 10.1016/j.ocemod.2015.07.011
Bellucci, A., Gualdi, S., Masina, S., Storto, A., Scoccimarro, E., Cagnazzo, C., et al. (2011). Decadal climate predictions with a coupled OAGCM initialized with oceanic reanalyses. Clim. Dyn. 40, 1483–1497. doi: 10.1007/s00382-012-1468-z
Blockley, E. W., and Peterson, K. A. (2018). Improving Met Office seasonal predictions of Arctic sea ice using assimilation of CryoSat-2 thickness. Cryosphere 12, 3419–3438. doi: 10.5194/tc-12-3419-201
Breivik, Ø, Mogensen, K. J., Bidlot, R., Balmaseda, M. A., and Janssen, P. A. (2015). Surface wave effects in the NEMO ocean model: forced and coupled experiments. J. Geophys. Res. Oceans 120, 2973–2992. doi: 10.1002/2014JC010565
Bricaud, C., Garric, G., Drillet, Y., Zuo, H., Storto, A., Peterson, K. A., et al. (2018). The copernicus marine environment monitoring service ocean state report. J. Operat. Oceanogr. 11(Suppl. 1), S49–S53. doi: 10.1080/1755876X.2018.1489208
Caldwell, P. C., Merrifield, M. A., and Thompson, P. R. (2015). Sea Level Measured by Tide Gauges from Global Oceans — the Joint Archive for Sea Level holdings (NCEI Accession 0019568), Version 5.5. Silver Spring, MD: NOAA. doi: 10.7289/V5V40S7W
Candille, G., Brankart, J.-M., and Brasseur, P. (2015). Assessment of an ensemble system that assimilates Jason-1/Envisat altimeter data in a probabilistic model of the North Atlantic ocean circulation. Ocean Sci. 11, 425–438. doi: 10.5194/os-11-425-2015
Canter, M., Barth, A., and Beckers, J.-M. (2016). Correcting circulation biases in a lower-resolution global general circulation model with data assimilation. Ocean Dyn. 67, 1–18. doi: 10.1007/s10236-016-1022-3
Chevallier, M., Smith, G. C., Dupont, F., Lemieux, J.-F., Forget, G., and Fujii, Y. (2017). Intercomparison of the Arctic sea ice cover in global ocean-sea ice reanalyses from the ORA-IP project. Clim. Dyn. 49, 1107–1136. doi: 10.1007/s00382-016-2985-y
Cipollone, A., Masina, S., Storto, A., and Iovino, D. (2017). Benchmarking the mesoscale variability in global ocean eddy-permitting numerical systems. Ocean Dyn. 67, 1313–1333. doi: 10.1007/s10236-017-1089-5
Compo, G. P., Whitaker, J. S., Sardeshmukh, D. P., Matsui, N., Allan, R. J., and Yin, X. (2011). The Twentieth Century reanalysis project. Q. J. R. Meteorol. Soc. 137, 1–28. doi: 10.1002/qj.776
Counillon, F., Keenlyside, N., Bethke, I., Wang, Y., Billeau, S., Shen, M. L., et al. (2016). Flow-dependent assimilation of sea surface temperature in isopycnal coordinates with the Norwegian climate prediction model. Tellus A 68, 1–17. doi: 10.3402/tellusa.v68.32437
Curry, J. A., Bentamy, M. A., Bourassa, A., Bourras, D., Bradley, E. F., and Brunke, M. (2004). SEAFLUX. Bull. Am. Meteor. Soc. 85, 409–424. doi: 10.1175/BAMS-85-3-409
de Boisséson, E., Balmaseda, M. A., and Mayer, M. (2017). Ocean heat content variability in an ensemble of twentieth century ocean reanalyses. Clim. Dyn. 50, 3783–3798. doi: 10.1007/s00382-017-3845-0
De Mey-Frémaux, P., Ayoub, N., Barth, A., Brewin, R., Charria, G., Campuzano, F., et al. (2019). Model-observations synergy in the coastal ocean. Front. Mar. Sci. 6:436. doi: 10.3389/fmars.2019.00436
Dee, D. P. (2005). Bias and data assimilation. Q. J. R. Meteorol. Soc. 131, 3323–3343. doi: 10.1256/qj.05.137
Dee, D. P., Uppala, S. M., Simmons, A. J., Berrisford, P., Poli, P., and Kobayashi, S. (2011). The ERA-Interim reanalysis: configuration and performance of the data assimilation system. Q. J. R. Meteorol. Soc. 137, 553–597. doi: 10.1002/qj.828
Desroziers, G., Berre, L., Chapnik, B., and Poli, P. (2005). Diagnosis of observation, background and analysis-error statistics in observation space. Q. J. R. Meteorol. Soc. 131, 3385–3396. doi: 10.1256/qj.05.108
Doblas-Reyes, F. J., García-Serrano, J., Lienert, F., Biescas, A. P., and Rodrigues, L. R. L. (2013). Seasonal climate predictability and forecasting: status and prospects. WIREs Clim. Change 4, 245–268. doi: 10.1002/wcc.217
Domingues, C., and Palmer, M. (2015). The IQuOD initiative: towards an international quality controlled ocean database. Clivar. Exchanges 19, 38–40.
Duan, Y., Liu, H., Yu, W., and Hou, Y. (2016). The mean properties and variations of the Southern Hemisphere subpolar gyres estimated by simple ocean data assimilation (SODA) products. Acta Oceanol. Sin. 3, 8–13. doi: 10.1007/s13131-016-0901-2
Evans, D. G., Toole, J., Forget, G., Zika, J. D., Naveira Garabato, A. C., Nurser, A. G., et al. (2017). Recent wind-driven variability in atlantic water mass distribution and meridional overturning circulation. J. Phys. Oceanogr. 47, 633–647. doi: 10.1175/JPO-D-16-0089.1
Feng, X., Haines, K., Liu, C., de Boisséson, E., and Polo, I. (2018). Improved SST-precipitation intraseasonal relationships in the ECMWF coupled climate reanalysis. Geophys. Res. Lett. 45, 3664–3672. doi: 10.1029/2018GL077138
Forget, G. (2010). Mapping Ocean Observations in a dynamical framework: a 2004–06 Ocean Atlas. J. Phys. Oceanogr. 40, 1201–1221. doi: 10.1175/2009JPO4043.1
Forget, G., Campin, J.-M., Heimbach, P., Hill, C. N., Ponte, R. M., et al. (2015a). ECCO version 4: an integrated framework for non-linear inverse modeling and global ocean state estimation. Geosci. Model Dev. 8, 3071–3104. doi: 10.5194/gmd-8-3071-2015
Forget, G., Ferreira, D., and Liang, X. (2015b). On the observability of turbulent transport rates by Argo: supporting evidence from an inversion experiment. Ocean Sci. 11, 839–853. doi: 10.5194/os-11-839-2015
Forget, G., and Ponte, R. M. (2015). The partition of regional sea level variability. Prog. Oceanogr. 137, 173–195. doi: 10.1016/j.pocean.2015.06.002
CrossRef Full Text doi: 10.1016/j.pocean.2015.06.002
Fujii, Y., Cummings, J., Xue, Y., Schiller, A., Lee, T., and Balmaseda, M. A. (2015). Evaluation of the Tropical Pacific Observing System from the ocean data assimilation perspective. Q. J. R. Meteorol. Soc. 141, 2481–2496. doi: 10.1002/qj.2579
Fujii, Y., Remy, E., Remy, H., Oke, P. R., Halliwell, G. R., Gasparin, F., et al. (2019). Observing system evaluation based on ocean data assimilation and prediction systems: on-going challenges and future vision for designing/supporting ocean observational networks. Front. Mar. Sci. 6:417. doi: 10.3389/fmars.2019.00417
Fukumori, I., Heimbach, P., Ponte, R. M., and Wunsch, C. (2018). A dynamically consistent, multi-variable ocean climatology. Bull. Amer. Meteor. Soc. 99, 2107–2128. doi: 10.1175/bams-d-17-0213.1
Garric, G., Hernandez, O., Bricaud, C., Storto, A., Peterson, K. A., and Zuo, H. (2018). Arctic Ocean freshwater content, in: the copernicus marine environment monitoring service ocean state report, issue 2. J. Operat. Oceanogr. 11(Suppl. 1), S70–S78. doi: 10.1080/1755876X.2018.1489208
Gasparin, F., Guinehut, S., Mao, C., Mirouze, I., Rémy, E., King, R. R., et al. (2019). Requirements for an Integrated in situ Atlantic ocean observing system from coordinated observing system simulation experiments. Front. Mar. Sci. 6:83. doi: 10.3389/fmars.2019.00083
Giese, B. S., Seidel, H. F., Compo, G. P., and Sardeshmukh, P. D. (2016). An ensemble of ocean reanalyses for 1815–2013 with sparse observational input. J. Geophys. Res. Oceans 121, 6891–6910. doi: 10.1002/2016JC012079
Guemas, V., Blanchard-Wrigglesworth, E., Chevallier, M., Day, J. J., Déqué, M., Doblas-Reyes, F. J., et al. (2016). A review on Arctic sea-ice predictability and prediction on seasonal to decadal time-scales. Q. J. R. Meteorol. Soc. 142, 546–561. doi: 10.1002/qj.2401
Hartfield, G., Blunden, J., and Arndt, D. S. (2018). A Look at 2017: takeaway points from the State of the climate supplement. Bull. Am. Meteor. Soc. 99, 1527–1539. doi: 10.1175/BAMS-D-18-0173.1
Jackson, L., Dubois, C., Masina, S., Storto, A., and Zuo, H. (2018). Atlantic meridional overturning circulation. in copernicus marine service ocean state report, issue 2. J. Operat. Oceanogr. 11(Suppl.1), S65–S66. doi: 10.1080/1755876X.2018.1489208
Jackson, L. C., Peterson, K. A., Roberts, C. D., and Wood, R. A. (2016). Recent slowing of Atlantic overturning circulation as a recovery from earlier strengthening. Nat. Geosci. 9, 518–522. doi: 10.1038/ngeo2715
Johnson, G. C., Lyman, J. M., Boyer, T., Cheng, L., Domingues, C. M., Gilson, J., et al. (2018). Global oceans: ocean heat content. In state of the climate in 2017. Bull. Am. Meteorol. Soc. 99, S72–S77. doi: 10.1175/2018BAMSStateoftheClimate.1
Johnson, S. J., Stockdale, T. N., Ferranti, L., Balmaseda, M. A., Molteni, F., Magnusson, L., et al. (2018). SEAS5: the new ECMWF seasonal forecast system. Geosci. Model Dev. 12, 1087–1117. doi: 10.5194/gmd-2018-228
Karl, T. R., Diamond, H. J., Bojinski, S., Butler, J. H., Dolman, H., and Haeberli, W. (2010). Observation needs for climate information, prediction and application: capabilities of existing and future observing systems. Proc. Environ. Sci. 1, 192–205. doi: 10.1016/j.proenv.2010.09.013
Kimmritz, M., Counillon, F., Bitz, C. M., Massonnet, F., Bethke, I., and Gao, Y. (2018). Optimising assimilation of sea ice concentration in an Earth system model with a multicategory sea ice model. Tellus A 70, 1–23. doi: 10.1080/16000870.2018.1435945
Koenig, Z., Provost, C., Sennéchael, N., Garric, G., and Gascard, J. C. (2017a). The Yermak pass branch: a major pathway for the Atlantic Water north of Svalbard? J. Geophys. Res. Oceans 122, 9332–9349. doi: 10.1002/2017JC013271
Koenig, Z., Provost, C., Villacieros-Robineau, N., Sennéchael, N., Meyer, A., Lellouche, J.-M., et al. (2017b). Atlantic Waters inflow north of Svalbard: insights from IAOOS observations and Mercator Ocean global operational system during N-ICE2015. JGR Oceans 122, 1254–1273. doi: 10.1002/2016JC012424
Laloyaux, P., de Boisseson, E., Balmaseda, M., Bidlot, J.-R., Broennimann, S., Buizza, R., et al. (2018). CERA-20C: a coupled reanalysis of the twentieth century. J. Adv. Model. Earth Syst. 10, 1172–1195. doi: 10.1029/2018MS001273
Lea, D. J., Drecourt, J., Haines, K., and Martin, M. J. (2008). Ocean altimeter assimilation with observational- and model-bias correction. Q. J. R. Meteorol. Soc. 134, 1761–1774. doi: 10.1002/qj.320
Legeais, J. F., Ablain, M., Zawadzki, L., Zuo, H., Johannessen, J. A., and Scharffenberg, M. G. (2018). An improved and homogeneous altimeter sea level record from the ESA climate change initiative. Earth Syst. Sci. Data 10, 281–301. doi: 10.5194/essd-10-281-2018
Lellouche, J.-M., Drévillon, M., Régnier, C., Greiner, E., Garric, G., et al. (2013). Evaluation of global monitoring and forecasting systems at Mercator Océan. Ocean Sci. 9, 57–81. doi: 10.5194/os-9-57-2013
Lellouche, J.-M., Greiner, E., Le Galloudec, O., Garric, G., Regnier, C., Drevillon, M., et al. (2018). Recent updates on the copernicus marine service global ocean monitoring and forecasting real-time 1/12° high resolution system. Ocean Sci. 14, 1093–1126. doi: 10.5194/os-14-1093-2018
Vidar, S. L., Solfrid, S. H., Skogen, M. D., Svendsen, E., Wehde, H., and Bertino, L. (2016). An Assessment of the added value from data assimilation on modelled Nordic Seas hydrography and ocean transports. Ocean Model. 99, 43–59. doi: 10.1016/j.ocemod.2015.12.010
Lisæter, K. A., Evensen, G., and Laxon, S. (2007). Assimilating synthetic CryoSat sea ice thickness in a coupled ice-ocean model. J. Geophys. Res. 112:C07023. doi: 10.1029/2006JC003786
Macdonald, A. M. (1998). The global ocean circulation: a hydrographic estimate and regional analysis. Prog. Oceanogr. 41, 281–382. doi: 10.1016/S0079-6611(98)00020-2
Masina, S., and Storto, A. (2017). Reconstructing the recent past ocean variability: status and perspective. J. Mar. Res. 75, 727–764. doi: 10.1357/002224017823523973
Masina, S., Storto, A., Ferry, N., Valdivieso, M., Haines, K., Balmaseda, M., et al. (2017). An ensemble of eddy-permitting global ocean reanalyses from the MyOcean project. Clim. Dyn. 49, 813–841. doi: 10.1007/s00382-015-2728-5
Massonnet, F., Mathiot, P., Fichefet, T., Goosse, H., König Beatty, C., Vancoppenolle, M., et al. (2013). A model reconstruction of the Antarctic sea ice thickness and volume changes over 1980–2008 using data assimilation. Ocean Model. 64, 67–75. doi: 10.1016/j.ocemod.2013.01.003
Mathiot, P., König Beatty, C., Fichefet, T., Goosse, H., Massonnet, F., and Vancoppenolle, M. (2012). Better constraints on the sea-ice state using global sea-ice data assimilation. Geosci. Model Dev. 5, 1501–1515. doi: 10.5194/gmd-5-1501-2012
Mayer, M., Alonso Balmaseda, M., and Haimberger, L. (2018). Unprecedented 2015/2016 Indo-Pacific heat transfer speeds up tropical Pacific heat recharge. Geophys. Res. Lett. 45, 3274–3284. doi: 10.1002/2018GL077106
Mayer, M., Haimberger, L., Edwards, J. M., and Hyder, P. (2017). Toward consistent diagnostics of the coupled atmosphere and ocean energy budgets. J. Clim. 30, 9225–9246. doi: 10.1175/JCLI-D-17-0137.1
Melia, N., Haines, K., and Hawkins, E. (2015). Improved Arctic sea ice thickness projections using bias corrected CMIP5 simulations. Cryosphere 9, 2237–2251. doi: 10.5194/tc-9-2237-2015
Mignac, D., Ferreira, D., and Haines, K. (2018). South Atlantic meridional transports from NEMO-based simulations and reanalyses. Ocean Sci. 14, 53–68. doi: 10.5194/os-14-53-2018
Miyoshi, T., and Kondo, K. (2013). A multi-scale localization approach to an ensemble Kalman filter. SOLA 9, 170–173. doi: 10.2151/sola.2013-038
Moore, A. M., Martin, M. J., Akella, S., Arango, H. G., Balmaseda, M., Bertino, L., et al. (2019). Synthesis of ocean observations using data assimilation for operational, real-time and reanalysis systems: a more complete picture of the state of the ocean. Front. Mar. Sci. 6:90. doi: 10.3389/fmars.2019.00090
Mu, L., Yang, Q., Losch, M., Losa, S. N., Ricker, R., Nerger, L., et al. (2018). Improving sea ice thickness estimates by assimilating CryoSat-2 and SMOS sea ice thickness data simultaneously. Q. J. R. Meteorol. Soc. 144, 529–538. doi: 10.1002/qj.3225
Mulholland, D. P., Haines, K., and Balmaseda, M. A. (2016). Improving seasonal forecasting through tropical ocean bias corrections. Q. J. R. Meteorol. Soc. 142, 2797–2807. doi: 10.1002/qj.2869
Oke, P. R., Larnicol, G., Fujii, Y., Smith, G. C., Lea, D. J., and Guinehut, S. (2015). Assessing the impact of observations on ocean forecasts and reanalyses: part 1, Global studies. J. Operat. Oceanogr. 8, s49–s62. doi: 10.1080/1755876X.2015.1022067
PAGES2k Consortium (2017). A global multiproxy database for temperature reconstructions of the Common Era. Sci. Data 4:170088. doi: 10.1038/sdata.2017.88
Park, J.-Y., Stock, C. A., Yang, X., Dunne, J. P., Rosati, A., John, J., et al. (2018). Modeling global ocean biogeochemistry with physical data assimilation: a pragmatic solution to the equatorial instability. J. Adv. Model. Earth Syst. 10, 891–906. doi: 10.1002/2017MS001223
Pellichero, V., Sallée, J. B., Schmidtko, S., Roquet, F., and Charrassin, J.-B. (2017). The ocean mixed layer under Southern Ocean sea-ice: seasonal cycle and forcing. J. Geophys. Res. 122, 1608–1633. doi: 10.1002/2016jc011970
Penny, S. G., Akella, R., Sergey, F., Yosuke, F., Alicia, K., and Malaquias, P. (2017). Coupled Data Assimilation for Integrated Earth System Analysis and Prediction: Goals, Challenges, and Recommendations. World Weather Research Programme (WWRP) Report 2017-3. Geneva: World Meteorological Organization.
Penny, S. G., Behringer, W. D., Carton, J. A., and Kalnay, E. (2015). A hybrid global ocean data assimilation system at NCEP. Mon. Wea. Rev. 143, 4660–4677. doi: 10.1175/MWR-D-14-00376.1
Poli, P., Hersbach, H., Tan, D. G. H., Dee, D. P., Thépaut, J.-N., and Simmons, A. (2013). The data assimilation system and initial performance evaluation of the ECMWF pilot reanalysis of the 20th-century assimilating surface observations only (ERA-20C). ECMWF ERA Rep. 14:59.
Potter, G. L., Carriere, L., Hertz, J., Bosilovich, M., Duffy, D., Lee, T., et al. (2018). Enabling reanalysis research using the collaborative reanalysis technical environment (CREATE). Bull. Am. Meteor. Soc. 99, 677–687. doi: 10.1175/BAMS-D-17-0174.1
Ricker, R., Hendricks, S., Kaleschke, L., Tian-Kunze, X., King, J., et al. (2017). A weekly Arctic sea-ice thickness data record from merged CryoSat-2 and SMOS satellite data. Cryosphere 11, 1607–1623. doi: 10.5194/tc-11-1607-2017
Rodwell, M. J., Lang, S. T. K., Ingleby, N. B., Bormann, N., Hólm, E., Rabier, F., et al. (2016). Reliability in ensemble data assimilation. Q. J. R. Meteorol. Soc. 142, 443–454. doi: 10.1002/qj.2663
Roemmich, D., Alford, M. H., Claustre, H., Johnson, K. S., King, B., Moum, J., et al. (2019). On the future of Argo: a global, full-depth, multi-disciplinary array. Front. Mar. Sci. (in press). doi: 10.3389/fmars.2019.00439
Sakov, P., Counillon, F., Bertino, L., Lister, K. A., Oke, P. R., and Korablev, A. (2012). TOPAZ4: an ocean-sea ice data assimilation system for the North Atlantic and Arctic. Ocean Sci. 8, 633–656. doi: 10.5194/os-8-633-2012
Schweiger, A., Lindsay, R., Zhang, J., Steele, M., Stern, H., and Kwok, R. (2011). Uncertainty in modeled Arctic sea ice volume. J. Geophys. Res. 116:C00D06. doi: 10.1029/2011JC007084
Shao, C., Xuan, L., Cao, Y., Cui, X., and Gao, S. (2015). Impact of argo observation on the regional ocean reanalysis of China coastal waters and adjacent seas: a twin-experiment study. Adv. Meteorol. 2015:793825. doi: 10.1155/2015/793825
Stammer, D., Ueyoshi, K., Kohl, A., Large, W. G., Josey, S. A., and Wunsch, C. (2004). Estimating air-sea fluxes of heat, freshwater, and momentum through global ocean data assimilation. J. Geophys. Res. 109: C05023. doi: 10.1029/2003JC002082
Storto, A., and Masina, S. (2016). C-GLORSv5: an improved multipurpose global ocean eddy-permitting physical reanalysis. Earth Syst. Sci. Data 8, 679–696. doi: 10.5194/essd-8-679-2016
Storto, A., Masina, S., Balmaseda, M., Guinehut, S., Xue, Y., Szekely, T., et al. (2017). Steric sea level variability (1993–2010) in an ensemble of ocean reanalyses and objective analyses. Clim. Dyn. 49, 709–729. doi: 10.1007/s00382-015-2554-9
Storto, A., Masina, S., and Navarra, A. (2016). Evaluation of the CMCC eddy-permitting global ocean physical reanalysis system (C-GLORS, 1982–2012) and its assimilation components. Q. J. R. Meteorol. Soc. 142, 738–758. doi: 10.1002/qj.2673
Storto, A., Oddo, P., Cipollone, A., Mirouze, I., and Lemieux-Dudon, B. (2018b). Extending an oceanographic variational scheme to allow for affordable hybrid and four-dimensional data assimilation. Ocean Model. 128, 67–86. doi: 10.1016/j.ocemod.2018.06.005
Storto, A., Masina, S., Simoncelli, S., Iovino, D., Cipollone, A., Drevillon, M., et al. (2018a). The added value of the multi-system spread information for ocean heat content and steric sea level investigations in the CMEMS GREP ensemble reanalysis product. Clim. Dyn. 53, 287–312. doi: 10.1007/s00382-018-4585-5
Talley, L. D., Feely, R. A., Sloyan, B. M., Wanninkhof, R., Baringer, M. O., and Bullister, J. L. (2016). Changes in ocean heat, carbon content, and ventilation: review of the first decade of global repeat hydrography (GO-SHIP). Ann. Rev. Mar. Sci. 8, 185–215. doi: 10.1146/annurev-marine-052915-100829
Tardif, R., Hakim, G. J., and Snyder, C. (2015). Coupled atmosphere–ocean data assimilation experiments with a low-order model and CMIP5 model data. Clim. Dyn. 45:1415. doi: 10.1007/s00382-014-2390-3
Toyoda, T., Fujii, Y., Kuragano, T., Masafumi, K., Yoichi, I., Shuhei, M., et al. (2017). Intercomparison and validation of the mixed layer depth fields of global ocean syntheses. Clim. Dyn. 49, 753–773. doi: 10.1007/s00382-015-2637-7
Trenberth, K. E., Fasullo, J. T., von Schuckmann, K., and Cheng, L. (2016). Insights into Earth’s Energy Imbalance from Multiple Sources. Clim. J. 29, 7495–7505. doi: 10.1175/JCLI-D-16-0339.1
Uotila, P., Goosse, H., Haines, K., Matthieu, C., Antoine, B., Clément, B., et al. (2018). An assessment of ten ocean reanalyses in the polar regions. Clim. Dyn. 52, 1613–1650. doi: 10.1007/s00382-018-4242-z
Valdivieso, M., Haines, K., Balmaseda, M., Chang, Y.-S., Drevillon, M., Ferry, N., et al. (2017). An assessment of air–sea heat fluxes from ocean and coupled reanalyses. Clim. Dyn. 49, 983–1008. doi: 10.1007/s00382-015-2843-3
von Schuckmann, K., Le Traon, P.-Y., Smith, N., Pascual, A., Brasseur, P., and Fennel, K. (2018a). Copernicus marine service ocean state report. J. Operat. Oceanogr. 11, s1–s142. doi: 10.1080/1755876X.2018.1489208
von Schuckmann, K., Storto, A., Simoncelli, S., Raj, R., Samuelsen, A., and de Pascual Collar, A. (2018b). Ocean heat content; in: copernicus marine service ocean state report, issue 2. J. Operat. Oceanogr. 11, s41–s45. doi: 10.1080/1755876X.2018.1489208
Weber, R. J., Carrassi, A., and Doblas-Reyes, F. J. (2015). Linking the anomaly initialization approach to the mapping paradigm: a proof-of-concept study. Mon. Wea. Rev. 143, 4695–4713. doi: 10.1175/MWR-D-14-00398.1
Worby, A. P., Geiger, C. A., Paget, M. J., Woert, M. L. V., Ackley, S. F., and DeLiberty, T. L. (2008). Thickness distribution of Antarctic sea ice. J. Geophys. Res. 113:C05S92. doi: 10.1029/2007JC004254
Wunsch, C., and Heimbach, P. (2007). Practical global oceanic state estimation. Physica D 230, 197–208. doi: 10.1016/j.physd.2006.09.040
Xie, J., Counillon, F., and Bertino, L. (2018). Impact of assimilating a merged sea ice thickness from CryoSat-2 and SMOS in the Arctic reanalysis. Cryosphere 12, 3671–3691. doi: 10.5194/tc-12-3671-2018
Xue, Y., Wen, C., Kumar, A., Balmaseda, M., Fujii, Y., Alves, O., et al. (2017). A real-time ocean reanalyses intercomparison project in the context of tropical pacific observing system and ENSO monitoring. Clim. Dyn. 49, 3647–3672. doi: 10.1007/s00382-017-3535-y
Yan, Y., Barth, A., Beckers, J. M., Candille, G., Brankart, J. M., and Brasseur, P. (2015). Ensemble assimilation of ARGO temperature profile, sea surface temperature, and altimetric satellite data into an eddy permitting primitive equation model of the North Atlantic Ocean. J. Geophys. Res. Oceans 120, 5134–5157. doi: 10.1002/2014JC010349
Yang, C., Masina, S., and Storto, A. (2017). Historical ocean reanalyses (1900–2010) using different data assimilation strategies. Q. J. R. Meteorol. Soc. 143, 479–493. doi: 10.1002/qj.2936
Zhang, S., Chang, Y.-S., Yang, X., and Rosati, A. (2013). Balanced and coherent climate estimation by combining data with a biased coupled model. J. Clim. 27, 1302–1314. doi: 10.1175/JCLI-D-13-00260.1
Zhang, X., Sun, C., Liu, C., Zhang, L., Shao, C., Zhang, X., et al. (2017). Evaluation of the impact of argo data on ocean reanalysis in the Pacific Region. Adv. Meteorol. 2017, 7314106. doi: 10.1155/2017/7314106
Zilberman, N. V. (2017). Deep argo: sampling the total ocean volume in state of the climate in 2016. Bull. Am. Meteorol. Soc. 98, S73–S74. doi: 10.1175/2017BAMSStateoftheClimate.1
Zuo, H., Balmaseda, M. A., and Mogensen, K. (2017). The new eddy-permitting ORAP5 ocean reanalysis: description, evaluation and uncertainties in climate signals. Clim. Dyn. 49, 791–811. doi: 10.1007/s00382-015-2675-1
Zuo, H., Balmaseda, M. A., Mogensen, K., and Tietsche, S. (2018). OCEAN5: the ECMWF Ocean Reanalysis System and its Real-Time analysis component, ECMWF Tech Memo 823. Reading: ECMWF.
Keywords: data assimilation, uncertainty, inter-comparisons, observational requirements, historical reanalyses
Citation: Storto A, Alvera-Azcárate A, Balmaseda MA, Barth A, Chevallier M, Counillon F, Domingues CM, Drevillon M, Drillet Y, Forget G, Garric G, Haines K, Hernandez F, Iovino D, Jackson LC, Lellouche J-M, Masina S, Mayer M, Oke PR, Penny SG, Peterson KA, Yang C and Zuo H (2019) Ocean Reanalyses: Recent Advances and Unsolved Challenges. Front. Mar. Sci. 6:418. doi: 10.3389/fmars.2019.00418
Received: 30 October 2018; Accepted: 05 July 2019;
Published: 31 July 2019.
Edited by:
Laura Lorenzoni, University of South Florida, Tampa, United StatesReviewed by:
Nadya Vinogradova Shiffer, National Aeronautics and Space Administration (NASA), United StatesJoaquin A. Trinanes, University of Santiago de Compostela, Spain
Copyright © 2019 Storto, Alvera-Azcárate, Balmaseda, Barth, Chevallier, Counillon, Domingues, Drevillon, Drillet, Forget, Garric, Haines, Hernandez, Iovino, Jackson, Lellouche, Masina, Mayer, Oke, Penny, Peterson, Yang and Zuo. This is an open-access article distributed under the terms of the Creative Commons Attribution License (CC BY). The use, distribution or reproduction in other forums is permitted, provided the original author(s) and the copyright owner(s) are credited and that the original publication in this journal is cited, in accordance with accepted academic practice. No use, distribution or reproduction is permitted which does not comply with these terms.
*Correspondence: Andrea Storto, YW5kcmVhLnN0b3J0b0BjbXJlLm5hdG8uaW50