- 1National Weather Service, National Oceanic and Atmospheric Administration, Silver Spring, MD, United States
- 2Pacific Marine Environmental Laboratory, National Oceanic and Atmospheric Administration, Seattle, WA, United States
- 3Tsunami Aware and Prepare, Melbourne, VIC, Australia
- 4Servicio Hidrográfico y Oceanográfico de la Armada, Valparaíso, Chile
- 5Ministry of Civil Defence and Emergency Management, Wellington, New Zealand
- 6GNS Science, Wellington, New Zealand
- 7Japan Meteorological Agency, Tokyo, Japan
- 8Caribbean Tsunami Warning Program, National Oceanic and Atmospheric Administration, Mayagüez, PR, United States
- 9International Tsunami Information Center, National Weather Service, National Oceanic and Atmospheric Administration/Intergovernmental Oceanographic Commission, United Nations Educational, Scientific and Cultural Organization, Honolulu, HI, United States
- 10Pacific Tsunami Warning Center, National Oceanic and Atmospheric Administration, Honolulu, HI, United States
- 11Bureau of Meteorology, Melbourne, VIC, Australia
- 12The Agency for the Assessment and Application of Technology, Jakarta, Indonesia
- 13Commissariat à l’Énergie Atomique et aux Energies Alternatives, Arpajon, France
It is possible that no catastrophe has mobilized the global ocean science and coastal emergency management communities more than the 2004 Indian Ocean tsunami. Though the Pacific tsunami threat was recognized, and a warning system had been in place since 1965, there was no warning system in the Indian Ocean, and almost 230,000 people perished. More broadly, the event highlighted critical gaps in global tsunami science and observation systems. In 2004, real-time coastal and deep-ocean observation systems were almost non-existent. Tsunami sources were inferred based on rough seismic parameters. Since then, tremendous strides have been made under the auspices of IOC/UNESCO toward better understanding tsunami mechanisms, deploying advanced real-time tsunami observation systems, and establishing tsunami warning and mitigation systems for the four main ocean basins at risk from tsunamis. Nevertheless, significant detection, measurement, and forecast uncertainties remain to meet emergency response and community needs. A new generation of ocean sensing capabilities presents an opportunity to address several of these uncertainties. Ocean bottom pressures can be measured over dense, multisensor grids linking stand-alone buoy systems with emerging capabilities like fiber-optic cables. The increasing number of coastal sea-level stations provides the higher time and space resolution needed to better verify forecasts and account for local variability. In addition, GNSS sensors may be able to provide solid-earth data needed to define seismic tsunami sources more precisely in the short timescales required. When combined with advances in seismology, other emerging techniques, and state-of-the-art modeling and computational resources, these capabilities will enable more timely and accurate tsunami detection, measurement, and forecasts. Because of these advances in detection and measurement, the opportunity exists to greatly reduce and/or quantify uncertainties associated with forecasting tsunamis. Providing more timely and accurate information related to tsunami location, arrival time, height, inundation, and duration would improve public trust and confidence and fundamentally alter tsunami emergency response. Additionally, this capability could be integrated with related fields (e.g., storm surge, sea-level rise, tide predictions, and ocean forecasting) to develop and deploy one continuous, real-time, accurate depiction of the always moving boundary that separates ocean from coast and, sometimes, life from death.
Introduction
To emergency managers charged with protecting populations from weather-related hazards, uncertainty is a formidable challenge. Numerical weather prediction models require detailed initialization data, and associated instrumentation, over massive domains to produce accurate forecasts. Plus, no matter how well these models represent initial conditions, uncertainties inevitably grow over the period of a model run. Resulting errors create public doubt and can lead to inconsistent responses no matter how much urgency emergency managers convey.
Similar challenges exist in the tsunami community, though the uncertainties manifest differently. Tsunamis propagate and amplify at reliable rates in the deep ocean based on depth. Assuming all other variables are known, tsunami wave heights at any time and location in the open ocean can be reliably traced back to the wave conditions at the source.1 Accurately defining these conditions, the source parameters, is therefore of utmost importance.
We recognize there are other sources of forecast errors unrelated to the tsunami source that must be considered. The complex modification of tsunami behavior when it reaches shallow water due to non-linear interactions and the often sparse coverage of high-resolution bathymetric data can lead to significant differences in forecast versus observed coastal wave heights. Reducing these errors will require dedicated, multiscale coastal mapping efforts, higher density of coastal observation systems, and increased sophistication of numerical simulations. The primary focus of this paper, however, is to comprehensively address one of the major limitations to accurate tsunami forecasts: the inability to quickly measure and represent the tsunami source.
Although principles of tsunami generation and propagation have long been understood, before the 2004 Indian Ocean tsunami, little real-time information was available regarding tsunami size and character, even for large ocean-wide tsunamis (Bernard et al., 2010). This was because there was no comprehensive global tsunami detection and measurement capability. There was no way to directly observe tsunamis until they reached coastal sea-level stations. Even then, data were typically not available in real time. It often took a day or more to accurately reconstruct critical tsunami source parameters using retrospective seismic analysis and sea-level observations (see orange line, Figure 1).
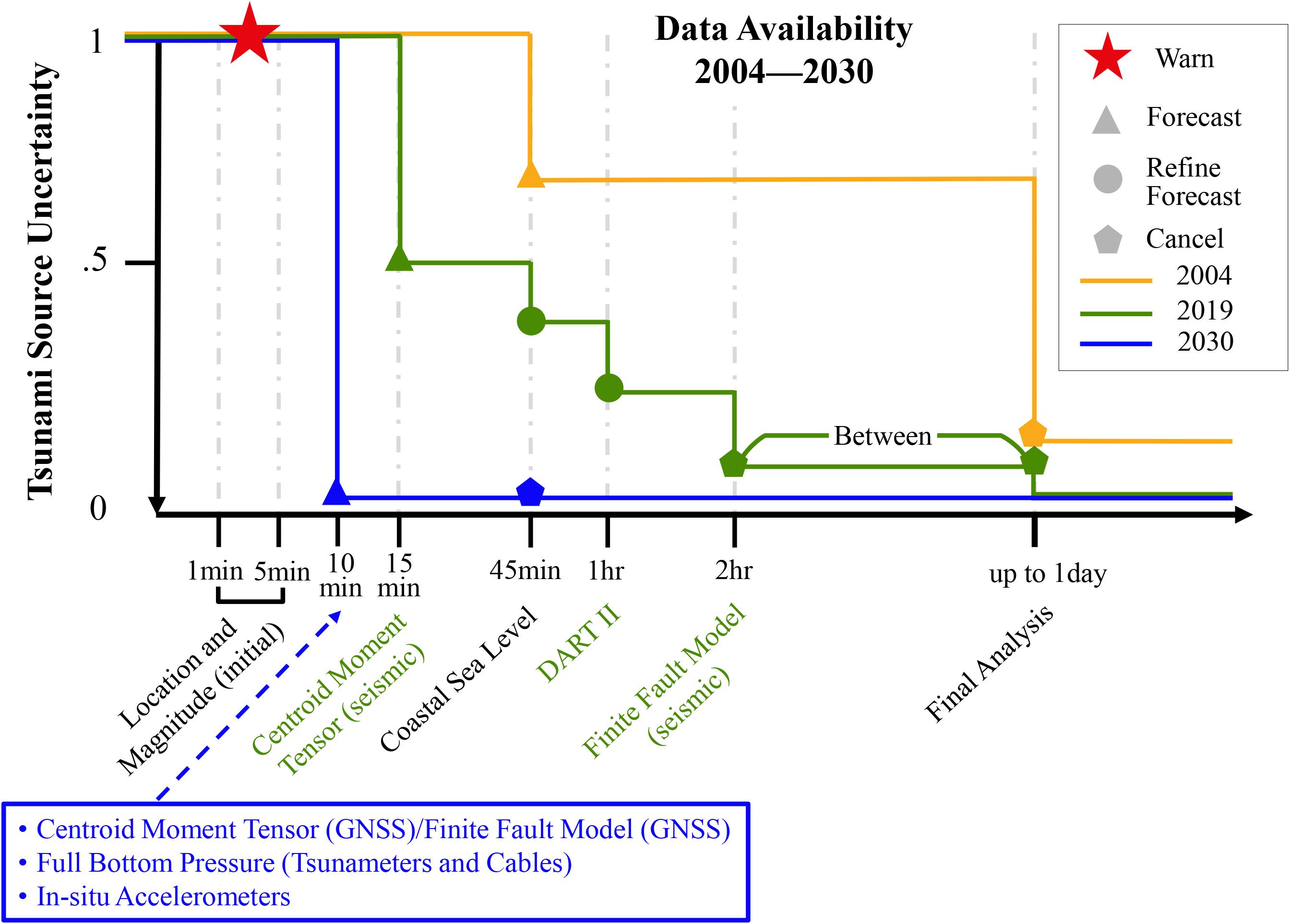
Figure 1. Generalized relationship between tsunami source uncertainty and time after earthquake origin for three different time frames. The orange line represents tsunami source uncertainty levels prior to 2004, the green line represents tsunami source uncertainty levels at present (2019), and the blue line represents tsunami source uncertainty levels achievable with the ocean sensing and analysis techniques advocated for in this paper. Initial earthquake location and magnitude is considered “fully uncertain” in terms of solving tsunami source parameters for the purposes of this depiction.
Fortunately, great strides have been made to reduce forecast uncertainties for tsunamis generated more than 3 h tsunami travel time away (distant-source or far-field tsunamis) (Bernard and Titov, 2015). Since the 2004 Indian Ocean tsunami, global tsunami detection, forecast, and warning capabilities have significantly expanded due to increased governmental support and international collaboration and data sharing. Greater availability of real-time seismic data and enhanced seismic analysis now enable estimation of earthquake forcing mechanisms within 2–3 h, and sometimes much sooner (see green line, Figure 1). Approximately 60 deep-ocean observation buoy systems and hundreds of tsunami-capable coastal sea-level stations ensure most large tsunamis will be detected and measured with sufficient time to alert distant coastlines.
Once measured in the deep ocean, tsunami arrival time, height, and duration at the coast can be accurately forecast using shallow-water wave equations (Thomson et al., 2011; Rabinovich et al., 2013). Given sufficient bathymetric data and computational resources, inundation can also be reliably calculated at the coast by nested-grid non-linear modeling (Hébert et al., 2001). Notably, lack of high-resolution bathymetric data is a problem for many ocean forecasting applications, including, tsunami warning.
Despite recent improvements to sensing and analysis capabilities, there are still large forecast uncertainties in the near field, where local-source tsunamis occur. Such tsunamis are particularly dangerous because they may reach a coast in less than 1 h, often less than 30 min, after generation. In extreme cases, they may strike in as little as 5–10 min.
Since it presently takes hours to precisely determine an earthquake’s forcing mechanism, and observations are limited, emergency managers, particularly in the near field, face large impact uncertainties when deciding how to protect their communities. They must develop and execute preplanned protocols based on broad—sometimes false—assumptions about a tsunami’s potential height and inundation. This can lead to over or under warning, the latter being particularly dangerous.
Fortunately, methods to more precisely and quickly measure tsunami sources are emerging. This will allow for development and delivery of accurate forecasts in time for emergency managers to take decisive action (Williamson and Newman, 2018). However, realizing this vision will require a reimagining and realignment of techniques, procedures, and observation networks.
Rapid seismic analysis is critical for issuing initial alerts, and efforts to refine and accelerate these techniques remain crucial. To directly infer tsunami sources in real time, however, more than traditional seismic analysis is needed.
This includes augmenting traditional seismic analysis with emerging capabilities, like using ground displacement data from the ever-expanding Global Navigation Satellite System (GNSS). We expect this to lead to more reliable tsunami source estimates where geography and/or instrument density support it.
In locations not supported by land-based GNSS-derived estimates, some countries are deploying advanced bottom pressure recorders (BPRs) on stand-alone deep-ocean buoy systems and, in Japan, Canada, and the United States, on limited-area cabled observation systems (Thomson et al., 2011; Rabinovich and Eblé, 2015). These instruments filter out seismic noise, allowing their placement much closer to likely seismic sources in the deep ocean, thereby reducing the time to measure the first wave from over an hour to tens of minutes.
This deep-ocean sensing strategy must be supported by high-density coastal observational data (Intergovernmental Oceanographic Commission [IOC], 2006, 2009, 2016). An expanded array of tsunami-capable coastal sea-level stations would help constrain tsunami sources and provide verification of real-time coastal wave height and inundation forecasts.
Most of these capabilities are currently available to the global tsunami warning and mitigation system or will be soon. When fully implemented, we expect a significant drop in tsunami source uncertainty, which will allow production of a dynamically based tsunami forecast within 10–15 min of tsunami generation (provided it is generated within a known source area).
As promising as these capabilities are, to more accurately measure and characterize all tsunami sources, we must look beyond them. Land-based GNSS and seismic processing techniques cannot directly measure seafloor deformation in most cases. This requires an in situ submarine network of localized, real-time displacement sensors deployed directly over a source.
Government and commercial entities regularly deploy submarine fiber-optic cables that could be important contributors to real-time tsunami detection and measurement. These cables cover key portions of nearly every tsunamigenic submarine zone in the world. Instrumenting these cables with real-time BPRs would greatly enhance the ability to verify wave propagation data and assimilate them into forecast models (Howe et al., 2018).
Direct instrumentation of fiber-optic cables requires commitments and resources beyond what regional tsunami warning and mitigation systems could provide on their own. Still, we can imagine a dynamic network of ocean observing systems (e.g., instrumented fiber-optic cables augmented by advanced stand-alone deep-ocean buoy systems) that could greatly reduce tsunami source uncertainty within minutes of generation, regardless of source location. If realized, the global tsunami warning and mitigation system would be able to deliver meaningful and accurate hydrodynamic tsunami forecasts within 10 min of generation (see blue line, Figure 1).
Other emerging techniques may also support the tsunami warning process. Satellite altimetry, infrasound arrays, and coastal high-frequency radar can provide important information (e.g., Barrick, 1979). A tsunami’s distinct signature can even be detected in the ionosphere.
We are careful not to oversimplify the threat or overpromise the timeliness and accuracy that tsunami warning centers can deliver. There will always be locations too close to a tsunami source to consider anything other than full evacuation once a potentially tsunamigenic earthquake is detected. But for most tsunami-vulnerable coastlines, being able to accurately determine tsunami source parameters, in the targeted time frame, would drastically change how emergency managers coordinate and execute their responses.
Emergency managers would no longer need to consider multiple scenarios to account for uncertainties. More importantly, the public could respond more quickly and appropriately based on improved trust and confidence in the alerts. Ultimately, these changes could save lives.
Nevertheless, significant obstacles remain. For instance, few countries can afford to develop and deploy dedicated tsunami observation systems. Traditionally, fielded ocean observing systems are operated by commercial enterprises and academic organizations for non-tsunami-specific purposes. There are likely limited incentives for them to add such instrumentation to their systems much less operationally support it. Even if a global observational grid of sufficient density to fully constrain tsunami sources in real time was achieved, the need to condition the public to act quickly and appropriately based on the enhanced products would remain.
Despite these challenges, there has never been a greater opportunity to pursue sweeping tsunami forecast improvements, especially in the near field. This paper describes how the global tsunami warning and mitigation system could achieve such improvements.
Benefits of Reducing Tsunami Forecast Uncertainty
Reducing tsunami forecast uncertainty supports all aspects of emergency management. Ultimately, it will provide assurance to at-risk communities and help them with their decision-making and community response.
In the context of tsunami emergency and risk management, timely response is critical to saving lives and preventing loss. Political leaders and the public expect timely, accurate, and effective tsunami alerts. However, these expectations cannot currently be fully met. While considerable improvements to tsunami warning and mitigation systems have saved many lives, recent tsunamis have still resulted in significant casualties or unnecessary evacuations and subsequent complacency. Examples of such events include:
• February 27, 2010 M8.82 Maule, Chile earthquake and tsunami—156 deaths (National Centers for Environmental Information/World Data Service [NCEI/WDS], n.d.).
• March 11, 2011 M9.1 Tōhoku, Japan earthquake and tsunami—18,453 deaths (National Centers for Environmental Information/World Data Service [NCEI/WDS], n.d.).
• November 13, 2016 M7.8 Kaikoura, New Zealand earthquake and tsunami—Late threat identification, protracted warnings, inconsistent response.
• September 8, 2017 M8.2 Chiapas, Mexico earthquake—Uncertain threat resulting in protracted or unnecessary warnings that caused disruption.
• January 23, 2018 M7.9 Kodiak Island, Alaska, United States earthquake—Uncertain threat resulting in protracted or unnecessary warnings that caused disruption.
This section describes the landscape within which the tsunami emergency and risk management community currently operates and how it could be improved if tsunami warning centers were able to deliver timelier, more accurate forecasts.
Emergency Response
The Problem
Tsunamis are low-frequency, high-consequence events that can cause widespread loss of life, injuries, and physical and environmental damage and disruption. Because of the possibility of devastating impacts, tsunami warning centers and emergency management agencies treat the potential for a tsunami seriously.
As suggested in this section’s introduction, a large submarine earthquake often serves as the first indicator of a potential tsunami. When such an earthquake occurs near a coast, which is the case most of the time due to the location of tectonic plate boundaries (the primary source of tsunamigenic earthquakes), warning centers have mere minutes to identify and assess the source and provide alerts before the first waves arrive. Even for communities farther away from a tsunami source, time for accurate assessments is limited as tsunamis can travel at speeds of over 800 km/h in the deep ocean.
Once a tsunami warning has been issued, community evacuation can take considerable time. So warning centers and emergency management agencies and authorities nearest an earthquake’s epicenter have to act quickly and make initial warning decisions based solely on estimated earthquake location, magnitude, and depth. These preliminary assessments are inevitably relatively crude and contain a significant degree of uncertainty. For this reason, emergency managers use cautious preplanned responses as the basis for their initial decisions. This often results in under, protracted, or over warning, each of which may have negative repercussions.
It takes considerable time—often hours—after a warning is first issued before observations are made and the threat is more fully understood. This often results in cancellation of the warning, but in the interim leads to unnecessary widespread disruption, including over evacuation, economic loss, mass inconvenience, and inconsistent responses. The cumulative effect is diminished public confidence in official alerts and the responsible authorities.
The Need
For alerts to be effective, they must be timely, relevant, accurate, detailed, clear, effectively communicated, and trusted. The first three of these elements (timely, relevant, and accurate) result directly from how well the tsunami source is understood and characterized, while the remaining four elements (detailed, clear, effectively communicated, and trusted) have large dependencies on the first three. We therefore consider the first three in detail:
• Timely: For emergency managers and the public to be able to take timely action during a tsunami, especially a local-source tsunami, warning centers need to be able to issue a “best estimate” forecast within 5–10 min of an earthquake based on all available information.3 To be relevant and accurate, this forecast requires a relatively high degree of certainty. However, in general, warning centers cannot provide this level of certainty within 10 min due to current challenges related to tsunami source characterization and observational gaps noted in this paper’s introduction.
• Relevant: From an emergency management perspective, relevance is determined by the nature and extent of the tsunami threat, which may range from strong currents and anomalous ocean conditions to significant land inundation. The decision to issue alerts and the associated advice and instructions must therefore be relevant to each situation. For example, if inundation is forecast, instructions to immediately evacuate to high ground may be appropriate. Alternatively, if strong currents are anticipated but there is not a perceived threat to land, a notice advising caution in and around the water may suffice. In response to a potential tsunami threat, emergency managers need to decide what actions to take and the instructions to issue to their constituents, if any. Existing forecast uncertainties complicate these decisions.
• Accurate: When a tsunami threatens, emergency managers and the supported public require a forecast that accurately identifies the scope and scale of the event and associated alert level(s). Accurate forecasts can help communities avoid unnecessary disruption while at the same time ensure response resources are directed and focused on the most at-risk areas. Forecast accuracy is particularly important in urban areas and tourist destinations, where overestimating the threat can strain the usually limited emergency management resources available at short notice and cause ancillary safety concerns. Currently, warning centers, in general, are not able to provide enough information about the level or extent of inundation within the desired time frame (i.e., within 5–10 min of event origin) to support effective response.
Application of More Timely, Higher Certainty Forecasts
The quality of public alerts largely depends on the three elements described in the previous subsection. Once the challenges to these elements are overcome, emergency managers will be confident that during a tsunami they will receive information in a timely manner and with a degree of certainty that supports decision-making. This confidence will enable them to refine their planning and procedures to support better responses. They will be able to tailor public alerts to the required level of detail and provide enough specificity in their instructions. Effective alerts, in turn, will enhance public and political confidence in the responsible authorities, underpin successful community response, and, ultimately, save lives.
Nevertheless, albeit to a lesser degree, some uncertainties will remain. Emergency managers and the public will need to be made aware of these uncertainties so they can consider them in their decision-making.
Tsunami Risk Management
What we know about tsunamis is important for managing the risk before disaster strikes. Tsunami hazard and risk assessment (e.g., inundation and evacuation modeling) depends on scientific information, and even the most sophisticated scientific knowledge has some degree of uncertainty. This does not mean the information is unreliable, but this uncertainty strongly influences the risk management process.
Risk management is by its very nature about managing uncertainty. Decision makers have been writing policy, developing management frameworks, and issuing advice to protect at-risk communities for a long time based on limited information or uncertain hazard and risk assessments.
Addressing uncertainty associated with tsunamis extends beyond emergency response. Better characterization of tsunami sources is an important preparedness activity that, once fully realized, will contribute to a better understanding of the risk from local-, regional-,4 and distant-source tsunamis. This will allow for risk-informed decision-making in all aspects of emergency management.
In 2011, the Tōhoku, Japan earthquake and tsunami exceeded the previously recognized level of risk. Despite the region’s high level of tsunami preparedness, the tsunami protection measures (e.g., barriers and evacuation planning) were insufficient (Mori et al., 2011; Suppasri et al., 2012). This event clearly illustrates the high degree of uncertainty that still exists in tsunami hazard and risk assessments used for pre-event decision-making. As such, emergency management and monitoring agencies have to acknowledge and accommodate uncertainty in their tsunami response planning activities.
End-to-End Benefits of Reducing Uncertainty
In summary, while our understanding of tsunamis will continue to evolve and challenge how we detect and measure tsunami sources and forecast the resulting waves, it is important to acknowledge that 100% certainty may never be achieved. Still, coordinated efforts to deliver more accurate tsunami impact forecasts would fundamentally improve the end-to-end tsunami risk and emergency management system. Benefits include the following:
• More decisive and effective public response to emergency management direction: Consistently demonstrating that emergency management instructions are appropriate and based on high-confidence forecasts can instill confidence in the public to act decisively when a tsunami threatens.
• More refined tsunami hazard and risk assessments linked to tsunami forecasts: A key requirement for effective preparedness and disaster reduction is an understanding of how a tsunami will impact individual coastlines before it strikes.
• Improved community preparedness: Refined, accurate tsunami inundation and evacuation modeling through better-informed scenario simulations can support actions before and after a tsunami emergency.
• Strengthened public education programs and messaging: The more that is known about potential tsunami impacts, the better education programs and action messages can be tailored to ensure official messages are relied on as trusted and accurate.
• Assured governments and policy makers: More confidence and certainty provide transparency and trust when decisions on processes, policies, plans, risk management frameworks, or investments depend on reliable, risk-based information.
• Increased investment in risk reduction, ocean-based science research, and targeted mitigation strategies: The prospect of reduced uncertainties associated with tsunami forecasts may make research sponsors more likely to support tsunami-focused applications and broader sea-level-related activities.
The Sendai Framework for Disaster Risk Reduction
Focusing on reducing tsunami forecast uncertainty also supports the goals and intentions of the Sendai Framework for Disaster Risk Reduction5 (agreed to internationally by Member States). Specifically relevant is “Target G: Substantially increase the availability of and access to multi-hazard early warning systems and disaster risk information and assessments to the people by 2030.” The framework aims to achieve “substantial reduction of disaster risk and loss to life, livelihoods and health, and in the economic, physical, social, cultural and environmental assets of persons, businesses, communities and countries.” Key components of Target G focus on improved detection, monitoring, analysis, and forecasting of hazards and possible consequences, and dissemination and communication, by an official source, of authoritative, timely, accurate, and actionable alerts and associated information on likelihood and impact (United Nations International Strategy for Disaster Reduction [UNISDR], 2015).
Addressing Tsunami Detection, Measurement, and Forecasting Uncertainties
In 2004, the tsunami threat in the Pacific was acknowledged and much better understood than in the Indian Ocean given its recent history of large tsunamis and basin-wide warning system. At that time, tsunami forecasts and alerts were most effective for distant-source tsunamis, were based primarily on preconceived assumptions and historical analogs, and were very broad-brush, which often resulted in over warning. Advancements since 2004 include methods for better tsunami source characterization (mainly through more sophisticated seismic analysis) that in combination with faster numerical tsunami forecast models and denser sea-level observation networks significantly improved the global tsunami warning and mitigation system’s distant-source tsunami capability.
The opportunity now exists to greatly enhance this capability and to produce more accurate, near-real-time inundation forecasts for local-source tsunamis. In this section, we describe recent and coming advances that could result in fundamental improvements to the ability to more rapidly and accurately constrain tsunami sources, thereby greatly reducing tsunami forecast errors. Of course, no technique for characterizing tsunamis stands alone. The observation systems we currently collectively leverage, or plan to, are listed in Tables 1A,B and discussed in the following subsections.
Inferring Tsunami Source Parameters From Solid-Earth Measurements
If key tsunami source parameters, including generating mechanisms, are known, then a tsunami can be computed with reasonable accuracy. Most often, the mechanism is vertical displacement of the sea over a large area caused by an earthquake. To accurately forecast tsunami impacts at the coast following a large earthquake, warning centers need to estimate how the earthquake deformed the seafloor. Tsunami forecast models can then simulate tsunami propagation toward coastlines and guide warning decisions. Model guidance can also inform decisions for coasts that should be unaffected, allowing activities there to proceed without disruption.
Outside of a few limited areas, there are currently no practical ways to directly measure earthquake-induced seafloor displacement. It must be inferred from other data. Procedures for approximating a tsunami source from an earthquake’s seismic signals have evolved over many decades.
Today, seismic waves can be analyzed quickly to determine an earthquake’s preliminary location and depth (hypocenter), magnitude, and origin time. Assuming an earthquake ruptures with the same mechanism as past large earthquakes in its hypocentral region, the rupture is largely uniform across the fault, and the hypocenter represents the center of the rupture, seafloor deformation can be estimated. However, this initial estimation has large uncertainties because the actual fault mechanism may be different, the rupture may not be uniform, the earthquake may propagate along the fault, and the hypocenter may not be where the main rupture initiated. These uncertainties are even more typical and problematic for very large events (i.e., those greater than M8.6).
Research aimed at better inferring fault and rupture planes using seismic analysis is promising, but implementation is not currently widespread. Even when these improved rapid seismic assessment techniques are applied, significant initial uncertainties will remain, so tsunami forecasts based solely on this type of analysis must be used with caution.
Additional seismic analysis normally produces a centroid moment tensor (CMT)—the first indication of an earthquake’s mechanism—in 20–30 min. For earthquakes with moment magnitudes6 from the M7s to lower M8s, the CMT information is usually sufficient to estimate seafloor deformation for tsunami forecast purposes. In most cases, the fault ruptures of these earthquakes are not so large (a few tens to a couple hundred kilometers) that rupture disparities significantly affect tsunamis.
However, for the largest earthquakes, ruptures measure from hundreds to more than a thousand kilometers. For these events, the aforementioned seismic analyses are inadequate. They do not describe earthquake complexities (e.g., homogeneous vs. complex slip distribution) with enough detail to accurately reproduce a tsunami in a forecast model in a timely manner, particularly in the near field. Hébert et al. (2007) and Hébert and Schindelé (2015) showed that for regional- and distant-source tsunamis, wave height estimates based on an homogeneous source could be as much as two to three times different than those based on a complex slip distribution source.
Therefore, new data and methods—seismic and non-seismic—are needed to more precisely characterize rupture complexities of very large earthquakes within a few minutes to produce more accurate tsunami forecasts from numerical models.
Seismic Analysis
Earthquakes generate more than 80% of all tsunamis worldwide (National Centers for Environmental Information/World Data Service [NCEI/WDS], n.d.) and are responsible for the majority of deadly tsunamis in recorded history. Seismology provides the first indication that a potentially tsunamigenic earthquake has occurred. Seismic waves travel much faster than tsunami waves, and the dense global network of seismic stations enables detection and location of most tsunamigenic earthquakes within about 5 min, anywhere on Earth.
Once a potentially tsunamigenic earthquake is detected, warning centers aim to disseminate tsunami forecasts as soon as possible. Numerical forecast models rely on the estimation of seafloor displacement (Okada, 1985) or measurement of sea-level changes. Since direct measurements are not available in the early stages of an event, seismological proxies of seafloor uplift based on analysis of fault location, size, and type are used to infer those changes and drive initial forecasts.
Thus, the seismic source must be characterized as soon as possible. This process currently involves the following:
• Stage 1 (0–5 min): Detection of earthquake: First indication of tsunamigenic potential based on limited observation. Initial seismic analysis, including very uncertain estimates of size (magnitude), location, and tsunamigenic potential.
• Stage 2 (5–10 min): Confirmed basic earthquake parameters: Improved estimation of location, depth, and size (magnitude) based on enough observations to begin to decrease the uncertainty of tsunamigenic potential. Initial issuance of tsunami alerts using assumptions of earthquake type based on the source region’s tectonics is possible.
• Stage 3 (20–30 min): Improved seismic source parameters: Source characterization, including good estimates of location, depth, size (moment), fault angles (strike, dip, rake), type (strike-slip, normal, or reverse), and slowness, but not extent or rupture details. This allows generation of the first reliable tsunami forecasts.
• Stage 4 (hours to days): Full characterization of seismic source rupture parameters: including location, depth, size (width, length), fault angles and type, geometry, extent, and rupture details, including amount and variation of slip. This allows generation of very reliable tsunami forecasts.
After these stages, seismological uncertainty is as low as possible. Any further significant reduction of uncertainty requires dedicated research.
Although the stages are sequential, the total elapsed time is what matters most. This is where research can deliver large gains. The challenge is to get to Stage 4 much more quickly. The suggested maximum elapsed time is as close to 5 min as possible, but the target for the next decade is 10 min (Intergovernmental Oceanographic Commission [IOC], 2018). This target is unlikely to be met using seismology alone. However, use of seismology in combination with other techniques and technologies [see section “Global Navigation Satellite System (GNSS)”] can be a major factor in reducing tsunami source uncertainty.
Nevertheless, in the last decade, huge advances have been made in seismic analysis techniques used to describe earthquake source parameters more accurately and with greater speed, and this trend is expected to continue. Examples of developing techniques include the use of strong-motion centroid and seismic energy back-projection techniques, which can be used to provide better estimates of the size and geometry of an earthquake’s rupture. Appendix contains more information and references about these developments and other examples of seismic analysis techniques and developments being applied to quickly determine an earthquake’s tsunamigenic potential.
Global Navigation Satellite System (GNSS)
A large number of GNSS stations currently produce continuous positions for many purposes. Of these stations, an estimated 3,500 could support tsunami warning by helping forecasters infer seafloor displacement in real time (Timothy Melbourne, personal communication, June 5, 2018) (see Figure 2). Location and data availability are key constraints on the usability of these stations for this purpose. To use GNSS data to characterize tsunamigenic earthquakes, stations must be near potential tsunami sources. While a significant number of stations are used for tectonic research, not all currently report in real time. To be useful for tsunami warning purposes, GNSS stations typically feature a 1-Hz sampling rate with a delay in the transmission of processed data of approximately 5 s.
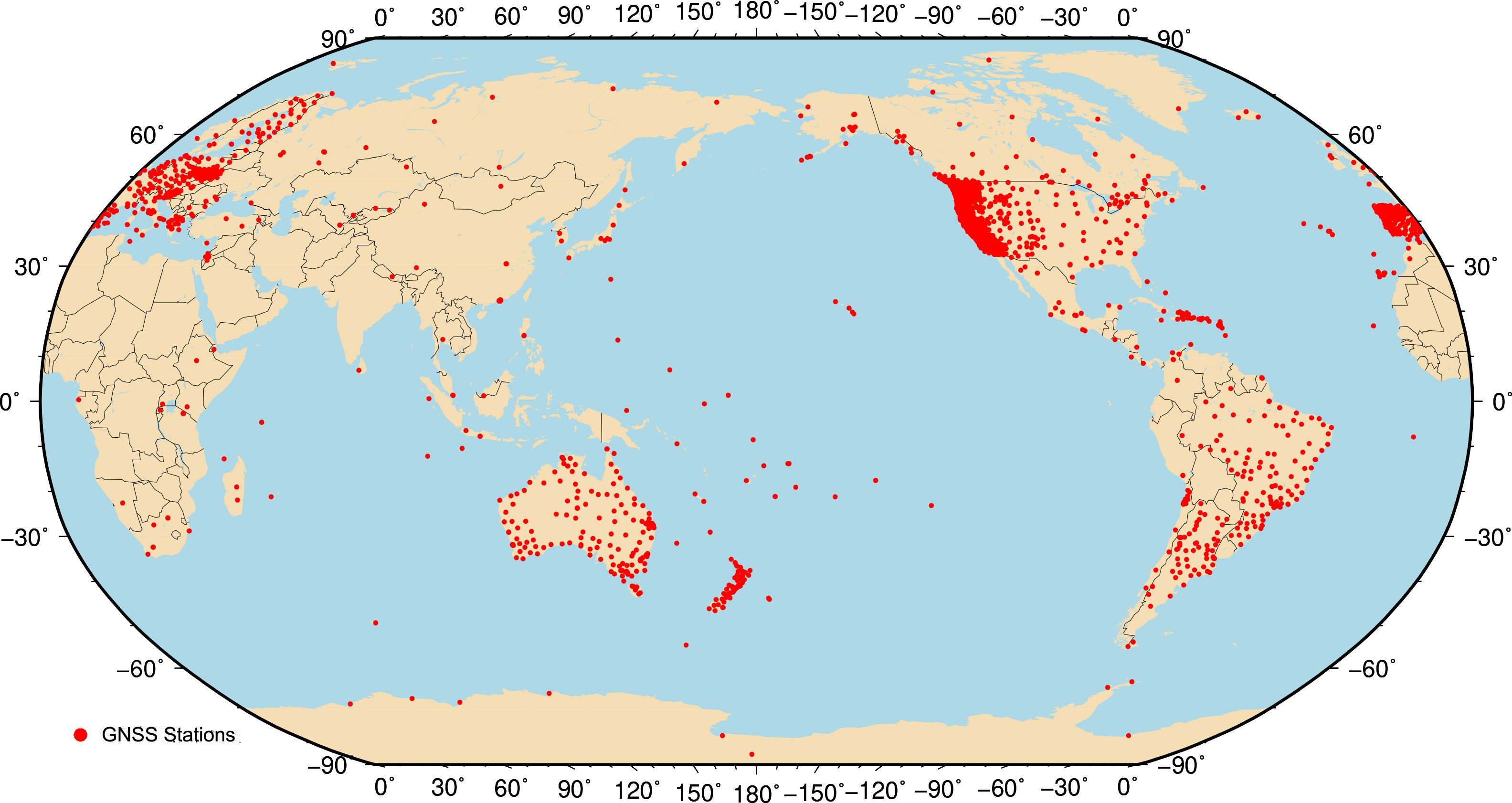
Figure 2. This map illustrates the location of 2,260 real-time GNSS stations from public networks around the world. GNSS stations in non-public networks (e.g., in Japan, Mexico, and Chile) are not shown here but could also support tsunami warning. Source: Pacific Northwest Geodetic Array/Central Washington University.
Several tsunami warning centers are currently investigating use of GNSS-derived earthquake magnitudes and other fault parameters. The initial focus of the United States warning centers is on the Cascadia subduction zone, the potential source of a large earthquake that would have the greatest tsunami impact on United States and Canadian coasts. Once fully implemented, this methodology could be extended to other tsunami source zones where GNSS data exist.
If significant numbers of useable GNSS stations are near a fault zone, then it is possible to produce a finite fault model for an earthquake within a few minutes. Such a model would fully describe the fault parameters, providing spatial detail on the fault geometry and amount of slip and enable seafloor displacement to be inferred. This represents one of the few ways to achieve a very high level of forecast certainty before sea-level measurements are available. Thus, a combination of advances in seismic analysis and GNSS techniques is likely to significantly improve the tsunami warning process in the near future.
The use of GNSS data to derive a seismic source necessary for tsunami forecasting is expected to proceed in three steps. During Step 1, an estimate of earthquake magnitude will be computed based on ground displacement (peak ground displacement magnitude, Mpgd) and hypocenter location. The Mpgd technique has demonstrated it is capable of consistently producing quick and accurate magnitude estimates for earthquakes larger than M6.0 (Melgar et al., 2015).
Step 2 will be initiated before completion of Step 1. In Step 2, static offset measurements reported by GNSS stations will be used to compute a CMT solution that contains information about the earthquake’s focal mechanism. This information is vitally important for determining the earthquake’s tsunamigenic potential. Steps 1 and 2 are expected to be completed 2–3 min after first rupture.
Step 3 is also based on the use of GNSS static offsets. It involves performing a non-uniform slip inversion over the region after the CMT solution is generated. The inversion that best fits observations is the preferred solution. The results of this type of analysis, called a finite fault solution, are particularly relevant for earthquakes with slip distributions concentrated on the shallow part of the fault since they have the most potential to generate large tsunamis not adequately captured by the overall CMT solution.
In most cases, Steps 1, 2, and 3 are performed almost simultaneously, and the respective solutions are expected to become available only seconds apart. There may be situations, however, in which an Mpgd solution with an assumed focal mechanism based on a historical catalog of events may be more accurate than a real-time CMT or finite fault solution if the number of available GNSS stations is small.7
Measuring Tsunamis in the Deep Ocean
Even with a well-constrained seismic solution, the correlation to a tsunami is not absolute (Titov et al., 2016). Tsunami parameters are uncertain until direct measurements are available. Typically, these measurements come from bottom pressure sensors contained in a BPR.8
Bottom Pressure Recorders
Deep-ocean BPRs provide direct detection and measurement of tsunamis. These instruments sit on the seafloor and communicate with surface buoys to transmit data in real time. Since the deep-ocean tsunami signal is not contaminated by coastal processes that typically affect coastal sea-level stations, these deep-ocean measurements are invaluable for tsunami warning decision-making and direct verification of tsunami modeling accuracy.
Traditionally, BPRs have been placed on the seafloor as part of discrete, widely spaced buoy systems. Developed by the U.S. National Oceanic and Atmospheric Administration (NOAA), Deep-ocean Assessment and Reporting of Tsunami (DART) buoy systems constituted the first operational network of instruments designed to measure tsunamis in the deep ocean (Bernard and Meinig, 2011; Rabinovich and Eblé, 2015) (see Figure 3). DART systems and similar deep-ocean observation buoy systems are made by a number of manufacturers and are generally called tsunameters. Today, more than 60 individual tsunameters are deployed worldwide.
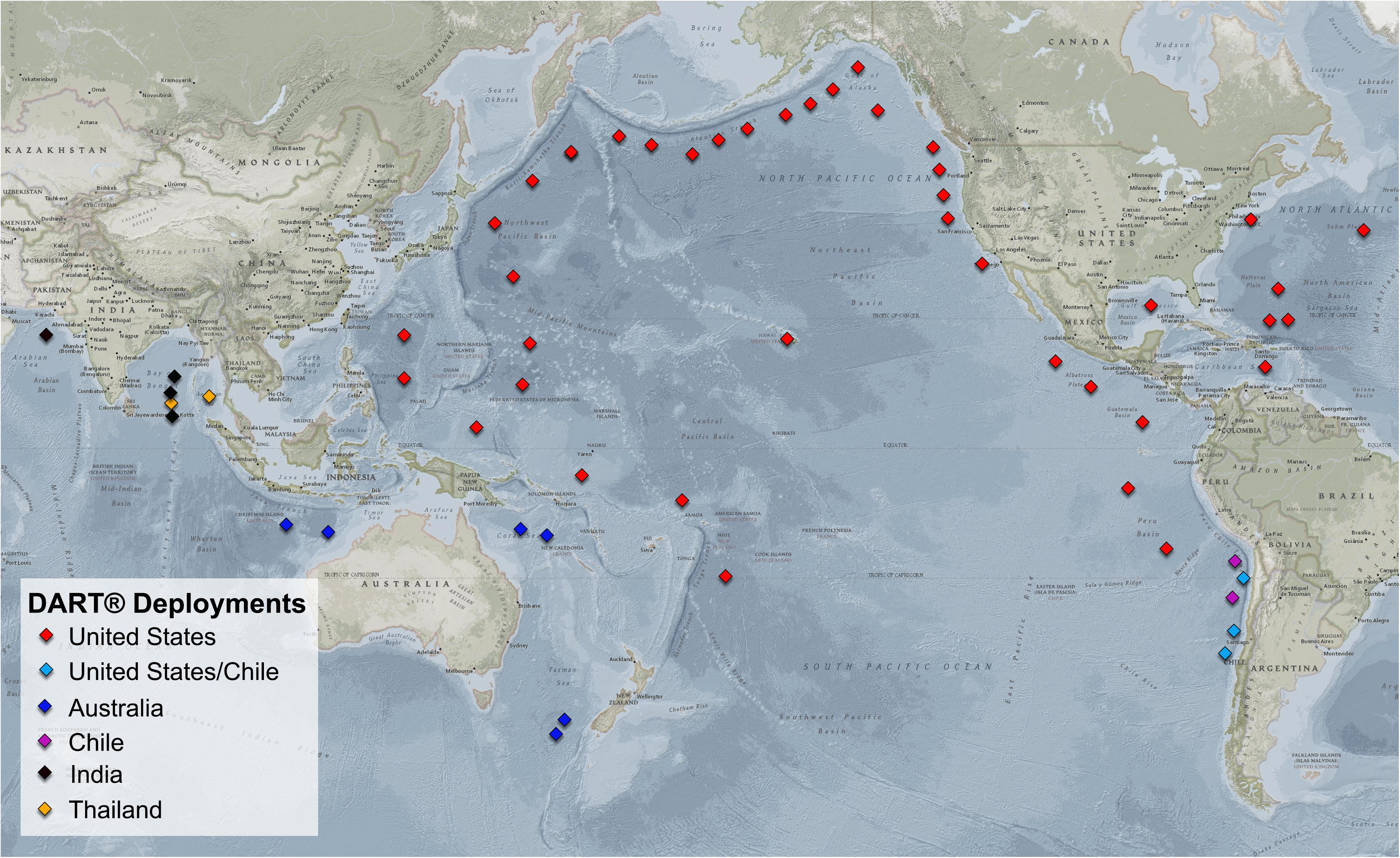
Figure 3. This map provides a visual summary of the DART system network as of November 2018. The DART network includes United States systems as well as systems owned and operated by Australia, Chile, India, and Thailand. Source: Adapted from NOAA National Centers for Environmental Information.
Dedicated undersea cabled observation systems that link BPRs have been successfully installed in Japan, Canada, and the United States and represent a major step forward in early tsunami detection and measurement (Rabinovich and Eblé, 2015). Even more promising is the opportunity to leverage the network of transoceanic fiber-optic submarine telecommunications cables, which are continually refreshed and expanded on 10–20 year cycles. By equipping these cables with environmental sensors such as BPRs and accelerometers, we could see major improvements in the ability to rapidly detect and measure tsunamis in the open ocean (You, 2010; Butler et al., 2014; Howe et al., 2018).
It is important to note a recent development that will likely lead to deployment of more BPRs, some suitable for tsunami warning purposes. A new in situ calibration method has been devised that largely removes sensor calibration drift, reducing it from tens of cm/year to ∼1 mm/year (Wilcock et al., 2017). This development will likely lead to deployment of bottom pressure sensors to monitor ocean circulation and for long-term climate monitoring purposes (Pugh and Woodworth, 2014; Hughes et al., 2018).
Tsunameters
A tsunameter is composed of a surface buoy that contains communications packages and a BPR that measures the pressure on the seafloor created by the weight of the water column above it. A tsunameter can detect and measure tsunamis as small as 1 mm in 6,000 m of water. As tsunami detectors, tsunameters have a number of advantages over traditional coastal sea-level stations:
• Tsunameters are deployed in the deep ocean, far from coastlines that can generate local wave reflections, trapping, local resonance, and other effects, which tend to obscure an original tsunami signal.
• Deployment of tsunameters on the offshore (deep ocean) side of a trench takes advantage of faster deep-ocean propagation speeds, making it possible to record a tsunami signal at a tsunameter before it arrives at a coastal sea-level station.
• Tsunameters support the use of linear inversion techniques (Percival et al., 2009). This allows for much more accurate tsunami source reconstruction than is possible from coastal sea-level station data.
• Tsunameters naturally filter wind waves and other high-frequency noise out of the pressure signal (i.e., mechanical filters or post-processing are not required). This results in a higher quality signal-to-noise ratio, which is key to tsunami detection.
The time it takes a tsunameter to detect a tsunami is based on proximity of the BPR to the tsunami’s source. However, since seismic and tsunami waves are both generated in the source region, most tsunameters are deployed a sufficient distance away from the source to reduce overlap of seismic and tsunami signals. Given the differential in their propagation speeds (∼3 km/s for seismic waves, ∼0.2 km/s for tsunami waves), the waves ultimately separate naturally. Nonetheless, this is a limiting factor in tsunameter positioning as it introduces a time delay of approximately 20–30 min between earthquake origin and tsunami detection (Mofjeld, 2009).
The latest generation of DART systems (DART 4G) is designed to address this latency problem (Meinig et al., 2001; Bernard and Meinig, 2011). DART 4G systems feature a higher sampling rate (1 Hz) than previous generations, which allows for the resolution of both seismic and hydrodynamic waves. The new systems then apply a low-pass filter to the total signal, filtering seismic noise from the tsunami waves. This allows positioning of BPRs much closer to sources (e.g., a subduction zone), eliminating the need to wait for the seismic and hydrodynamic signals to separate naturally.
Cabled observation systems
Connecting BPRs by dedicated undersea cabled observation systems is another way to monitor offshore tsunamis with accuracies equal to or better than stand-alone systems. Because the data-transmission delay is less than 1 s, observation data are available in real time.
A significant advantage of cabled observations is the ability to continuously supply a large amount of power to BPRs since they are connected to land stations through the cable. This allows a constant stream of high-sample-rate data to warning centers. Also, since BPRs are on the seafloor and require no ocean surface communication links (unlike tsunameters), accidents and theft are unlikely. Dense arrays are possible and have been implemented in Japan, as described below, as well as Canada and the United States. The primary infrastructure of these systems is based on submarine telecommunications cabled systems with extremely high reliability (one failure in 25 years).
The disadvantage is the high capital cost. To mitigate this, BPRs must be made as reliable as the basic infrastructure and/or multiple BPRs must be deployed for redundancy and to minimize maintenance costs. Regardless, cabled observation systems likely have lower lifetime costs than alternatives, e.g., buoy-based, ship-maintained systems.
The 2011 Tôhoku, Japan earthquake and tsunami reaffirmed the need for BPRs in Japan. To improve the observation network, the National Research Institute for Earth Science and Disaster Prevention (NIED) installed the Seafloor Observation Network for Earthquakes and Tsunamis along the Japan Trench (S-net) off the Pacific coast of east Japan (Kanazawa, 2013). S-net consists of 150 tsunami observation modules hardwired into the system, five landing stations, and connecting cables providing coverage for the coast and the seafloor near the trench. When fully operational, it is expected that S-net will allow tsunami detection and characterization 25 min earlier than without it.
In addition, the Japan Agency for Marine-Earth Science and Technology installed the Dense Oceanfloor Network System for Earthquakes and Tsunamis (DONET) off the Pacific coast of southwest Japan (Takahashi et al., 2017) (see Figure 4). Operated by NIED, DONET consists of 51 tsunami observation modules with plug-and-play nodes and landing stations (Kaneda, 2013).
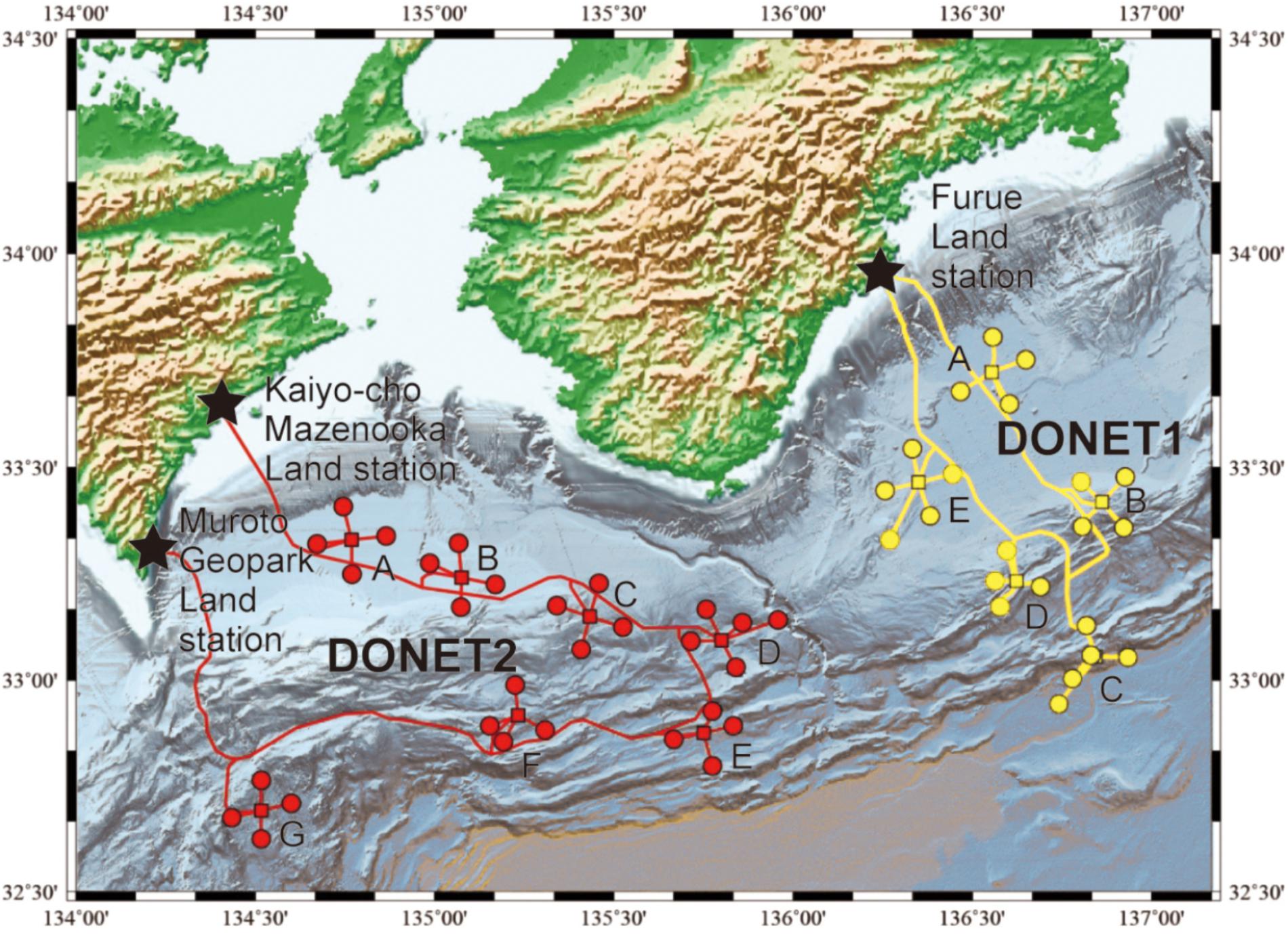
Figure 4. Map of DONET installation. DONET1 and DONET2 observatories are shown with yellow and red circles, respectively. Stars are land stations. Letters indicate nodes (branching apparatuses). Source: Adapted from Takahashi et al. (2017).
During a tsunami, data collected by these cabled observation systems are sent to the Japan Meteorological Agency (JMA), which is responsible for issuing tsunami alerts.
Similar systems, though smaller scale, are deployed in Canada (North East Pacific Time-series Underwater Networked Experiments, or NEPTUNE) and the United States (Ocean Observatories Initiative) (Thomson et al., 2011; Rabinovich et al., 2013; Fine et al., 2015). The U.S. National Tsunami Warning Center is using some of the data from these systems in its forecast and warning operations.
Though effective, due to the costs, it is unlikely that tsunami-dedicated cabled observation systems will ever cover a significant percentage of the world’s tsunamigenic regions. To address this, the International Telecommunication Union, the Intergovernmental Oceanographic Commission of the United Nations Educational, Scientific and Cultural Organization (IOC/UNESCO), and the World Meteorological Organization established a Joint Task Force in 2012 to develop and implement the Science Monitoring and Reliable Telecommunications (SMART) cable initiative.
The goal of the SMART initiative is to integrate scientific sensors for ocean and climate monitoring and disaster risk reduction, including tsunamis, into the repeaters of new fiber-optic telecommunications cables (Howe et al., 2016, Tilmann et al., 2017). Over the normal one- or two-decade refresh cycle for such systems, global coverage could be built up. If industry and government would instrument their fiber-optic cables with BPRs and accelerometers, particularly in locations important for tsunami detection, it would greatly improve the world’s ability to rapidly detect and measure tsunamis (Butler et al., 2014; Barnes et al., 2016; Ranasinghe et al., 2017; Barnes, 2018). Figure 5 illustrates the proximity of existing cable networks to tsunami source areas and existing tsunameters.
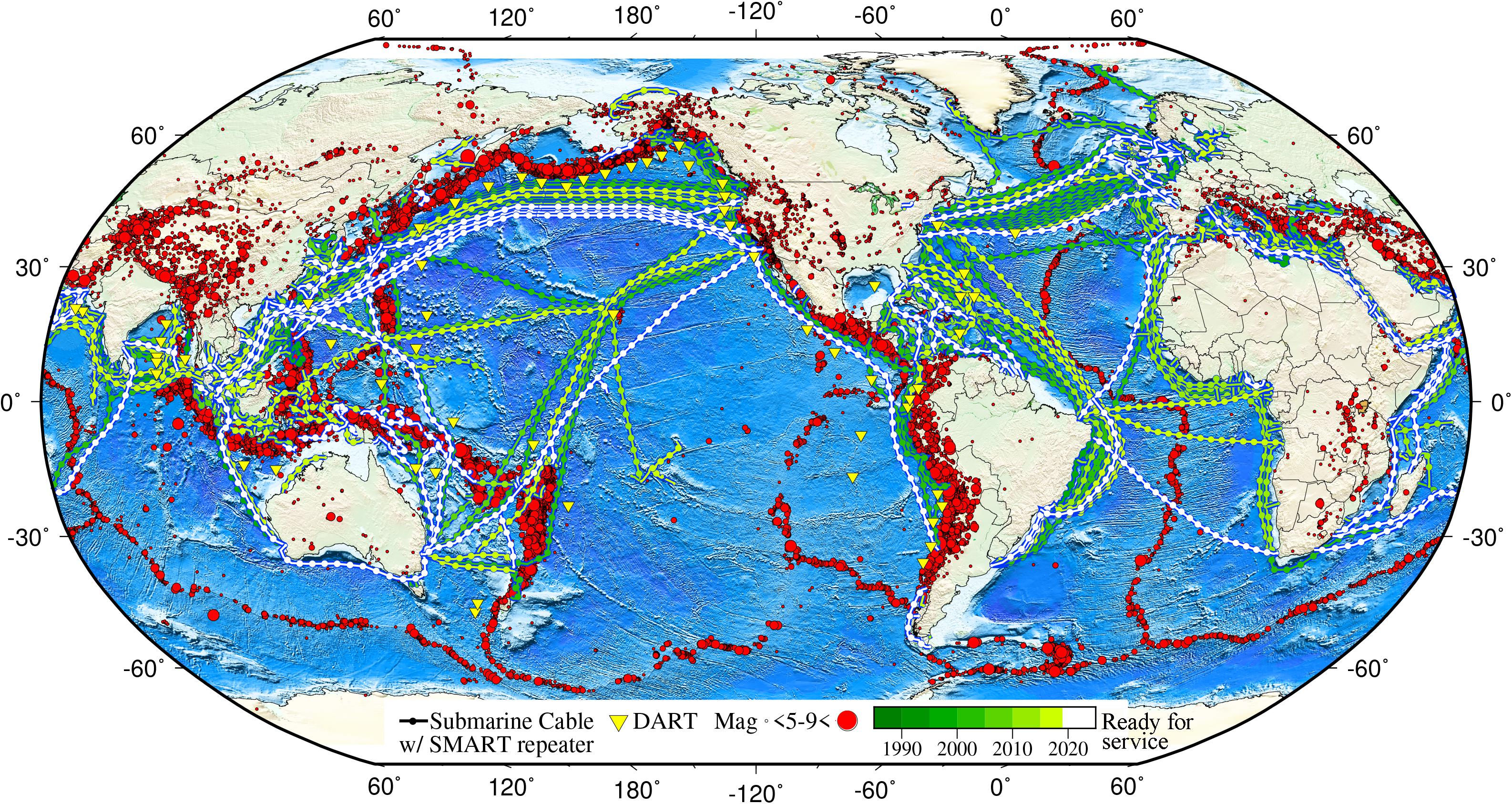
Figure 5. Current and planned submarine cables, locations and magnitudes of historical seismic events, and the existing tsunameter network. Environmental sensors (pressure, acceleration, temperature) can be added to the cable repeaters every ∼100 km, gradually obtaining real-time global coverage (for clarity, repeaters are shown only every 300 km). Map by M. Chandler using GMT. Cable data: TeleGeography’s Telecom Resources licensed under Creative Commons ShareAlike. DART system data: NOAA National Data Buoy Center. Seismic data: United States Geological Survey Earthquake Catalog.
A new distributed sensing technology based on using optical fibers themselves as sensors is also in development. A particularly attractive application includes using a combination of forward transmission optical interferometry and absolute time measurement (Marra et al., 2018) to passively use existing transoceanic fiber-optic cables as a continuously distributed ground deformation sensing network from which seismic or bottom pressure information could be inferred.
Measuring Tsunamis at the Coast
Real-time tsunami observations at the coast are essential for reducing associated tsunami forecast uncertainties and will become more important as techniques to better characterize tsunami sources emerge. Coastal observations are an important means of:
• Verifying tsunami generation and validating the need for alerts,
• Providing information on the actual coastal response to deep-ocean propagating tsunamis,
• Constraining deep-ocean propagation and coastal inundation forecasts,
• Monitoring tsunami propagation across impacted ocean basins,
• Monitoring tides and their addition to or subtraction from tsunami-induced sea-level changes,
• Enabling cancellation of tsunami alerts,
• Guiding local emergency management decision-making regarding “all clear” advice,
• Validating tsunami forecast models in real time,
• Improving forecast accuracy for future events, and
• Evaluating long-term tsunami risk for planning purposes.
Coastal observations of tsunamis are also important for historical record keeping. This informs hazard and risk assessments that help identify at-risk communities and supports community education and preparedness.
Coastal Sea-Level Stations
Sea-level stations have long been used to monitor sea level at the coast to prepare tide predictions at prescribed locations for maritime navigation (Pugh and Woodworth, 2014). They have also proven valuable in documenting sea-level variability and the rate of sea-level change relative to land benchmarks (Church and White, 2011).
Coastal sea-level data that are distributed in real time are extremely valuable for the tsunami warning process, in particular for the verification of tsunami generation and impact on local sea level (see Figure 6). This is especially true as techniques are developed to improve tsunami forecast accuracy through faster source characterization. As modeling and computing capabilities continue to evolve, it may be possible to use data from coastal sea-level stations to further constrain tsunami sources, thereby improving tsunami forecasts, including inundation.
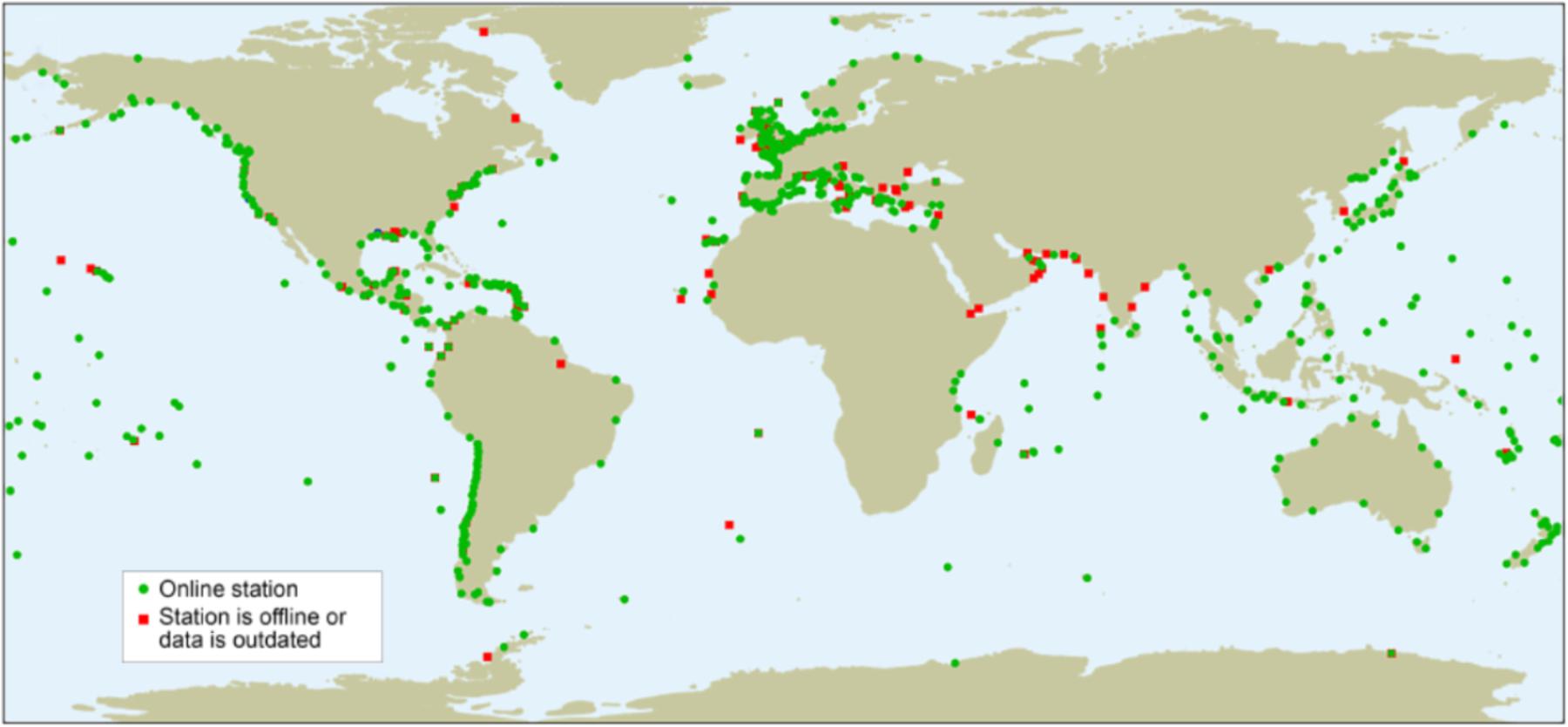
Figure 6. Coverage of available real-time coastal sea-level data (green dots) as of February 2019. (Red dots indicate data outages.) Note large gaps around the world where data is not available in real time. Source: IOC/UNESCO Sea Level Station Monitoring Facility.
Sea-level data have played an important role in the reduction of forecast uncertainties associated with complex bathymetry (Sahal et al., 2009; Vela et al., 2014). In the Pacific, several decades of tsunami records have allowed the application of specific amplification factors at locations with coastal sea-level stations (Jamelot and Reymond, 2015). In these cases, results compare favorably to those obtained by applying a modified Green’s law; observed amplitudes then normally show errors of a factor of less than two.
Elsewhere, where there are no records of tsunamis at coastal sea-level stations, coastal amplification factors have been derived by comparing nested-grid modeling output to modified Green’s law results (Gailler et al., 2018). This method is best suited for small basins like the Mediterranean and Caribbean and near-field coastlines, where nested-grid computations are not feasible in real time.
Once a tsunami alert is issued, warning centers use sea-level data to update (i.e., downgrade, upgrade, expand, narrow) or cancel it. Below are examples of coastal sea-level station observations that influenced tsunami messages.
• On January 10, 2018, an earthquake with an original magnitude of 7.8 registered in the Northwest Caribbean. Within 5 min, the Pacific Tsunami Warning Center (PTWC) issued tsunami messages to its partners in the region. Because of the lack of sea-level data in the epicentral region, it took almost 1 h to confirm tsunami generation, but it was very small and was not a threat. Soon after, the PTWC issued its final messages for the event. A shorter detection time would have reduced the anxiety and actions observed.
• For the M8.6 North Sumatra, Indonesia event of April 11, 2012, and the M7.8 Southwest Sumatra, Indonesia event of March 2, 2016, since the earthquakes were far away from the main fault lines and there were no precomputed scenarios, the Joint Australian Tsunami Warning Centre (JATWC) issued precautionary advice for parts of Australia near the sources in the Indian Ocean. The waves observed at nearby coastal sea-level stations did not meet warning-level criteria, so the JATWC downgraded and then cancelled the alerts as soon as possible to avoid further unnecessary actions from emergency services and the public.
• In the M7.9 Solomon Islands event of February 6, 2013, the JATWC correctly notified the public that there was no threat based on observations from coastal sea-level stations. The continued monitoring of coastal sea-level station data as waves formed and propagated toward Australia helped confirm the accuracy of the original decision as the sea-level anomalies did not meet the criteria for an actionable alert. Coastal sea-level stations from the Solomon Islands to Vanuatu to Australia’s Rosslyn Bay recorded these anomalies.
• In the M8.4 Coquimbo, Chile event on September 16, 2015, the availability of 40 coastal sea-level stations in contrast to the 20 stations available for the M8.8 Maule, Chile event on February 27, 2010, led to more effective messaging and response.
Other Emerging Techniques for Detecting and Measuring Tsunamis
There are a number of other instruments or applications developed for, or capable of, gathering information that can be helpful in detecting and measuring tsunamis. While the following techniques are not expected to support the immediate goal of precise real-time tsunami measurement or source characterization, they are of promising value for event reconstruction (satellite altimetry), confirming tsunami generation (ionospheric perturbations and infrasound), and detecting tsunamis in surface wave reflections (coastal radar).
Satellite Altimetry
The use of satellite altimeters to measure and analyze changes in sea level has been successfully demonstrated following recent tsunamis such as the 2004 Indian Ocean and 2011 Tōhoku, Japan tsunamis (Gower, 2005; Smith et al., 2005; Hébert et al., 2007; Song et al., 2012). Satellite altimeter data are direct measurements of changes in sea level. Consequently, the process to constrain a tsunami source from these data is very similar to that currently employed with tsunameter data by some operational forecast systems.
Satellite altimetry has the advantage of providing both spatial and temporal variation not present in the static time series reported by tsunameters. As a case in point, a number of researchers used sea-level data collected by the Topex-Poseidon, Envisat, and Jason 1 missions processed after the 2004 Indian Ocean tsunami to constrain tsunami initial conditions and model propagation throughout the Indian Ocean (Gower, 2005; Smith et al., 2005; Hébert et al., 2007). Seismologists also used altimetry data to complement seismic observations in characterizing the 2004 earthquake’s source (Geist et al., 2007).
Disadvantages associated with this technology include limitations in sensitivity, limited satellite coverage, and extended data processing times (especially for small tsunamis). Thus, operational use of satellite altimetry in real-time tsunami forecasting is not currently a viable solution.
Ionospheric Perturbations
Tsunamis also create disturbances in the ionosphere and are being captured by the ever-increasing arrays of GNSS, such as the Global Positioning System (GPS), Globalnaya Navigazionnaya Sputnikovaya Sistema (GLONASS), and, in the future, Galileo (Occhipinti et al., 2018; Rakoto et al., 2018). The ability of tsunamis to excite internal gravity waves in the atmosphere has been recognized since the early seventies (Hines, 1972).
Long-period tsunamis transfer a small fraction of their energy to the surrounding air (Artru et al., 2005), generating perturbations that propagate both horizontally and vertically in the atmosphere in the form of internal gravity waves. These waves eventually reach the ionosphere where they can be detected by a number of different sensors. Some of these sensors are mounted on altimetry satellites and GPS-satellite systems that measure total electron content in the ionosphere or on ground-based ionospheric Doppler sounders and airglow cameras.
These technologies have successfully identified and even mapped ionospheric perturbations generated by recent tsunamis (Rolland et al., 2010; Occhipinti, 2015). However, the potential for the use of these observations in real-time tsunami forecasting remains unclear. This is because the horizontal propagation velocity of ionospheric internal gravity waves is similar to that of a propagating tsunami in the deep ocean. Since ionospheric waves have to propagate vertically to the upper layers of the atmosphere, there is an unavoidable delay between tsunami arrival and detection of its trailing internal gravity waves in the ionosphere.
In addition to tsunami-generated internal gravity waves, higher-frequency acoustic waves and acoustic gravity waves are also generated and propagated to the ionosphere by the Rayleigh waves generated at an earthquake’s epicenter. These acoustic waves travel much faster than tsunami waves (∼3.5 km/s) (Occhipinti, 2015) and can potentially supplement seismic information to help characterize the seismic source, thereby contributing to more accurate tsunami forecasts.
Infrasound
It has long been known that earthquakes and tsunamis emit inaudible frequencies (infrasound) that can be detected by infrasound arrays from regional to far distances, as reported by Le Pichon et al. (2002, 2005) in regard to the June 23, 2001 Arequipa, Peru earthquake; March 27, 1964 Alaska, United States earthquake; and August 18, 1959 Montana, United States earthquake. A review of the monitoring results in Diego Garcia also detected the infrasound in the 2004 Indian Ocean earthquake and tsunami. In 2005, the Diego Garcia infrasound station and stations in Palau and Madagascar also detected earthquakes in Nias and Mentawai, Indonesia, on March 28 and April 10, respectively (Garcés et al., 2005).
The idea of using infrasound as part of the global tsunami warning and mitigation system has been widely studied. Bittner et al. (2010) reported that infrasound waves affect the temperature pattern of the stratosphere to an altitude of 87 km. Infrasound waves propagate much faster than tsunami waves, making them attractive to warning centers as an independent means of confirming tsunami generation. However, additional development, testing, and evaluation of the application of infrasound to tsunami detection and forecasting are needed before this technique can be incorporated into tsunami warning center operations.
Coastal Radar
Shore-based high-frequency (HF) radar has proven to be an effective tool to measure ocean currents. The principle is based on the ability of these systems to measure the Doppler shift induced on the radar signal by the orbital velocities of water particles. This alteration of the reflectivity signal can then be inverted to obtain values of ocean current velocities in the radial direction along the line-of-sight of the radar. The use of this technology to detect approaching tsunamis was suggested by Barrick (1979) in the 1970s and has recently received new emphasis following the successful detection of propagating tsunamis in the near and far field during recent events (Hinata et al., 2011; Lipa et al., 2011; Dzvonkovskaya, 2012).
One of the advantages of tsunami detection via HF radar is its ability to provide detection over a large area as opposed to the point-based measurements of other instruments (e.g., tsunameters, GNSS, coastal sea-level stations). Current HF radar systems have a range of several hundreds of kilometers (Grilli et al., 2016) with a wide sweep angle potentially covering a substantial amount of the typical generation area of tsunamis triggered by subduction zone earthquakes.
Based on recent detection of propagating tsunamis, the role of HF radar in tsunami forecasting has been proposed primarily as an early warning tool capable of detecting an approaching tsunami. However, its ability to provide accurate and detailed tsunami height information over an earthquake rupture area still needs to be explored. For tsunami warning centers to use HF radar for this purpose, in addition to the inversion of tsunami velocities from radar signals, a second inversion is necessary to infer tsunami heights from the detected velocity field. However, the ability of current physical models to perform this inversion accurately enough to fully capture all tsunami generation processes at the source remains to be assessed.
Since the 2004 tsunami, interest in HF radar as an additional tool for tsunami detection has increased. Nevertheless, the data are not currently widely used in real-time tsunami forecast and warning operations.
Modeling and Computational Advances
Operational tsunami forecast systems have developed over the last decade following operational deployment of ocean observing systems such as tsunameters and tsunami capable coastal sea-level stations. While such forecast systems can provide forecast solutions based on rapid seismic assessment of tsunamigenic earthquakes, their strength relies on the ability to generate forecast solutions based on sea-level observations collected by tsunameters and reported in real time. It is in this mode of operation that such systems reach their highest levels of accuracy.
Today, one of the most advanced operational tsunami forecast systems is deployed in the United States at NOAA’s tsunami warning centers. The current version of the United States system relies on the linear behavior of tsunamis in the deep ocean to construct an initial ocean surface deformation consistent with tsunameter observations via a linear combination of precomputed tsunami unit sources. Once appropriate initial conditions have been established, a deep-ocean propagation solution is automatically generated from the database of unit sources, while the non-linear tsunami propagation in shallow water and inundation values are computed in real time for select communities (Gica et al., 2008; Titov, 2009; Bernard and Titov, 2015).
There are two advantages to using precomputed unit sources. First, they expedite numerical computation of real-time tsunami propagation in the deep ocean by performing straightforward calculations on readily available solutions. Second, they provide the precomputed time series necessary to perform an inversion from tsunameter data. These time series are combined to fit observations during the inversion process to reconstruct the tsunami source.
There are also two main limitations to using precomputed unit sources. The most significant shortcoming is the possibility of a tsunamigenic earthquake that does not conform to either the parameters or locations in the database of precomputed unit sources (e.g., April 11, 2012 Sumatra, Indonesia; March 2, 2016 Southwest Sumatra, Indonesia; January 23, 2018 Kodiak Island, Alaska, United States). The other important limitation is the current latency of the reception of tsunameter data. Given the current density of tsunameters and the fact that most operational systems do not have the rapid detection capabilities of the DART 4G system, there is an underlying latency in the reception of sea-level observations. This slows formulation of tsunameter-based forecasts and is particularly problematic in the near field.
To improve the capabilities and address the shortcomings of the United States forecast system, state-of-the-art high-performance computing technology is being introduced. In 2019, the United States tsunami warning centers will deploy a new version of the forecast system, which is based on a version of the Method of Splitting Tsunamis (MOST) numerical model (Titov and González, 1997; De la Asunción et al., 2013; Titov et al., 2016) that takes advantage of the massive parallel architecture of modern graphics processing units (GPUs). Other tsunami warning centers in Japan and Europe are in the process of adopting similar GPU technology for tsunami forecasting (González-Vida et al., 2019).
Graphics processing units will allow the forecast system to compute up to 60 times faster than the previous version and are particularly efficient at computing problems with a relatively small computational load and minimal input/output demands but that require a fast solution turnaround (seconds to minutes), as is the case in tsunami forecasting. This means the warning centers can conduct computations in-house significantly faster than at centralized supercomputer centers, which are generally geared toward running models with less stringent turnaround requirements than the warning centers.
These enhanced computing capabilities will address the main two shortcomings associated with using precomputed unit sources by enabling the warning centers to perform real-time deep-ocean computations if a tsunamigenic earthquake does not conform to the hypocentral parameters of precomputed unit sources. In such a situation, the warning centers will be able to calculate a seismic solution (moment tensor or finite fault) or compute a new cluster of unit sources in the region where their analysis suggests the earthquake occurred and then generate a tsunameter-based inversion using the newly computed unit sources in near real time. These two processes could also be combined, generating an initial forecast based on a seismic inversion with updates provided when tsunameter data become available.
In regard to the computation of new clusters of unit sources, the system is being designed to further expedite computations of tsunameter-based inversions. Calculations of each unit source will be restricted in the newly defined cluster to a region around the epicenter that only includes data from tsunameters close to the source. Once a time series for each unit source in this region has been computed and time series from all unit sources at each of the nearby tsunameters are available, an initial ocean surface deformation can be computed and inverted and a single basin-wide tsunami propagation solution can be performed. This will eliminate the need for a full-basin computation from each unit source.
Preliminary implementation of this approach was tested using a set of newly defined unit sources up to 2 h of tsunami travel time away from the epicenter. It demonstrated that a regional solution for each unit source in the new set can be performed in approximately 10 s. These regional computations can be initiated before tsunameter data are available, further reducing the total computational time requirement.
Consequently, the introduction of new high-performance computing capabilities to the United States forecast system, in conjunction with the use of the efficient calculation strategies described above, eliminates the first of the two main shortcomings in today’s forecast system, the handling of events not available in the precomputed database. In addition, it has the potential to largely reduce the problem of rapid forecast availability in the near field when used in combination with other near-field detection systems such as GNSS or DART 4G.
Other Sea-Level and Geophysical Applications
Enhancements to the coverage and real-time availability of sea level (deep ocean and coastal) and GNSS data will provide identifiable multidisciplinary benefits to many other applications. Some of these applications are noted below and in Tables 1A,B.
• Monitoring sea-level variations and inundation for climate research and forecasting.
• Assimilating data to support deep-ocean and coastal forecasting systems.
• Enhancing tide predictions at individual locations for navigation and maritime applications.
• Researching and operationally supporting other coastal hazard applications, such as storm surge inundation and warning.
• Researching changes in deep-ocean properties associated with climate change.
• Defining maritime boundaries more accurately.
• Monitoring tectonic plate movements.
• Conducting geodetic and land information surveys.
• Addressing other geophysical and seismic phenomena.
• Leveraging available ship-time resources for installation and maintenance.
• Improving tide measurements and datums.
• Validating satellite altimeter data.
• Improving understanding of ocean circulation.
• Supporting research and warning on related hazards such as meteotsunamis and infragravity waves.
Challenges, Roadblocks, and Remaining Gaps Related to Reducing Uncertainties
In this paper, we demonstrated that, with sufficient observations, tools exist to greatly reduce long-standing uncertainties associated with tsunami forecasts and that resolving these uncertainties would fundamentally change how emergency managers coordinate tsunami response, particularly in the near field. Today, we have neither all the required data sets nor the means to globally implement these tools. Some of the more pressing challenges are discussed below.
GNSS
We believe focusing on GNSS-derived displacement data rather than traditional seismic analysis is the best near-term strategy for rapidly reducing tsunami source uncertainties. However, the challenges are not trivial.
Coastal GNSS stations must be dense enough and configured so they can reliably represent complex seafloor deformations. The required station density does not exist, and may never exist, along many dangerous subduction zones, such as the Aleutian, Kermadec, and Sumatra trenches. In some locations with high-density coastal GNSS coverage, such as the Japan Trench, the relatively long distance between the coast and the primary tsunami source region limits the degree to which coastal displacements can accurately represent offshore deformation.
Some locations, such as the Cascadia subduction zone, New Zealand’s Hikurangi fault zone, and the Chilean trench, are well suited for GNSS source estimate techniques because there are dense coastal GNSS networks in close proximity to these tsunamigenic regions. However, these are also the locations where tsunami travel times between source and coast are the shortest (Power et al., 2016). It is of utmost importance that tsunami forecast information in these regions is timely and accurate.
Seismology
Although we suggest above a focus on GNSS techniques to reduce uncertainty, the first identification of most tsunamis will continue to rely on seismology. As demonstrated in Section “Seismic Analysis” and Appendix, seismic detection and characterization techniques continue to develop and when combined with GNSS will be important for quickly reducing initial forecast uncertainty. Increasing the timeliness and accuracy of seismic analysis continues to present challenges and may require direct instrumentation of oceanic fault zones.
Land-Based Seismic Stations
Installation of new seismic stations and real-time access to data from more existing seismic stations are needed, particularly in low-density regions. Quicker and more accurate seismic analysis will remain important in issuing the first messages after a potentially tsunamigenic earthquake.
Tsunameters
DART 4G systems eliminate seismic noise, allowing them to be sited closer to tsunami sources to reduce detection times. However, these systems and systems like them are expensive and in some locations may provide more limited coverage than their predecessors, requiring deployment of additional systems.
Coastal Sea-Level Stations
Even if it is possible, through a combination of GNSS techniques and near-field tsunameters, to get to a point where tsunami sources can be mostly constrained within 10 min of origin, a significant level of uncertainty would remain. This is particularly true for complex inundation zones, where uncertainties can become large due to insufficient bathymetric resolution or other complicating factors such as stream outflow. Therefore, real-time coastal sea-level station data remain vital. To further support verification of the new forecasts, access to real-time data from higher densities of coastal sea-level stations is required (Intergovernmental Oceanographic Commission [IOC], 2006). In addition, it must be recognized that sea-level stations near an earthquake’s epicenter may be partially or totally destroyed by an earthquake and/or tsunami as demonstrated by the 2010 Maule, Chile earthquake and tsunami (Intergovernmental Oceanographic Commission [IOC], 2010).
Cabled Observation Systems
Attaching BPRs and accelerometers to cables would provide important improvements in timeliness and accuracy needed to precisely measure tsunami sources. This is key to achieving the level of forecast certainty needed to transform global tsunami response from a series of preplanned responses based on worst-case scenarios.
Adding BPRs to commercial fiber-optic cables at large scales is likely outside the reach of the traditional tsunami warning and mitigation capability. We therefore call on the broader ocean observations community to work with us to encourage the submarine telecommunications industry to consider installing instrumentation, such as bottom pressure sensors and accelerometers, on their cables to help achieve our goals.
Observational Data Accessibility
We expect to see continued densification of seismic, deep-ocean, and coastal observing networks in the coming years. It will be of critical importance that governments ensure data from these networks are shared through standard international paths of dissemination to inform global tsunami forecast and warning applications. Equally important is that Member States realize there is a need to maintain these observing networks over the long-term to the required standards for this and other applications.
Bathymetric Data
Accurate tsunami inundation forecasts require high-resolution bathymetric data. The resolution required for tsunami inundation forecasting is approximately 10 m. Where this resolution exists, it is often for populated coastlines. In other instances, it is not available due to security reasons. The ocean observations community should work together to increase the amount of and access to high-resolution bathymetric data to support the many beneficial applications for society.9
Time-Distance Problem
Independent of efforts to better constrain the tsunami source, there are many sections of populated coastlines where there may never be sufficient time to produce forecasts. In these places, predetermined responses based on initial seismic analysis, rapid alerting infrastructures, and a public understanding of natural warnings may forever be required.
Other Tsunami Sources
While the primary focus of this effort has been to better constrain tsunami sources in the world’s known subduction zones, we recognize that dangerous tsunamis can be created outside these zones that may require different detection and measurement strategies. Two recent events in Indonesia illustrate the need to better detect and measure all tsunamis, regardless of their source:
• On September 28, 2018, a M7.5 earthquake struck near Sulawesi. Indonesia’s Agency for Meteorology, Climatology, and Geophysics (BMKG) issued tsunami alerts based on magnitude and location alone. These alerts were cancelled once the source mechanism was determined to be strike slip (such earthquakes rarely generate destructive tsunamis), tsunami heights at Marmuju were below the threshold for an alert, and the alert period had passed. There was no real-time consideration that the strike-slip event could have induced a tsunami in the relatively shallow Palu Bay over 200 km away.
• On December 22, 2018, a tsunami occurred in the Sunda Strait that was likely caused by the Anak Krakatau volcano. Since it was not a seismic event and there were no tsunameters in the vicinity, there were no indications that a tsunami had been generated until it arrived at the coast.
Moving Forward: The United Nations Decade of Ocean Science for Sustainable Development
In February 2018, IOC/UNESCO held a workshop to review the latest developments in tsunami early warning to enhance community response. Participants discussed the remaining scientific challenges to meeting identified emergency response/mitigation needs. Following the discussion, the IOC/UNESCO Working Group on Tsunami and Other Hazards Related to Sea-level Warning and Mitigation System (TOWS-WG) debated the outcomes of the workshop (Intergovernmental Oceanographic Commission [IOC], 2018).
The consensus opinion from the workshop was that while there are, and likely always will be, large uncertainties associated with tsunami impacts in the immediate near field (i.e., within 5–10 min of generation), reliable, accurate, and more timely forecasts can be produced if we pursue improved means of constraining tsunami sources. Most notably, this would entail new ocean and coastal observation techniques and technologies or better assimilation of data from existing observation systems (Intergovernmental Oceanographic Commission [IOC], 2018). Greater forecast certainty would allow decision makers to transition from broad, preplanned responses designed for worst-case scenarios to more measured actions that better reflect the actual hazard.
This all requires improving both the science and response aspects of the global tsunami warning and mitigation system. Most of the focus of this paper has been on the scientific and observational advances needed to reduce forecast uncertainty. We recognize, however, that any step forward in this regard must be accompanied by a similar increase in community preparedness. This includes conducting training and exercises for at-risk communities, identifying evacuation routes, building vertical evacuation structures, revising regulations to accommodate specific local inundation vulnerabilities, and developing curricula that include information about local geophysical hazards.
Adding to the challenge, we strive to make these advances during a time in which we could see reduced government support for building capacity for tsunami resilience and coastal community awareness and preparedness as the memories of the devastating 2004 Indian Ocean and 2011 Tōhoku, Japan tsunamis fade. To be successful, we will need continued and enhanced engagement from governments, research institutes and universities, industry, communities, the media, and other interested parties.
Finally, we must ensure common technical standards are defined and adhered to. While technological development continues to accelerate for much of the world, many tsunami-prone countries are significantly more limited in terms of capability and capacity to implement new science applications. Therefore, measures must be put in place to ensure that tsunami warning and mitigation system development in all IOC/UNESCO Member States meets minimum standards for commonality, connectivity, and integration.
Standardization of formats is also essential to ensure tsunami warning centers can ingest and analyze data in as little as 5–10 min and to maintain uniform long-term archives. IOC/UNESCO and other relevant international agencies contributing to the global coastal and ocean observing networks must work closely together to facilitate this level of standardization among Member States.
For all of these reasons, the announcement of the United Nations Decade of Ocean Science for Sustainable Development could not have come at a more critical juncture in terms of providing opportunities to leverage capabilities and organizations normally outside the relatively narrow global tsunami community of interest. This initiative will provide the opportunity for industry and government entities to engage with the scientific community in ways never seen before.
Of the approaches discussed here, instrumenting commercial fiber-optic cables with BPRs and accelerometers is the most promising, but it comes with complexities and costs—costs unlikely to ever be fully offset by revenue from the scientific data stream. However, rising sea levels and the increasing risks to coastal populations are driving national governments, regional development agencies, and industry consortiums to recognize a larger business case for contributing to this once-in-a-generation opportunity. Drastically reducing the potential for great loss of life and mitigating the devastation of tsunamis resonates across a broad range of global enterprises. The global tsunami warning and mitigation community stands ready to assist governments and private sector actors in leveraging this enormous potential, whether through the Decade of Ocean Science or other similar interdisciplinary collaborations.
Summary
Tsunami forecast and warning efforts have traditionally relied on seismic cueing matched to precomputed scenarios that include broad worst-case assumptions. This is particularly true for local-source tsunamis. For these potentially catastrophic events, real-time numerical tsunami models are not yet able to produce sufficiently reliable forecast information.
However, with the combination of emerging solid-earth and sea-level observation technologies, advanced analysis techniques, and innovative modeling and computational strategies, the ability to directly measure or tightly infer tsunami sources is within reach. This means it could be possible for tsunami warning centers around the world to deliver accurate tsunami arrival, height, and inundation forecasts within minutes—not hours—of generation along the majority of the world’s most exposed coastlines.
With this information, emergency managers will be able to prescribe precise actions with the confidence that the forecasts will closely match observations. Communities will not have to endure hours of uncertainty before fully comprehending the threat. The vision is that emergency managers could replace their current one-size-fits-all worst-case mitigation procedures with decisive, scientifically informed responses that precisely map to the actual threat.
We are confident that driving down tsunami source and related inundation forecast uncertainties can lead to a full reconsideration of how tsunamis are mitigated globally. By connecting tsunami warning and emergency management specialists around the world with the broader ocean observations community, including governments and industry, this end state could be achieved far sooner and more effectively than it could be by the global tsunami warning and mitigation community alone.
Author Contributions
All authors listed have made a substantial, direct and intellectual contribution to the work, and approved it for publication.
Funding
DA was supported by the National Oceanic and Atmospheric Administration with this report being PMEL contribution number 4987.
Conflict of Interest Statement
The authors declare that the research was conducted in the absence of any commercial or financial relationships that could be construed as a potential conflict of interest.
Acknowledgments
The authors would like to specifically thank the input and/or expert review from the following key individuals: Tim Melbourne (Central Washington University), Ken McPherson (U.S. National Tsunami Warning Center), Lara Bland (GNS Science, Wellington, New Zealand), Bruce Howe (University of Hawaii), Preston Thomas (Thomas Strategies), Bernardo Aliaga (IOC/UNESCO), Thorkild Aarup (IOC/UNESCO), Rahmat Triyono (Indonesia Tsunami Early Warning System, Agency for Meteorology, Climatology, and Geophysics), Thorne Lay (University of California, Santa Cruz), Srinivasa Tummala (IOC/UNESCO), Allison Allen (NOAA/National Weather Service), and Weniza (Indonesia Tsunami Early Warning System, Agency for Meteorology, Climatology, and Geophysics). In addition, the authors would like to especially recognize the efforts of Christa Rabenold of the NOAA/National Weather Service Tsunami Program staff. Christa’s editorial and subject matter expertise were critical to this manuscript’s development and construction.
Footnotes
- ^ Tsunamis can be described mathematically as solutions to hyperbolic partial differential equations. This is a unique characteristic of hyperbolic systems and stands in contrast to other geophysical processes defined by parabolic or elliptic equations.
- ^ Where used in this paper, M denotes moment magnitude (Mw).
- ^ The 5–10 min target does not negate the need to continue outreach and education efforts focused on natural warnings, which are the primary warnings for local-source tsunamis. Natural warnings include strong or long earthquake shaking, a sudden rise or fall of the ocean, and unusual ocean sounds.
- ^ A regional-source tsunami is between 1 and 3 h tsunami travel time away.
- ^ Information about the Sendai framework is online at https://www.unisdr.org/we/coordinate/sendai-framework.
- ^ See Appendix to learn more about moment magnitude.
- ^ It should be noted that while a single GNSS station can be sufficient for estimating the Mpgd of a seismic event, the need for a sufficiently high signal-to-noise ratio limits the allowable distance between the recording station and the epicenter. This minimum distance will vary with the magnitude of the event, but if one considers only events larger than Mw 7 to be of tsunamigenic interest, then the estimated minimum distance for Mpgd estimation is approximately 200 km (Brendan Crowell, personal communication, March, 2019).
- ^ This paper uses “bottom pressure recorder,” but it is synonymous with other terms, including “ocean bottom pressure gauge.”
- ^ Seabed 2030 is a project to bring together and share all available bathymetric data. For more information, see https://seabed2030.gebco.net/.
References
Aki, K. (1966). Generation and propagation of G waves from the Niigata earthquake of June 14, 1964: Part 2. Estimation of earthquake moment, released energy and stress-strain drop from G wave spectrum. Bull. Earthq. Res. Inst. 44, 73–88.
Artru, J., Lognonne, P., Occhipinti, G., Crespon, F., and Garcia, R. (2005). Tsunami detection in the ionosphere. Space Res. Today 163, 23–27. doi: 10.1016/S0045-8732(05)80048-8
Barnes, C. R. (2018). “Quantum leap in platforms of opportunity: Telecommunication cables,” in Challenges and Innovations in Ocean in Situ Sensors: Measuring Inner Ocean Processes and Health in the Digital Age, eds E. Delory and J. Pearlman (Amsterdam: Elsevier Science), 33–44.
Barnes, C. R., Meldrum, D. T., and Ota, H. (2016). “Emerging subsea networks: New market opportunities for, and societal contributions from, SMART cable systems,” in Proceedings of SubOptic 2016, Dubai.
Barrick, D. E. (1979). A coastal radar system for tsunami warning. Remote Sens. Environ. 8, 353–358. doi: 10.1016/0034-4257(79)90034-8
Bernard, E., and Meinig, C. (2011). “History and future of deep-ocean tsunami measurements,” in Proceedings of Oceans’11 MTS/IEEE, Kona.
Bernard, E., and Titov, V. V. (2015). Evolution of tsunami warning systems and products. Philos. Trans. R. Soc. Lond. A. 373:20140371. doi: 10.1098/rsta.2014.0371
Bernard, E. N., Meinig, C., Titov, V. V., O’Neil, K., Lawson, R., Jarrott, K., et al. (2010). “Tsunami resilient communities,” in Proceedings of OceanObs’09: Sustained Ocean Observations and Information for Society, Venice.
Bittner, M., Höppner, K., Pilger, C., and Schmidt, C. (2010). Mesopause temperature perturbations caused by infrasonic waves as a potential indicator for the detection of tsunamis and other geohazards. Nat. Hazards Earth Syst. Sci. 10, 1431–1442. doi: 10.5194/nhess-10-1431-2010
Butler, R., Cochran, E., Collins, J., Eblé, M., Evans, J., Favali, P., et al. (2014). The Scientific and Societal Case for the Integration of Environmental Sensors into New Submarine Telecommunication Cables. ITU/WMO/UNESCO IOC Joint Task Force report. Paris: UNESCO.
Church, J. A., and White, N. J. (2011). Sea-level rise from the late 19th to the early 21st century. Surveys Geophys. 32, 585–602. doi: 10.1007/s10712-011-9119-1
De la Asunción, M., González, J. M., and Castro, M. J. (2013). CUDA Implementation of the Propagation Module of the MOST Model. Seattle, WA: NOAA Center for Tsunami Research.
Dzvonkovskaya, A. (2012). “Ocean surface current measurements using HF radar during the 2011 Japan tsunami hitting Chilean coast,” in Geoscience and Remote Sensing Symposium (IGARSS), 2012 IEEE International Geoscience and Remote Sensing Symposium, Munich, 7605–7608. doi: 10.1109/IGARSS.2012.6351867
Fine, I. V., Cherniawsky, J. Y., Thomson, R. E., Rabinovich, A. B., and Krassovski, M. V. (2015). Observations and numerical modeling of the 2012 Haida Gwaii tsunami off the coast of British Columbia. Pure Appl. Geophys. 172, 699–718. doi: 10.1007/s00024-014-1012-7
Fry, B., Gledhill, K. R., Kaiser, A. E., Francois-Holden, C., Power, W. L., McCurrach, S.-J., et al. (2018). Toward operational tsunami early warning in New Zealand. Paper Presented at AGU Fall Meeting 2018, Washington DC.
Gailler, A., Hébert, H., Schindelé, F., and Reymond, D. (2018). Coastal amplification laws for the French tsunami Warning Center: numerical modeling and fast estimate of tsunami wave heights along the French Riviera. Pure Appl. Geophys. 175, 1429–1444. doi: 10.1007/s00024-017-1713-9
Garcés, M., Caron, P., Hetzer, C., Pichon, A. L., Bass, H., Drob, D., et al. (2005). Deep infrasound radiated by the Sumatra earthquake and tsunami. Eos 86, 317–320. doi: 10.1029/2005EO350002
Geist, E. L., Titov, V. V., Arcas, D., Pollitz, F. F., and Bilek, S. L. (2007). Implications of the 26 December 2004 Sumatra–Andaman earthquake on tsunami forecast and assessment models for great subduction-zone earthquakes. Bull. Seismol. Soc. Am. 97, S249–S270. doi: 10.1785/0120050619
Gica, E., Spillane, M., Titov, V. V., Chamberlin, C., and Newman, J. C. (2008). Development of the Forecast Propagation Database for NOAA’s Short-term Inundation Forecast for Tsunamis (SIFT). NOAA Tech. Memo. OAR PMEL-139. Seattle, WA: U.S. Dept. of Commerce, National Oceanic and Atmospheric Administration, Office of Oceanic and Atmospheric Research, Pacific Marine Environmental Laboratory.
González-Vida, J. M., Macías, J., Castro, M. J., Sánchez-Linares, C., de la Asunción, M., Ortega, S., et al. (2019). The Lituya Bay landslide-generated mega-tsunami. Numerical simulation and sensitivity analysis. Nat. Hazards Earth Syst. Sci. 19, 369–388. doi: 10.5194/nhess-19-369-2019
Gower, J. (2005). Jason1 detects the 26 December 2004 tsunami. Eos 86, 37–38. doi: 10.1029/2005EO040002
Grilli, S. T., Shelby, M., Grilli, A., Guerin, C.-A., Grosdidier, S., and Insua, T. (2016). “Algorithms for tsunami detection by high frequency radar: Development and case studies for tsunami impact in British Columbia, Canada,” in Proceedings of the 26th International Ocean and Polar Engineering Conference, Rhodes, 807–814.
Guilbert, J., Vergoz, J., Schisselé, E., Roueff, A., and Cansi, Y. (2005). Use of hydroacoustic and seismic arrays to observe rupture propagation and source extent of the Mw = 9.0 Sumatra earthquake. Geophys. Res. Lett. 32:L15310. doi: 10.1029/2005GL022966
Hébert, H., Heinrich, P., Schindelé, F., and Piatanesi, A. (2001). Far-field simulation of tsunami propagation in the Pacific Ocean: impact on the marquesas islands (French Polynesia). J. Geophys. Res. 106, 9161–9177. doi: 10.1029/2000JC000552
Hébert, H., and Schindelé, F. (2015). Tsunami impact computed from offshore modeling and coastal amplification laws: insight from the 2004 indian ocean tsunami. Pure Appl. Geophys. 172, 3385–3407. doi: 10.1007/s00024-15-1136-4
Hébert, H., Sladen, A., and Schindelé, F. (2007). Numerical modeling of the great 2004 indian ocean tsunami: focus on the Mascarene Islands. Bull. Seismol. Soc. Am. 97, S208–S222. doi: 10.1785/0120050611
Hinata, H., Fujii, S., Furukawa, K., Kataoka, T., Miyata, M., Kobayashi, T., et al. (2011). Propagating tsunami wave and subsequent resonant response signals detected by HF radar in the Kii Channel, Japan. Estuar. Coast. Shelf Sci. 95, 268–273. doi: 10.1016/j.ecss.2011.08.009
Howe, B. M., Aucan, J., and Tilmann, F. (2016). Submarine cable systems for future societal needs. Eos 97, doi: 10.1029/2016EO056781
Howe, B. M., Tilmann, F., and The Joint Task Force for Smart Cables (2018). “Using submarine telecommunications cables to improve monitoring tsunamis and earthquakes: The SMART Cable concept,” in Poster presented at IOC/UNESCO Symposium: Advances in Tsunami Warning to Enhance Community Responses, Paris.
Hughes, C. W., Williams, J., Blaker, A., Coward, A., and Stepanov, V. (2018). A window on the deep ocean: the special value of ocean bottom pressure for monitoring the large-scale, deep-ocean circulation. Prog. Oceanogr. 161, 19–46. doi: 10.1016/j.pocean.2018.01.011
Intergovernmental Oceanographic Commission [IOC] (2006). Manual on Sea Level Measurement and Interpretation. Volume IV–An Update to 2006. Paris: UNESCO.
Intergovernmental Oceanographic Commission [IOC] (2009). Tsunami Early Warning and Mitigation System in the North Eastern Atlantic, the Mediterranean and Connected Seas, NEAMTWS, Implementation Plan. Paris: UNESCO.
Intergovernmental Oceanographic Commission [IOC] (2010). 27 February 2010 Chile Earthquake and Tsunami Event—Post-Event Assessment of PTWS Performance. Paris: UNESCO.
Intergovernmental Oceanographic Commission [IOC] (2016). Manual on Sea-level Measurements and Interpretation, Volume V: Radar Gauges. Paris: UNESCO.
Intergovernmental Oceanographic Commission [IOC] (2018). IOC/UNESCO Symposium on Advances in Tsunami Warning to Enhance Community Responses, 12–14 February 2018, Summary Statement. Paris: UNESCO.
Jamelot, A., and Reymond, D. (2015). New tsunami forecast tools for the French Polynesia tsunami warning system Part II: numerical modelling and tsunami height estimation. Pure Appl. Geophys. 172, 805–819. doi: 10.1007/s00024-014-0997-2
Kanazawa, T. (2013). “Japan trench earthquake and tsunami monitoring network of cable-linked 150 ocean bottom observatories and its impact to earth disaster science,” in IEEE International Underwater Technology Symposium, Tokyo
Kaneda, Y. (2013). Real time monitoring systems and advanced simulation researches for Earthquakes/Tsunami disaster mitigation. Paper Presented at AGU Fall Meeting 2013, San Francisco.
Kao, H., and Shan, S.-J. (2004). The source-scanning algorithm: mapping the distribution of seismic sources in time and space. Geophys. J. Int. 157, 589–594. doi: 10.1111/j.1365-246X.2004.02276.x
Kao, H., Wang, K., Chen, R. Y., Wada, I., He, J., and Malone, S. D. (2008). Identifying the rupture plane of the 2001 Nisqually, Washington, earthquake. Bull. Seismol. Soc. Am. 98, 1546–1558. doi: 10.1785/0120070160
Le Pichon, A., Guilbert, J., Vega, A., Garcés, M., and Brachet, N. (2002). Ground-coupled air waves and diffracted infrasound from the Arequipa earthquake of June 23, 2001. Geophys. Res. Lett. 29, 33-1–33-4. doi: 10.1029/2002GL015052
Le Pichon, A., Herry, P., Mialle, P., Vergoz, J., Brachet, N., Garcés, M., et al. (2005). Infrasound associated with 2004–2005 large Sumatra earthquakes and tsunami. Geophys. Res. Lett. 32:L19802. doi: 10.1029/2005GL023893
Lipa, B., Barrick, D., Saitoh, S.-I., Ishikawa, Y., Awaji, T., Largier, J., et al. (2011). Japan tsunami current flows observed by HF radars on two continents. Remote Sens. 3, 1663–1679. doi: 10.3390/rs3081663
Marra, G., Clivati, C., Luckett, R., Tampellini, A., Kronjäger, J., Wright, L., et al. (2018). Ultrastable laser interferometry for earthquake detection with terrestrial and submarine cables. Science 361, 486–490. doi: 10.1126/science.aat4458
Meinig, C., Eblé, M. C., and Stalin, S. E. (2001). “System development and performance of the Deep-ocean Assessment and Reporting of Tsunamis (DART) system from 1997–2001,” in Proceedings of the International Tsunami Symposium 2001 (ITS 2001), Seattle, WA.
Melgar, D., Crowell, B. W., Geng, J., Allen, R. M., Bock, Y., Riquelme, S., et al. (2015). Earthquake magnitude calculation without saturation from the scaling of peak ground displacement. Geophys. Res. Lett. 42, 5197–5205. doi: 10.1002/2015GL064278
Mofjeld, H. O. (2009). “Tsunami measurements,” in Tsunamis, The Sea, Vol. 15, eds A. Robinson and E. Bernard (Cambridge, MA: Harvard University Press), 201–235.
Mori, N., Takahashi, T., Yasuda, T., and Yanagisawa, H. (2011). Survey of 2011 Tohoku earthquake tsunami inundation and run-up. Geophys. Res. Lett. 38:L00G14. doi: 10.1029/2011GL049210
National Centers for Environmental Information/World Data Service [NCEI/WDS] (n.d.). Global Historical Tsunami Database. Avbailable at: https://www.ngdc.noaa.gov/hazard/tsu_db.shtml (accessed August, 2018).
Occhipinti, G. (2015). “The seismology of the Planet Mongo: The 2015 ionospheric seismology review,” in Subduction Dynamics: From Mantle Flow to Mega Disasters, eds G. Morra, D. A. Yuen, S. D. King, S. M. Lee, and S. Stein (Hoboken, NJ: John Wiley and Sons), 169–182. doi: 10.1002/9781118888865
Occhipinti, G., Manta, F., Rolland, L., Watada, S., Makela, J. J., Hill, E., et al. (2018). “Ionospheric detection of tsunami earthquakes: observation, modeling and ideas for future early warning,” in Poster presented at IOC/UNESCO Symposium on Advances in Tsunami Warning to Enhance Community Responses, Paris.
Okada, Y. (1985). Surface deformation due to shear and tensile faults in a half-space. Bull. Seismol. Soc. Am. 75, 1135–1154.
Percival, D. B., Arcas, D., Denbo, D. W., Eble, M. C., Gica, E., Mofjeld, H. O., et al. (2009). Extracting Tsunami Source Parameters Via Inversion of DART Buoy Data. NOAA Tech. Memo. OAR PMEL-144. Seattle, WA: U.S. Dept. of Commerce, National Oceanic and Atmospheric Administration, Office of Oceanic and Atmospheric Research, Pacific Marine Environmental Laboratory.
Power, W. L., Wallace, L. M., Mueller, C., Henrys, S. A., Clark, K. J., Fry, B., et al. (2016). Understanding the potential for tsunami generated by earthquakes on the southern Hikurangi subduction interface. N. Z. J. Geol. Geophys. 59, 70–85. doi: 10.1080/00288306.2015.1127825
Pugh, D., and Woodworth, P. (2014). Sea-Level Science: Understanding Tides, Surges, Tsunamis and Mean Sea-Level Changes. Cambridge: Cambridge University Press.
Rabinovich, A. B., and Eblé, M. C. (2015). Deep ocean measurements of tsunami waves. Pure Appl. Geophys. 172, 3281–3312. doi: 10.1007/s00024-015-1058-1
Rabinovich, A. B., Thomson, R. E., and Fine, I. V. (2013). The 2010 Chilean tsunami off the west coast of Canada and the northwest coast of the United States. Pure Appl. Geophys. 170, 1529–1565. doi: 10.1007/s00024-012-0541-1
Rakoto, V., Lognonné, P., Rolland, L., and Coisson, P. (2018). “Tsunami wave height estimation from GPS-derived ionospheric data,” in Poster Presented at IOC/UNESCO Symposium on Advances in Tsunami Warning to Enhance Community Responses, Paris.
Ranasinghe, N., Rowe, C., Syracuse, E., Larmat, C., and Begnaud, M. (2017). Enhanced global seismic resolution using transoceanic SMART cables. Seismol. Res. Lett. 89, 77–85. doi: 10.1785/0220170068
Roch, J., Schindelé, F., Rivera, L., and Duperray, P. (2016). Very fast characterization of fault parameters through W-phase centroid inversion in the context of tsunami warning. Pure Appl. Geophys. 173, 3881–3893. doi: 10.1007/s00024-016-1258-3
Rolland, L. M., Occhipinti, G., Lognonne, P., and Loevenbruck, A. (2010). Ionospheric gravity waves detected offshore Hawaii after tsunamis. Geophys. Res. Lett. 37:L17101. doi: 10.1029/2010GL044479
Sahal, A., Roger, J., Allgeyer, S., Lemaire, B., Hébert, H., Schindelé, F., et al. (2009). The tsunami triggered by the 21 May 2003 Boumerdes-Zemmouri (Algeria) earthquake: field investigations on the French Mediterranean coast and tsunami modelling. Nat. Hazards Earth Syst. Sci. 9, 1823–1834. doi: 10.5194/nhess-9-1823-2009
Smith, W. H. F., Scharroo, R., Titov, V. V., Arcas, D., and Arbic, B. K. (2005). Satellite altimeters measure tsunami. Oceanography 18, 11–13. doi: 10.5670/oceanog.2005.62
Song, Y. T., Fukumori, I., Shum, C. K., and Yi, Y. (2012). Merging tsunamis of the 2011 Tohoku-Oki earthquake detected over the open ocean. Geophys. Res. Lett. 39:L05606. doi: 10.1029/2011GL050767
Suppasri, A., Shuto, N., Imamura, F., Koshimura, S., Mas, E., and Yalciner, A. C. (2012). Lessons learned from the 2011 great east japan tsunami: performance of tsunami countermeasures, coastal buildings, and tsunami evacuation in Japan. Pure Appl. Geophys. 170, 993–1018. doi: 10.1007/s00024-012-0511-7
Takahashi, N., Imai, I., Ishibashi, M., Sueki, K., Obayashi, R., Tanabe, T., et al. (2017). Real-time tsunami prediction system using DONET. J. Disaster Res. 12, 766–774.
Thomson, R. E., Fine, I. V., Rabinovich, A. B., Mihaly, S. F., Davis, E. E., Heesemann, M., et al. (2011). Observations of the 2009 Samoa tsunami by the NEPTUNE-Canada cabled observatory: test data for an operational regional tsunami forecast model. Geophys. Res. Lett. 38:L11701. doi: 10.1029/2011GL046728
Tilmann, F., Howe, B. M., and Butler, R. (2017). Commercial underwater cable systems could reduce disaster impact. Eos 98, doi: 10.1029/2017EO069575
Titov, V., and González, F. I. (1997). Implementation and Testing of the Method of Splitting Tsunami (MOST) Model. NOAA Tech. Memo. ERL PMEL-112. Seattle, WA: U.S. Dept. of Commerce, National Oceanic and Atmospheric Administration, Office of Oceanic and Atmospheric Research, Pacific Marine Environmental Laboratory.
Titov, V. V. (2009). “Tsunami forecasting,” in Tsunamis, The Sea, Vol. 15, eds A. Robinson and E. Bernard (Cambridge, MA: Harvard University Press), 371–400.
Titov, V. V., Song, Y. T., Tang, L., Bernard, E. N., Bar-Sever, Y., and Wei, Y. (2016). Consistent estimates of tsunami energy show promise for improved early warning. Pure Appl. Geophys. 173, 3863–3880. doi: 10.1007/s00024-016-1312-1
United Nations International Strategy for Disaster Reduction [UNISDR] (2015). Sendai Framework for Disaster Risk Reduction 2015–2030. Geneva: UNISDR.
Vela, J., Pérez-Gómez, B., González, M., Otero, L., Olabarrieta, M., Canals, M., et al. (2014). Tsunami resonance in palma bay and harbor, majorca Island, as induced by the 2003 Western Mediterranean Earthquake. J. Geol. 122, 165–182. doi: 10.1086/675256
Wilcock, W. S. D., Manalang, D., Harrington, M., Cram, G., Tilley, J., Burnett, J., et al. (2017). A seafloor test of the A-0-A approach to calibrating pressure sensors for vertical geodesy. Poster Presented at AGU Fall Meeting 2017, New Orleans.
Williamson, A. L., and Newman, A. V. (2018). Suitability of open-ocean instrumentation for use in near-field tsunami early warning along seismically active subduction zones. Pure Appl. Geophys. 1–16. doi: 10.1007/s00024-018-1898-6
You, J. Y. (2010). Harnessing telecoms cables for science. Nature 466, 690–691. doi: 10.1038/466690a
Appendix: Advances in Seismic Estimation of the Tsunami Source
Earthquake magnitude is the most basic factor in determining an earthquake’s tsunamigenic potential. Since the start of instrumental seismology, seismologists have created numerous magnitude scales to serve as a proxy for earthquake size. Each scale has its own strengths and applicability.
The Richter surface wave magnitude (Ms) was long used to characterize the size of great earthquakes and infer their tsunamigenic potential. However, this scale is insufficient to resolve magnitudes that reach the mid-8 range (known as magnitude saturation), which is far below the true magnitude of the most destructive tsunamigenic earthquakes.
To address the saturation problem, Aki (1966) introduced the moment magnitude (Mw) scale in the 1960s. While other scales saturate at much lower magnitudes, the Mw scale is able to measure truly great earthquakes, such as the 1960 M9.5 Chile earthquake. In addition, it was the first scale to have a physical and geological meaning—the area of the fault times the slip across the fault times the shear modulus of the material containing the fault.
Since magnitude scales are logarithmic (i.e., with each whole number increase in magnitude, the energy release increases about 32 times), it is critical to know an earthquake’s true magnitude, its Mw, in order to estimate its tsunamigenic potential. The Mw scale provided a fundamentally more useful measure of relating earthquake magnitude to tsunamigenic potential.
Below are some more recent examples of seismic analysis techniques that further refine and/or speed up tsunami source estimates.
• Mwp: Initial routine measurements of Mw for large earthquakes were based on analyses of seismograms from key stations but were not available until many hours or even days after an earthquake—not soon enough to be useful for tsunami warning. Recently, techniques have been developed to more quickly estimate Mw from the initial P-wave arrivals on broadband seismometers. This Mw scale, known as Mwp, can be produced just minutes after an earthquake. This is the scale now used by warning centers around the world for initial tsunami assessments.
• W-phase CMT: The seismic “W-phase” is a very long-period seismic signal (100–1,000 s period) that travels in Earth’s mantle and arrives just after the P-wave. Within 20–30 min of earthquake origin, tsunami forecasters can now invert the seismic “W-phase” to produce a more reliable Mw estimate and identify the seismic mechanism by generating what is known as the W-phase CMT (Roch et al., 2016). Tsunami earthquakes, which are earthquakes that generate larger tsunamis than their Mw would otherwise suggest (Kanamori, 1972), generate large W-phase waves.
• Rupture extension and location: Fast processing of seismic and hydroacoustic array data can provide fast rupture location and extension (Guilbert et al., 2005).
• Strong-motion centroid: Modern strong-motion sensor networks provide a continuous measurement of large ground motions, which can be used to estimate the extent and size of a rupture. This has proven effective for earthquakes like the 2016 Kaikoura event in New Zealand (Fry et al., 2018). Traditional seismic techniques failed to characterize this earthquake as tsunamigenic due to its complicated rupture process, which involved approximately 20 faults and an onshore epicenter.
• Back-projected energy: Summing back-projected energy from strong-motion stations can provide estimates of the rupture extent and centroid (e.g., Kao and Shan, 2004; Kao et al., 2008). Applying back projection to the 2016 Kaikoura earthquake results in an estimated rupture that scales to M7.9 within 2 min of the earthquake’s origin compared to the global M7.8 estimated 10+ min after origin. In retrospect, this magnitude and mapped rupture extent could have formed the basis of a warning within 5 min of origin. Back-projection techniques, when combined with assumptions about fault geometry, may prove useful for tsunami warning purposes, as it is possible to use the centroid estimates they produce, coupled with empirical tsunami relationships, to produce initial estimates of the likely areas of impact (Fry et al., 2018).
Keywords: tsunami, detection, forecast, warning, mitigation, near field, uncertainty
Citation: Angove M, Arcas D, Bailey R, Carrasco P, Coetzee D, Fry B, Gledhill K, Harada S, von Hillebrandt-Andrade C, Kong L, McCreery C, McCurrach S-J, Miao Y, Sakya AE and Schindelé F (2019) Ocean Observations Required to Minimize Uncertainty in Global Tsunami Forecasts, Warnings, and Emergency Response. Front. Mar. Sci. 6:350. doi: 10.3389/fmars.2019.00350
Received: 29 October 2018; Accepted: 06 June 2019;
Published: 25 June 2019.
Edited by:
Sanae Chiba, Japan Agency for Marine-Earth Science and Technology, JapanReviewed by:
José Pinho, University of Minho, PortugalBegoña Pérez-Gómez, Independent Researcher, Madrid, Spain
Copyright © 2019 Angove, Arcas, Bailey, Carrasco, Coetzee, Fry, Gledhill, Harada, von Hillebrandt-Andrade, Kong, McCreery, McCurrach, Miao, Sakya and Schindelé. This is an open-access article distributed under the terms of the Creative Commons Attribution License (CC BY). The use, distribution or reproduction in other forums is permitted, provided the original author(s) and the copyright owner(s) are credited and that the original publication in this journal is cited, in accordance with accepted academic practice. No use, distribution or reproduction is permitted which does not comply with these terms.
*Correspondence: Michael Angove, bWljaGFlbC5hbmdvdmVAbm9hYS5nb3Y=