- 1NOAA/AOML, Miami, FL, United States
- 2GEOMAR Helmholtz Centre for Ocean Research Kiel, Kiel, Germany
- 3Kiel University, Kiel, Germany
- 4Application Laboratory, Japan Agency for Marine-Earth Science and Technology, Yokohama, Japan
- 5Departamento de Física de la Tierra y Astrofísica, Universidad Complutense de Madrid, Madrid, Spain
- 6Instituto de Geociencias IGEO, UCM-CSIC, Madrid, Spain
- 7IRD, LEGOS, Mercator Océan, Ramonville-Saint-Agne, France
- 8Department of Oceanography, Federal University of Pernambuco, Recife, Brazil
- 9Department of Oceanography, Federal University of Santa Catarina, Florianópolis, Brazil
- 10Kiel Marine Science, Christian-Albrechts-Universität zu Kiel, Kiel, Germany
- 11Department of Physical Oceanography, Woods Hole Oceanographic Institution, Woods Hole, MA, United States
- 12LOCEAN-IPSL, Pierre and Marie Curie University, Paris, France
- 13Faculdade de Oceanografia, BrOA, Universidade do Estado do Rio de Janeiro, Rio de Janeiro, Brazil
- 14NOAA/PMEL, Seattle, WA, United States
- 15UMR5318 CECI CNRS-CERFACS, Toulouse, France
- 16Climate and Ecosystem Sciences Division, Lawrence Berkeley National Laboratory, Berkeley, CA, United States
- 17Center for Marine Meteorology, Météo-France, Brest, France
- 18Rosenstiel School of Marine and Atmospheric Science, University of Miami, Miami, FL, United States
- 19CIEnAm, Departamento de Química Analítica, Universidade Federal da Bahia, Salvador, Brazil
- 20Centre IRD de Bretagne, Technopole Pointe du Diable, Plouzané, France
- 21Faculty of Oceanography, University of Calabar, Calabar, Nigeria
- 22Collecte Localisation Satellites, Ramonville-Saint-Agne, France
- 23Department of Oceanography and Atmospheric Sciences, Texas A&M University, College Station, TX, United States
- 24Physical Oceanography Laboratory, Qingdao Collaborative Innovation Center of Marine Science and Technology, Ocean University of China, Qingdao, China
- 25Direction de la Recherche, Météo-France, Toulouse, France
- 26NOAA/NCEP Climate Prediction Center, College Park, MD, United States
- 27LEGOS, CNRS/IRD/UT/CNES, Toulouse, France
- 28Department of Oceanography, University of Cape Town, Cape Town, South Africa
- 29Department of Atmospheric and Oceanic Science, University of Maryland, College Park, MD, United States
- 30Mercator Ocean, Ramonville-Saint-Agne, France
- 31JPL, NASA, Pasadena, CA, United States
- 32Center for Weather Forecast and Climate Studies – CPTEC, National Institute for Space Research (INPE), Cachoeira Paulista, Brazil
- 33Geophysical Institute, University of Bergen, Bergen, Norway
- 34Bjerknes Centre for Climate Research, Bergen, Norway
- 35Centro Euro-Mediterraneo sui Cambiamenti Climatici, Bologna, Italy
- 36Centre for Maritime Research and Experimentation (CMRE), La Spezia, Italy
- 37LOCEAN-IPSL, Sorbonne Universités (UPMC, Univ. Paris 06), CNRS/IRD/MNHN, Paris, France
- 38Laboratoire de Météorologie Dynamique, CNRS, ENS, UMR Ecole Polytech 8539, Paris, France
- 39CIMAS, University of Miami, Miami, FL, United States
- 40Department for Environment, Food and Rural Affairs (Defra), London, United Kingdom
- 41College of Earth, Ocean, and Atmospheric Sciences, Oregon State University, Corvallis, OR, United States
- 42School of Environmental Sciences, University of East Anglia, Norwich, United Kingdom
- 43ESP, University Cheikh Anta Diop (UCAD), Laboratoire de Physique de l’Atmosphère et de l’Océan Siméon Fongang, Dakar, Senegal
- 44National Centre for Atmospheric Science (NCAS), Department of Meteorology, University of Reading, Reading, United Kingdom
- 45MARE, Marine and Environmental Sciences Centre, University of Lisbon, Lisbon, Portugal
- 46Department of Chemistry, Covenant University, Ota, Nigeria
- 47Research Laboratory of Mathematics and Mathematical Physics, University of Abomey-Calavi, Cotonou, Benin
- 48Directorate General for Science, Technology and Nuclear Development of the Brazilian Navy, Rio de Janeiro, Brazil
- 49Laboratoire d’Océanographie Physique et Spatiale (LOPS), Université de Bretagne Occidentale, CNRS, Ifremer, IRD, Brest, France
The tropical Atlantic is home to multiple coupled climate variations covering a wide range of timescales and impacting societally relevant phenomena such as continental rainfall, Atlantic hurricane activity, oceanic biological productivity, and atmospheric circulation in the equatorial Pacific. The tropical Atlantic also connects the southern and northern branches of the Atlantic meridional overturning circulation and receives freshwater input from some of the world’s largest rivers. To address these diverse, unique, and interconnected research challenges, a rich network of ocean observations has developed, building on the backbone of the Prediction and Research Moored Array in the Tropical Atlantic (PIRATA). This network has evolved naturally over time and out of necessity in order to address the most important outstanding scientific questions and to improve predictions of tropical Atlantic severe weather and global climate variability and change. The tropical Atlantic observing system is motivated by goals to understand and better predict phenomena such as tropical Atlantic interannual to decadal variability and climate change; multidecadal variability and its links to the meridional overturning circulation; air-sea fluxes of CO2 and their implications for the fate of anthropogenic CO2; the Amazon River plume and its interactions with biogeochemistry, vertical mixing, and hurricanes; the highly productive eastern boundary and equatorial upwelling systems; and oceanic oxygen minimum zones, their impacts on biogeochemical cycles and marine ecosystems, and their feedbacks to climate. Past success of the tropical Atlantic observing system is the result of an international commitment to sustained observations and scientific cooperation, a willingness to evolve with changing research and monitoring needs, and a desire to share data openly with the scientific community and operational centers. The observing system must continue to evolve in order to meet an expanding set of research priorities and operational challenges. This paper discusses the tropical Atlantic observing system, including emerging scientific questions that demand sustained ocean observations, the potential for further integration of the observing system, and the requirements for sustaining and enhancing the tropical Atlantic observing system.
Introduction
Many developing countries surrounding the tropical Atlantic Ocean face societal challenges that are compounded by climate variability and change (Figure 1). Rainfall in South America and West Africa and Atlantic hurricanes are highly sensitive to conditions in the tropical Atlantic. These conditions are driven by complex interactions between the ocean, atmosphere, and land, and between other ocean basins and the tropical Atlantic. Strong and societally relevant variability occurs on seasonal to multidecadal timescales. The background state on which these fluctuations occur is changing, as are the two-way connections between the tropical Atlantic and the Pacific. In the tropical Atlantic Ocean, significant changes in physical and biogeochemical variables, including temperature, oxygen, nutrient availability and pH, are also occurring, and it is unclear how these changes will affect marine ecosystems and biodiversity. Short-term predictions and longer decadal and century-scale projections of the tropical Atlantic are made more challenging by persistent biases in climate models that have seen little progress over the past two decades.
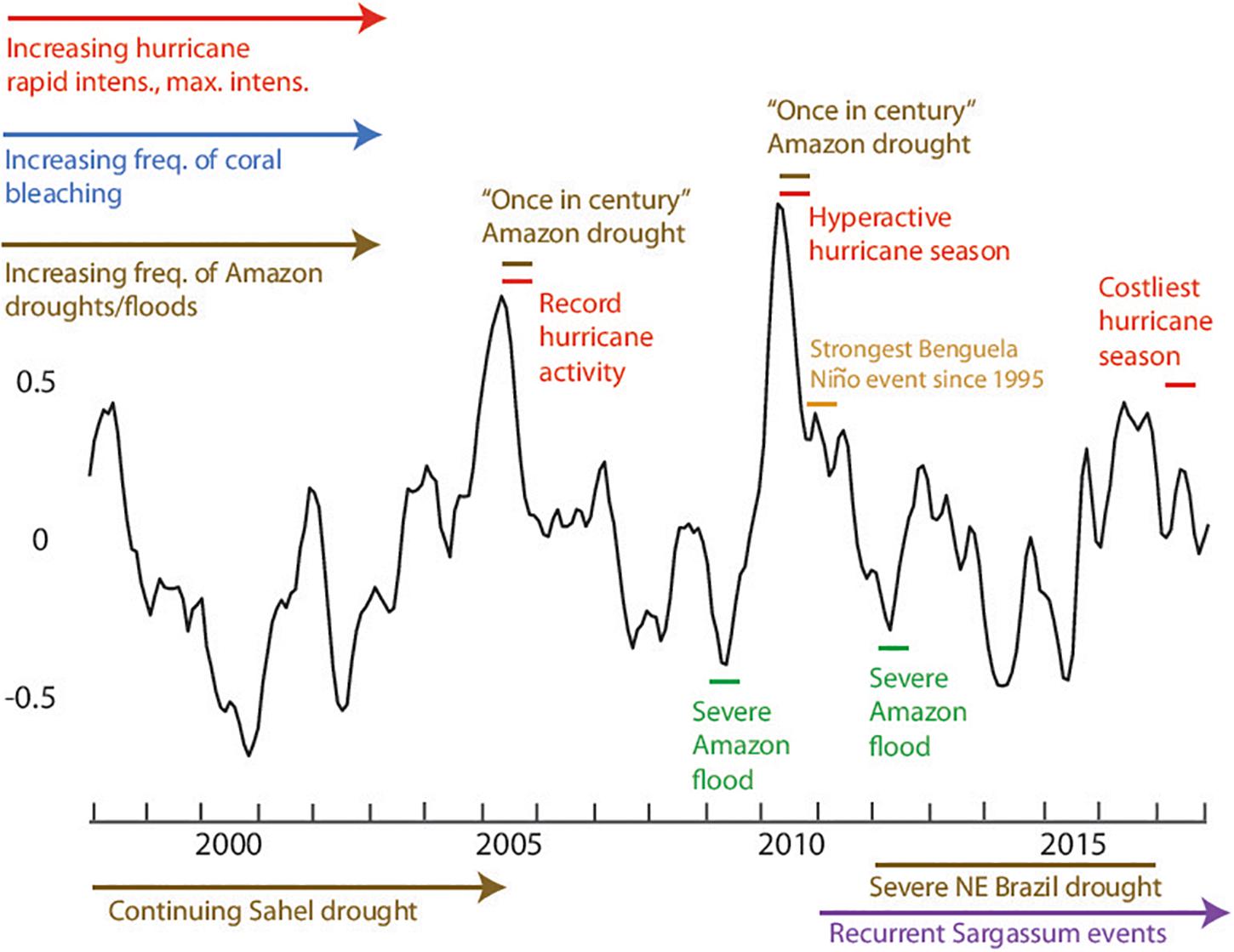
Figure 1. Summary of notable tropical Atlantic societal impacts over the past 20 years. Black line is the monthly SST anomaly, relative to the 1998–2017 mean climatology, averaged between 5°N–25°N and 30°W–60°W.
The tropical Atlantic observing system has progressed substantially over the past 20 years (Figure 2), yet many challenges remain. Observing systems must continue to monitor the climate system and provide measurements to aid in weather and climate prediction, scientific research, and ocean state estimates. There are new emerging threats that will require additional scientific knowledge and monitoring capabilities. These include the increasing occurrence of weather and climate extremes and tipping points in ocean biogeochemistry and ecosystems. This paper summarizes the societal issues that demand tropical Atlantic climate monitoring and prediction, their scientific drivers, and the current observing system. It concludes with key recommendations for the future tropical Atlantic observing system.
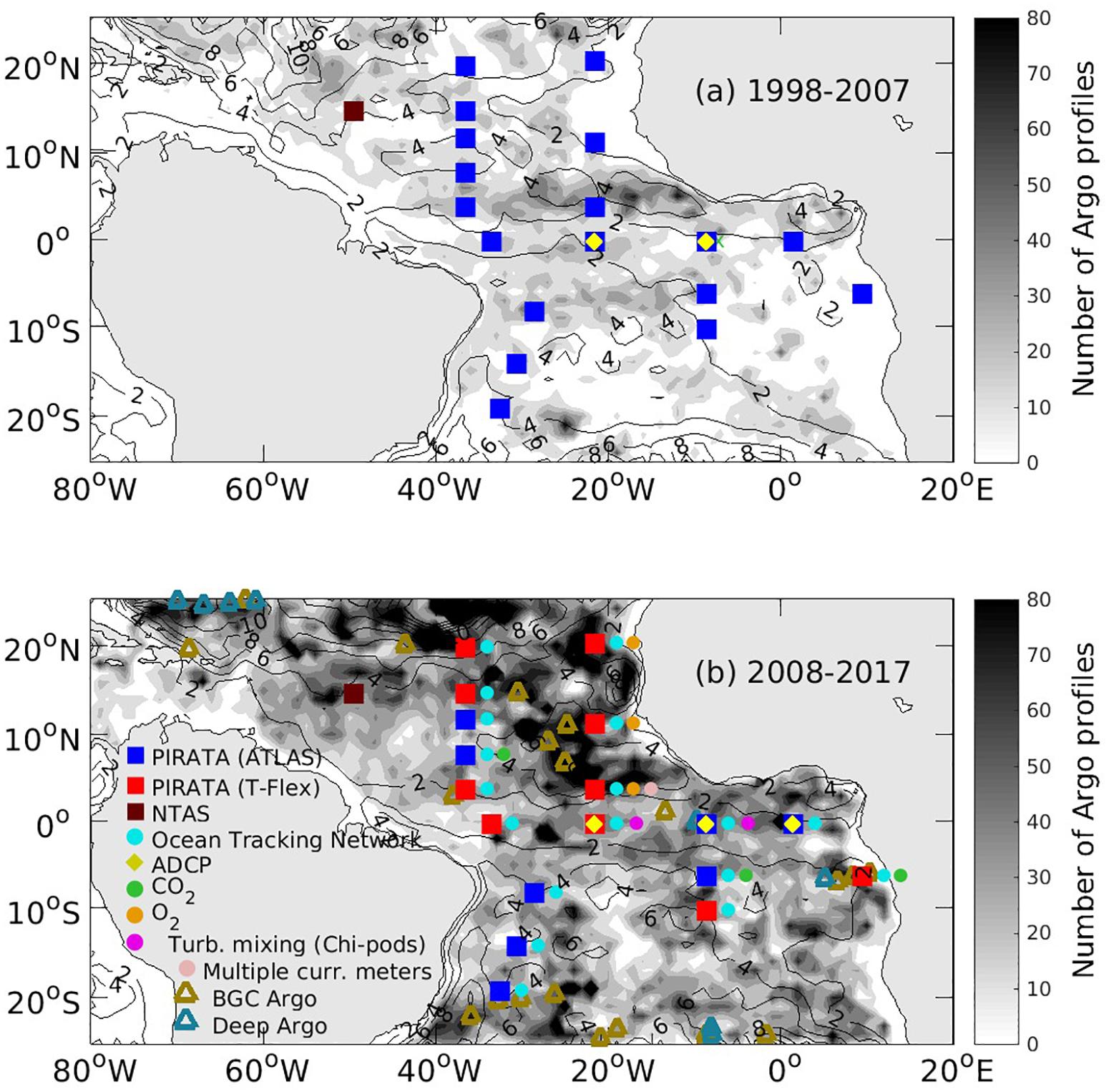
Figure 2. Key elements of the tropical Atlantic in situ observing system during (a) 1998–2007 and (b) 2008–2017. Gray shading represents the number of Argo profiles made in each 1° box. Contours show the average number of hourly surface drifter observations made in each 1° box per month. Squares show moored buoy locations, circles indicate additional measurements made from the moorings, and triangles show locations of deep Argo and biogeochemical Argo floats during September 2018.
Societal Drivers
Tropical Atlantic variability (TAV) influences a wide range of societally important phenomena on different timescales that span the physical, biogeochemical, and ecological systems and their interactions. This section summarizes the key societal drivers in the tropical Atlantic.
Rainfall
One of the most important climate- and weather-related societal drivers is continental rainfall. The impacts of tropical Atlantic climate variability on rainfall are strongest in South America and West Africa. The West African monsoon (WAM) brings most of the rainfall to West Africa during the year. Its onset is typically in late June or early July and it ends normally from late September to October (Sultan and Janicot, 2003). Knowledge of the development of the WAM is important for agricultural planning, as an unanticipated late onset and early demise of the monsoon can lead to crop failure. There is stronger interannual variability at the Guinea Coast compared to the Sahel, and rainfall anomalies often extend zonally across West Africa (Giannini et al., 2005), affecting large populations. A recent example is the 2011–2012 severe Sahel drought and famine, which was caused by below-normal and erratic rainfall in 2011 and poor harvests in 2011 and 20121.
The Sahel region also experiences strong rainfall variability on decadal and multidecadal timescales. There was a period of severe drought between the 1960s and 1980s that strongly impacted West African agriculture and economies. Rainfall in the Sahel has increased since the 1980s, but has not recovered to pre-drought levels (Nicholson et al., 2000; Dong and Sutton, 2015; Berntell et al., 2018). Through its impacts on soil moisture, vegetation, and albedo, the rainfall received during a given year affects the likelihood of drought the following year, acting to enhance decadal-multidecadal variations of Sahel rainfall (Zeng et al., 1999). The long-term trend of Sahel precipitation in response to the intensification of the hydrological cycle due to climate change is still unclear and may have significant consequences for West African populations (Druyan, 2011; Monerie et al., 2016).
The semiarid region of Northeast Brazil has also experienced strong rainfall variations and extreme droughts. This region is highly susceptible to drought because its short March–May rainy season is dependent on seasonal sea surface temperature (SST) and rainfall patterns in the tropical Atlantic. During 2011–2016, Northeast Brazil experienced its most severe and prolonged drought since 1981 (Brito et al., 2018). Prior to the recent drought period, Northeast Brazil experienced a severe flood in 2009 that has been linked to anomalous SSTs in the tropical Atlantic (Foltz et al., 2012). There is strong interannual and decadal variability of Northeast Brazil rainfall (Nobre and Shukla, 1996), along with a downward long-term trend of precipitation and increasing trend in air temperature (Lacerda et al., 2015).
During the years 2005–2012, the Amazon region experienced some of the most severe droughts and floods in its recorded history. The two record droughts of 2005 and 2010 were regarded as “once in a century” climate extremes at the times of their occurrences and were linked to anomalous SSTs in the tropical Atlantic (Figure 1; Marengo et al., 2008; Zeng et al., 2008; Lewis et al., 2011). The droughts occurred during the dry season (June–September), when the ecosystem is particularly vulnerable to stressors. Normally the Amazon is a carbon sink, absorbing CO2 from the atmosphere. However, during 2005–2008 the Amazon was a net carbon source due to drought-stressed and dying trees, combined with increased occurrence of fires (Zeng et al., 2008; Yang et al., 2018). At the other extreme, the Amazon floods of 2009 and 2012 were the largest going back several decades (Satyamurty et al., 2013; Filizola et al., 2014) and left hundreds of thousands homeless. Since the 1970s there has been an upward trend in the year-to-year variability of Amazon River discharge, following a period with no trend during 1904 through the 1960’s (Satyamurty et al., 2013; Barichivich et al., 2018). It is unclear whether the recent increase in variability is part of a longer trend driven by climate change or due to natural variability.
In summary, there is a strong societal need for accurate predictions of rainfall to improve agricultural productivity, allow for more efficient use of water resources, and protect homes and infrastructure against floods. There is also a need to mitigate disease outbreaks, which commonly occur following large floods. Rainfall predictions are needed on many different climate timescales, ranging from intraseasonal to multidecadal. Climate change projections of rainfall and the occurrences of droughts, floods, and extremes in rainfall intensity are also a necessity for developing countries surrounding the tropical Atlantic Ocean.
Tropical Cyclones
Tropical cyclones (TCs) are one of the deadliest and most destructive hazards in the tropics and subtropics. Threats include storm surge, damaging winds, and inland flooding from rainfall. Developing countries and low-lying coastal areas are particularly vulnerable. Since 2005, there have been eight Atlantic hurricanes that have resulted in at least $25 billion in damages, including Harvey, Irma, and Maria in 2017 and Florence in 20182,3. Adjusted for inflation, 9 of the 10 costliest hurricanes have occurred since 2004. The increasing destruction is likely a result of coastal population growth in the United States as well as natural and human-induced changes in the large-scale hurricane environment that can influence TC intensity, rapid intensification (RI), translation speed, and rainfall (Goldenberg et al., 2001; Knutson et al., 2010; Kossin, 2017; Scoccimarro et al., 2017; Balaguru et al., 2018; Kossin, 2018; Wang et al., 2018).
The Atlantic basin as a whole has experienced large variations in TC activity on interannual and longer timescales. The 2005, 2010, and 2017 Atlantic hurricane seasons were extremely active, with 28, 19, and 17 cyclones of at least tropical storm strength, respectively. The 2005 and 2017 seasons were the costliest on record at the time of occurrence. There has also been significant decadal-multidecadal variability of TC activity in the Atlantic, with above-normal activity during the 1940s and 50s, below-normal from the 60s to the early 90s, and above-normal since the mid-90s (Goldenberg et al., 2001). The magnitude of hurricane RI (increase in maximum wind speed of at least 25 kt in 24 h) has increased in the central and eastern tropical Atlantic since the 1980s (Balaguru et al., 2018). Whether a storm will undergo RI is particularly difficult to predict (Kaplan et al., 2010). When RI occurs before landfall, destruction and loss of life can be catastrophic.
Ultimately, in terms of seasonal prediction, what matters most for coastal residents and planning agencies is the number and severity of land-falling TCs. There have been marked changes in Atlantic land-falling TCs in the past several decades (Wang et al., 2011; Kossin, 2017). However, the number of landfalling TCs is very difficult to predict. There are indications that TC activity and intensification before landfall may be increasing in the Atlantic due to global warming (Emanuel, 2005, 2017; Webster et al., 2005; Elsner et al., 2008).
In summary, there is a need for improved intraseasonal and seasonal predictions of TC activity, including landfalls, and more reliable decadal-multidecadal projections. There are also uncertainties related to how Atlantic TC activity will change in response to global warming. This is particularly important for highly populated low-lying coastal areas in the southeastern United States, which will likely become more susceptible to storm surge inundation as sea level rises. Improved intraseasonal and seasonal predictions and longer-term projections will allow coastal communities to prepare and allocate resources for post-storm recovery.
Biogeochemistry
One of the important unknowns in the future global carbon budget is the extent to which the ocean sink keeps pace with anthropogenic CO2 emissions. Present-day observations and models show that the ocean sink has increased along with CO2 emissions and is currently absorbing about 28% of anthropogenic CO2 emissions annually (Le Quéré et al., 2018). The amount of CO2 that can be emitted by fossil fuel burning and industrial uses while limiting global surface temperature rise to within 2°C and stabilizing atmospheric CO2 levels is critically dependent on the magnitude of this ocean sink4. The tropical Atlantic is the second largest source, after the tropical Pacific, of oceanic CO2 to the atmosphere, releasing about 0.10 Pg C yr−1 in the 18°S–18°N region (Landschützer et al., 2014). While uptake of anthropogenic CO2 by the ocean regulates the atmospheric CO2 concentration, it also leads to ocean acidification, with significant but poorly understood consequences for marine organisms and ecosystems (Feely et al., 2004; Bates, 2007). Carbon trends remain unclear because of short records and high natural variability in the tropical Atlantic, though there are indications of significant decadal variations that have implications for anthropogenic CO2 uptake (Park and Wanninkhof, 2012). There is also significant interannual variability of air-sea CO2 fluxes in the tropical Atlantic that is closely linked to climate variability (Lefèvre et al., 2013; Ibánhez et al., 2017).
Oxygen minimum zones (OMZs) are found at intermediate depths (100–900 m) in the eastern tropical oceans off the equator (Karstensen et al., 2008). The OMZs in the eastern tropical Atlantic are split into shallow (100 m) and deep (400 m) branches (Monteiro et al., 2008; Brandt et al., 2015) that are caused primarily by enhanced biological productivity/consumption and sluggish ventilation, respectively. The shallow OMZs overlap with the euphotic zone and hence have a direct impact on ecosystems, carbon export, and the release of CO2 and other climate-relevant trace gases like N2O to the atmosphere. Since the 1960’s the OMZs in the eastern tropical Atlantic have been expanding (Stramma et al., 2008; Brandt et al., 2015), with far-reaching consequences for tropical ecosystems (Stramma et al., 2012; Gilly et al., 2013), nutrient cycling and resilience, as well as goods and services, including food production through fisheries and aquaculture, ecosystem conservation, and climate regulation (Diaz and Rosenberg, 2008; Stramma et al., 2012; Craig and Bosman, 2013; Kalvelage et al., 2013; Martinez-Rey et al., 2015; Arevalo-Martinez et al., 2015).
The carbon and oxygen cycles and ecosystems in coastal regions are influenced by river outflow and upwelling. The tropical Atlantic receives about 25% of the global riverine freshwater discharge from three large rivers (Amazon, Congo, and Orinoco; Dai and Trenberth, 2002). The rivers also deliver high loads of nutrients, which lead to high oceanic productivity near the river mouths. The tropical Atlantic includes two major eastern boundary upwelling systems that support some of the world’s most productive fisheries: the Canary and the Benguela (Chaigneau et al., 2009). These systems are particularly vulnerable to ongoing warming, deoxygenation and acidification (Gruber, 2011). It is important to understand what drives biological production within these regions in order to understand ecosystem dynamics and also to constrain the regional and global carbon cycles. There are several important factors that control biological production in eastern upwelling regions, including along-shore winds, eddy activity, and mixed layer depth (Lachkar and Gruber, 2012). Close to coastal areas, high productivity and local oxygen depletion are found, with concentrations below 30 μmol kg−1, and intense respiration and remineralisation, associated with high organic matter input, has been observed (Chen and Borges, 2009). Anoxic conditions regularly occur at the Namibian shelf (Brüchert et al., 2006; Mohrholz et al., 2008) and have been found more recently at the Senegalese shelf (Machu et al., 2019) and within mesoscale eddies (Karstensen et al., 2015; Schütte et al., 2016b). Low oxygen conditions affect the carbon cycle, ecosystems and fisheries. Trace elements (e.g., Zn, Fe, Co, and Mn) affect biomass and the turnover rate of phytoplankton, and ultimately the productivity of entire food webs. The cycling of these micronutrients is thus critically linked to carbon cycling.
Ecosystems and Pollution
Many coastal communities surrounding the tropical Atlantic Ocean rely on seafood for sustenance. The importance of fisheries in the tropical Atlantic can be demonstrated most easily by the total catch and dependence on the region. Approximately 10 million tons of seafood (from 87.2 million tons of global marine capture production) were harvested in the Central and South Atlantic in 20165. The fishing sector is also very important in tropical Atlantic coastal countries. There are 8 million fishers in Africa, Latin America, and the Caribbean, though not all of them are operating in the Atlantic; many foreign fleets (Korean, Chinese, Russian, European) also use tropical Atlantic resources. Threats to fisheries include overfishing, pollution, and invasive species.
The frequency of marine heatwaves has increased significantly in the tropical North Atlantic during the past 35 years and is expected to increase further in response to global warming (Oliver et al., 2018). Marine heatwaves are defined as anomalously warm SST that lasts for five or more days, with temperatures warmer than the 90th percentile based on a 30-year historical baseline period (Hobday et al., 2016). They can have detrimental effects on marine organisms, including coral bleaching, disease outbreaks, and forced migration (Comte and Olden, 2017; Hughes et al., 2018). Future responses of marine organisms to climate change and the implications for the biogeochemical cycles and fisheries are unclear.
Since 2011, there has been an increase in the abundance of Sargassum in the western tropical North Atlantic, often resulting in mass “beaching” events in the Caribbean (Wang and Hu, 2017) and also in western Africa and Brazil. These events can have significant negative impacts on local economies and ecology (Hu et al., 2016). It is unclear what has caused the increase in Sargassum in the western tropical North Atlantic and Caribbean. Hypotheses include changes in upper-ocean temperature and nutrients or anomalous winds and ocean currents. Previous events such as the Deepwater Horizon drilling rig explosion can also have serious negative consequences for local ecosystems and economies and require knowledge of the ocean circulation.
Transport and fishing vessels in the Atlantic are sources of marine pollution such as plastics, hydrocarbons, and particulate materials. Overall, there are relatively few records of pollutants that have emissions high enough to cause harmful consequences in the open ocean. Mercury and plastic pollution, nevertheless, show us that adverse effects of those pollutants in marine ecosystems can be widespread. Plastic is a pervasive pollutant of high concern. Its use has increased 20 times in the past 50 years and is expected to double in the next 20 years6. A high amount of plastic materials escapes collection systems, generating significant risks for marine biota. Their threats to marine life are primarily mechanical, due to ingestion of plastics and entanglement (Derraik, 2002). It has also been shown that contaminants from plastic debris may leach into seawater or be ingested by marine organisms (Romera-Castillo et al., 2018), creating a new risk route.
Ecosystems are highly dependent on nutrient availability and biogeochemistry, which themselves are closely linked to the physical state of the ocean. There is a strong need to monitor fish stocks and understand their variability and response to internal and external stressors. External threats include overfishing, invasive species, global warming, deoxygenation, and pollution. Observations are needed for monitoring and scientific understanding, and models that incorporate biogeochemistry and ecosystems are critical for informing scientists and policy-makers of future changes to tropical Atlantic ecosystems.
Science Drivers
This section summarizes the key scientific phenomena and processes that affect the societally relevant issues presented in the previous section.
Modes of Variability and Tropical Cyclones
Many societally relevant phenomena in the tropical Atlantic region, such as droughts, floods, TCs, and marine heat waves, are linked to TAV. TAV involves coupled ocean-atmosphere processes and their interactions, most notably, fluctuations of the trade winds, SST, and rainfall (Figure 3; Xie and Carton, 2004). There is also significant external forcing of TAV from ENSO, the North Atlantic Oscillation, and the South Atlantic Anticyclone (Enfield and Mayer, 1997; Czaja et al., 2002; Chang et al., 2006; Illig and Dewitte, 2006; Lübbecke et al., 2010; Lübbecke and McPhaden, 2012). Interannual variability of the tropical Atlantic can be described in terms of two main climate modes: the Atlantic Zonal Mode (AZM) and the Atlantic Meridional Mode (AMM). The AZM, also commonly referred to as the Atlantic Niño and Atlantic equatorial mode, is associated with SST anomalies near the equator, peaking in the eastern basin (Zebiak, 1993; Polo et al., 2008; Lübbecke et al., 2018), while the AMM is characterized by a cross-equatorial gradient of SST and wind anomalies (Ruiz-Barradas et al., 2000; Chiang and Vimont, 2004). Their patterns and seasonality are depicted in Figure 4.
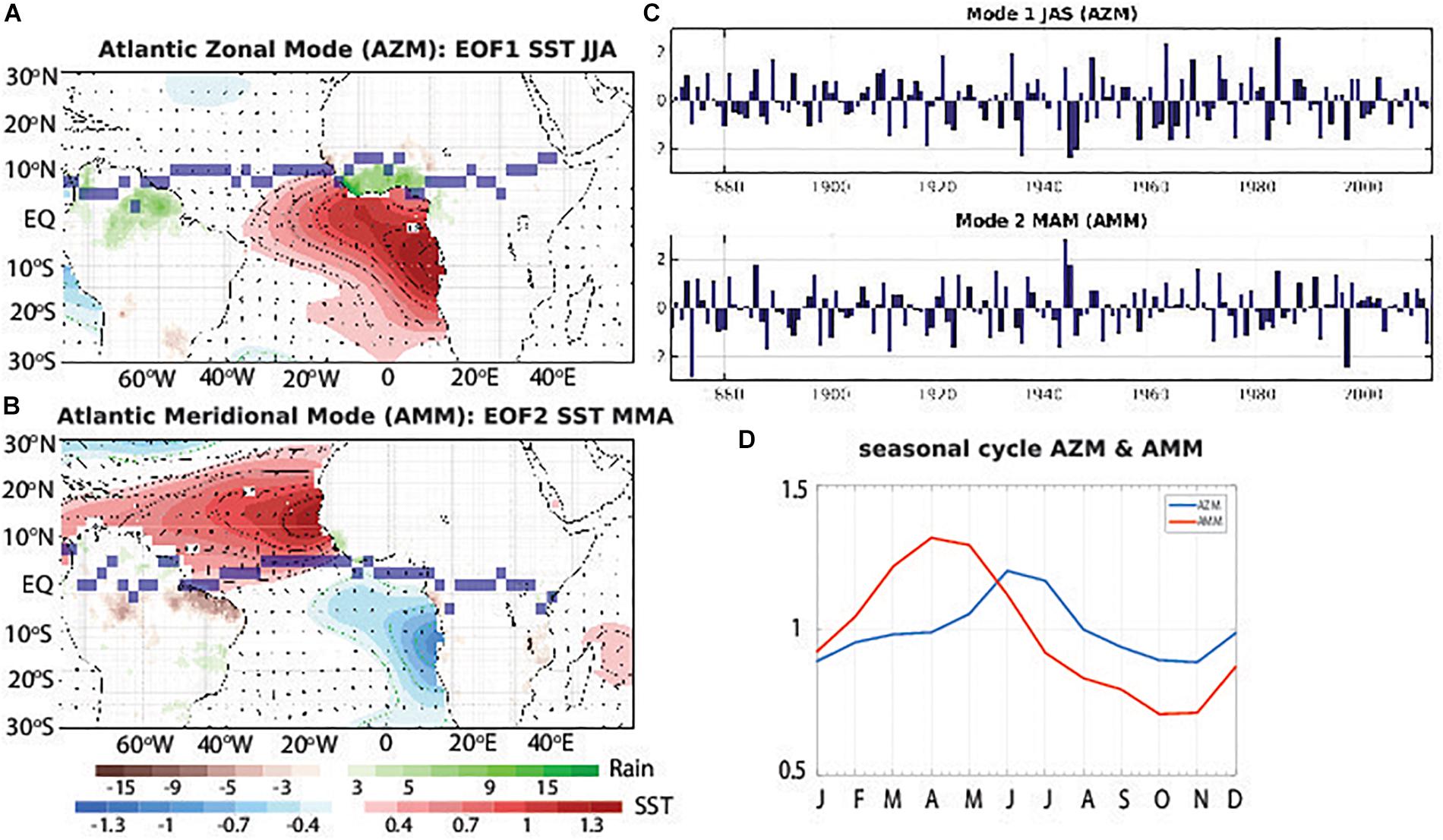
Figure 4. Leading modes of tropical Atlantic SST variability. Shown are the composites of events characterized by a principal component value > 1 standard deviation, minus those events in which the principal component has values < –1 standard deviation. The modes are calculated from Had-SST data (°C) using an 11-year high pass filter. (A) Composite of the leading mode in June–July–August for SST (shading over ocean), rainfall in cm mo−1 from University of Delaware (shading over land; data are available from http://climate.geog.udel.edu/~climate/html_pages/Global2011/README.GlobalTsP2011.html), and surface wind from NCEP 20th Century Reanalysis. (B) As in panel (A) but for the second mode. Purple shading in panels (A) and (B) indicates the seasonal mean position of the ITCZ. (C) Interannual times series of the first and second EOF modes. (D) Evolution of the standard deviation of the leading EOF (blue) and second EOF (red) corresponding to the Atlantic Zonal Mode (AZM) and Atlantic Meridional Mode (AMM), respectively.
On interannual timescales, the AZM affects the WAM (Sultan and Janicot, 2003; Polo et al., 2008; Losada et al., 2010; Nicholson, 2013). A warm phase of the AZM shifts the intertropical convergence zone (ITCZ) anomalously to the south during June–August, increasing rainfall over the Gulf of Guinea (Figure 4A; Janicot et al., 1998; Okumura and Xie, 2004; Polo et al., 2008; Joly and Voldoire, 2010; Losada et al., 2010; Rodríguez-Fonseca et al., 2011, 2015). A positive AZM is also associated with a late onset of the WAM, though eastern tropical North Atlantic SST also plays a role (Brandt et al., 2011a). An earlier development of a warm phase of the AZM can enhance the southward migration of the ITCZ, bringing excess rainfall to the Brazilian Amazon and Northeast Brazil (Torralba et al., 2015). The tropical Pacific also influences rainfall in these regions (Moron et al., 1995; Nobre and Shukla, 1996; Janicot et al., 2001; Rowell, 2001) and affects the AZM (Chang et al., 2006).
A positive AMM favors an earlier migration of the ITCZ northward during April–May, shortening the rainfall season over the Amazon region and Brazilian Northeast and leading to severe droughts over the Northeast (Figures 4B,C; Nobre and Shukla, 1996; Hastenrath, 2006; Kucharski et al., 2008; Liebmann and Mechoso, 2011; Rodrigues et al., 2011). The opposite is generally true for negative AMM phases (Foltz et al., 2012; Rodrigues and McPhaden, 2014). There is a strong relationship between the AMM and Atlantic hurricane activity (Kossin and Vimont, 2007) due to the AMM’s influence on SST, position of the ITCZ, strength of vertical wind shear, and humidity (DeMaria and Kaplan, 1994).
Atlantic Multidecadal Variability (AMV; Kerr, 2000) modulates Sahel rainfall through its control of the Atlantic ITCZ (Knight et al., 2006; Zhang and Delworth, 2006; Mohino et al., 2011; Dieppois et al., 2015). There is also strong evidence linking decadal-multidecadal tropical Atlantic SST variability to hurricane activity (Goldenberg et al., 2001; Latif et al., 2007; Balaguru et al., 2018), but the drivers of this SST variability are not well known (Yang, 1999; Chang et al., 2000; Tanimoto and Xie, 2002; Evan et al., 2011; Booth et al., 2012; Clement et al., 2015). Several studies have demonstrated that there is potential for improved seasonal predictions of hurricane landfall frequencies and intensities if the predicted atmospheric steering flow, wind shear, and SST patterns are taken into account (Wang et al., 2011; Kossin, 2017), further emphasizing the importance of tropical Atlantic SST variations and their interactions with the atmosphere.
The influence of interannual TAV on the West African and South American monsoons is not stationary and can be modulated by its interactions with variability from other tropical oceans (Losada et al., 2012; Torralba et al., 2015). TAV also has robust imprints on global climate. The AZM impacts the Indian summer monsoon (ISM), altering the ENSO-ISM connection (Kucharski et al., 2007, 2008; Wang et al., 2009; Barimalala et al., 2012, 2013). Summer equatorial variability associated with the AZM is also highly correlated with the next winter’s ENSO (Polo et al., 2008; Rodríguez-Fonseca et al., 2009; Ding et al., 2012; Keenlyside et al., 2013; Martín-Rey et al., 2015). The AZM-ENSO relationship is strongest during negative AMV phases (Martín-Rey et al., 2014; Polo et al., 2015a), when equatorial Atlantic SST variability is enhanced (Martín-Rey et al., 2018). A possible connection between the tropical North Atlantic and tropical Pacific variability has also been suggested (Wu et al., 2007; Ham et al., 2013a,b; Wang et al., 2017), and it seems to be more active during positive AMV phases (Wang et al., 2017). However, global warming may also affect this teleconnection (Dong and Zhou, 2014; Liu and Sui, 2014). Improved understanding of interactions between the tropical oceans is needed to advance seasonal to decadal climate predictions and climate change projections (Cai et al., 2019). Sustained observations in the tropical Atlantic are a key requirement for achieving this goal.
Despite significant progress in understanding TAV and its impacts during the last decades, many open questions remain. Dynamical mechanisms for the generation of the AZM (Keenlyside and Latif, 2007; Polo et al., 2015b; Jouanno et al., 2017) have been questioned and, mostly based on model simulations, important roles for thermodynamic processes proposed (Nnamchi et al., 2015, 2016). The importance of thermodynamic forcing is likely amplified in models with enhanced SST bias (Jouanno et al., 2017). Advection and equatorial deep jets (EDJ; vertically alternating zonal currents) also affect the AZM (Brandt et al., 2011b; Richter et al., 2013).
Another open question concerns the relationship between the AZM and other modes of climate variability in the tropical Atlantic. Warm events that occur in the southeastern tropical Atlantic off Angola and Namibia have been termed Benguela Niños (Shannon et al., 1986). They have a pronounced impact on fisheries in coastal areas (e.g., Boyer and Hampton, 2001) and rainfall over south-western Africa (Rouault et al., 2003). SST anomalies in the eastern equatorial to subtropical South Atlantic that covary with anomalies of opposite sign in the southwestern subtropical South Atlantic have been described as the South Atlantic Ocean Dipole (SAOD, Venegas et al., 1996; Morioka et al., 2011; Nnamchi et al., 2011, 2016; Rouault et al., 2018). In addition to having similar climatic impacts on adjacent continents as the AZM, the SAOD has been linked to the Antarctic Oscillation and rainfall anomalies over the southern parts of Africa and South America (Nnamchi et al., 2011; Morioka et al., 2011, 2014). The Benguela Niño and SAOD have been linked to the AZM (Lübbecke et al., 2010; Richter et al., 2010; Nnamchi et al., 2016, 2017; Rouault et al., 2018), but it is unclear whether the Benguela Niño and AZM are part of the same climate mode or closely related but distinct modes (Polo et al., 2008; Goubanova et al., 2013; Bachèlery et al., 2016a,b; Illig et al., 2018a,b; Illig and Bachèlery, 2019). Intraseasonal wind bursts seem to play an important role in AZM evolution during some years (Marin et al., 2009; Herbert and Bourlès, 2018). The AZM has been shown to impact surface chlorophyll-a concentration in the eastern equatorial Atlantic (Grodsky et al., 2008), but its broader effect on primary productivity is still unknown.
Other key questions relate to the nature and importance of specific SST feedbacks onto the atmosphere. Bjerknes and wind-evaporation-SST feedbacks in particular are considered to be essential elements of the AZM and AMM, respectively. Both feedbacks involve the horizontal adjustment of the sea level pressure gradients to SST gradients (Lindzen and Nigam, 1987; Young, 1987). However, they are complicated by other factors such as the vertical adjustment of the planetary boundary layer to SST (Sweet et al., 1981; Hayes et al., 1989; Wallace et al., 1989) and the effects of higher atmospheric levels on surface pressure (Richter et al., 2014a; Diakhaté et al., 2016).
There are many open questions regarding the way the spatial patterns of the SST modes (Losada and Rodríguez-Fonseca, 2016), their interactions with the extratropical Atlantic and other tropical basins (Czaja et al., 2002; Losada et al., 2012), and changes in the climatological background states can affect rainfall regimes (Suarez-Moreno and Rodríguez-Fonseca, 2018) and TC activity (Latif et al., 2007; Vecchi and Soden, 2007; Kossin, 2017). There is still a lot of uncertainty with respect to the air-sea interactions linking the AZM and AMM (Servain et al., 1999; Andreoli and Kayano, 2003; Foltz and McPhaden, 2010a,b; Richter et al., 2013, 2014a; Burmeister et al., 2016) and the processes responsible for the AMM, including its relation to coastal and open-ocean upwelling (Doi et al., 2009; Evan et al., 2011; Foltz et al., 2012; Rugg et al., 2016).
Tropical cyclones are strongly influenced by the underlying SST, which in turn is affected by TAV. TCs also typically induce a cold wake of upper-ocean temperatures that can provide a negative feedback on their intensities. The strength of the feedback depends on a storm’s intensity and translation speed as well as the ocean heat content and salinity structure, which vary regionally and on seasonal to multidecadal timescales (Shay et al., 2000; Balaguru et al., 2012, 2015, 2018). This is especially true in the northwestern tropical Atlantic, where the Amazon-Orinoco plume increases salinity stratification, limiting hurricane-induced SST cooling (Figure 5; Balaguru et al., 2012; Grodsky et al., 2012b; Domingues et al., 2015). The impact of interannual to multidecadal changes in upper-ocean temperature and salinity stratification on TCs’ cold wakes and intensities has only begun to be explored (Huang et al., 2015; Balaguru et al., 2016). A complicating factor in the study of TAV and TC activity is that some of the largest SST biases in global climate models occur in this region (see section “Predictability and Model Biases”).
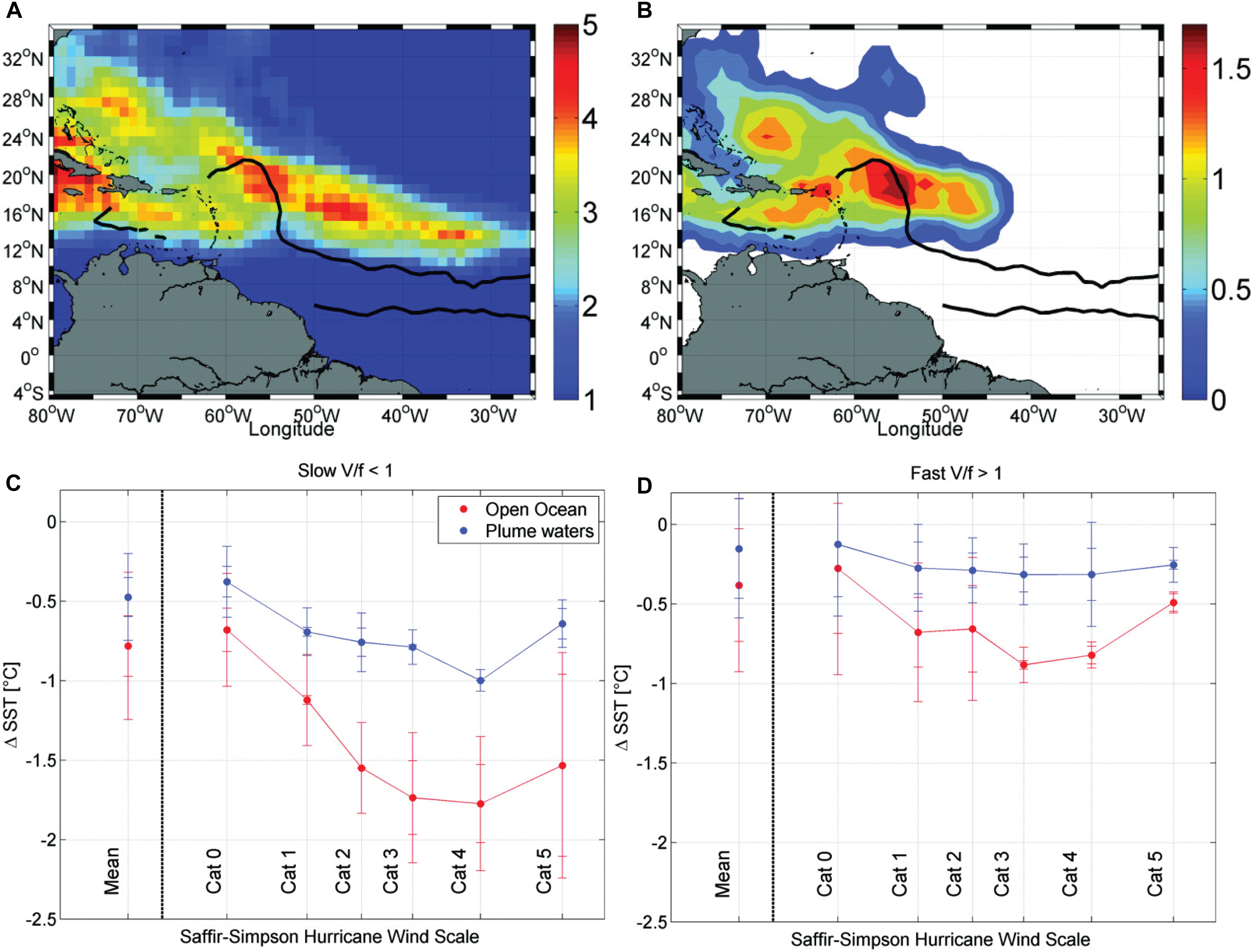
Figure 5. The number of 1950–2010 “best track” TCs per 1° box (smoothed by a 3° × 3° block average) (A) that evolve as categories 4 and 5 somewhere along their path and (B) that intensified locally to categories 4 and 5. The black curve shows the historical extent of the Amazon–Orinoco river plume during the hurricane peak season (August to October). (C,D) Mean sea surface cooling induced by North-Atlantic hurricanes as a function of the Saffir-Simpson wind scale, with error bars showing the 90 and 95% significance levels. Responses estimated over open ocean waters (red dots) are distinguished from those evaluated within the historical plume region (blue dots). (C) Slow-moving (or low latitude) tropical cyclones with V/f < 1 (V is translation speed and f is Coriolis parameter), (D) fast moving (or high-latitude) storms with V/f > 1. Adapted from Reul et al. (2014).
All of the aforementioned climate variations occur in a changing climate. Significant trends in tropical Atlantic SST, surface salinity, upper-ocean heat content, winds, cloudiness, and rainfall have emerged in the past decade (Tokinaga and Xie, 2011; Durack et al., 2012; Servain et al., 2014). It is unclear how these changes are affecting continental rainfall and the frequencies of droughts and floods (Elsner et al., 2008; Trenberth et al., 2014). There is robust forcing of the tropical Atlantic from the tropical Pacific on interannual and longer timescales (Enfield and Mayer, 1997; Chiang et al., 2002; Villamayor and Mohino, 2015). These teleconnections are likely to change with the varying mean states of the Pacific and Atlantic. The frequency of extreme El Niño and La Niña events is likely to increase (Cai et al., 2014, 2015a,b), which will affect the tropical North Atlantic ocean-atmosphere system. An improved understanding of TAV and its interactions with a warming climate can also potentially improve ENSO seasonal forecasts (Keenlyside et al., 2013; Martín-Rey et al., 2015; Dommenget and Yu, 2017). There is growing evidence that Atlantic hurricane activity will be affected by climate change (Grossmann and Morgan, 2011; Walsh et al., 2016; Sobel et al., 2016). Theory and numerical models predict that as SST rises, the maximum potential intensity that storms can reach will increase, enabling more powerful hurricanes (Emanuel, 1999; Elsner et al., 2008). Climate models predict increases in wind shear in some portions of the Atlantic hurricane development region (Latif et al., 2007; Vecchi and Soden, 2007), which would, however, act to decrease overall Atlantic storm activity.
Observational needs for tropical Atlantic modes of variability and TCs include long data records of upper-ocean and near-surface atmospheric parameters. These are required for improved understanding, monitoring, and predictability on seasonal to multidecadal timescales, including global warming. Of particular importance are dense measurements in the oceanic mixed layer and of air-sea heat, moisture, and momentum fluxes. The northwestern and southeastern tropical Atlantic should be high priority regions for additional measurements because of their importance for TCs and the equatorial Atlantic modes of variability, respectively.
Processes That Affect Upper-Ocean Temperature and Salinity
It is essential to understand and monitor changes in tropical Atlantic SST and near-surface salinity in order to advance understanding of the climate system, improve models, and address many of the outstanding societal challenges described in Section “Societal Drivers”. The tropical Atlantic seasonal cycle, TAV, and TC activity are driven to a large extent by changes in SST. Upper-ocean salinity affects vertical mixing and SST (Breugem et al., 2008; Balaguru et al., 2012) and is an important indicator of changes in the hydrological cycle (Durack et al., 2012). Many of the processes that control near-surface temperature and salinity (vertical mixing, air-sea fluxes) also drive variations of biogeochemical quantities such as CO2, O2, and nutrients. These parameters are discussed only briefly here and in more detail in Section “Biogeochemistry, Ecosystems, and Pollution”.
Observational and modeling studies of the equatorial mixed layer heat balance have demonstrated the importance of vertical turbulent mixing for generating seasonal cooling of SST in the equatorial Atlantic (Foltz et al., 2003; Peter et al., 2006). Progress has been made identifying processes responsible for the seasonal cycle of vertical turbulent mixing such as shear from the background currents, intraseasonal tropical instability waves (TIWs) and wind-driven waves, and the diurnal cycle in the mixed layer (Foltz et al., 2003; Jouanno et al., 2011; Giordani et al., 2013; Hummels et al., 2013; Wenegrat and McPhaden, 2015; Scannell and McPhaden, 2018). Away from the equator, surface heat fluxes play a more dominant role (Nobre et al., 2012; Foltz et al., 2013, 2018; Cintra et al., 2015; Nogueira Neto et al., 2018). However, there remain significant seasonal variations in the heat budget residuals (i.e., changes in mixed layer heat content that cannot be explained by the net surface heat flux) at some off-equatorial locations, implying that vertical mixing and other processes may be important (Figure 6; Foltz et al., 2018). The residuals are particularly large in eastern upwelling regions, consistent with previous studies (Foltz et al., 2013; Faye et al., 2015; Scannell and McPhaden, 2018). Outside of upwelling regions, there is evidence that near-inertial wave-induced mixing can have a significant impact on SST (Jochum et al., 2013). There is debate about the impact of salinity stratification on vertical mixing and SST in the Amazon-Orinoco River plume region of the northwestern tropical Atlantic (Balaguru et al., 2012; Hernandez et al., 2016). An ongoing challenge is to correctly represent ocean-wave-atmosphere coupling, which is important because of its impacts on turbulent heat and momentum fluxes (Belcher et al., 2012; Qiao et al., 2016; Reichl et al., 2016; Aijaz et al., 2017; Stoney et al., 2017; Bruneau et al., 2018). The parameterizations used in coupled climate and hurricane forecast models often have not been confirmed by observations. Uncertainties remain in large part because of very few long time series (one year or longer) of vertical mixing and its driving forces (e.g., current shear, temperature and salinity stratification) at off-equatorial locations.
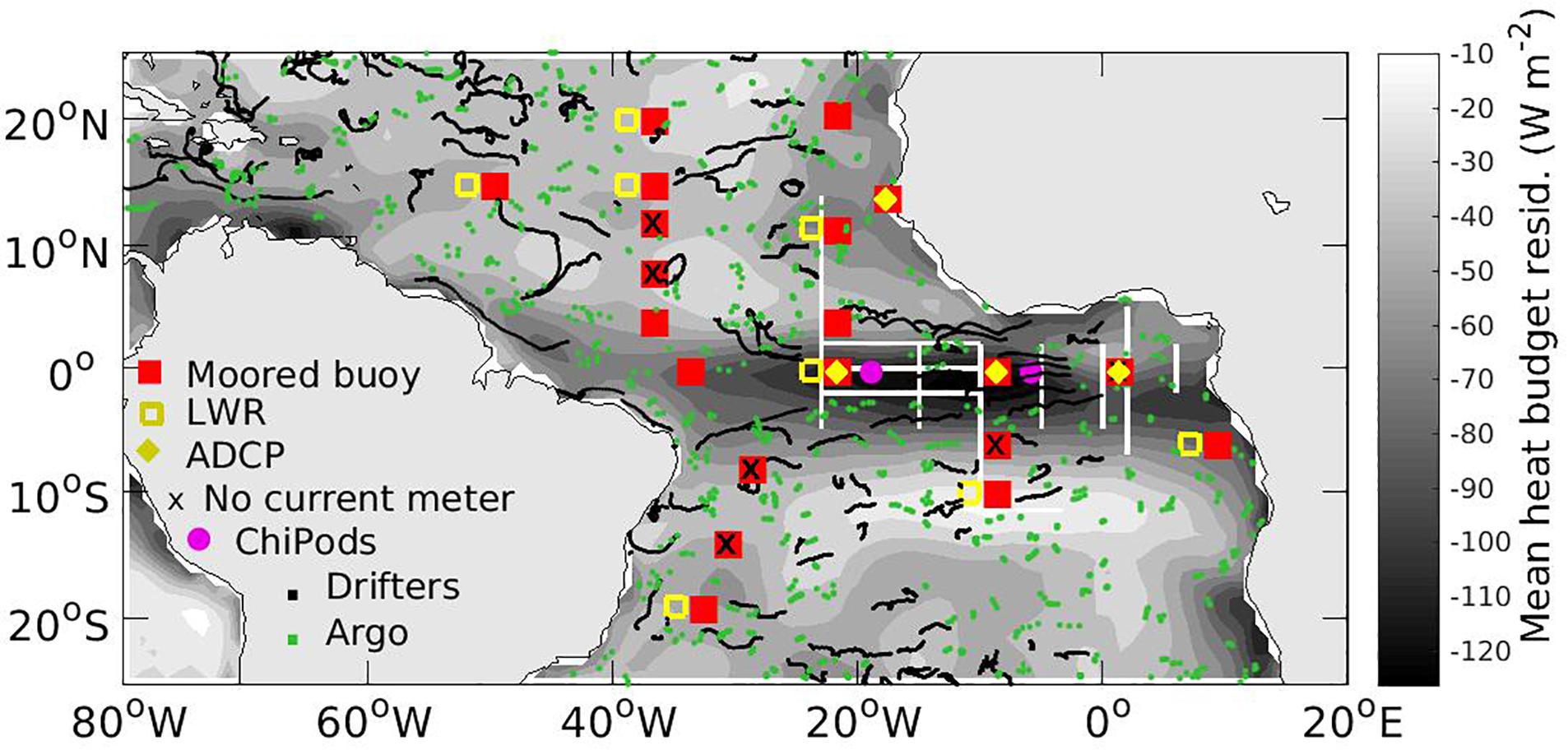
Figure 6. Elements of the mixed layer heat budget and vertical mixing observing system. Black trajectories show the tracks of all surface drifters during August 2018. Green dots indicate locations of Argo floats in August 2018. Yellow squares and diamonds show mooring locations with longwave radiation sensors and ADCPs, respectively. Purple circles indicate locations with turbulence measurements, and a black ‘x’ indicates that the mooring does not have a near-surface current meter. Shading shows the annual mean heat budget residual (2003–2017), an estimate of the sum of horizontal heat advection and vertical turbulent cooling, calculated using TropFlux surface heat fluxes, blended satellite microwave-infrared SST, and mixed layer depth calculated from monthly gridded EN4 ocean temperature and salinity. White lines show the locations of ship-based and glider-based microstructure measurements conducted during 2005–2011.
One major difference between the mixed layer heat and salinity budgets is that for the salinity budget, horizontal advection is generally much more important (Foltz and McPhaden, 2008; Da-Allada et al., 2013, 2017; Camara et al., 2015). This is due to multiple factors, including stronger spatial gradients of the surface freshwater flux due to precipitation and river outflow and the fact that sea surface salinity (SSS) anomalies are not damped by the atmosphere, in contrast to SST anomalies. On interannual timescales, there is some evidence that changes in ocean circulation dominate in the western tropical Atlantic (Coles et al., 2013; Foltz et al., 2015). However, interannual variations in Amazon outflow can also contribute (Zeng et al., 2008). The balance between ocean dynamics and changes in Amazon discharge is still not well understood. For example, the Amazon plume covered less area during the Amazon flood year of 2012 than in 2011 (Grodsky et al., 2014).
Turbulent mixing in the upper thermocline above the upper continental slopes and shelves of the eastern boundary upwelling regions is a dominant process bringing colder, nutrient-rich water from the deeper ocean to the surface mixed layer. Mixing is enhanced due to tide-topography interaction that largely sustains the elevated productivity in the Atlantic’s eastern boundary upwelling regions (Schafstall et al., 2010). In the deeper thermocline away from continental margins and varying topography, turbulent mixing processes are weak. Nevertheless, about 30% of the oxygen consumed in the OMZs of the eastern tropical Atlantic is replenished by interior ocean mixing processes sustained through internal wave-wave interaction (Brandt et al., 2015).
Since direct measurements of surface heat fluxes are available only at limited locations, on basin and global scales they are estimated. The estimation contains uncertainty, which hampers our ability to accurately quantify air-sea thermodynamic interactions and heat budgets in the tropical Atlantic (Frankignoul and Kestenare, 2005; Pinker et al., 2014; Bentamy et al., 2017). Surface turbulent fluxes are computed via bulk flux parameterizations using surface meteorological variables that can be obtained from ship reports and satellite remote sensing. Uncertainties in near-surface air temperature and humidity are the leading sources of uncertainties for satellite-derived products (Prytherch et al., 2015). This is mainly because satellites cannot retrieve the variables a few meters above the sea surface and instead rely on empirically derived algorithms applied to total-column water vapor or precipitable water (Liu et al., 1991).
The spread in net surface heat flux (Qnet) mean values is large across the entire tropical basin (standard deviation > 20 W m−2 based on 12 different products; Figure 7). In the tropical Atlantic away from the equatorial cold tongue, the standard deviation of Qnet is as large as the ensemble-mean Qnet. All Qnet products have problems achieving a balanced energy budget at the ocean surface, with an overestimation of the downward heat input to the ocean ranging from 5 to 20 W m−2 (Yu, 2019). For reanalysis fluxes, there are major uncertainties in tropical shortwave and longwave radiation associated with the long-standing problems of parameterizing tropical convective clouds and low-altitude stratocumulus clouds in reanalysis models (Trolliet et al., 2018). For satellite fluxes, major uncertainties are related to turbulent bulk flux parameterization schemes and also satellite retrieval algorithms. In situ data are crucial for anchoring global efforts to develop a global climate observing system (Weatherhead et al., 2018) and discriminate the imbalance in the Earth’s radiation budget as the climate warms (Kato et al., 2013). Both reanalysis and satellite fluxes are much in need of in situ validation data in climatologically overcast regions such as the northeastern and particularly the southeastern tropical Atlantic (Zuidema et al., 2016b). Direct measurements of the surface heat flux have also proven valuable for diagnosing air-sea coupling in the southwestern tropical Atlantic near the Brazilian coast (Chaves and Nobre, 2004; De Almeida et al., 2007).
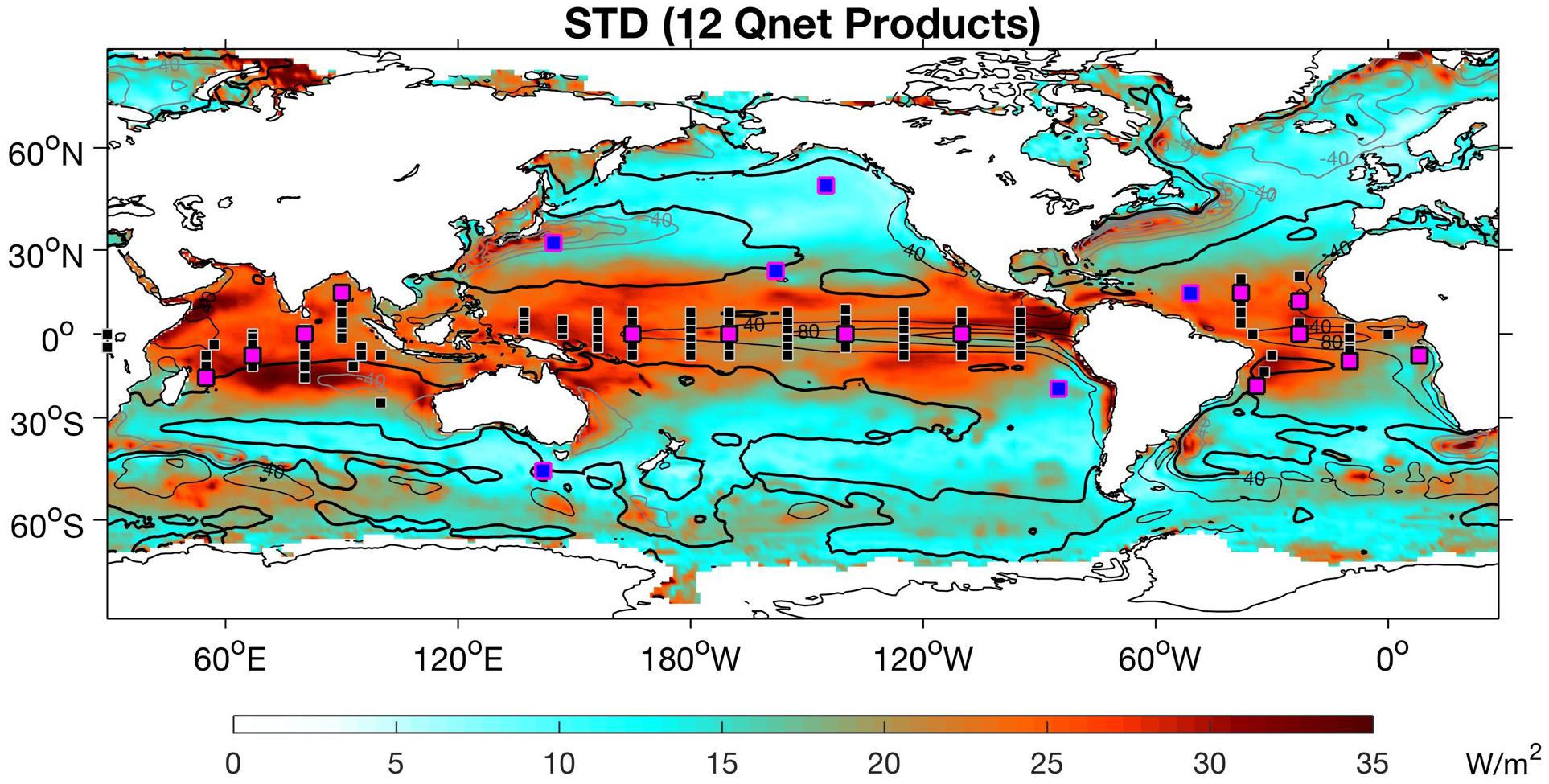
Figure 7. Standard deviations between 12 mean surface heat flux (Qnet) products (colors) superimposed on the ensemble mean Qnet (contours; zero lines are highlighted). The means for all products are constructed over the same 10-year period between 2001 and 2010. Black squares denote the tropical moored arrays (PIRATA, TAO/TRITON, and RAMA) with net heat flux reference sites denoted by large magenta squares. Blue squares outlined in magenta denote OceanSITES buoys (NTAS in the tropical Atlantic). Adapted from Yu (2019).
To make further progress on understanding and monitoring upper-ocean processes, continued measurements of ocean temperature, salinity, and velocity are needed. These observations must be capable of resolving the oceanic mixed layer and upper thermocline on diurnal to decadal timescales. Measurements of turbulent mixing and the processes that drive it, including vertical current shear, stratification, and surface buoyancy and momentum fluxes, are required both in the equatorial and off-equatorial regions. In situ measurements of surface fluxes are required throughout the tropical Atlantic and especially in cloudy regimes such as the northeastern and southeastern tropical Atlantic, and basin-scale measurements of surface parameters such as SST, surface salinity, winds, and currents are extremely valuable.
Ocean Circulation
The circulation of the tropical Atlantic Ocean is an important measure of the state of the climate system. The mean circulation sets the background conditions for the distributions of heat, salt, carbon, oxygen, nutrients, and other tracers. Due to its connection with subtropical and higher latitude regions, the tropical Atlantic circulation also affects the distributions of these quantities outside of the tropical belt. The Atlantic Meridional Overturning Circulation (AMOC) and subtropical cells (STCs) are examples of basin-wide and regional circulations, respectively, that are influenced by and interact with the circulation in the tropical Atlantic and affect tropical Atlantic climate and extreme weather (Zhang et al., 2003; Knight et al., 2005; Zhang and Delworth, 2006). At the thermocline level, the Equatorial Undercurrent (EUC) flows eastward and supplies equatorial upwelling. The strength of the EUC undergoes a strong seasonal cycle in response to wind forcing (Johns et al., 2014; Brandt et al., 2016). Interannual variability in the equatorial and eastern boundary circulation due to equatorial and coastally trapped waves define the propagation characteristics of warm and cold anomalies of the AZM and Benguela Niño (Lübbecke et al., 2010).
The eastern tropical Atlantic on both sides of the equator is occupied by OMZs, which result from sluggish ventilated shadow zones (Luyten et al., 1983) situated equatorward of the subtropical gyres. In addition to vertical mixing and lateral eddy fluxes (Fischer et al., 2013; Hahn et al., 2014), latitudinally alternating zonal jets play a dominant role in the ventilation of the Atlantic OMZs (Brandt et al., 2010, 2012). A unique feature of the eastern tropical North Atlantic is the presence of dead-zones that can develop in closed mesoscale eddy cores. Oxygen can drop to zero in these eddies (Karstensen et al., 2015), substantially lower than the average value of 40 μmol kg−1. These events act to intensify the OMZ in the upper 200 m and have severe direct impacts on the local ecosystem (Schütte et al., 2016b).
At the equator, EDJs contribute to the ventilation of the eastern basin (Brandt et al., 2012). The shorter period of EDJs in the Atlantic compared to the Pacific, combined with the strong and shallow EUC in the eastern equatorial Atlantic, enables EDJs to influence interannual climate variability in the Atlantic (Brandt et al., 2011b). On intraseasonal timescales, variability in the equatorial Atlantic is dominated by TIWs in the western and central part and by wind-driven waves in the east (Athie and Marin, 2008). These waves significantly contribute to the upper-ocean heat and freshwater budgets through their influence on horizontal and vertical mixing (Foltz et al., 2003; Jochum et al., 2004; Hummels et al., 2013) and provide energy to EDJs via downward propagating Yanai beams (Tuchen et al., 2018). Moreover, there is evidence that mesoscale ocean dynamics significantly affect the tropical Atlantic Ocean and overlying atmospheric variability across a large range of time scales from daily to interannual and interdecadal (Seo et al., 2007; Chelton and Xie, 2010). Eddies are generated in the eastern side of the basin connecting the eastern boundary upwelling systems with the open oceans, transporting oxygen-poor and nutrient-rich waters into the oligotrophic ocean and impacting the mean state (Schütte et al., 2016a,b). At the western boundary, North Brazil Current (NBC) rings (Figure 8) and Deep Western Boundary Current (DWBC) eddies are generated and are responsible for part of the water mass transport within the tropical AMOC (Goni and Johns, 2001; Dengler et al., 2004; Goes et al., 2009). NBC rings interact with the Amazon and Orinoco river plumes, modifying barrier layers and thus affecting TC intensification.
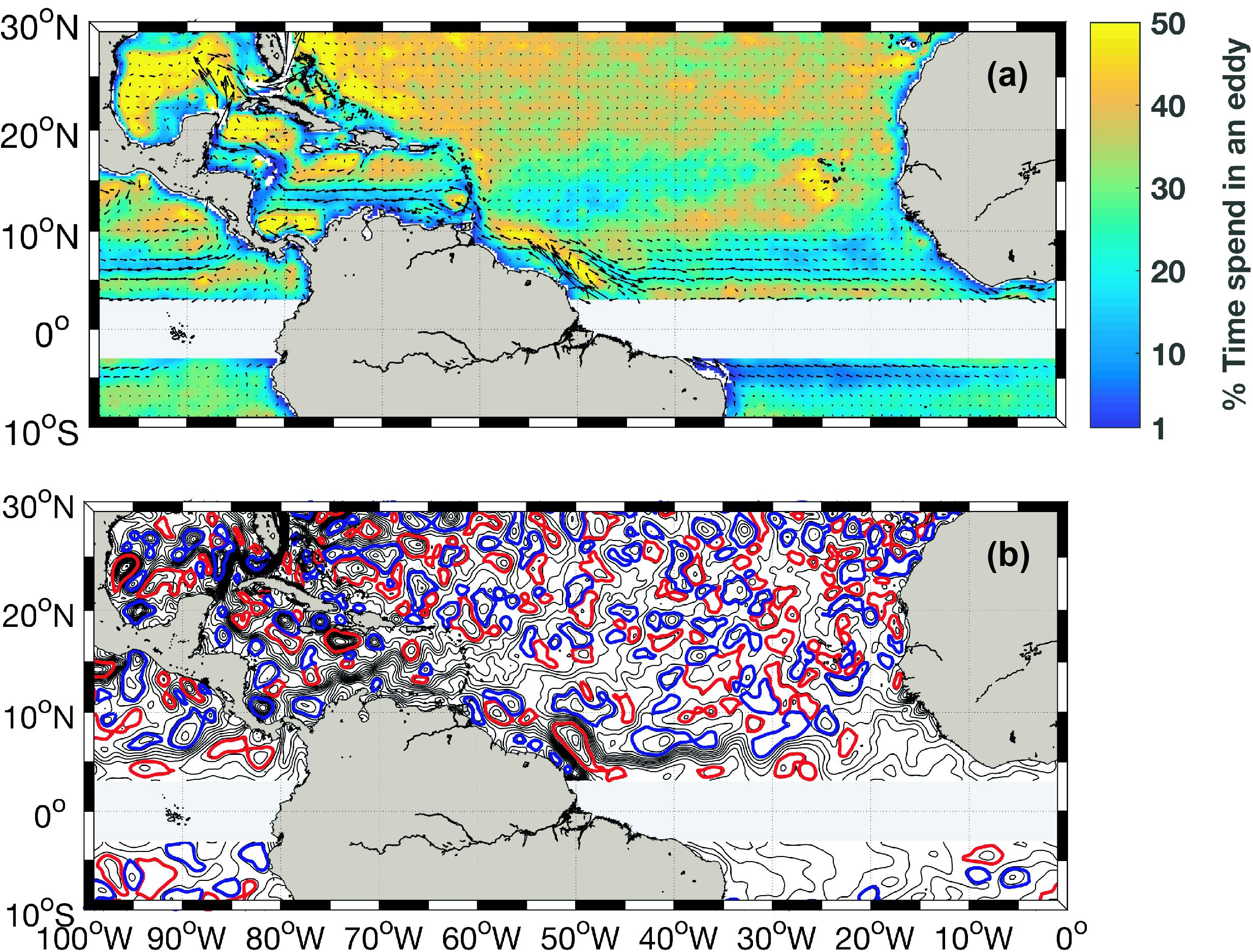
Figure 8. (a) Shading shows the percentage of time each 1° × 1° box is within an eddy, based on the eddy detection algorithm of Laxenaire et al. (2019). Vectors represent the annual mean surface currents from Aviso Ssalto/Duacs and distributed by the Copernicus Marine Environment Monitoring Service (http://marine.copernicus.eu/) in the version released in April 2014 (Duacs/Aviso+, 2014; Pujol et al., 2016). (b) Absolute Dynamic Topography (ADT) field from Aviso Ssalto/Duacs, based on satellite altimetry on July 1, 2017, showing a well-defined North Brazil Current retroflection and North Equatorial Countercurrent in the western tropical North Atlantic. ADT is plotted every 2.5 cm. Red and blue contours indicate the maximum geostrophic velocities of anticyclonic and cyclonic eddies, respectively.
Major remaining questions regarding ocean circulation include (1) the role of eddies in the transport of ocean heat, salinity, and biogeochemical properties, (2) the three-dimensional structure and temporal variability of the AMOC in the tropical Atlantic, and (3) the importance of equatorial waves and the deep ocean circulation for interannual-decadal variability of the AZM. In addition, climate change will alter the tropical Atlantic circulation and its interaction with the atmosphere. Increased upper-ocean stratification, changes in wind forcing, and a changing AMOC will affect the three-dimensional transport of heat, salt, and tracers as well as regional variations in sea level, regional distributions of water mass boundaries, and shifts in ecosystems. Coupled model simulations indicate a general warming in the tropical Atlantic, with reduced seasonal cycle and interannual variability, in response to an AMOC weakening (Chang et al., 2008). Continued monitoring is needed to assess climate change impacts and validate models.
Needs for ocean circulation include basin-scale observations to measure the AMOC and the near-surface ocean circulation. The measurements must span multiple decades in order to monitor long-term changes and must be capable of resolving velocity fluctuations from mesoscale eddies and other transient phenomena, especially near the western boundary and in the equatorial waveguide.
Predictability and Model Biases
Predicting tropical Atlantic climate is challenging, and the difficulty is exacerbated by persistent coupled climate model biases. These biases have received much attention but seen little improvement (Davey et al., 2002; Richter and Xie, 2008; Richter et al., 2014b; Giarolla et al., 2015; Zuidema et al., 2016b). The tropical Atlantic biases weaken the Atlantic’s global impact in models (McGregor et al., 2018). Predicting the AZM is particularly challenging, with dynamical forecasts often matched or even outperformed by persistence forecasts (Stockdale et al., 2006; Richter et al., 2017). It has been suggested that the AZM may be predictable with an anomaly correlation coefficient of 0.55 at 4 months lead (Ding et al., 2010). While most prediction models drop well below that by lead month 3 (Richter et al., 2017), some promising systems show skill in predicting the AZM in summer when initialized on May 1 (Prodhomme et al., 2016). To what extent model biases contribute to the poor skill in predicting the AZM is unclear, and very few studies have addressed this problem.
Variability of SST and other climatic variables in the northern tropical Atlantic is of great interest because most hurricanes form there. Hurricane-permitting global climate model simulations are becoming increasingly feasible (Patricola et al., 2014; Wehner et al., 2014; Walsh et al., 2015; Haarsma et al., 2016), but tend to overpredict hurricane activity if oceanic feedbacks are neglected (Zarzycki, 2016; Li and Sriver, 2018). Coupled atmosphere-ocean simulations are therefore needed for hurricane projections. However, the cool SST bias in the northern tropical Atlantic, common to coupled models (Richter, 2015; Zuidema et al., 2016b), can cause a 65% under-representation of Atlantic hurricane activity (Hsu et al., 2019). This suggests that the northern tropical Atlantic is a good target for coupled model improvement and possibly more ocean observations.
Models typically produce too weak of a cold tongue that also appears too late in the year, while placing cool SST in the western equatorial Atlantic warm pool, defined as the area with SSTs above 28.5°C. One contribution to the insufficient cold tongue development in models is the equatorial westerly wind bias in March–May (Figure 9), which deepens the thermocline and inhibits cooling during the subsequent June–August upwelling season (Richter and Xie, 2008; Richter et al., 2012). It may be linked to the erroneous southward shift in the Atlantic ITCZ, which is also present in atmosphere-only simulations (Figure 9B; Richter et al., 2014b), and the misrepresentation of convective momentum transport in the lower troposphere (Zermeño-Diaz and Zhang, 2013; Richter et al., 2014b). Tackling these problems will require detailed observations of the lower troposphere to gather observations that can guide error diagnosis and efforts toward improving convective parameterizations.
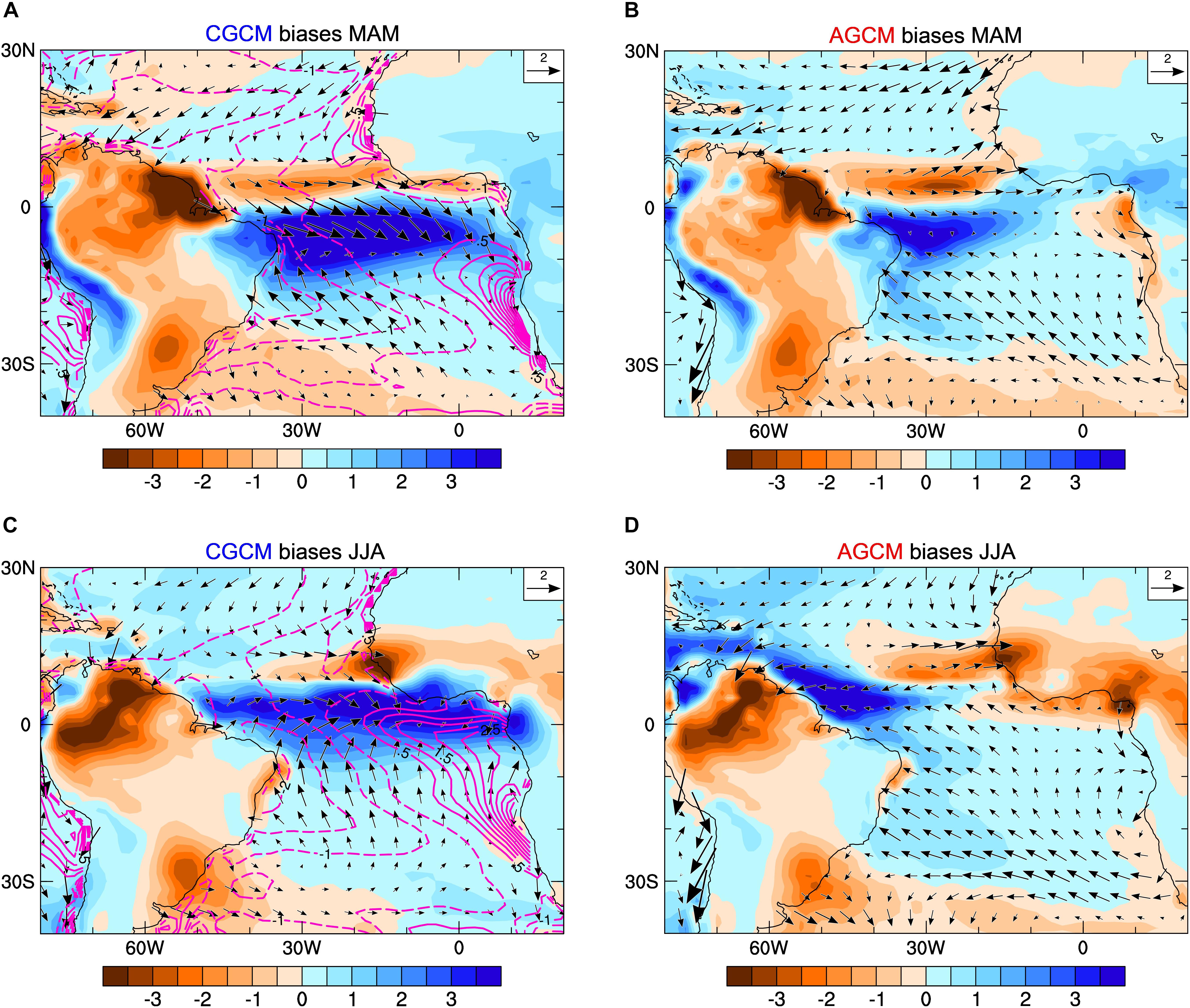
Figure 9. Biases of precipitation (shading; mm day−1), surface winds (vectors), and SST (contours; K) for an ensemble of CMIP5 models. Panels show the biases for (A) control experiment in March–April–May using coupled general circulation models (CGCM), (B) experiment AMIP [atmospheric general circulation models (AGCM) only] in MAM, (C) control experiment in June–July–August, and (D) experiment AMIP in JJA. The reference data sets are the Global Precipitation Climatology Project (Adler et al., 2003) for precipitation, the European Centre for Medium-Range Weather Forecasts Interim Reanalysis (Dee et al., 2011) for surface winds, and Reynolds optimally interpolated dataset (Reynolds et al., 2002) for SST.
Another factor that may contribute to the cold tongue bias is insufficient representation of the oceanic thermocline (Hazeleger and Haarsma, 2005; Xu et al., 2014b), which may be related to deficiencies in vertical mixing parameterizations. Another possibility is an overly weak AMOC, which generates a cold SST bias in the North Atlantic and warm bias in the Atlantic cold tongue (section “Ocean Circulation”; Wang et al., 2014). Poor representation of the AZM in many models appears related to the under-representation of the thermocline feedback associated with an overly deep mixed layer and too weak upwelling in the eastern Atlantic (Ding et al., 2015a,b; Deppenmeier et al., 2016; Dippe et al., 2018; Jouanno et al., 2017).
The warm SST bias in the Benguela coastal upwelling region (Figure 9) is more severe than that at the equator, exceeding 2.5°C in the multi-model average of atmosphere-ocean general circulation models (AOGCMs) from the Coupled Model Intercomparison Project Phase 5 (CMIP5; Toniazzo and Woolnough, 2014; Richter, 2015; Zuidema et al., 2016a). Upwelling is driven by southerly winds associated with the Benguela low-level coastal jet, with oceanic currents and the location of the Angola-Benguela frontal zone sensitive to the jet’s wind-stress curl (Colberg and Reason, 2006; Fennel et al., 2012). There are two main causes of the model errors in this region: (1) equatorial biases (Rouault et al., 2007), and (2) errors in local winds, especially the Benguela Jet (Xu et al., 2014a; Koseki et al., 2018; Voldoire et al., 2019) and the Benguela Current (Grodsky et al., 2012a; Muñoz et al., 2012). Some uncertainty remains in the actual near-coastal wind-stress curl, owing to the lack of reliable satellite data and atmospheric observations near land (Desbiolles et al., 2016). Many data products poorly represent the atmospheric and oceanic circulations in the region (Large and Yeager, 2008; Patricola and Chang, 2017; Tchipalanga et al., 2018), underscoring the need for updated products at higher resolution.
Relatively little is known about the vertical structure and seasonality of the poleward Angola current (Tchipalanga et al., 2018), though data from recently deployed buoys in the region are beginning to shed some light on this (Kopte et al., 2017). Likewise, the low-level atmospheric jet along the Angola-Namibia coast is relatively poorly observed. As a consequence, even the theory underpinning the existence of the Angola current is not completely settled (Junker et al., 2015). Based on reliable SST observations, we know that the Angola current and the associated ABF are placed too far south in GCMs by about 10° of latitude (Xu et al., 2014a; Koseki et al., 2018). In the absence of reliable theoretical and observational guidance, it is difficult to alleviate this problem.
The southeastern tropical Atlantic is home to one of the largest low-latitude semi-permanent stratocumulus decks on the globe. The large-scale meteorology that affects low clouds in this region is influenced by strong coupling between the Atlantic basin and neighboring continents (Adebiyi et al., 2015; Adebiyi and Zuidema, 2016). Both the northeastern and southeastern Atlantic low clouds are affected by large dust and smoke outflows off of continental Africa. Because of the large spatial extent of the shortwave-absorbing aerosols, they are capable of influencing the interhemispheric energy balance, the location of the ITCZ, and regional precipitation patterns (Jones et al., 2009; Randles and Ramaswamy, 2010; Sakaeda et al., 2011). Positive dust and cloud feedbacks on SST and climate have been established at interannual and decadal timescales in both hemispheres (Evan et al., 2011; Bellomo et al., 2015; Myers et al., 2018) and are capable of amplifying the AMV (Bellomo et al., 2016; Yuan et al., 2016). How the corresponding smaller-scale aerosol-cloud interactions are interwoven with the individual radiative aerosol and cloud feedbacks remains a topic of research (Zuidema et al., 2016b).
One common attribution of the warm SST biases in the Southeast Atlantic is an under-representation of the marine stratocumulus deck, which then allows too much sunlight to reach the ocean surface. This introduces a positive feedback: the warmer ocean surface reduces the lower tropospheric stability that maintains the low cloud deck (Klein and Hartmann, 1993; Huang et al., 2007; Hu et al., 2008), further reducing the low cloud cover. Even when the CMIP surface radiative forcing is correct, the cloud cover is still typically too low (de Szoeke et al., 2012), likely due to an underestimation of optically thin stratiform clouds (Nam et al., 2012; Zuidema et al., 2012; Delgadillo et al., 2018; Wood et al., 2018). However, two findings complicate this interpretation. One is that the turbulent surface fluxes often overcompensate for the enhanced shortwave warming (de Szoeke et al., 2010). The other is that even for AMIP simulations in which the SST is specified, the cloud radiative effect on the surface is still underestimated, with bias reductions of only ∼25% (Zuidema et al., 2016a). This suggests the atmospheric component of the coupled models is the primary culprit (Lauer and Hamilton, 2013), with the ocean playing a vital but secondary role (Richter, 2015). Several studies have reported improved Atlantic equatorial SST, precipitation, and wind climatologies after improving their cloud radiative biases (Hu et al., 2008; Wahl et al., 2011), and efforts to improve cloud representation in models are ongoing (Bodas-Salcedo et al., 2011; Qu et al., 2014; Dal Gesso et al., 2015; Neggers, 2015; Webb et al., 2017). The cause of parameterization biases is often model specific (Medeiros et al., 2012), and ongoing observations of the boundary layer thermodynamic structure and its cloud, precipitation, and aerosol vertical structure, including the diurnal cycle, are needed for continued model improvement. Such observational datasets remain sparse for the Southeast Atlantic.
An observational network aimed at understanding and reducing model biases should include comprehensive measurements of the lower troposphere as well as surface heat and freshwater fluxes, wind stress, and ocean currents, and mixing in the equatorial and southeastern tropical Atlantic. Targeted measurements of clouds and lower tropospheric winds, temperature, and humidity in the Atlantic ITCZ region would also be beneficial.
Data Assimilation
The tropical Atlantic observing system is not designed to resolve all important spatial and temporal scales of variability. However, observations are used in data assimilative models that merge observations with ocean general circulation models forced by numerical weather prediction (NWP) fields or atmospheric reanalyses. These ocean state estimates reconstruct a dynamically consistent past evolution of the ocean, adding significant value to the existing collection of measurements. Through their inclusion in assimilative models, observations are also a valuable component of medium- to long-range prediction systems. It is therefore important that the design of the observing system takes into account the strengths, weaknesses, and future directions of analysis, reanalysis, and forecast systems.
Data from the tropical Atlantic observing system are not only used in oceanic data assimilation, but also in atmospheric analyses. From a NWP perspective, Poli (2018) analyzed the impact of observations on the ECMWF operational data assimilation scheme and showed that surface pressure measurements from PIRATA buoys have a higher positive impact per datum than many other types of observations (satellite, upper-air, land surface, or sea surface other than buoys). For instance, the impact of surface pressure is one order of magnitude larger than the impact of wind measured on the same buoys. However, satellite scatterometer, altimeter, and synthetic aperture radar data are providing valuable assimilation constraints for wind and sea-state forecasts by NWP systems (Isaksen and Janssen, 2004; Dragani et al., 2015).
Most tropical Atlantic Ocean short term prediction systems are global, eddy-permitting, and assimilate available remote sensing and in situ data transmitted in real-time. These systems are based on multivariate three-dimensional variational (3DVAR) and 4DVAR and ensemble approaches. They currently assimilate satellite altimetry, radiometry, and imagery to correct the surface model’s sea level, temperature, and chlorophyll content. There have been some successful attempts to assimilate microwave data from the Soil Moisture and Ocean Salinity (SMOS), Aquarius, and Soil Moisture Active Passive (SMAP) satellites to correct SSS (Martin, 2016).
With the advent of coupled analysis and reanalysis systems, it is likely that the optimization of the tropical Atlantic observing system will become increasingly important. From an uncoupled ocean perspective, there is also a strong impact from water mass observations over the full water column, which have been shown to improve reanalysis fields locally by several units in temperature and salinity (Oke et al., 2015; Turpin et al., 2016; Gasparin et al., 2018). The vertical structure of currents and water mass properties at depth, especially near the thermocline, is drastically improved with the assimilation of in situ ocean data. For instance, Busalacchi (1996) showed that the direct assimilation of sea level and thermocline depth observations improves the upper-ocean structure in the equatorial Atlantic. The assimilation of PIRATA data was also found to improve significantly the intra-seasonal variability of upper-ocean temperature (Belyaev et al., 2001), while the impact on seasonal to longer-range prediction systems needs to be investigated and quantified in more detail. In order to guide observing system agencies and improve the use of observations in ocean models, multisystem approaches, mostly based on observing system experiments and reanalysis intercomparisons, are increasingly being used. Sophisticated (e.g., variational) quality control procedures have also shown promise for handling different observing networks in the tropical Atlantic, where, for example, large freshwater variability due to river outflow and the presence of the ITCZ may make conventional quality control methods ineffective (Storto, 2016).
At NOAA/NCEP, monthly ocean state monitoring is performed as part of a Real-Time Multiple Ocean Reanalysis Intercomparison Project (RT-ORA-IP). The intercomparison, based on nine operational systems, was motivated by the need to study the influences of the tropical Pacific observing system on uncertainties in the tropical Pacific Ocean state estimation (Xue et al., 2017). This project has been expanded to cover the tropical Atlantic and shows that overall, in the equatorial Atlantic the agreement between reanalyses is often reasonable. However, locally the spread between estimates can reach 2°C in the thermocline7.
Sustaining and enhancing measurements is important to reduce such discrepancies among the reanalysis products. For example, the RT-ORA-IP exercise illustrates that in spite of a reasonably well captured thermocline structure along the equatorial band, heat content anomalies close to the mouth of the Amazon show strong disagreement due to large differences in river discharge applied in the different reanalyses and the associated resultant differences in local ocean circulation. Ocean prediction and reanalysis systems will greatly benefit from a sustained observing network in this area and, similarly, in the Gulf of Guinea. Most data assimilation systems also suffer from overly simplistic balance (cross-covariance) formulations close to the equator that may jeopardize the assimilation of local observations. These difficulties often result from extensions of geostrophic balance using empirical approximations (e.g., Weaver et al., 2005). Thus, data assimilation systems must evolve and account for the complex, time-varying balance in the tropics.
The skills of ecosystem models depend on physical forcing (e.g., temperature and currents) and biogeochemical variables (e.g., primary production and dissolved oxygen concentration), as well as bottom parameters for models that include coastal and shelf slopes. Whereas in ocean models’ operational systems data are assimilated to correct biases, biological and fisheries data are often assimilated into models to optimize their parameters. Fish stock assessment models have developed quantitative methods to optimize the population dynamics parameters of exploited species based mainly on catch and fishing effort data, and in some cases tagging data (Maunder and Punt, 2013). Conversely, ecosystem models have rarely included such quantitative approaches. The Spatial Ecosystem and Population Dynamics Model (SEAPODYM) is an example of a modeling framework for the dynamics of phytoplankton, zooplankton, and micronekton (small but actively swimming organisms) and the detailed dynamics of key exploited fish populations (e.g., tunas, swordfish, mackerel) and their fisheries. Parameters of each model component (zooplankton, micronekton, exploited species and fisheries) are estimated using observations and data assimilation methods. A recent major development is the use of acoustic observations at multiple frequencies rather than a single one (usually 38 kHz) to reconstruct a proxy of micronekton biomass, since various species or groups of species can be distinguished by their specific responses in frequency space (Verma et al., 2017; Proud et al., 2018).
In addition to reanalysis intercomparison, Observing System Simulation Experiments (OSSEs) have been carried out recently within the Horizon 2020 AtlantOS project (2014–2019, Visbeck et al., 2015), based on a multi-system approach including both satellite and in situ observations. Temperature and salinity errors were reduced by 5–10% in the upper 2000 m with an enhancement of Argo sampling in equatorial regions and by around 20–30% in the deep ocean due to the implementation of deep Argo (Gasparin et al., 2019). The present tropical mooring array provides invaluable time series for evaluation of models and assimilation systems, the latter being primarily impacted in the region of the moorings. The high temporal sampling rates of moorings are not exploited in most current assimilation systems, and the mooring impact could potentially be larger with better adapted and more advanced assimilation systems. While data assimilation techniques and OSSEs are less mature for biogeochemical observations, dedicated experiments suggest that assimilation of biogeochemical Argo data will complement satellite surface color data by improving model estimates of oxygen, nutrients, carbon, and chlorophyll throughout the water column. Such dedicated activities require large time commitments and dedicated infrastructure, including running research and development versions of operational ocean analysis and forecasting systems. They also need careful planning and guidance to focus on observing system contributions at specific spatial and temporal scales and desired process-oriented metrics.
The observing system requirements for data assimilation are broad, and work is underway to quantify the specific needs. Measurements of temperature and salinity in the deep ocean and surface atmospheric pressure appear to be top priorities for improving operational data assimilation and ocean state estimates. Measurements in the northwestern and southeastern tropical Atlantic are very important. Uncertainties in ocean reanalyses are largest in these regions due to sparse observations, large model errors and uncertainties in river run-off as well as air-sea heat, moisture, and momentum fluxes. Direct measurements of biogeochemical and biological parameters are needed throughout the tropical Atlantic to improve their representations in ocean and coupled models. Future coordination between modeling and data assimilation experts and observational experts is essential for proper design and interpretation of OSSEs, especially in order to extract compelling messages on the ability of the ocean observing system to resolve certain processes.
Biogeochemistry, Ecosystems, and Pollution
Interannual variability of air-sea CO2 fluxes is closely linked to climate variability (Lefèvre et al., 2013; Ibánhez et al., 2017). Thus, improving understanding and monitoring of tropical Atlantic climate will improve knowledge and predictability of CO2 fluxes. Carbon trends remain unclear because of the relatively short time records and high variability of the tropical Atlantic, but observations in the western tropical North Atlantic show a slower increase of seawater pCO2 than the atmospheric growth rate from 2002 to 2009 (Park and Wanninkhof, 2012) followed by a large increase almost twice the atmospheric growth rate from 2010 to 2018 (Wanninkhof et al., in preparation), suggesting strong decadal variability.
Based on oxygen observations from repeat ship sections in the OMZ of the eastern tropical North Atlantic for the recent decade (2006–2015), Hahn et al. (2017) suggested the existence of strong oxygen variations over this decade that are superimposed on the multi-decadal deoxygenation pattern (Stramma et al., 2009; Brandt et al., 2015; Santos et al., 2016). Ocean warming and the related solubility effect are responsible for about half of the multi-decadal oxygen decline in the upper 1200 m (Schmidtko et al., 2017). Mechanisms that are responsible for the other half are unclear and are likely related to changes in ventilation and circulation (Brandt et al., 2015; Oschlies et al., 2018), though changes in biological activity cannot be ruled out. Oxygen changes in the tropical North Atlantic during the past decade are most likely associated with changes in the eddy-driven zonal current bands at intermediate depths and a shoaling of the wind-driven thermocline circulation, but other processes, such as variations in the intensity of the mesoscale eddy field, may contribute (Hahn et al., 2017).
Other main scientific questions are related to the roles of natural and anthropogenic processes that affect physical and biogeochemical changes on different timescales and their impact on marine ecosystems and biodiversity. In the context of climate change, ocean acidification is a key concern (Feely et al., 2004; de Carvalho-Borges et al., 2018). In many regions of the tropical Atlantic, the rate at which pH is declining, its causes, and its impacts on CaCO3 shell-forming species are not well known. One of the longest moored pCO2 time series in the tropical Atlantic is located at 6°S, 10°W and began in 2006. Acidification has been examined at this site, though the record is not long enough to detect any increase in CO2 (or decrease of pH) given the strong natural variability of CO2 (Lefevre et al., 2016). Documentation of the fate of organic matter (OM) in OMZs is also of paramount importance. Organic matter produced in the well-lit layer could be either preserved and exported, or degraded and available for marine organisms. OMZs are expected to strengthen the biological carbon pump, as surface-produced OM is better preserved due to oxygen deficiency. This assumption does not account for the intense microbial activity, which may foster OM degradation or remineralisation (Bretagnon et al., 2018). An estimation of the particle flux attenuation from satellite observations has been developed (Bretagnon, 2018) and may lead to improved predictions of oxygen inventory trends. It would also help to study the impact of oxygen variability on the remineralisation efficiency.
In the eastern tropical Atlantic, estimates of the biological pump and its dependence on oxygen availability are complicated by the presence of mesoscale eddies, which contain shallow (40–100 m) suboxic environments (Karstensen et al., 2015). In these structures the vertical distributions of particulate and dissolved OM show higher concentrations in the surface mixed layer (0–70 m). Inside the eddies’ cores, oxygen consumption can be an order of magnitude higher than the average values for the North Atlantic, and the downward flux of organic matter exceeds typical values found in the open ocean (Fiedler et al., 2016). A current lack of monitoring of biological variables and their controls makes it challenging to determine how the ecosystems will cope with the changing conditions.
In models, interannual variations of temperature, nitrate, and oxygen concentrations along the southwestern coast of Africa are primarily controlled by oceanic teleconnections associated with equatorial wave variability and along-shore water mass transport (Mohrholz et al., 2008; Bachèlery, 2016; Bachèlery et al., 2016a,b; Koungue et al., 2017). Equatorially forced waves propagate along the southwestern African coast, triggering substantial thermocline, halocline, and nutricline displacements and affecting the local marine ecosystems balance, while tropical nutrient-rich and low-oxygen water masses may occasionally penetrate southward over large distances along the coast. Observations are needed to verify model results and the sensitivity of biogeochemistry and ecosystems to local and remote forcing.
For fisheries, the resource, commercially important fish stocks, needs to be assessed in terms of current stock status, including age structure and recruitment (i.e., the production of offspring). Thus, there are clear direct monitoring needs: reporting catches, including determination of age, length and weight of samples of each commercial species and the respective effort to harvest them. In addition, many countries perform fisheries independent activities such as trawl surveys, hydroacoustic surveys, and egg and larvae surveys, for independent measures of the state of a given fish stock. However, to understand the mid- to long-term development of stocks, information on the ecosystem besides the development of prey and predators is needed. This includes phytoplankton, zooplankton, micronekton, fish, shellfish, benthic organisms (corals, sponges, etc.) and megafauna (seabirds and marine mammals) on the biological side, and changes in the marine environment either through climate variability or global warming induced long-term trends (see discussion of ecosystem models and assimilation in Section “Data Assimilation”). Pollutants such as mercury and plastics can negatively impact ecosystems, and emerging contaminants such as gadolinium, silver, and platinum need close attention, as their fate, toxicity and distributions are not well known (Hatje et al., 2018; Henderson et al., 2018). Monitoring of trace-metal cycles, and novel approaches to assess their interaction with ecosystems, will be required as the ocean responds to anthropogenic stressors. A list of human activities that exert pressures on the ocean environment and have negative impacts on ecosystems can be found in Table 1.
The observing needs for biogeochemistry, ecosystems, and pollution include the development of long time series of key variables such as oxygen, CO2, chlorophyll, nutrients, and commercial and endangered species, for monitoring and understanding seasonal, interannual, decadal, and longer timescale changes. These measurements are especially important in OMZs, upwelling and other near-coastal regions, and at key sites to monitor ocean acidification. Harmful pollutants such as plastics must be monitored, and internationally integrated fish stock and endangered species surveys are also a high priority.
The Existing Observing System
This section summarizes the existing tropical Atlantic observing system and explores the extent to which it meets the needs of the science drivers put forth in Section “Science Drivers”.
In situ Observations
Moored Buoys
The Prediction and Research Moored Array in the Tropical Atlantic (PIRATA) serves as the backbone of the in situ observing system (Figure 2; Bourlès et al., 2008, 2019). It was initiated in 1997 and now consists of 18 moorings spanning the basin. All moorings measure ocean temperature in the upper 500 m, salinity in the upper 120 m, and near-surface air temperature, relative humidity, wind velocity, rainfall, and incident shortwave radiation. The vertical spacing of temperature sensors varies between moorings and is typically 5–20 m in the upper 40 m, with 20 m intervals down to 140 m and 40–200 m resolution between 140 and 500 m. Conductivity is additionally measured with 5–20 m spacing in the upper 40 m and 20–80 m spacing down to 120 m. Several moorings also measure downward longwave radiation, atmospheric pressure, and ocean currents at a depth of 10 m or 12 m. Daily averaged data are transmitted in real-time. A transition to the next-generation tropical flex moorings (T-Flex) began in 2015. Currently, 10 PIRATA buoys have been upgraded and the remaining eight will follow (Figure 2). The main advantage of T-Flex is that much larger amounts of data can be transmitted. At present, all T-Flex moorings send hourly averages in real-time.
At the equator, several subsurface acoustic Doppler current profiler (ADCP) moorings are maintained as part of PIRATA (Bourlès et al., 2019). They measure currents typically from depths of about 30 to 300 m. Several German and French projects have deployed additional deep and intermediate-depth current meters to the ADCP moorings (Brandt et al., 2006; Bunge et al., 2008). Other projects have also taken advantage of the PIRATA moorings as platforms of opportunity. Beginning in 2014, all buoys have been equipped with acoustic receivers at a depth of 200 m as a contribution to the Ocean Tracking Network (OTN8). The 10°W and 23°W equatorial moorings have been equipped with turbulence sensors (χpods) as part of an Oregon State University (OSU) Ocean Mixing Group program that will run for 5 years. In 2017, 10 additional point acoustic current meters were implemented at 4°N, 23°W between 7 and 87 m depths for the NOAA/AOML Tropical Atlantic Current Observations Study (TACOS) experiment, and a subset of those sensors was redeployed in 2018.
PIRATA has also supported biogeochemical measurements. Since 2006 at 6°S, 10°W and since 2008 at 8°N, 38°W, CO2 Carbon Interface Ocean Atmosphere (CARIOCA) sensors have been measuring the fugacity of CO2 (fCO2) at a depth of about 1 m. In 2017, a new CARIOCA sensor was implemented at 6°S, 8°E. Since 2008, the moorings at 4°N, 23°W and 12°N, 23°W have measured dissolved oxygen (O2) at depths of 300 and 500 m in order to monitor the OMZ. O2 sensors were recently added at 20.5°N, 23°W (Bourlès et al., 2019).
Other moorings in the tropical Atlantic include the Northwest Tropical Atlantic Station for air-sea flux measurements (NTAS) at 15°N, 51°W, the Melax air-sea buoy in the Senegalese part of the Canary Current Upwelling System at 14°N, 17°W, the Cape Verde Ocean Observatory (CVOO) at 17.6°N 24.3°W, and several meteorological and wave buoys maintained by the National Data Buoy Center (NDBC). NTAS has been operational since 2001 and measures the same parameters as the PIRATA buoys (surface meteorology including longwave radiation and atmospheric pressure), plus ocean currents from a point meter at 10 m and an upward-looking ADCP at 100 m, and enhanced vertical resolutions of temperature and salinity. The Melax buoy is on the continental shelf and measures the same parameters and oxygen at the seafloor. CVOO is part of the Cape Verde Observatory, which consists of operational atmospheric and oceanic monitoring sites for climate-relevant environmental parameters in the tropical eastern North Atlantic Ocean. Most NDBC moorings measure wind velocity, atmospheric pressure, and SST. Some buoys additionally measure significant wave height and direction and relative humidity. Data from NTAS and the NDBC moorings are transmitted in real-time, whereas only atmospheric data, SST, and surface salinity are relayed in real-time from Melax.
Argo
Argo is a global array of autonomous floats that sample the upper 2000 m of the ocean (Jayne et al., 2017). The floats drift at a depth of 1000 m and make profiles of temperature, salinity, and pressure in the upper 2000 m typically every 10 days. Measurements began in the early 2000’s and there are currently approximately 4000 floats in the global ocean. About 800 floats must be deployed each year to maintain the array.
Argo is expanding its measurement capabilities to include full-depth (4000–6000 m) profiles (Zilberman and Roemmich, 2017). The deep Argo array currently consists of 69 floats, 9 of which are in the tropical Atlantic (Figure 2B). The project’s goal is to deploy 1228 deep Argo floats globally, each with the ability to measure temperature, salinity, and pressure to within ±0.001°C, ±0.002 psu, and ± 3 dbar, respectively, improving on the standard float accuracies of temperature and salinity. Increased accuracy is required in order to resolve very small variations that can exert a large influence on global and regional mass, heat, and freshwater budgets.
The biogeochemical (BGC) Argo program was developed to improve scientific understanding and monitoring of the ocean’s carbon uptake, oxygen variability, nitrate cycle, ocean acidification, the biological carbon pump, and phytoplankton communities (Gruber et al., 2010). Profiles of biogeochemical (BGC) parameters are currently made by 329 Argo floats in the global ocean, of which 22 are in the tropical Atlantic (Figure 2B). The measured parameters include oxygen, chlorophyll-a, suspended particles, nitrate, pH, and downwelling irradiance. Suspended particles include phytoplankton, their microscopic predators, as well as bacteria that decompose organic material. Downwelling irradiance in the ocean enables estimates of the concentrations of chlorophyll and dissolved organic matter and the amount of light available for primary production.
Repeat Hydrographic Surveys and Ships of Opportunity
The Global Ocean Ship-based Hydrographic Investigations Program (GO-SHIP) conducts high-quality, full-depth measurements in the global ocean approximately once per decade (Figure 10). The measurements have high spatial and vertical resolutions and measure many different physical, chemical, and biological variables, including heat, freshwater, carbon, oxygen, nutrients and transient tracers. These measurements are used to document ocean changes throughout the water column and are especially important for monitoring the deep ocean below 2 km, which is not sampled globally by profiling floats. Different national and international programs contribute to hydrographic and velocity measurements along repeat sections near the western (5°S, 11°S) and eastern (18°N, 11°S, 23°S) boundaries (Figure 10).
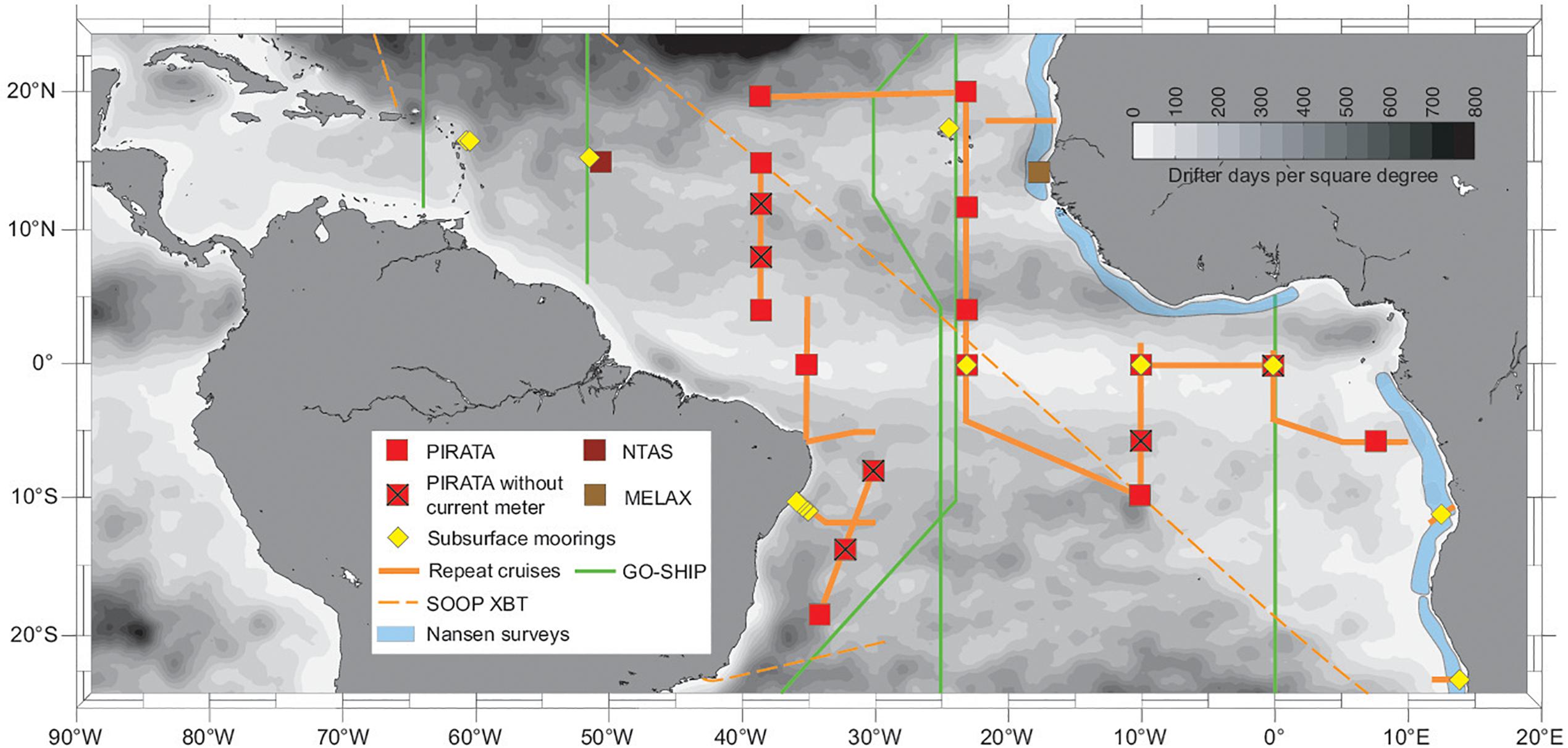
Figure 10. The tropical Atlantic observing system as implemented for the study of ocean circulation and upwelling. Shading indicates total number of days any surface drifter was in each 1° × 1° box through February 2018. Surface drifter measurements in the tropical Atlantic began in 1997. Solid orange lines show repeat cruise tracks, mainly for servicing moorings, dashed orange lines show XBT lines, blue shading indicate regions where Nansen surveys (near-coastal physical, chemical, and biological sampling from a research vessel) have been conducted, and green lines show full-depth repeat hydrography cruise tracks (GO-SHIP). The brown square shows the location of the coastal air-sea buoy Melax, yellow diamonds indicate where subsurface velocity and hydrographic moorings have been deployed, and other symbols are as in Figure 6.
The ship-of-opportunity program (SOOP) acquires measurements from volunteer merchant ships that regularly traverse certain shipping routes. Currently, there are three transects in the tropical Atlantic, each repeated approximately every 3 months (Figure 10). XBTs are deployed 10 to 35 km apart to measure temperature in the upper 760 m, from which mesoscale eddy variability and the larger-scale ocean circulation can be deduced (Goes et al., 2013). Thermosalinographs (TSGs), instruments mounted close to the water intake of ships to continuously measure SSS and SST, record measurements along two SOOP transects. Surface ocean CO2 fugacity (fCO2), temperature, and salinity are also measured on some voluntary observing ships. The number of measurements of fCO2 increased from about 0.3 million per year during 1995–2000 to 1.1 million per year during 2005–2012 (Bakker et al., 2016).
Annual PIRATA servicing cruises provide opportunities for repeated shipboard measurements. These consist primarily of conductivity-temperature-depth (CTD) casts, often with measurements of O2 and currents from lowered ADCPs (Figure 10). PIRATA cruises also allow for deployments of Argo floats, surface drifters, radiosondes, and ozonesondes, and for water sample analysis to determine concentrations of O2, CO2, and chlorophyll. In 2017 and 2018, full-depth CTD-O2-ADCP casts, along with water sample analyses of salinity, O2, pH, nutrients, and trace elements, were performed during the western Atlantic PIRATA servicing cruises. Flux tower measurements of momentum, humidity, and CO2 at the ocean-atmosphere interface were also made at times. Multi-frequency transects collected during the regular maintenance cruises of the PIRATA network since 2015 are a key piece of a growing acoustic network for estimating the global biomass of micronekton, one of the less known components of the ocean ecosystem.
Surface Drifting Buoys
Global Drifter Program (GDP) observations from buoys drogued at 15 m were first collected in the tropical Atlantic in 1990, and the array was sustained beginning in 1997 (Figure 2). Since 1998, the array in the tropical Atlantic has averaged 92 drifters, and since 2005 drifter measurements have been approximately hourly. Most deployments are conducted from the expendable bathythermograph (XBT) line AX8, which runs from Cape Town to the U.S. east coast, from research vessels servicing PIRATA, and from Brazilian Navy vessels. Sustaining drifter observations in the tropical Atlantic is difficult as it is a region of net surface divergence.
In addition to the standard drifter measurements of SST, surface currents, and barometric pressure, drifters have also been developed to measure subsurface temperature, surface and subsurface salinity, wind velocity, and directional wave spectra. However, few of these observations are being collected by drifters in the tropical Atlantic. Surface velocity estimates can also be calculated from Argo float trajectories while they are transmitting their data at the surface (Lebedev et al., 2007). As Argo transitions to Iridium data transmission, the floats will spend less time at the surface and thus be more strongly affected by high-frequency motion than was the case with floats using Service Argos. It is unclear how useful such measurements will be.
Boundary Current Arrays
An array consisting of four velocity moorings has been installed at the Brazilian continental slope along 11°S from 2000 to 2004 and since 2013, measuring the shallow and DWBC (Schott et al., 2005; Hummels et al., 2015). A single mooring was also deployed to observe the Angola Current. The mooring consists of an ADCP covering the upper 500 m at 11°S, 13°E and has been recording data since 2013, showing a weak and highly variable southward flow of the Angola Current (Kopte et al., 2018). In addition to the boundary current arrays at 11°S, pressure-equipped inverted echo sounders have been deployed at 300 and 500 m on the continental margins on each side of the basin since 2013 to enable comprehensive AMOC estimates at this latitude. Since 2000, a mooring array has been maintained at 16°N that includes velocity measurements covering the DWBC east of Guadeloupe and additional geostrophic moorings measuring the deep flow between the continental slope and the mid-Atlantic ridge (Send et al., 2011; Frajka-Williams et al., 2018). The 16°N and 11°S arrays contribute to the AMOC observing system and can be used to investigate links between the subtropical North Atlantic array at 26.5°N (RAPID/MOCHA/WBTS) and the South Atlantic array at 34.5°S (SAMBA). Figure 10 shows the locations of the boundary current arrays.
Satellite Observations
Most satellites provide measurements in real-time or near real-time, and the records for many parameters extend back at least 20 years. SST is obtained from passive infrared and microwave radiometers. By combining data from several satellites with in situ measurements, daily maps of SST at high spatial resolution (0.25° or better) are possible going back to 1997, when microwave measurements started. Estimates of tropical rainfall and precipitable water are also available from microwave sensors. Satellite-based measurements of SSS began in 2009 with the launch of the SMOS sensor. SSS was measured by Aquarius during 2011–2015 and is currently measured by the SMAP radiometer. From these satellites, complete coverage of the tropical Atlantic is achieved in approximately one week at a spatial resolution of 1/2°–1°. Real-time blended SSS products show promise for monitoring conditions in the tropical Atlantic (Xie et al., 2014). Measurements of surface chlorophyll-a concentration, clouds, atmospheric temperature and moisture profiles, and aerosol optical depth are made routinely using infrared sensors such as the Moderate Resolution Imaging Spectroradiometer (MODIS). Complete coverage of the tropical Atlantic is achieved in approximately 2–8 days. The geostationary MeteoSat satellite provides full disk coverage at half-hourly resolution, though retrieval products are more limited. Several satellites are equipped with radar altimeters that measure sea surface height, from which the sea level anomaly with respect to the geoid can be calculated. Full coverage of the tropical Atlantic is achieved in less than 3 days.
Satellite observations have greatly improved the coverage, resolution, and accuracy of surface buoyancy and momentum flux estimates (Yu, 2018). Turbulent heat, moisture, and momentum fluxes are computed from bulk flux algorithms (Fairall et al., 2003) using surface wind speed and direction, SST, near-surface air temperature, and humidity as input. Wind speed and direction have been provided by scatterometers on a series of satellite missions. These include the European Remote sensing Satellite (ERS)-1 (1992–1996) and ERS-2 (1995–2000), the NASA SeaWinds-1 scatterometer on the QuikSCAT satellite (1999–2009), the European Space Agency’s (ESA’s) series of three Advanced Scatterometers (ASCAT) onboard the MetOp satellites (2006 onward), and OceanSat-2 (OSCAT; 2009–2014) and SCATSAT-1 (2016 onward) by the Indian Space Research Organization. Scatterometers measure the effects of centimeter-scale roughness caused by surface stress, but the present retrieval algorithms generate estimates of surface wind, not wind stress, because there are no suitable surface wind stress ground-truths for calibration. Instead, wind retrievals are calibrated to the equivalent neutral-stability wind 10 m above the local-mean sea surface (Liu and Tang, 1996). The 10-m equivalent neutral wind speed differs from the 10-m wind speed measured by anemometers. These differences are a function of atmospheric stratification and are normally on the order of 0.2 m s−1. The differences may also reflect differences in observing platforms. For example, in situ wind measurements are relative to a fixed-earth reference, while satellite winds are relative to surface currents. For winds less than about 3 m s−1 or greater than 20 m s−1, the uncertainties are generally larger. Low-wind retrievals are often problematic because the weak backscatter signal is confounded by noise, and the empirical scatterometer algorithms are not sufficiently calibrated at high winds due to the lack of in situ measurements.
The remote sensing of SST uses space-borne infrared and microwave radiometers to detect thermally emitted radiation from the ocean surface. Infrared radiometers such as the five-channel Advanced Very High Resolution Radiometer (AVHRR) use wavelength bands that have high transmissivity in the cloud-free atmosphere. However, clouds are opaque to infrared radiation and can effectively mask the radiation emitted from the ocean’s surface. Because of the cloud effect, it takes one or two weeks to obtain a complete global SST field from AVHRR even though the satellite orbits the Earth 14 times each day and has a 2399-km-wide swath. In contrast, clouds have little effect on microwave radiometers so that microwave SST retrievals can be made under all rain-free weather conditions. The TRMM microwave imager (TMI) was launched in 1997 and was the first satellite sensor capable of accurately measuring SST through clouds. The low-inclination equatorial orbit, however, limits TMI’s coverage to the 38°S–38°N latitude band. Global through-cloud measurements of SST were made possible by the Advanced Microwave Scanning Radiometer (AMSR) onboard NASA’s EOS Aqua spacecraft (AMSR-E, 2002–2011) and the AMSR-2 onboard the Japan Aerospace Exploration Agency’s (JAXA’s) Global Change Observation Mission – Water (GCOM-W1) spacecraft (2012 onward).
Near-surface air humidity and temperature cannot be retrieved directly by satellites. Instead, these variables are estimated from satellite-measured total column water vapor or total precipitable water (PW) (Liu, 1986; Liu et al., 1991) using passive microwave radiometers such as Special Sensor Microwave Imager and Sounder (SSM/I and SSMIS, respectively), AMSR-E, and AMSR-2. The launch of the Advanced Microwave Sounding Unit (AMSU) on the NOAA series of polar orbiting meteorological satellites in May 1998 provided profiles of temperature and humidity that have been used to improve estimates of near-surface humidity and temperature (Jackson et al., 2006). Despite significant progress, these variables remain the leading source of error in satellite-based surface heat flux products.
Satellites measure downwelling and upwelling solar radiation and upwelling longwave radiation at the top of the atmosphere (TOA). The radiation budget at the ocean’s surface is not remotely sensed. Instead it is estimated from radiative transfer calculations that use satellite-derived TOA irradiance, cloud and aerosol properties, and the atmospheric state from either satellites or reanalysis. Surface radiation budget estimates are produced by the Clouds and the Earth’s Radiant Energy System (CERES) Energy Balanced and Filled (EBAF) radiative transfer model (Kato et al., 2013). The CERES experiment was developed to measure TOA radiative fluxes and to determine radiative fluxes within the atmosphere and at the surface. CERES instruments were launched aboard the Tropical Rainfall Measuring Mission (TRMM) in November 1997, on the EOS Terra satellite in December 1999, and on the EOS Aqua spacecraft in 2002.
Precipitation at the ocean’s surface is retrieved from variables that are highly correlated with rainfall, including infrared and microwave brightness temperature, as well as visible and near-infrared albedo. Infrared techniques are based on the premise that surface rainfall is related to cloud-top properties observed from space, while microwave techniques relate rainfall to microwave emission from rain drops and scattering from ice. Microwave observations are available from SSM/I, AMSU-B, and the TRMM spacecrafts. TRMM is equipped with the first spaceborne precipitation radar (PR) along with a microwave radiometer (TMI) and a visible/infrared radiometer (VIRS), thus allowing the estimation of rain profiles in addition to surface precipitation. Information from all three sensors is optimally merged to produce a three-hour precipitation field at 0.25° spatial resolution over the tropics and subtropics. The Global Precipitation Measurement (GPM) mission launched in February 2014 and carries a dual-frequency precipitation radar and microwave imager, which is able to sense total precipitation within all cloud layers. GPM extends the capabilities of TRMM sensors, including sensing light rain, and for the first time, is able to quantify microphysical properties of precipitation particles.
Strengths and Weaknesses of the Current Observing System
There is a high degree of integration among the various sustained observing components so that weaknesses of some are compensated by the strengths of others. Satellites give global coverage but only at the surface of the ocean and generally do not resolve timescales less than one day. In contrast, Argo provides subsurface information, but at coarser horizontal resolution than satellites. Moored surface buoys have even coarser horizontal and vertical resolutions than Argo, but uniquely make co-located high-temporal-resolution measurements of the upper ocean and near-surface atmosphere. In situ data are critical for validating and calibrating satellite retrievals, and satellite data are useful for filling temporal gaps in mooring surface data and for providing information to fill the large spatial gaps between moorings and other in situ surface measurements. There is therefore a high degree of complementarity between satellite and in situ measurements. To measure ocean circulation, surface drifters have basin-wide coverage, complementing sparse moored measurements from single-point and profiling current meters. Measurements from XBTs are able to resolve the vertical structures of eddies and give estimates of large-scale ocean transport as quasi-synoptic snapshots, while transport arrays provide estimates of AMOC components including the deep ocean or full-depth mass, heat, and salinity transports at key locations. The existing integration can be seen as a strength of the present observing system. The remainder of this section focuses on gaps in the observing system in the context of the different science drivers presented in Section “Science Drivers”.
In the tropical North Atlantic, PIRATA moorings are located in the central and eastern basin (east of 40°W), where seasonal and interannual SST signals are largest, especially those associated with the AMM and AZM. However, PIRATA moorings do not sample the warm pool region to the west, where there are strong mesoscale eddies (Figure 8), seasonal variations of upper-ocean salinity stratification that affect SST and TCs, and strong multidecadal variations of upper-ocean heat content and SST (Figure 11). Moorings also do not resolve well the equatorial waveguide, especially meridionally, or the southeastern tropical Atlantic, where there are large SST signals associated with Benguela Niños, model biases, and complex ocean-atmosphere interactions involving winds, currents, SST, clouds, and aerosols. In these regions, enhanced mooring coverage may be part of the solution, but other measurements will also be needed. An effective multiplatform approach might include (1) denser sampling from Argo floats where more information on the large-scale subsurface ocean is most needed, such as the equatorial and northwestern tropical Atlantic, (2) additional moorings at key locations where upper-ocean processes and air-sea fluxes are poorly understood, such as downstream from the Amazon-Orinoco low-salinity plume and in the southeastern Atlantic stratus deck region, and (3) ocean gliders and satellite measurements of surface currents that can most effectively sample fronts and eddies.
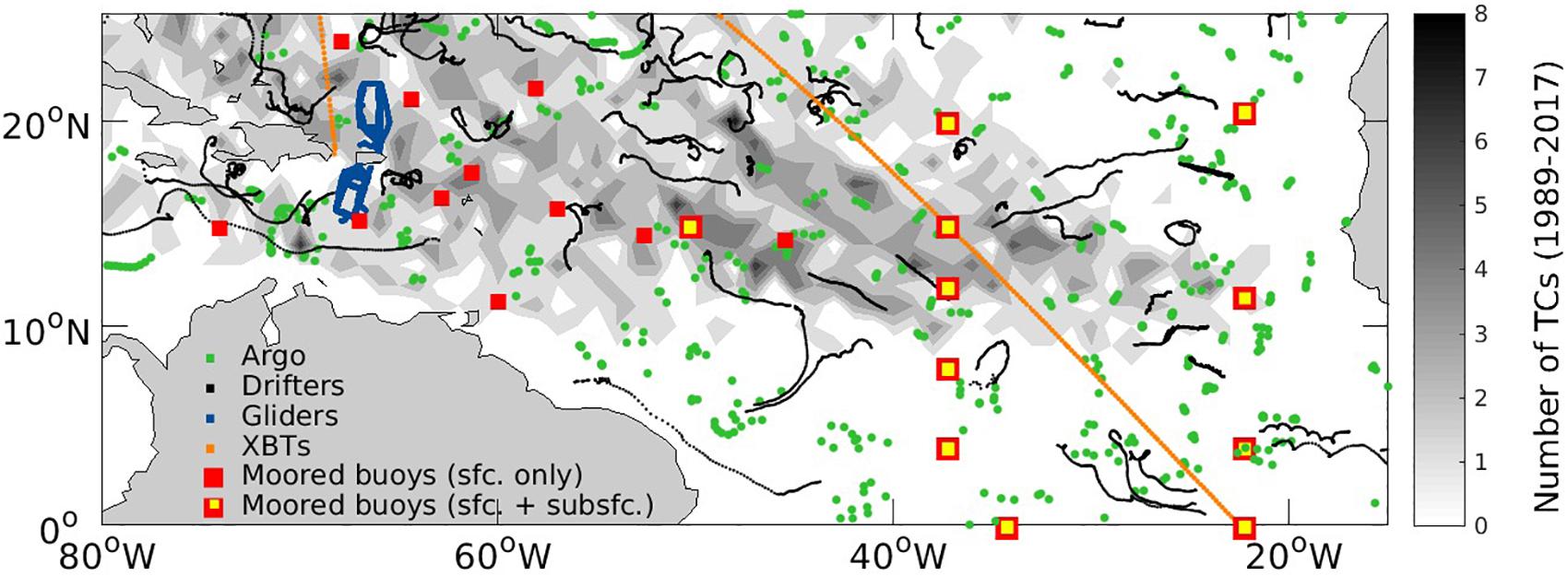
Figure 11. Key components of the sustained ocean observing system for extreme weather. Shading shows the number of tropical cyclones that passed through each 1°×1° box during 1989–2017, based on “best track” data. Black lines show the trajectories of all surface drifters during August 2018. Green dots show all Argo profiles made during August 2018. Orange lines indicate XBT lines, which are repeated approximately every 3 months, and blue lines represent ocean glider tracks during 2014–2018. Red squares indicate moored buoys with surface measurements, and yellow squares show moorings that additionally measure subsurface temperature, salinity, and/or velocity.
Improvements must be made to the observing system to advance monitoring and understanding of the processes that affect SST and surface salinity. Moorings remain a key source of co-located upper ocean and air-sea flux measurements that are extremely useful for this purpose. However, the vertical resolutions of temperature sensors on PIRATA moorings are not high enough to resolve the vertical structure of the mixed layer diurnal cycle, which can affect the mean state climate and vertical mixing (Ham et al., 2009; Hummels et al., 2013). The vertical spacing of conductivity sensors on most moorings is much too coarse for accurate resolution of the mixed layer and salinity stratification required for heat and salinity budget analyses. Vertical turbulent mixing plays an important role in the equatorial mixed layer heat and salinity budgets, and this has motivated multi-year measurements of mixing at some PIRATA locations. There are strong indications that mixing is also important at off-equatorial locations and that the driving processes are very different than those operating at the equator. However, no long-term measurements of horizontal velocity, vertical shear, and turbulent mixing exist in the tropical Atlantic outside the equatorial band (Figure 6). Vertical velocity in the upper ocean provides important preconditioning for turbulent mixing through its impact on stratification, yet direct measurements of this quantity are lacking. There are very few measurements of surface wave height and spectra in the tropical Atlantic, which are needed to improve forecasts and to advance understanding of wave-induced upper-ocean mixing. There are large uncertainties in surface turbulent heat fluxes derived from satellite and reanalysis data (Figure 7), and it is unclear how well existing bulk formulas perform, especially outside of the equatorial region. It is difficult to close the heat and salinity budgets at many PIRATA mooring locations because at present less than half of the moorings have direct measurements of longwave radiation and ocean currents.
A more complete observing system for the upper ocean will likely include measurements from many different platforms, in addition to addressing the gaps in mooring observations described above. Emerging technologies are showing promise for obtaining surface flux and upper-ocean measurements from autonomous moving platforms (Voosen, 2018). Gliders have already proven valuable for measuring upper-ocean turbulence and the factors that control it (St. Laurent and Merrifield, 2017). Satellite-based retrievals of surface radiative and turbulent fluxes show considerable skill (Yu and Weller, 2007; Trolliet et al., 2018) and are likely to improve further if satellite and in situ measurements are maintained.
Much of the present observing system was designed before coupled model biases emerged as a scientific research priority. As a result, efforts to alleviate model biases are hampered by inadequate in situ measurements (Tchipalanga et al., 2018). A more comprehensive and multifaceted observational approach is needed to make progress on this difficult problem. Based on the leading theories of what generates coupled model biases, such an improved system may include (1) arrays of near-coastal buoys and ocean gliders in the Benguela upwelling region, (2) multiplatform measurements of turbulent mixing and the processes that drive it in the equatorial Atlantic, (3) direct measurements of turbulent and radiative air-sea fluxes in the northeastern and southeastern tropical Atlantic from autonomous vehicles and moored buoys, (4) enhanced measurements of the lower troposphere in the Atlantic ITCZ region, possibly as part of a process study.
In general, biogeochemical quantities are severely undersampled compared to many physical parameters, especially in the tropical South Atlantic (Figure 12). Oxygen and fCO2 are not monitored by many elements of the tropical Atlantic observing system. Due to the importance of the OMZ for marine habitat compression and air-sea CO2 flux for the tracking of the ocean CO2 sink, measurements of oxygen and fCO2 co-located with physical variables should become the norm. Expanded measurements of other biogeochemical parameters, such as nutrients, pH, and ocean color, are also needed to monitor biogeochemical cycles and acidification. These may be achieved through (1) the continuation and expansion of biogeochemical Argo and in situ measurements from research vessels, (2) the addition of biogeochemical sensors to existing moorings, and (3) development of new technologies to measure biogeochemical parameters from autonomous and remotely controlled vehicles. Some PIRATA cruises have only recently begun to measure biogeochemical and biological parameters such as nutrients, pH/alkalinity, and phytoplankton, while others measure only temperature, salinity, and oxygen. Traditional zooplankton sampling with nets requires extra ship time, complicating its implementation. Possible alternatives include emerging technologies such as the underwater vision profiler (UVP) that can be lowered to 6000 m on a CTD rosette. In addition to taking pictures of larger particles or organisms that would normally be sampled using nets, it also captures fragile zooplankton and phytoplankton species normally not recorded in net samples (Biard et al., 2016) and has been deployed in the tropical Atlantic (Kiko et al., 2017). Alternatively, acoustic sampling of zooplankton and fish can be performed from autonomous vehicles or gliders (Lembke et al., 2018). Thus, for PIRATA the ocean biogeochemistry and ecosystem observation capabilities have not yet been fully explored.
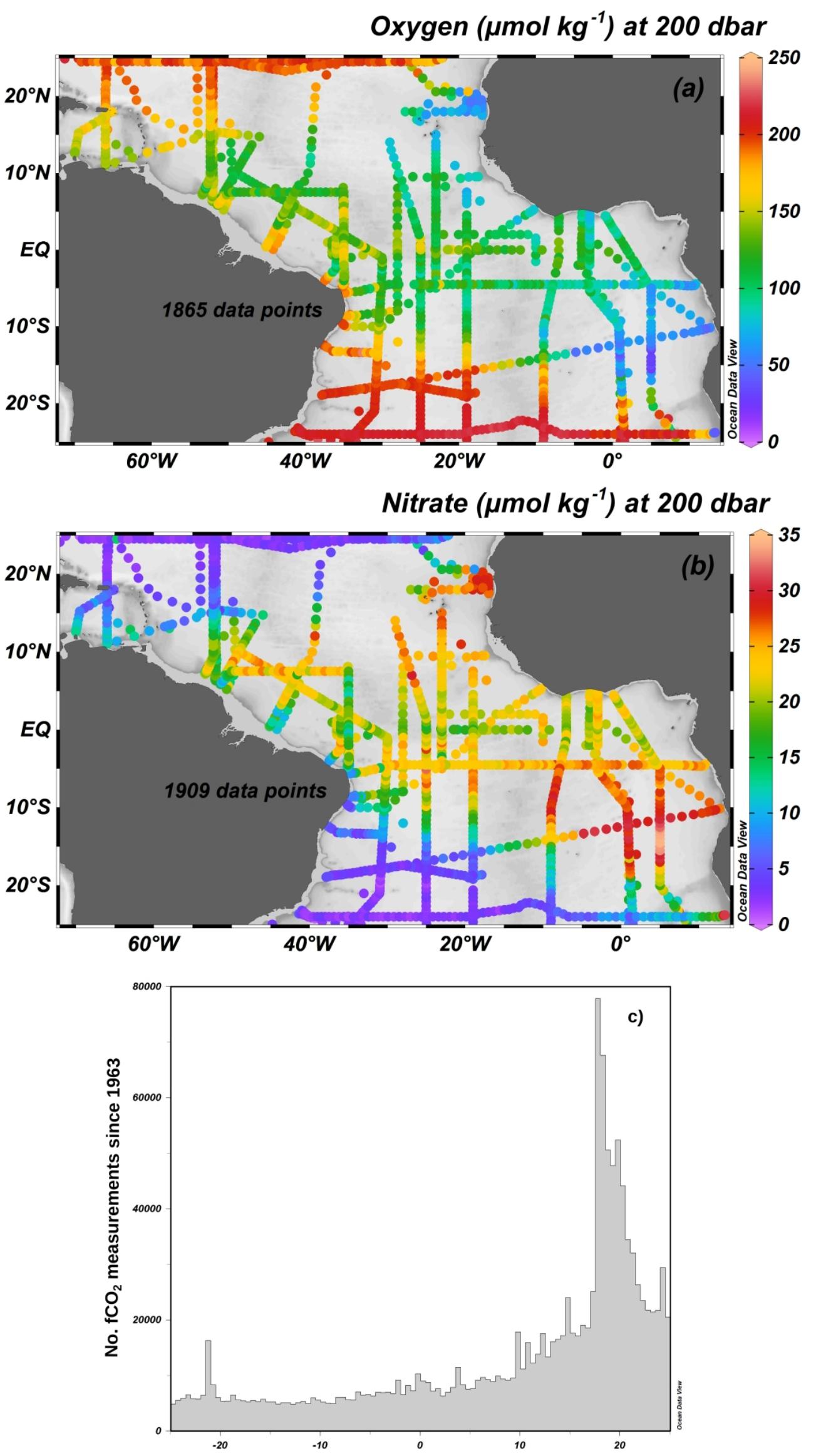
Figure 12. Number of historical in situ measurements of (a) oxygen and (b) nitrate at 200 dbar acquired during repeat hydrographic cruises (GLODAP v2 data product, Olsen et al., 2016). Note that the color scales are different in panels (a,b). (c) Total number of surface CO2 fugacity (fCO2) measurements since 1963 across all longitudes in the tropical Atlantic for each 0.5° latitude bin (SOCATv6, Bakker et al., 2016).
A current lack of monitoring of biological variables also makes it challenging to determine how ecosystems will cope with changing physical and biogeochemical conditions. Many of the methods to improve biogeochemical sampling also apply to biological sampling. Complicating biological measurements is the need for a multidisciplinary approach that includes observations of physical, biogeochemical, and biological parameters. Integration of biogeochemical and biological measurements into PIRATA and Argo may be one way forward, and PIRATA and Argo would also benefit from greater international governance of biogeochemical and biological observations. Currently, there is no long-term commitment to fund these observations continuously, only to define what constitutes biogeochemical measurements and what should be measured. In addition, the biological sampling network would greatly benefit from improved international coordination and capacity building. Currently, satellite retrievals of chlorophyll and ocean color are limited by clouds, especially in the ITCZ, providing additional motivation for in situ measurements this region.
For ocean state estimates, the PIRATA array is too sparse and the measurements are too shallow to provide constraints on the deeper ocean. Deeper measurements, including a more complete AMOC monitoring system, are needed to constrain simulations of the AMOC, sea level rise, heat and freshwater storage, and the global energy imbalance. Currently, the deep ocean (>2000 m) is very poorly sampled, severely limiting the accuracy with which these quantities can be calculated. Global arrays such as Argo, and especially the developing deep Argo program, are likely to be most useful for this purpose. Direct measurements of the AMOC from in situ monitoring arrays are also needed for validation of ocean state estimates. More comprehensive design, analysis, and intercomparison of OSSEs should be explored in order to quantify the number, type, and spatial locations of additional measurements that would most likely improve ocean data assimilation and state estimates.
The tropical Atlantic observing system generates large quantities of data covering a wide range of temporal and spatial scales and across many platforms. A key challenge is to ensure consistent data processing, archiving, availability, and visibility within and across all datasets. This is important not only to increase the usability of the data for advancement of scientific knowledge, but also to improve data assimilation and predictability of weather, climate, and ecosystems. A consistent data record is also needed to evaluate the performance of the observing system over time and recommend changes to it. The archiving and availability of data has progressed over the past decade because of several developments: (1) the use of digital object identifiers (DOIs) for datasets has expanded, (2) scientific journals have started requiring statements of data accessibility, (3) funding agencies require data management and archiving plans, and (4) common data formats such as NetCDF have gained wider acceptance. However, many important datasets remain difficult to find or are stored in formats that are difficult to read or do not contain adequate metadata. A push for open data availability and visibility and consistent archiving is needed and may be achieved through a combination of approaches, including continuation of those listed above.
Recommendations for the Future Observing System
Based on the science drivers and gaps in the observing system identified in Sections “Science Drivers” and “Strengths and Weaknesses of the Current Observing System”, below is a list of key recommendations for the future tropical Atlantic observing system. The recommendations include readiness levels (RL) to indicate the ease with which they could be implemented: (1) No additional action needed other than sustained funding, (2) Implementation in progress, (3) Additional funding required for implementation, (4) Scientific guidance/design and funding required. See also Bourlès et al. (2019) for a discussion of recommendations specifically for PIRATA.
• Most importantly, maintain observing systems that have proven their long-term value for scientific research, monitoring, and operational forecasts and analyses. These long records in the tropical Atlantic are extremely important for observing ocean-atmosphere variability on interannual to multidecadal timescales and changes in response to global warming. Continuity of satellite records is critical for maintaining basin-wide surface observations. In situ data provide information on the subsurface ocean and high-accuracy measurements near the air-sea interface that can be used to calibrate and validate satellite measurements and to bridge gaps between satellite missions. RL1
• Improve the vertical sampling on PIRATA moorings in the mixed layer and immediately below; add new sensors for ocean velocity and surface fluxes; develop new satellite measuring capabilities. Presently, the sensor spacing is too coarse to accurately calculate mixed layer depth, stratification beneath the mixed layer, and the vertical structure of the diurnal cycle of temperature near the surface. It is also recommended that every mooring have at least one single-point current meter and a downwelling longwave radiation sensor. These measurements are needed to compute temperature and salinity advection and the net surface heat flux, important components of the mixed layer heat and salinity budgets. Augmenting moorings with barometers will aid NWP and data assimilation. Proposed satellite missions such as the Sea surface KInematics Multiscale monitoring of ocean surface currents (SKIM) have great potential to aid research, data assimilation, and forecasts. Their development should be continued. RL3,4
• Sustained measurements of upper-ocean mixing are needed, along with the processes that drive it, such as current shear and surface waves. This could be achieved through moored microstructure, ocean velocity, and surface wave measurements at one or two PIRATA locations on the equator and one or two locations off the equator, together with targeted deployments of autonomous vehicles and gliders. RL4
• Monitoring of deep ocean temperature and salinity must be continued and expanded. These measurements, mainly from deep Argo and some moorings, are critical for assessing long-term changes in the ocean’s heat storage and mass balance, and the earth’s energy imbalance. They also help to monitor and understand the AMOC. RL2
• Sampling of biogeochemical and biological parameters from floats, research vessels, ships of opportunity, and moorings must be continued and expanded, especially in the tropical South Atlantic. These measurements are required for monitoring and understanding the carbon cycle and OMZ dynamics. They must be acquired concurrently with physical parameters such as temperature, salinity, and ocean velocity. RL2,3
• The moored observing system should be extended to the northwestern tropical Atlantic warm pool (AWP) and southeastern tropical Atlantic (STA), and the use of other in situ platforms should be expanded. The AWP is a region through which a high percentage of land-falling hurricanes pass. There is strong upper-ocean salinity stratification and energetic mesoscale fronts and eddies that affect air-sea fluxes and mixing and yet are poorly understood. The combination of moorings that include subsurface ocean measurements, and other platforms such as gliders and Argo, would advance knowledge and predictability of landfalling hurricanes. Augmenting the in situ observing system in the STA will help to improve understanding of the AZM, Benguela Niños, and coupled model biases. This could be achieved through a denser network of Argo floats and PIRATA moorings near the equator and additional PIRATA moorings in the southeastern tropical Atlantic. Routine servicing cruises to new moorings should be used to measure the diurnal cycle of the cloudy boundary layer. RL4
• More widespread use of autonomous platforms and gliders is recommended, especially in western and eastern boundary regions and on the continental shelf, where satellite data is unavailable or uncertain and subsurface data are sparse. Augmenting autonomous platforms and surface drifters with additional meteorological measurements, such as atmospheric pressure, winds, air temperature, and relative humidity, would be very beneficial for improving weather forecasts and air-sea flux calculations. RL2,3
• Extensions of oceanographic surveys for commercial and endangered species are needed. In many cases this will require additional capacity-building in African nations. Another related requirement is the integration of individual surveys into a larger observational system that considers the requirements of different user groups, including society, the private sector, and the scientific community. The value of all ocean observations must be communicated more widely and clearly to society. RL3,4
• The measurements of micronekton collected during the regular maintenance cruises of the PIRATA network are one on the less known components of the ocean ecosystem. These measurements must be continued and expanded to other platforms when feasible. Routine measurements of plastics during repeated PIRATA services cruises are also highly recommended, given their detrimental impacts on ecosystems. RL2,3
• Though not part of the sustained observing system, process studies are essential to improve model parameterizations, identify new scientific phenomena, and develop and test new technologies. Process studies often measure many variables at high resolution for a limited amount of time. The information gained can inform scaled-back versions for integration into the sustained observing system and point to ways in which the tropical Atlantic observing system can be adapted for the future. RL4
PIRATA and other fixed moorings are unique among the observing systems because they can be placed at predefined locations and do not drift. Following the first recommendation in the list above, there are compelling reasons to sustain the current configuration of moorings. PIRATA resolves the fast zonal propagation of oceanic signals along the equator, while at off-equatorial locations they provide essential measurements across a wide range of climate regimes. These include the low-wind, high-rainfall ITCZ; the northeastern and southeastern areas with cool SSTs, low clouds, and large concentrations of aerosols; and the northwestern tropical Atlantic, characterized by strong upper-ocean salinity stratification, energetic mesoscale variability, and poorly understood shallow convection in the atmosphere. Furthermore, there are large disparities between off-equatorial winds measured directly by PIRATA and estimated by satellites and model-based reanalyses (Bentamy and Fillon, 2012), emphasizing the importance of maintaining the long records from all PIRATA moorings. Coupled ocean-atmosphere data assimilation, a promising approach to improve oceanic and atmospheric analyses and coupled model forecasts, is in its infancy (Zhang et al., 2007; Lu et al., 2015). The most valuable data for evaluating and improving the methodologies are co-located time series of oceanic and atmospheric data from fixed-point moorings. For forecast improvements, both a long history (with a record of many past events to evaluate forecast skill) and reliable real-time data (to initialize forecasts) are critical.
Conclusion
The tropical Atlantic represents a unique and complex mix of many interacting oceanic and climate modes of variability that fluctuate across a wide variety of timescales. A large and diverse group of human populations depends on the tropical Atlantic Ocean for sustenance and recreation and is adversely affected by climate-driven changes in precipitation patterns, extreme weather and ocean ecosystems. Therefore, the demand for information about the tropical Atlantic Ocean, including subseasonal to seasonal predictions and longer-term projections in a changing climate, is only expected to increase in the future.
There has been substantial progress in building and maintaining the sustained observing system over the past 20 years. We must continue to maintain the valuable elements of the observing system, building on the successful history of international and multidisciplinary cooperation. Critical gaps in the observing system need to be filled. This may be accomplished in part through enhancements to autonomous observing networks and investments in new technologies. The tropical Atlantic is strongly influenced by conditions in the Pacific and Indian Oceans as well as the extratropical North and South Atlantic. Therefore, the success of the tropical Atlantic observing system depends in part on the maintenance and evolution of those observing systems. Continued international cooperation and observing system integration are essential in order to maintain and improve monitoring, prediction, and scientific understanding of the tropical Atlantic Ocean and related changes in weather, climate, and ecosystems.
Author Contributions
BR-F, RR, PB, MDe, IR, NL, LY, JOS, and FH led the writing of individual sections of the manuscript. GF combined all sections and wrote the full manuscript. All authors contributed to writing the sections and/or revising the full manuscript.
Funding
MM-R received funding from the MORDICUS grant under contract ANR-13-SENV-0002-01 and the MSCA-IF-EF-ST FESTIVAL (H2020-EU project 797236). GF, MG, RLu, RP, RW, and CS were supported by NOAA/OAR through base funds to AOML and the Ocean Observing and Monitoring Division (OOMD; fund reference 100007298). This is NOAA/PMEL contribution #4918. PB, MDe, JH, RH, and JL are grateful for continuing support from the GEOMAR Helmholtz Centre for Ocean Research Kiel. German participation is further supported by different programs funded by the Deutsche Forschungsgemeinschaft, the Deutsche Bundesministerium für Bildung und Forschung (BMBF), and the European Union. The EU-PREFACE project funded by the EU FP7/2007–2013 programme (Grant No. 603521) contributed to results synthesized here. LCC was supported by the UERJ/Prociencia-2018 research grant. JOS received funding from the Cluster of Excellence Future Ocean (EXC80-DFG), the EU-PREFACE project (Grant No. 603521) and the BMBF-AWA project (Grant No. 01DG12073C).
Conflict of Interest Statement
The authors declare that the research was conducted in the absence of any commercial or financial relationships that could be construed as a potential conflict of interest.
The reviewer, SC, declared a shared affiliation, with no collaboration, with one of the authors, BB, to the handling Editor at the time of review.
Footnotes
- ^ https://news.un.org/en/story/2012/05/411752-un-relief-coordinator-warns-over-humanitarian-crisis-africas-drought-hit-sahel
- ^ https://www.nhc.noaa.gov/news/UpdatedCostliest.pdf
- ^ https://en.wikipedia.org/wiki/List_of_costliest_Atlantic_hurricanes
- ^ https://unfccc.int/process/conferences/pastconferences/paris-climate-change-conference-november-2015/paris-agreement
- ^ http://www.fao.org/fishery/statistics/global-capture-production/en
- ^ https://www.ellenmacarthurfoundation.org/assets/downloads/EllenMacArthurFoundation_TheNewPlasticsEconomy_Pages.pdf
- ^ http://www.cpc.ncep.noaa.gov/products/GODAS/multiora93_body.html
- ^ http://oceantrackingnetwork.org/
References
Adebiyi, A., and Zuidema, P. (2016). The role of the southern African easterly jet in modifying the southeast Atlantic aerosol and cloud environments. Q. J. R. Meteorol. Soc. 142, 1574–1589. doi: 10.1002/qj.2765
Adebiyi, A., Zuidema, P., and Abel, S. (2015). The convolution of dynamics and moisture with the presence of shortwave absorbing aerosols over the southeast Atlantic. J. Clim. 28, 1997–2024. doi: 10.1175/JCLI-D-14-00352.1
Adler, R. F., Huffman, G. J., Chang, A., Ferraro, R., Xie, P. P., Janowiak, J., et al. (2003). The version 2 global precipitation climatology project (GPCP) monthly precipitation analysis (1979-present). J. Hydrometeorol. 4, 1147–1167. doi: 10.1175/1525-7541(2003)004<1147:TVGPCP>2.0.CO;2
Aijaz, S., Ghantous, M., Babanin, A. V., Ginis, I., Thomas, B., and Wake, G. (2017). Nonbreaking wave-induced mixing in upper ocean during tropical cyclones using coupled hurricane-ocean-wave modeling. J. Geophys. Res. Oceans 122, 3939–3963. doi: 10.1002/2016JC012219
Andreoli, R. V., and Kayano, M. T. (2003). Evolution of the equatorial and dipole modes of the sea-surface temperature in the tropical Atlantic at decadal scale. Meteorol. Atmos. Phys. 83, 277–285.
Arevalo-Martinez, D. L., Kock, A., Loscher, C. R., Schmitz, R. A., and Bange, H. W. (2015). Massive nitrous oxide emissions from the tropical South Pacific Ocean. Nat. Geosci. 8, 530–533. doi: 10.1038/NGEO2469
Athie, G., and Marin, F. (2008). Cross-equatorial structure and temporal modulation of intraseasonal variability at the surface of the Tropical Atlantic Ocean. J. Geophys. Res.-Oceans 113:C08020. doi: 10.1029/2007JC004332
Bachèlery, M.-L. (2016). Variabilité côtière physique et biogéochimique en Atlantique Sud-Est : rôle du forçage atmosphérique local versus téléconnexion océanique. Ph.D. thesis, Toulouse: Laboratoire d’Etude en Geophysique et Océanographie Spatiale (LEGOS), University of Paul Sabatier, 215.
Bachèlery, M.-L., Illig, S., and Dadou, I. (2016a). Interannual variability in the south-east atlantic ocean, focusing on the benguela upwelling system: remote versus local forcing. J. Geophys. Res. 121, 284–310. doi: 10.1002/2015JC011168
Bachèlery, M.-L., Illig, S., and Dadou, I. (2016b). Forcings of nutrient, oxygen and primary production interannual variability in the south-east Atlantic ocean. Geophys. Res. Lett. 43, 8617–8625. doi: 10.1002/2016GL070288
Bakker, D. C. E., Pfeil, B., Landa, C. S., Metzl, N., O’Brian, K. M., Olsen, A., et al. (2016). A multi-decade record of high-quality fCO2 data in version 3 of the Surface Ocean CO2 Atlas (SOCAT). Earth Syst. Sci. Data 8, 383–413. doi: 10.5194/essd-8-383-2016
Balaguru, K., Chang, P., Saravanan, R., Leung, L. R., Xu, Z., Li, M. K., et al. (2012). Ocean barrier layers’ effect on tropical cyclone intensification. Proc. Natl. Acad. Sci. U.S.A. 109, 14343–14347. doi: 10.1073/pnas.1201364109
Balaguru, K., Foltz, G. R., and Leung, L. R. (2018). Increasing magnitude of hurricane rapid intensification in the central and eastern tropical Atlantic. Geophys. Res. Lett. 45, 4328–4247. doi: 10.1029/2018GL077597
Balaguru, K., Foltz, G. R., Leung, L. R., D’Asaro, E. A., Emanuel, K. A., Liu, H., et al. (2015). Dynamic potential intensity: an improved representation of the ocean’s impact on tropical cyclones. Geophys. Res. Lett. 42, doi: 10.1002/2015GL064822
Balaguru, K., Foltz, G. R., Leung, L. R., and Emanuel, K. A. (2016). Global warming-induced upper-ocean freshening and the intensification of super typhoons. Nat. Commun. 7:13670. doi: 10.1038/ncomms13670
Barichivich, J., Gloor, E., Peylin, P., Brienen, R. J. W., Schongart, J., Espinoza, J. C., et al. (2018). Recent intensification of Amazon flooding extremes driven by strengthened Walker circulation. Sci. Adv. 2018:4. doi: 10.1126/sciadv.aat8785
Barimalala, R., Bracco, A., and Kucharski, F. (2012). The representation of the South Tropical Atlantic teleconnection to the Indian Ocean in the AR4 coupled models. Clim. Dynam. 38, 1147–1166. doi: 10.1007/s00382-011-1082-5
Barimalala, R., Bracco, A., Kucharski, F., McCreary, J. P., and Crise, A. (2013). Arabian sea ecosystem responses to the South Tropical Atlantic teleconnection. J. Mar. Syst. 117, 14–30. doi: 10.1016/j.jmarsys.2013.03.002
Bates, N. R. (2007). Interannual variability of the oceanic CO2 sink in the subtropical gyre of the North Atlantic Ocean over the last 2 decades. J. Geophys. Res. 112, C09013. doi: 10.1029/2006JC003759
Belcher, S. E., Grant, A. L. M., Hanley, K. E., Kemper, B. F., Roekel, L. V., Sullivan, P. P., et al. (2012). A global perspective on Langmuir turbulence in the ocean surface boundary layer. Geophys. Res. Lett. 39:L18605. doi: 10.1029/2012GL052932
Bellomo, K., Clement, A. C., Mauritsen, T., Radel, G., and Stevens, B. (2015). The influence of cloud feedbacks on equatorial Atlantic variability. J. Clim. 28, 2725–2744. doi: 10.1175/JCLI-D-14-00495.1
Bellomo, K., Clement, A. C., Murphy, L. N., Polvani, L. M., and Cane, M. A. (2016). New observational evidence for a positive cloud feedback that amplifies the Atlantic Multidecadal Oscillation. Geophys. Res. Lett. 43, 9852–9859. doi: 10.1002/2016GL069961
Belyaev, K. P., Tanajura, C., and O’Brien, J. J. (2001). A data assimilation method used with an ocean circulation model and its application to the tropical Atlantic. Appl. Mathemat. Modell. 25, 655–670. doi: 10.1016/S0307-904X(01)00003-8
Bentamy, A., and Fillon, D. C. (2012). Gridded surface wind fields from metop/ASCAT measurements. Int. J. Rem. Sens. 33, 1729–1754. doi: 10.1080/01431161.2011.600348
Bentamy, A., Piollé, J. F., Grouazel, A., Danielson, R., Gulev, S., Paul, F., et al. (2017). Review and assessment of latent and sensible heat flux accuracy over the global oceans. Remote Sens. Environ. 201, 196–218. doi: 10.1016/j.rse.2017.08.016
Berntell, E., Zhang, Q., Chafik, L., and Körnich, H. (2018). Representation of multidecadal sahel rainfall variability in 20th century reanalyses. Sci. Rep. 8:10937. doi: 10.1038/s41598-018-29217-9
Biard, T., Stemmann, L., Picheral, M., Mayot, N., Vandromme, P., Hauss, H., et al. (2016). In situ imaging reveals the biomass of giant protists in the global ocean. Nature 532, 504–507. doi: 10.1038/nature17652
Bodas-Salcedo, A., Webb, M. J., Bony, S., Chepfer, H., Dufresne, J. L., Klein, S. A., et al. (2011). COSP: satellite simulation software for model assessment. B. Am. Meteorol. Soc. 92:1023. doi: 10.1175/2011BAMS2856.1
Booth, B. B. B., Dunstone, N. J., Halloran, P. R., Andrews, T., and Bellouin, N. (2012). Aerosols implicated as a prime driver of twentieth-century North Atlantic climate variability. Nature 484, 228–232. doi: 10.1038/nature10946
Bourlès, B., Araujo, M., McPhaden, M. J., Brandt, P., Foltz, G. R., Lumpkin, R., et al. (2019). PIRATA: A sustained observing system for tropical Atlantic climate research and forecasting. Earth Space Sci. 6. doi: 10.1029/2018EA000428
Bourlès, B., Lumpkin, R., McPhaden, M. J., Hernandez, F., Nobre, P., Campos, E., et al. (2008). The PIRATA program: history, accomplishments, and future directions. Bull. Am. Meteorol. Soc. 89, 1111–1125. doi: 10.1175/2008BAMS2462.1
Boyer, D. C., and Hampton, I. (2001). An overview of the living marine resources of Namibia. S. Afr. J. Mar. Sci. 23, 5–35. doi: 10.2989/025776101784528953
Brandt, P., Bange, H. W., Banyte, D., Dengler, M., Didwischus, S. H., Fischer, T., et al. (2015). On the role of circulation and mixing in the ventilation of oxygen minimum zones with a focus on the eastern tropical North Atlantic. Biogeosciences 12, 489–512. doi: 10.5194/bg-12-489-2015
Brandt, P., Caniaux, G., Bourlès, B., Lazar, A., Dengler, M., Funk, A., et al. (2011a). Equatorial upper-ocean dynamics and their interaction with the West African monsoon. Atmos. Sci. Lett. 12, 24–30. doi: 10.1002/asl.287
Brandt, P., Funk, A., Hormann, V., Dengler, M., Greatbatch, R. J., and Toole, J. M. (2011b). Interannual atmospheric variability forced by the deep equatorial Atlantic Ocean. Nature 473, 497–500. doi: 10.1038/nature10013
Brandt, P., Claus, M., Greatbatch, R. J., Kopte, R., Toole, J. M., Johns, W. E., et al. (2016). Annual and semi-annual cycle of equatorial Atlantic circulation associated with basin mode resonance. J. Phys. Oceanogr. 46, 3011–3029. doi: 10.1175/JPO-D-15-0248.1
Brandt, P., Greatbatch, R. J., Claus, M., Didwischus, S. H., Hormann, V., and Funk, A. (2012). Ventilation of the equatorial Atlantic by the equatorial deep jets. J. Geophys. Res.-Oceans 117:C12015. doi: 10.1029/2012JC008118
Brandt, P., Hormann, V., Kortzinger, A., Visbeck, M., Krahmann, G., Stramma, L., et al. (2010). Changes in the ventilation of the oxygen minimum zone of the tropical North Atlantic. J. Phys. Oceanogr. 40, 1784–1801. doi: 10.1175/2010JPO4301.1
Brandt, P., Schott, F. A., Provost, C., Kartavtseff, A., Hormann, V., Bourlès, B., et al. (2006). Circulation in the central equatorial Atlantic: mean and intraseasonal to seasonal variability. Geophys. Res. Lett. 33:L07609. doi: 10.1029/2005GL025498
Bretagnon, M., (2018) Inférence De l’activité Biogéochimique Dans La Zone de Minimum d’Oxygène du Pérou, PhD Thesis, University of Toulouse, Toulouse.
Bretagnon, M., Paulmier, A., Garçon, V., Dewitte, B., Illig, S., Lebond, N., et al. (2018). Modulation of the vertical particules transfer efficiency in the oxygen minimum zone off Peru. Biogeosciences 15, 5093–5111. doi: 10.5194/bg-15-5093-2018
Breugem, W.-P., Chang, P., Jang, C. J., Mignot, J., and Hazeleger, W. (2008). Barrier layers and tropical Atlantic SST biases in coupled GCMs. Tellus A 60, 885–897. doi: 10.1111/j.1600-0870.2008.00343.x
Brito, S. S. B., Cunha, A. P. M. A., Cunningham, C. C., Alvalá, R. C., Marengo, J. A., and Carvalho, M. A. (2018). Frequency, duration and severity of drought in the Semiarid Northeast Brazil region. Int. J. Climatol. 38, 517–529. doi: 10.1002/joc.5225
Brüchert, V., Currie, B., Peard, K. R., Lass, U., Endler, R., Dübecke, A., et al. (2006). “Biogeochemical and physical control of shelf anoxia and water column hydrogen sulphide in the Benguela coastal upwelling system,” in Past and Present Water Column Anoxia, ed. L. N. Neretin (New York, NY: Springer), 161–193.
Bruneau, N., Toumi, R., and Wang, S. (2018). Impact of wave whitecapping on land falling tropical cyclones. Sci. Rep. 8:652. doi: 10.1038/s41598-017-19012-3
Bunge, L., Provost, C., Hua, B. L., and Kartavtseff, A. (2008). Variability at intermediate depths at the equator in the Atlantic Ocean in 2000–06: annual cycle, equatorial deep jets, and intraseasonal meridional velocity fluctuations. J. Phys. Oceanogr. 38, 1794–1806. doi: 10.1175/2008JPO3781.1
Burmeister, K., Brandt, P., and Lübbecke, J. F. (2016). Revisiting the cause of the eastern equatorial Atlantic cold event in 2009. J. Geophys. Res. 121, 4777–4789. doi: 10.1002/2016JC011719
Busalacchi, A. J. (1996). Data assimilation in support of tropical ocean circulation studies. Elsevier Oceanogr. Ser. 61, 235–270. doi: 10.1016/S0422-9894(96)80012-2
Cai, W., Borlace, S., Lengaigne, M., van Rensch, P., Collins, M., Vecchi, G., et al. (2014). Increasing frequency of extreme El Niño events due to greenhouse warming. Nat. Clim. Change 4, 111–116. doi: 10.1038/nclimate2100
Cai, W., Santoso, A., Wang, G., Yeh, S.-W., An, S.-I., Cobb, K., et al. (2015a). ENSO and global warming. Nat. Clim. Change 5, 849–859. doi: 10.1038/nclimate2743
Cai, W., Wang, G., Santoso, A., McPhaden, M. J., Wu, L., Jin, F.-F., et al. (2015b). Increasing frequency of extreme La Niña events induced by greenhouse warming. Nat. Clim. Change 5, 132–137. doi: 10.1038/nclimate2492
Cai, W., Wu, L., Lengaigne, M., Li, T., McGregor, S., and Kug, J.-S. (2019). Pantropical climate interactions. Science 363:eaav4236. doi: 10.1126/science.aav4236
Camara, I., Kolodziejczyk, N., Mignot, J., Lazar, A., and Gaye, A. T. (2015). On the seasonal variations of salinity of the tropical Atlantic mixed layer. J. Geophys. Res. Oceans 120, 4441–4462. doi: 10.1002/2015JC010865
Chaigneau, A., Eldin, G., and Dewitte, B. (2009). Eddy activity in the four major upwelling systems from satellite altimetry (1992-2007). Progr. Oceanogr. 83, 117–123. doi: 10.1016/j.pocean.2009.07.012
Chang, P., Fang, Y., Saravanan, R., Ji, L., and Seidel, H. (2006). The cause of the fragile relationship between the Pacific El Nino and the Atlantic Nino. Nature 443, 324–328. doi: 10.1038/nature05053
Chang, P., Saravanan, R., Ji, L., and Hegerl, G. C. (2000). The effect of local sea surface temperatures on atmospheric circulation over the tropical Atlantic sector. J. Clim. 13, 2195–2216. doi: 10.1175/1520-0442(2000)013<2195:TEOLSS>2.0.CO;2
Chang, P., Zhang, R., Hazeleger, W., Wen, C., Wan, X., Ji, K., et al. (2008). Oceanic link between abrupt changes in the North Atlantic ocean and the African monsoon. Nat. Geosci. 1, 444–448. doi: 10.1038/ngeo218
Chaves, R. R., and Nobre, P. (2004). Interactions between sea surface temperature over the South Atlantic Ocean and the South Atlantic convergence zone. Geophys. Res. Lett. 31:L03204. doi: 10.1029/2003GL018647
Chelton, D. B., and Xie, S. P. (2010). Coupled ocean-atmosphere interaction at oceanic mesoscales. Oceanography 23, 52–69. doi: 10.5670/oceanog.2010.05
Chen, C. T. A., and Borges, A. V. (2009). Reconciling opposing views on carbon cycling in the coastal ocean: continental shelves as sinks and near-shore ecosystems as sources of atmospheric CO2. Deep Sea Res. II 56, 578–590. doi: 10.1016/j.dsr2.2009.01.001
Chiang, J. C., and Vimont, D. J. (2004). Analogous Pacific and Atlantic meridional modes of tropical atmosphere–ocean variability. J. Clim. 17, 4143–4158. doi: 10.1175/JCLI4953.1
Chiang, J. C. H., Kushnir, Y., and Giannini, A. (2002). Deconstructing atlantic intertropical convergence zone variability: influence of the local cross-equatorial sea surface temperature gradient and remote forcing from the eastern equatorial Pacific. J. Geophys. Res. 107, doi: 10.1029/2000JD000307
Cintra, M. M., Lentini, C. A. D., Servain, J., Araujo, M., and Marone, E. (2015). Physical processes that drive the seasonal evolution of the Southwestern Tropical Atlantic Warm Pool. Dynam. Atmos. Oceans 72, 1–11. doi: 10.1016/j.dynatmoce.2015.08.001
Clement, A., Bellomo, K., Murphy, L. N., Cane, M. A., Mauritsen, T., Radel, G., et al. (2015). The atlantic multidecadal oscillation without a role for ocean circulation. Science 350, 320–324. doi: 10.1126/science.aab3980
Colberg, F., and Reason, C. J. C. (2006). A model study of the Angola Benguela frontal zone: sensitivity to atmospheric forcing. Geophys. Res. Lett. 33, L19608. doi: 10.1029/2006GL027463
Coles, V. J., Brooks, M. T., Hopkins, J., Stukel, M. R., Yager, P. L., and Hood, R. R. (2013). The pathways and properties of the Amazon River Plume in the tropical North Atlantic Ocean. J. Geophys. Res. Oceans 118, 6894–6913. doi: 10.1002/2013JC008981
Comte, L., and Olden, J. D. (2017). Climatic vulnerability of the world’s freshwater and marine fishes. Nat. Clim. Change 7, 718–722. doi: 10.1038/nclimate3382
Craig, J. K., and Bosman, S. H. (2013). Small spatial scale variation in fish assemblage structure in the vicinity of the northwestern gulf of Mexico hypoxic zone. Estuaries Coasts 36, 268–285. doi: 10.1007/s12237-012-9577-9
Czaja, A., Van der Vaart, P., and Marshall, J. (2002). A diagnostic study of the role of remote forcing in tropical Atlantic variability. J. Clim. 15, 3280–3290. doi: 10.1175/1520-0442(2002)015<3280:ADSOTR>2.0.CO;2
Da-Allada, C. Y., Alory, G., du Penhoat, Y., Kestenare, E., Durand, F., and Hounkonnou, N. M. (2013). Seasonal mixed-layer salinity balance in the tropical Atlantic Ocean: mean state and seasonal cycle. J. Geophys. Res. 118, 332–345. doi: 10.1029/2012JC008357
Da-Allada, C. Y., Jouanno, J., Gaillard, F., Kolodziejczyk, N., Maes, C., Reul, N., et al. (2017). Importance of the equatorial undercurrent on the sea surface salinity in the eastern equatorial Atlantic in boreal spring. J. Geophys. Res. Oceans 122, 521–538. doi: 10.1002/2016JC012342
Dai, A., and Trenberth, K. E. (2002). Estimates of freshwater discharge from continents: latitudinal and seasonal variations. J. Hydrometeorol. 3, 660–687. doi: 10.1175/1525-7541(2002)003<0660:EOFDFC>2.0.CO;2
Dal Gesso, S., Van der Dussen, J. J., Siebesma, A. P., De Roode, S. R., Boutle, I. A., Kamae, Y., et al. (2015). A singlecolumn model intercomparison on the stratocumulus representation in present-day and future climate. J. Adv. Model. Earth Syst. 7, 617–647. doi: 10.1002/2014MS000377
Davey, M. K., Huddleston, M., Sperber, K. R., Braconnot, P., Bryan, F., Chen, D., et al. (2002). STOIC: a study of coupled model climatology and variability in tropical ocean regions. Clim. Dynam. 18, 403–420. doi: 10.1007/s00382-001-0188-6
De Almeida, R. A. F., Nobre, P., Haarsma, R. J., and Campos, E. J. D. (2007). Negative ocean-atmosphere feedback in the South Atlantic Convergence Zone. Geophys. Res. Lett. 34:L18809. doi: 10.1029/2007GL030401
de Carvalho-Borges, M., Orselli, I. B. M., Ferreira, M. L. D., and Kerr, R. (2018). Seawater acidification and anthropogenic carbon distribution on the continental shelf and slope of the western South Atlantic Ocean. J. Mar. Syst. 187, 62–81. doi: 10.1016/j.jmarsys.2018.06.008
de Szoeke, S. P., Fairall, C. W., Zuidema, P., Wolfe, D. E., and Bariteau, L. (2010). Surface flux observations on the southeastern tropical Pacific ocean and attribution of SST errors in coupled ocean–atmosphere models. J. Clim. 23, 4152–4174. doi: 10.1175/2010JCLI3411.1
de Szoeke, S. P., Yuter, S., Mechem, D., Fairall, C. W., Burleyson, C., and Zuidema, P. (2012). Observations of stratocumulus clouds and their effect on the eastern Pacific surface heat budgetalong 20 S J. Climate. 25, 8542–8567. doi: 10.1175/JCLI-D-11-00681.1
Dee, D. P., Uppala, S. M., Simmons, A. J., Berrisford, P., Poli, P., and Kobayashi, S. (2011). The ERA-Interim reanalysis: configuration and performance of the data assimilation system. Q. J. R. Meteorol. Soc. 137, 553–597. doi: 10.1002/qj.828
Delgadillo, R., Voss, K., and Zuidema, P. (2018). Characteristics of optically-thin coastal Florida cumuli from surface-based lidar measurements. J. Geophys. Res, 123, 10,591–10,605. doi: 10.1029/2018JD028867
DeMaria, M., and Kaplan, J. (1994). A statistical hurricane intensity prediction scheme (SHIPS) for the Atlantic basin. Wea. Forecast. 9, 209–220. doi: 10.1175/1520-0434(1994)009<0209:ASHIPS>2.0.CO;2
Dengler, M., Schott, F. A., Eden, C., Brandt, P., Fischer, J., and Zantopp, R. J. (2004). Break-up of the Atlantic deep western boundary current into eddies at 8 degrees. Nature 432, 1018–1020. doi: 10.1038/nature03134
Deppenmeier, A.-L., Haarsma, R. J., and Hazeleger, W. (2016). The Bjerknes feedback in the tropical Atlantic in CMIP5 models. Clim. Dyn. 7:2691. doi: 10.1007/s00382-016-2992-z
Derraik, J. G. B. (2002). The pollution of the marine environment by plastic debris: a review. Mar. Pollut. Bull. 44, 842–852. doi: 10.1016/S0025-326X(02)00220-5
Desbiolles, F., Blanke, B., Bentamy, A., and Roy, C. (2016). Response of the Southern Benguela upwelling system to fine-scale modifications of the coastal wind. J. Mar. Syst. 156, 46–55. doi: 10.1016/j.jmarsys.2015.12.002
Diakhaté, M., de Coetlogon, G., Lazar, A., and Gaye, A. T. (2016). Intra-seasonal SST variability within tropical Atlantic upwellings. 2016. Q. J. R. Meteorol. Soc. 142, 372–386. doi: 10.1002/qj.2657
Diaz, R. J., and Rosenberg, R. (2008). Spreading dead zones and consequences for marine ecosystems. Science 321, 926–929. doi: 10.1126/science.1156401
Dieppois, B., Durand, A., Fournier, M., Diedhiou, A., Fontaine, B., Massei, N., et al. (2015). Low-frequency variability and zonal contrast in Sahel rainfall and Atlantic sea surface temperature teleconnections during the last century. Theor. Appl. Climatol. 121, 139–155. doi: 10.1007/s00704-014-1229-5
Ding, H., Keenlyside, N., Latif, M., Park, W., and Wahl, S. (2015a). The impact of mean state errors on the simulated equatorial atlantic interannual variability. JGR Oceans 120, 1133–1151.
Ding, H., Greatbatch, R. J., and Latif, M. (2015b). The impact of sea surface temperature bias on equatorial Atlantic interannual variability in partially coupled model experiments. Geophys. Res. Lett. 42, 5540–5546. doi: 10.1002/2015GL064799
Ding, H., Keenlyside, N. S., and Latif, M. (2010). Equatorial Atlantic internannual variability: role of heat content. J. Geophys. Res. 115:C09020. doi: 10.1029/2010JC006304
Ding, H., Keenlyside, N. S., and Latif, M. (2012). Impact of the equatorial Atlantic on the El Niño southern oscillation. Clim. Dynam. 38, 1965–1972. doi: 10.1007/s00382-011-1097-y
Dippe, T. R., Greatbatch, J., and Ding, H. (2018). On the relationship between Atlantic Niño variability and ocean dynamics. Clim. Dynam. 51, 597–612. doi: 10.1007/s00382-017-3943-z
Doi, T., Tozuka, T., and Yamagata, T. (2009). Interannual variability of the guinea dome and its possible link with the atlantic meridional mode. Clim. Dynam. 33, 985–998. doi: 10.1007/s00382-009-0574-z
Domingues, R., Goni, G., Bringas, F., Lee, S. K., Kim, H.-S., Halliwell, G., et al. (2015). Upper ocean response to Hurricane Gonzalo 2014: salinity effects revealed by targeted and sustained underwater glider observations. Geophys. Res. Lett. 42, 7131–7138. doi: 10.1002/2015GL065378
Dommenget, D., and Yu, Y. (2017). The effects of remote SST forcings on ENSO dynamics, variability and diversity. Clim. Dynam. 49, 2605–2624. doi: 10.1007/s00382-016-3472-1
Dong, B., and Sutton, R. (2015). Dominant role of greenhouse-gas forcing in the recovery of Sahel rainfall. Nat. Clim. Change 5, 757–760. doi: 10.1038/nclimate2664
Dong, L., and Zhou, T. (2014). The formation of the recent cooling in the eastern tropical Pacific Ocean and the associated climate impacts: a competition of global warming, IPO, and AMO. J. Geophys. Res. Atmos. 119, 11–272. doi: 10.1002/2013JD021395
Dragani, R., Abdalla, S., Engelen, R. J., Inness, A., and Thépaut, J.-N. (2015). Ten years of ENVISAT observations at ECMWF: a review of activities and lessons learnt. Q. J. R. Meteorol. Soc. 141, 598–610. doi: 10.1002/qj.2380
Druyan, L. M. (2011). Studies of 21st-century precipitation trends over West Africa. Int. J. Climatol. 31, 1415–1424. doi: 10.1002/joc.2180
Duacs/Aviso+ (2014), Liu and Tang (1996). (2014). A New Version of SSALTO/Duacs Products Available in April 2014. Version 1.1,CNES. Absolute dynamic topography and surface geostrophic currents. Available at http://www.aviso.altimetry.fr/fileadmin/documents/data/duacs/Duacs2014.pdf
Durack, P. J., Wijffels, S. E., and Matear, R. J. (2012). Ocean salinities reveal strong global water cycle intensification during 1950 to 2000. Science 336, 455–458. doi: 10.1126/science.1212222
Elsner, J. B., Kossin, J. P., and Jagger, T. H. (2008). The increasing intensity of the strongest tropical cyclones. Nature 455, 92–95. doi: 10.1038/nature07234
Emanuel, K. (2017). Will global warming make hurricane forecasting more difficult? Bull. Am. Meteorol. Soc. 98, 495–501. doi: 10.1175/BAMS-D-16-0134.1
Emanuel, K. A. (1999). Thermodynamic control of hurricane intensity. Nature 401, 665–669. doi: 10.1038/44326
Emanuel, K. A. (2005). Increasing destructiveness of tropical cyclones over the past 30 years. Nature 436, 686–688. doi: 10.1038/nature03906
Enfield, D. B., and Mayer, D. A. (1997). Tropical Atlantic sea surface temperature variability and its relation to El Nino Southern Oscillation. J. Geophys. Res. 102, 929–945. doi: 10.1029/96JC03296
Evan, A. T., Foltz, G. R., Zhang, D., and Vimont, D. J. (2011). Influence of African dust on ocean-atmosphere variability in the tropical Atlantic. Nat. Geosci. 4, 762–765. doi: 10.1038/ngeo1276
Fairall, C. W., Bradley, E. F., Hare, J. E., Grachev, A. A., and Edson, J. B. (2003). Bulk parameterization of air-sea fluxes: updates and verification for the COARE algorithm. J. Clim. 16, 571–591. doi: 10.1175/1520-0442(2003)016<0571:BPOASF>2.0.CO;2
Faye, S., Lazar, A., Sow, B., and Gaye, A. T. (2015). A model study of the seasonality of sea surface temperature and circulation in the Atlantic North-Eastern Tropical Upwelling System. Front. Phys. 3:76. doi: 10.3389/fphy.2015.00076
Feely, R. A., Sabine, C. L., Lee, K., Berelson, W., Kleypas, J., Fabry, V. J., et al. (2004). Impact of anthropogenic CO2 on the CaCO3 system in the oceans. Science 305, 362–366. doi: 10.1126/science.1097329
Fennel, W., Junker, T., Schmidt, M., and Mohrholz, V. (2012). Response of the Benguela upwelling systems to spatial variations in the wind stress. Cont. Shelf Res. 45, 65–77. doi: 10.1016/j.csr.2012.06.004
Fiedler, B., Grundle, D. S., Schütte, F., Karstensen, J., Löscher, C. R., Hauss, H., et al. (2016). Oxygen utilization and downward carbon flux in an oxygen-depleted eddy in the eastern tropical North Atlantic. Biogeosciences 13, 5633–5647. doi: 10.5194/bg-13-5633-2016
Filizola, N., Latrubesse, E. M., Fraizy, P., Souza, R., Guimaraes, V., and Guyot, J.-L. (2014). Was the 2009 flood the most hazardous or the largest ever recorded in the Amazon? Geomorphology 215, 99–105. doi: 10.1016/j.geomorph.2013.05.028
Fischer, T., Banyte, D., Brandt, P., Dengler, M., Krahmann, G., Tanhua, T., et al. (2013). Diapycnal oxygen supply to the tropical North Atlantic oxygen minimum zone. Biogeosciences 10, 5079–5093. doi: 10.5194/bg-10-5079-2013
Foltz, G. R., Grodsky, S. A., Carton, J. A., and McPhaden, M. J. (2003). Seasonal mixed layer heat budget of the tropical Atlantic Ocean. J. Geophys. Res.-Oceans 108:3146. doi: 10.1029/2002JC001584
Foltz, G. R., and McPhaden, M. J. (2008). Seasonal mixed layer salinity balance of the tropical North Atlantic Ocean. J. Geophys. Res. Oceans 113:C02013. doi: 10.1029/2007JC004178
Foltz, G. R., and McPhaden, M. J. (2010a). Abrupt equatorial wave-induced cooling of the Atlantic cold tongue in 2009. Geophys. Res. Lett. 37:L24605. doi: 10.1029/2010GL045522
Foltz, G. R., and McPhaden, M. J. (2010b). Interaction between the Atlantic meridional and Nino modes. Geophys. Res. Lett. 37:L18604. doi: 10.1029/2010GL044001
Foltz, G. R., McPhaden, M. J., and Lumpkin, R. (2012). A strong Atlantic meridional mode event in 2009: the role of mixed layer dynamics. J. Clim. 25, 363–380. doi: 10.1175/JCLI-D-11-00150.1
Foltz, G. R., Schmid, C., and Lumpkin, R. (2013). Seasonal cycle of the mixed layer heat budget in the Northeastern Tropical Atlantic Ocean. J. Clim. 26, 8169–8188. doi: 10.1175/JCLI-D-13-00037.1
Foltz, G. R., Schmid, C., and Lumpkin, R. (2015). Transport of surface freshwater from the equatorial to the subtropical North Atlantic Ocean. J. Phys. Oceanogr. 45, 1086–1102. doi: 10.1175/JPO-D-14-0189.1
Foltz, G. R., Schmid, C., and Lumpkin, R. (2018). An enhanced PIRATA dataset for tropical atlantic ocean-atmosphere research. J. Clim. 31, 1499–1524. doi: 10.1175/JCLI-D-16-0816.1
Frajka-Williams, E., Lankhorst, M., Koelling, J., and Send, U. (2018). Coherent circulation changes in the deep north atlantic from 16 degrees N and 26 degrees N transport arrays. J. Geophys. Res.-Oceans 123, 3427–3443. doi: 10.1029/2018JC013949
Frankignoul, C., and Kestenare, E. (2005). Air–sea interactions in the tropical Atlantic: a view based on lagged rotated maximum covariance analysis. J. Clim. 18, 3874–3890. doi: 10.1175/JCLI3498.1
Gasparin, F., Greiner, E., Lellouche, J. M., Legalloudec, O., Garric, G., and Drillet, Y. (2018). A large-scale view of oceanic variability from 2007 to 2015 in the global high resolution monitoring and forecasting system at Mercator Ocean. J. Mar. Sys. 187, 260–276. doi: 10.1016/j.jmarsys.2018.06.015
Gasparin, F., Guinehut, S., Mao, C., Mirouze, I., Remy, E., King, R., et al. (2019). Requirements for an integrated in situ atlantic ocean observing system from coordinated observing system simulation experiments. Front. Mar. Sci. 6:83. doi: 10.3389/fmars.2019.00083
Giannini, A., Saravanan, R., and Chang, P. (2005). Dynamics of the boreal summer African monsoon in the NSIPP1 atmospheric model. Clim. Dyn. 25, 517–535. doi: 10.1007/s00382-005-0056-x
Giarolla, E., Siqueira, L. S. P., Bottino, M. J., Malagutti, M., Capistrano, V. B., and Nobre, P. (2015). Equatorial Atlantic Ocean dynamics in a coupled ocean-atmosphere model simulation. Ocean Dyn. 65, 831–843. doi: 10.1007/s10236-015-0836-8
Gilly, W. F., Beman, J. M., Litvin, S. Y., and Robison, B. H. (2013). Oceanographic and biological effects of shoaling of the oxygen minimum zone. Annu. Rev. Mar. Sci. 5, 393–420. doi: 10.1146/annurev-marine-120710-100849
Giordani, H., Caniaux, G., and Voldoire, A. (2013). Intraseasonal mixed-layer heat budget in the equatorial Atlantic during the cold tongue development in 2006. J. Geophys. Res. 118, 650–671. doi: 10.1029/2012JC008280
Goes, M., Goni, G., Hormann, V., and Perez, R. C. (2013). Variability of the Atlantic off-equatorial eastward currents during 1993–2010 using a synthetic method. J. Geophys. Res. Oceans 118, 3026–3045. doi: 10.1002/jgrc.20186
Goes, M., Marshall, D. P., and Wainer, I. (2009). Eddy formation in the tropical atlantic induced by abrupt changes in the meridional overturning circulation. J. Phys. Oceanogr. 39, 3021–3031. doi: 10.1175/2009JPO4004.1
Goldenberg, S. B., Landsea, C. W., Mestas-Nuñez, A. M., and Gray, W. M. (2001). The recent increase in Atlantic hurricane activity: causes and implications. Science 293, 474–479. doi: 10.1126/science.1060040
Goni, G. J., and Johns, W. E. (2001). A census of North Brazil current rings observed from TOPEX/POSEIDON altimetry: 1992-1998. Geophys. Res. Lett. 28, 1–4. doi: 10.1029/2000GL011717
Goubanova, K., Illig, S., Machu, E., Garcon, V., and Dewitte, B. (2013). SST subseasonal variability in the central Benguela upwelling system as inferred from satellite observations (1999-2009). J. Geophys. Res. Oceans 118, 4092–4110. doi: 10.1002/jgrc.20287
Grodsky, S. A., Carton, J. A., and McClain, C. R. (2008). Variability of upwelling and chlorophyll in the equatorial Atlantic. Geophys. Res. Lett. 35, L03610. doi: 10.1029/2007GL032466
Grodsky, S. A., Carton, J. A., Nigam, S., and Okumura, Y. M. (2012a). Tropical Atlantic biases in CCSM4. J. Clim. 25, 3684–3701. doi: 10.1175/JCLI-D-11-00315.1
Grodsky, S. A., Reul, N., Lagerloef, G., Reverdin, G., Carton, J. A., Chapron, B., et al. (2012b). Haline hurricane wake in the Amazon/Orinoco plume: AQUARIUS/SACD and SMOS observations. Geophys. Res. Lett. 39:L20603. doi: 10.1029/2012GL053335
Grodsky, S. A., Reverdin, G., Carton, J. A., and Coles, V. J. (2014). Year-to-year salinity changes in the Amazon plume: contrasting 2011 and 2012 aquarius/SACD and SMOS satellite data. Remote Sens. Environ. 140, 14–22. doi: 10.1016/j.rse.2013.08.033
Grossmann, I., and Morgan, M. G. (2011). Tropical cyclones, climate change, and scientific uncertainty: what do we know, what does it mean, and what should be done? Clim. Change 108, 543–579. doi: 10.1007/s10584-011-0020-1
Gruber, N. (2011). Warming up, turning sour, losing breath: ocean biogeochemistry under global change. Philos. Trans. R. Soc. A 369, 1980–1996. doi: 10.1098/rsta.2011.0003
Gruber, N., Doney, S., Emerson, S., Gilbert, D., Kobayashi, T., Körtzinger, A., et al. (2010). “Adding oxygen to Argo: developing a global in-situ observatory for ocean deoxygenation and biogeochemistry,” in Proceedings of the “OceanObs’09: Sustained Ocean Observations and Information for Society”, eds J. Hall, D. E. Harrison, and D. Stammer (Venice-Lido: Venice Convention Centre), doi: 10.5270/OceanObs09.cwp.39
Haarsma, R. J., Roberts, M. J., Vidale, P. L., Senior, C. A., Bellucci, A., Bao, Q., et al. (2016). High resolution model intercomparison project (HighResMIP v1.0) for CMIP6. Geosci. Model Dev. 9, 4185–4208. doi: 10.5194/gmd-9-4185-2016
Hahn, J., Brandt, P., Greatbatch, R. J., Krahmann, G., and Kortzinger, A. (2014). Oxygen variance and meridional oxygen supply in the Tropical North East Atlantic oxygen minimum zone. Clim. Dyn. 43, 2999–3024. doi: 10.1007/s00382-014-2065-0
Hahn, J., Brandt, P., Schmidtko, S., and Krahmann, G. (2017). Decadal oxygen change in the eastern tropical North Atlantic. Ocean Sci. 13, 551–576. doi: 10.5194/os-13-551-2017
Ham, Y.-G., Kug, J.-S., Kang, I.-S., Jin, F.-F., and Timmermann, A. (2009). Impact of diurnal atmosphere-ocean coupling on tropical climate simulations using a coupled GCM. Clim. Dyn. 34, 905–917. doi: 10.1007/s00382-009-0586-8
Ham, Y. G., Kug, J. S., Park, J. Y., and Jin, F. F. (2013a). Sea surface temperature in the north tropical Atlantic as a trigger for El Niño/Southern oscillation events. Nat. Geosci. 6, 112. doi: 10.1038/ngeo1686
Ham, Y.-G., Kug, J.-S., and Park, J.-Y. (2013b). Two distinct roles of Atlantic SSTs in ENSO variability: north tropical Atlantic SST and Atlantic Niñ;o. Geophys. Res. Lett. 40, 4012–4017. doi: 10.1002/grl.50729
Hastenrath, S. (2006). Circulation and teleconnection mechanisms of Northeast Brazil droughts. Prog. Oceanogr. 70, 407–415. doi: 10.1016/j.pocean.2005.07.004
Hatje, V., Lamborg, C. H., and Boyle, E. A. (2018). Trace metal contaminants: human footprint on the ocean. Elements 14, 403–408. doi: 10.2138/gselements.14.6.403
Hayes, S. P., McPhaden, M. J., and Wallace, J. M. (1989). The influence of sea- surface temperature on surface wind in the eastern equatorial Pacific: weekly to monthly variability. J. Clim. 2, 1500–1506. doi: 10.1175/1520-0442(1989)002<1500:TIOSST>2.0.CO;2
Hazeleger, W., and Haarsma, R. J. (2005). Sensitivity of tropical Atlantic climate to mixing in a coupled ocean-atmosphere model. Clim. Dynam. 25, 387–399. doi: 10.1007/s00382-005-0047-y
Henderson, G. M., Achterberg, E. P., and Bopp, L. (2018). Changing trace element cycles in the 21st century ocean. Elements 14, 409–413. doi: 10.2138/gselements.14.6.409
Herbert, G., and Bourlès, B. (2018). Impact of intraseasonal wind bursts on sea surface temperature variability in the far eastern tropical Atlantic Ocean during boreal spring 2005 and 2006: focus on the mid-May 2005 event. Ocean Sci. 14, 2018. doi: 10.5194/os-14-849-2018
Hernandez, O., Jouanno, J., and Durand, F. (2016). Do the Amazon and Orinoco freshwater plumes really matter for hurricane-induced ocean surface cooling? J. Geophys. Res. 121, 2119–2141. doi: 10.1002/2015JC011021
Hobday, A. J., Alexander, L. V., Perkins, S. E., Smale, D. A., Straub, S. C., Oliver, E. C. J., et al. (2016). A hierarchical approach to defining marine heatwaves. Prog. Oceanogr. 141, 227–238. doi: 10.1016/j.pocean.2015.12.014
Hsu, W.-C., Patricola, C. M., and Chang, P. (2019). The impact of climate model sea surface temperature biases on tropical cyclone simulations. Clim. Dyn. (in press). doi: 10.1007/s00382-018-4577-5
Hu, C., Murch, B., Barnes, B. B., Wang, M., Marechal, J.-P., Franks, J., et al. (2016). Sargassum watch warns of incoming seaweed. Eos Trans. AGU 97, doi: 10.1029/2016EO058355
Hu, Z.-Z., Huang, B., and Pegion, K. (2008). Low cloud errors over the southeastern Atlantic in the NCEP CFS and their association with lower-tropospheric stability and air-sea interaction. J. Geophys. Res. 113:D12114. doi: 10.1029/2007JD009514
Huang, B., Hu, Z.-Z., and Jha, B. (2007). Evolution of model systematic errors in the tropical Atlantic basin from coupled climate hindcasts. Clim. Dyn. 28, 661–682. doi: 10.1007/s00382-006-0223-8
Huang, P., Lin, I. I., Chou, C., and Huang, R. H. (2015). Change in ocean subsurface environment to suppress tropical cyclone intensification under global warming. Nat. Commun. 6:7188. doi: 10.1038/ncomms13670
Hughes, T. P., Anderson, K. D., Connolly, S. R., Heron, S. F., Kerry, J. T., Lough, J. M., et al. (2018). Spatial and temporal patterns of mass bleaching of corals in the Anthropocene. Science 359, 80–83. doi: 10.1126/science.aan8048
Hummels, R., Brandt, P., Dengler, M., Fischer, J., Araujo, M., Veleda, D., et al. (2015). Interannual to decadal changes in the western boundary circulation in the Atlantic at 11 degrees S. Geophys. Res. Lett. 42, 7615–7622. doi: 10.1002/2015GL065254
Hummels, R., Dengler, M., and Bourlés, B. (2013). Seasonal and regional variability of upper ocean diapycnal heat flux in the Atlantic Cold Tongue. Prog. Oceanogr. 111, 52–74. doi: 10.1016/j.pocean.2012.11.001
Ibánhez, J. S. P., Flores, M., and Lefèvre, N. (2017). Collapse of the tropical and subtropical North Atlantic CO2 sink in boreal spring of 2010. Sci. Rep. 7, 1–9. doi: 10.1038/srep41694
Illig, S., and Bachèlery, M. L. (2019). Propagation of subseasonal equatorially-forced coastal trapped waves down to the benguela upwelling system. Sci. Rep. 9:5306. doi: 10.1038/s41598-019-41847-1
Illig, S., Cadier, E., Bachèlery, M.-L., and Kersalé, M. (2018a). Subseasonal coastal-trapped wave propagations in the southeastern Pacific and Atlantic Oceans: 1. A new approach to estimate wave amplitude. J. Geophys. Res. Ocean 123, 3915–3941. doi: 10.1029/2017JC013539
Illig, S., Bachèlery, M.-L., and Cadier, E. (2018b). Subseasonal coastal-trapped wave propagations in the southeastern Pacific and Atlantic Oceans: 2. Wave characteristics and connection with the equatorial variability. J. Geophys. Res. Ocean 123, 3942–3961. doi: 10.1029/2017JC013540
Illig, S., and Dewitte, B. (2006). Local coupled equatorial variability versus remote ENSO forcing in an intermediate coupled model of the tropical atlantic. J. Clim. 19, 5227–5252. doi: 10.1175/JCLI3922.1
Isaksen, L., and Janssen, P. A. E. M. (2004). Impact of ERS scatterometer winds in ECMWF’s assimilation system. Quart. J. R. Meteorol. Soc. 130, 1793–1814. doi: 10.1256/qj.03.110
Jackson, D. L., Wick, G. A., and Bates, J. J. (2006). Near-surface retrieval of air temperature and specific humidity using multisensor microwave satellite observations. J. Geophys. Res. 111:D10306. doi: 10.1029/2005JD006431
Janicot, S., Harzallah, A., Fontaine, B., and Moron, V. (1998). West African monsoon dynamics and eastern equatorial Atlantic and Pacific SST anomalies (1970-88). J. Clim. 11, 1874–1882. doi: 10.1175/1520-0442-11.8.1874
Janicot, S., Trzaska, S., and Poccard, I. (2001). Summer Sahel-ENSO teleconnection and decadal time scale SST variations. Clim. Dyn. 18, 303–320. doi: 10.1007/s003820100172
Jayne, S. R., Roemmich, D. H., Zilberman, N. V., Riser, S. C., Johnson, K. S., Johnson, G. C., et al. (2017). The argo program: present and future. Oceanography 30, 18–28. doi: 10.5670/oceanog.2017.213
Jochum, M., Briegleb, B. P., Danabasoglu, G., Large, W. G., Norton, N. J., Jayne, S. R., et al. (2013). The impact of oceanic near-inertial waves on climate. J. Climate 26, 2833–2844. doi: 10.1175/JCLI-D-12-00181.1
Jochum, M., Malanotte-Rizzoli, P., and Busalacchi, A. (2004). Tropical instability waves in the Atlantic ocean. Ocean Model. 7, 145–163. doi: 10.1016/S1463-5003(03)00042-8
Johns, W. E., Brandt, P., Bourlès, B., Tantet, A., Papapostolou, A., and Houk, A. (2014). Zonal structure and seasonal variability of the Atlantic equatorial undercurrent. Clim. Dynam. 43, 3047–3069. doi: 10.1007/s00382-014-2136-2
Joly, M., and Voldoire, A. (2010). Role of the Gulf of Guinea in the inter-annual variability of the West African monsoon: what do we learn from CMIP3 coupled simulations? Int. J. Climatol. 30, 1843–1856.
Jones, A., Haywood, J., and Boucher, O. (2009). Climate impacts of geoengineering marine stratocumulus clouds. J. Geophys. Res. 114:D10106. doi: 10.1029/2008JD011450
Jouanno, J., Hernandez, O., and Sanchez-Gomez, E. (2017). Equatorial Atlantic interannual variability and its relation to dynamic and thermodynamic processes. Earth Syst. Dynam. 8, 1061–1069. doi: 10.5194/esd-8-1061-2017
Jouanno, J., Marin, F., duPenhoat, Y., Sheinbaum, J., and Molines, J. (2011). Seasonal heat balance in the upper 100 m of the Equatorial Atlantic Ocean. J. Geophys. Res. 116, C09003. doi: 10.1029/2010JC006912
Junker, T., Schmidt, M., and Mohrholz, V. (2015). The relation of wind stress curl and meridional transport in the Benguela upwelling system. J. Mar. Sys. 143, 1–6. doi: 10.1016/j.jmarsys.2014.10.006
Kalvelage, T., Lavik, G., Lam, P., Contreras, S., Arteaga, L., Loscher, C. R., et al. (2013). Nitrogen cycling driven by organic matter export in the South Pacific oxygen minimum zone. Nat. Geosci. 6, 228–234. doi: 10.1038/ngeo1739
Kaplan, J., DeMaria, M., and Knaff, J. A. (2010). A revised tropical cyclone rapid intensification index for the atlantic and eastern north pacific basins. Wea Forecast. 25, 220–241. doi: 10.1175/2009WAF2222280.1
Karstensen, J., Fiedler, B., Schutte, F., Brandt, P., Körtzinger, A., Fischer, G., et al. (2015). Open ocean dead zones in the tropical North Atlantic Ocean. Biogeosciences 12, 2597–2605. doi: 10.5194/bg-12-2597-2015
Karstensen, J., Stramma, L., and Visbeck, M. (2008). Oxygen minimum zones in the eastern tropical Atlantic and Pacific. Prog. Oceanogr. 77, 331–350. doi: 10.1016/j.pocean.2007.05.009
Kato, S., Loeb, N. G., Rose, F. G., Doelling, D. R., Rutan, D. A., Caldwell, T. E., et al. (2013). Surface irradiances consistent with CERES-derived top-of-atmosphere shortwave and longwave irradiances. J. Clim. 26, 2719–2740. doi: 10.1175/JCLI-D-12-00436.1
Keenlyside, N. S., Ding, H., and Latif, M. (2013). Potential of equatorial Atlantic variability to enhance El Niño prediction. Geophys. Res. Lett. 40, 2278–2283. doi: 10.1002/grl.50362
Keenlyside, N. S., and Latif, M. (2007). Understanding equatorial Atlantic interannual variability. J. Clim. 20, 131–142. doi: 10.1175/JCLI3992.1
Kerr, R. A. (2000). A North Atlantic climate pacemaker for the centuries. Science 288, 1984–1985. doi: 10.1126/science.288.5473.1984
Kiko, R., Biastoch, A., Brandt, P., Cravatte, S., Hauss, H., Hummels, R., et al. (2017). Biological and physical influences on marine snowfall at the equator. Nat. Geosci. 10, 852–858. doi: 10.1038/ngeo3042
Klein, S. A., and Hartmann, D. L. (1993). The seasonal cycle of low stratiform clouds. J. Clim. 6, 1587–1606. doi: 10.1175/1520-0442(1993)006<1587:TSCOLS>2.0.CO;2
Knight, J. R., Allan, R. J., Folland, C. K., Vellinga, M., and Mann, M. E. (2005). A signature of persistent natural thermohaline circulation cycles in observed climate. Geophys. Res. Lett. 32:L20708. doi: 10.1029/2005GL024233
Knight, J. R., Folland, C. K., and Scaife, A. A. (2006). Climate impacts of the Atlantic multidecadal oscillation. Geophys. Res. Lett. 33:L17706. doi: 10.1029/2006GL026242
Knutson, T. R., McBride, J. L., Chan, J., Emanuel, K., Holland, G., Landsea, C., et al. (2010). Tropical cyclones and climate change. Nat. Geosci. 3, 157–163. doi: 10.1038/NGEO779
Kopte, R., Brandt, P., Claus, M., Greatbatch, R. J., and Dengler, M. (2018). Role of equatorial basin-mode resonance for the seasonal variability of the angola current at 11 degrees S. J. Phys. Oceanogr. 48, 261–281. doi: 10.1175/JPO-D-17-0111.1
Kopte, R., Brandt, P., Dengler, M., Tchipalanga, P. C. M., Macueria, M., and Ostrowski, M. (2017). The angola current: flow and hydrographic characteristics as observed at 11 degrees S. J. Geophys. Res.-Oceans 122, 1177–1189. doi: 10.1002/2016JC012374
Koseki, S., Keenlyside, N., Demissie, T., Toniazzo, T., Counillon, F., Bethke, I., et al. (2018). Causes of the large warm bias in the Angola–Benguela frontal zone in the norwegian earth system model. Clim. Dynam. 50, 4651–4670. doi: 10.1007/s00382-017-3896-2
Kossin, J. P. (2017). Hurricane intensification along United States coast suppressed during active hurricane periods. Nature 541, 390–393. doi: 10.1038/nature20783
Kossin, J. P. (2018). A global slowdown of tropical cyclone translation speed. Nature 558, 104–107. doi: 10.1038/s41586-018-0158-3
Kossin, J. P., and Vimont, D. J. (2007). A more general framework for understanding Atlantic hurricane variability and trends. Bull. Am. Meteorol. Soc. 88, 1767–1781. doi: 10.1175/BAMS-88-11-1767
Koungue, R. A. I., Illig, S., and Rouault, M. (2017). Role of interannual Kelvin wave propagations in the equatorial Atlantic on the Angola Benguela Current system. J. Geophys. Res. 122:46854703. doi: 10.1002/2016JC012463
Kucharski, F., Bracco, A., Yoo, J. H., and Molteni, F. (2007). Low-frequency variability of the Indian monsoon-ENSO relationship and the tropical Atlantic: the “weakening” of the 1980s and 1990s. J. Clim. 20, 4255–4266. doi: 10.1175/JCLI4254.1
Kucharski, F., Bracco, A., Yoo, J. H., and Molteni, F. (2008). Atlantic forced component of the Indian monsoon interannual variability. Geophys. Res. Lett. 35, L04706. doi: 10.1029/2007GL033037
Lacerda, F. F., Nobre, P., Sobral, M. C., Lopes, G. M. B., Chou, S. C., Assad, E. D., et al. (2015). Long-term temperature and rainfall trends over Northeast Brazil and cape verde. J. Earth Sci. Clim. Change 6:296. doi: 0.4172/2157-7617.1000296
Lachkar, Z., and Gruber, N. (2012). A comparative study of biological production in eastern boundary upwelling systems using an artificial neural network. Biogeosciences 9, 293–308. doi: 10.5194/bg-9-293-2012
Landschützer, P., Gruber, N., Bakker, D. C. E., and Schuster, U. (2014). Recent variability of the global ocean carbon sink. Glob. Biogeochem. Cycles 28, 927–949. doi: 910.1002/2014GB004853
Large, W. G., and Yeager, S. G. (2008). The global climatology of an interannually varying air-sea flux data set. Clim. Dynam. 33, 341–364. doi: 10.1007/s00382-008-0441-3
Latif, M., Keenlyside, N., and Bader, J. (2007). Tropical sea surface temperature, vertical wind shear, and hurricane development. Geophys. Res. Lett. 34:L01710. doi: 10.1029/2006GL027969
Lauer, A., and Hamilton, K. (2013). Simulating clouds with global climate models: a comparison of CMIP5 results with CMIP3 and satellite data. J. Clim. 26, 3823–3845. doi: 10.1175/JCLI-D-12-00451.1
Laxenaire, R., Speich, S., Blanke, B., Chaigneau, A., Pegliasco, C., and Stegner, A. (2019). Anticyclonic eddies connecting the western boundaries of Indian and Atlantic oceans. J. Geophys. Res. 123, 7651–7677. doi: 10.1029/2018JC014270
Le Quéré, C., Andrew, R. M., Friedlingstein, P., Sitch, S., Pongratz, J., Manning, A. C., et al. (2018). Global carbon budget 2017. Earth Syst. Sci. Data 10, 405–448. doi: 10.5194/essd-10-405-2018
Lebedev, K., Yoshinari, H., Maximenko, N. A., and Hacker,P. W. (2007). YoMaHa’07: velocity data assessed from trajectories of Argo floats at parking level and at the sea surface. IPRC Tech. Note 4:16.
Lefèvre, N., Caniaux, G., Janicot, S., and Gueye, A. K. (2013). Increased CO2 outgassing in February-May 2010 in the tropical Atlantic following the 2009 Pacific El Niño. J. Geophys. Res. 118, 1645–1657. doi: 10.1002/jgrc.20107
Lefevre, N., Veleda, D., Araujo, M., and Caniaux, G. (2016). Variability and trends of carbon parameters at a time series in the eastern tropical Atlantic. Tellus B 68:30305. doi: 10.3402/tellusb.v68.30305
Lembke, C., Lowerre-Barbieri, S., Mann, D., and Taylor, J. C. (2018). Using three acoustic technologies on underwater gliders to survey fish. Mar. Technol. Soc. J. 52, 39–52. doi: 10.4031/MTSJ.52.6.1
Lewis, S. L., Brando, P. M., Phillips, O. L., van der Heijden, G. M. F., and Nepstad, D. (2011). The 2010 Amazon drought. Science 331, 554–555. doi: 10.1126/science.1200807
Li, H., and Sriver, R. L. (2018). Tropical cyclone activity in the high-resolution community earth system model and the impact of ocean coupling. J. Adv. Model. Earth Syst. 10, 165–186. doi: 10.1002/2017MS001199
Liebmann, B., and Mechoso, C. R. (2011). “The south American monsoon system,” in The Global Monsoon System: Research and Forecast, ed. C.-P. Chang (Singapore: World Scientific Series on Asia-Pacific Weather and Climate), 137–157. doi: 10.1142/9789814343411_0009
Lindzen, R. S., and Nigam, S. (1987). On the role of sea surface temperature gradients in forcing low level winds and convergence in the Tropics. J. Atmos. Sci. 44, 2418–2436. doi: 10.1175/1520-0469(1987)044<2418:OTROSS>2.0.CO;2
Liu, P., and Sui, C. H. (2014). An observational analysis of the oceanic and atmospheric structure of global-scale multi-decadal variability. Adv. Atmos. Sci. 31, 316–330. doi: 10.1007/s00376-013-2305-y
Liu, W. T. (1986). Statistical relation between monthly mean precipitable water and surface-level humidity over global oceans. Mon. Weather Rev. 114, 1591–1602. doi: 10.1175/1520-0493(1986)114<1591:SRBMMP>2.0.CO;2
Liu, W. T., and Tang, W. (1996). Equivalent Neutral Wind. Pasadena, CA: JPL Publication, 17. Available at: https://ntrs.nasa.gov/archive/nasa/casi.ntrs.nasa.gov/19970010322.pdf.
Liu, W. T., Tang, W., and Niiler, P. P. (1991). Humidity profiles over oceans. J. Clim. 4, 1023–1034. doi: 10.1175/1520-0442(1991)004<1023:HPOTO>2.0.CO;2
Losada, T., and Rodríguez-Fonseca, B. (2016). Tropical atmospheric response to decadal changes in the Atlantic Equatorial Mode. Clim. Dynam. 47, 1211–1224. doi: 10.1007/s00382-015-2897-2
Losada, T., Rodriguez-Fonseca, B., Janicot, S., Gervois, S., Chauvin, F., and Ruti, P. (2010). A multimodel approach to the Atlantic equatorial mode: impact on the West African monsoon. Clim. Dynam. 35, 29–43. doi: 10.1007/s00382-009-0625-5
Losada, T., Rodriguez-Fonseca, B., Mohino, E., Bader, J., Janicot, S., and Mechoso, C. R. (2012). Tropical SST and sahel rainfall: a non-stationary relationship. Geophys. Res. Lett. 39:L12705. doi: 10.1029/2012GL052423
Lu, F., Liu, Z., Zhang, S., and Liu, Y. (2015). Strongly coupled data assimilation using leading averaged coupled covariance (LACC). Part II: CGCM applications. Mon. Wea. Rev. 143, 4645–4659. doi: 10.1175/MWR-D-15-0088.1
Lübbecke, J., and McPhaden, M. J. (2012). On the inconsistent relationship between Atlantic and Pacific Niños. J. Clim. 25, 4294–4303. doi: 10.1175/JCLI-D-11-00553.1
Lübbecke, J. F., Boning, C. W., Keenlyside, N. S., and Xie, S. P. (2010). On the connection between Benguela and equatorial Atlantic Ninos and the role of the South Atlantic Anticyclone. J. Geophys. Res.-Oceans 115, C09015. doi: 10.1029/2009JC005964
Lübbecke, J. F., Rodriguez-Fonseca, B., Richter, I., Martin-Rey, M., Losada, T., Polo, I., et al. (2018). Equatorial Atlantic variability-Modes, mechanisms, and global teleconnections. Wires Clim. Change 9, e527. doi: 10.1002/wcc.527
Luyten, J. R., Pedlosky, J., and Stommel, H. (1983). The ventilated thermocline. J. Phys. Oceanogr. 13, 292–309. doi: 10.1175/1520-0485(1983)013<0292:TVT>2.0.CO;2
Machu, E., Capet, X., Estrade, P. A., Ndoye, S., Brajard, J., Baurand, F., et al. (2019). First evidence of anoxia and nitrogen loss in the Southern Canary upwelling system. Geophys. Res. Lett. 46, 2619–2627. doi: 10.1029/2018GL079622
Marengo, J. A., Nobre, C. A., Tomasella, J., Oyama, M. D., Sampaio, de Oliveira, G., et al. (2008). The drought of Amazonia in 2005. J. Clim. 21, 495–516. doi: 10.1175/2007JCLI1600.1
Marin, F., Caniaux, G., Bourlès, B., Giordani, H., Gouriou, Y., and Key, E. (2009). Why were sea surface temperatures so different in the Eastern Equatorial Atlantic in June 2005 and 2006. J. Phys. Oceanogr. 39, 1416–1431. doi: 10.1175/2008JPO4030.1
Martin, M. J. (2016). Suitability of satellite sea surface salinity data for use in assessing and correcting ocean forecasts. Remote Sens. Environ. 180, 305–319. doi: 10.1016/j.rse.2016.02.004
Martinez-Rey, J., Bopp, L., Gehlen, M., Tagliabue, A., and Gruber, N. (2015). Projections of oceanic N2O emissions in the 21st century using the IPSL Earth system model. Biogeosciences 12, 4133–4148. doi: 10.5194/bg-12-4133-2015
Martín-Rey, M., Polo, I., Rodríguez-Fonseca, B., Losada, T., and Lazar, A. (2018). Is there evidence of changes in tropical Atlantic variability modes under AMO phases in the observational record? J. Clim. 31, 515–536. doi: 10.1175/JCLI-D-16-0459.1
Martín-Rey, M., Rodríguez-Fonseca, B., and Polo, I. (2015). Atlantic opportunities for ENSO prediction. Geophys. Res. Lett. 42, 6802–6810. doi: 10.1002/2015GL065062
Martín-Rey, M., Rodríguez-Fonseca, B., Polo, I., and Kucharski, F. (2014). On the Atlantic–Pacific Niños connection: a multidecadal modulated mode. Clim. Dynam. 43, 3163–3178. doi: 10.1007/s00382-014-2305-3
Maunder, M. N., and Punt, A. E. (2013). A review of integrated analysis in fisheries stock assessment. Fish. Res. 142, 61–74. doi: 10.1016/j.fishres.2012.07.025
McGregor, S., Stuecker, M. F., Kajtar, J. B., England, M. H., and Collins, M. (2018). Model tropical Atlantic biases underpin diminished Pacific decadal variability. Nat. Clim. Change 8, 493–498. doi: 10.1038/s41558-018-0163-4
Medeiros, B., Williamson, D. L., Hannay, C., and Olson, J. G. (2012). Southeast pacific stratocumulus in the community atmosphere model. J. Clim. 25, 6175–6192. doi: 10.1175/JCLI-D-11-00503.1
Mohino, E., Janicot, S., and Bader, J. (2011). Sahel rainfall and decadal to multi-decadal sea surface temperature variability. Clim. Dynam. 37, 419–440. doi: 10.1007/s00382-010-0867-2
Mohrholz, V., Bartholomae, C. H., van der Plas, A. K., and Lass, H. U. (2008). The seasonal variability of the northern Benguela undercurrent and its relation to the oxygen budget on the shelf. Cont. Shelf Res. 28, 424–441. doi: 10.1016/j.csr.2007.10.001
Monerie, P. A., Biasutti, M., and Roucou, P. (2016). On the projected increase of Sahel rainfall during the late rainy season. Int. J. Clim. 36, 4373–4383. doi: 10.1002/joc.4638
Monteiro, P. M. S., van der Plas, A. K., Mélice, J. L., and Florenchie, P. (2008). Interannual hypoxia variability in a coastal upwelling system: Ocean-shelf exchange, climate and ecosystem-state implications. Deep Sea Res. I 55, 435–450. doi: 10.1016/j.dsr.2007.12.010
Morioka, Y., Masson, S., Terray, P., Prodhomme, C., Behera, S. K., and Masumoto, Y. (2014). Role of tropical SST variability on the formation of subtropical dipoles. J. Clim. 27, 4486–4507. doi: 10.1175/JCLI-D-13-00506.1
Morioka, Y., Tozuka, T., and Yamagata, T. (2011). On the growth and decay of the subtropical dipole mode in the South Atlantic. J. Clim. 24, 5538–5554. doi: 10.1175/2011JCLI4010.1
Moron, V., Bigot, S., and Roucou, P. (1995). Rainfall variability in subequatorial America and Africa and relationships with the main sea-surface temperature modes (1951–1990). Int. J. Climatol. 15, 1297–1322. doi: 10.1002/joc.3370151202
Muñoz, E., Weijer, W., Grodsky, S. A., Bates, S. C., and Wainer, I. (2012). Mean and variability of the tropical Atlantic Ocean in the CCSM4. J. Clim. 25, 4860–4882. doi: 10.1175/JCLI-D-11-00294.1
Myers, T. A., Mechoso, DeFlorio, C. R., and Clim Dyn, M. J. (2018). Importance of positive cloud feedback for tropical Atlantic interhemispheric climate variability. 51:1707. doi: 10.1007/s00382-017-3978-1
Nam, C., Bony, S., Dufresne, J.-L., and Chepfer, H. (2012). The ‘too few, too bright’ tropical low-cloud problem in CMIP5 models. Geophys. Res. Lett. 39:L21801. doi: 10.1029/2012GL053421
Neggers, R. A. J. (2015). Attributing the behavior of low-level clouds in large scale models to subgrid scale parameterizations. J. Adv. Model. Earth Syst. 7, 2029–2043. doi: 10.1002/2015MS000503
Nicholson, S. E. (2013). The West African sahel: a review of recent studies on the rainfall regime and its interannual variability. ISRN Meteorol. 2013:453521. doi: 10.1155/2013/453521
Nicholson, S. E., Some, B., and Kone, B. (2000). An analysis of recent rainfall conditions in West Africa, including the rainy seasons of the 1997 El Nino and the 1998 La Nina years. J. Clim. 13, 2628–2640. doi: 10.1175/1520-0442(2000)013<2628:AAORRC>2.0.CO;2
Nnamchi, H. C., Kucharski, F., Keenlyside, N. S., and Farneti, R. (2017). Analogous seasonal evolution of the South Atlantic SST dipole indices. Atmos. Sci. Lett. 18, 396–402. doi: 10.1002/asl.781
Nnamchi, H. C., Li, J., and Anyadike, R. N. C. (2011). Does a dipole mode really exist in the South Atlantic Ocean? J. Geophys. Res. 116:D15104. doi: 10.1029/2010JD015579
Nnamchi, H. C., Li, J., Kucharski, F., Kang, I., Keenlyside, N. S., Chang, P., et al. (2016). An equatorial–extratropical dipole structure of the Atlantic Niño. J. Clim. 29, 7295–7311. doi: 10.1175/JCLI-D-15-0894.1
Nnamchi, H. C., Li, J., Kucharski, F., Kang, I.-S., Keenlyside, N. S., Chang, P., et al. (2015). Thermodynamic controls of the Atlantic Niño. Nat. Commun. 6:8895. doi: 10.1038/ncomms9895
Nobre, P., De Almeida, R. A., Malagutti, M., and Giarolla, E. (2012). Coupled ocean-atmosphere variations over the South Atlantic Ocean. J. Clim. 25, 6349–6358. doi: 10.1175/JCLI-D-11-00444.1
Nobre, P., and Shukla, J. (1996). Variations of sea surface temperature, wind stress and rainfall over the tropical Atlantic and South America. J. Clim. 9, 2464–2479. doi: 10.1175/1520-0442(1996)009<2464:VOSSTW>2.0.CO;2
Nogueira Neto, A. V., Giordani, H., Caniaux, G., and Araujo, M. (2018). Seasonal and interannual mixed-layer heat budget variabili ty in the western tropical Atlantic from Argo floats (2007–2012). J. Geophys. Res. Oceans 123, doi: 10.1029/2017JC013436
Oke, P. R., Larnicol, G., Fujii, Y., Smith, G. C., Lea, D. J., Guinehut, S., et al. (2015). Assessing the impact of observations on ocean forecasts and reanalyses: part 1, global studies. J. Operat. Oceanogr. 8, s49–s62. doi: 10.1080/1755876X.2015.1022067
Okumura, Y., and Xie, S. P. (2004). Interaction of the Atlantic equatorial cold tongue and the African monsoon. J. Clim. 17, 3589–3602. doi: 10.1175/1520-0442(2004)017<3589:IOTAEC>2.0.CO;2
Oliver, E. C., Donat, M. G., Burrows, M. T., Moore, P. J., Smale, D. A., Alexander, L. V., et al. (2018). Longer and more frequent marine heatwaves over the past century. Nat. Commun. 9:1324. doi: 10.1038/s41467-018-03732-9
Olsen, A., Key, R. M., van Heuven, S., Lauvset, S. K., Velo, A., Lin, X., et al. (2016). The Global Ocean Data Analysis Project version 2 (GLODAPv2) – an internally consistent data product for the world ocean. Earth Syst. Sci. Data 8, 297–323. doi: 10.5194/essd-8-297-2016
Oschlies, A., Brandt, P., Stramma, L., and Schmidtko, S. (2018). Drivers and mechanisms of ocean deoxygenation. Nat. Geosci. 11, 467–473. doi: 10.1038/s41561-018-0152-2
Park, G.-H., and Wanninkhof, R. (2012). A large increase of the CO2 sink in the western tropical North Atlantic from 2002 to 2009. J. Geophys. Res. 117:C080029. doi: 10.1029/2011JC007803
Patricola, C. M., and Chang, P. (2017). Structure and dynamics of the benguela low-level coastal jet. Clim. Dynam. 49, 2765–2788. doi: 10.1007/s00382-016-3479-7
Patricola, C. M., Saravanan, R., and Chang, P. (2014). The impact of the El Niño-southern oscillation and atlantic meridional mode on seasonal atlantic tropical cyclone activity. J. Clim. 27, 5311–5328. doi: 10.1175/JCLI-D-13-00687.1
Peter, A. C., Le Hénaff, M., Du Penhoat, Y., Menkes, C. E., Marin, F., Vialard, J., et al. (2006). A model study of the seasonal mixed layer heat budget in the equatorial Atlantic. J. Geophys. Res. 111:C06014. doi: 10.1029/2005JC003157
Pinker, R. T., Bentamy, A., Katsaros, K. B., Ma, Y., and Li, C. (2014). Estimates of net heat fluxes over the Atlantic Ocean. J. Geophys. Res. Oceans 119, 410–427. doi: 10.1002/2013JC009386
Poli, P. (2018). Note on the Impact of Meteorological Data from PIRATA Moorings on Global Weather Forecasts, ed. Centre de Météorologie Marine. Météo-France: Centre de Météorologie Marine, 6. doi: 10.5281/zenodo.1164619
Polo, I., Martin-Rey, M., Rodriguez-Fonseca, B., Kucharski, F., and Mechoso, C. R. (2015a). Processes in the Pacific La Niña onset triggered by the Atlantic Niño. Clim. Dynam. 44, 115–131. doi: 10.1007/s00382-014-2354-7
Polo, I., Lazar, A., Rodriguez-Fonseca, B., and Mignot, J. (2015b). Growth and decay of the equatorial Atlantic SST mode by means of closed heat budget in a coupled general circulation model. Front. Earth Sci. 3:37. doi: 10.3389/feart.2015.00037
Polo, I., Rodríguez-Fonseca, B., Losada, T., and García-Serrano, J. (2008). Tropical Atlantic variability modes (1979–2002). Part I: time-evolving SST modes related to West African rainfall. J. Clim. 21, 6457–6475. doi: 10.1175/2008JCLI2607.1
Prodhomme, C., Batté, L., Massonnet, F., Davini, P., Bellprat, P., Guemas, V., et al. (2016). Benefits of increasing the model resolution for the seasonal forecast quality in EC-Earth. J. Clim. 29, 9141–9162. doi: 10.1175/JCLI-D-16-0117.1
Proud, R.,Kloser, R. J., Cox, M. J., and Brierley, A. S. (2018). From siphonophores to deep scattering layers: uncertainty ranges for the estimation of global mesopelagic fish biomass. ICES J. Mar. Sci. fsy037. doi: 10.1093/icesjms/fsy037
Prytherch, J., Kent, E. C., Fangohr, S., and Berry, D. I. (2015). A comparison of SSM/I-derived global marine surface-specific humidity datasets. Int. J. Climatol. 35, 2359–2381. doi: 10.1002/joc.4150
Pujol, M.-I., Faugère, Y., Taburet, G., Dupuy, S., Pelloquin, Ablain, C., et al. (2016). DUACS DT2014: the new multi-mission altimeter data set reprocessed over 20 years. Ocean Sci. 12, 1067–1090. doi: 10.5194/os-12-1067-2016
Qiao, F., Yuan, Y., Deng, J., Dai, D., and Song, Z. (2016). Wave-turbulence interaction-induced vertical mixing and its effects in ocean and climate models. Philos. Trans. R. Soc. A 374:20150201. doi: 10.1098/rsta.2015.0201
Qu, X., Hall, A., Klein, S. A., and Caldwell, P. M. (2014). On the spread of changes in marine low cloud cover in climate model simulations of the 21st century. Clim. Dynam. 42, 2603–2626. doi: 10.1007/s00382-013-1945-z
Randles, C. A., and Ramaswamy, V. (2010). Direct and semi-direct impacts of absorbing biomass burning aerosol on the climate of southern Africa: a geophysical fluid dynamics laboratory GCM sensitivity study. Atmos. Chem. Phys. 10, 9819–9831. doi: 10.5194/acp-10-9819-2010
Reichl, B. G., Ginis, I., Hara, T., Thomas, B., Kukulka, T., and Wang, D. (2016). Impact of sea-state-dependent Langmuir turbulence on the ocean response to a tropical cyclone. Mon. Weather Rev. 144, 4569–4590. doi: 10.1175/MWR-D-16-0074.1
Reul, N., Quilfen, Y., Chapron, B., Fournier, S., Kudryavtsev, V., and Sabia, R. (2014). Multisensor observations of the Amazon-Orinoco river plume interactions with hurricanes. J. Geophys. Res. 119, 8271–8295. doi: 10.1002/2014JC010107
Reynolds, R. W., Rayner, N. A., Smith, T. M., Stokes, D. C., and Wang, W. Q. (2002). An improved in situ and satellite SST analysis for climate. J. Clim. 15, 1609–1625. doi: 10.1175/1520-0442(2002)015<1609:AIISAS>2.0.CO;2
Richter, I. (2015). Climate model biases in the eastern tropical oceans: causes, impacts and ways forward. WIREs Clim. Change 6, 345–358. doi: 10.1002/wcc.338
Richter, I., Behera, S. K., Doi, T., Taguchi, B., Masumoto, Y., and Xie, S. P. (2014a). What controls equatorial Atlantic winds in boreal spring? Clim. Dyn. 43, 3091–3104. doi: 10.1007/s00382-014-2170-0
Richter, I., Xie, S. P., Behera, S. K., Doi, T., and Masumoto, Y. (2014b). Equatorial Atlantic variability and its relation to mean state biases in CMIP5. Clim. Dynam. 42, 171–188. doi: 10.1007/s00382-012-1624-5
Richter, I., Behera, S. K., Masumoto, Y., Taguchi, B., Komori, N., and Yamagata, T. (2010). On the triggering of Benguela Niños: remote equatorial versus local influences. Geophys. Res. Lett. 37:L20604. doi: 10.1029/2010GL044461
Richter, I., Behera, S. K., Masumoto, Y., Taguchi, B., Sasaki, H., and Yamagata, T. (2013). Multiple causes of interannual sea surface temperature variability in the equatorial Atlantic Ocean. Nat. Geosci. 643. doi: 10.1038/ngeo1660
Richter, I., Doi, T., Behera, S. K., and Keenlyside, N. (2017). On the link between mean state biases and prediction skill in the tropics: an atmospheric perspective. Clim. Dynam. 50, 3355–3374. doi: 10.1007/s00382-017-3809-4
Richter, I., and Xie, S.-P. (2008). On the origin of equatorial Atlantic biases in coupled general circulation models. Clim. Dynam. 31, 587–598. doi: 10.1007/s00382-008-0364-z
Richter, I., Xie, S.-P., Wittenberg, A. T., and Masumoto, Y. (2012). Tropical Atlantic biases and their relation to surface wind stress and terrestrial precipitation. Clim. Dynam. 38, 985–1001. doi: 10.1007/s00382-011-1038-9
Rodrigues, R. R., Haarsma, R. J., Campos, E. J. D., and Ambrizzi, T. (2011). Impacts of inter-El Niño variability on the tropical atlantic and northeast Brazil climate. J. Clim. 24, 3402–3422. doi: 10.1175/2011JCLI3983.1
Rodrigues, R. R., and McPhaden, M. J. (2014). Why did the 2011-12 La Niña cause a severe drought in the Brazilian Northeast? Geophys. Res. Lett. 41, 1012–1018. doi: 10.1002/2013GL058703
Rodríguez-Fonseca, B., Polo, I., García-Serrano, J., Losada, T., Mohino, E., Mechoso, C. R., et al. (2009). Are Atlantic Niños enhancing Pacific ENSO events in recent decades? Geophys. Res. Lett. 36:20705.
Rodríguez-Fonseca, B., Janicot, S., Mohino, E., Losada, T., Bader, J., Caminade, C., et al. (2011). Interannual and decadal SST-forced responses of the West African monsoon. Atmos. Sci. Lett. 12, 67–74. doi: 10.1002/asl.308
Rodríguez-Fonseca, B., Mohino, E., Mechoso, C. R., Caminade, C., Biasutti, M., Gaetani, M., et al. (2015). Variability and predictability of West African droughts: a review on the role of sea surface temperature anomalies. J. Clim. 28, 4034–4060. doi: 10.1175/JCLI-D-14-00130.1
Romera-Castillo, C., Pinto, M., Langer, T. M., Alvarez-Salgado, X. A., and Herndl, G. J. (2018). Dissolved organic carbon leaching from plastics stimulates microbial activity in the ocean. Nat. Commun. 9:1430. doi: 10.1038/s41467-018-03798-5
Rouault, M., Florenchie, P., Fauchereau, N., and Reason, C. J. C. (2003). South east tropical Atlantic warm events and southern African rainfall. Geophys. Res. Lett. 30:8009. doi: 10.1029/2003GL014840
Rouault, M., Illig, S., Bartholomae, C., Reason, C. J. C., and Bentamy, A. (2007). Propagation and origin of warm anomalies in the Angola Benguela upwelling system in 2001. J. Mar. Syst. 68, 473–488. doi: 10.1016/j.jmarsys.2006.11.010
Rouault, M., Illig, S., Lübbecke, J., and Koungue, R. A. I. (2018). Origin, development and demise of the 2010-2011 Benguela Niño. J. Mar. Syst. 188, 39–48. doi: 10.1016/j.jmarsys.2017.07.007
Rowell, D. P. (2001). Teleconnections between the tropical Pacific and the Sahel. Quart. J. Roy. Meteor. Soc. 127, 1683–1706. doi: 10.1002/qj.49712757512
Rugg, A., Foltz, G. R., and Perez, R. C. (2016). Role of mixed layer dynamics in tropical North Atlantic interannual sea surface temperature variability. J. Clim. 29, 8083–8101. doi: 10.1175/JCLI-D-15-0867.1
Ruiz-Barradas, A., Carton, J. A., and Nigam, S. (2000). Structure of interannual-to-decadal climate variability in the tropical Atlantic sector. J. Clim. 13, 3285–3297. doi: 10.1175/1520-0442(2000)013<3285:SOITDC>2.0.CO;2
Sakaeda, N., Wood, R., and Rasch, P. J. (2011). Direct and semidirect aerosol effects of southern African biomass burning aerosol. J. Geophys. Res. 116:D12205. doi: 10.1029/2010JD015540
Santos, G. C., Kerr, R., Azevedo, J. L. L., Mendes, C. R. B., and Da Cunha, L. C. (2016). Influence of Antarctic intermediate water on the deoxygenation of the Atlantic Ocean. Dynam. Atmos. Oceans 76, 72–82. doi: 10.1016/j.dynatmoce.2016.09.002
Satyamurty, P., da Costa, C. P. W., Manzi, A. O., and Candido, L. A. (2013). A quick look at the 2012 record flood in the Amazon Basin. Geophys. Res. Lett. 40, 1396–1401. doi: 10.1002/grl.50245
Scannell, H. A., and McPhaden, M. J. (2018). Seasonal mixed layer temperature balance in the southeastern tropical Atlantic. J. Geophys. Res. 123, 5557–5570. doi: 10.1029/2018JC014099
Schafstall, J., Dengler, M., Brandt, P., and Bange, H. (2010). Tidal induced mixing and diapycnal nutrient fluxes in the Mauritanian upwelling region. J. Geophys. Res. 115:C10014. doi: 10.1029/2009JC005940
Schmidtko, S., Stramma, L., and Visbeck, M. (2017). Decline in global oceanic oxygen content during the past five decades. Nature 542, 335–339. doi: 10.1038/nature21399
Schott, F. A., Dengler, M., Zantopp, R., Stramma, L., Fischer, J., and Brandt, P. (2005). The shallow and deep western boundary circulation of the South Atlantic at 5°-11°S. J. Phys. Oceanogr. 35, 2031–2053. doi: 10.1175/JPO2813.1
Schütte, F., Brandt, P., and Karstensen, J. (2016a). Occurrence and characteristics of mesoscale eddies in the tropical northeastern Atlantic Ocean. Ocean Sci. 12, 663–685. doi: 10.5194/os-12-663-2016
Schütte, F., Karstensen, J., Krahmann, G., Hauss, H., Fiedler, B., Brandt, P., et al. (2016b). Characterization of “dead-zone” eddies in the eastern tropical North Atlantic. Biogeosciences 13, 5865–5881. doi: 10.5194/bg-13-5865-2016
Scoccimarro, E., Villarini, G., Gualdi, S., Navarra, A., Vecchi, G., and Walsh, K. (2017). “Tropical cyclone rainfall changes in a warmer climate,” in Hurricanes and Climate Change, eds J. Collins and K. Walsh (Cham: Springer),243–255.
Send, U., Lankhorst, M., and Kanzow, T. (2011). Observation of decadal change in the Atlantic meridional overturning circulation using 10 years of continuous transport data. Geophys. Res. Lett. 38:L24606. doi: 10.1029/2011GL049801
Seo, H., Jochum, M., Murtugudde, R., Miller, A. J., and Roads, J. O. (2007). Feedback of tropical instability-wave-induced atmospheric variability onto the ocean. J. Clim. 20, 5842–5855. doi: 10.1175/JCLI4330.1
Servain, J., Caniaux, G., Kouadio, Y. K., McPhaden, M. J., and Araujo, M. (2014). Recent climatic trends in the tropical Atlantic. Clim. Dyn. 41, 3071–3089. doi: 10.1007/s00382-014-2168-7
Servain, J., Wainer, I., McCreary, J. P. Jr., and Dessier, A. (1999). Relationship between the equatorial and meridional modes of climatic variability in the tropical Atlantic. Geophys. Res. Lett. 26, 485–488. doi: 10.1029/1999GL900014
Shannon, L. V., Boyd, A. J., Bundrit, G. B., and Taunton-Clark, J. (1986). On the existence of an El Niño-type phenomenon in the Benguela system. J. Mar. Syst. 44, 495–520.
Shay, L. K., Goni, G. J., and Black, P. G. (2000). Effects of a warm oceanic feature on hurricane opal. Mon. Weater Rev. 128, 1366–1382. doi: 10.1175/1520-0493(2000)128<1366:EOAWOF>2.0.CO;2
Sobel, A. H., Camargo, S. J., Hall, T. M., Lee, C. Y., Tippett, M. K., and Wing, A. A. (2016). Human influence on tropical cyclone intensity. Science 353, 242–246. doi: 10.1126/science.aaf6574
St. Laurent, L., and Merrifield, S. (2017). Measurements of near-surface turbulence and mixing from autonomous ocean gliders. Oceanography 30, 116–125. doi: 10.5670/oceanog.2017.231
Stockdale, T. N., Balmaseda, M. A., and Vidard, A. (2006). Tropical Atlantic SST prediction with coupled ocean-atmosphere GCMs. J. Clim. 19, 6047–6061. doi: 10.1175/JCLI3947.1
Stoney, L., Walsh, K., Babanin, A. V., Ghantous, M., Govekar, P., and Young, I. (2017). Simulated ocean response to tropical cyclones: the effect of a novel parameterization of mixing from unbroken surface waves. J. Adv. Model. Earth Syst. 9, 759–780. doi: 10.1002/2016MS000878
Storto, A. (2016). Variational quality control of hydrographic profile data with non-Gaussian errors for global ocean variational data assimilation systems. Ocean Model. 104, 226–241. doi: 10.1016/j.ocemod.2016.06.011
Stramma, L., Johnson, G. C., Sprintall, J., and Mohrholz, V. (2008). Expanding oxygen-minimum zones in the tropical oceans. Science 320, 655–658. doi: 10.1126/science.1153847
Stramma, L., Prince, E. D., Schmidtko, S., Luo, J. G., Hoolihan, J. P., Visbeck, M., et al. (2012). Expansion of oxygen minimum zones may reduce available habitat for tropical pelagic fishes. Nat. Clim. Change 2, 33–37. doi: 10.1038/nclimate1304
Stramma, L., Visbeck, M., Brandt, P., Tanhua, T., and Wallace, D. (2009). Deoxygenation in the oxygen minimum zone of the eastern tropical North Atlantic. Geophys. Res. Lett. 36:L20607. doi: 10.1029/2009GL039593
Suarez-Moreno, R., and Rodríguez-Fonseca, B. (2018). Interdecadal changes in the leading ocean forcing of sahelian rainfall interannual variability: atmospheric dynamics and role of multidecadal SST background. J. Clim. 31, 6687–6710. doi: 10.1175/JCLI-D-17-0367.s1
Sultan, B., and Janicot, S. (2003). The West African monsoon dynamics. Part II: the “preonset” and “onset” of the summer monsoon. J. Clim. 16, 3407–3427. doi: 10.1175/1520-0442(2003)016<3407:TWAMDP>2.0.CO;2
Sweet, W., Fett, R., Kerling, J., and La Violette, P. (1981). Air-sea interaction effects in the lower troposphere across the north wall of the Gulf Stream. Mon. Weather Rev. 109, 1042–1052. doi: 10.1175/1520-0493(1981)109<1042:ASIEIT>2.0.CO;2
Tanimoto, Y., and Xie, S. P. (2002). Inter-hemispheric decadal variations in SST, surface wind, heat flux, and cloud cover over the Atlantic Ocean. J. Meteor. Soc. Jpn. 80, 1199–1219. doi: 10.2151/jmsj.80.1199
Tchipalanga, P., Dengler, M., Brandt, P., Kopte, R., Macuéria, M., Coelho, P., et al. (2018). Eastern boundary circulation and hydrography off Angola – building Angolan oceanographic capacities. Bull. Am. Met. Soc. 99, 1589–1605. doi: 10.1175/BAMS-D-17-0197.1
Tokinaga, H., and Xie, S. P. (2011). Weakening of the equatorial Atlantic cold tongue over the past six decades. Nat. Geosci. 4, 222–226. doi: 10.1038/ngeo1078
Toniazzo, T., and Woolnough, S. (2014). Development of warm SST errors in the southern tropical Atlantic in CMIP5 decadal hindcasts. Clim. Dynam. 43, 2889–2913. doi: 10.1007/s00382-013-1691-2
Torralba, V., Rodríguez-Fonseca, B., Mohino, E., and Losada, T. (2015). The non-stationary influence of the Atlantic and Pacific Niños on North Eastern South American rainfall. Front. Earth Sci. 3:55. doi: 10.3389/feart.2015.00055
Trenberth, K. E., Dai, A., Van Der Schrier, G., Jones, P. D., Barichivich, J., Briffa, K. R., et al. (2014). Global warming and changes in drought. Nat. Clim. Change 4, 17–22. doi: 10.1038/NCLIMATE2067
Trolliet, M., Walawender, J. P., Bourlès, B., Boilley, A., Trentmann, J., Blanc, P., et al. (2018). Downwelling surface solar irradiance in the tropical Atlantic Ocean: a comparison of re-analyses and satellite-derived data sets to PIRATA measurements. Ocean Sci. 14, 1021–1056. doi: 10.5194/os-14-1021-2018
Tuchen, F. P., Brandt, P., Claus, M., and Hummels, R. (2018). Deep intraseasonal variability in the central equatorial Atlantic. J. Phys. Oceanogr. 48, 2851–2865. doi: 10.1175/JPO-D-18-0059.1
Turpin, V., Remy, E., and Le Traon, P.-Y. (2016). How essential are Argo observations to constrain a global ocean data assimilation system? Ocean Sci. 12, 257–274. doi: 10.5194/os-12-257-2016
Vecchi, G. A., and Soden, B. J. (2007). Increased tropical Atlantic wind shear in model projections of global warming. Geophys. Res. Lett. 34:L08702. doi: 10.1029/2006gl028905
Venegas, S. A., Mysak, L. A., and Straub, D. N. (1996). Evidence for interannual and interdecadal climate variability in the South Atlantic. Geophys. Res. Lett. 23, 2673–2676. doi: 10.1029/96GL02373
Verma, A., Kloser, R. J., and Duncan, A. J. (2017). Potential use of broadband acoustic methods for micronekton classification. Acoust. Aust. 45, 353–361. doi: 10.1007/s40857-017-0105-8
Villamayor, J., and Mohino, E. (2015). Robust sahel drought due to the Interdecadal Pacific Oscillation in CMIP5 simulations. Geophys. Res. Lett. 42, 1214–1222. doi: 10.1002/2014GL062473
Visbeck, M., Araujo, M., Boetius, A., Buch, E., Claustre, H., Dabrowski, T., et al. (2015). More integrated and more sustainable atlantic ocean observing system (AtlantOS). CLIVAR Exch. 67, 18–20.
Voldoire, A., Exarchou, E., Sanchez-Gomez, E., Deppenmeier, A.-L., Frauen, C., Goubanova, K., et al. (2019). Role of wind stress in driving SST biases in the Tropical Atlantic. Clim. Dynam. (in press). doi: 10.1007/s00382-019-04717-0
Voosen, P. (2018). Saildrone fleet could help replace aging buoys. Science 359, 1082–1083. doi: 10.1126/science.359.6380.1082
Wahl, S., Latif, M., Park, W., and Keenlyside, N. (2011). On the tropical atlantic SST warm bias in the kiel climate model. Clim. Dynam. 36, 891–906. doi: 10.1007/s00382-009-0690-9
Wallace, J. M., Mitchell, T. P., and Deser, C. (1989). The influence of sea- surface temperature on surface wind in the eastern equatorial Pacific: seasonal and interannual variability. J. Clim. 2, 1492–1499. doi: 10.1175/1520-0442(1989)002<1492:TIOSST>2.0.CO;2
Walsh, K. J. E., Camargo, S. J., Vecchi, G. A., Daloz, A. S., Elsner, J., and Emanuel, K. (2015). Hurricanes and climate: the US CLIVAR working group on hurricanes. Bull. Am. Meteorol. Soc. 96, 997–1017. doi: 10.1175/BAMS-D-13-00242.1
Walsh, K. J. E., McBride, J. L., Klotzbach, P. J., Balachandran, S., Camargo, S. J., and Holland, G. (2016). Tropical cyclones and climate change. Wiley Interdiscipl. Rev. Clim. Change 7, 65–89. doi: 10.1002/wcc.371
Wang, C., Kucharski, F., Barimalala, R., and Bracco, A. (2009). Teleconnections of the tropical Atlantic to the tropical Indian and Pacific Oceans: a review of recent findings. Meteorol. Z. 18, 445–454. doi: 10.1127/0941-2948/2009/0394
Wang, C., Zhang, L., Lee, S.-K., Wu, L., and Mechoso, C. R. (2014). A global perspective on CMIP5 climate model biases. Nat. Clim. Change 4, 201–205. doi: 10.1038/nclimate2118
Wang, C. Z., Liu, H. L., Lee, S.-K., and Atlas, R. (2011). Impact of the Atlantic warm pool on United States landfalling hurricanes. Geophys. Res. Lett. 38:L19702. doi: 10.1029/2011GL049265
Wang, L., Yu, J. Y., and Paek, H. (2017). Enhanced biennial variability in the Pacific due to Atlantic capacitor effect. Nat. Commun. 8:14887. doi: 10.1038/ncomms14887
Wang, M. Q., and Hu, C. M. (2017). Predicting sargassum blooms in the caribbean sea from MODIS observations. Geophys. Res. Lett. 44, 3265–3273. doi: 10.1002/2017GL072932
Wang, S. Y. S., Zhao, L., Yoon, J. H., Klotzbach, P., and Gillies, R. R. (2018). Quantitative attribution of climate effects on Hurricane Harvey’s extreme rainfall in Texas. Environ. Res. Lett. 13:054014. doi: 10.1088/1748-9326/aabb85
Weatherhead, E. C., Wielicki, B. A., Ramaswamy, V., Abbott, M., Ackerman, T. P., Atlas, R., et al. (2018). Designing the climate observing system of the future. Earth’s Future 6, 80–102. doi: 10.1002/2017EF000627
Weaver, A. T., Deltel, C., Machu, E., Ricci, S., and Daget, N. (2005). A multivariate balance operator for variational ocean data assimilation. Q. J. R. Meteorol. Soc. 131, 3605–3625. doi: 10.1256/qj.05.119
Webb, M. J., Andrews, T., Bodas-Salcedo, A., Bony, S., Bretherton, C. S., Chadwick, R., et al. (2017). The cloud feedback model intercomparison project (CFMIP) contribution to CMIP6. Geosci. Model Dev. 10, 359–384. doi: 10.5194/gmd-10-359-2017
Webster, P. J., Holland, G. J., Curry, J. A., and Chang, H. R. (2005). Changes in tropical cyclone number, duration, and intensity in a warming environment. Science 309, 1844–1846. doi: 10.1126/science.1116448
Wehner, M., Reed, K., Prabhat, L. F., Bacmeister, J., Chen, C.-T., Paciorek, C., et al. (2014). The effect of horizontal resolution on simulation quality in the community atmospheric Model, CAM5.1. J. Model. Earth Syst. 06, 980–997. doi: 10.1002/2013MS000276
Wenegrat, J. O., and McPhaden, M. J. (2015). Dynamics of the surface layer diurnal cycle in the equatorial Atlantic Ocean (0, 23W). J. Geophys. Res. Oceans 120, 563–581. doi: 10.1002/2014JC010504
Wood, R., Kuan-Ting, O., Bretherton, C., Mohrmann, J., Albrecht, B., Zuidema, P., et al. (2018). Ultraclean layers and optically-thin clouds in the stratocumulus-to-cumulus transition: Part I. Observations. J. Atmos. Sci. 75, 1631–1652. doi: 10.1175/JAS-D-17-0213.1
Wu, L., He, F., Liu, Z., and Li, C. (2007). Atmospheric teleconnections of tropical Atlantic variability: Interhemispheric, tropical-extratropical, and cross-basin interactions. J. Clim. 20, 856–870. doi: 10.1175/JCLI4019.1
Xie, S.-P., and Carton, J. A. (2004). “Tropical Atlantic variability: patterns, mechanisms, and impacts,” in Earth’s Climate, eds C. Wang, S.-P. Xie, and J. A. Carton (Washington, D.C: American Geophysical Union).
Xie, T., Boyer, T., Bayler, E., Xue, Y., Byrne, D., Reagan, J., et al. (2014). An in situ – satellite blended analysis of global sea surface salinity. J. Geophys. Res. Oceans 119, 6140–6160. doi: 10.1002/2014JC010046
Xu, Z., Chang, P., Richter, I., and Kim, W. (2014a). Diagnosing southeast tropical Atlantic SST and ocean circulation biases in the CMIP5 ensemble. Clim. Dynam. 43, 3123–3145. doi: 10.1007/s00382-014-2247-9
Xu, Z., Li, M., Patricola, C. M., and Chang, P. (2014b). oceanic origin of southeast tropical atlantic biases. Clim. Dynam. 43, 2915–2930. doi: 10.1007/s00382-013-1901-y
Xue, Y., Wen, C., Kumar, A., Balmaseda, M., Fujii, Y., Alves, O., et al. (2017). A real-time ocean reanalyses intercomparison project in the context of tropical pacific observing system and ENSO monitoring. Clim. Dynam. 49, 3647–3672. doi: 10.1007/s00382-017-3535-y
Yang, Y., Saatchi, S. S., Xu, L., Yu, Y., Choi, S., Phillips, N., et al. (2018). Post-drought decline of the Amazon carbon sink. Nat. Comm. 9:3172. doi: 10.1038/s41467-018-05668-6
Yang, Y. J. (1999). A linkage between decadal climate variations in the Labrador Sea and the tropical Atlantic Ocean. Geophys. Res. Lett. 26, 1023–1026. doi: 10.1029/1999GL900181
Young, J. A. (1987). Boundary Layer Dynamics of Tropical and Monsoonal Flows. Monsoon Meteorology. Oxford: Oxford University Press, 461–500.
Yu, L. (2018). “Sea surface exchanges of momentum, heat, and freshwater determined by satellite remote sensing,” in Encyclopedia of Ocean Sciences, 3rd Edn, eds J. K. Cochran, H. J. Bokuniewicz, and P. L. Yager (Cambridge, MA: Academic Press).
Yu, L. (2019). Global air–sea fluxes of heat, fresh water, and momentum: energy budget closure and unanswered questions. Annu. Rev. Mar. Sci. 11, 227–248. doi: 10.1146/annurev-marine-010816-060704
Yu, L. S., and Weller, R. A. (2007). Objectively analyzed air-sea heat fluxes for the global ice–free oceans (1981–2005). Bull. American Meteorol. Soc. 88, 527–539. doi: 10.1175/BAMS-88-4-527
Yuan, T., Oreopoulos, L., Zelinka, M., Yu, H., Norris, J. R., Chin, M., et al. (2016). Positive low cloud and dust feedbacks amplify tropical North Alantic Multidecadal Oscillation. Geophys. Res. Lett. 43, 1349–1356. doi: 10.1002/2016GL067679
Zarzycki, C. M. (2016). Tropical cyclone intensity errors associated with lack of two-way ocean coupling in high-resolution global simulations. J. Clim. 29, 8589–8610. doi: 10.1175/JCLI-D-16-0273.1
Zebiak, S. E. (1993). Air–sea interaction in the equatorial Atlantic region. J. Climate 6, 1567–1586. doi: 10.1175/1520-0442(1993)006<1567:AIITEA>2.0.CO;2
Zeng, N., Neelin, J. D., Lau, K.-M., and Tucker, C. J. (1999). Enhancement of interdecadal climate variability in the Sahel by vegetation interaction. Science 286, 1537–1540. doi: 10.1126/science.286.5444.1537
Zeng, N., Yoon, J. H., Marengo, J. A., Subramaniam, A., Nobre, C. A., Mariotti, A., et al. (2008). Causes and impacts of the 2005 Amazon drought. Environ. Res. Lett. 3:014002. doi: 10.1088/1748-9326/3/1/014002
Zermeño-Diaz, D. M., and Zhang, C. (2013). Possible root causes of surface westerly biases over the equatorial Atlantic in global climate models. J. Clim. 26, 8154–8168. doi: 10.1175/JCLI-D-12-00226.1
Zhang, D., McPhaden, M. J., and Johns, W. E. (2003). Observational evidence for flow between the subtropical and tropical atlantic: the atlantic subtropical cells. J. Phys. Oceanogr. 13, 1783–1797. doi: 10.1175/2408.1
Zhang, R., and Delworth, T. L. (2006). Impact of atlantic multidecadal oscillations on India/Sahel rainfall and Atlantic hurricanes. Geophys. Res. Lett. 33:L17712. doi: 10.1029/2006GL026267
Zhang, S., Harrison, M. J., Rosati, A., and Wittenberg, A. (2007). System design and evaluation of coupled ensemble data assimilation for global oceanic climate studies. Mon. Wea. Rev. 135, 3541–3564. doi: 10.1175/MWR3466.1
Zilberman, N., and Roemmich, D. (2017). The argo program samples the deep ocean. US CLIVAR Variat. 2, 29–33.
Zuidema, P., Leon, D., Pazmany, A., and Cadeddu, M. (2012). Aircraft millimeter-wave passive sensing of cloud liquid water and water vapor during VOCALS-REx. Atmos. Chem. Phys. 12, 355–369. doi: 10.5194/acp-12-355-2012
Zuidema, P., Chang, P., Medeiros, B., Kirtman, B. P., Mechoso, R., Schneider, E. K., et al. (2016a). Challenges and prospects for reducing coupled climate model SST biases in the eastern tropical atlantic and pacific oceans: the U.S. CLIVAR eastern tropical oceans synthesis working group. Bull. Am. Meteorol. Soc. 97, 2305–2328. doi: 10.1175/BAMS-D-15-00274.1
Keywords: tropical Atlantic Ocean, observing system, weather, climate, hurricanes, biogeochemistry, ecosystems, coupled model bias
Citation: Foltz GR, Brandt P, Richter I, Rodríguez-Fonseca B, Hernandez F, Dengler M, Rodrigues RR, Schmidt JO, Yu L, Lefevre N, Da Cunha LC, McPhaden MJ, Araujo M, Karstensen J, Hahn J, Martín-Rey M, Patricola CM, Poli P, Zuidema P, Hummels R, Perez RC, Hatje V, Lübbecke JF, Polo I, Lumpkin R, Bourlès B, Asuquo FE, Lehodey P, Conchon A, Chang P, Dandin P, Schmid C, Sutton A, Giordani H, Xue Y, Illig S, Losada T, Grodsky SA, Gasparin F, Lee T, Mohino E, Nobre P, Wanninkhof R, Keenlyside N, Garcon V, Sánchez-Gómez E, Nnamchi HC, Drévillon M, Storto A, Remy E, Lazar A, Speich S, Goes M, Dorrington T, Johns WE, Moum JN, Robinson C, Perruche C, de Souza RB, Gaye AT, López-Parages J, Monerie P-A, Castellanos P, Benson NU, Hounkonnou MN, Duhá JT, Laxenaire R and Reul N (2019) The Tropical Atlantic Observing System. Front. Mar. Sci. 6:206. doi: 10.3389/fmars.2019.00206
Received: 31 October 2018; Accepted: 02 April 2019;
Published: 10 May 2019.
Edited by:
Gilles Reverdin, Centre National de la Recherche Scientifique (CNRS), FranceReviewed by:
Sophie E. Cravatte, Institut de Recherche pour le Développement (IRD), FranceLaurent Coppola, UMR7093 Laboratoire d’Océanographie de Villefranche (LOV), France
Copyright © 2019 Foltz, Brandt, Richter, Rodríguez-Fonseca, Hernandez, Dengler, Rodrigues, Schmidt, Yu, Lefevre, Da Cunha, McPhaden, Araujo, Karstensen, Hahn, Martín-Rey, Patricola, Poli, Zuidema, Hummels, Perez, Hatje, Lübbecke, Polo, Lumpkin, Bourlès, Asuquo, Lehodey, Conchon, Chang, Dandin, Schmid, Sutton, Giordani, Xue, Illig, Losada, Grodsky, Gasparin, Lee, Mohino, Nobre, Wanninkhof, Keenlyside, Garcon, Sánchez-Gómez, Nnamchi, Drévillon, Storto, Remy, Lazar, Speich, Goes, Dorrington, Johns, Moum, Robinson, Perruche, de Souza, Gaye, López-Parages, Monerie, Castellanos, Benson, Hounkonnou, Duhá, Laxenaire and Reul. This is an open-access article distributed under the terms of the Creative Commons Attribution License (CC BY). The use, distribution or reproduction in other forums is permitted, provided the original author(s) and the copyright owner(s) are credited and that the original publication in this journal is cited, in accordance with accepted academic practice. No use, distribution or reproduction is permitted which does not comply with these terms.
*Correspondence: G. R. Foltz, gregory.foltz@noaa.gov